Summary of Genome Annotation Assessment in Drosophila melanogaster
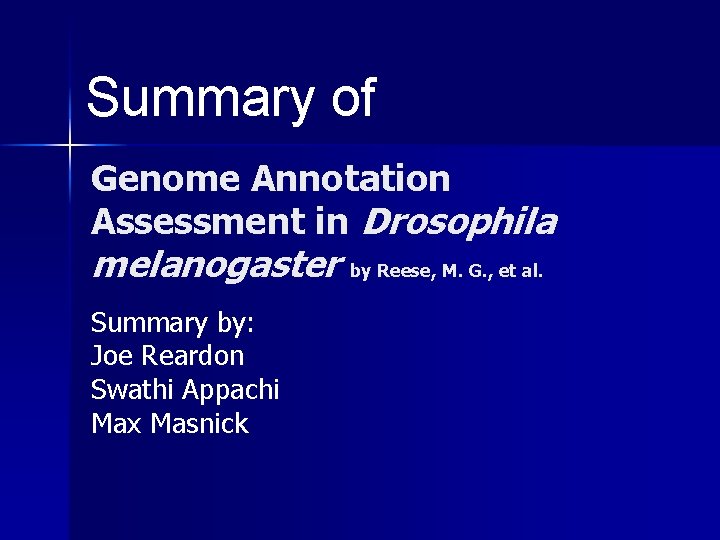
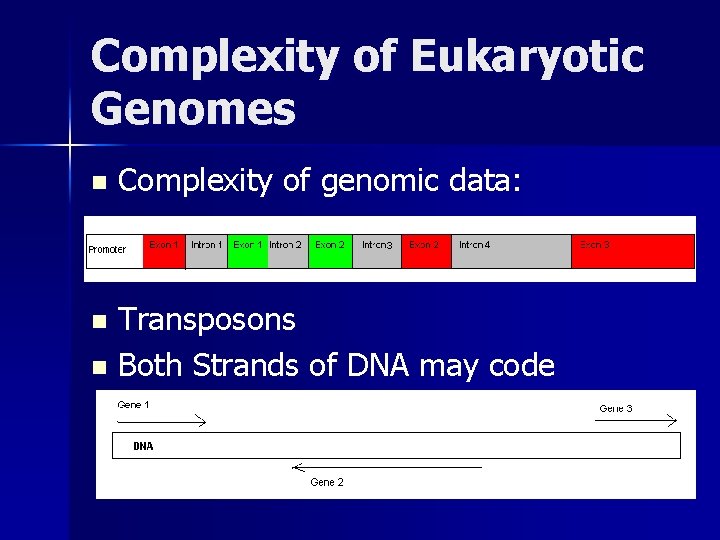
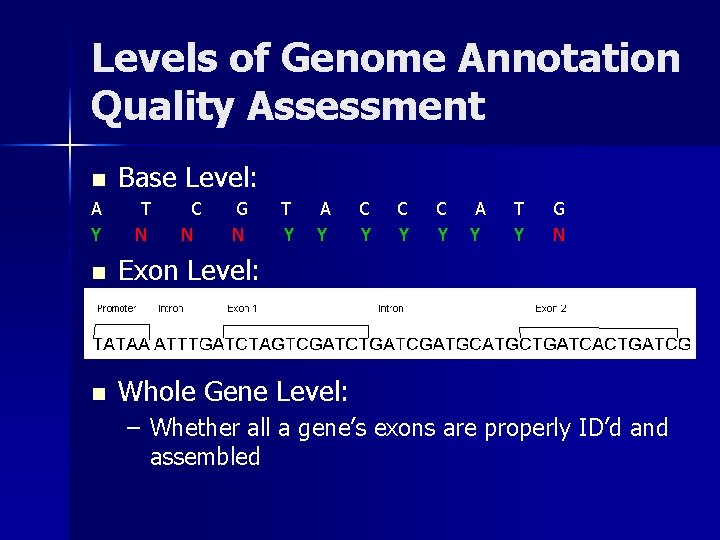
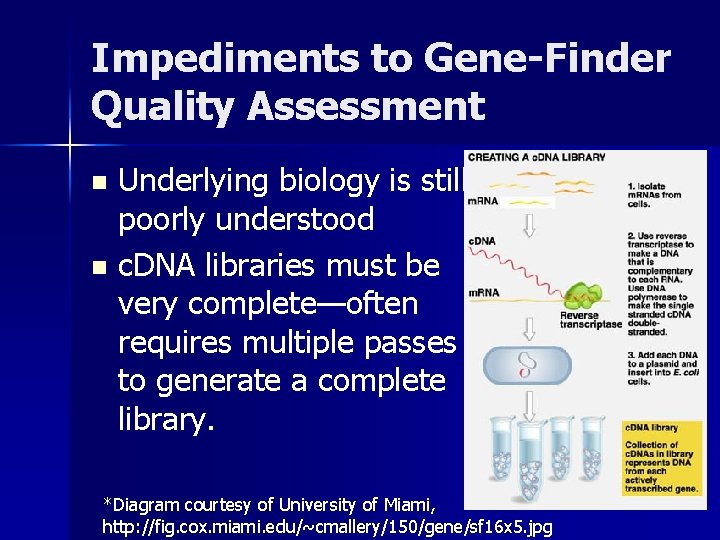
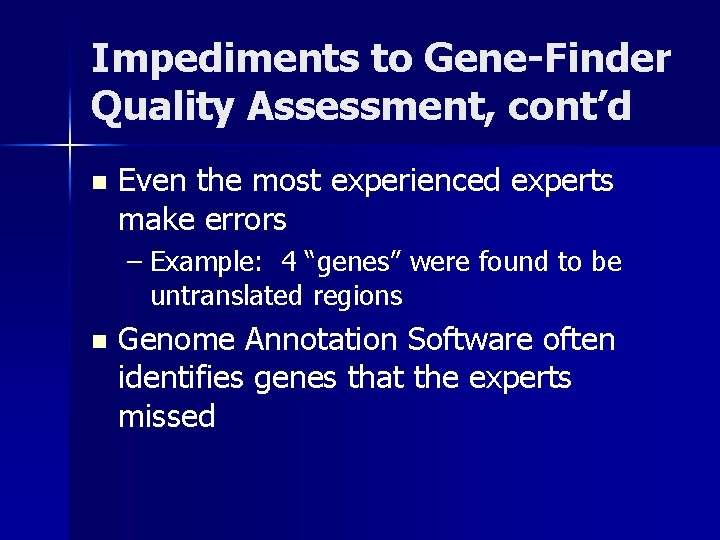
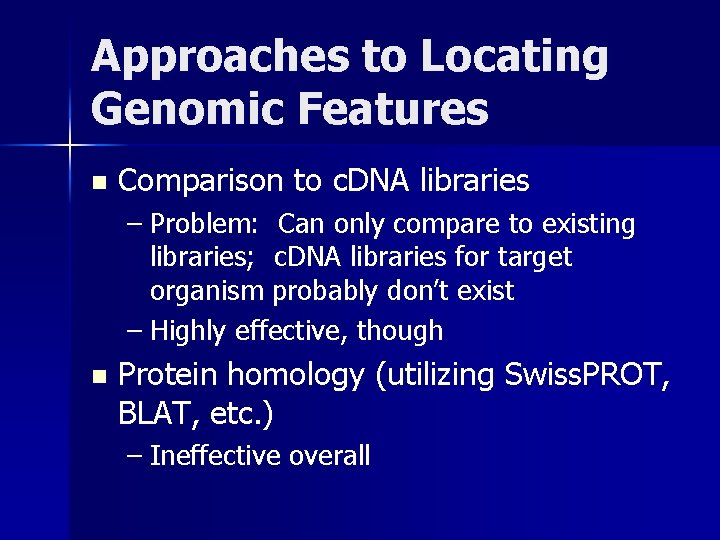
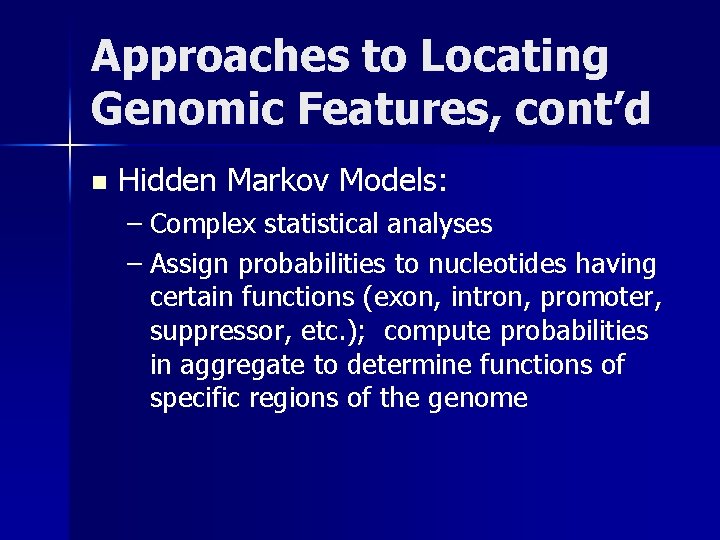
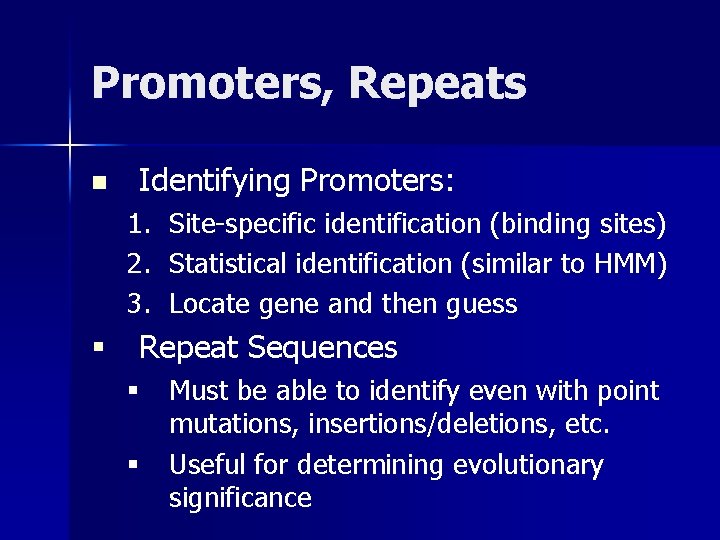
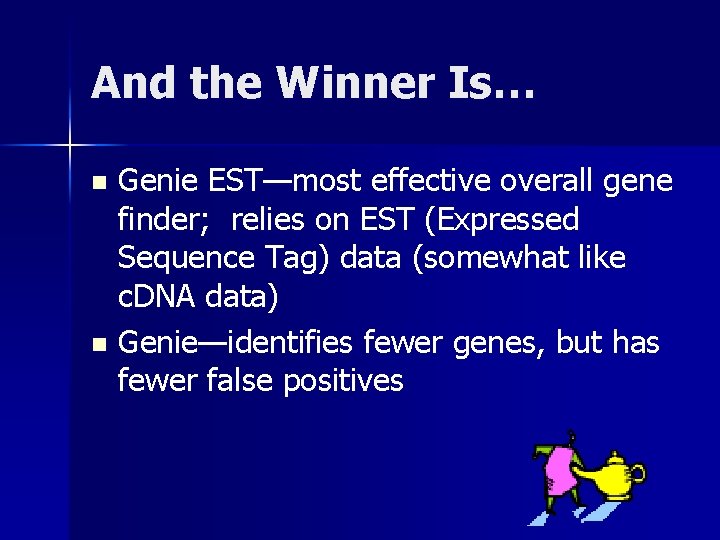
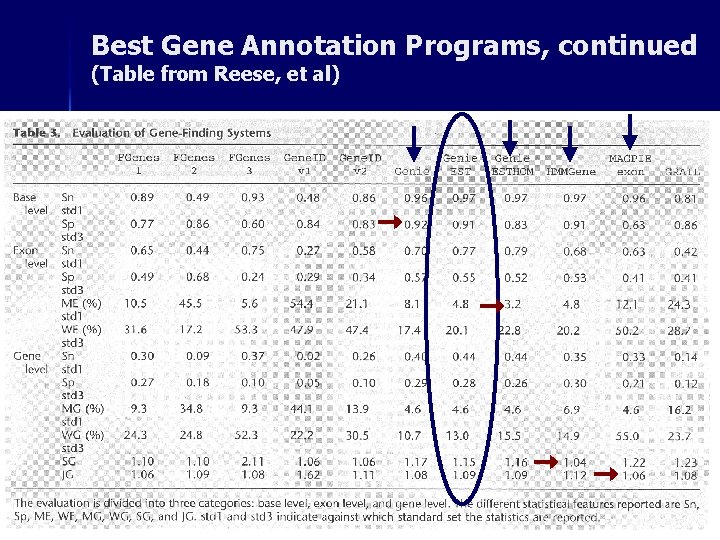
