UAV Research at the University of Cincinnati Dr
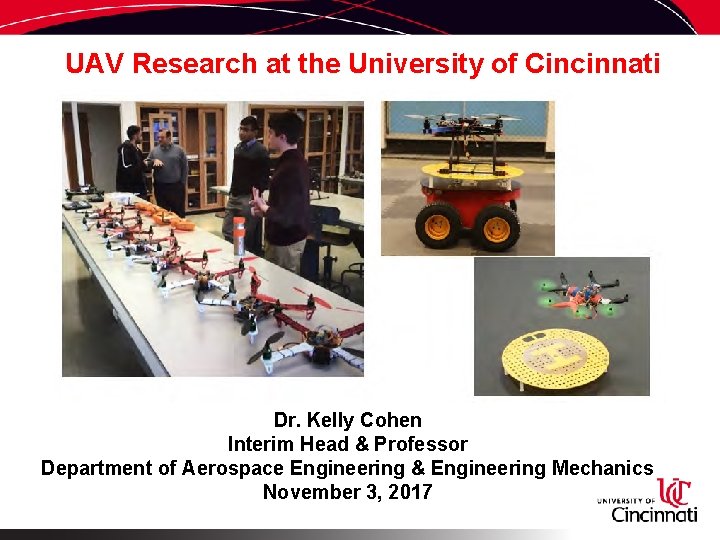
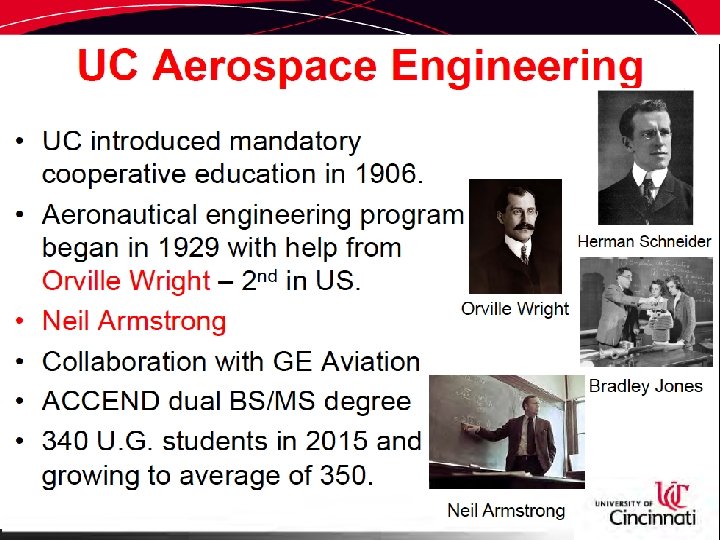
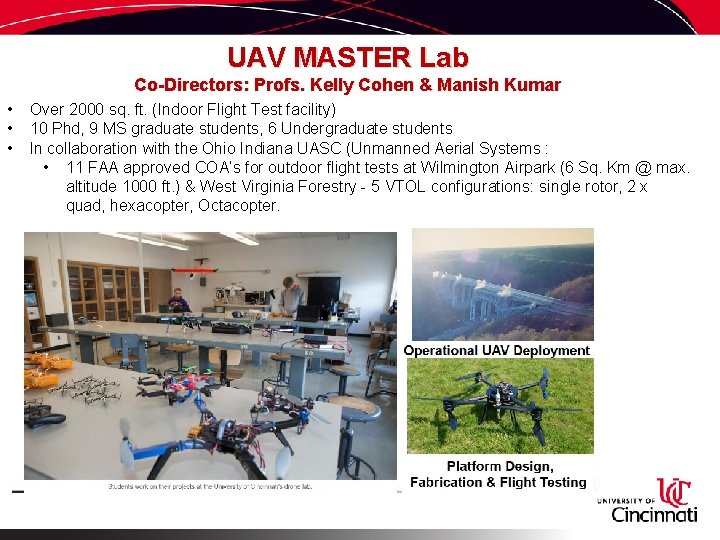
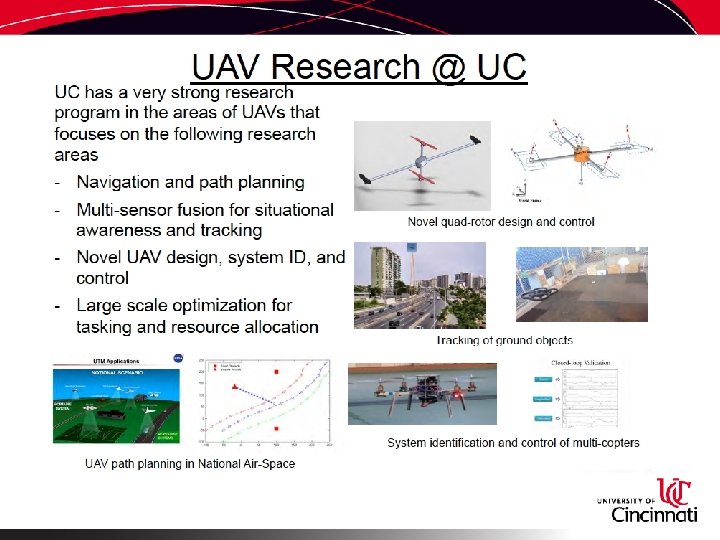
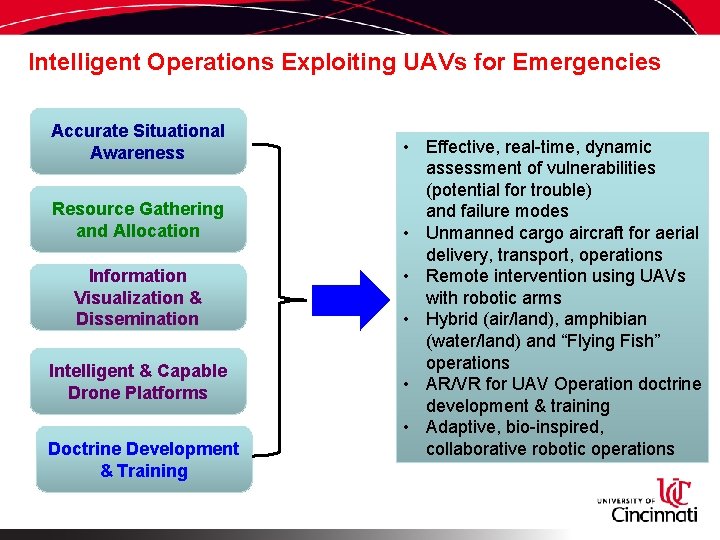
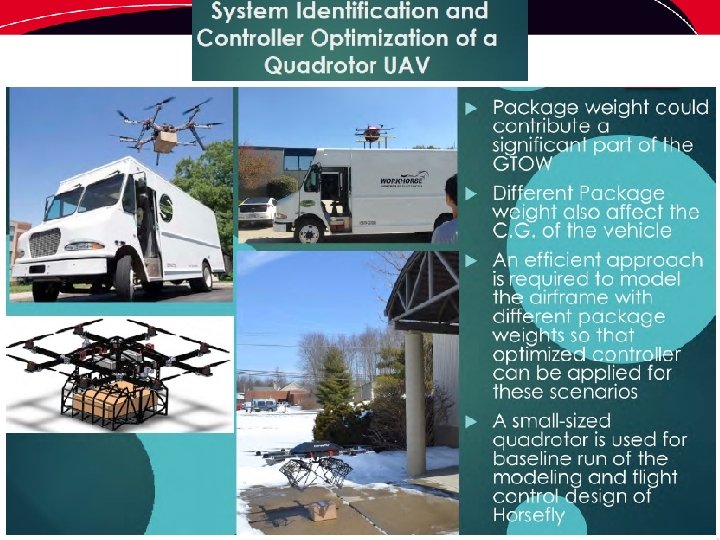
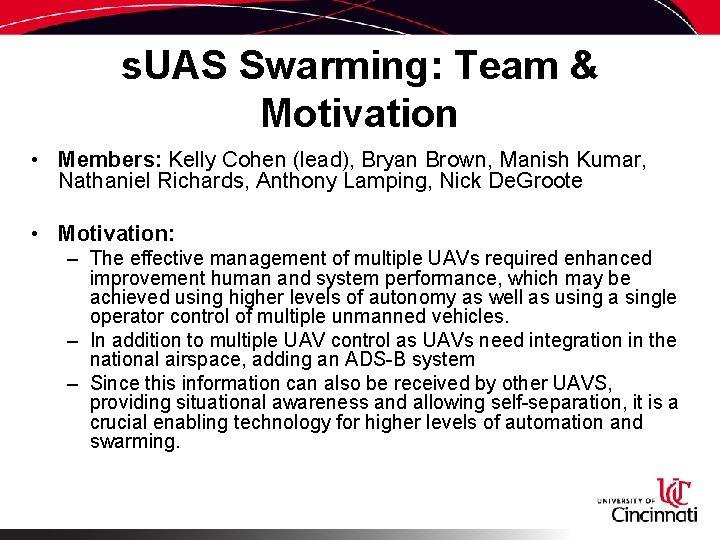
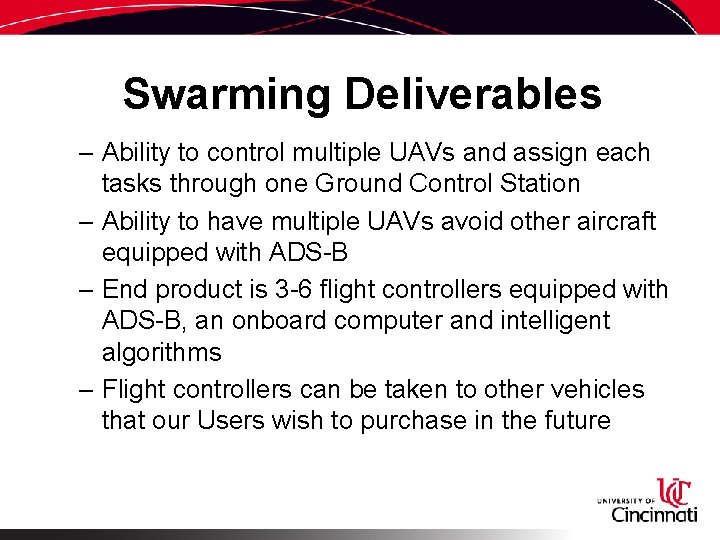
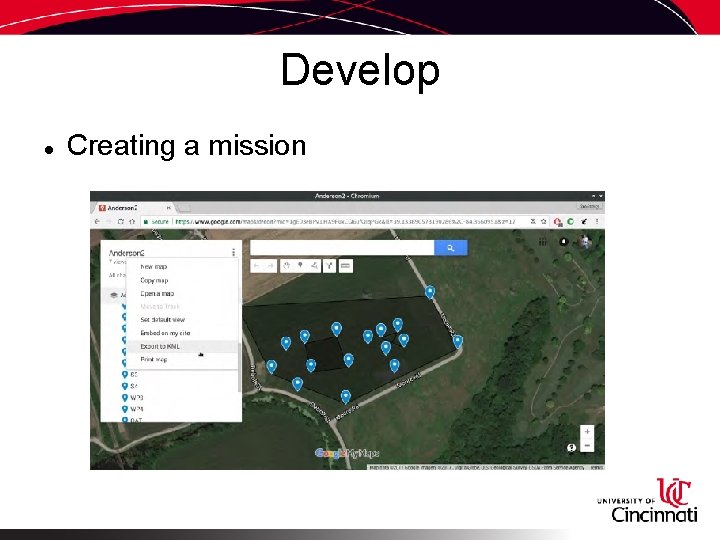
