Using Corpora for Language Research COGS 523 Lecture
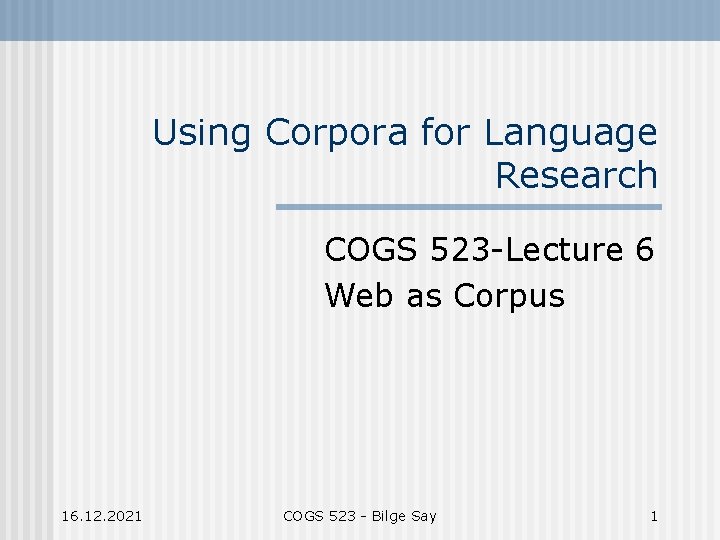
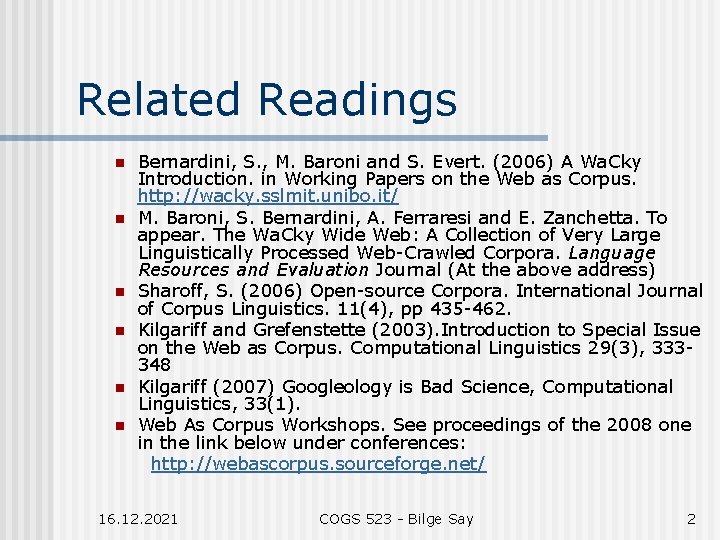
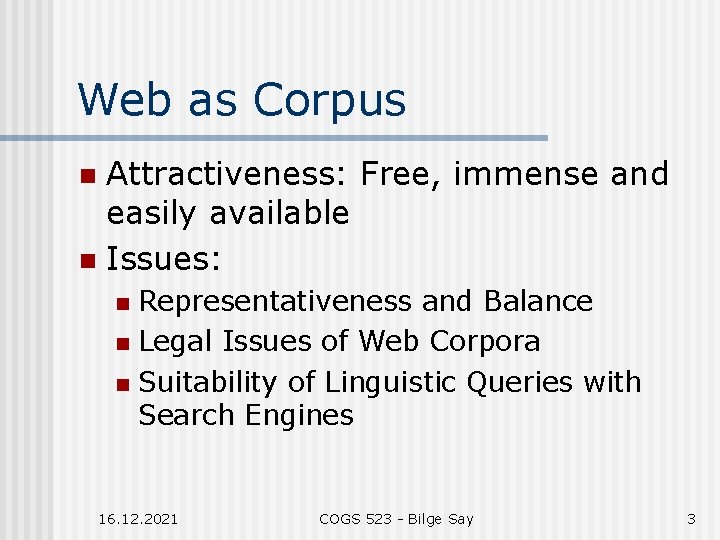
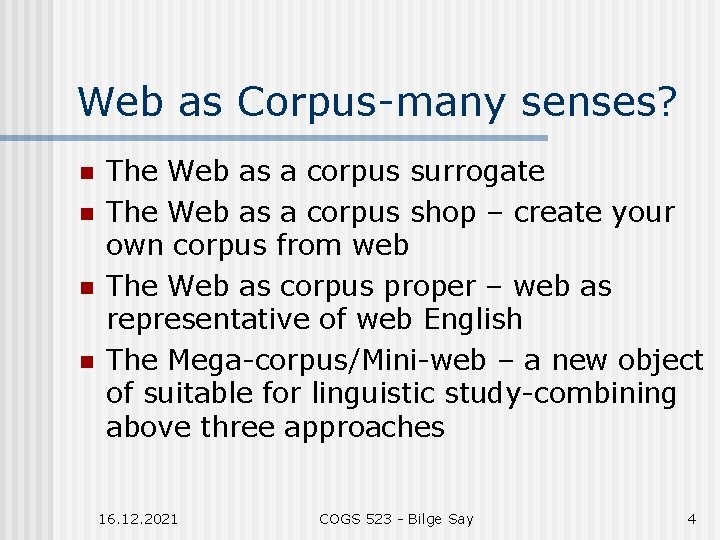
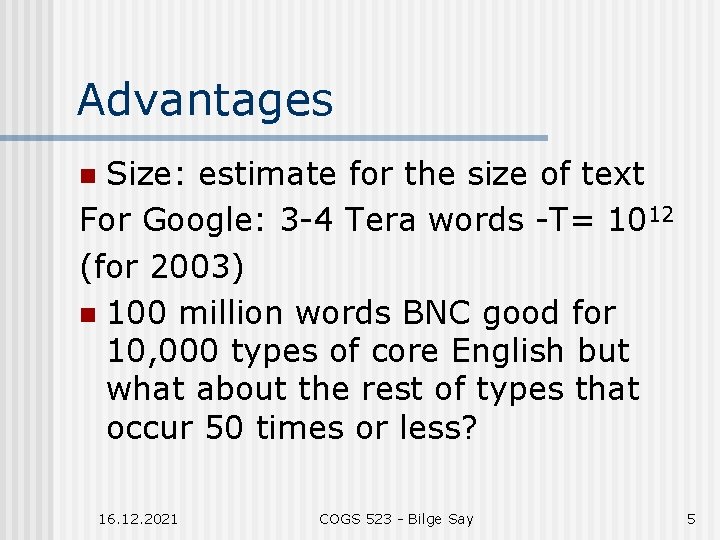
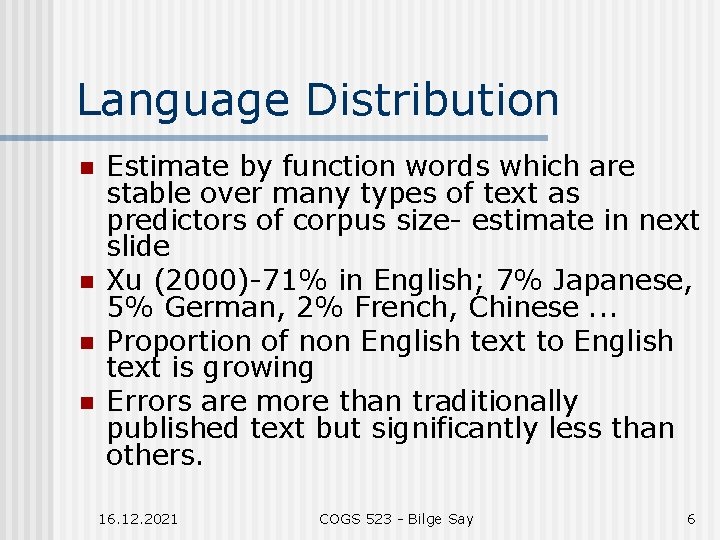
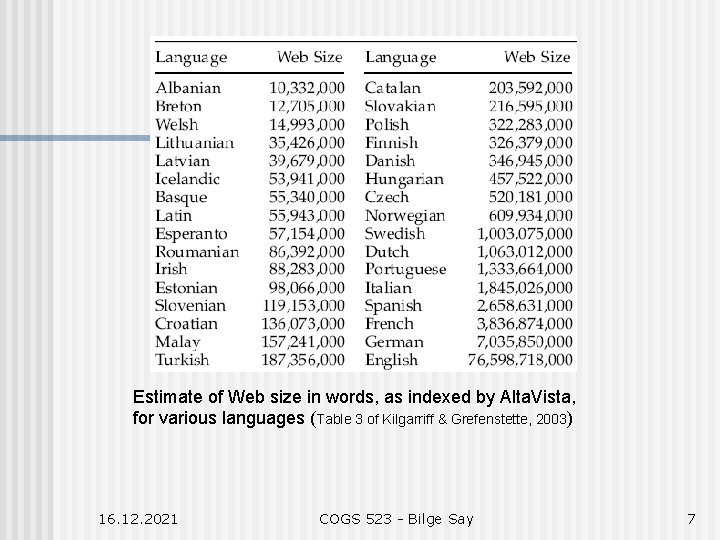
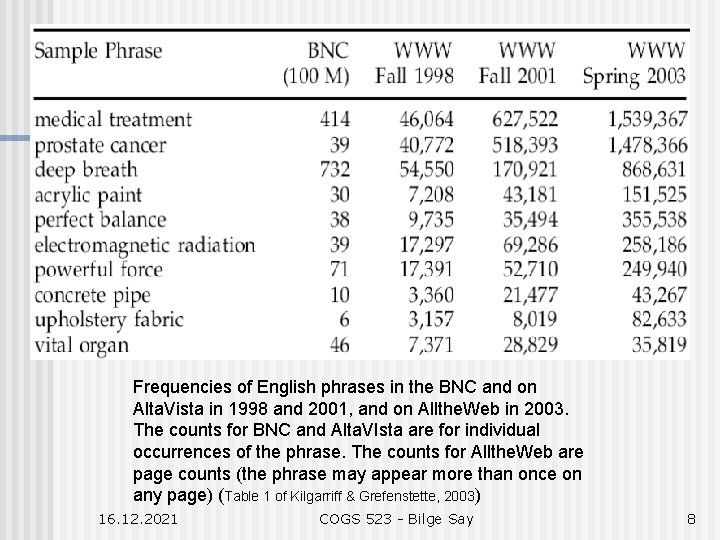
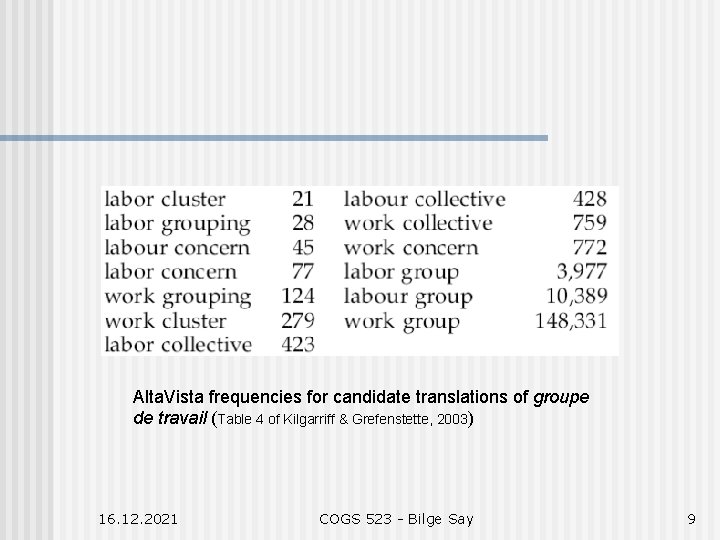
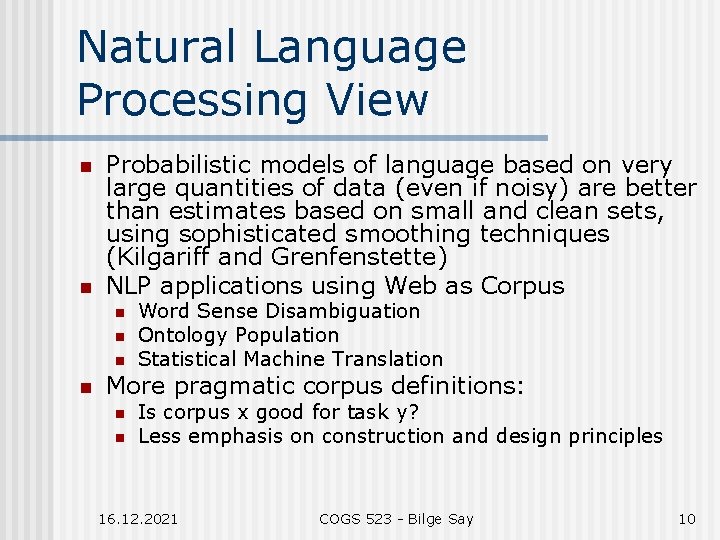
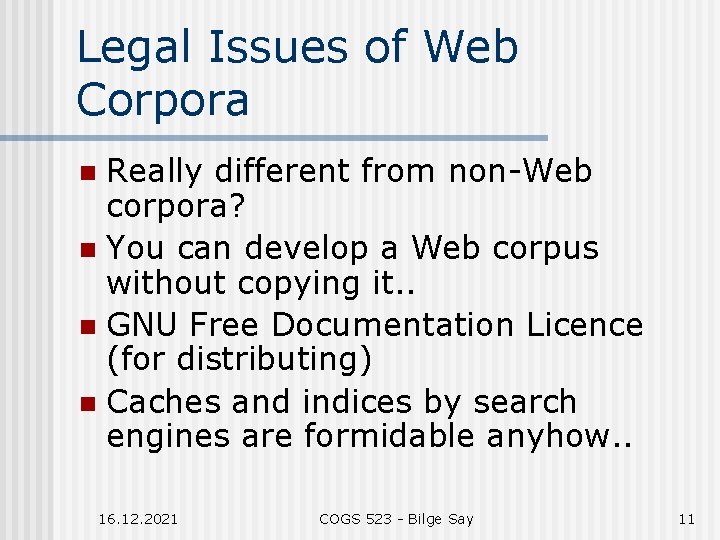
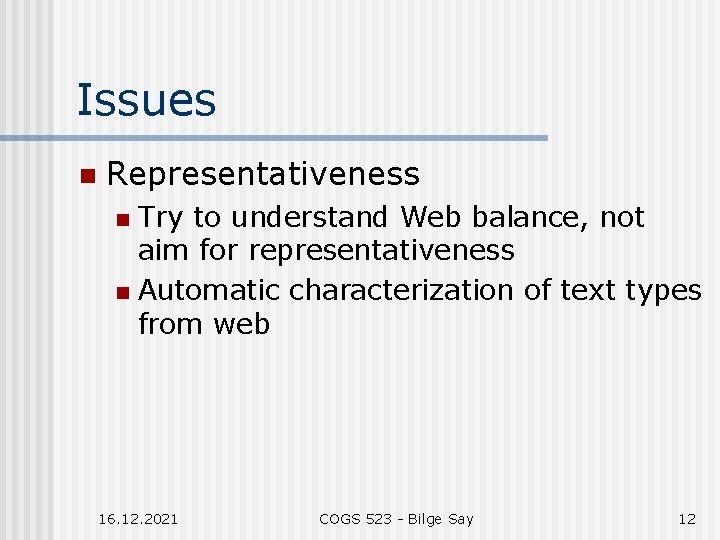
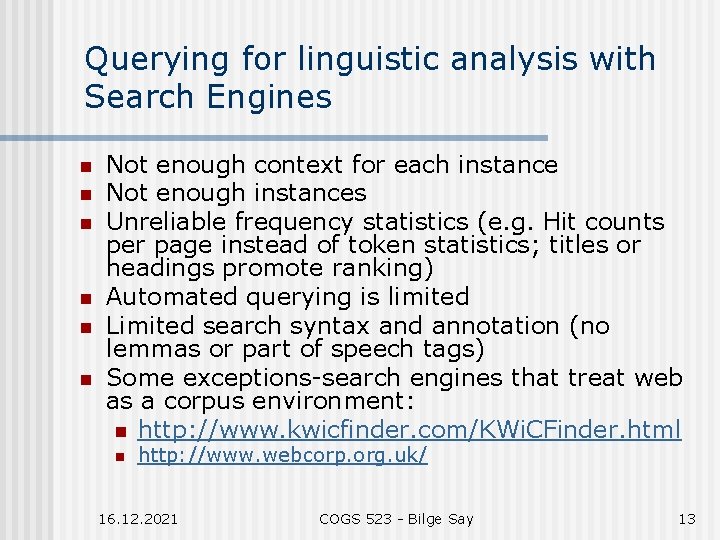
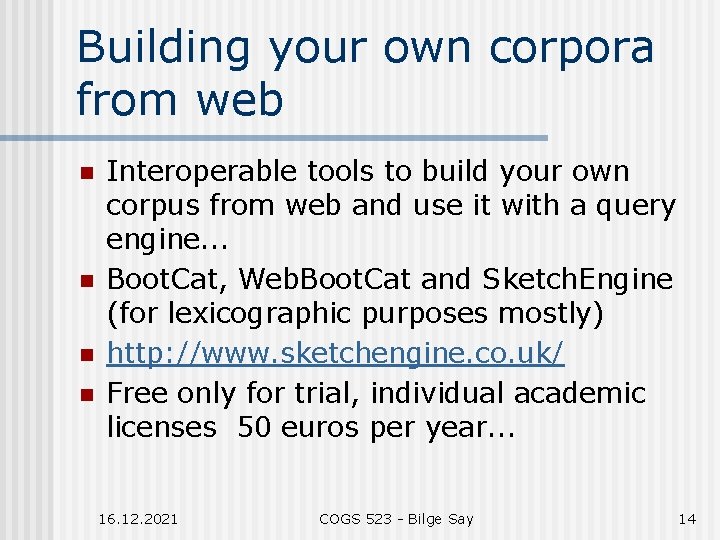
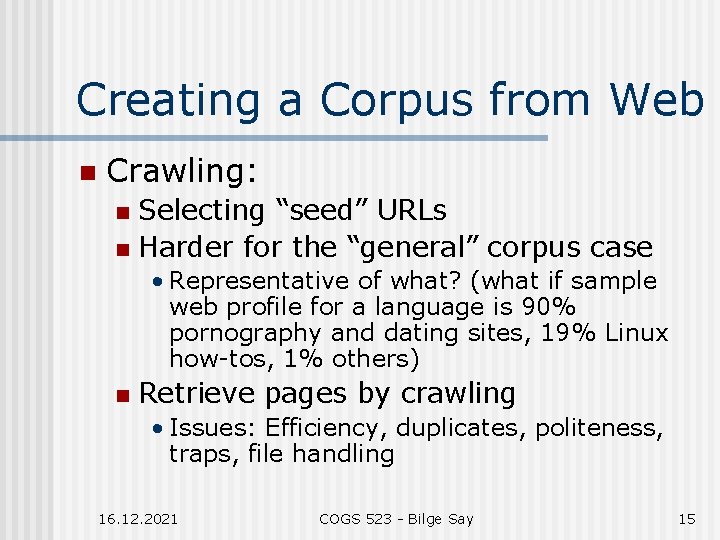
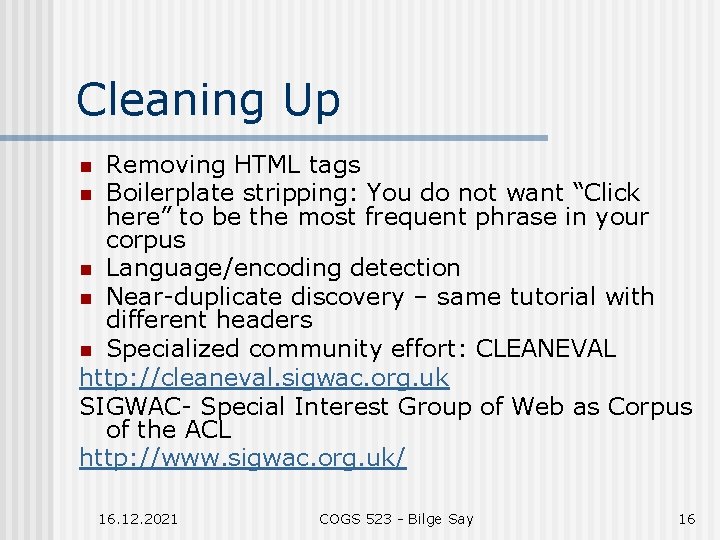
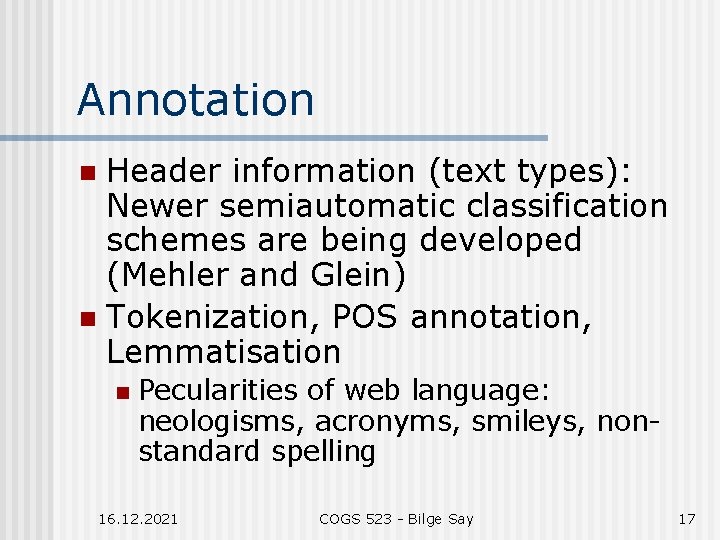
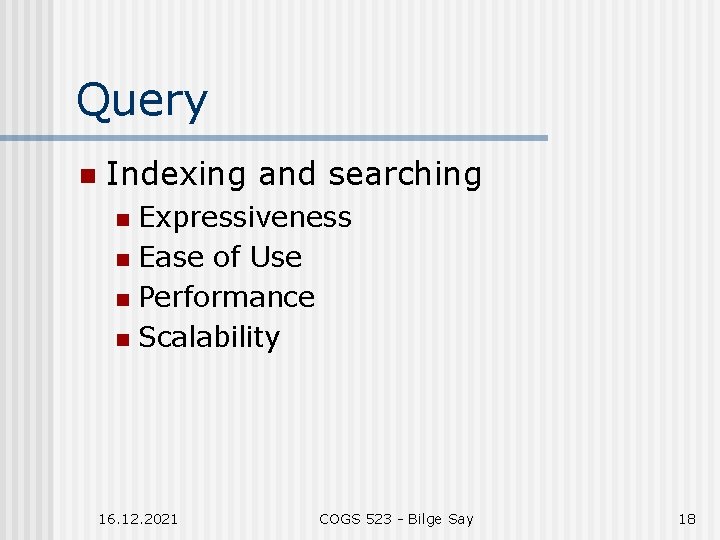
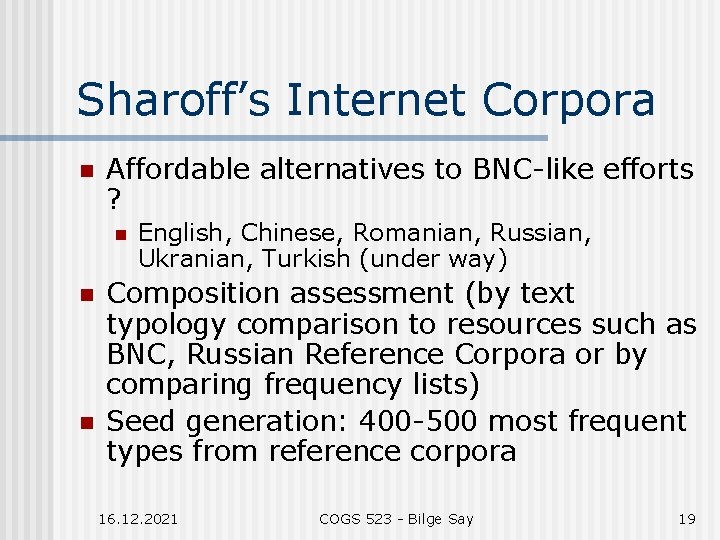
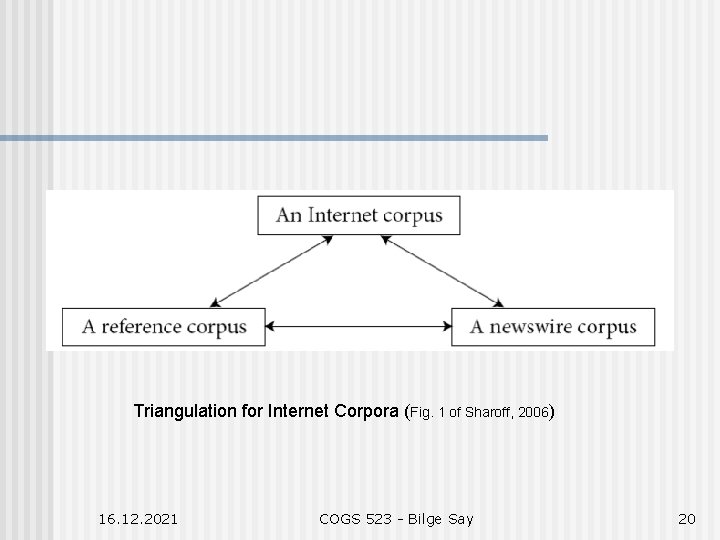
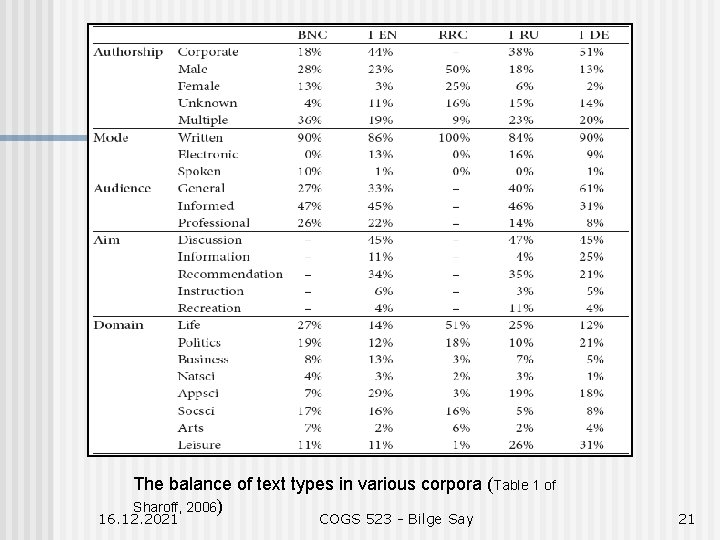
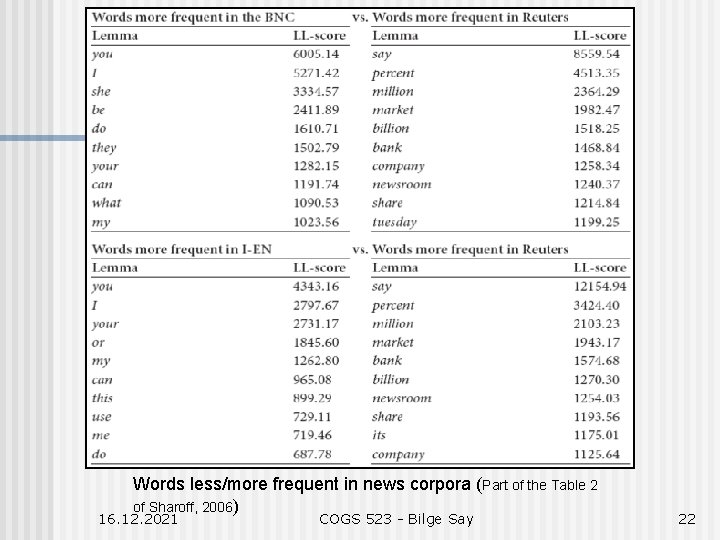
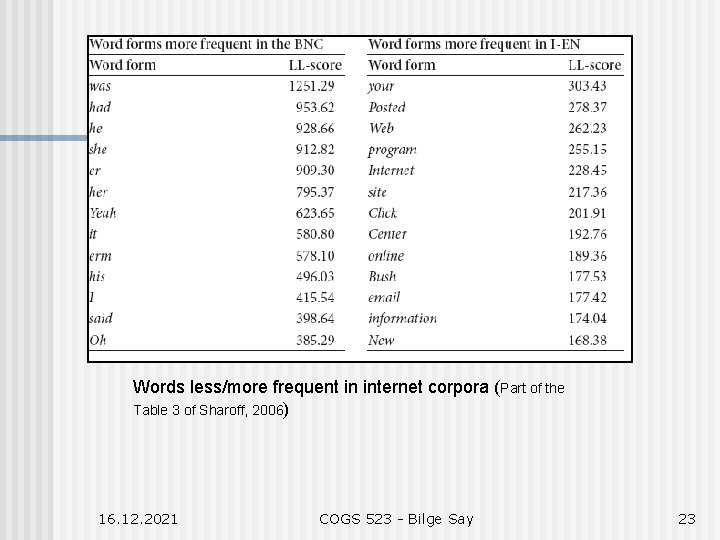
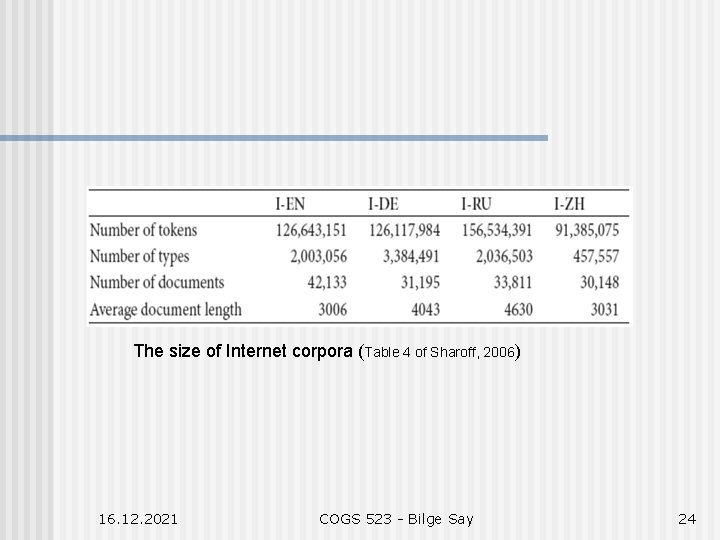
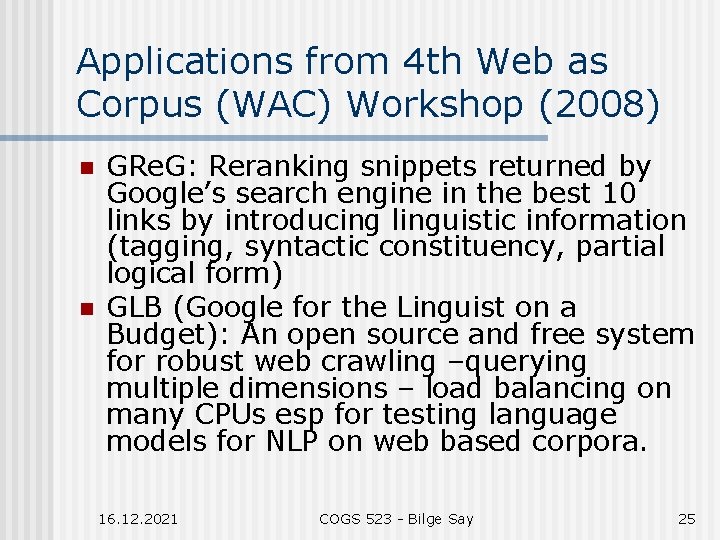
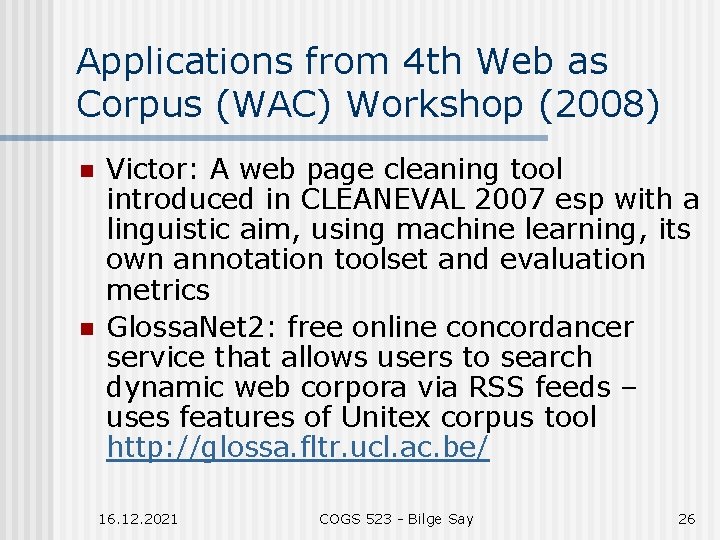
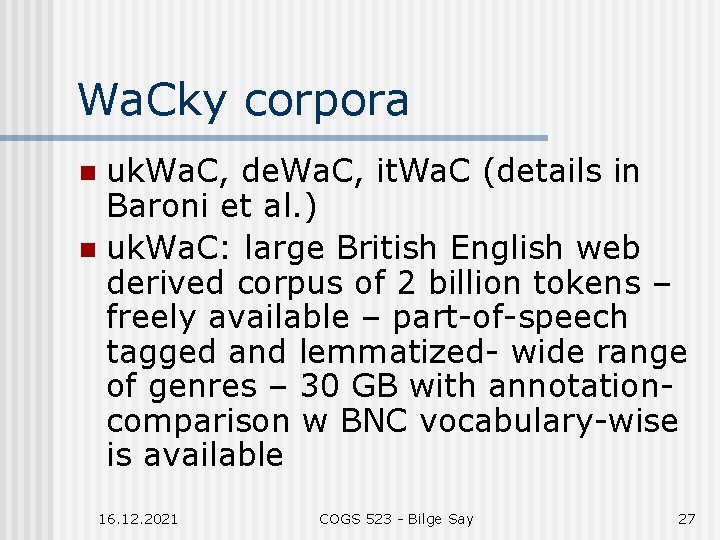
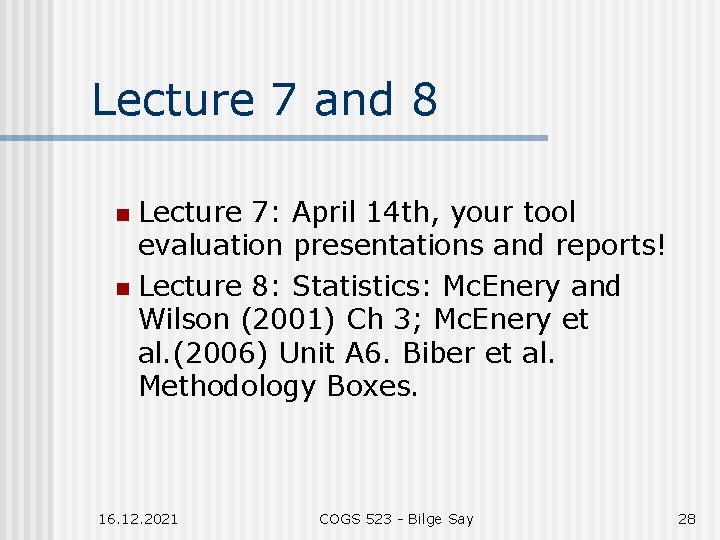
- Slides: 28
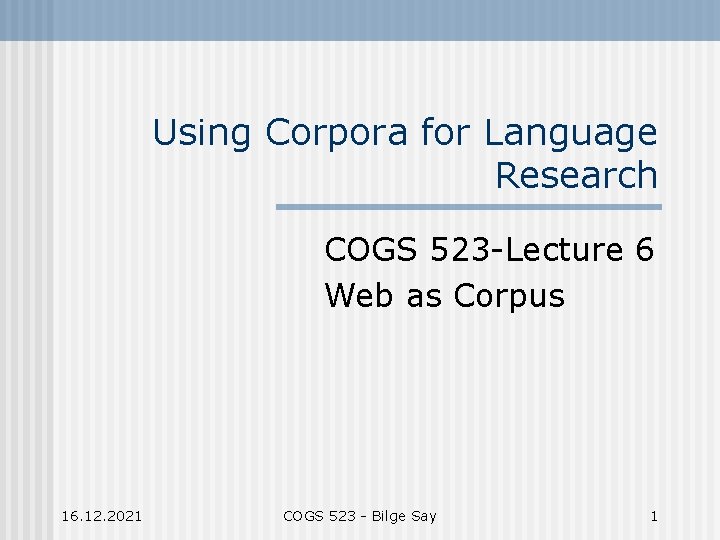
Using Corpora for Language Research COGS 523 -Lecture 6 Web as Corpus 16. 12. 2021 COGS 523 - Bilge Say 1
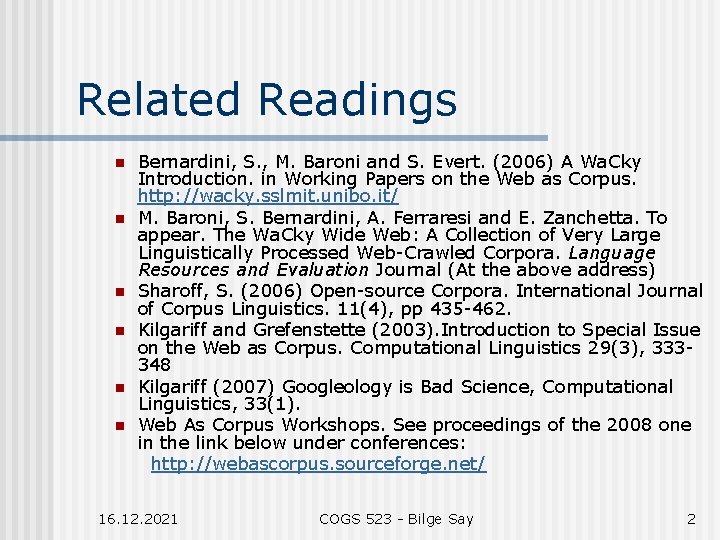