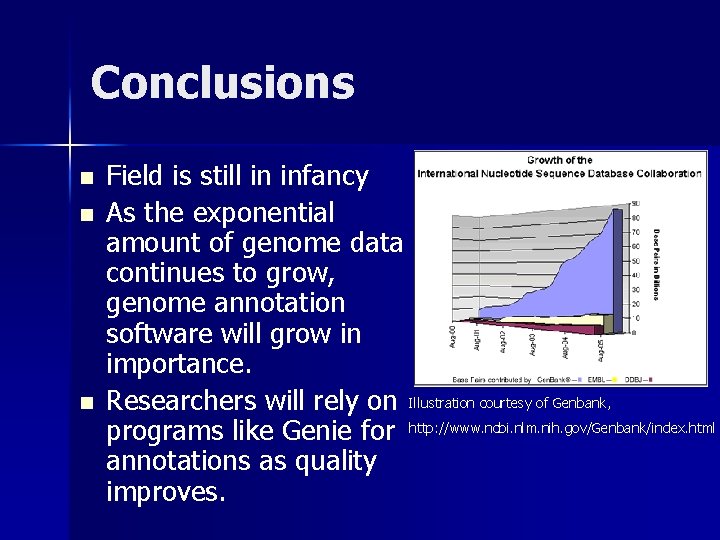
- Slides: 11
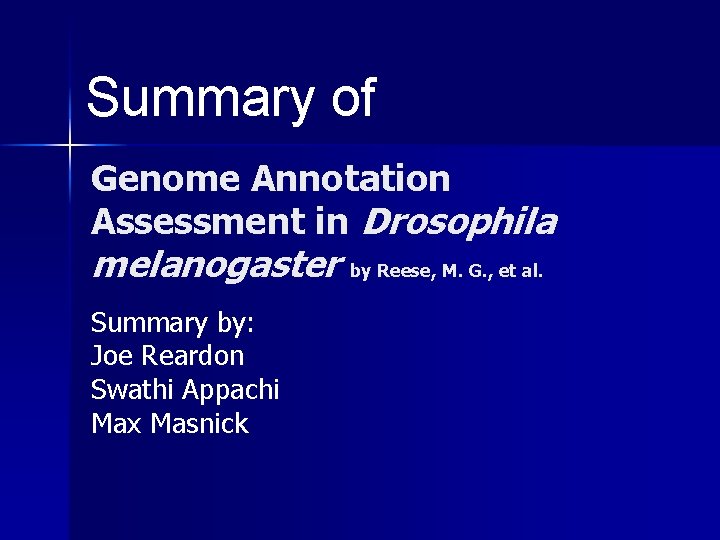
Summary of Genome Annotation Assessment in Drosophila melanogaster by Reese, M. G. , et al. Summary by: Joe Reardon Swathi Appachi Max Masnick
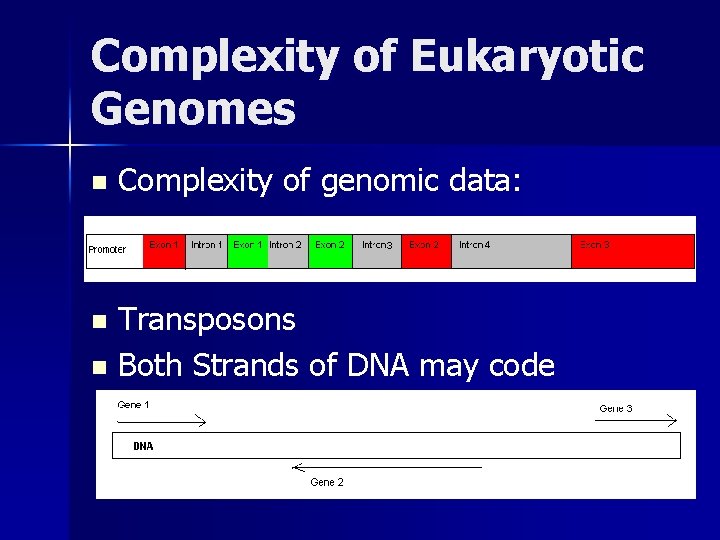
Complexity of Eukaryotic Genomes n Complexity of genomic data: Transposons n Both Strands of DNA may code n
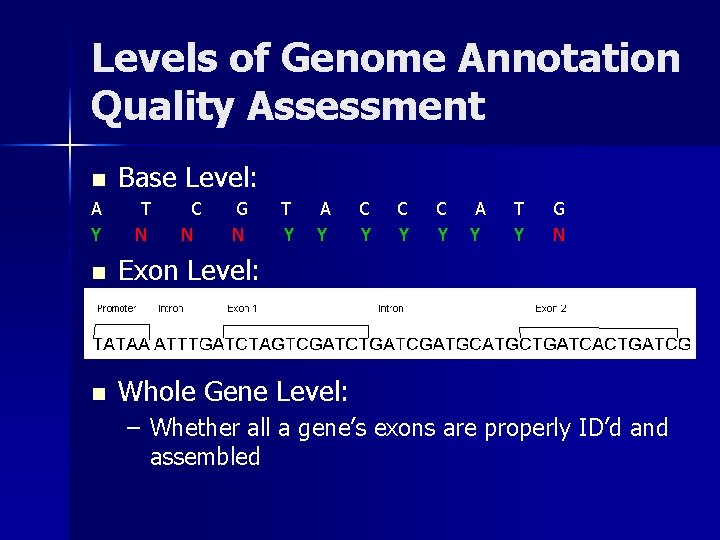
