Tesla Fastest Processor Adoption in HPC History http
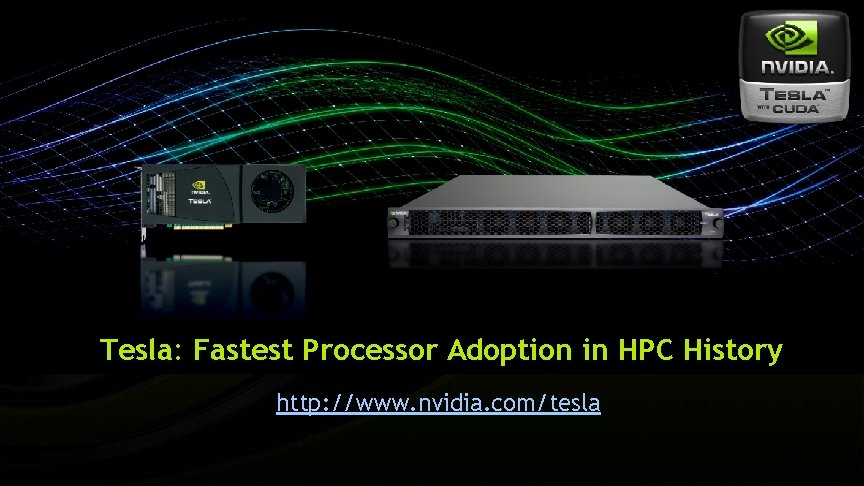
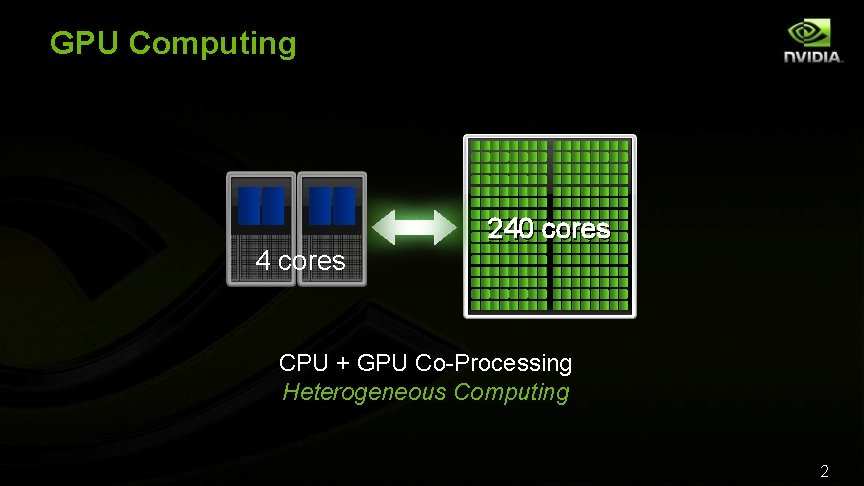
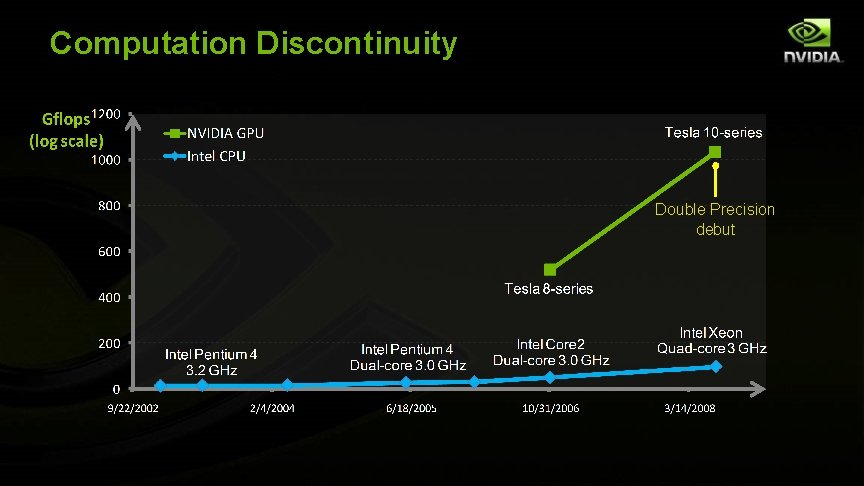
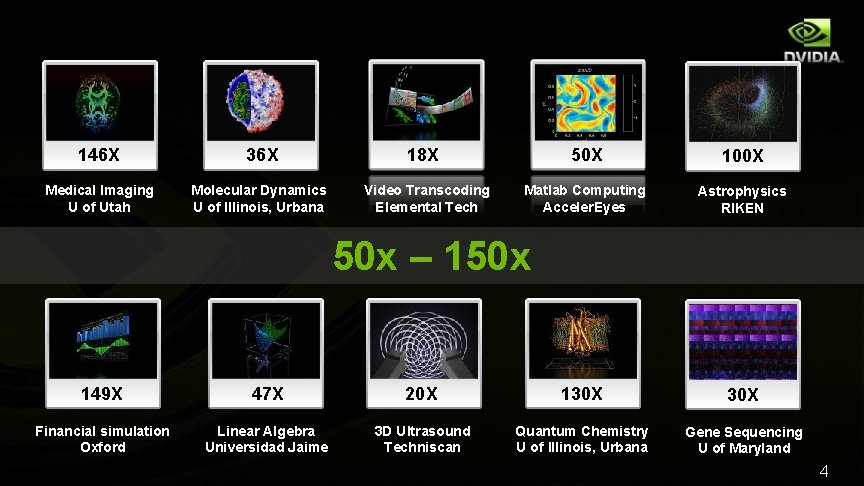
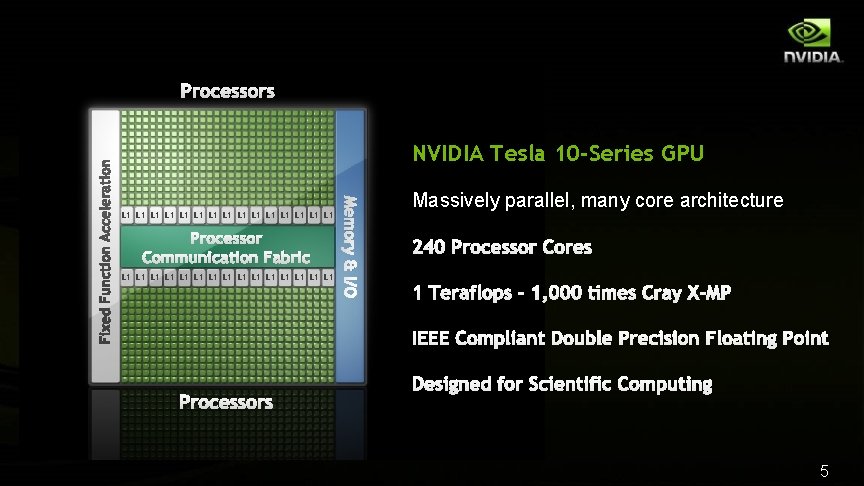
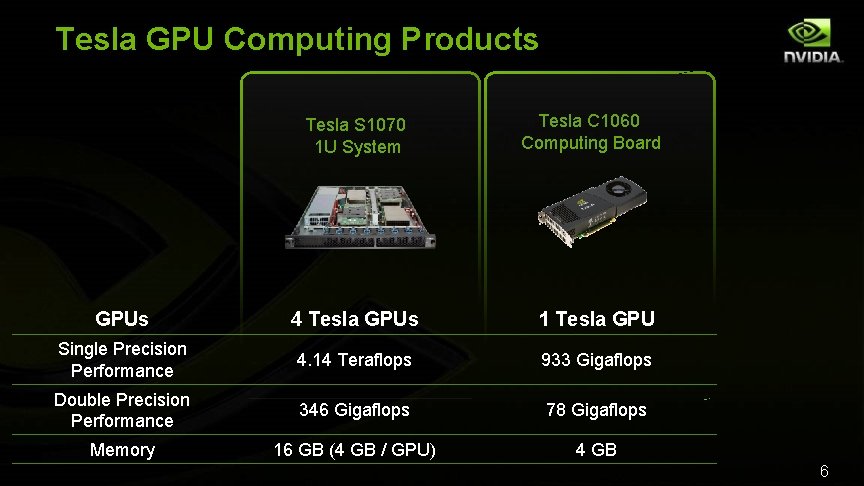
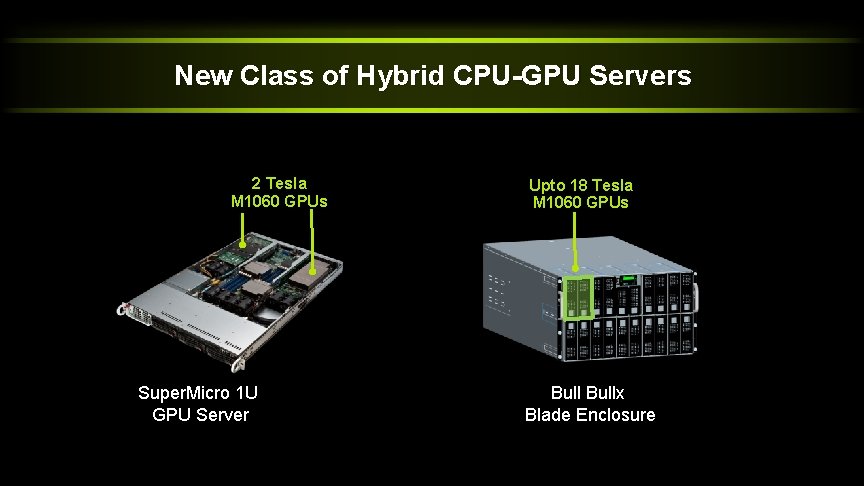
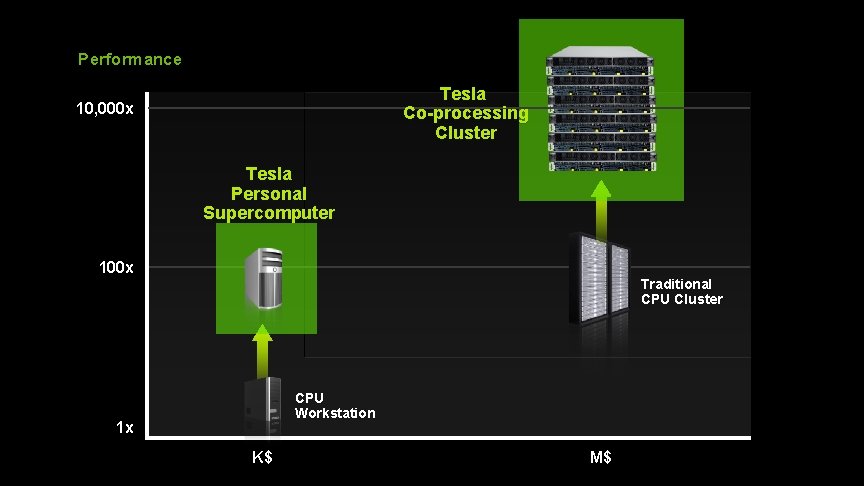
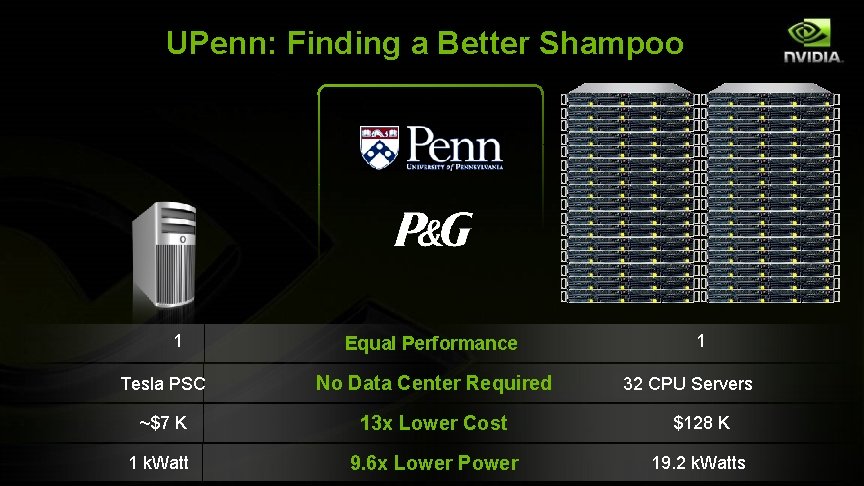
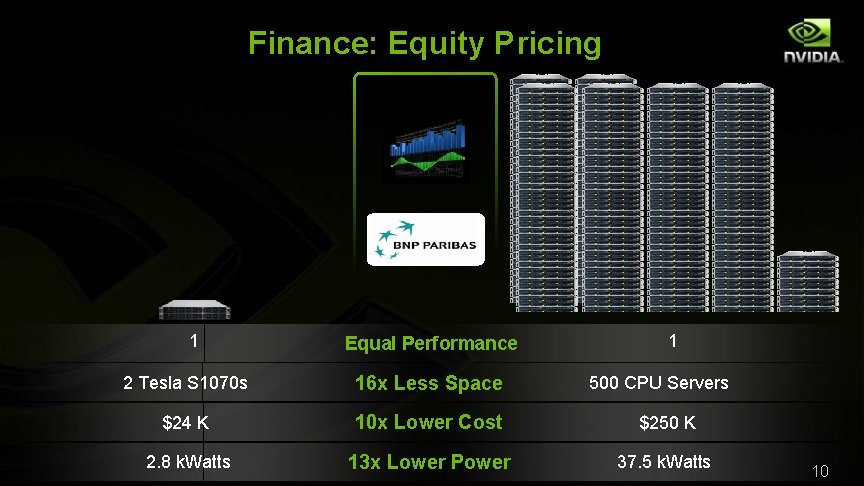
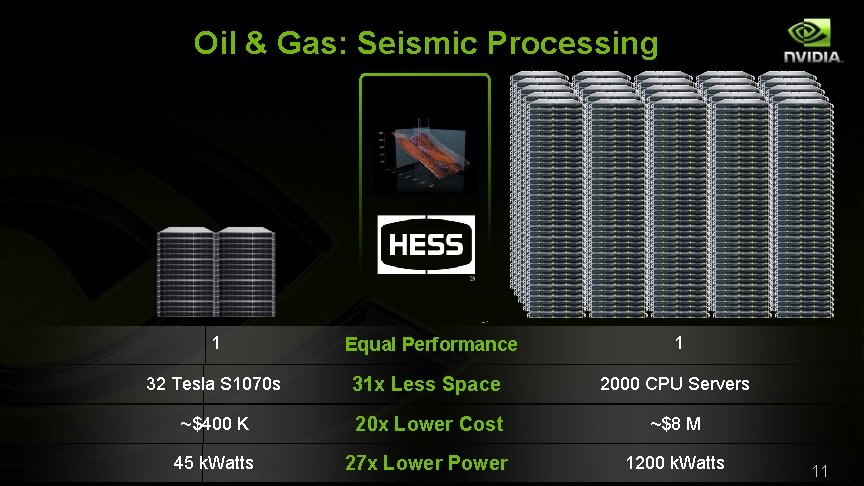
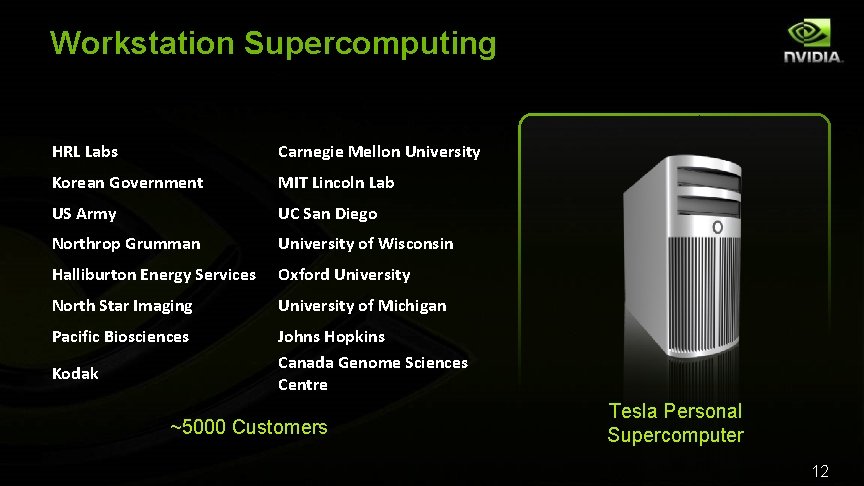
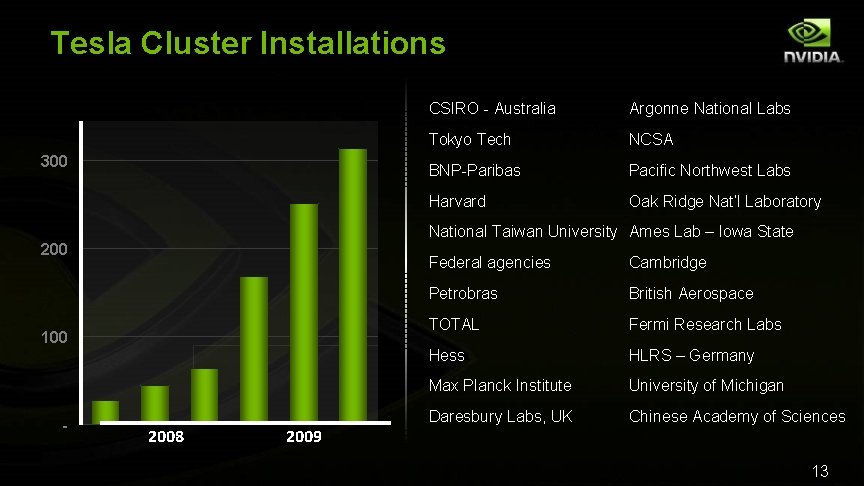
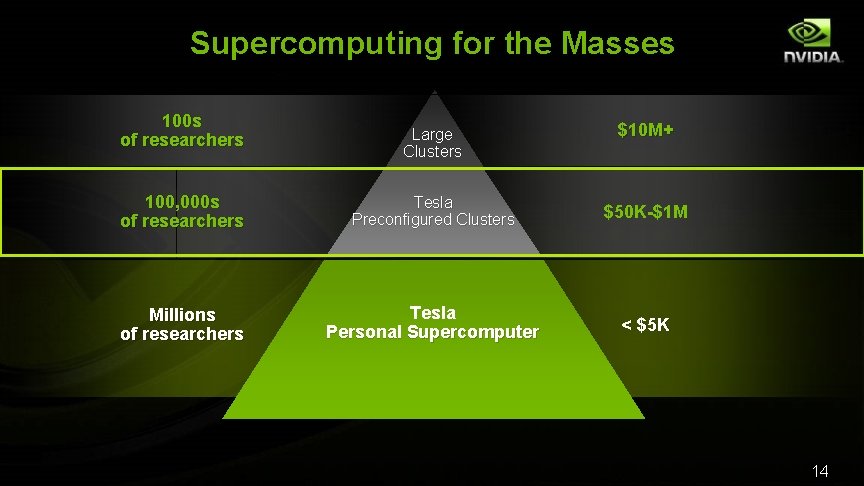
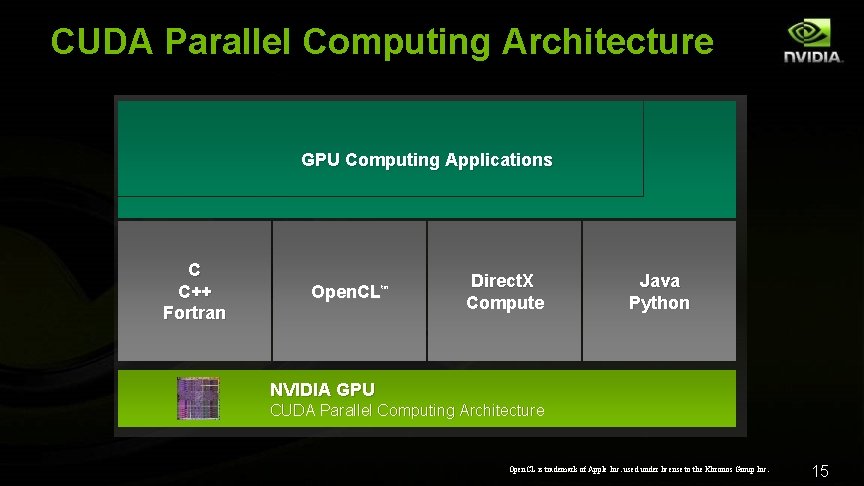
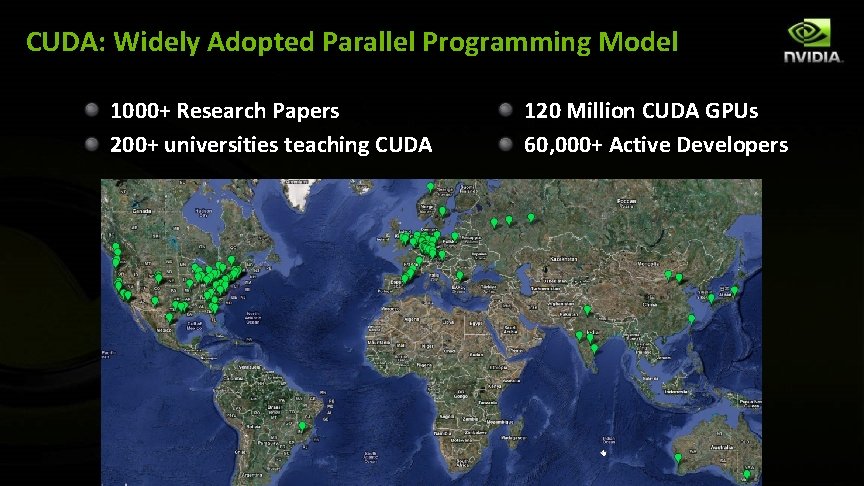
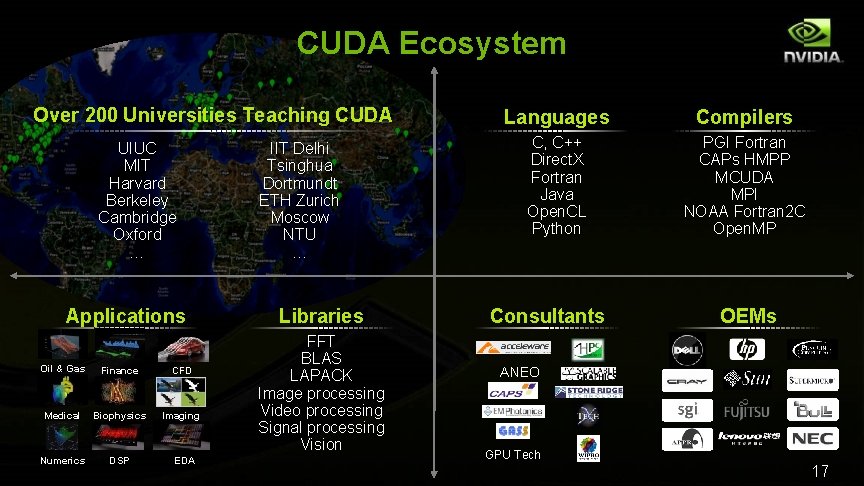
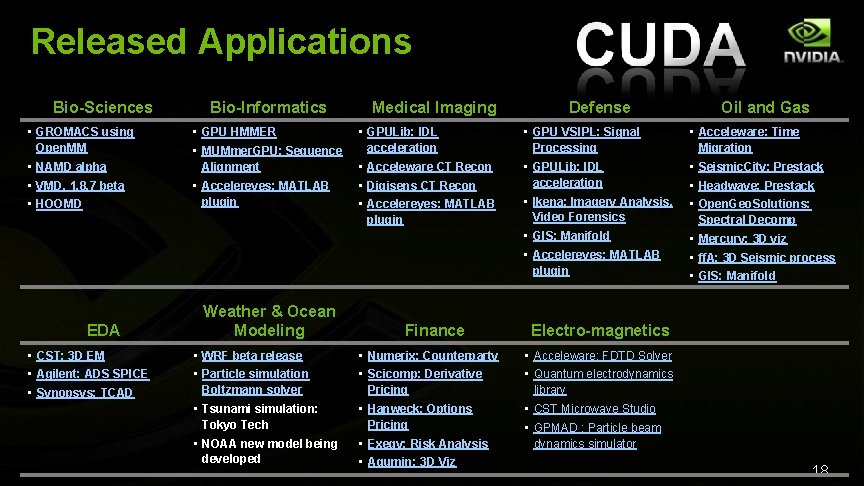
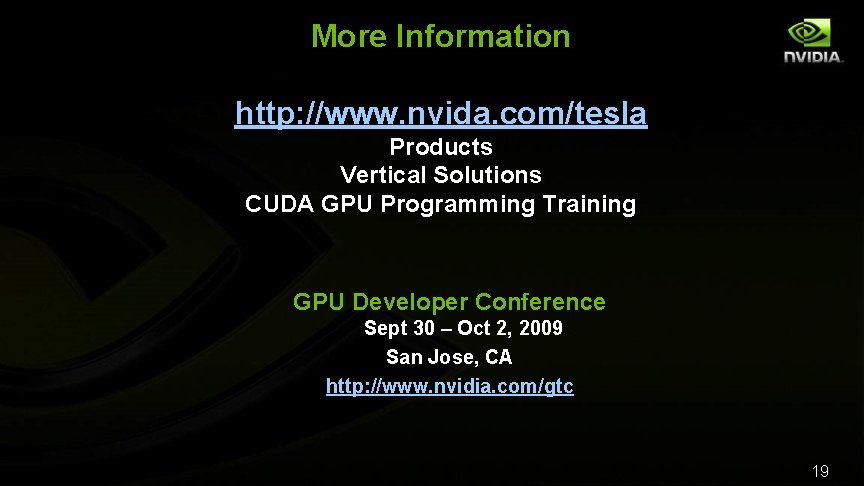
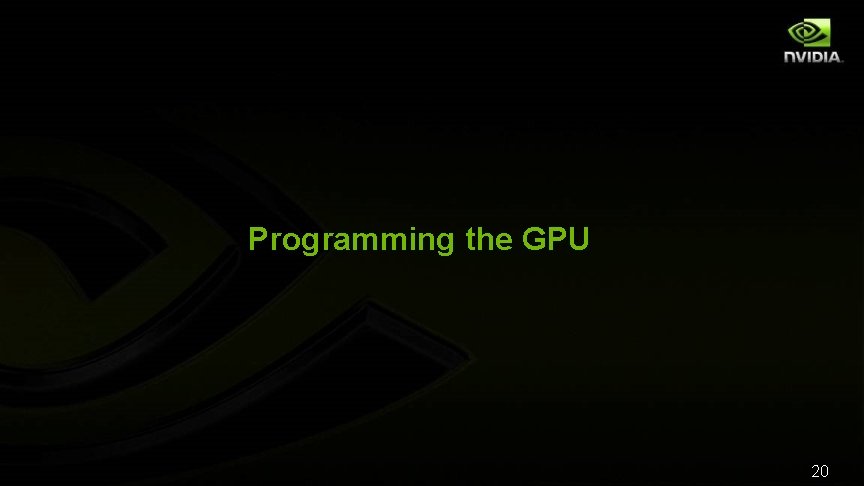
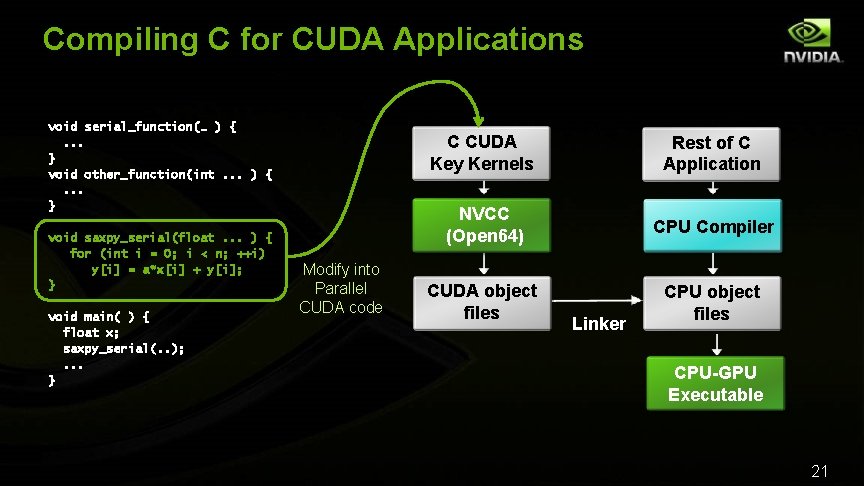
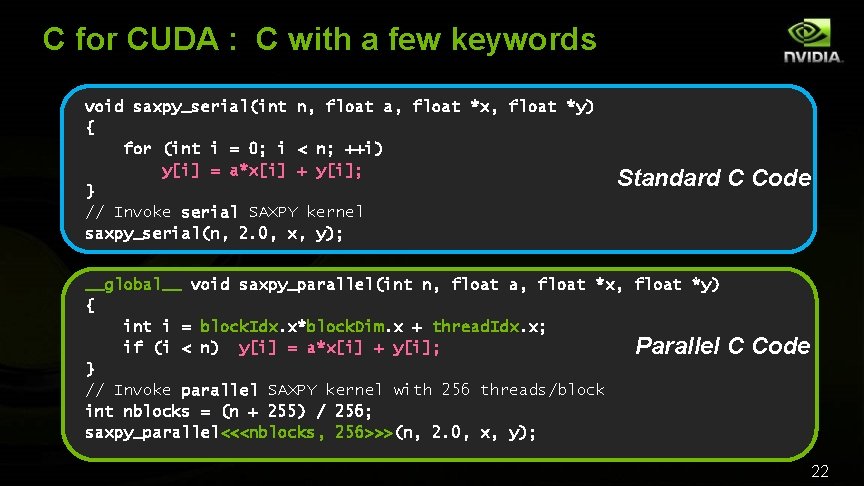
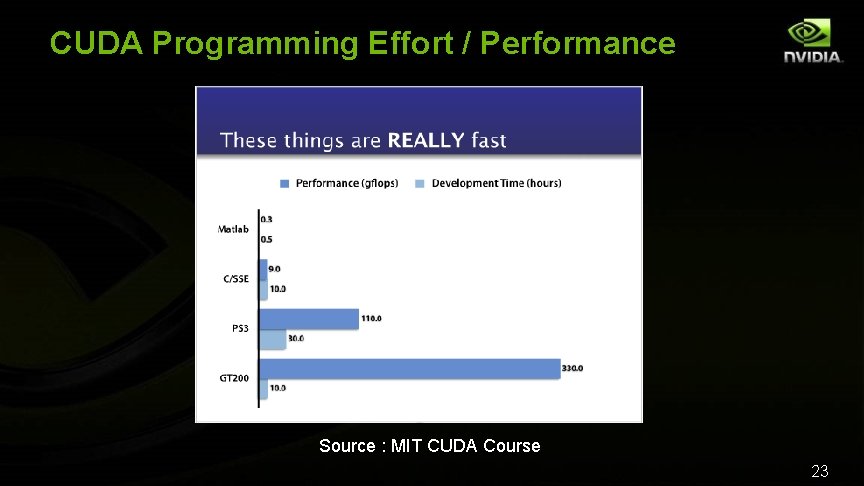
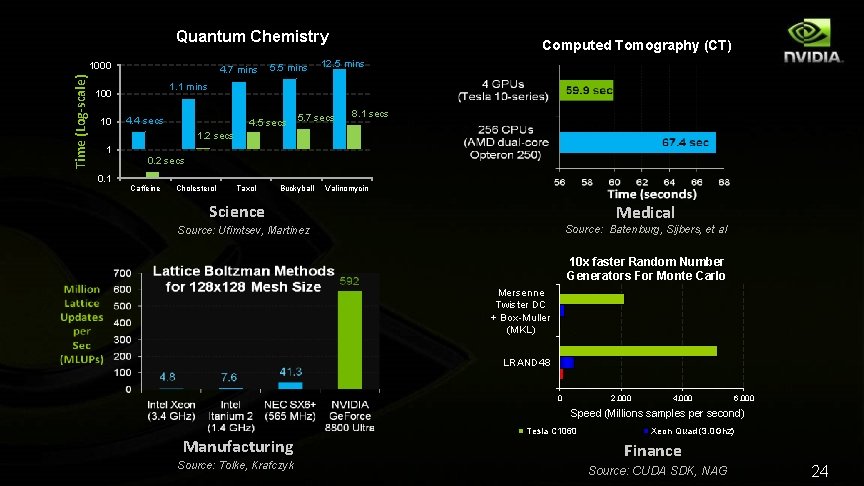
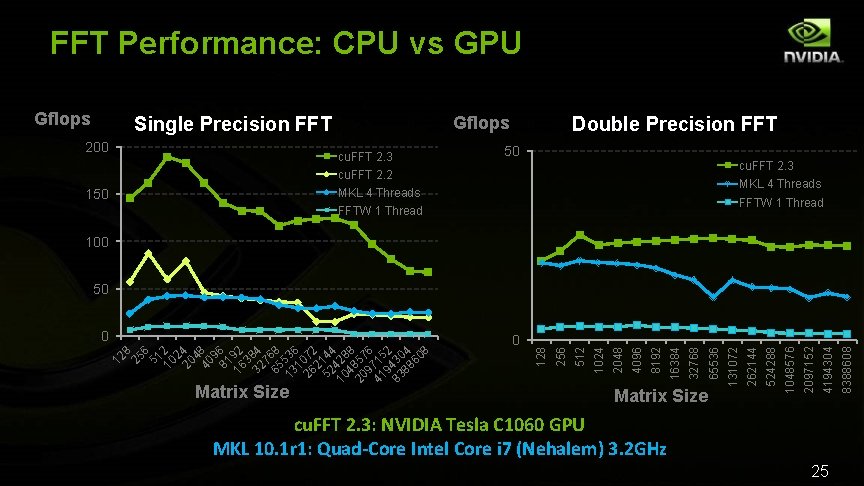
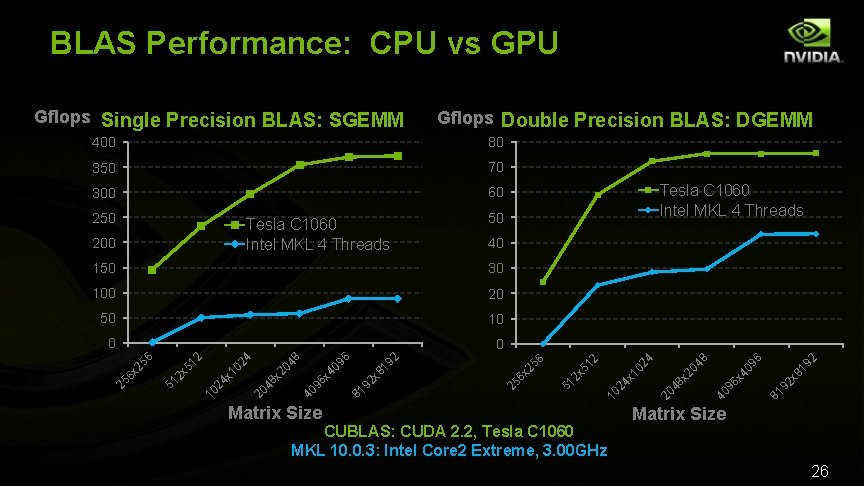
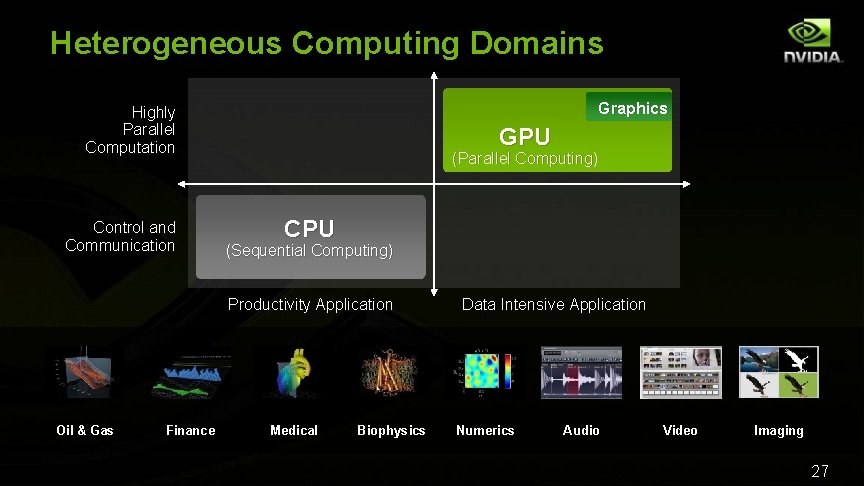
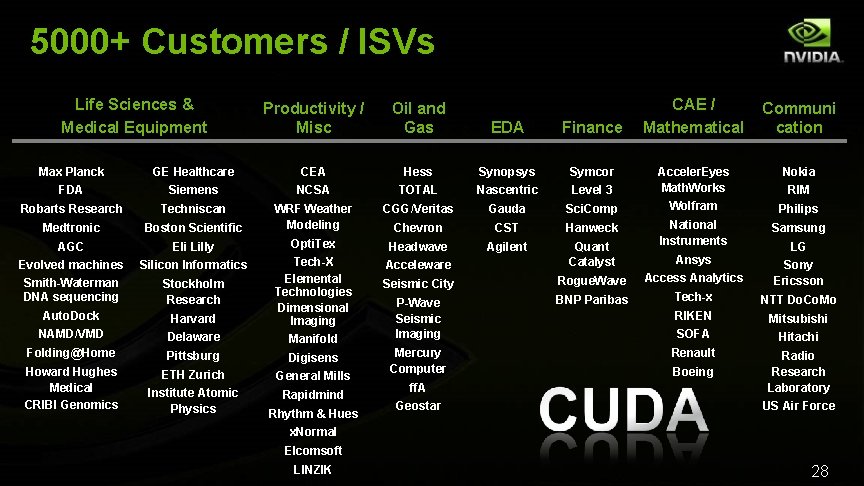
- Slides: 28
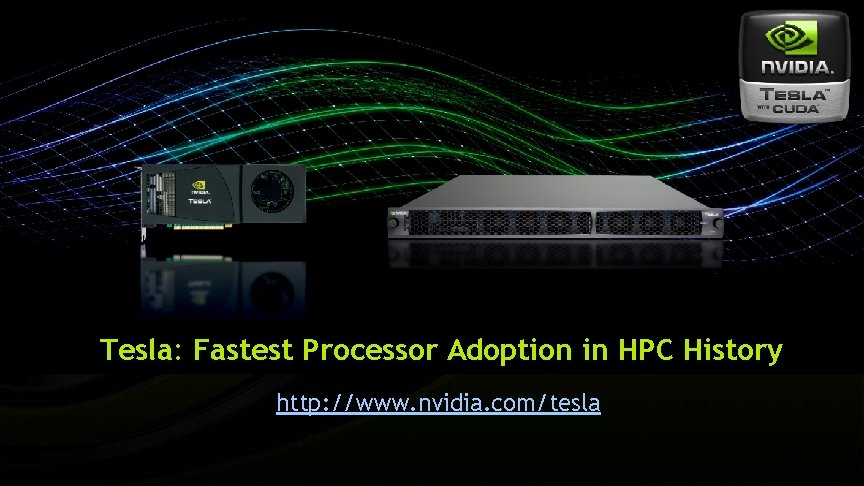
Tesla: Fastest Processor Adoption in HPC History http: //www. nvidia. com/tesla
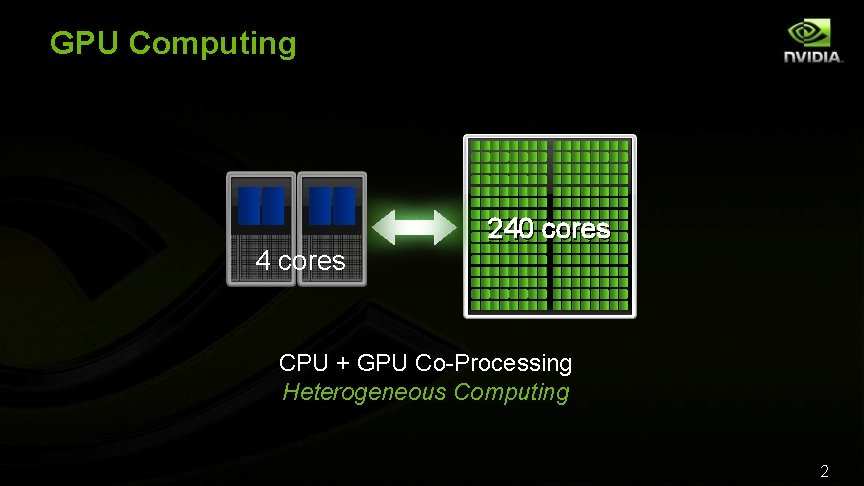
GPU Computing 4 cores 240 cores CPU + GPU Co-Processing Heterogeneous Computing 2
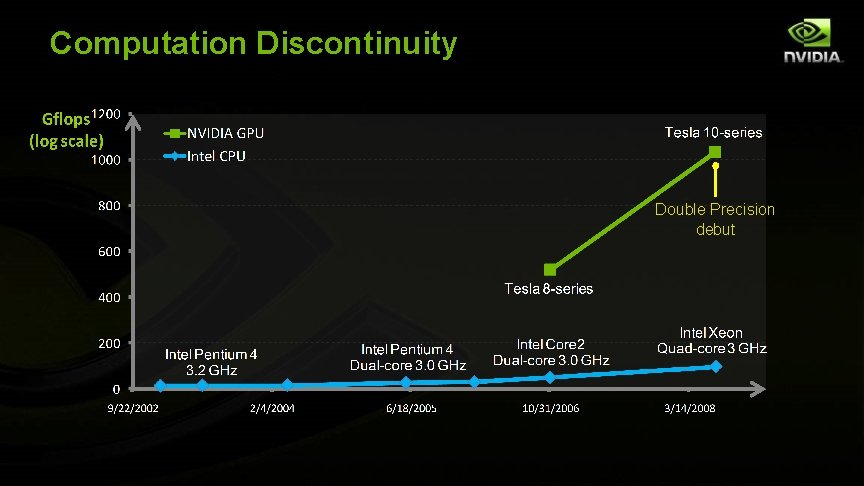
Computation Discontinuity Double Precision debut
