UNCERTAINTY AROUND MODELED LOSS ESTIMATES CAS Annual Meeting
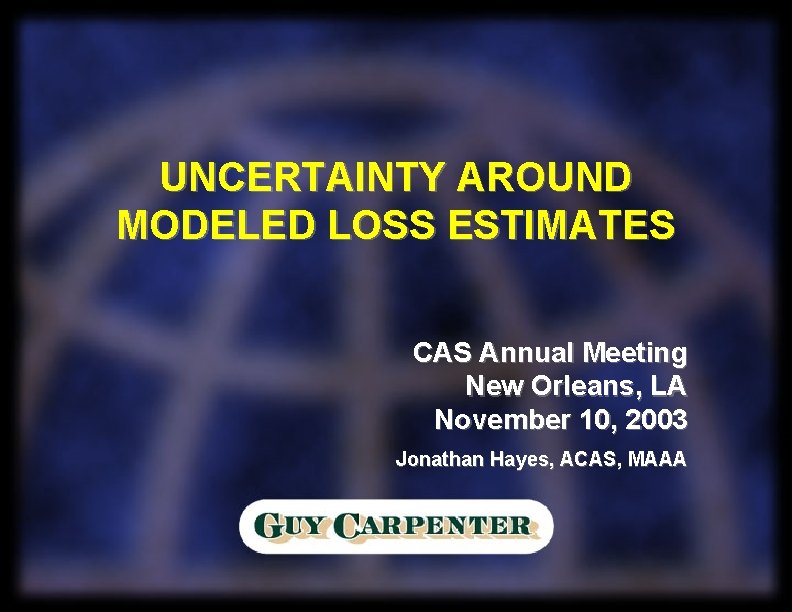
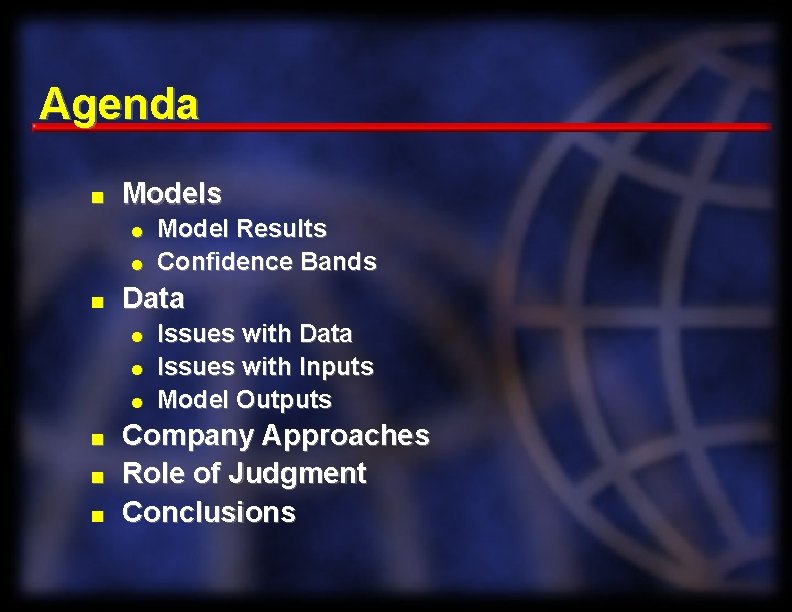
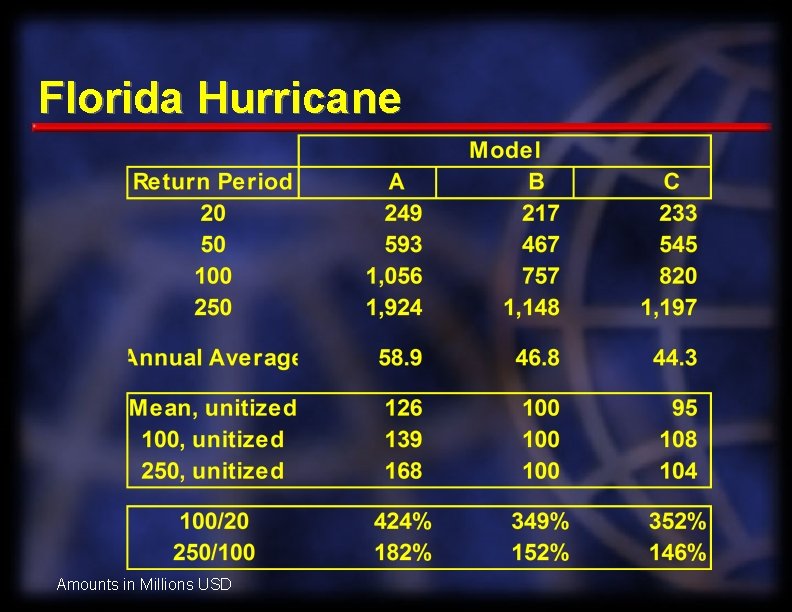
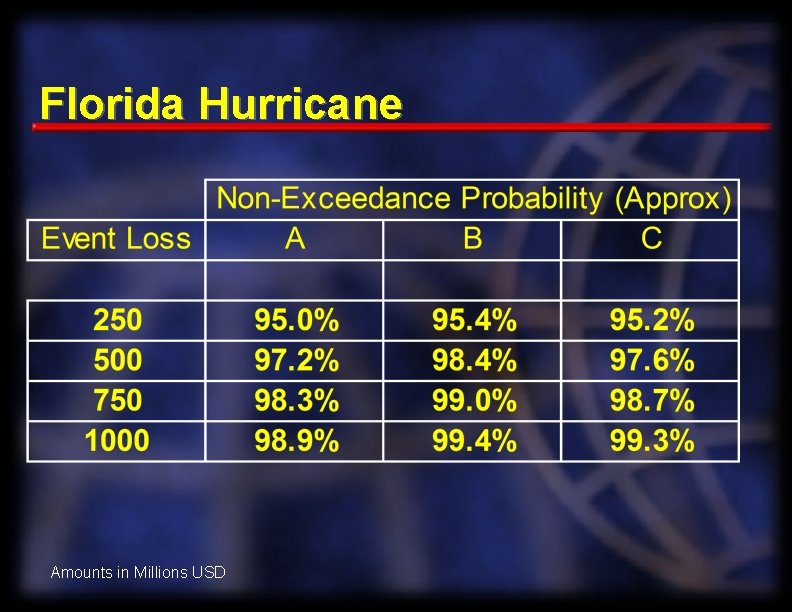
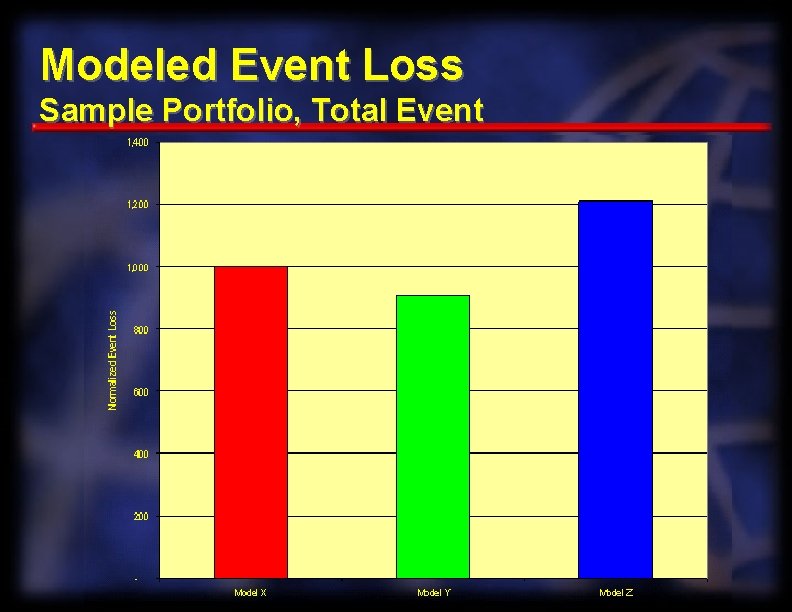
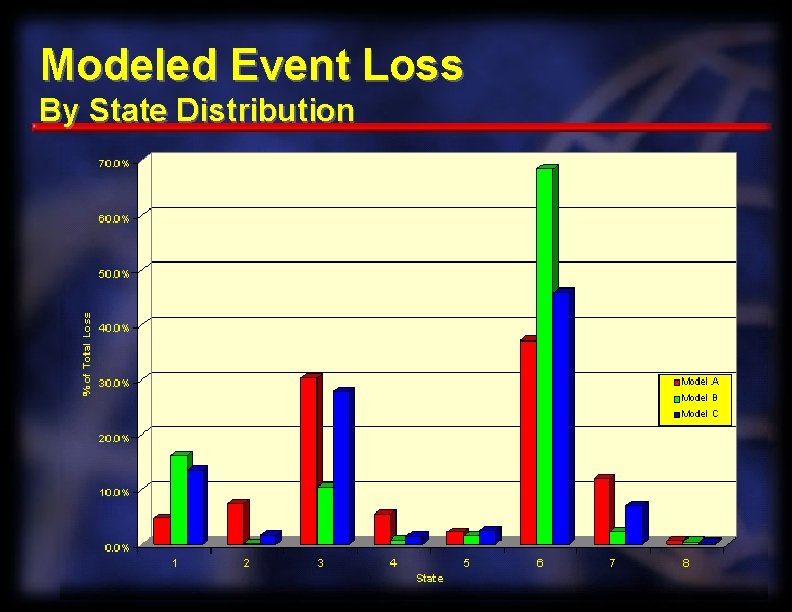
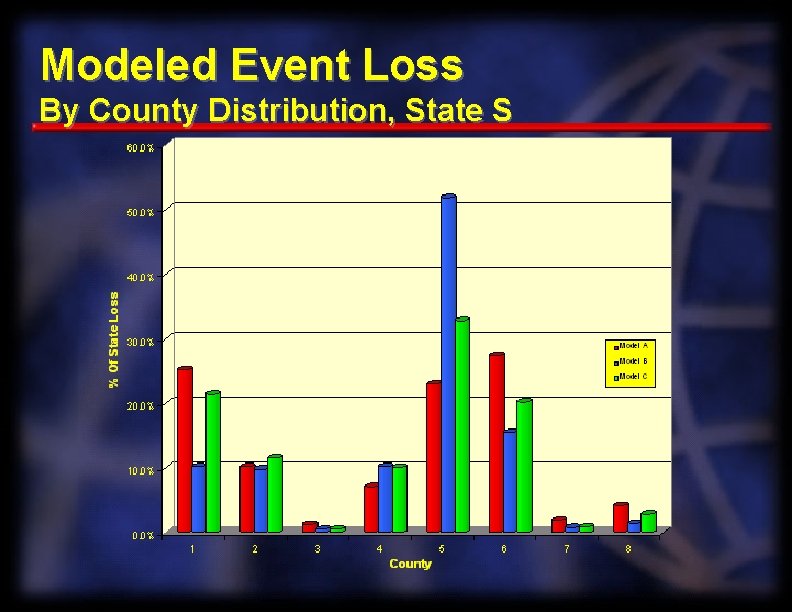
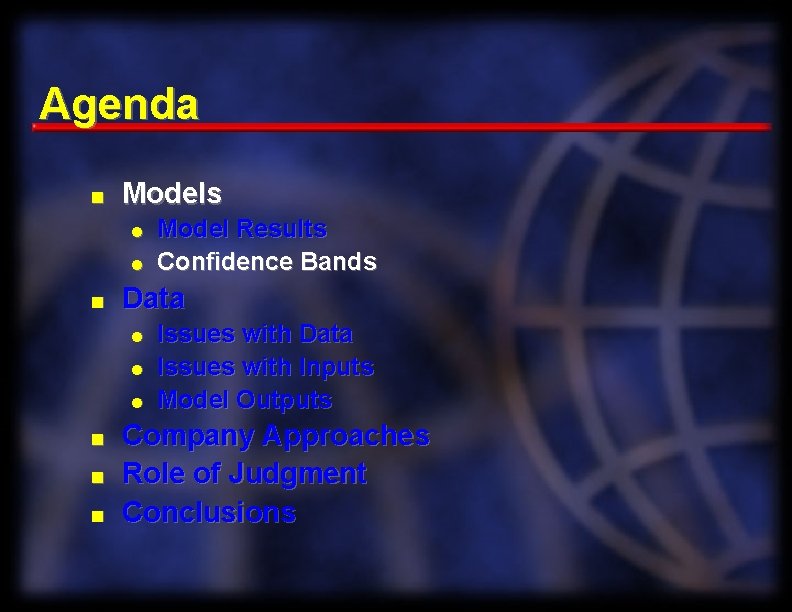
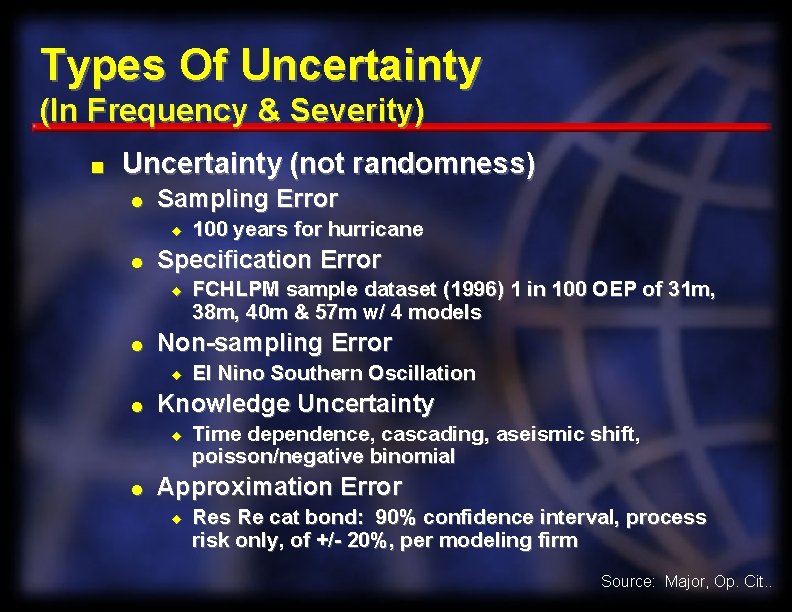
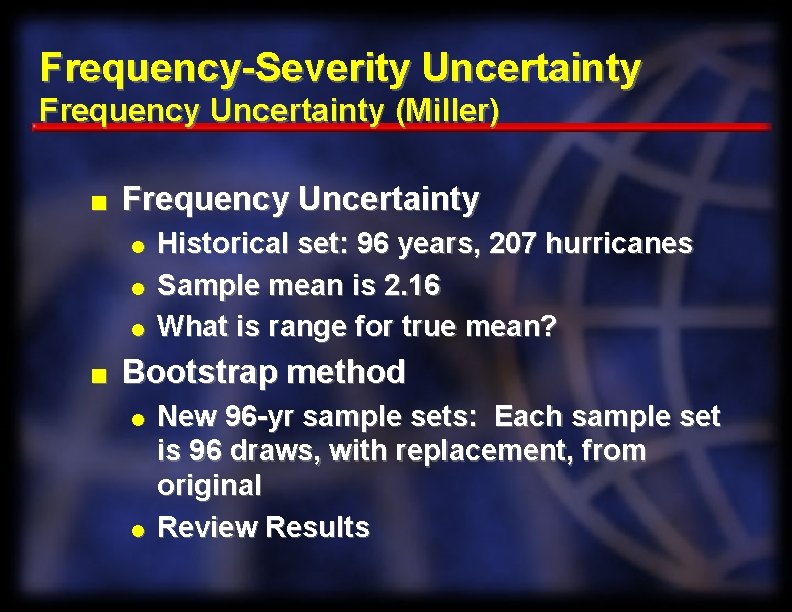
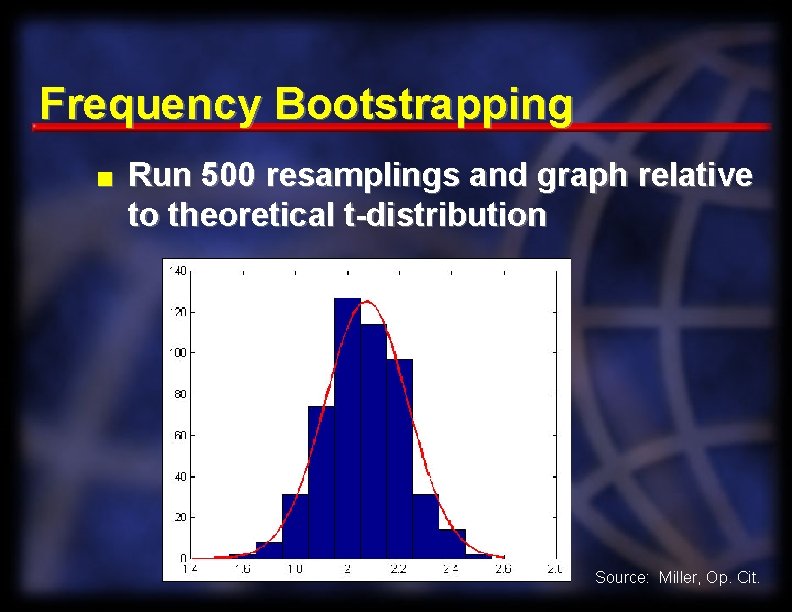
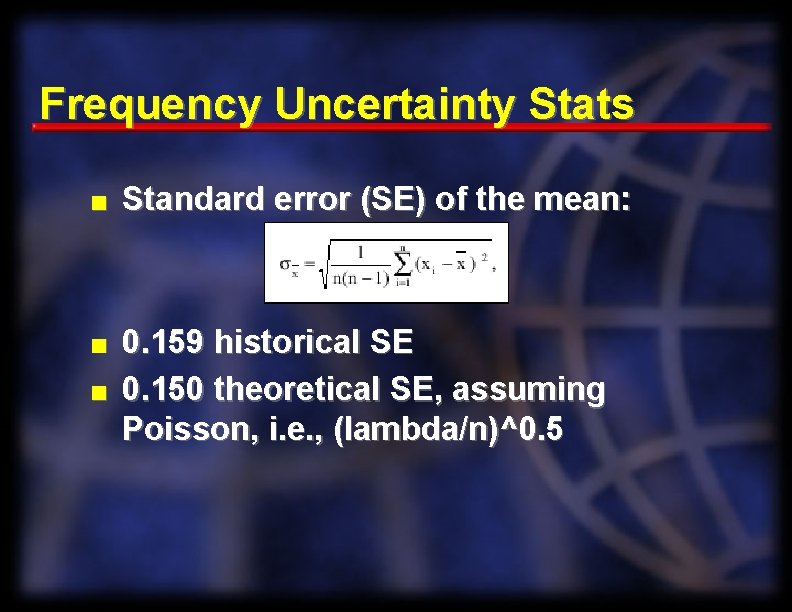