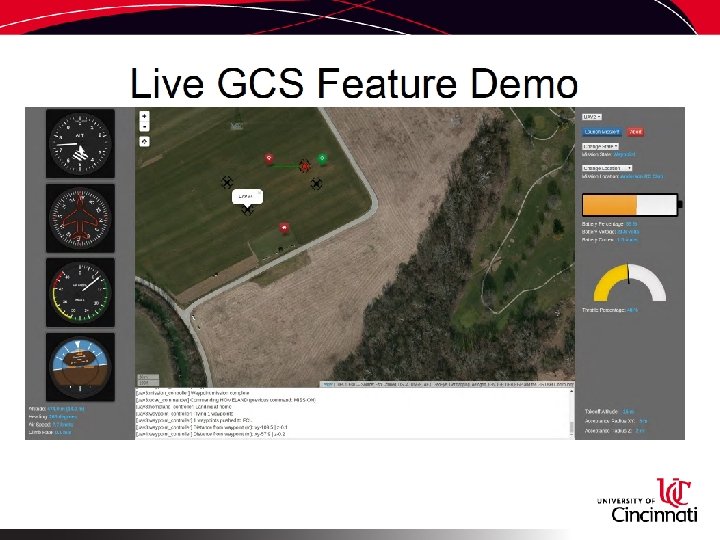
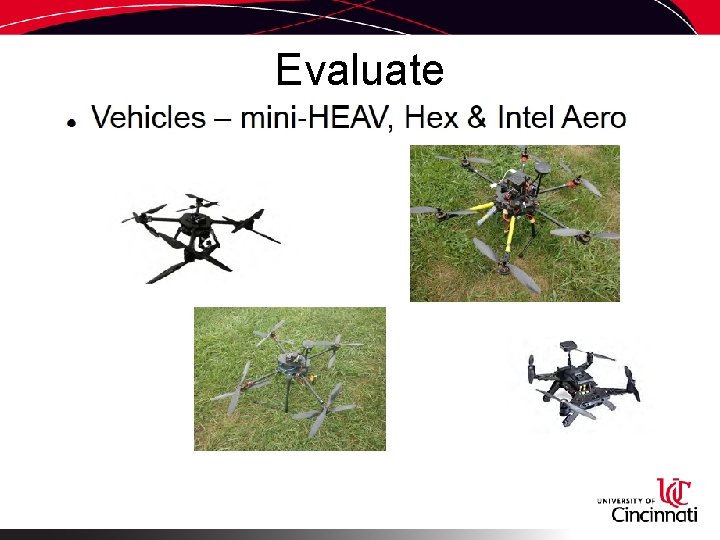
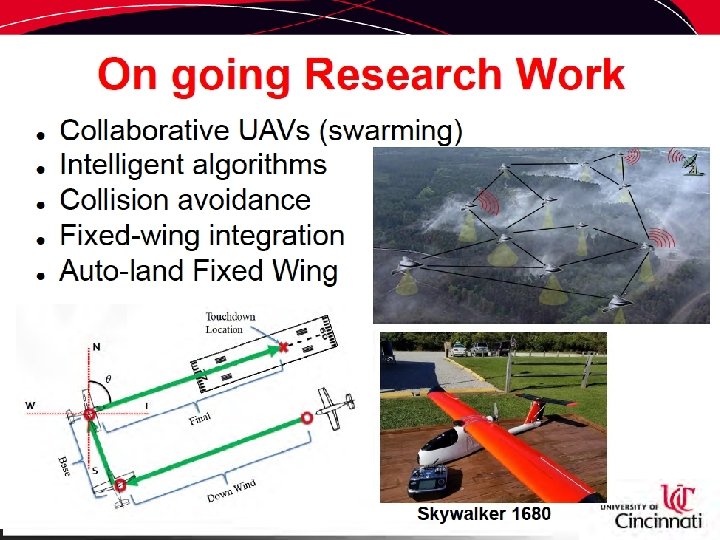
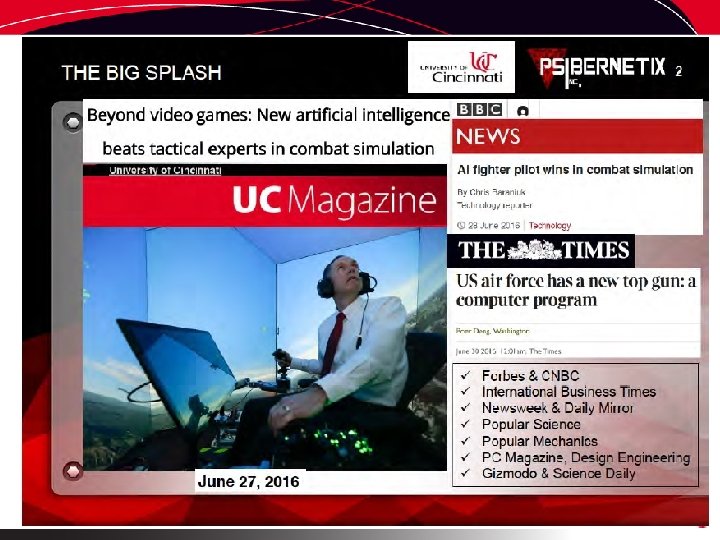
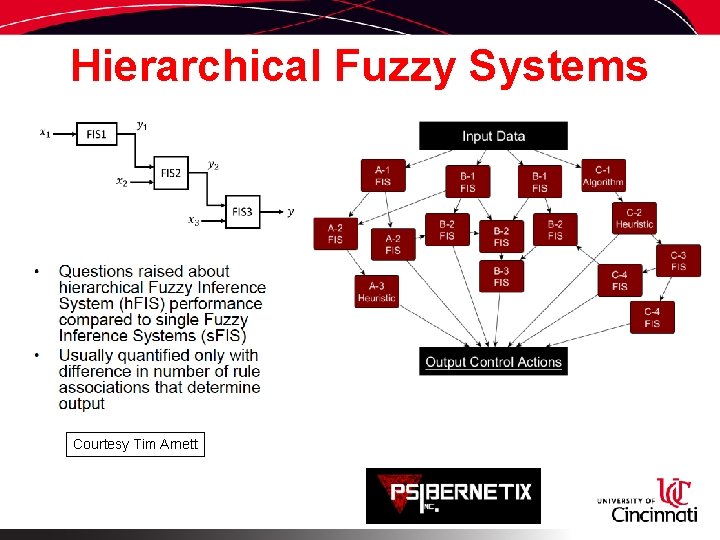
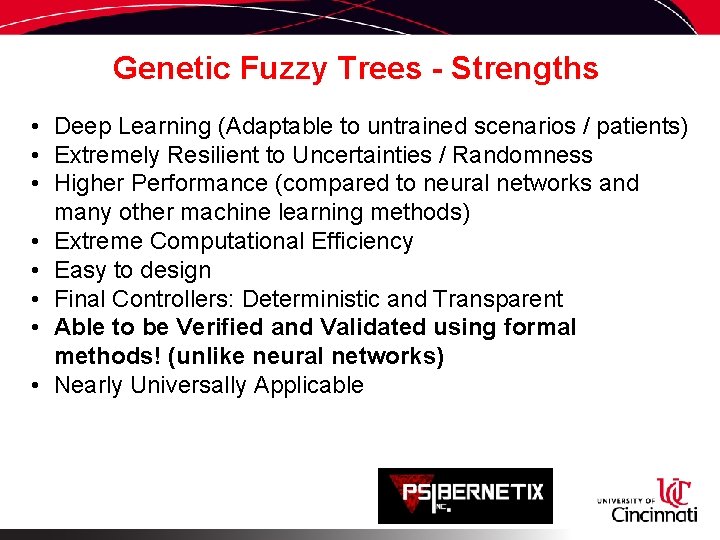
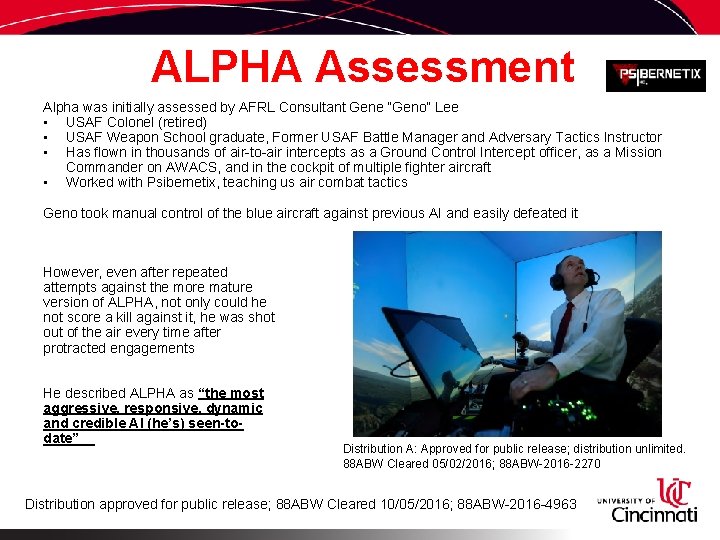
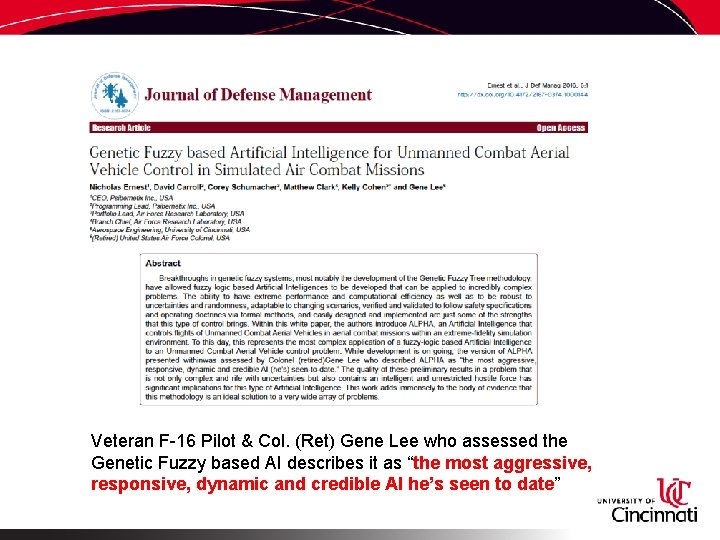
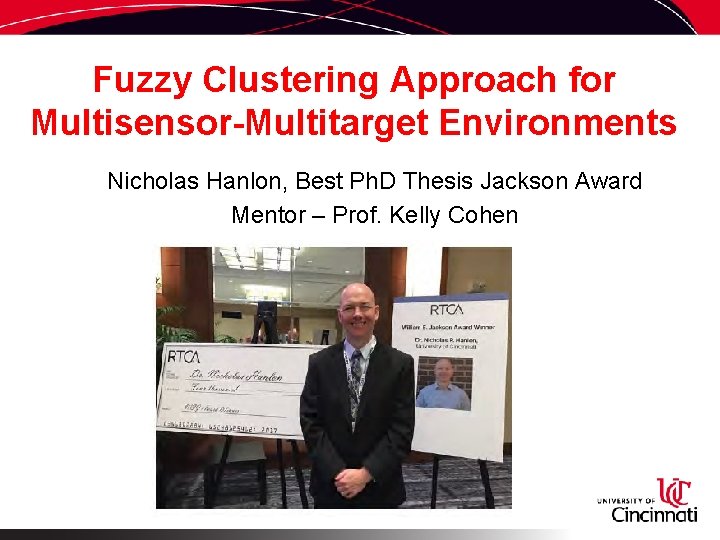
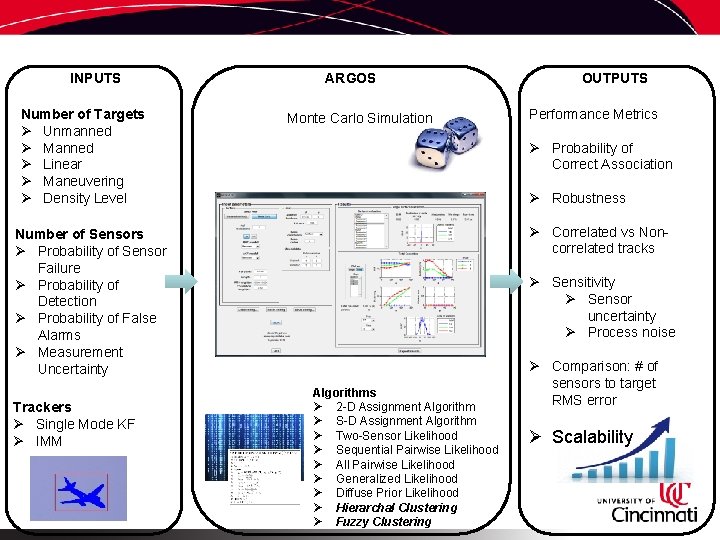