Related Readings n n n Bernardini, S. , M. Baroni and S. Evert. (2006) A Wa. Cky Introduction. in Working Papers on the Web as Corpus. http: //wacky. sslmit. unibo. it/ M. Baroni, S. Bernardini, A. Ferraresi and E. Zanchetta. To appear. The Wa. Cky Wide Web: A Collection of Very Large Linguistically Processed Web-Crawled Corpora. Language Resources and Evaluation Journal (At the above address) Sharoff, S. (2006) Open-source Corpora. International Journal of Corpus Linguistics. 11(4), pp 435 -462. Kilgariff and Grefenstette (2003). Introduction to Special Issue on the Web as Corpus. Computational Linguistics 29(3), 333348 Kilgariff (2007) Googleology is Bad Science, Computational Linguistics, 33(1). Web As Corpus Workshops. See proceedings of the 2008 one in the link below under conferences: http: //webascorpus. sourceforge. net/ 16. 12. 2021 COGS 523 - Bilge Say 2
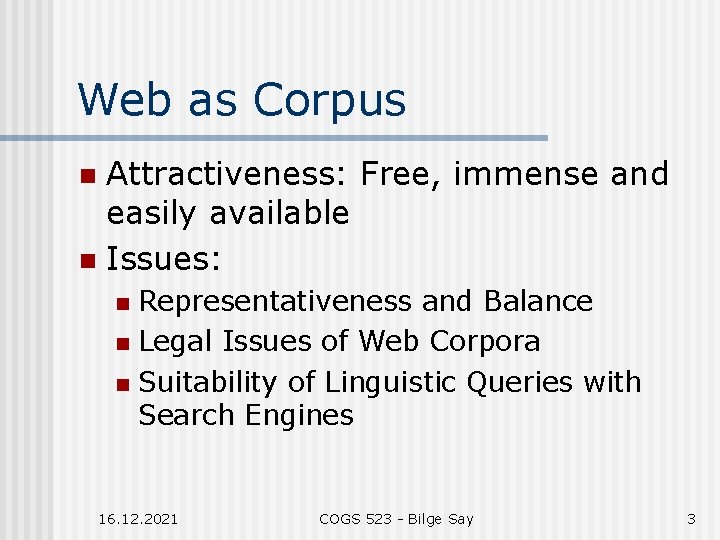
Web as Corpus Attractiveness: Free, immense and easily available n Issues: n Representativeness and Balance n Legal Issues of Web Corpora n Suitability of Linguistic Queries with Search Engines n 16. 12. 2021 COGS 523 - Bilge Say 3
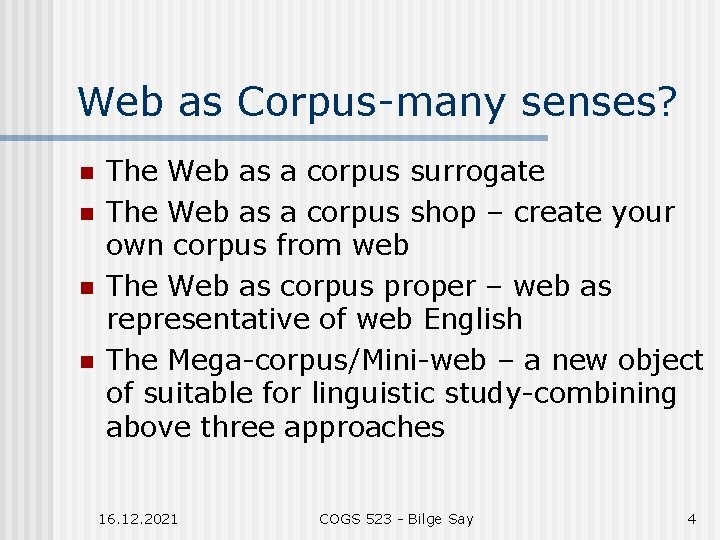
Web as Corpus-many senses? n n The Web as a corpus surrogate The Web as a corpus shop – create your own corpus from web The Web as corpus proper – web as representative of web English The Mega-corpus/Mini-web – a new object of suitable for linguistic study-combining above three approaches 16. 12. 2021 COGS 523 - Bilge Say 4