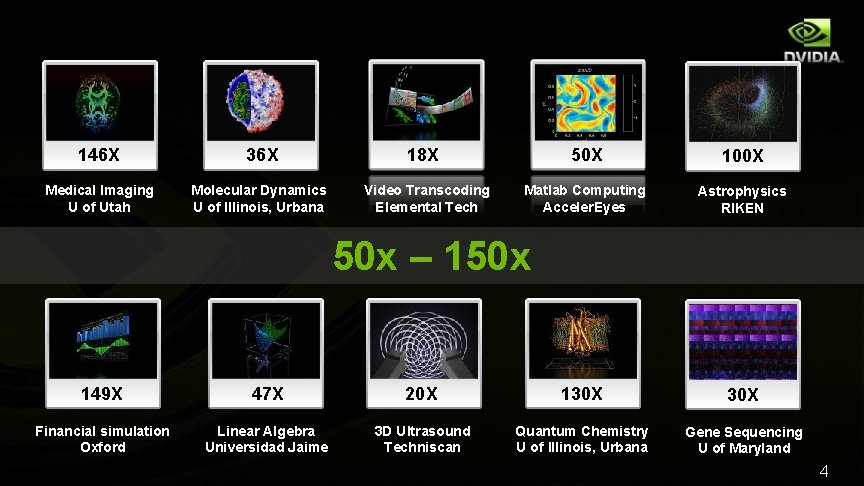
146 X 36 X 18 X 50 X 100 X Medical Imaging U of Utah Molecular Dynamics U of Illinois, Urbana Video Transcoding Elemental Tech Matlab Computing Acceler. Eyes Astrophysics RIKEN 50 x – 150 x 149 X 47 X 20 X 130 X Financial simulation Oxford Linear Algebra Universidad Jaime 3 D Ultrasound Techniscan Quantum Chemistry U of Illinois, Urbana Gene Sequencing U of Maryland 4
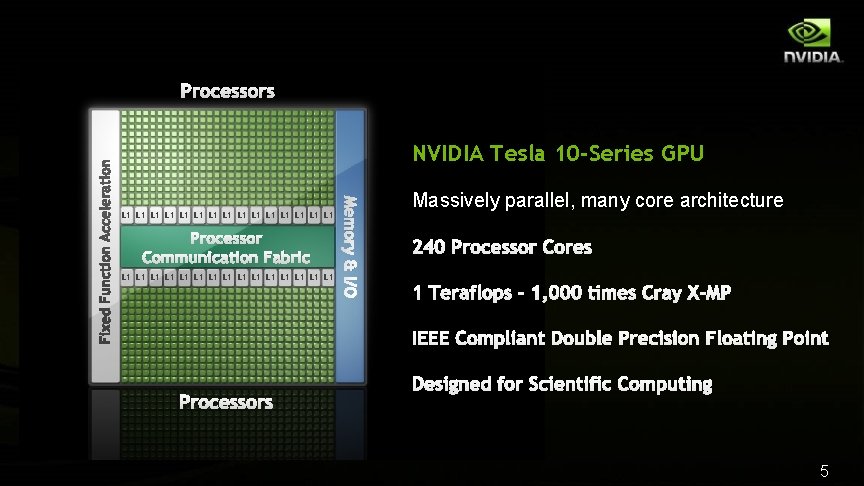
Processors NVIDIA Tesla 10 -Series GPU Processor Communication Fabric L 1 L 1 L 1 L 1 Memory & I/O L 1 L 1 L 1 L 1 Massively parallel, many core architecture 240 Processor Cores 1 Teraflops – 1, 000 times Cray X-MP IEEE Compliant Double Precision Floating Point Processors Designed for Scientific Computing 5
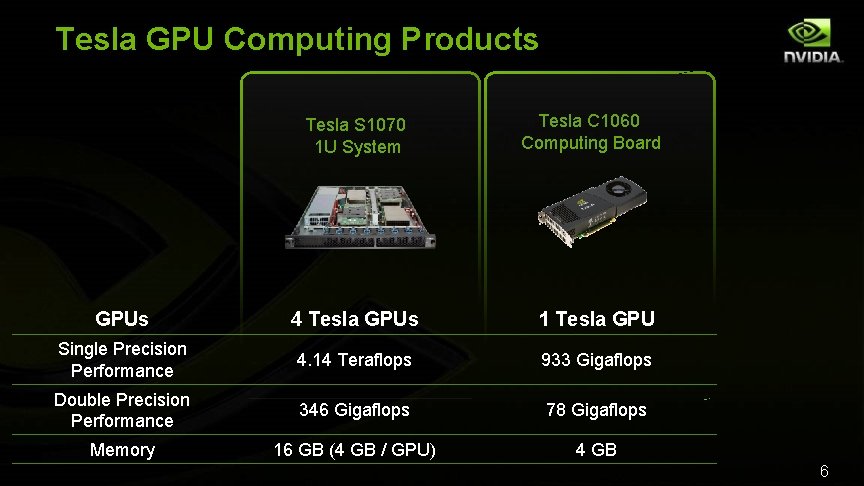
Tesla GPU Computing Products Tesla S 1070 1 U System Tesla C 1060 Computing Board GPUs 4 Tesla GPUs 1 Tesla GPU Single Precision Performance 4. 14 Teraflops 933 Gigaflops Double Precision Performance 346 Gigaflops 78 Gigaflops Memory 16 GB (4 GB / GPU) 4 GB 6
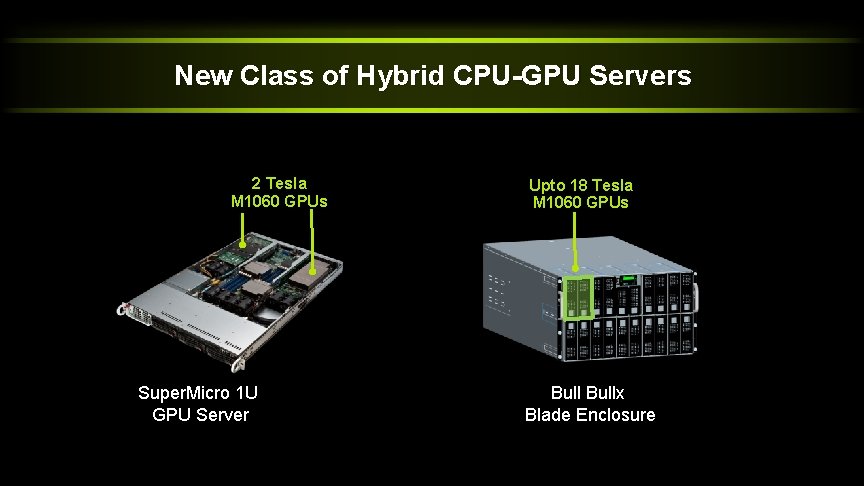
New Class of Hybrid CPU-GPU Servers 2 Tesla M 1060 GPUs Super. Micro 1 U GPU Server Upto 18 Tesla M 1060 GPUs Bullx Blade Enclosure 7
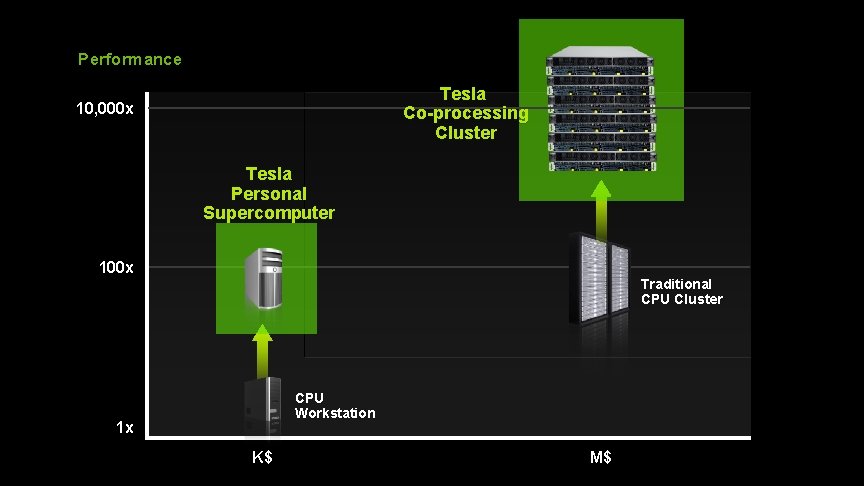
Performance Tesla Co-processing Cluster 10, 000 x Tesla Personal Supercomputer 100 x Traditional CPU Cluster CPU Workstation 1 x K$ M$ 8
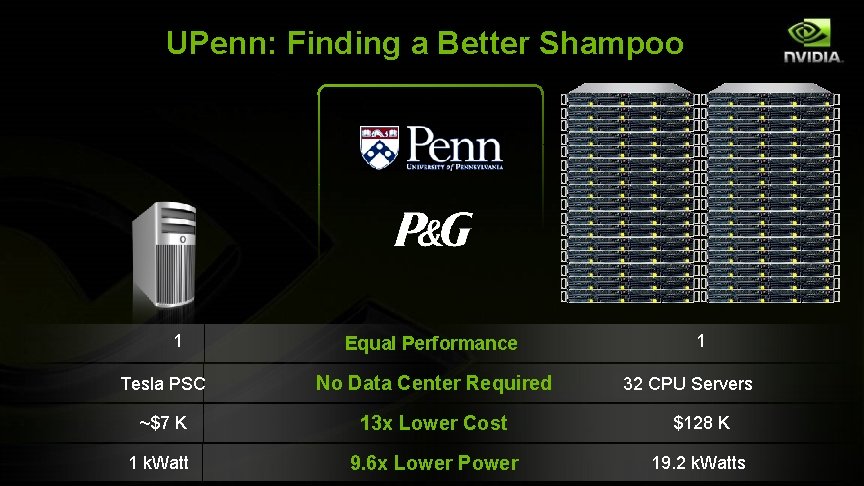