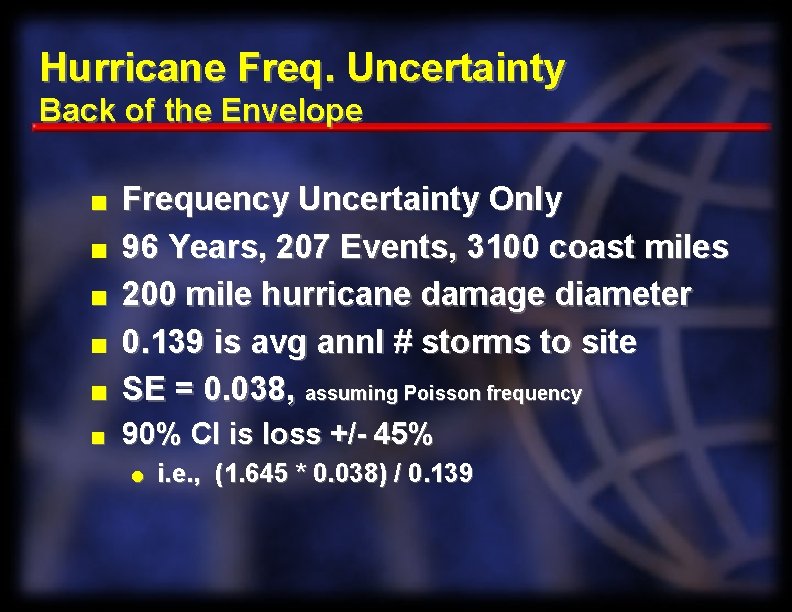
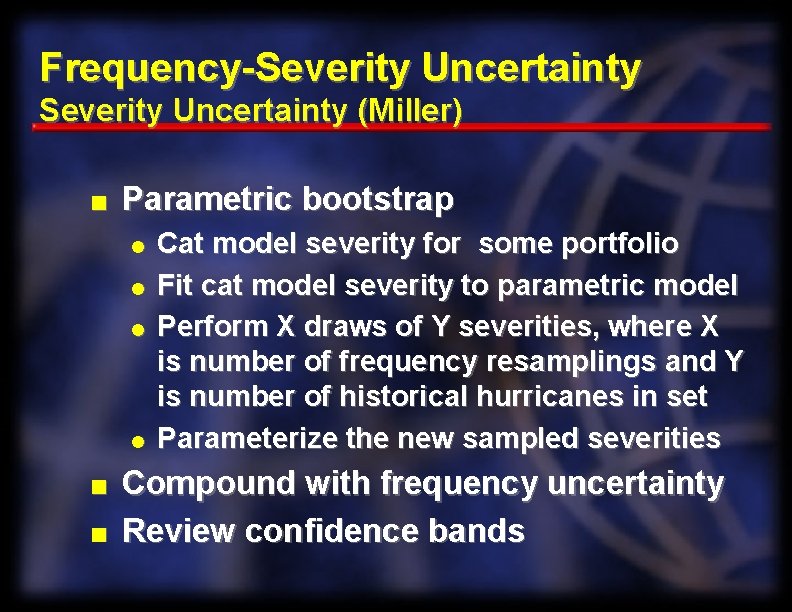
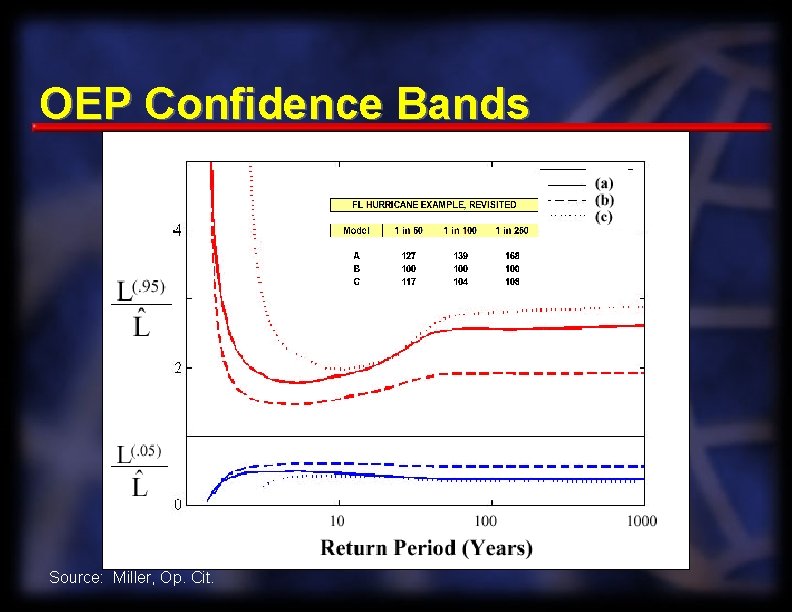
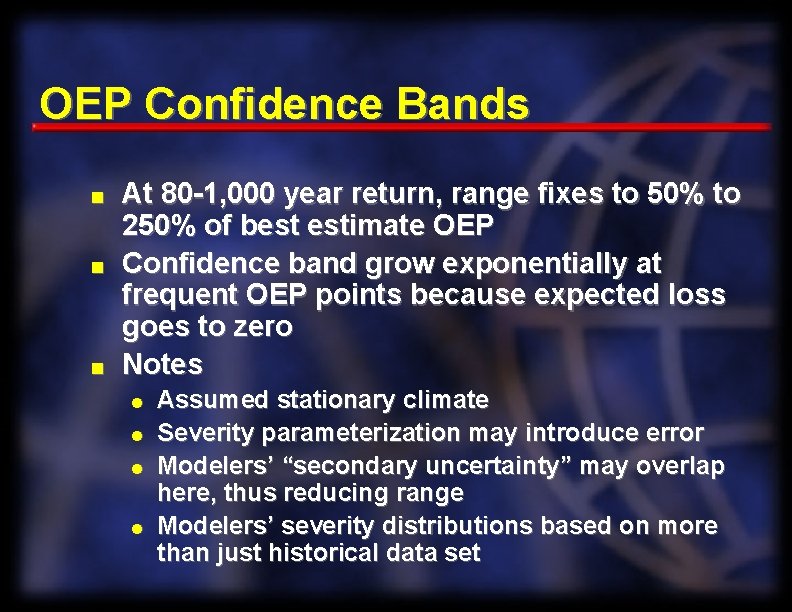
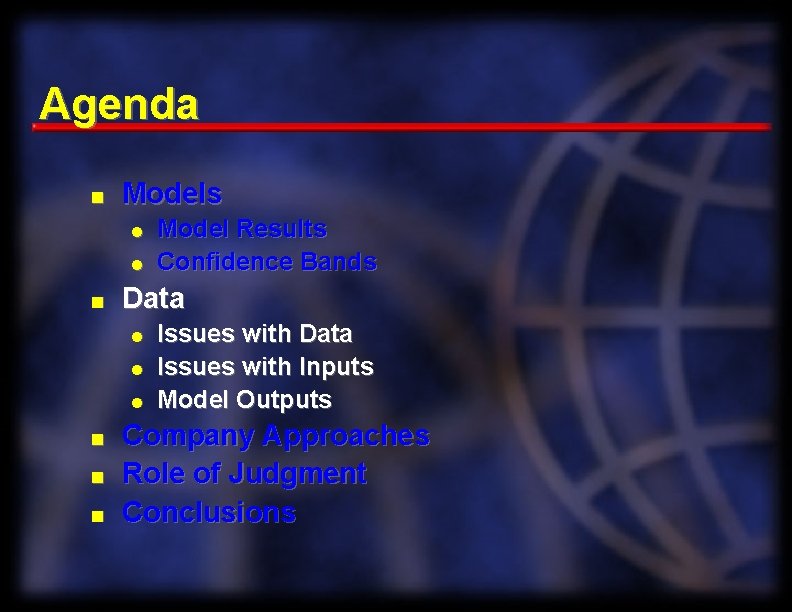
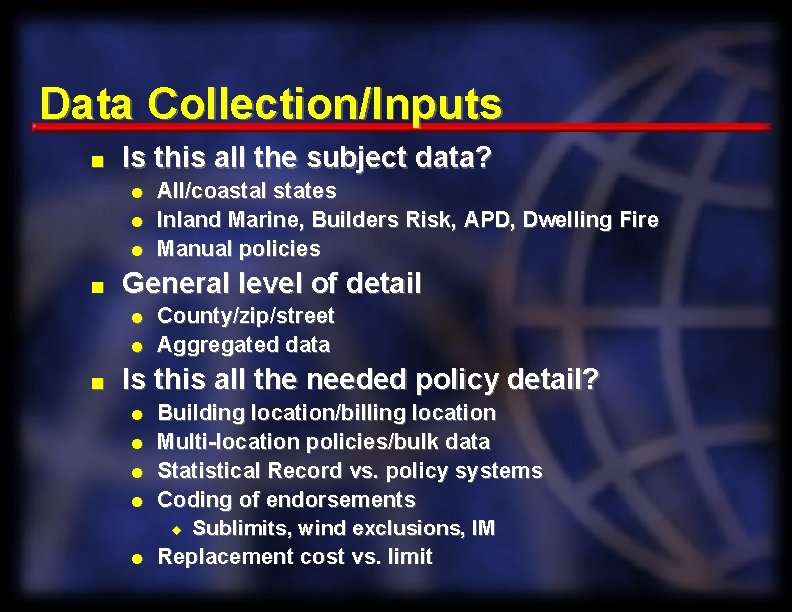
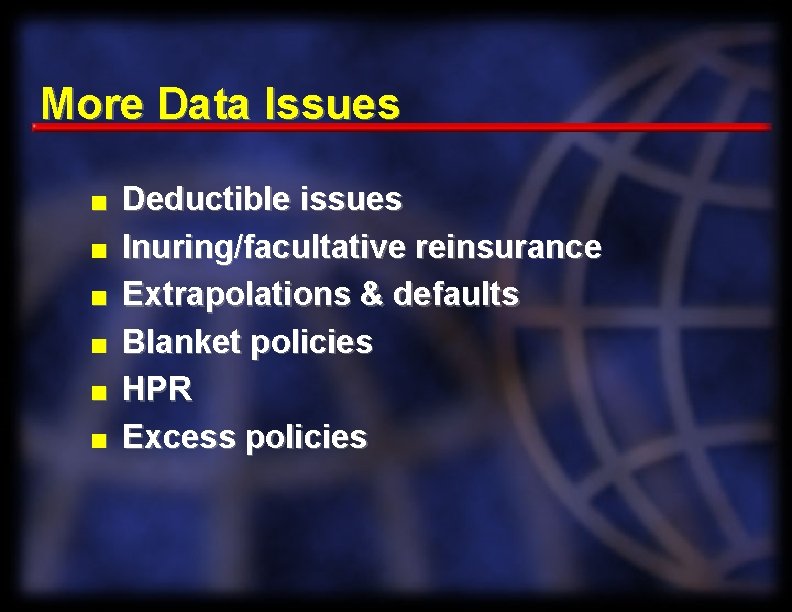
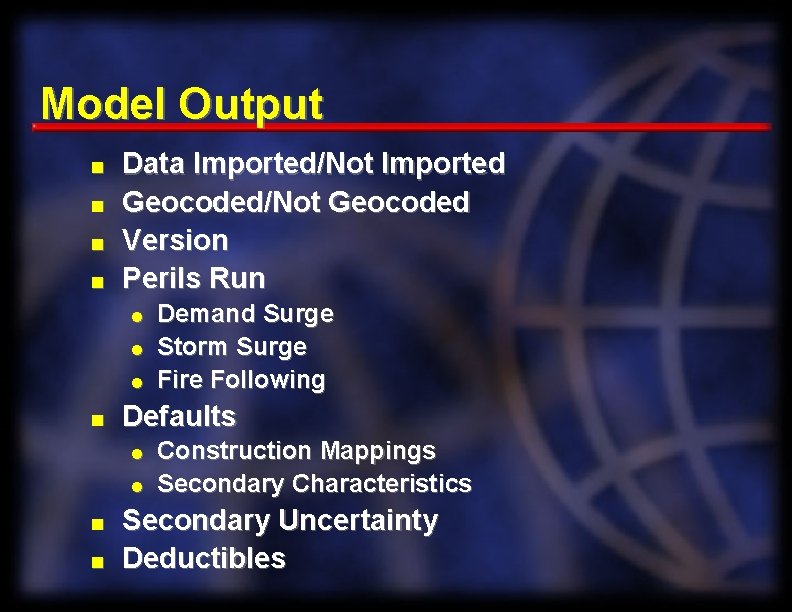
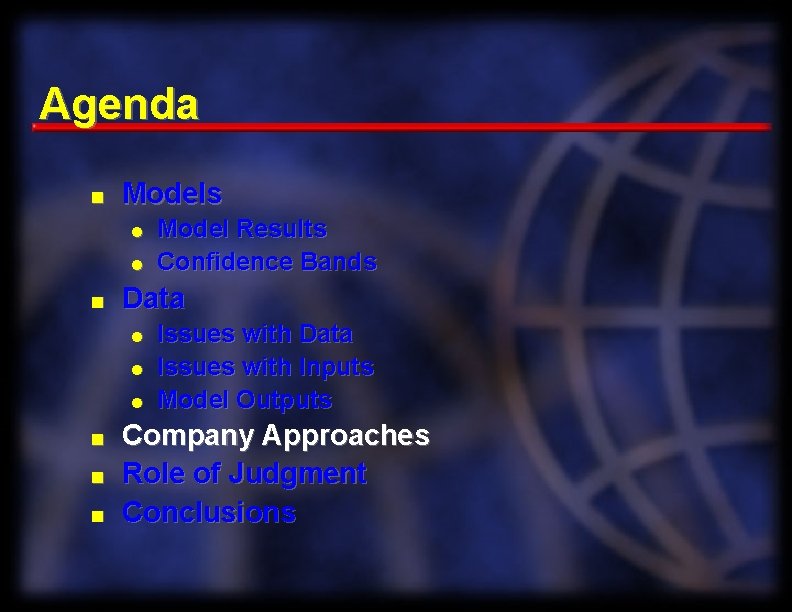
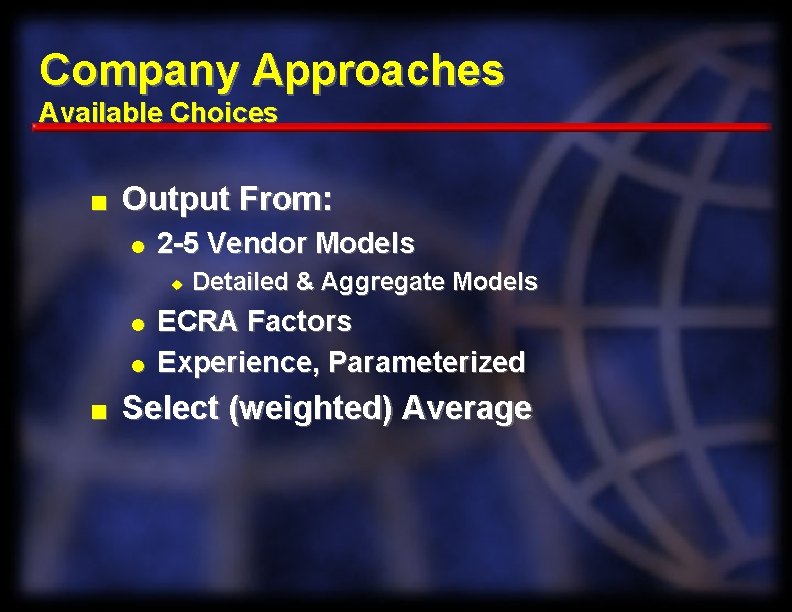
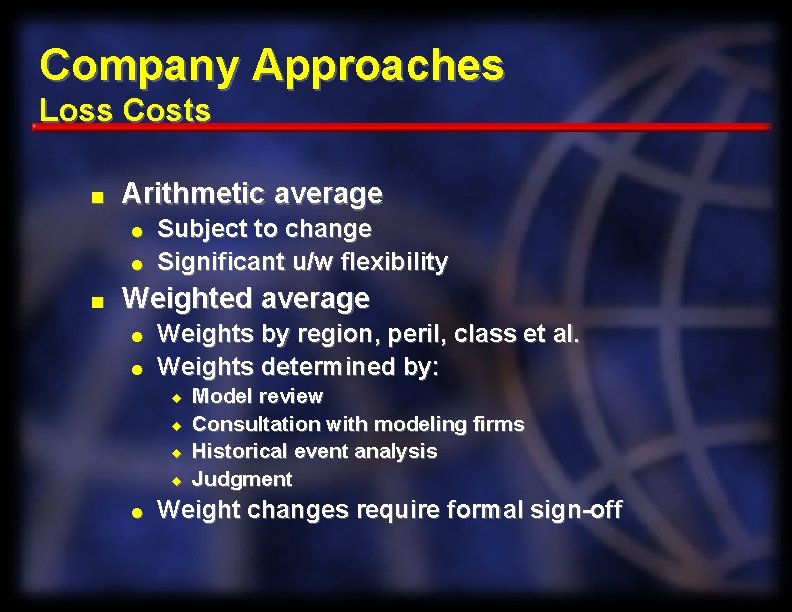
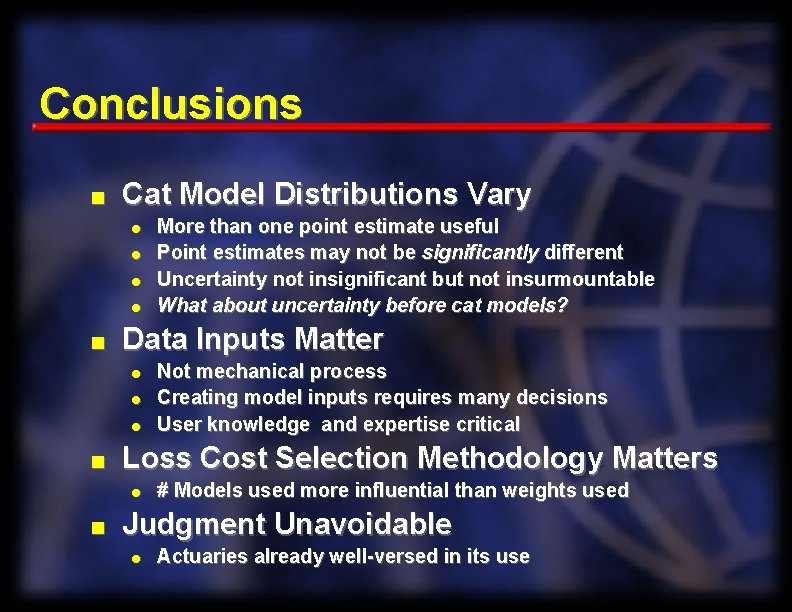
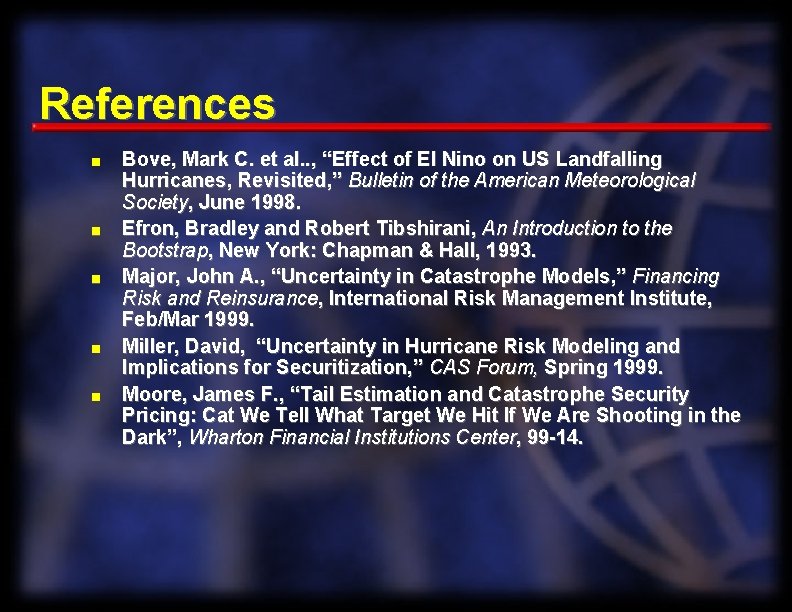