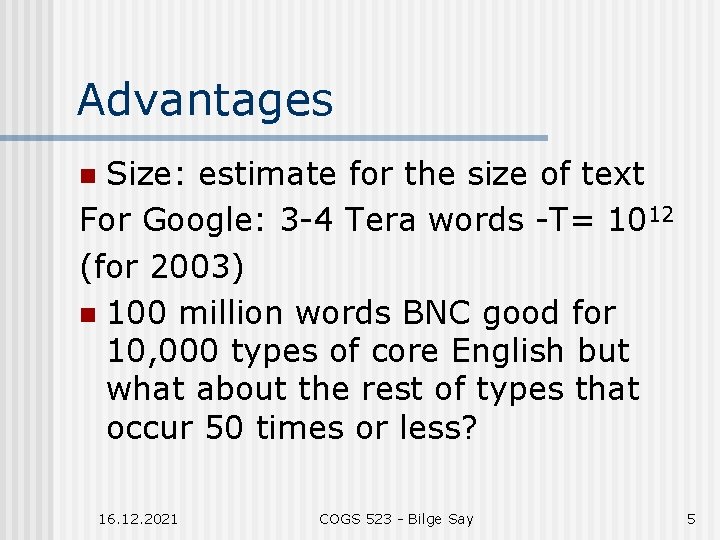
Advantages Size: estimate for the size of text For Google: 3 -4 Tera words -T= 1012 (for 2003) n 100 million words BNC good for 10, 000 types of core English but what about the rest of types that occur 50 times or less? n 16. 12. 2021 COGS 523 - Bilge Say 5
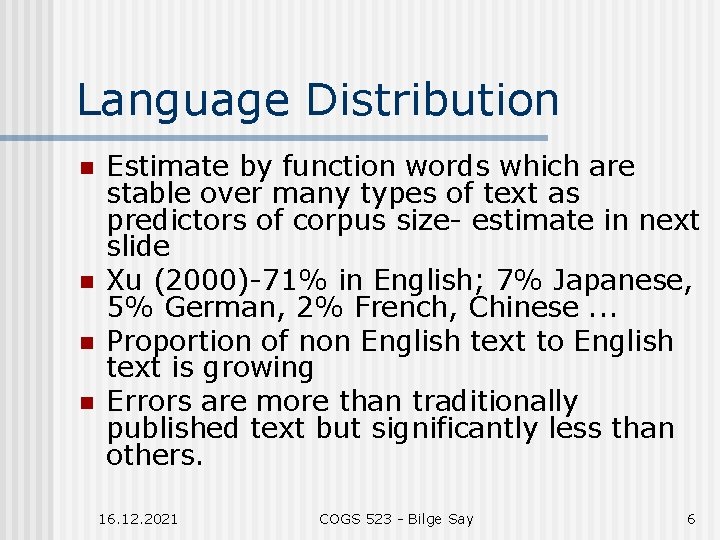
Language Distribution n n Estimate by function words which are stable over many types of text as predictors of corpus size- estimate in next slide Xu (2000)-71% in English; 7% Japanese, 5% German, 2% French, Chinese. . . Proportion of non English text to English text is growing Errors are more than traditionally published text but significantly less than others. 16. 12. 2021 COGS 523 - Bilge Say 6
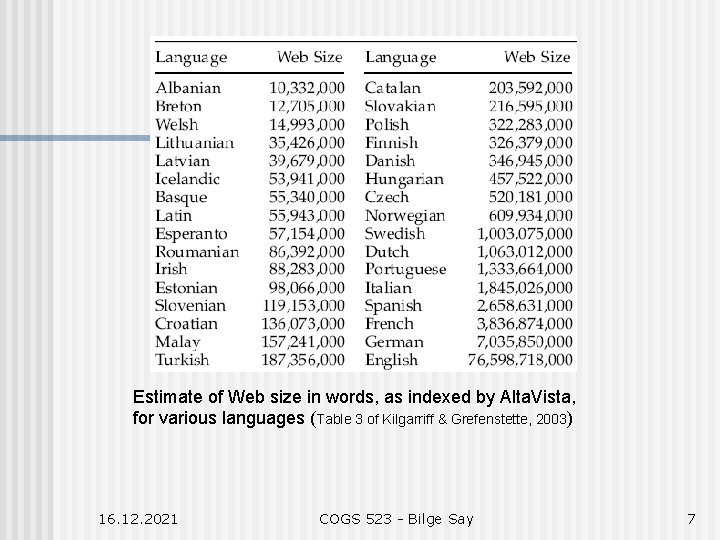
Estimate of Web size in words, as indexed by Alta. Vista, for various languages (Table 3 of Kilgarriff & Grefenstette, 2003) 16. 12. 2021 COGS 523 - Bilge Say 7
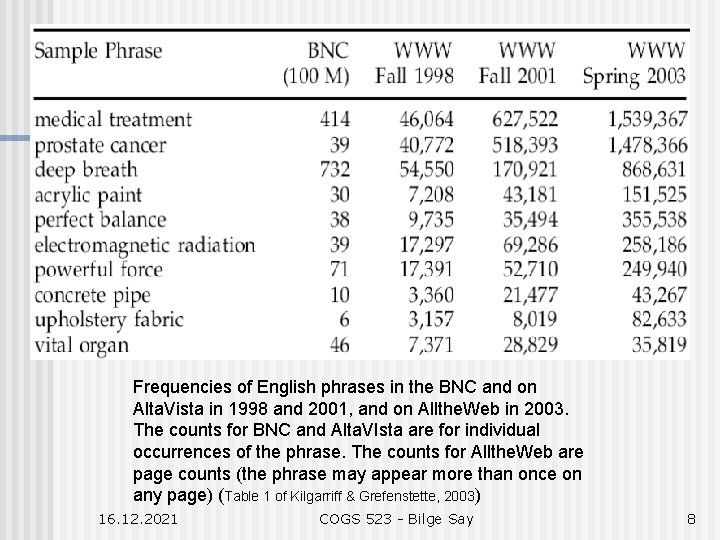
Frequencies of English phrases in the BNC and on Alta. Vista in 1998 and 2001, and on Allthe. Web in 2003. The counts for BNC and Alta. VIsta are for individual occurrences of the phrase. The counts for Allthe. Web are page counts (the phrase may appear more than once on any page) (Table 1 of Kilgarriff & Grefenstette, 2003) 16. 12. 2021 COGS 523 - Bilge Say 8