Levels of Genome Annotation Quality Assessment n A Y Base Level: T N C N G N T Y A Y n Exon Level: n Whole Gene Level: C Y C Y A Y T Y G N – Whether all a gene’s exons are properly ID’d and assembled
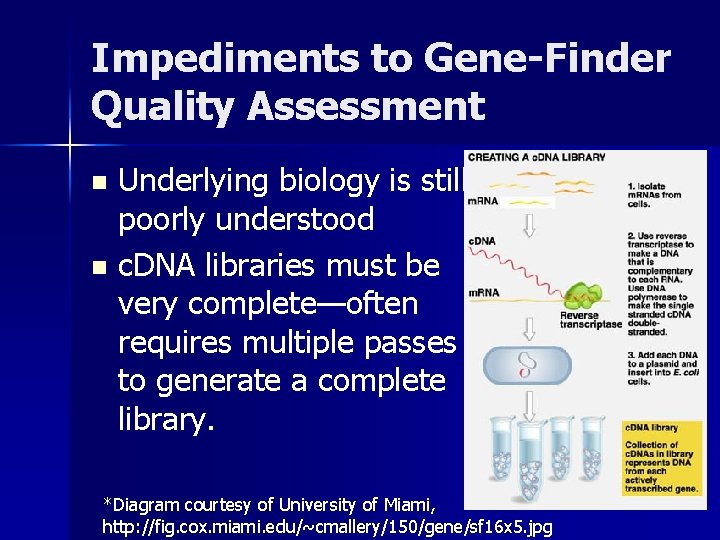
Impediments to Gene-Finder Quality Assessment Underlying biology is still poorly understood n c. DNA libraries must be very complete—often requires multiple passes to generate a complete library. n *Diagram courtesy of University of Miami, http: //fig. cox. miami. edu/~cmallery/150/gene/sf 16 x 5. jpg