UPenn: Finding a Better Shampoo 1 Equal Performance 1 Tesla PSC No Data Center Required ~$7 K 13 x Lower Cost $128 K 1 k. Watt 9. 6 x Lower Power 19. 2 k. Watts 32 CPU Servers
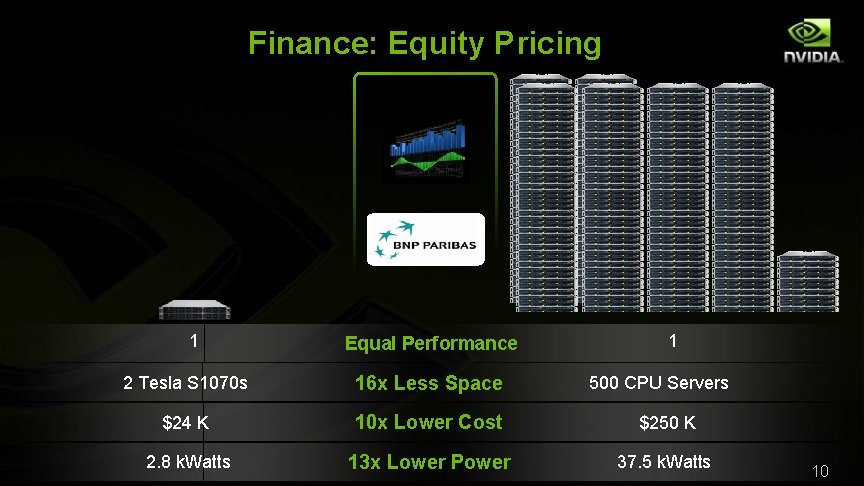
Finance: Equity Pricing 1 Equal Performance 1 2 Tesla S 1070 s 16 x Less Space $24 K 10 x Lower Cost $250 K 2. 8 k. Watts 13 x Lower Power 37. 5 k. Watts 500 CPU Servers 10
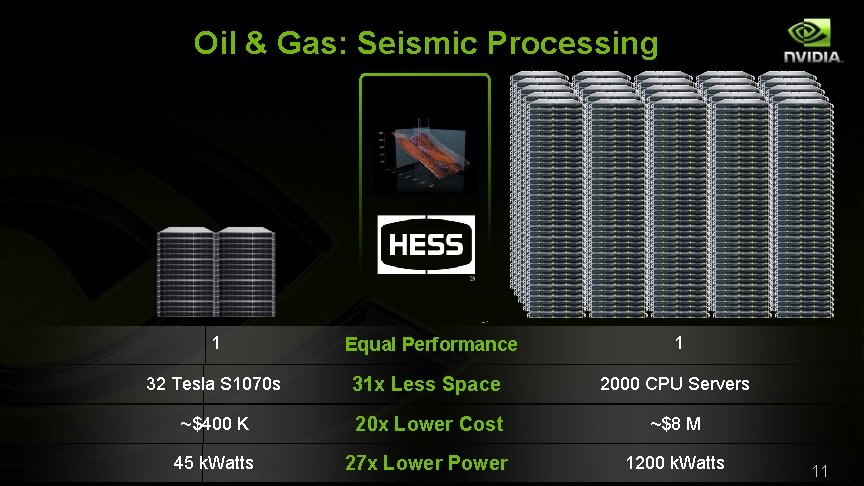
Oil & Gas: Seismic Processing 1 Equal Performance 1 32 Tesla S 1070 s 31 x Less Space 2000 CPU Servers ~$400 K 20 x Lower Cost ~$8 M 45 k. Watts 27 x Lower Power 1200 k. Watts 11
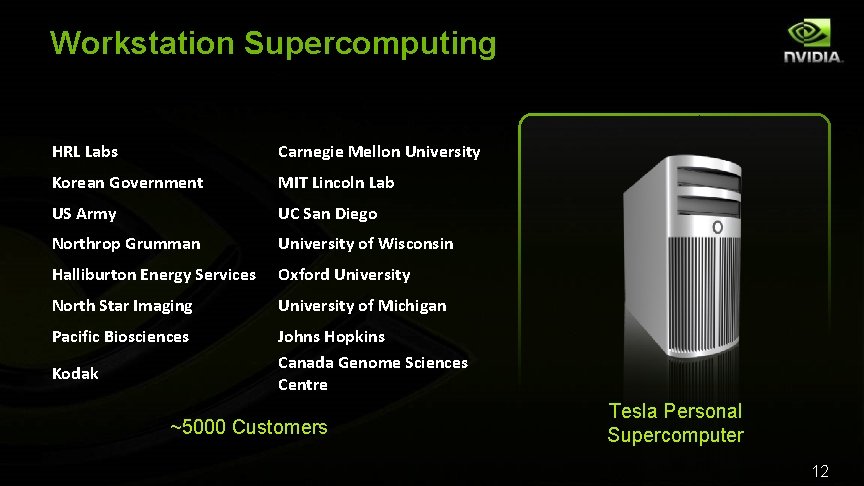
Workstation Supercomputing HRL Labs Carnegie Mellon University Korean Government MIT Lincoln Lab US Army UC San Diego Northrop Grumman University of Wisconsin Halliburton Energy Services Oxford University North Star Imaging University of Michigan Pacific Biosciences Johns Hopkins Canada Genome Sciences Centre Kodak ~5000 Customers Tesla Personal Supercomputer 12
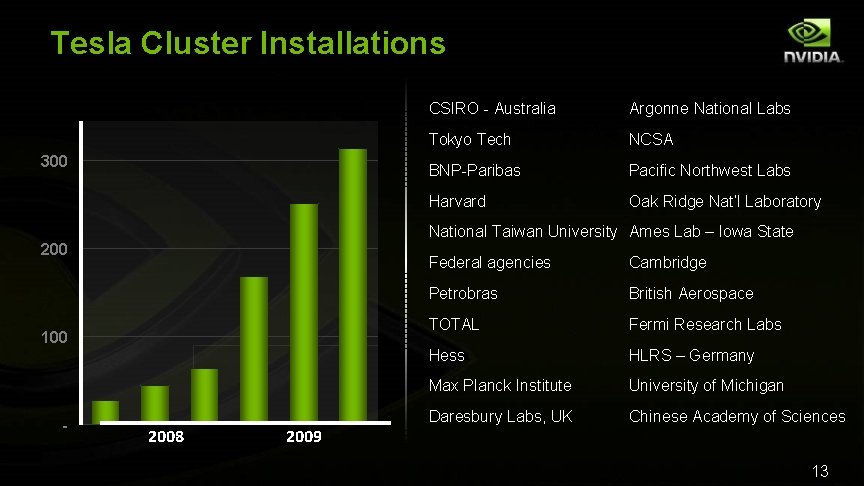
Tesla Cluster Installations 400 300 Argonne National Labs Tokyo Tech NCSA BNP-Paribas Pacific Northwest Labs Harvard Oak Ridge Nat’l Laboratory National Taiwan University Ames Lab – Iowa State 200 100 - CSIRO - Australia 2008 2009 Federal agencies Cambridge Petrobras British Aerospace TOTAL Fermi Research Labs Hess HLRS – Germany Max Planck Institute University of Michigan Daresbury Labs, UK Chinese Academy of Sciences 13
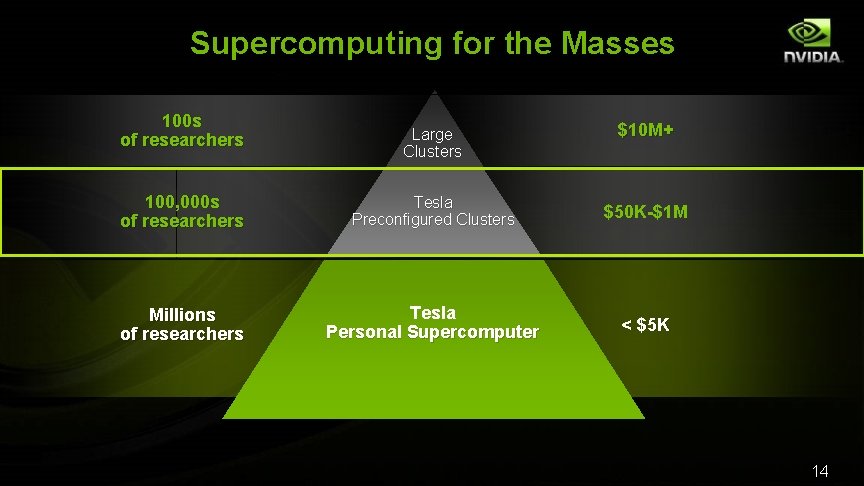