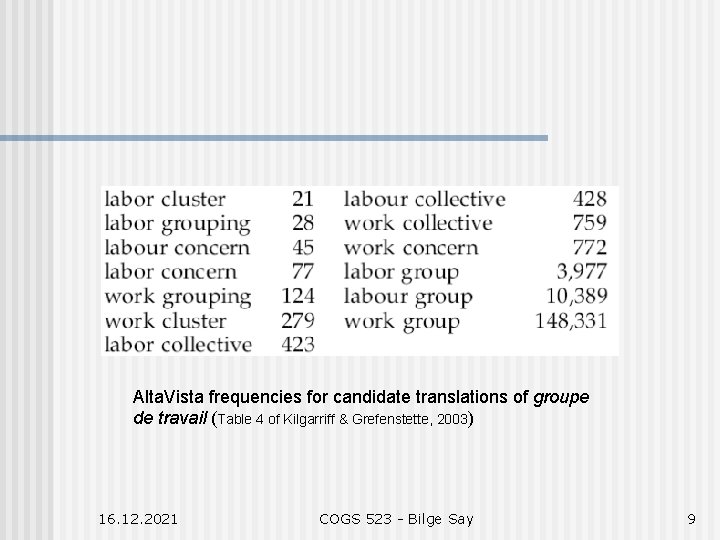
Alta. Vista frequencies for candidate translations of groupe de travail (Table 4 of Kilgarriff & Grefenstette, 2003) 16. 12. 2021 COGS 523 - Bilge Say 9
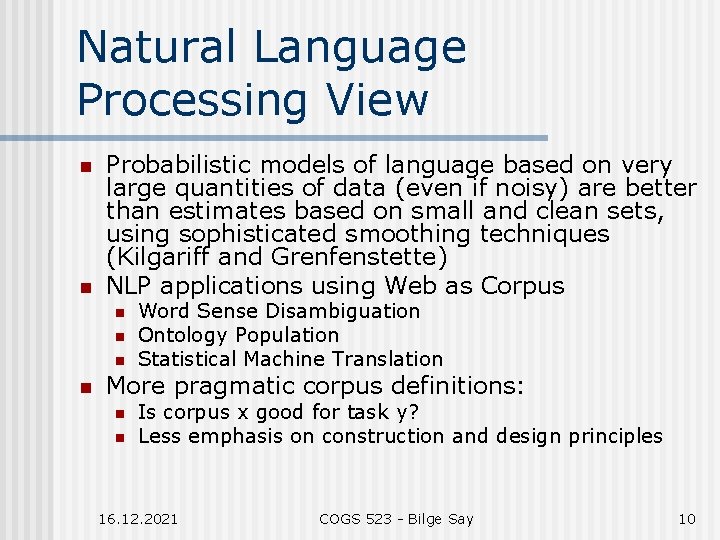
Natural Language Processing View n n Probabilistic models of language based on very large quantities of data (even if noisy) are better than estimates based on small and clean sets, using sophisticated smoothing techniques (Kilgariff and Grenfenstette) NLP applications using Web as Corpus n n Word Sense Disambiguation Ontology Population Statistical Machine Translation More pragmatic corpus definitions: n n Is corpus x good for task y? Less emphasis on construction and design principles 16. 12. 2021 COGS 523 - Bilge Say 10
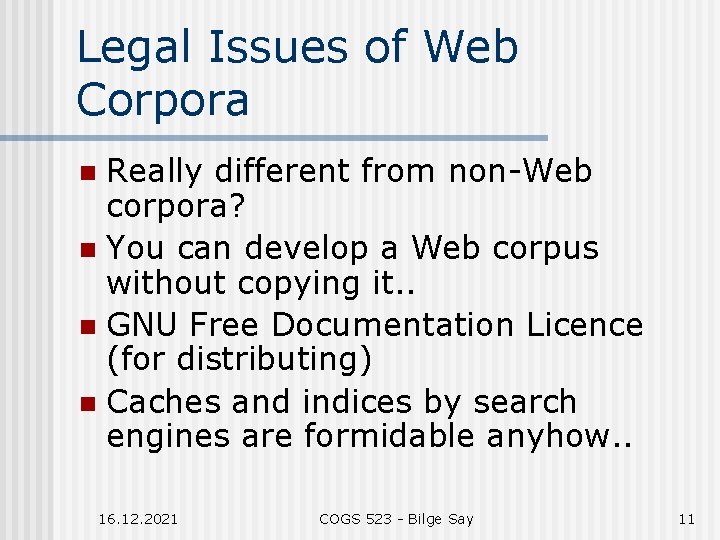
Legal Issues of Web Corpora Really different from non-Web corpora? n You can develop a Web corpus without copying it. . n GNU Free Documentation Licence (for distributing) n Caches and indices by search engines are formidable anyhow. . n 16. 12. 2021 COGS 523 - Bilge Say 11
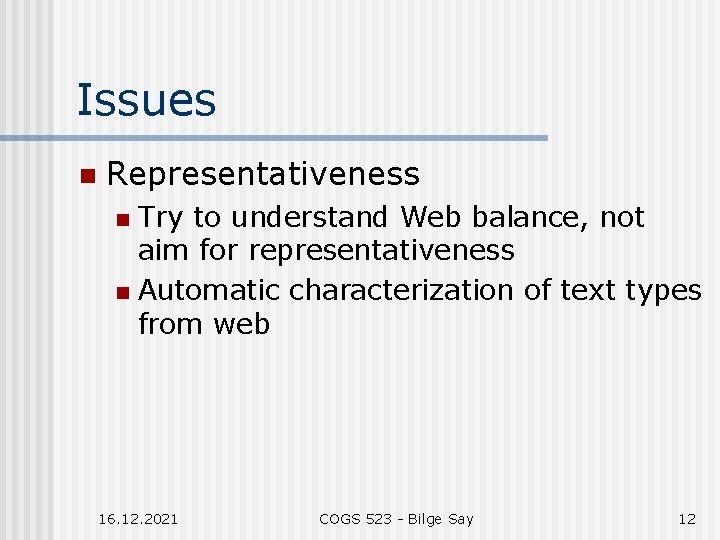
Issues n Representativeness Try to understand Web balance, not aim for representativeness n Automatic characterization of text types from web n 16. 12. 2021 COGS 523 - Bilge Say 12
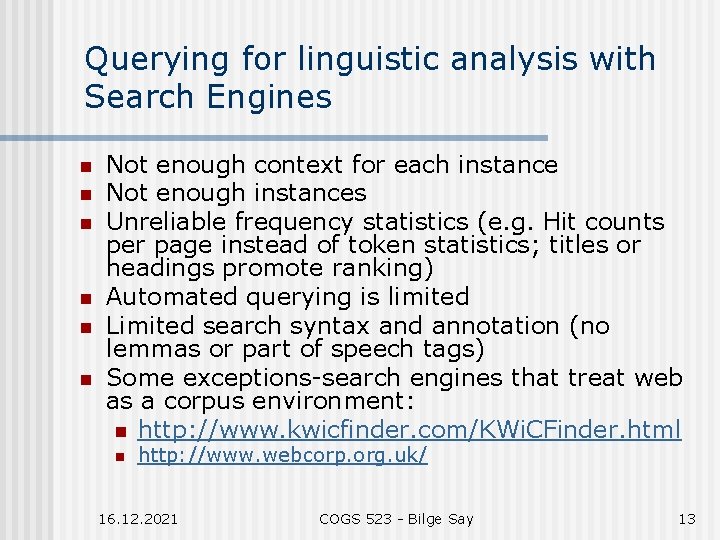