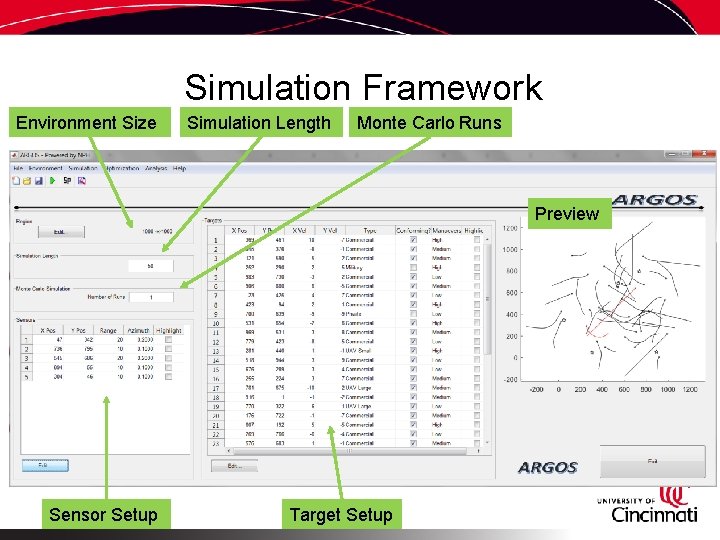
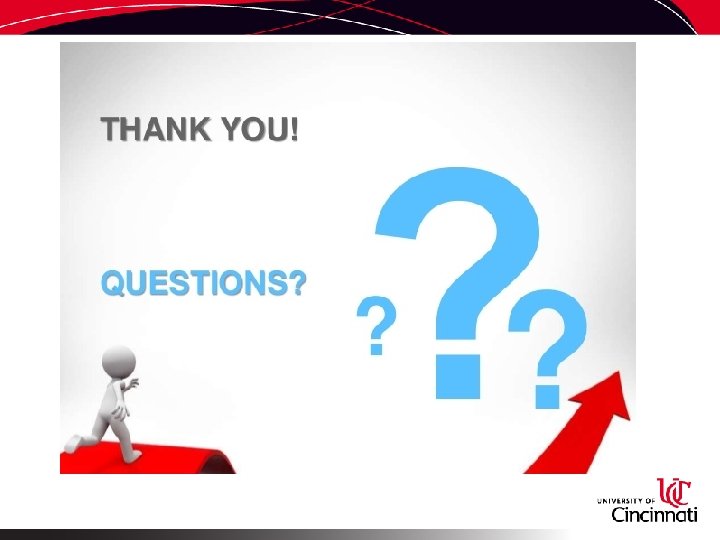
- Slides: 21
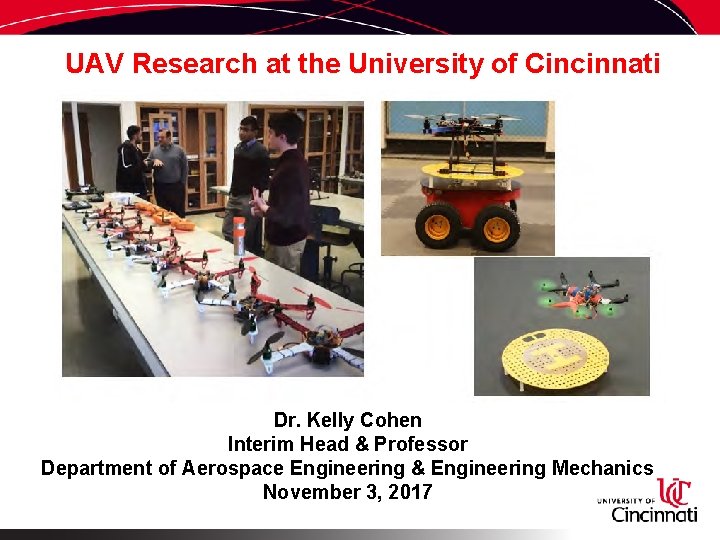
UAV Research at the University of Cincinnati Dr. Kelly Cohen Interim Head & Professor Department of Aerospace Engineering & Engineering Mechanics November 3, 2017
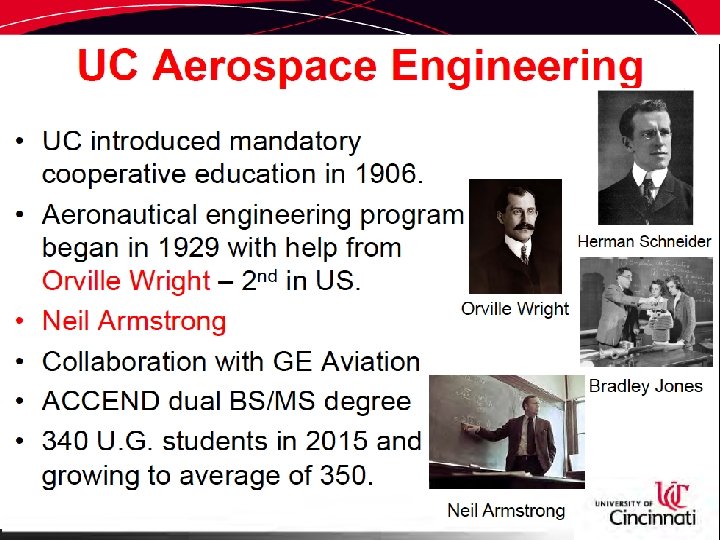
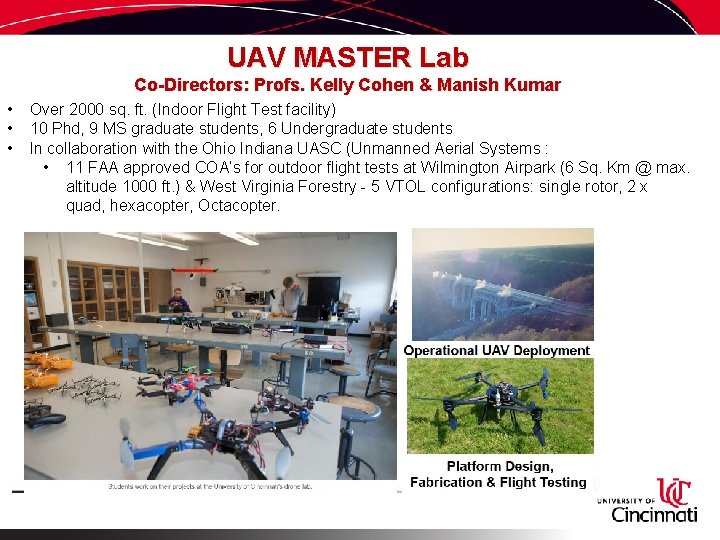
UAV MASTER Lab Co-Directors: Profs. Kelly Cohen & Manish Kumar • • • Over 2000 sq. ft. (Indoor Flight Test facility) 10 Phd, 9 MS graduate students, 6 Undergraduate students In collaboration with the Ohio Indiana UASC (Unmanned Aerial Systems : • 11 FAA approved COA’s for outdoor flight tests at Wilmington Airpark (6 Sq. Km @ max. altitude 1000 ft. ) & West Virginia Forestry - 5 VTOL configurations: single rotor, 2 x quad, hexacopter, Octacopter.
