Summarization and Personal Information Management Carolyn Penstein Ros
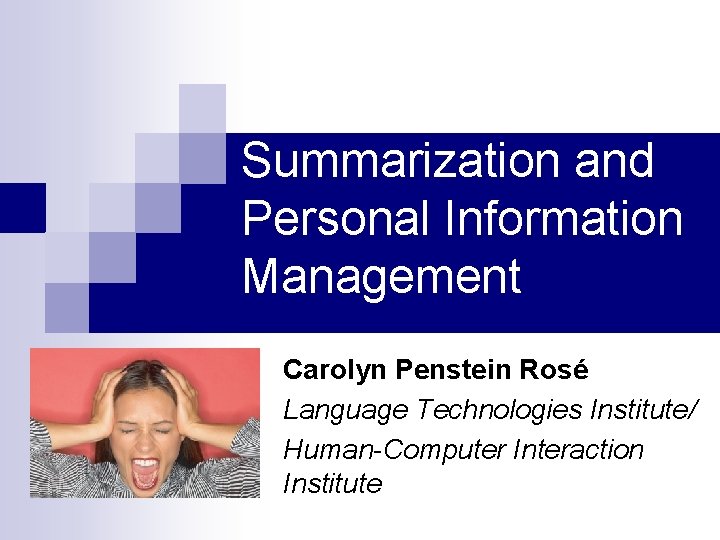
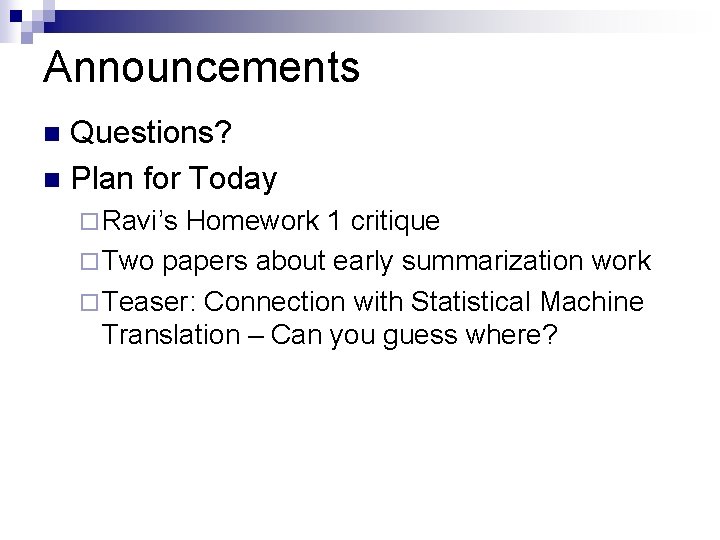
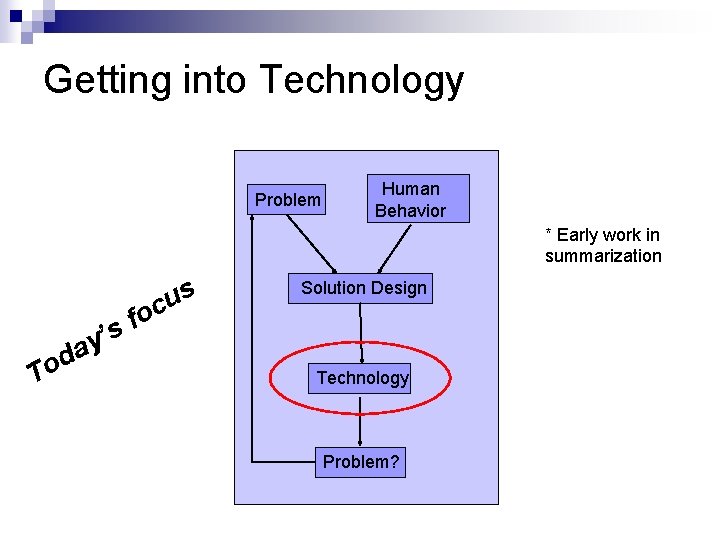