- Slides: 25
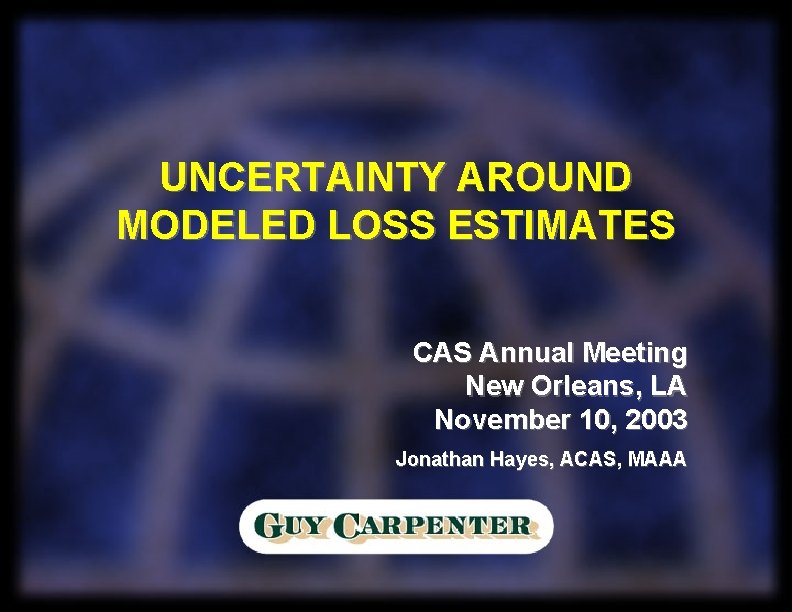
UNCERTAINTY AROUND MODELED LOSS ESTIMATES CAS Annual Meeting New Orleans, LA November 10, 2003 Jonathan Hayes, ACAS, MAAA
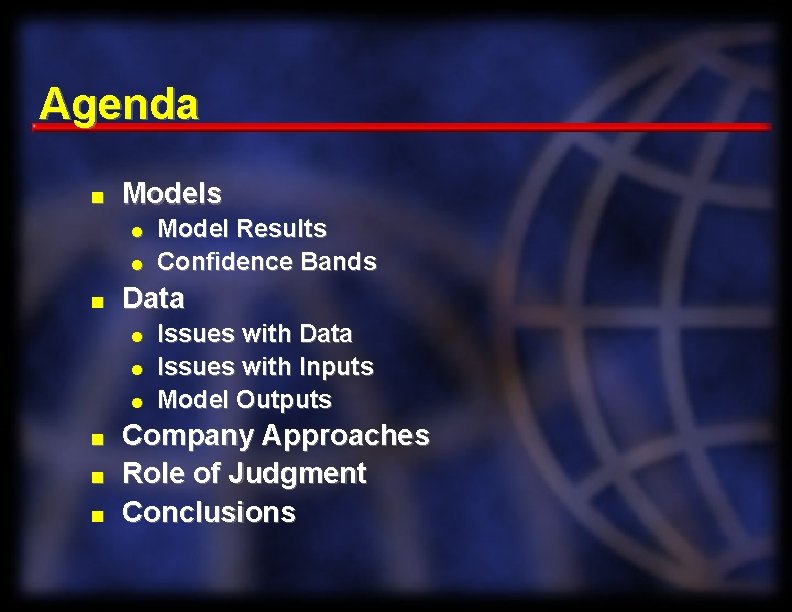
Agenda n Models l l n Data l l l n n n Model Results Confidence Bands Issues with Data Issues with Inputs Model Outputs Company Approaches Role of Judgment Conclusions
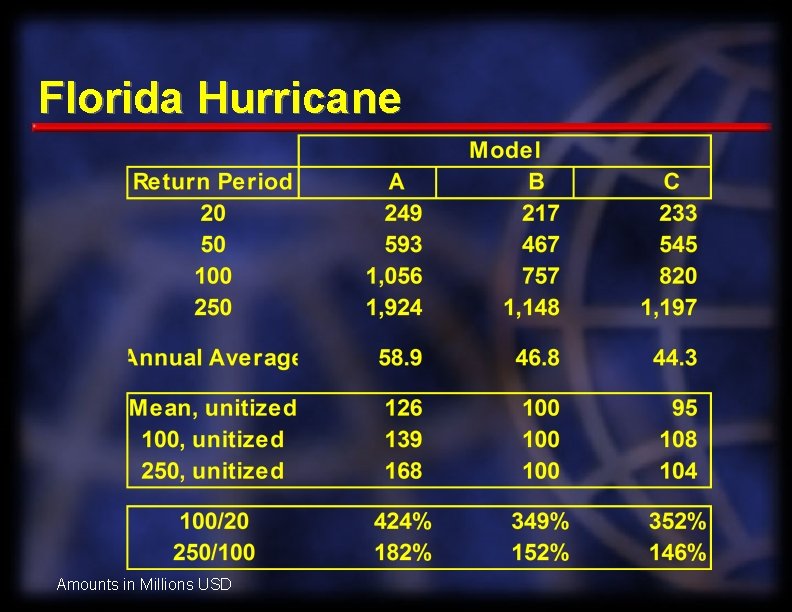
Florida Hurricane Amounts in Millions USD
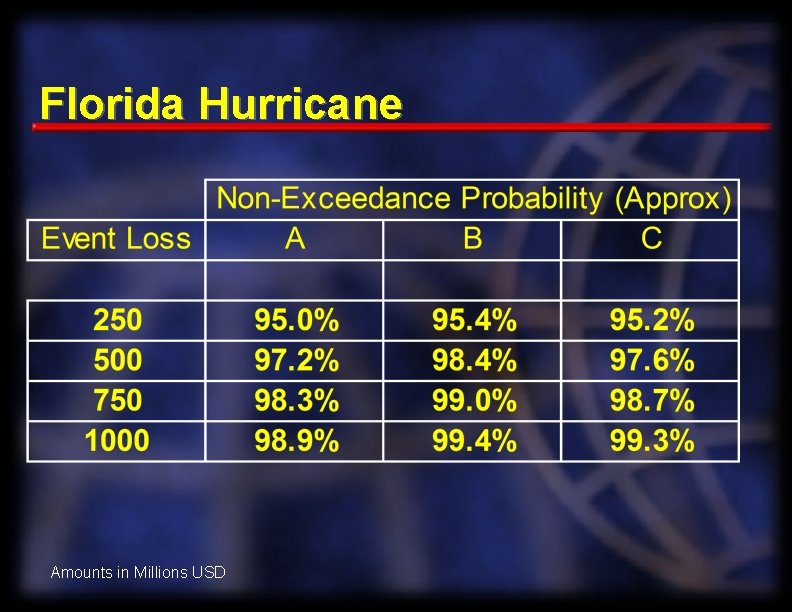