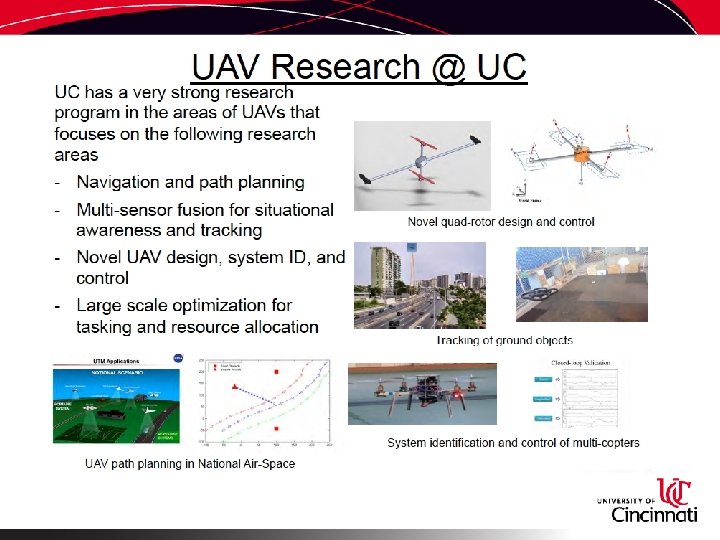
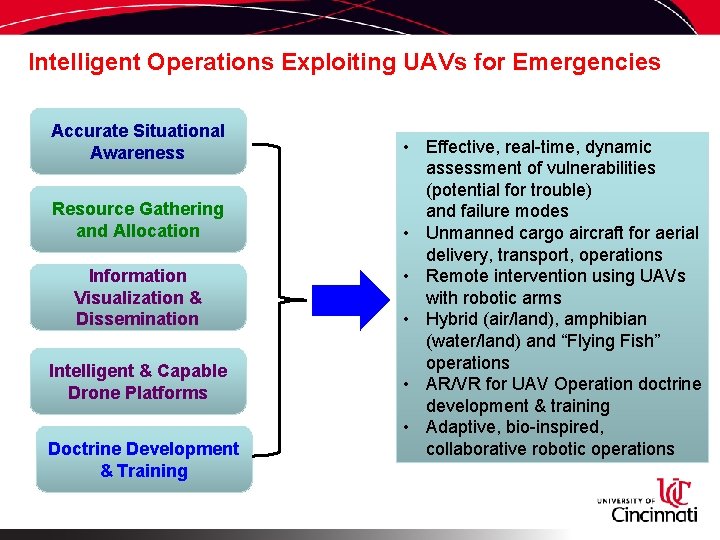
Intelligent Operations Exploiting UAVs for Emergencies Accurate Situational Awareness Resource Gathering and Allocation Information Visualization & Dissemination Intelligent & Capable Drone Platforms Doctrine Development & Training • Effective, real-time, dynamic assessment of vulnerabilities (potential for trouble) and failure modes • Unmanned cargo aircraft for aerial delivery, transport, operations • Remote intervention using UAVs with robotic arms • Hybrid (air/land), amphibian (water/land) and “Flying Fish” operations • AR/VR for UAV Operation doctrine development & training • Adaptive, bio-inspired, collaborative robotic operations
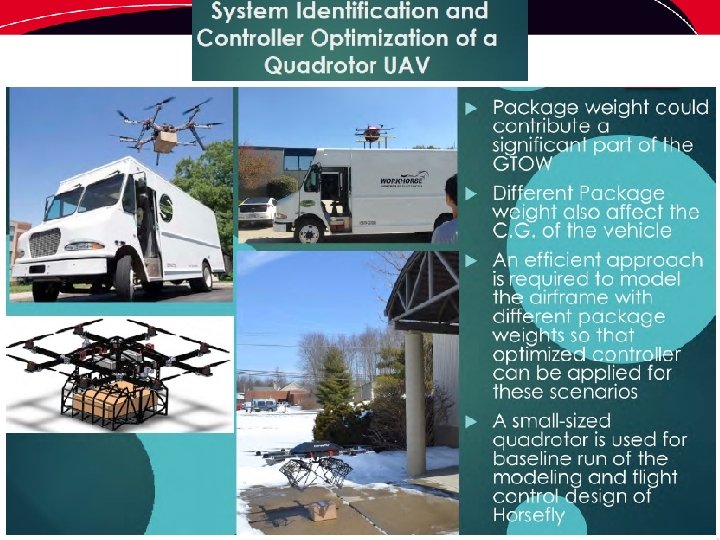
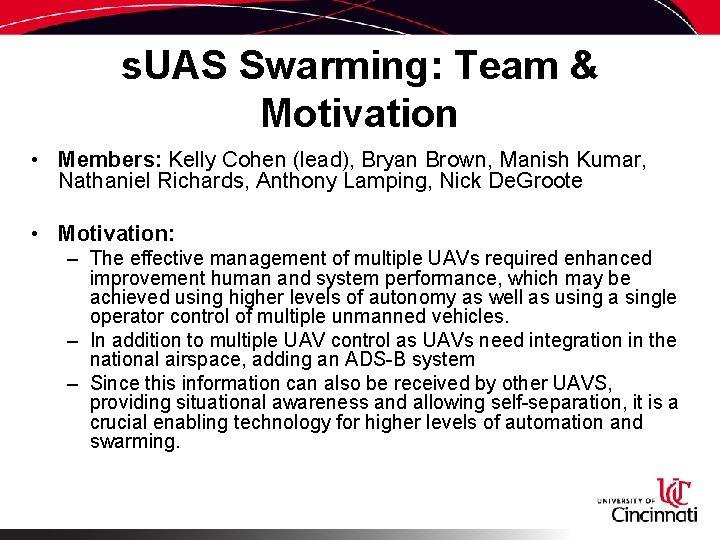
s. UAS Swarming: Team & Motivation • Members: Kelly Cohen (lead), Bryan Brown, Manish Kumar, Nathaniel Richards, Anthony Lamping, Nick De. Groote • Motivation: – The effective management of multiple UAVs required enhanced improvement human and system performance, which may be achieved using higher levels of autonomy as well as using a single operator control of multiple unmanned vehicles. – In addition to multiple UAV control as UAVs need integration in the national airspace, adding an ADS-B system – Since this information can also be received by other UAVS, providing situational awareness and allowing self-separation, it is a crucial enabling technology for higher levels of automation and swarming.