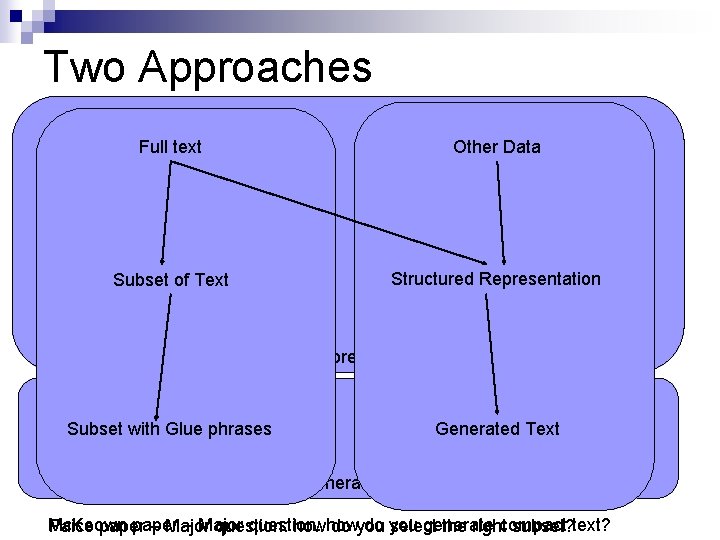
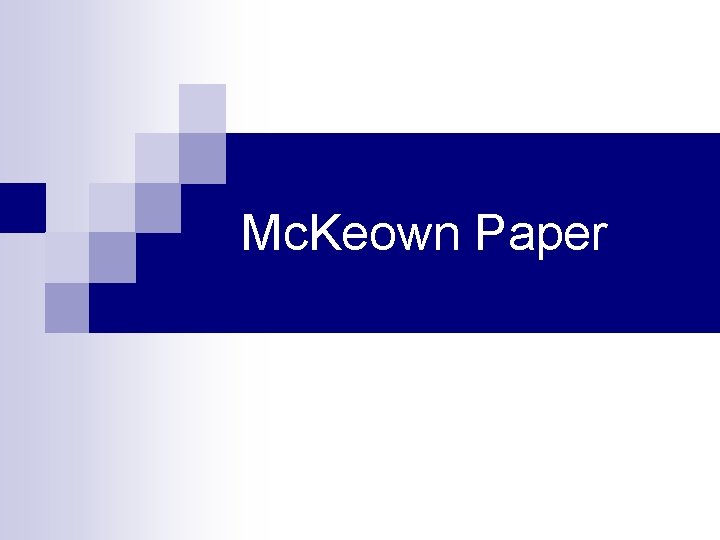
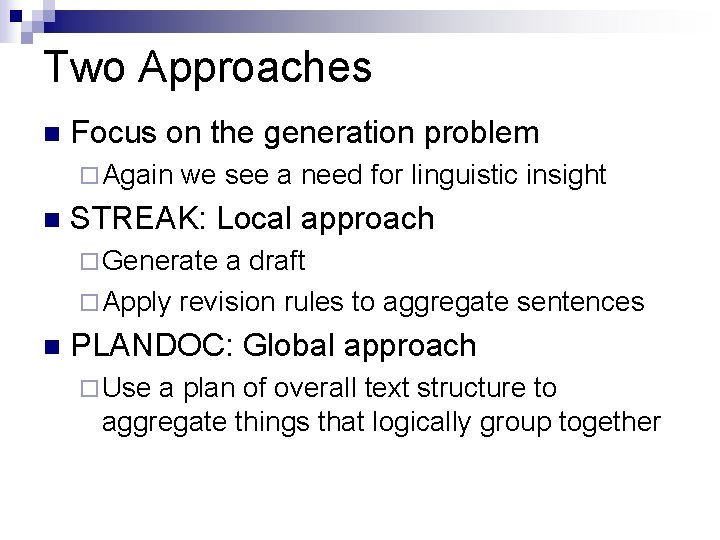
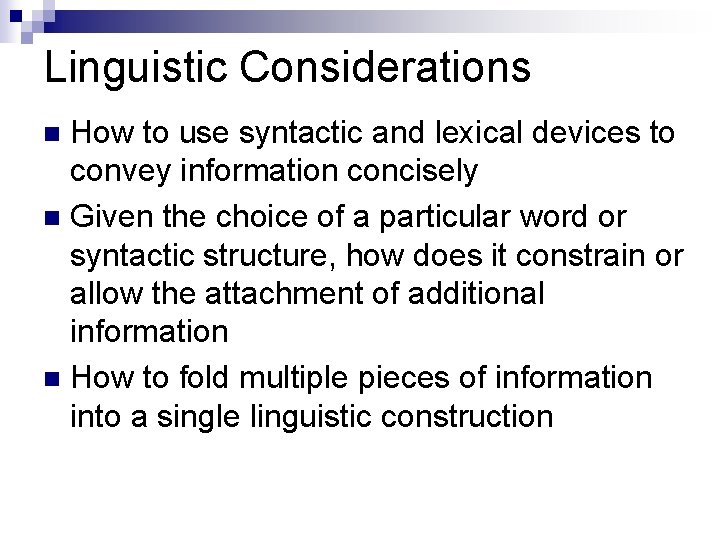