Supercomputing for the Masses 100 s of researchers Large Clusters $10 M+ 100, 000 s of researchers Tesla Preconfigured Clusters $50 K-$1 M Millions of researchers Tesla Personal Supercomputer < $5 K 14
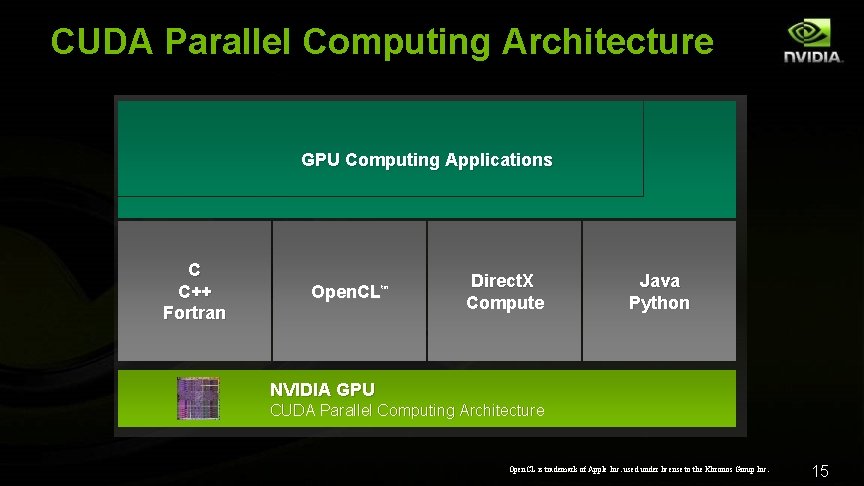
CUDA Parallel Computing Architecture GPU Computing Applications C C++ Fortran Open. CL tm Direct. X Compute Java Python NVIDIA GPU CUDA Parallel Computing Architecture Open. CL is trademark of Apple Inc. used under license to the Khronos Group Inc. 15
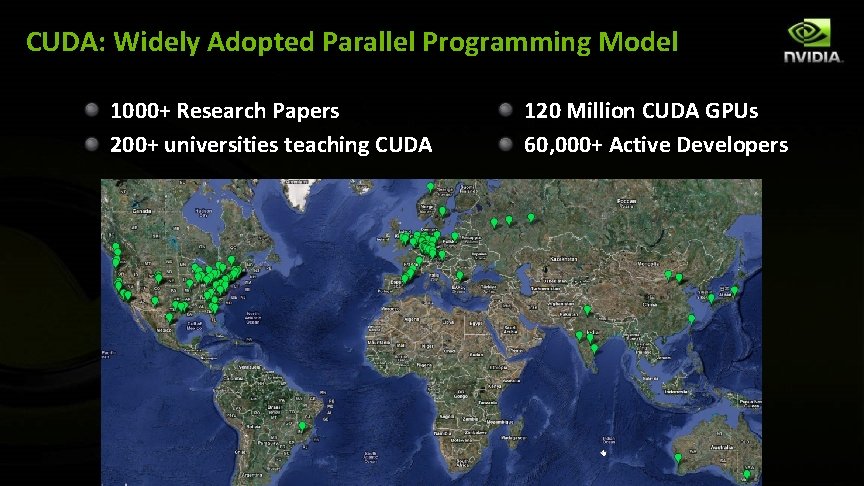
CUDA: Widely Adopted Parallel Programming Model 1000+ Research Papers 200+ universities teaching CUDA 120 Million CUDA GPUs 60, 000+ Active Developers
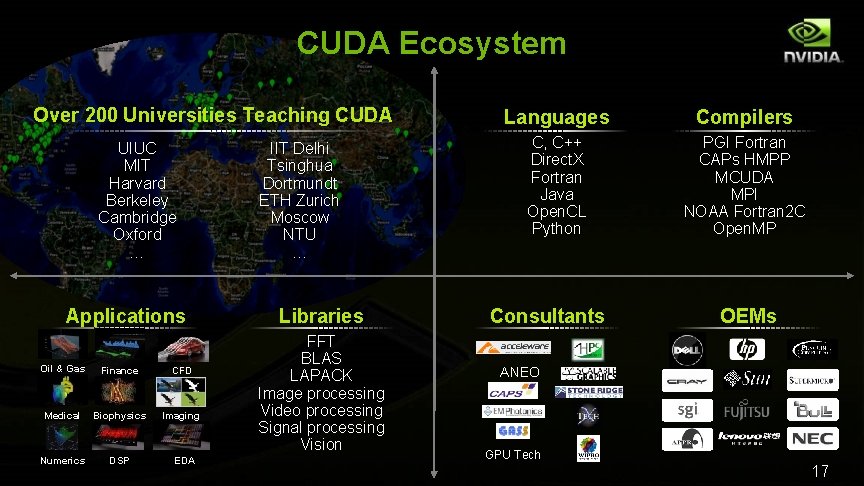
CUDA Ecosystem Over 200 Universities Teaching CUDA UIUC MIT Harvard Berkeley Cambridge Oxford … Applications Oil & Gas Finance CFD Medical Biophysics Imaging Numerics DSP EDA IIT Delhi Tsinghua Dortmundt ETH Zurich Moscow NTU … Libraries FFT BLAS LAPACK Image processing Video processing Signal processing Vision Languages Compilers C, C++ Direct. X Fortran Java Open. CL Python PGI Fortran CAPs HMPP MCUDA MPI NOAA Fortran 2 C Open. MP Consultants OEMs ANEO GPU Tech 17
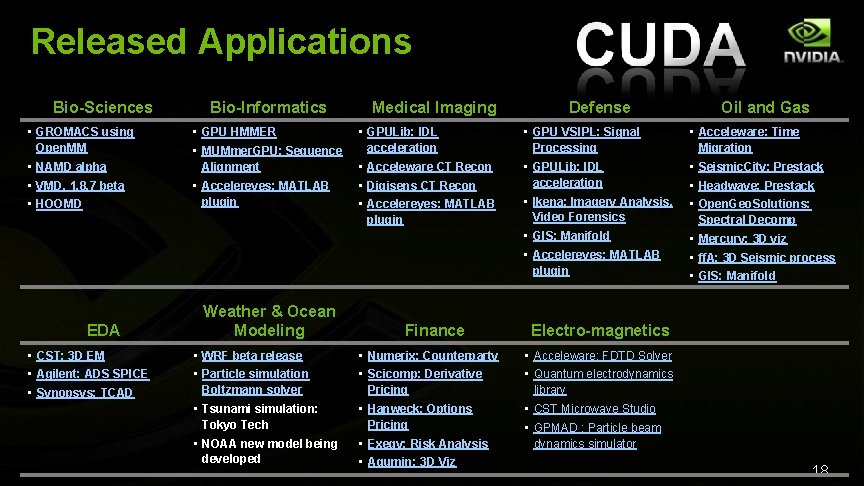
Released Applications Bio-Sciences • GROMACS using Open. MM • NAMD alpha • VMD, 1. 8. 7 beta • HOOMD EDA • CST: 3 D EM • Agilent: ADS SPICE • Synopsys: TCAD Bio-Informatics • GPU HMMER • MUMmer. GPU: Sequence Alignment • Accelereyes: MATLAB plugin Weather & Ocean Modeling • WRF beta release • Particle simulation Boltzmann solver • Tsunami simulation: Tokyo Tech • NOAA new model