Florida Hurricane Amounts in Millions USD
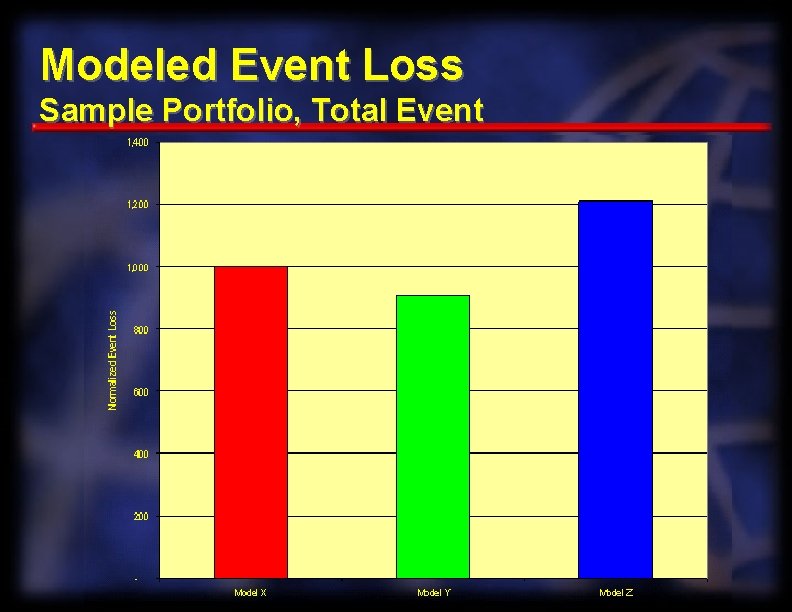
Modeled Event Loss Sample Portfolio, Total Event
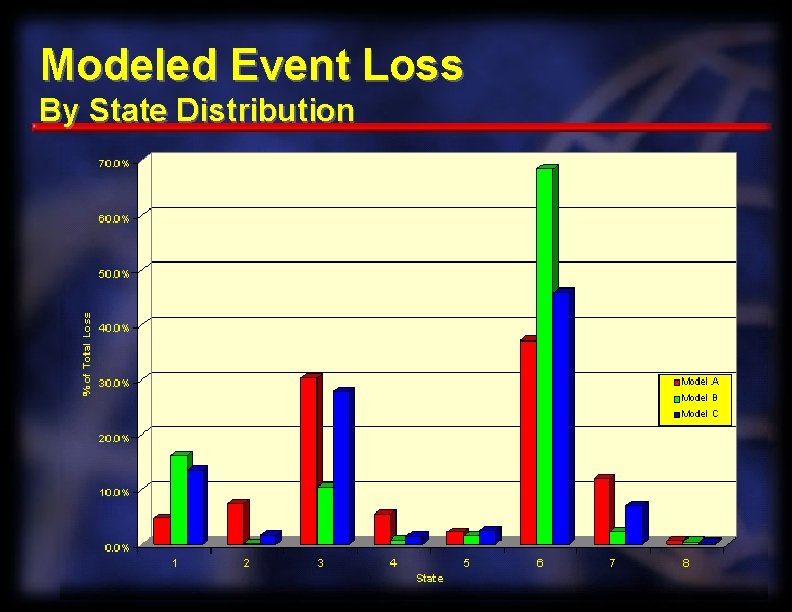
Modeled Event Loss By State Distribution
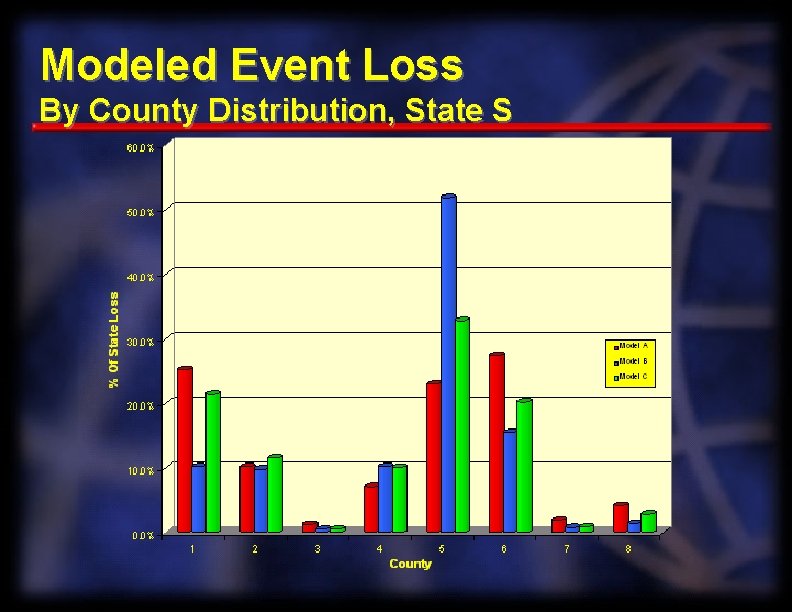
Modeled Event Loss By County Distribution, State S
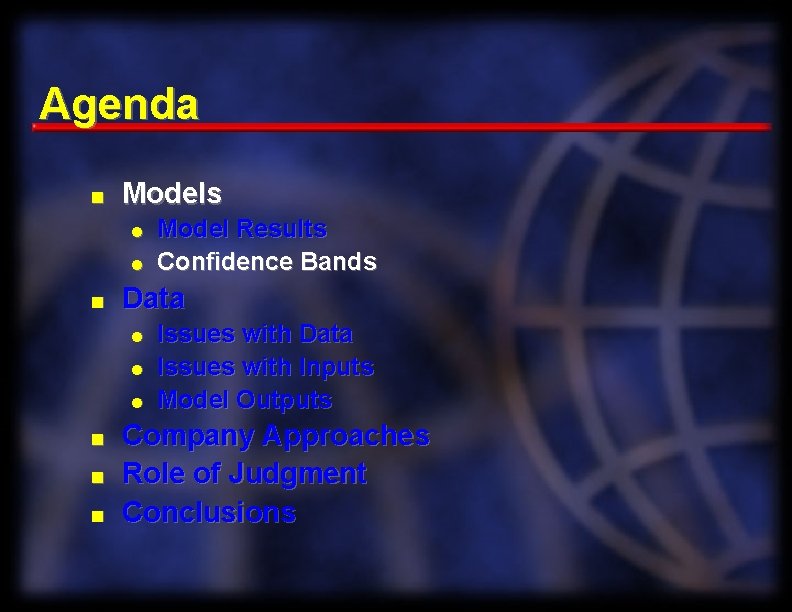
Agenda n Models l l n Data l l l n n n Model Results Confidence Bands Issues with Data Issues with Inputs Model Outputs Company Approaches Role of Judgment Conclusions
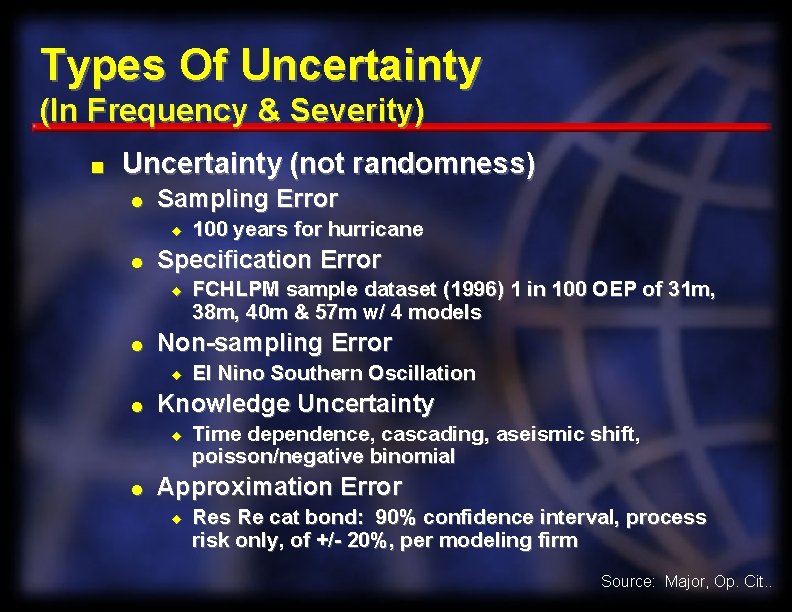
Types Of Uncertainty (In Frequency & Severity) n Uncertainty (not randomness) l Sampling Error u l Specification Error u l El Nino Southern Oscillation Knowledge Uncertainty u l FCHLPM sample dataset (1996) 1 in 100 OEP of 31 m, 38 m, 40 m & 57 m w/ 4 models Non-sampling Error u l 100 years for hurricane Time dependence, cascading, aseismic shift, poisson/negative binomial Approximation Error u Res Re cat bond: 90% confidence interval, process risk only, of +/- 20%, per modeling firm Source: Major, Op. Cit. .