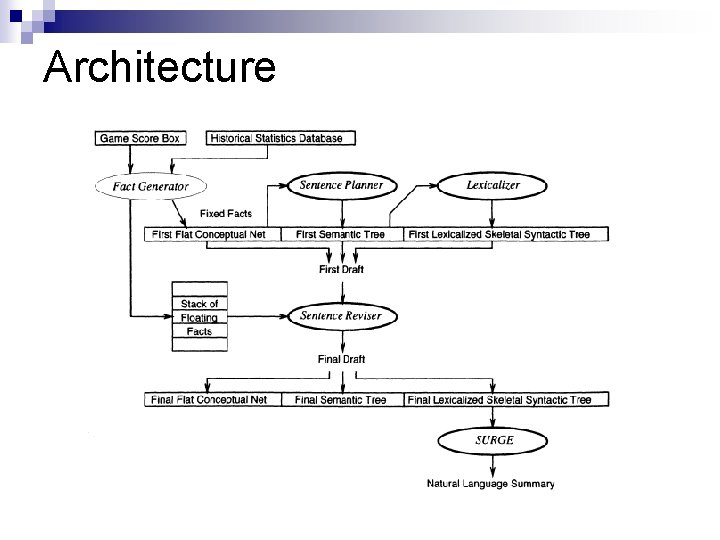
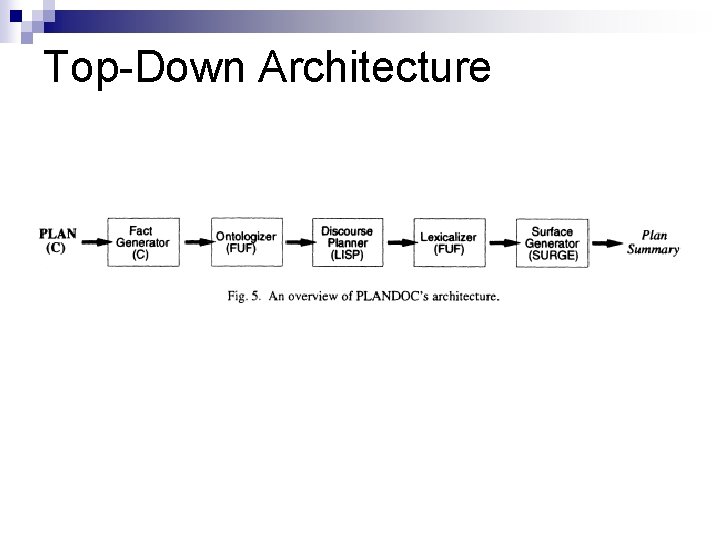
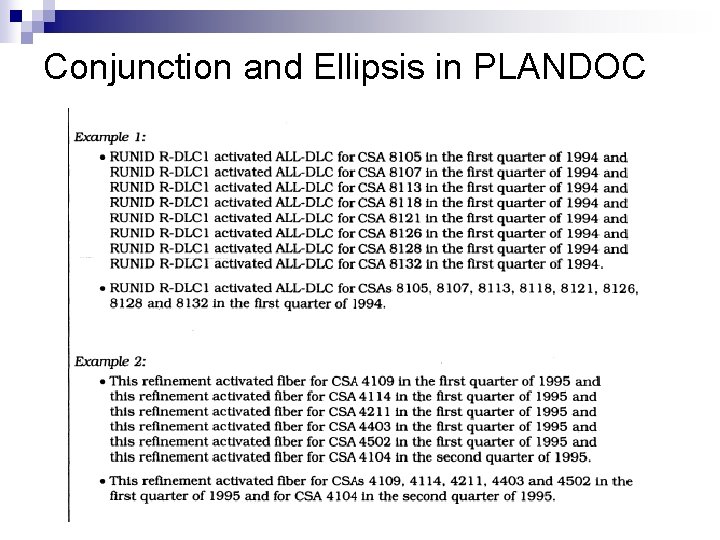
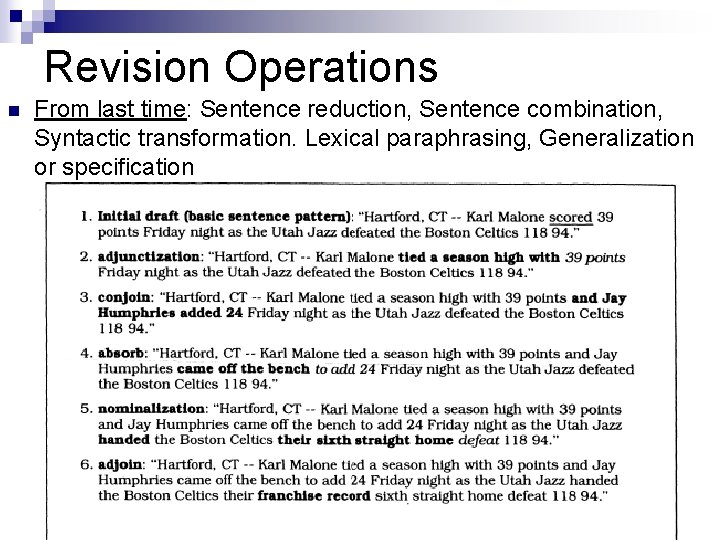