being developed Medical Imaging • GPULib: IDL acceleration • Acceleware CT Recon • Digisens CT Recon • Accelereyes: MATLAB plugin Finance • Numerix: Counterparty • Scicomp: Derivative Pricing • Hanweck: Options Pricing • Exegy: Risk Analysis • Aqumin: 3 D Viz Defense Oil and Gas • GPU VSIPL: Signal Processing • GPULib: IDL acceleration • Ikena: Imagery Analysis, Video Forensics • GIS: Manifold • Accelereyes: MATLAB plugin • Acceleware: Time Migration • Seismic. City: Prestack • Headwave: Prestack • Open. Geo. Solutions: Spectral Decomp • Mercury: 3 D viz • ff. A: 3 D Seismic process • GIS: Manifold Electro-magnetics • Acceleware: FDTD Solver • Quantum electrodynamics library • CST Microwave Studio • GPMAD : Particle beam dynamics simulator 18
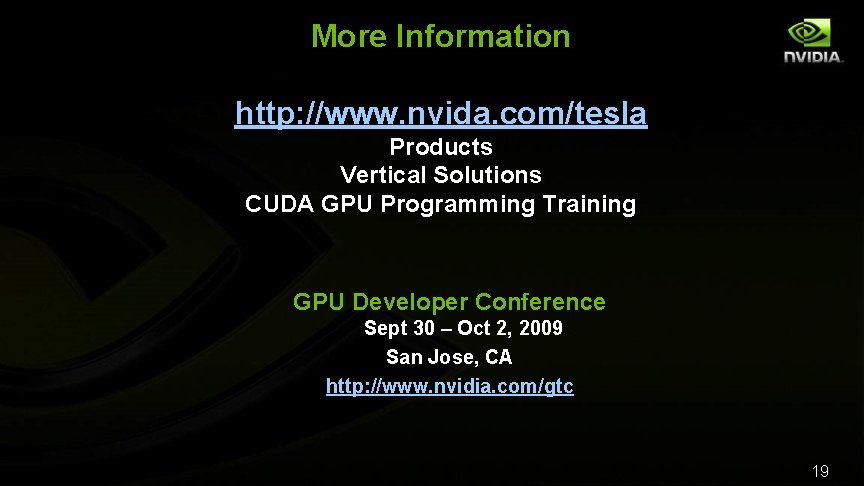
More Information http: //www. nvida. com/tesla Products Vertical Solutions CUDA GPU Programming Training GPU Developer Conference Sept 30 – Oct 2, 2009 San Jose, CA http: //www. nvidia. com/gtc 19
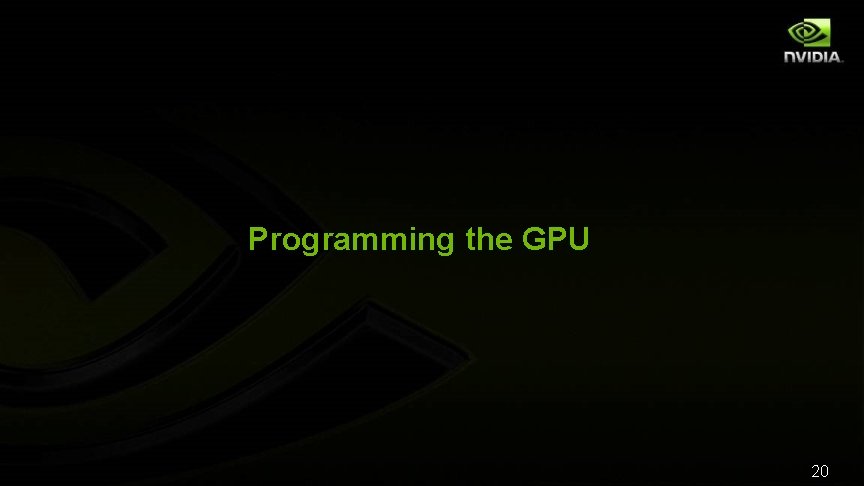
Programming the GPU 20
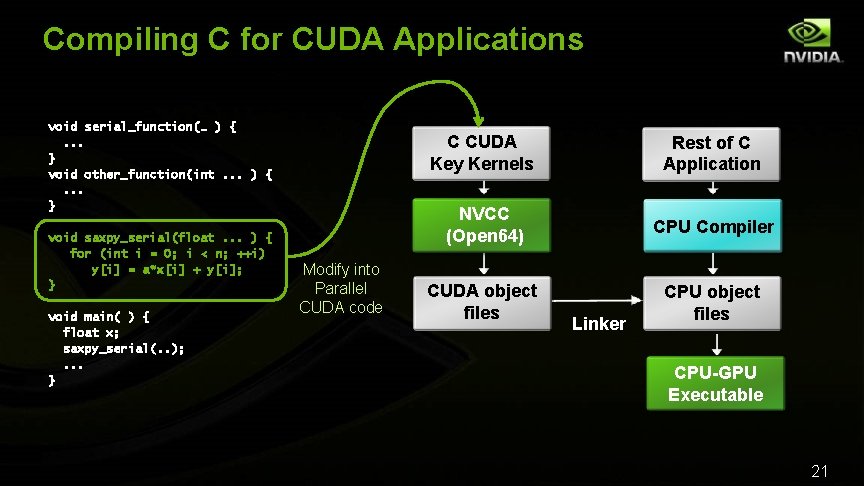
Compiling C for CUDA Applications void serial_function(… ) {. . . } void other_function(int. . . ) {. . . } void saxpy_serial(float. . . ) { for (int i = 0; i < n; ++i) y[i] = a*x[i] + y[i]; } void main( ) { float x; saxpy_serial(. . ); . . . } Modify into Parallel CUDA code C CUDA Key Kernels Rest of C Application NVCC (Open 64) CPU Compiler CUDA object files CPU object files Linker CPU-GPU Executable 21
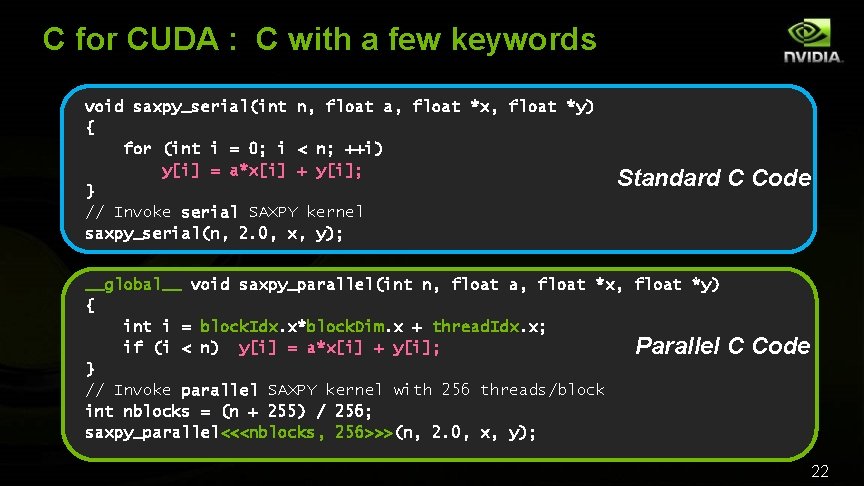