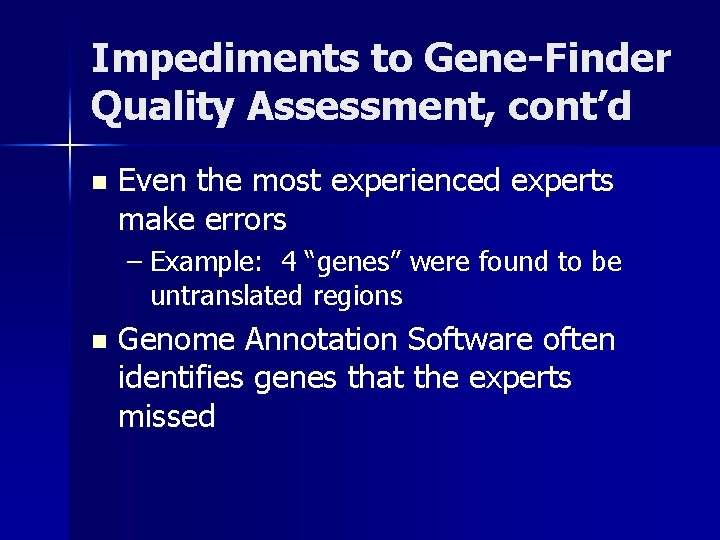
Impediments to Gene-Finder Quality Assessment, cont’d n Even the most experienced experts make errors – Example: 4 “genes” were found to be untranslated regions n Genome Annotation Software often identifies genes that the experts missed
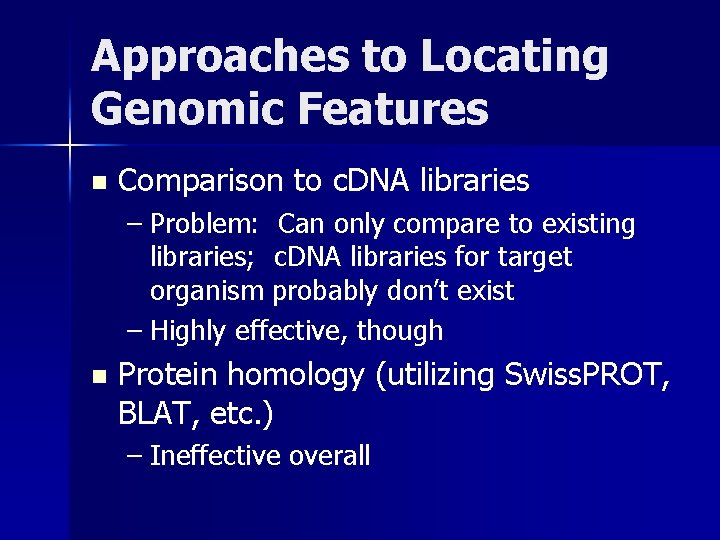
Approaches to Locating Genomic Features n Comparison to c. DNA libraries – Problem: Can only compare to existing libraries; c. DNA libraries