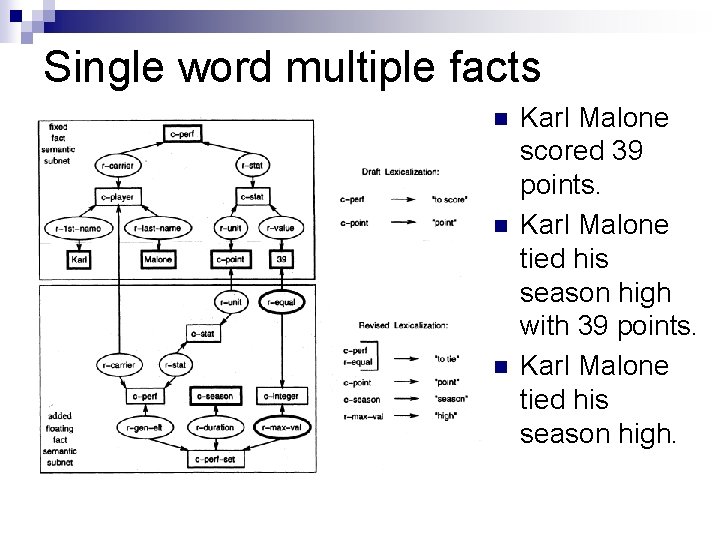
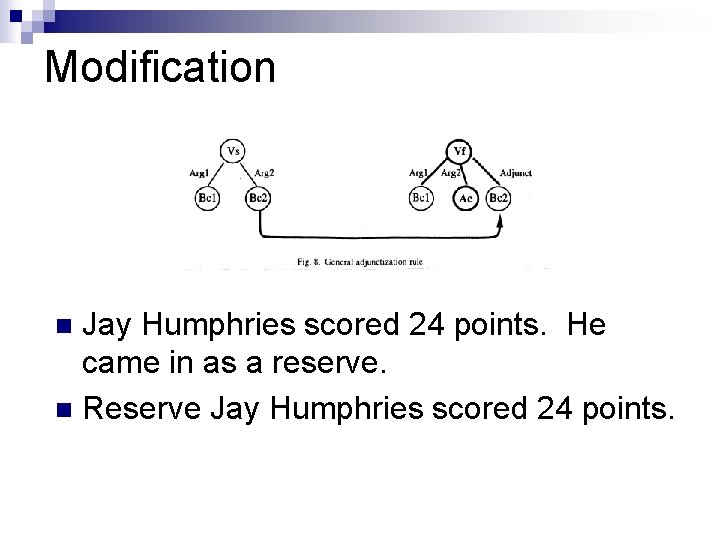
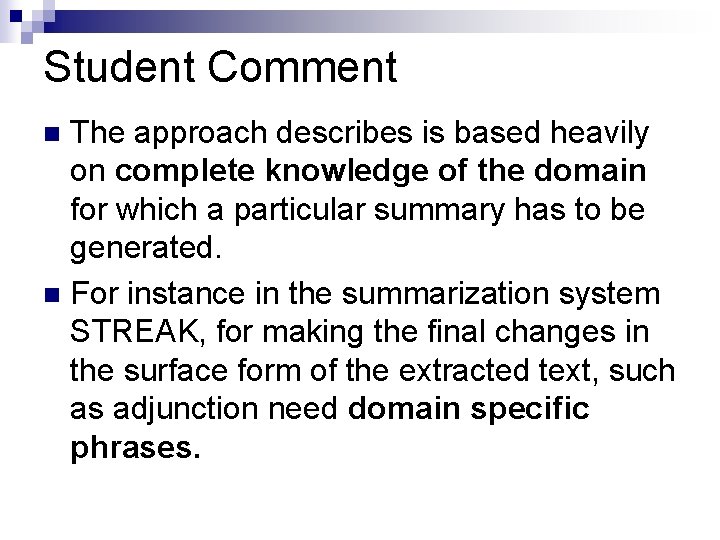
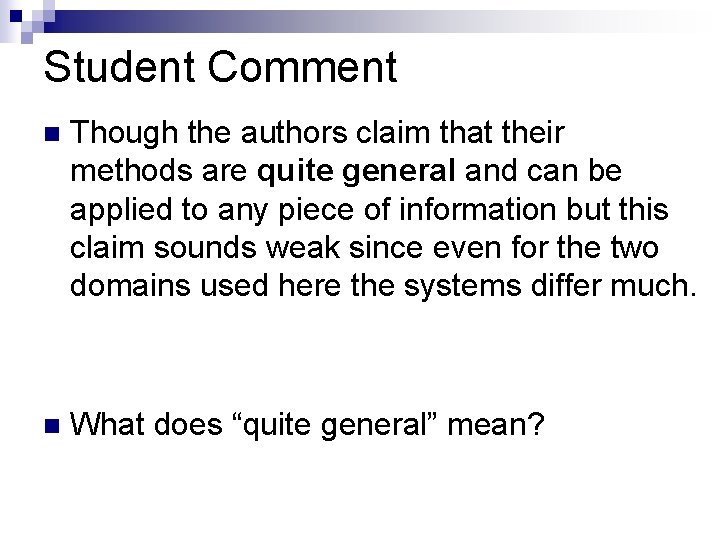