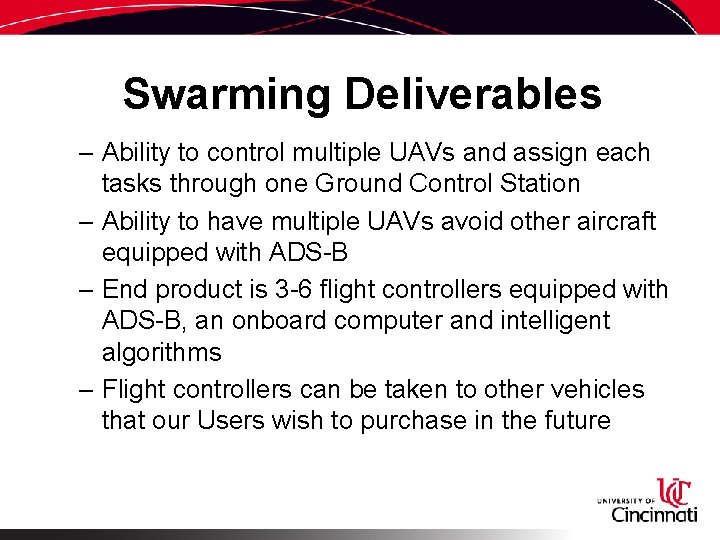
Swarming Deliverables – Ability to control multiple UAVs and assign each tasks through one Ground Control Station – Ability to have multiple UAVs avoid other aircraft equipped with ADS-B – End product is 3 -6 flight controllers equipped with ADS-B, an onboard computer and intelligent algorithms – Flight controllers can be taken to other vehicles that our Users wish to purchase in the future
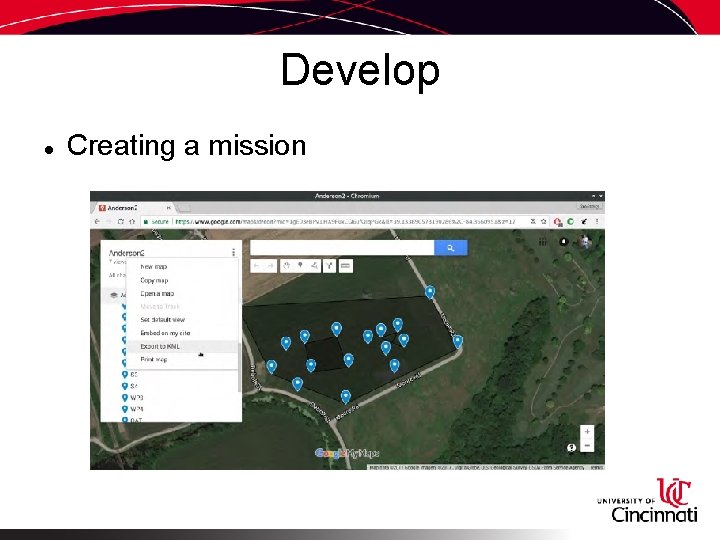
Develop Creating a mission
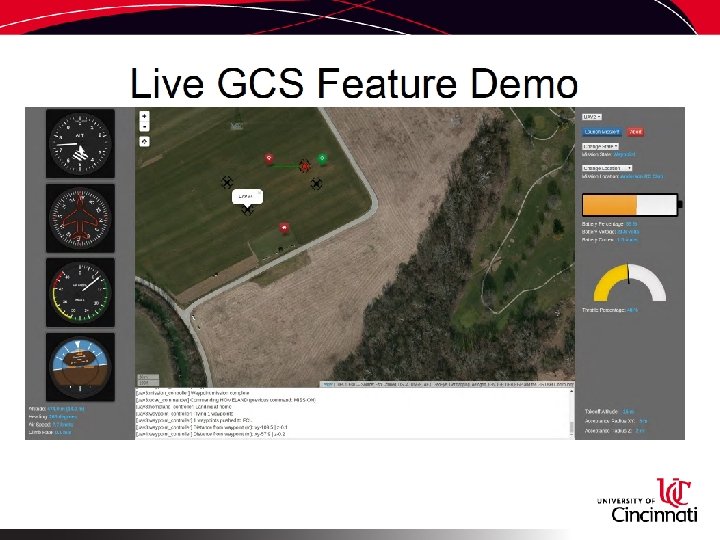
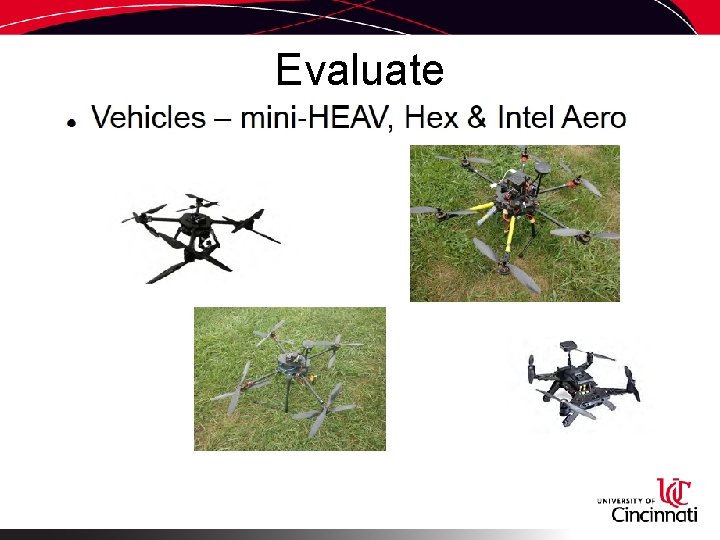
Evaluate
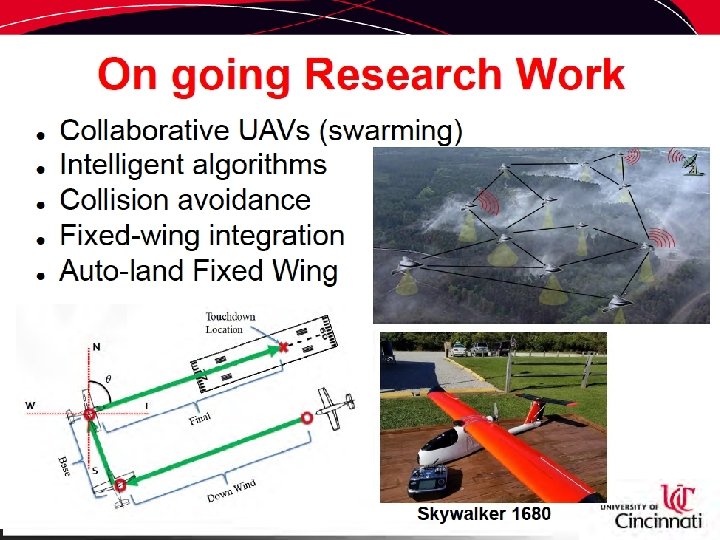
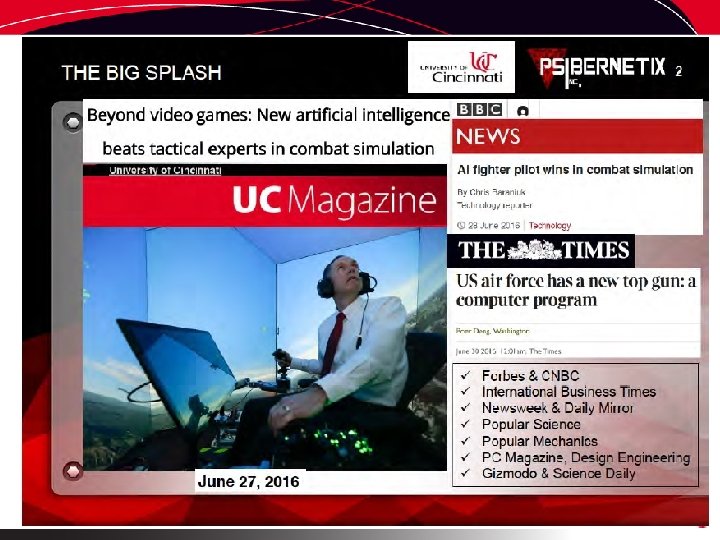
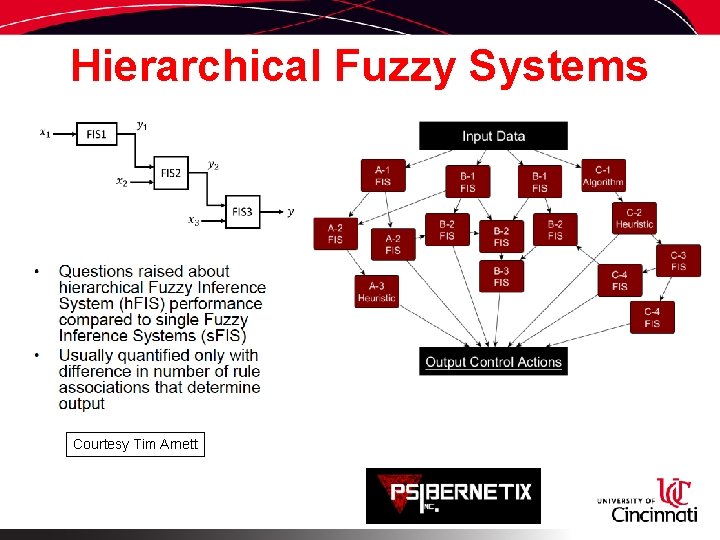
Hierarchical Fuzzy Systems Courtesy Tim Arnett
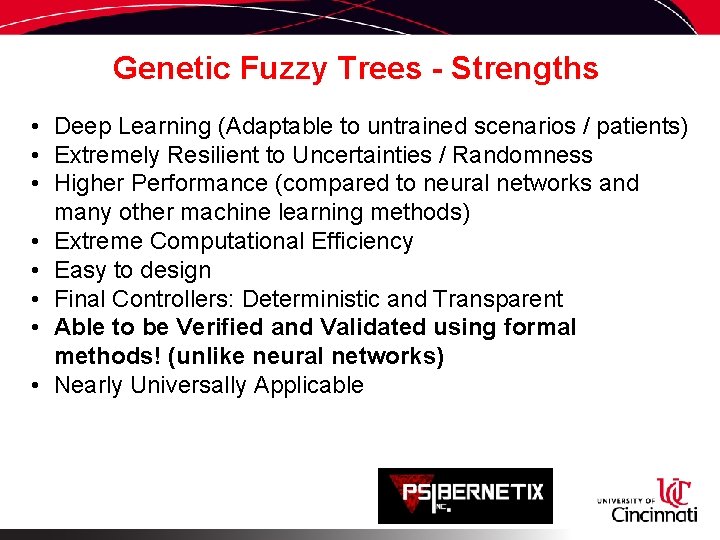
Genetic Fuzzy Trees - Strengths • Deep Learning (Adaptable to untrained scenarios / patients) • Extremely Resilient to Uncertainties / Randomness • Higher Performance (compared to neural networks and many other machine learning methods) • Extreme Computational Efficiency • Easy to design • Final Controllers: Deterministic and Transparent • Able to be Verified and Validated using formal methods! (unlike neural networks) • Nearly Universally Applicable
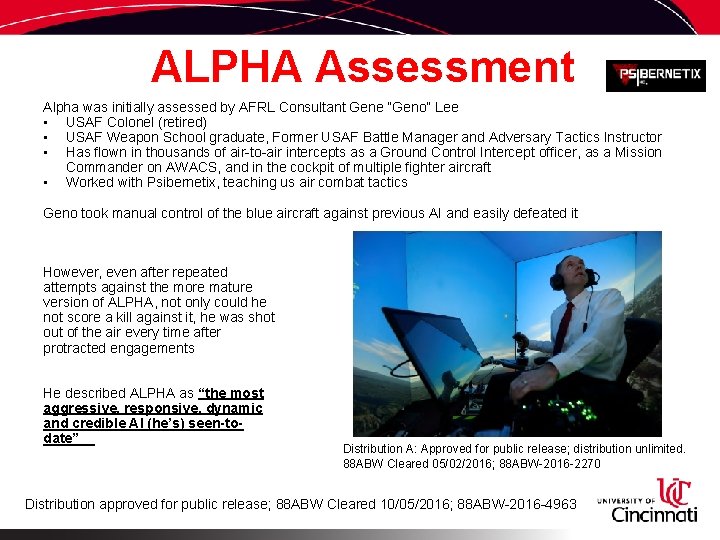