C for CUDA : C with a few keywords void saxpy_serial(int n, float a, float *x, float *y) { for (int i = 0; i < n; ++i) y[i] = a*x[i] + y[i]; } // Invoke serial SAXPY kernel saxpy_serial(n, 2. 0, x, y); Standard C Code __global__ void saxpy_parallel(int n, float a, float *x, float *y) { int i = block. Idx. x*block. Dim. x + thread. Idx. x; if (i < n) y[i] = a*x[i] + y[i]; Parallel } // Invoke parallel SAXPY kernel with 256 threads/block int nblocks = (n + 255) / 256; saxpy_parallel<<<nblocks, 256>>>(n, 2. 0, x, y); C Code 22
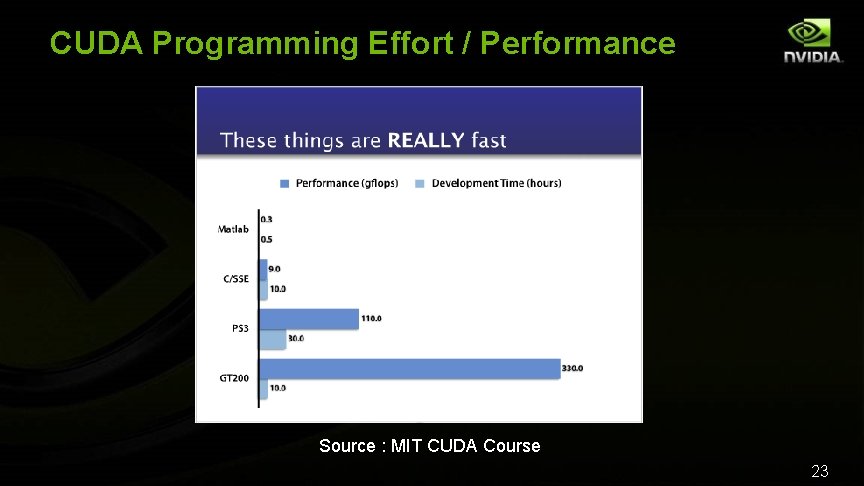
CUDA Programming Effort / Performance Source : MIT CUDA Course 23
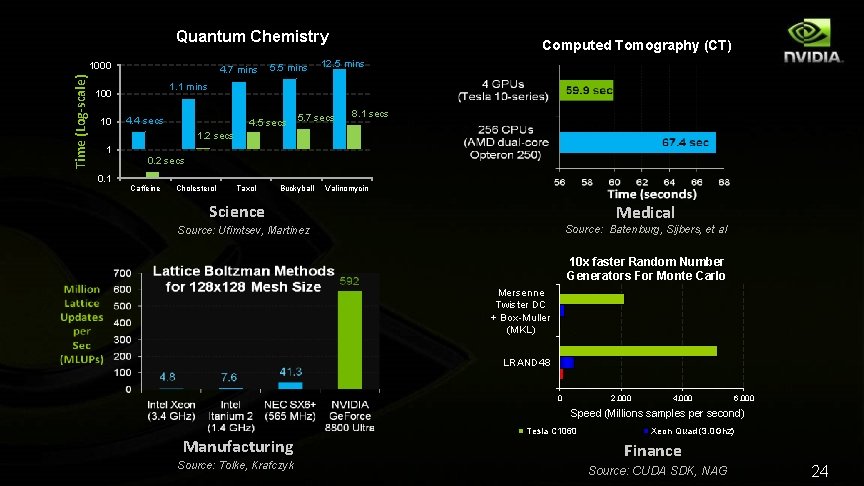
Quantum Chemistry Time (Log-scale) 1000 4. 7 mins 12. 5 mins 1. 1 mins 100 10 5. 5 mins Computed Tomography (CT) 4. 4 secs 4. 5 secs 5. 7 secs 8. 1 secs 1. 2 secs 1 0. 2 secs Caffeine Cholesterol Taxol Buckyball Valinomycin Science Medical Source: Batenburg, Sijbers, et al Source: Ufimtsev, Martinez 10 x faster Random Number Generators For Monte Carlo Mersenne Twister DC + Box-Muller (MKL) LRAND 48 0 2, 000 4, 000 6, 000 Speed (Millions samples per second) Manufacturing Source: Tolke, Krafczyk Tesla C 1060 Xeon Quad (3. 0 Ghz) Finance Source: CUDA SDK, NAG 24
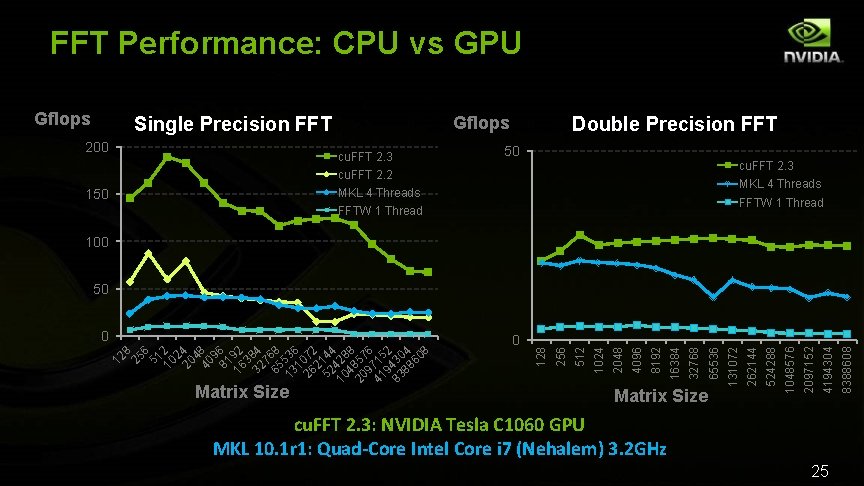
FFT Performance: CPU vs GPU Gflops Single Precision FFT 200 cu. FFT 2. 3 Double Precision FFT 50 cu. FFT 2. 3 cu. FFT 2. 2 MKL 4 Threads 150 FFTW 1 Thread 100 cu. FFT 2. 3: NVIDIA Tesla C 1060 GPU MKL 10. 1 r 1: Quad-Core Intel Core i 7 (Nehalem) 3. 