Querying for linguistic analysis with Search Engines n n n Not enough context for each instance Not enough instances Unreliable frequency statistics (e. g. Hit counts per page instead of token statistics; titles or headings promote ranking) Automated querying is limited Limited search syntax and annotation (no lemmas or part of speech tags) Some exceptions-search engines that treat web as a corpus environment: n http: //www. kwicfinder. com/KWi. CFinder. html n http: //www. webcorp. org. uk/ 16. 12. 2021 COGS 523 - Bilge Say 13
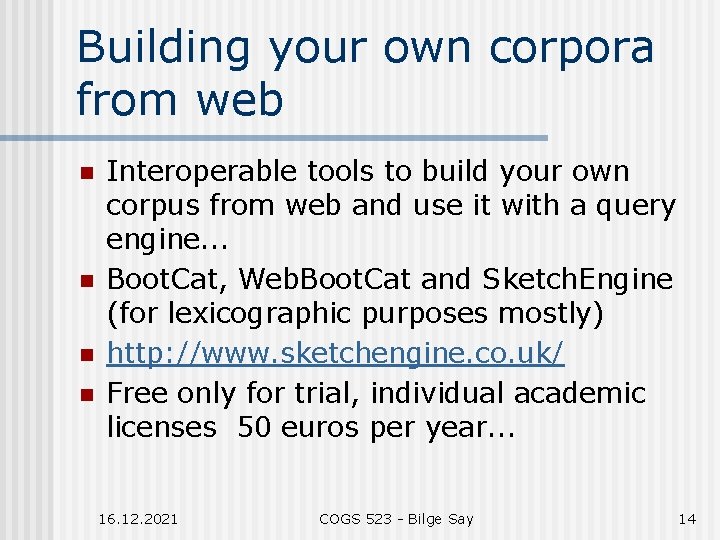
Building your own corpora from web n n Interoperable tools to build your own corpus from web and use it with a query engine. . . Boot. Cat, Web. Boot. Cat and Sketch. Engine (for lexicographic purposes mostly) http: //www. sketchengine. co. uk/ Free only for trial, individual academic licenses 50 euros per year. . . 16. 12. 2021 COGS 523 - Bilge Say 14
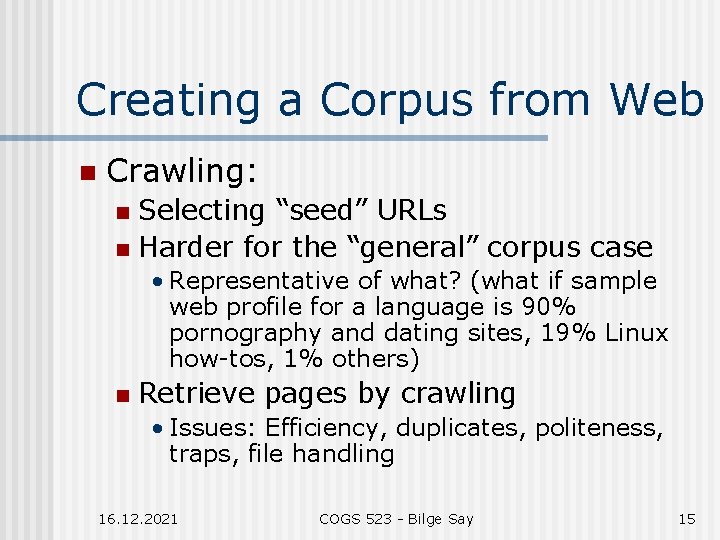
Creating a Corpus from Web n Crawling: Selecting “seed” URLs n Harder for the “general” corpus case n • Representative of what? (what if sample web profile for a language is 90% pornography and dating sites, 19% Linux how-tos, 1% others) n Retrieve pages by crawling • Issues: Efficiency, duplicates, politeness, traps, file handling 16. 12. 2021 COGS 523 - Bilge Say 15
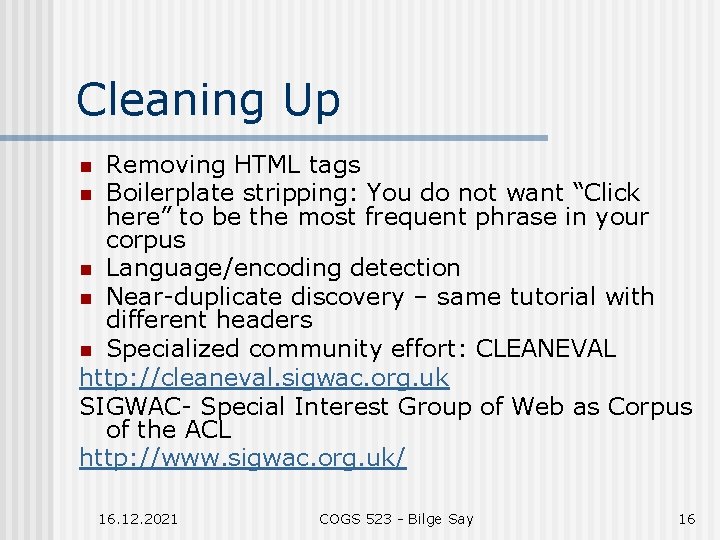