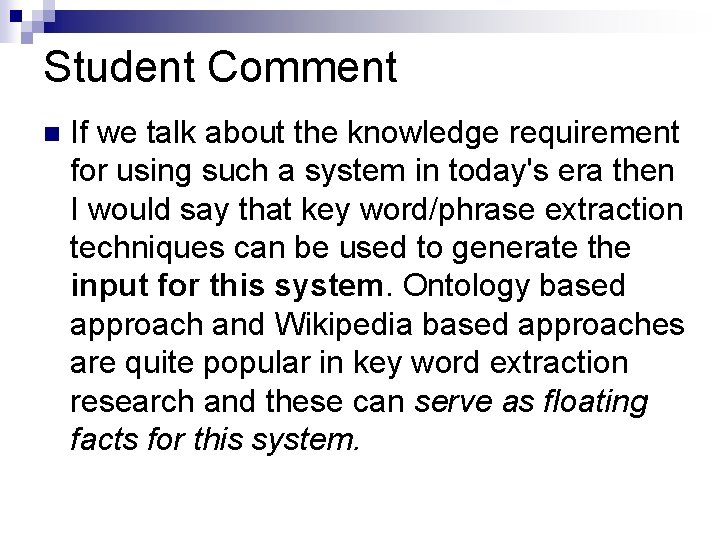
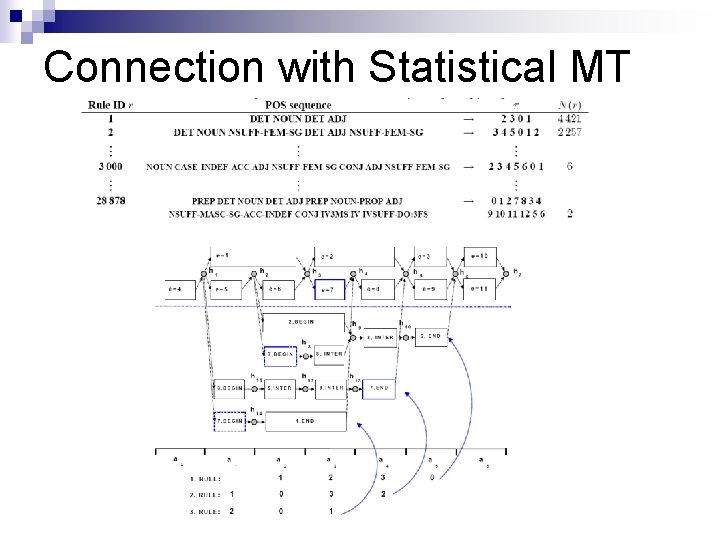
![Tracing the History of the Idea n [AI Planning] Chapman 1987: Partial Order Causal Tracing the History of the