2 GHz 25 8388608 4194304 2097152 1048576 524288 262144 65536 32768 16384 8192 4096 1024 2048 Matrix Size 131072 Matrix Size 512 12 8 25 6 51 10 2 2 20 4 4 40 8 9 81 6 16 92 3 32 84 7 65 68 13 536 1 26 072 2 52 144 10 428 4 8 20 857 9 6 41 715 9 2 83 430 88 4 60 8 0 256 0 128 50
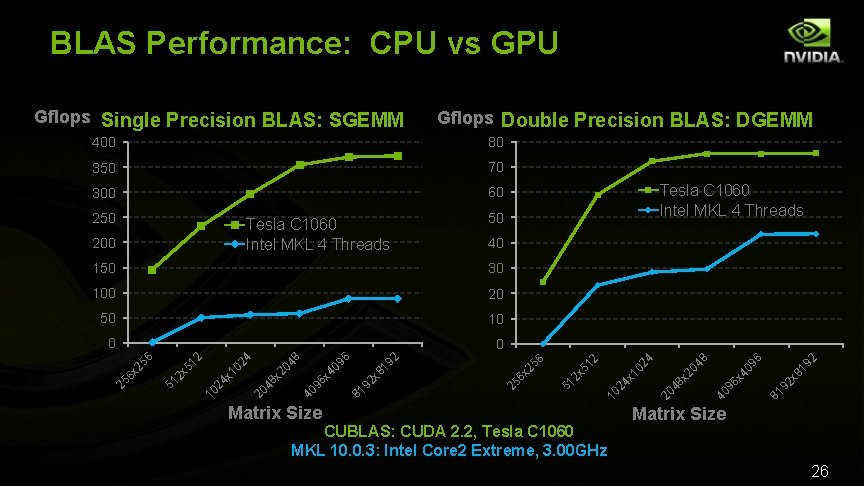
BLAS Performance: CPU vs GPU Gflops Double Precision BLAS: DGEMM 400 80 350 70 300 60 CUBLAS: CUDA 2. 2, Tesla C 1060 MKL 10. 0. 3: Intel Core 2 Extreme, 3. 00 GHz 19 2 x 8 81 92 09 6 x 4 04 8 40 96 25 6 x 19 2 x 8 81 92 x 4 40 96 x 2 20 48 10 24 51 2 x 25 6 x x 1 Matrix Size x 2 0 20 48 0 25 6 10 09 6 50 04 8 20 02 4 100 51 2 30 25 6 150 02 4 40 x 1 200 50 10 24 Tesla C 1060 Intel MKL 4 Threads 51 2 250 Tesla C 1060 Intel MKL 4 Threads 51 2 x Gflops Single Precision BLAS: SGEMM Matrix Size 26
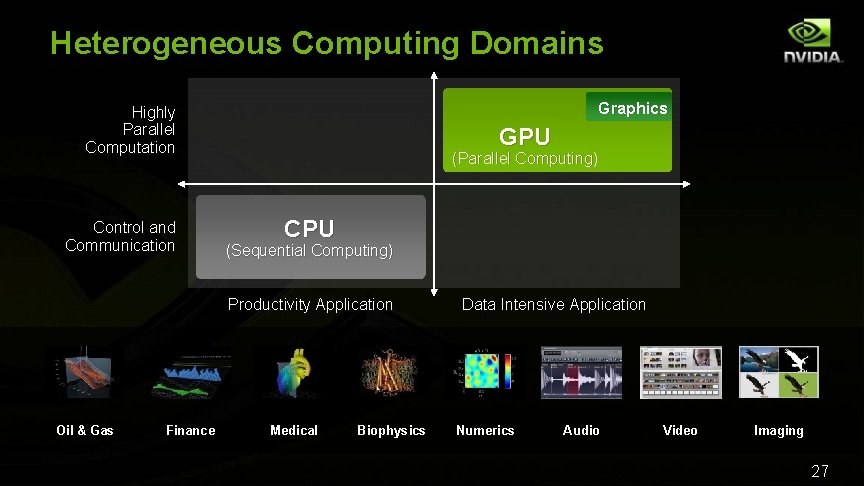
Heterogeneous Computing Domains Graphics Highly Parallel Computation Control and Communication GPU (Parallel Computing) CPU (Sequential Computing) Productivity Application Oil & Gas Finance Medical Biophysics Data Intensive Application Numerics Audio Video Imaging 27
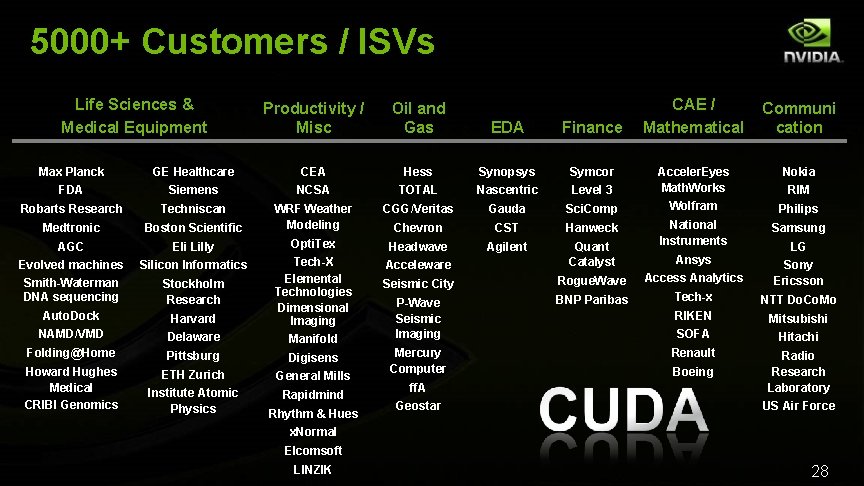
5000+ Customers / ISVs Life Sciences & Medical Equipment Productivity / Misc Oil and Gas EDA Finance CAE / Mathematical Communi cation Nokia Max Planck GE Healthcare CEA Hess Synopsys Symcor FDA Siemens NCSA TOTAL Nascentric Level 3 Acceler. Eyes Math. Works Robarts Research Techniscan CGG/Veritas Gauda Sci. Comp Wolfram Philips Medtronic Boston Scientific