Cleaning Up Removing HTML tags n Boilerplate stripping: You do not want “Click here” to be the most frequent phrase in your corpus n Language/encoding detection n Near-duplicate discovery – same tutorial with different headers n Specialized community effort: CLEANEVAL http: //cleaneval. sigwac. org. uk SIGWAC- Special Interest Group of Web as Corpus of the ACL http: //www. sigwac. org. uk/ n 16. 12. 2021 COGS 523 - Bilge Say 16
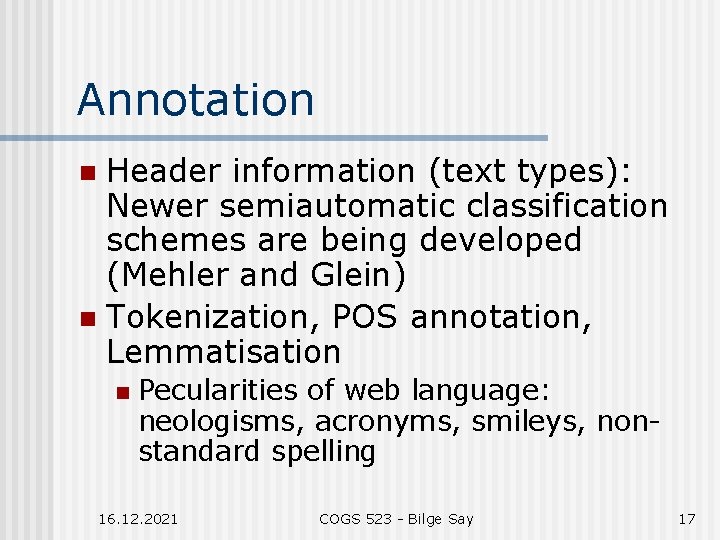
Annotation Header information (text types): Newer semiautomatic classification schemes are being developed (Mehler and Glein) n Tokenization, POS annotation, Lemmatisation n n Pecularities of web language: neologisms, acronyms, smileys, nonstandard spelling 16. 12. 2021 COGS 523 - Bilge Say 17
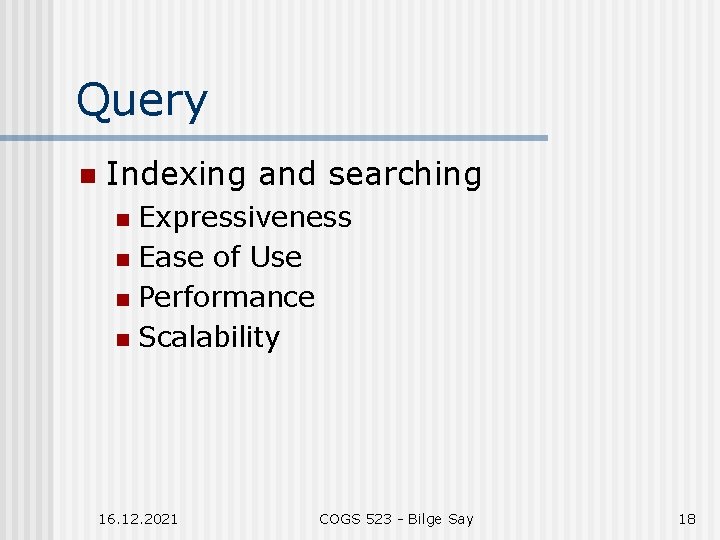
Query n Indexing and searching Expressiveness n Ease of Use n Performance n Scalability n 16. 12. 2021 COGS 523 - Bilge Say 18
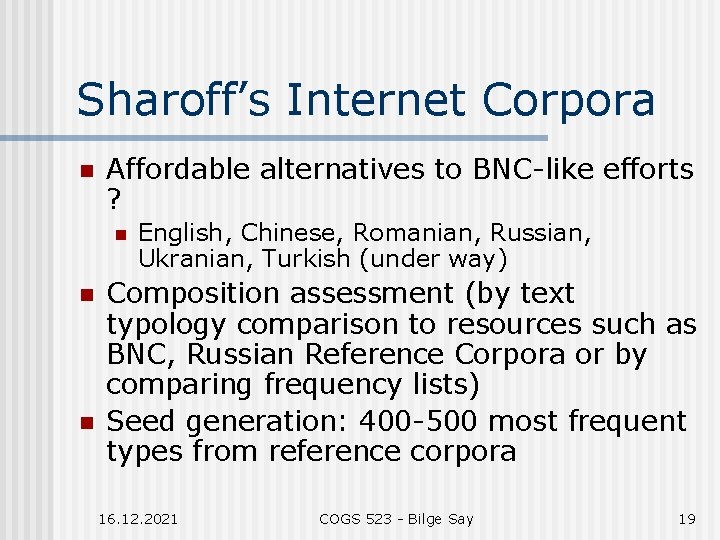
Sharoff’s Internet Corpora n Affordable alternatives to BNC-like efforts ? n n n English, Chinese, Romanian, Russian, Ukranian, Turkish (under way) Composition assessment (by text typology comparison to resources such as BNC, Russian Reference Corpora or by comparing frequency lists) Seed generation: 400 -500 most frequent types from reference corpora 16. 12. 2021 COGS 523 - Bilge Say 19
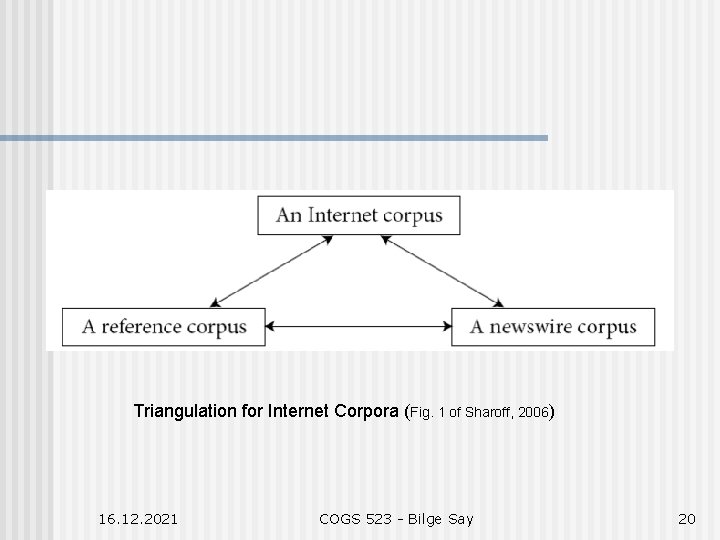
Triangulation for Internet Corpora (Fig. 1 of Sharoff, 2006) 16. 12. 2021 COGS 523 - Bilge Say 20
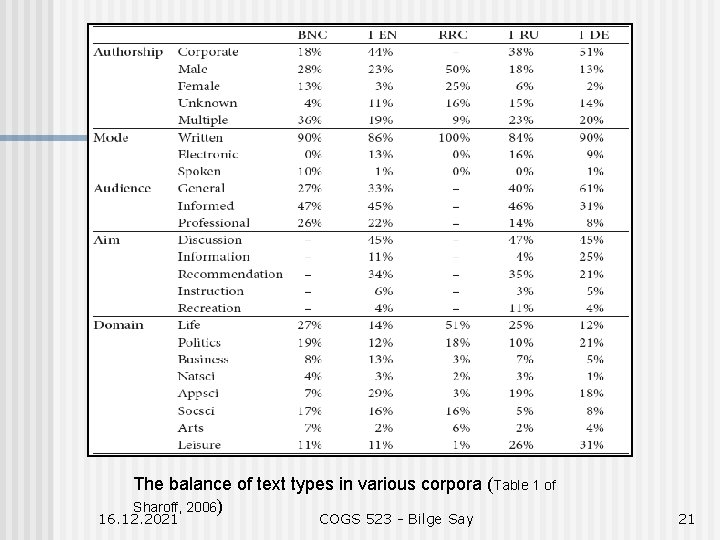