Idea n [AI Planning] Chapman 1987: Partial Order Causal](https://slidetodoc.com/presentation_image/871600129c11e575e377c2864b8b7c1a/image-18.jpg)
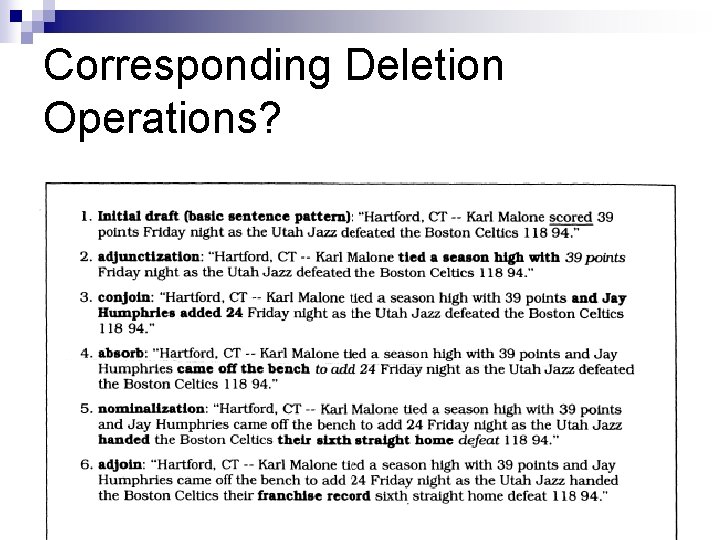