for target organism probably don’t exist – Highly effective, though n Protein homology (utilizing Swiss. PROT, BLAT, etc. ) – Ineffective overall
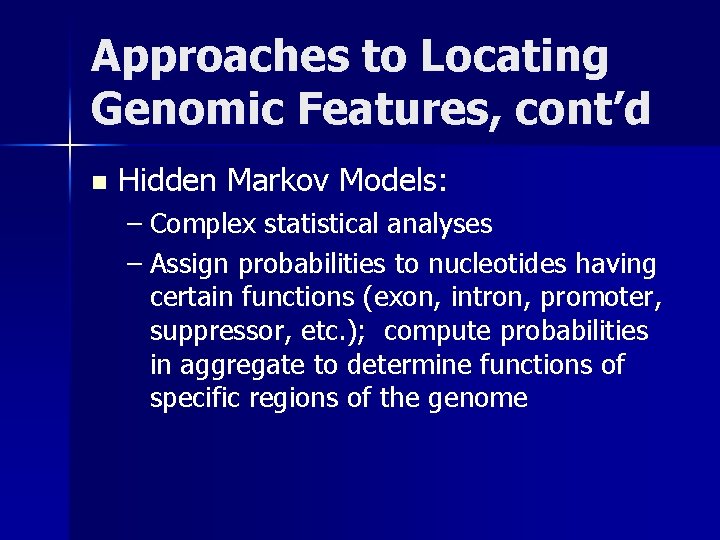
Approaches to Locating Genomic Features, cont’d n Hidden Markov Models: – Complex statistical analyses – Assign probabilities to nucleotides having certain functions (exon, intron, promoter, suppressor, etc. ); compute probabilities in aggregate to determine functions of specific regions of the genome