The balance of text types in various corpora (Table 1 of Sharoff, 2006) 16. 12. 2021 COGS 523 - Bilge Say 21
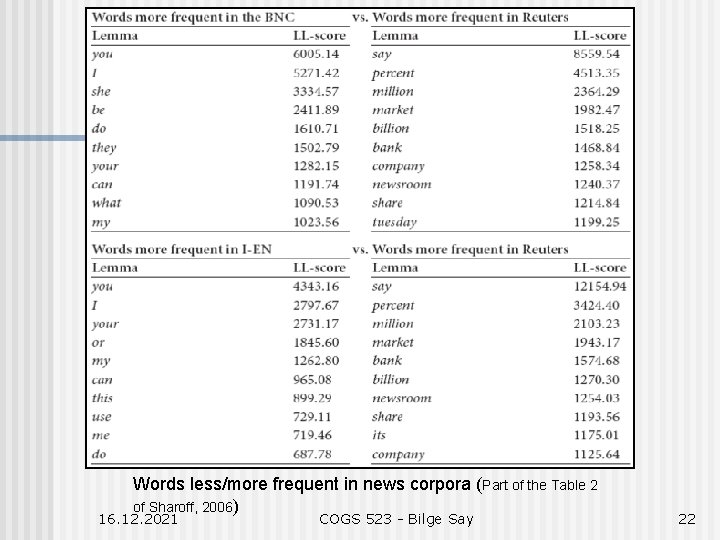
Words less/more frequent in news corpora (Part of the Table 2 of Sharoff, 2006) 16. 12. 2021 COGS 523 - Bilge Say 22
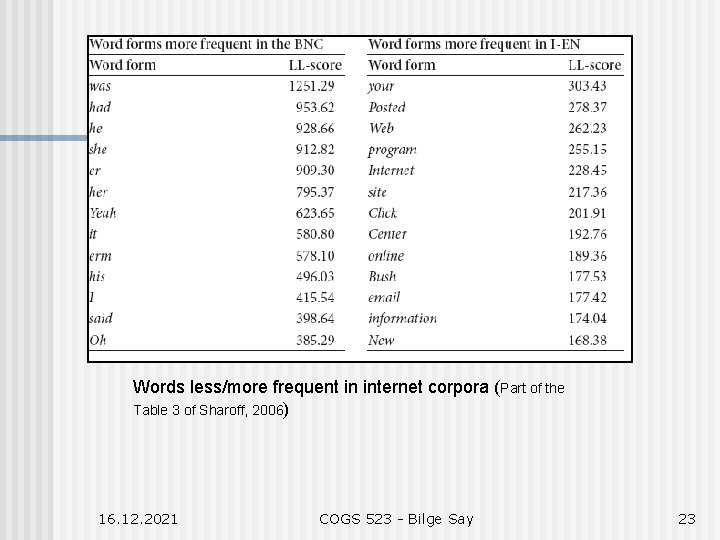
Words less/more frequent in internet corpora (Part of the Table 3 of Sharoff, 2006) 16. 12. 2021 COGS 523 - Bilge Say 23
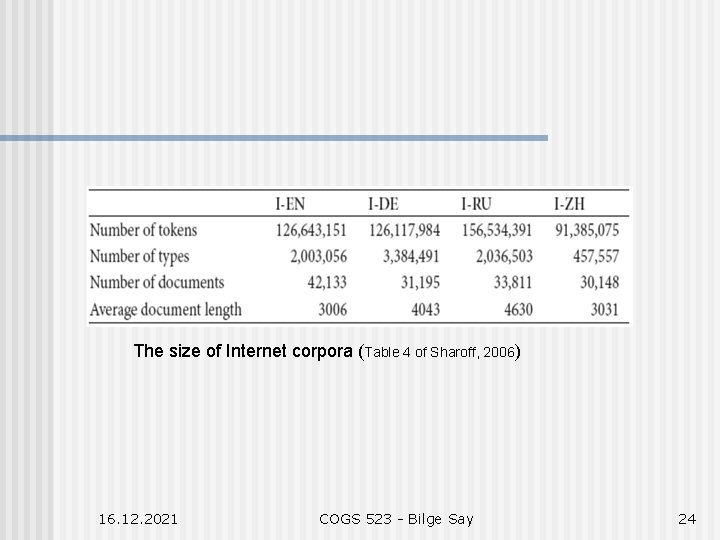
The size of Internet corpora (Table 4 of Sharoff, 2006) 16. 12. 2021 COGS 523 - Bilge Say 24
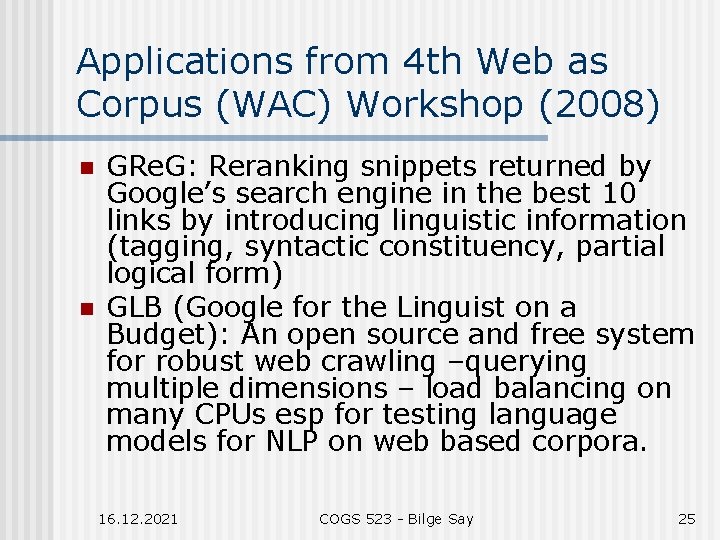
Applications from 4 th Web as Corpus (WAC) Workshop (2008) n n GRe. G: Reranking snippets returned by Google’s search engine in the best 10 links by introducing linguistic information (tagging, syntactic constituency, partial logical form) GLB (Google for the Linguist on a Budget): An open source and free system for robust web crawling –querying multiple dimensions – load balancing on many CPUs esp for testing language models for NLP on web based corpora. 16. 12. 2021 COGS 523 - Bilge Say 25
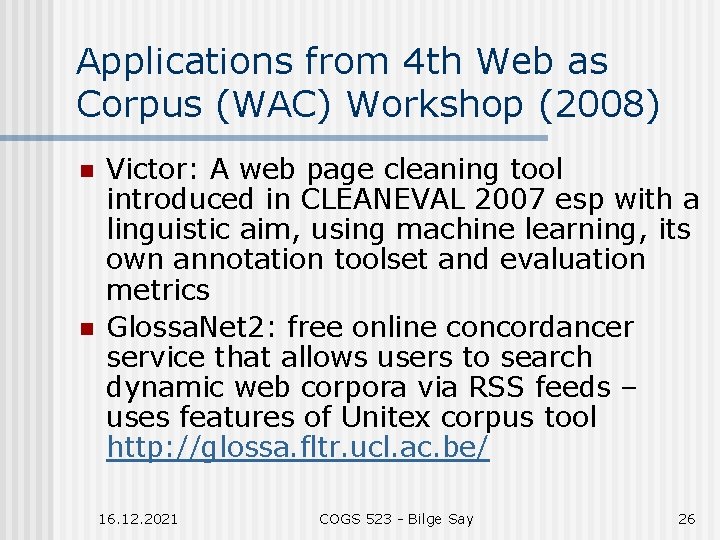
Applications from 4 th Web as Corpus (WAC) Workshop (2008) n n Victor: A web page cleaning tool introduced in CLEANEVAL 2007 esp with a linguistic aim, using machine learning, its own annotation toolset and evaluation metrics Glossa. Net 2: free online concordancer service that allows users to search dynamic web corpora via RSS feeds – uses features of Unitex corpus tool http: //glossa. fltr. ucl. ac. be/ 16. 12. 2021 COGS 523 - Bilge Say 26