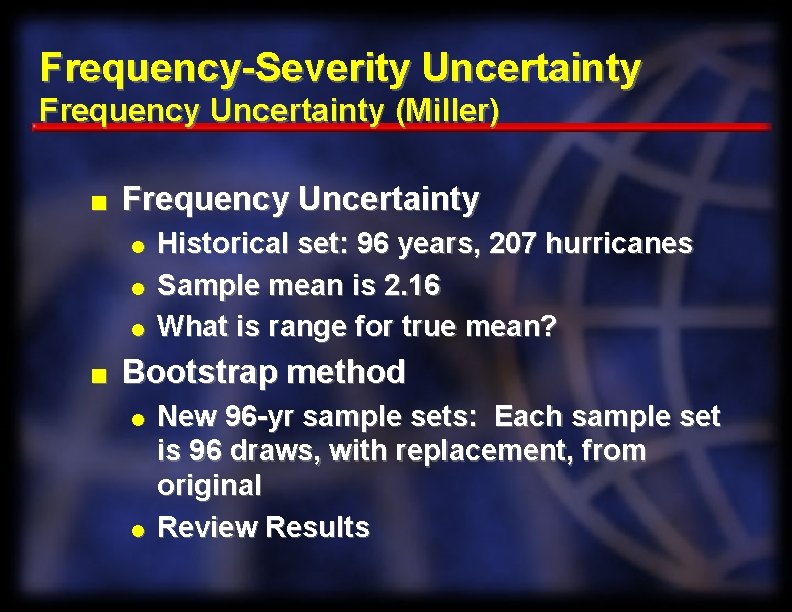
Frequency-Severity Uncertainty Frequency Uncertainty (Miller) n Frequency Uncertainty l l l n Historical set: 96 years, 207 hurricanes Sample mean is 2. 16 What is range for true mean? Bootstrap method l l New 96 -yr sample sets: Each sample set is 96 draws, with replacement, from original Review Results
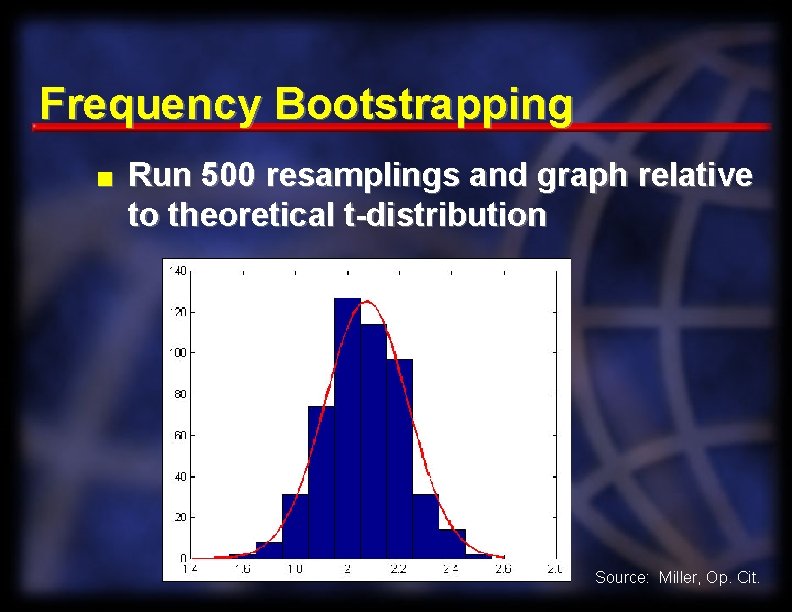
Frequency Bootstrapping n Run 500 resamplings and graph relative to theoretical t-distribution Source: Miller, Op. Cit.
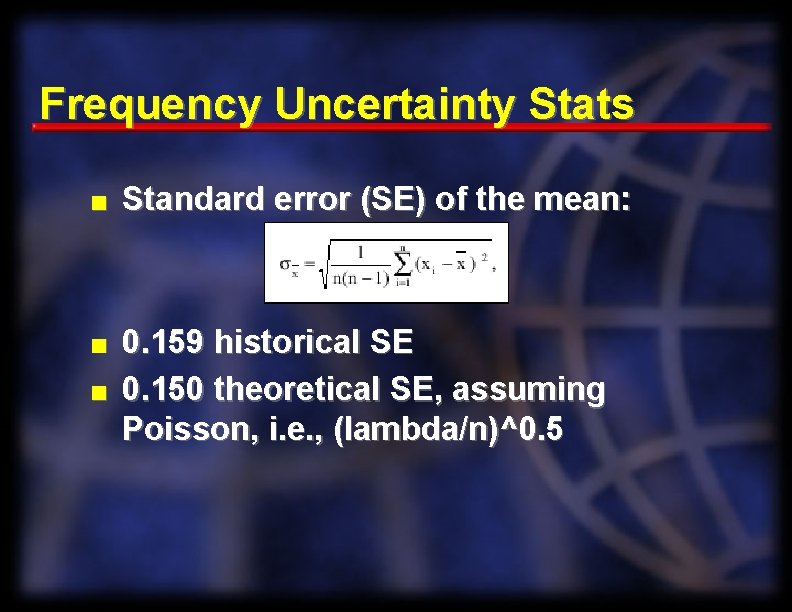
Frequency Uncertainty Stats n n n Standard error (SE) of the mean: 0. 159 historical SE 0. 150 theoretical SE, assuming Poisson, i. e. , (lambda/n)^0. 5
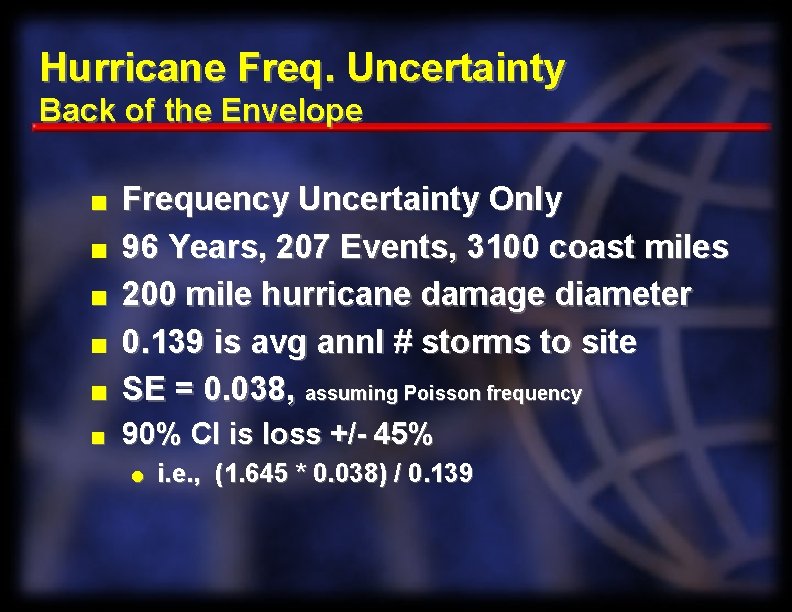
Hurricane Freq. Uncertainty Back of the Envelope n Frequency Uncertainty Only 96 Years, 207 Events, 3100 coast miles 200 mile hurricane damage diameter 0. 139 is avg annl # storms to site SE = 0. 038, assuming Poisson frequency n 90% CI is loss +/- 45% n n l i. e. , (1. 645 * 0. 038) / 0. 139
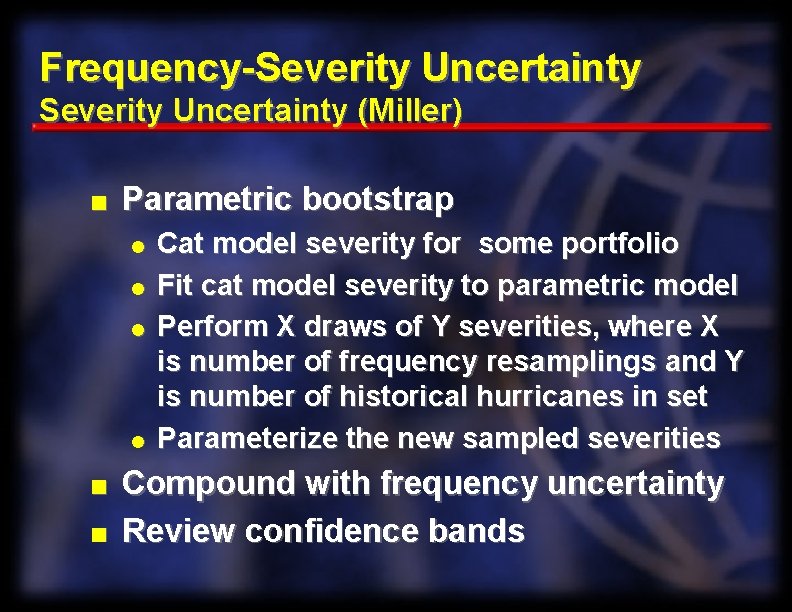
Frequency-Severity Uncertainty (Miller) n Parametric bootstrap l l n n Cat model severity for some portfolio Fit cat model severity to parametric model Perform X draws of Y severities, where X is number of frequency resamplings and Y is number of historical hurricanes in set Parameterize the new sampled severities Compound with frequency uncertainty Review confidence bands
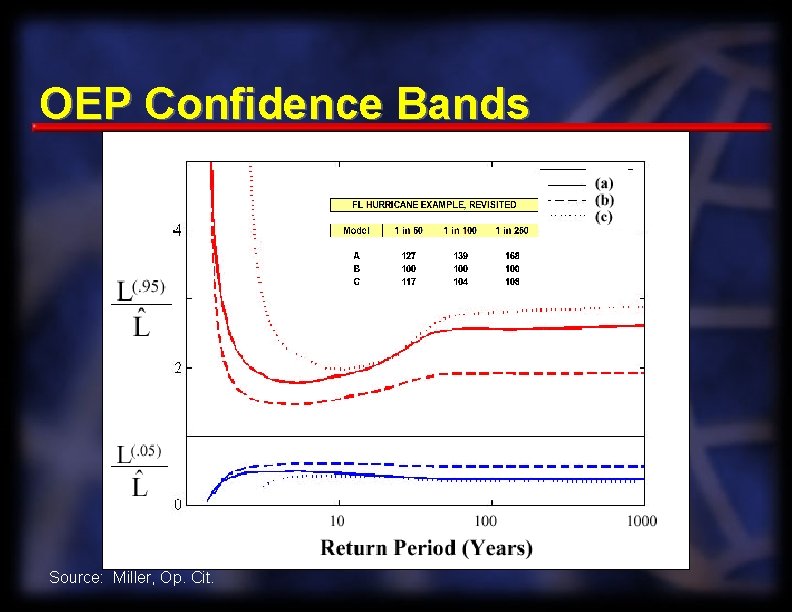
OEP Confidence Bands Source: Miller, Op. Cit.