ALPHA Assessment Alpha was initially assessed by AFRL Consultant Gene “Geno” Lee • USAF Colonel (retired) • USAF Weapon School graduate, Former USAF Battle Manager and Adversary Tactics Instructor • Has flown in thousands of air-to-air intercepts as a Ground Control Intercept officer, as a Mission Commander on AWACS, and in the cockpit of multiple fighter aircraft • Worked with Psibernetix, teaching us air combat tactics Geno took manual control of the blue aircraft against previous AI and easily defeated it However, even after repeated attempts against the more mature version of ALPHA, not only could he not score a kill against it, he was shot out of the air every time after protracted engagements He described ALPHA as “the most aggressive, responsive, dynamic and credible AI (he’s) seen-todate” Distribution A: Approved for public release; distribution unlimited. 88 ABW Cleared 05/02/2016; 88 ABW-2016 -2270 Distribution approved for public release; 88 ABW Cleared 10/05/2016; 88 ABW-2016 -4963
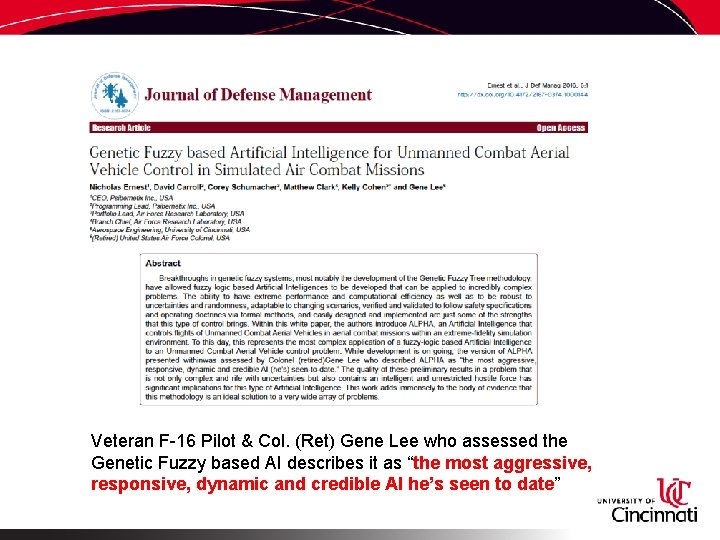
Veteran F-16 Pilot & Col. (Ret) Gene Lee who assessed the Genetic Fuzzy based AI describes it as “the most aggressive, responsive, dynamic and credible AI he’s seen to date”
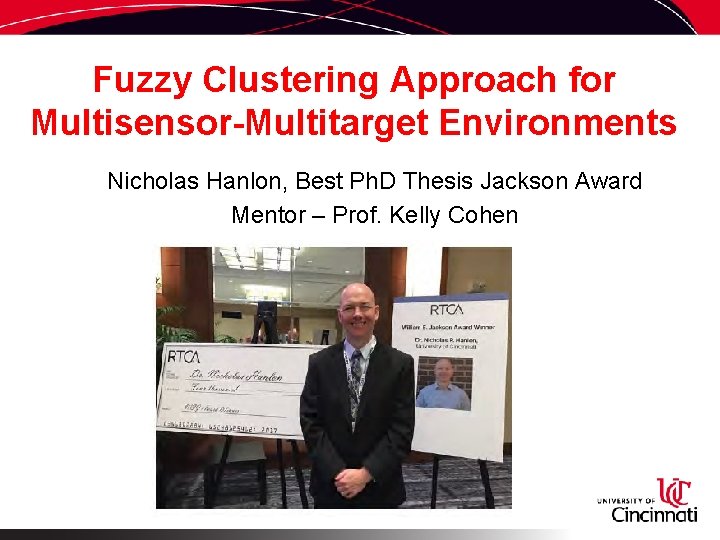
Fuzzy Clustering Approach for Multisensor-Multitarget Environments Nicholas Hanlon, Best Ph. D Thesis Jackson Award Mentor – Prof. Kelly Cohen
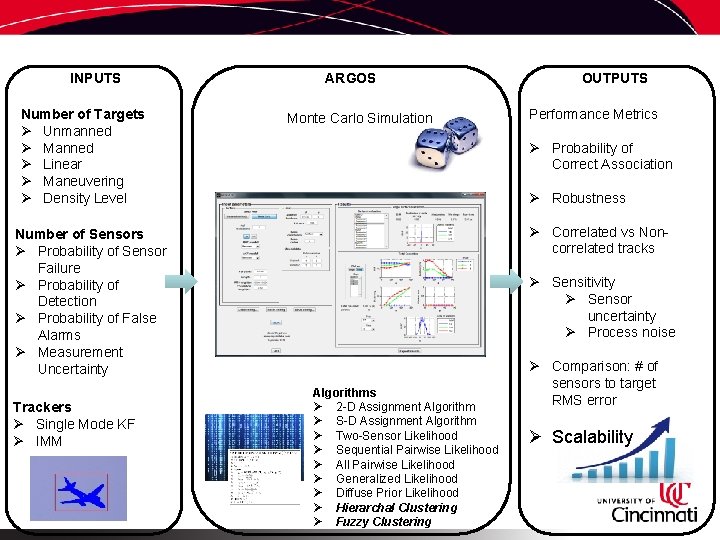