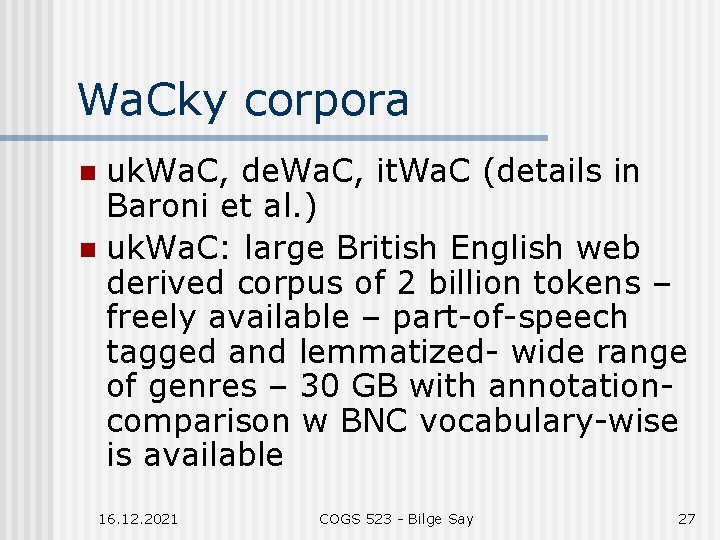
Wa. Cky corpora uk. Wa. C, de. Wa. C, it. Wa. C (details in Baroni et al. ) n uk. Wa. C: large British English web derived corpus of 2 billion tokens – freely available – part-of-speech tagged and lemmatized- wide range of genres – 30 GB with annotationcomparison w BNC vocabulary-wise is available n 16. 12. 2021 COGS 523 - Bilge Say 27
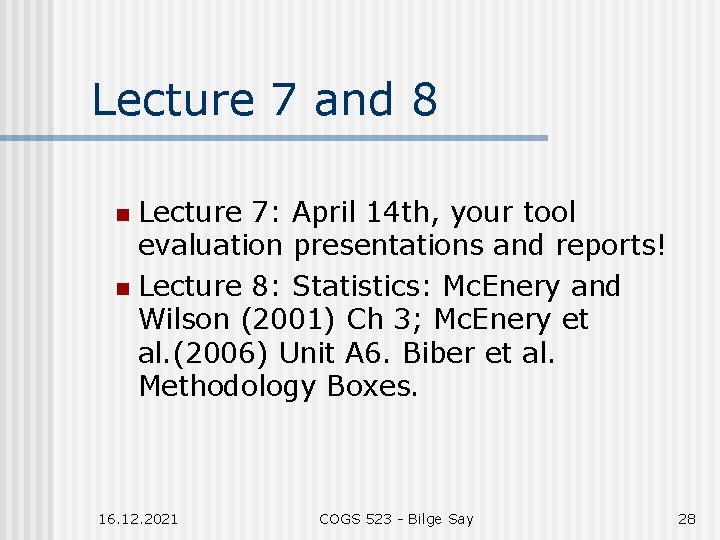
Lecture 7 and 8 Lecture 7: April 14 th, your tool evaluation presentations and reports! n Lecture 8: Statistics: Mc. Enery and Wilson (2001) Ch 3; Mc. Enery et al. (2006) Unit A 6. Biber et al. Methodology Boxes. n 16. 12. 2021 COGS 523 - Bilge Say 28
Income statement beginning inventory
Stand like the brave
Orf 523
Ba 523
Dpv08
Cs 523
Hymn 523
523
Comp 523 unc
01:640:244 lecture notes - lecture 15: plat, idah, farad
Meninge
Corpora quadrigemina
Dura mater
Vision cheval
Types of corpora
What is corpus
Medulla oblongata
Corpora quadrigemina pronunciation
Corpora amylacea
Corpora aranacea
What is corpus
Help
Motor sensory cranial nerves mnemonic
Research methods notes kenya
Slidetodoc downloader
Process of research definition
Fspos
Typiska drag för en novell
Nationell inriktning för artificiell intelligens