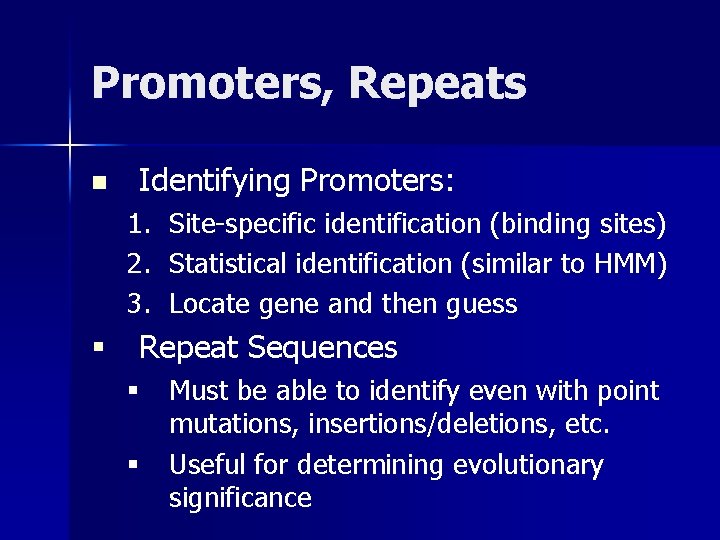
Promoters, Repeats n Identifying Promoters: 1. Site-specific identification (binding sites) 2. Statistical identification (similar to HMM) 3. Locate gene and then guess § Repeat Sequences § Must be able to identify even with point mutations, insertions/deletions, etc. § Useful for determining evolutionary significance
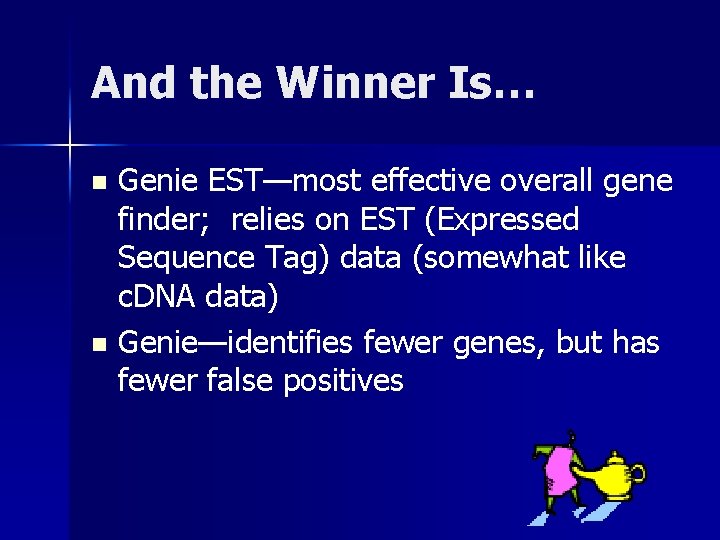