INPUTS Number of Targets Ø Unmanned Ø Manned Ø Linear Ø Maneuvering Ø Density Level ARGOS Monte Carlo Simulation Performance Metrics Ø Probability of Correct Association Ø Robustness Ø Correlated vs Noncorrelated tracks Number of Sensors Ø Probability of Sensor Failure Ø Probability of Detection Ø Probability of False Alarms Ø Measurement Uncertainty Trackers Ø Single Mode KF Ø IMM OUTPUTS Ø Sensitivity Ø Sensor uncertainty Ø Process noise Algorithms Ø 2 -D Assignment Algorithm Ø S-D Assignment Algorithm Ø Two-Sensor Likelihood Ø Sequential Pairwise Likelihood Ø All Pairwise Likelihood Ø Generalized Likelihood Ø Diffuse Prior Likelihood Ø Hierarchal Clustering Ø Fuzzy Clustering Ø Comparison: # of sensors to target RMS error Ø Scalability
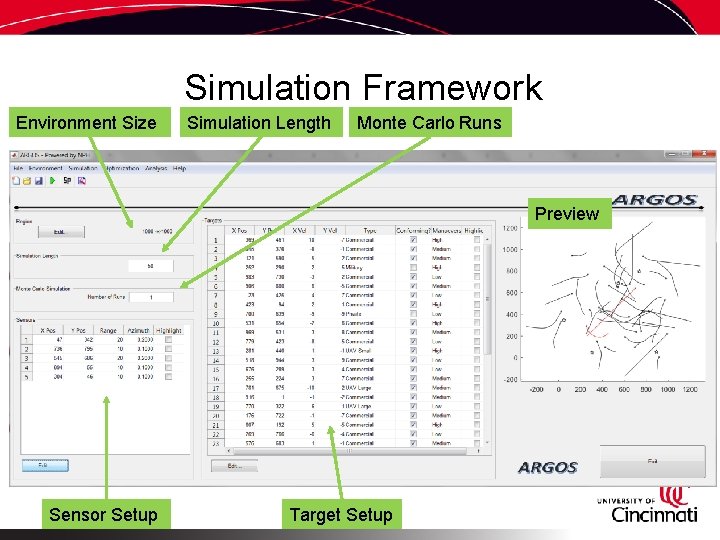
Simulation Framework Environment Size Simulation Length Monte Carlo Runs Preview Sensor Setup Target Setup
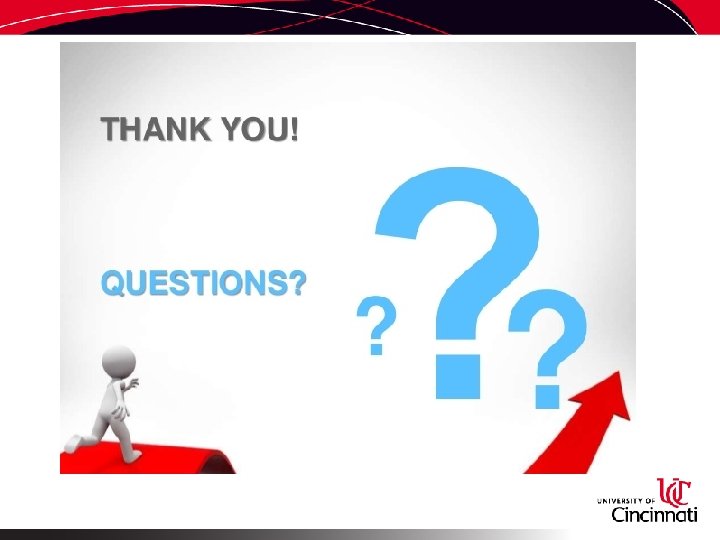
Pengertian uav
Classification of uav
Schulich uav
Uav airframe design
Uav
Satellite uav
University of cincinnati physicians inc
University of cincinnati nurse midwifery
University of cincinnati co op program
Cincinnati chemical engineering
University of cincinnati chemical engineering
University of cincinnati physicians inc
Soma sengupta university of cincinnati
Cincinnati prehospital stroke scale
Cincinnati prehospital stroke scale
Meetup.com cincinnati
Southwestern college cincinnati ohio
Ischaemic stroke types
Avpu skalası
Escala cincinnati
Cstars cincinnati
Cincinnati