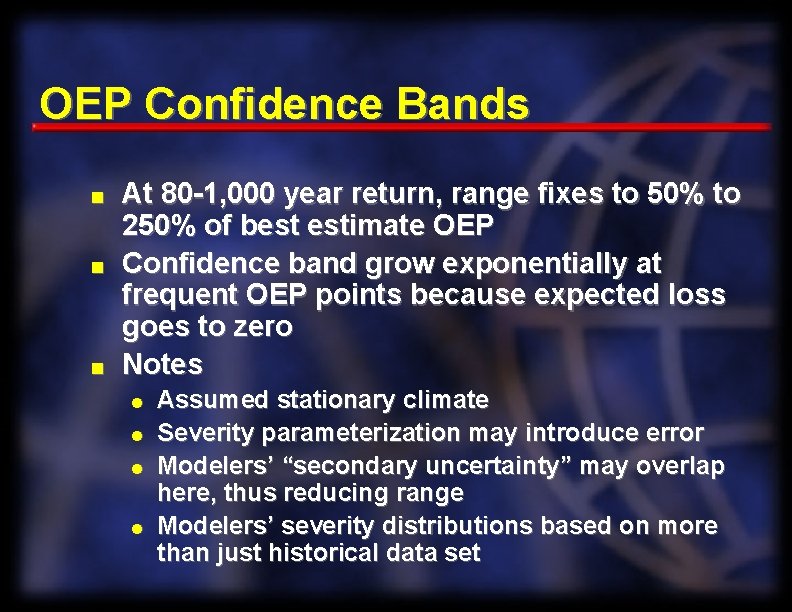
OEP Confidence Bands n n n At 80 -1, 000 year return, range fixes to 50% to 250% of best estimate OEP Confidence band grow exponentially at frequent OEP points because expected loss goes to zero Notes l l Assumed stationary climate Severity parameterization may introduce error Modelers’ “secondary uncertainty” may overlap here, thus reducing range Modelers’ severity distributions based on more than just historical data set
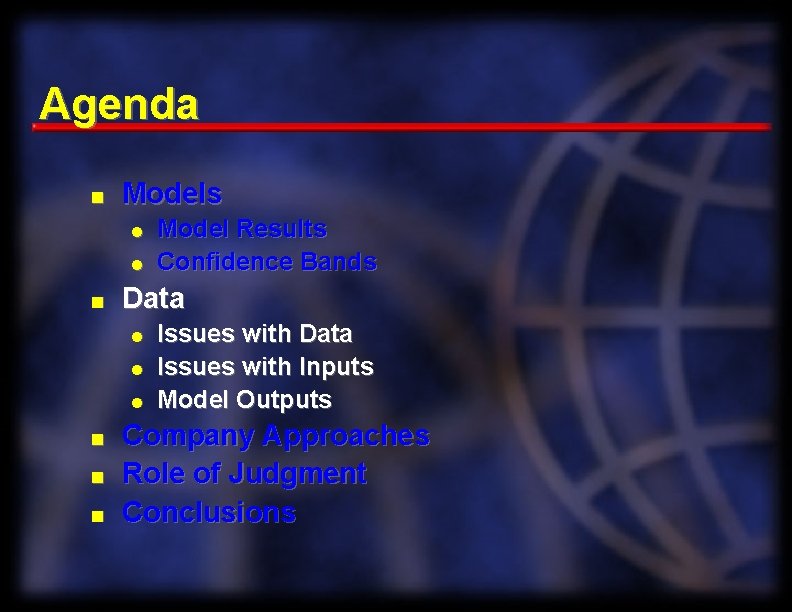
Agenda n Models l l n Data l l l n n n Model Results Confidence Bands Issues with Data Issues with Inputs Model Outputs Company Approaches Role of Judgment Conclusions
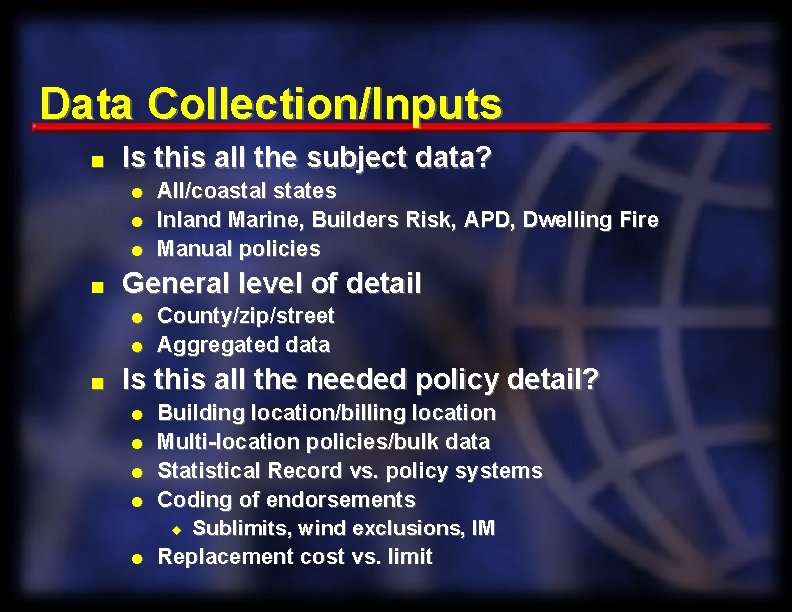
Data Collection/Inputs n Is this all the subject data? l l l n General level of detail l l n All/coastal states Inland Marine, Builders Risk, APD, Dwelling Fire Manual policies County/zip/street Aggregated data Is this all the needed policy detail? l l l Building location/billing location Multi-location policies/bulk data Statistical Record vs. policy systems Coding of endorsements u Sublimits, wind exclusions, IM Replacement cost vs. limit
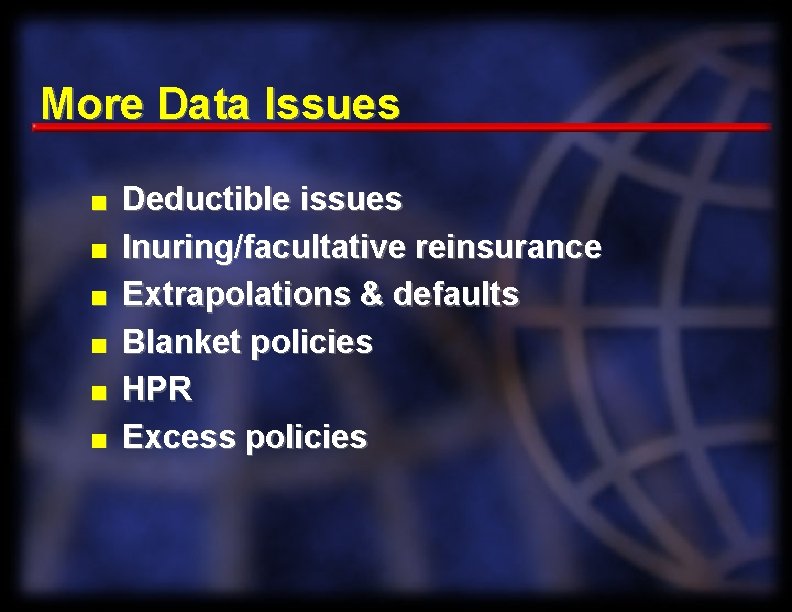
More Data Issues n n n Deductible issues Inuring/facultative reinsurance Extrapolations & defaults Blanket policies HPR Excess policies
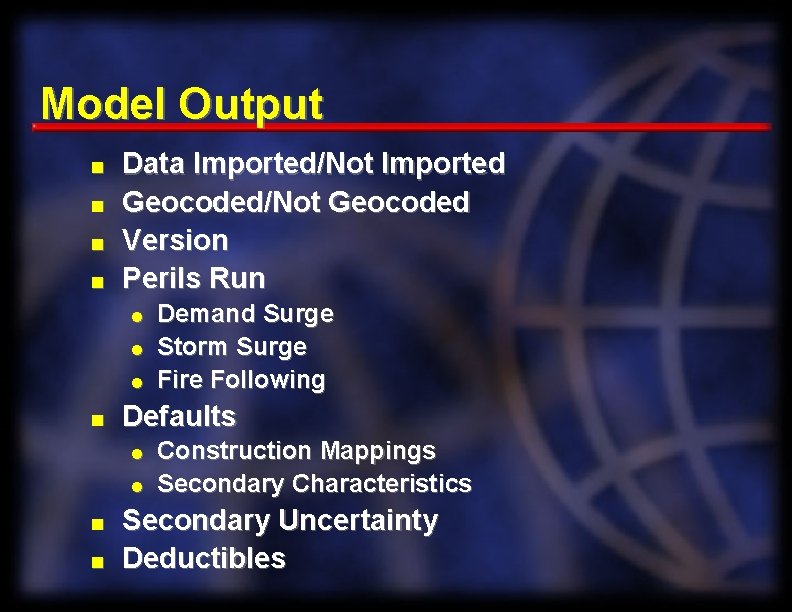
Model Output n n Data Imported/Not Imported Geocoded/Not Geocoded Version Perils Run l l l n Defaults l l n n Demand Surge Storm Surge Fire Following Construction Mappings Secondary Characteristics Secondary Uncertainty Deductibles
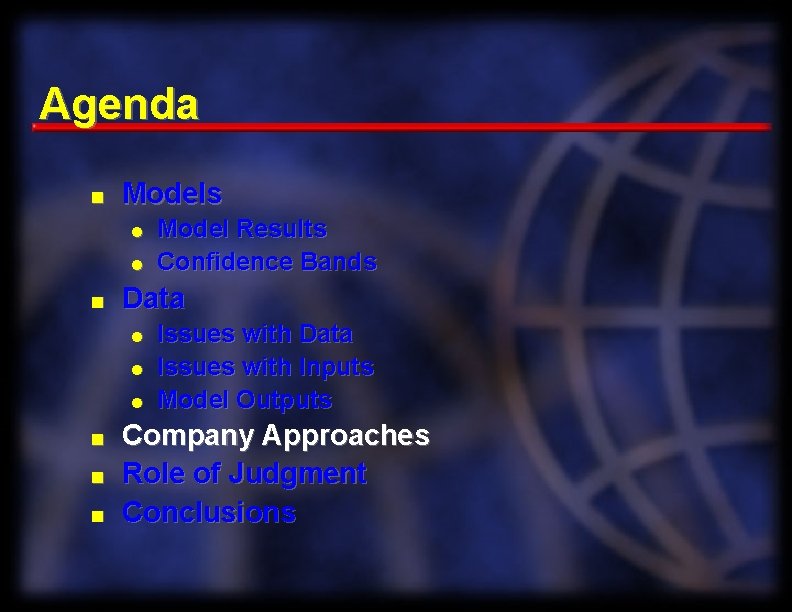
Agenda n Models l l n Data l l l n n n Model Results Confidence Bands Issues with Data Issues with Inputs Model Outputs Company Approaches Role of Judgment Conclusions
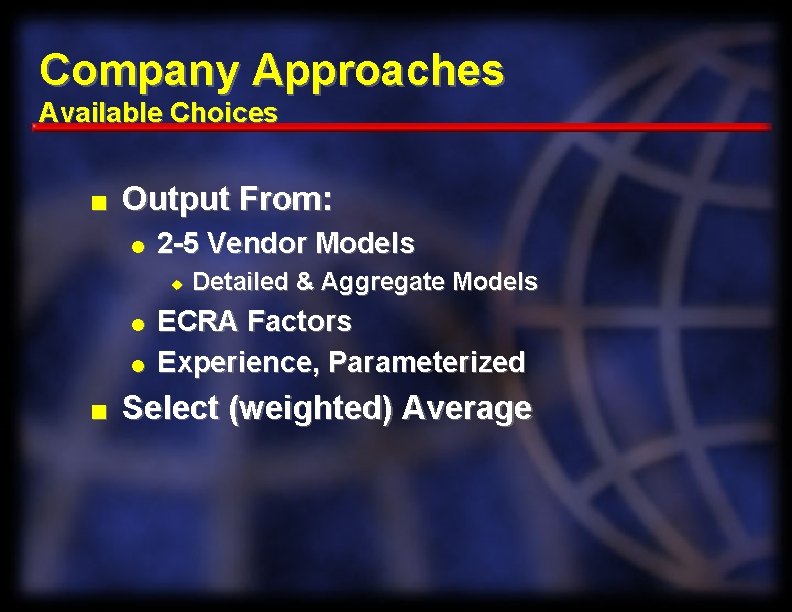
Company Approaches Available Choices n Output From: l 2 -5 Vendor Models u l l n Detailed & Aggregate Models ECRA Factors Experience, Parameterized Select (weighted) Average
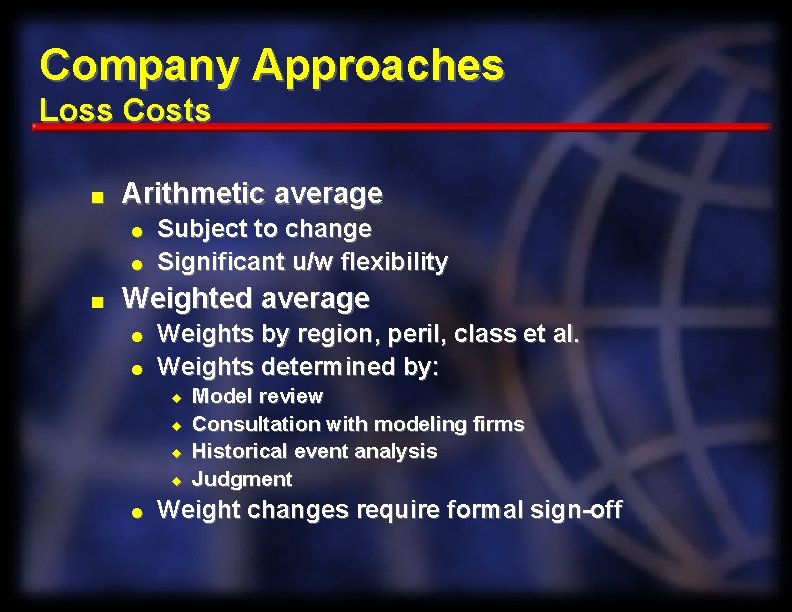
Company Approaches Loss Costs n Arithmetic average l l n Subject to change Significant u/w flexibility Weighted average l l Weights by region, peril, class et al. Weights determined by: u u l Model review Consultation with modeling firms Historical event analysis Judgment Weight changes require formal sign-off