And the Winner Is… Genie EST—most effective overall gene finder; relies on EST (Expressed Sequence Tag) data (somewhat like c. DNA data) n Genie—identifies fewer genes, but has fewer false positives n
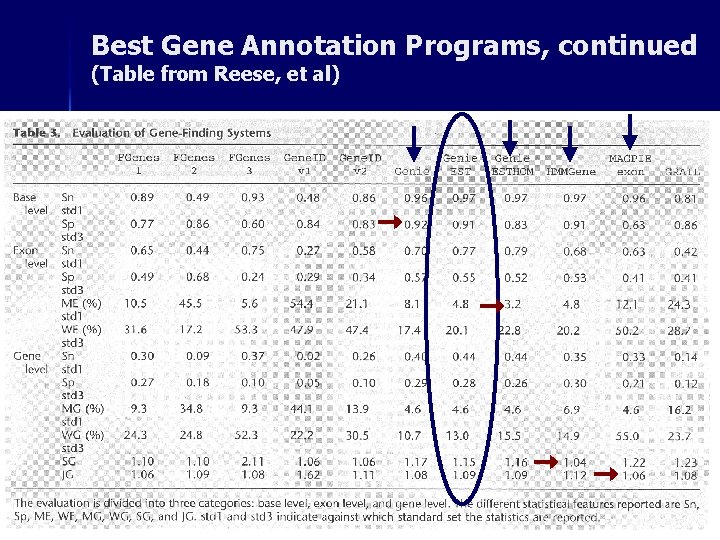
Best Gene Annotation Programs, continued (Table from Reese, et al)
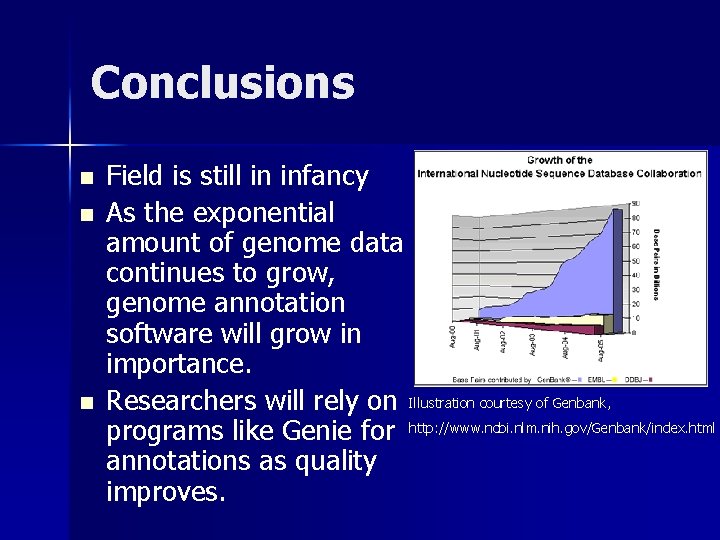
Conclusions n n n Field is still in infancy As the exponential amount of genome data continues to grow, genome annotation software will grow in importance. Researchers will rely on Illustration courtesy of Genbank, programs like Genie for http: //www. ncbi. nlm. nih. gov/Genbank/index. html annotations as quality improves.
Ufpa na drosophila melanogaster a cor do corpo
Mutación por sustitución
Drosophila melanogaster
Teoria cromozomiala a ereditatii
Genome assembly and annotation ppt
Scale annotation pipeline
Tomato genome browser
Genome-to-genome distance calculator
Hox gene mutation in drosophila
Oogenesi
Hox gene mutation in drosophila
Drosophila cinnabar