WRF Weather Modeling Chevron CST Hanweck Samsung AGC Eli Lilly Opti. Tex Headwave Agilent Evolved machines Smith-Waterman DNA sequencing Silicon Informatics Acceleware Ansys Seismic City Rogue. Wave Access Analytics Sony Ericsson Tech-x NTT Do. Co. Mo Harvard RIKEN Mitsubishi NAMD/VMD Delaware Manifold P-Wave Seismic Imaging BNP Paribas Auto. Dock Tech-X Elemental Technologies Dimensional Imaging Quant Catalyst National Instruments SOFA Hitachi Folding@Home Pittsburg Digisens Renault ff. A Radio Research Laboratory Geostar US Air Force Howard Hughes Medical CRIBI Genomics Stockholm Research ETH Zurich General Mills Institute Atomic Physics Rapidmind Rhythm & Hues Mercury Computer Boeing RIM LG x. Normal Elcomsoft LINZIK 28
Processor history
Jazelle instruction set
Pentium processor history
Multi core processor history
How is sound produced
What the theme of “excerpt from hattie big sky?”
Superlative the cold
Sound sol
Library.thinkquest.org 19537
Fastest way to get a job
Sound travels fastest through
The fastest land dwelling creature is the cheetah
Ecotourism is one of the fastest
Why dark chocolate melts faster
Skip search
Which liquid freezes the fastest
Fastest tap
Degree of comparison of evil
Mechanical reasoning
Sound travels fastest in
Fastest growing sport in america
Excerpt from hattie big sky answers
Define sound energy
Classification of structures
Normal fault
Http //mbs.meb.gov.tr/ http //www.alantercihleri.com
Http //siat.ung.ac.id atau http //pmb.ung.ac.id
User adoption portal
User adoption tracking