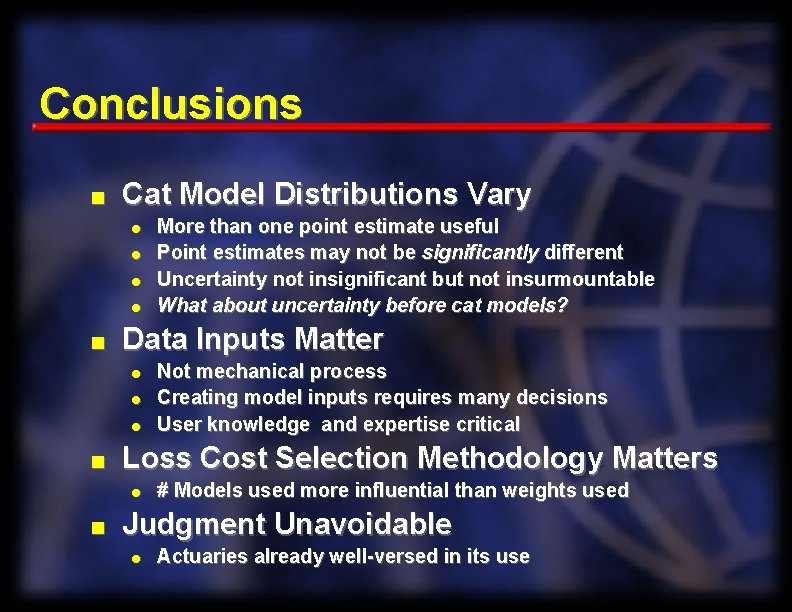
Conclusions n Cat Model Distributions Vary l l n Data Inputs Matter l l l n Not mechanical process Creating model inputs requires many decisions User knowledge and expertise critical Loss Cost Selection Methodology Matters l n More than one point estimate useful Point estimates may not be significantly different Uncertainty not insignificant but not insurmountable What about uncertainty before cat models? # Models used more influential than weights used Judgment Unavoidable l Actuaries already well-versed in its use
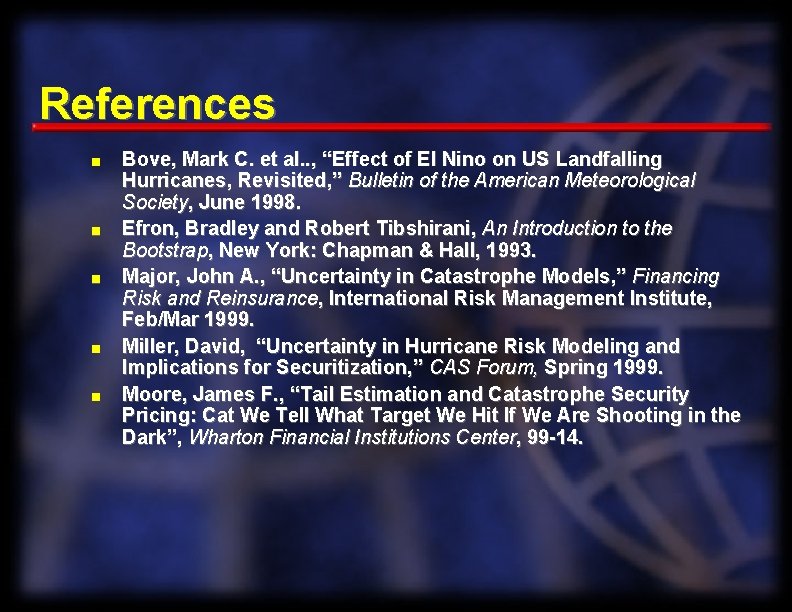
References n n n Bove, Mark C. et al. . , “Effect of El Nino on US Landfalling Hurricanes, Revisited, ” Bulletin of the American Meteorological Society, June 1998. Efron, Bradley and Robert Tibshirani, An Introduction to the Bootstrap, New York: Chapman & Hall, 1993. Major, John A. , “Uncertainty in Catastrophe Models, ” Financing Risk and Reinsurance, International Risk Management Institute, Feb/Mar 1999. Miller, David, “Uncertainty in Hurricane Risk Modeling and Implications for Securitization, ” CAS Forum, Spring 1999. Moore, James F. , “Tail Estimation and Catastrophe Security Pricing: Cat We Tell What Target We Hit If We Are Shooting in the Dark”, Wharton Financial Institutions Center, 99 -14.
Job order costing
Marquis company estimates that annual manufacturing
Who global estimates on prevalence of hearing loss 2020
Who global estimates on prevalence of hearing loss 2020
Annual loss expectancy
Catastrophe modeling
Scrap account
Cwemf annual meeting
Nrg oncology meeting 2017
American psychiatric association annual meeting 2020
How to run an annual general meeting
American epilepsy society annual meeting 2017
Grand lodge kentucky
Aashto annual meeting 2015
Nrg oncology meeting 2019
Informs annual meeting
Annual theory meeting
Scts annual meeting
Aupha annual meeting
Example of what goes around comes around
Martin luther king of hinduism
Building maintenance cost estimates
1/4percentage
Fermi estimate
Properties of least square regression line
Fermi problem