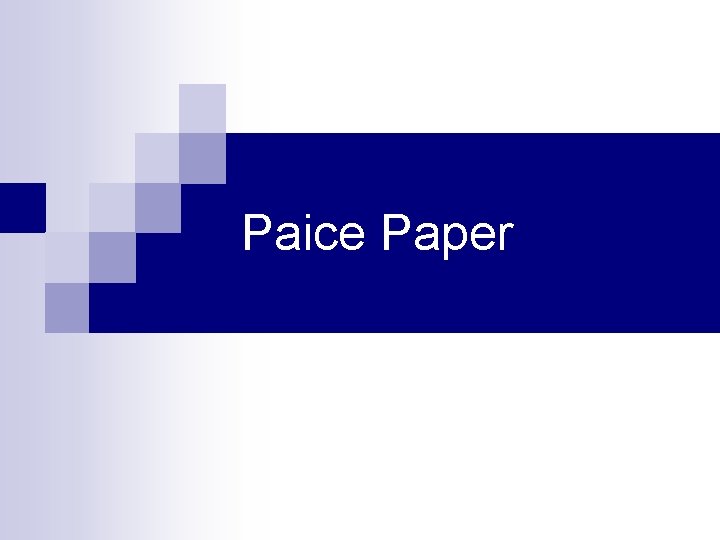
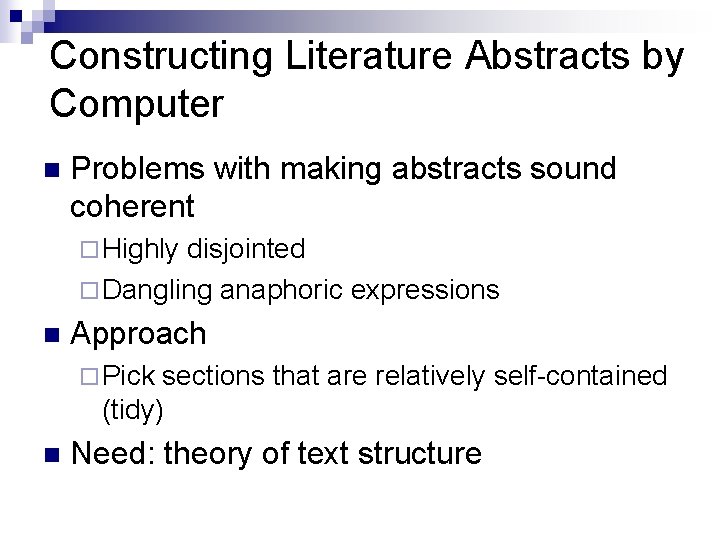
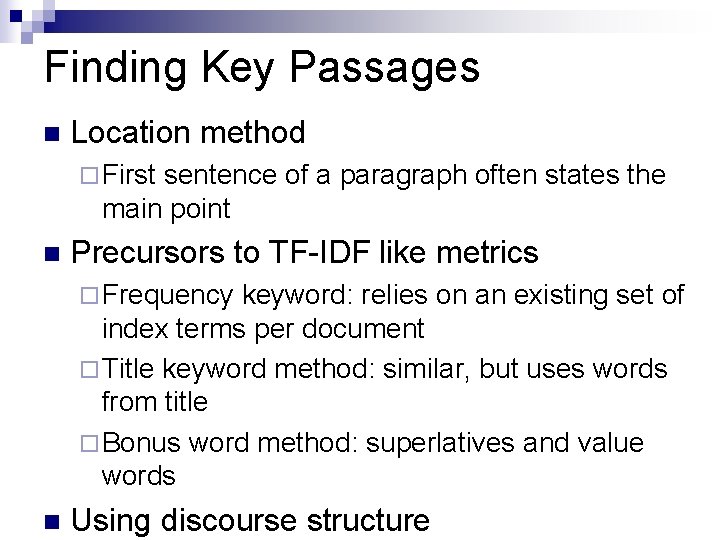
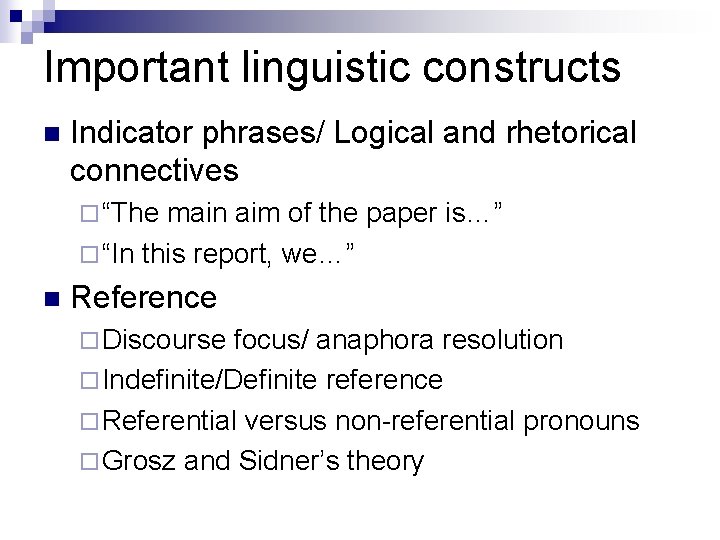
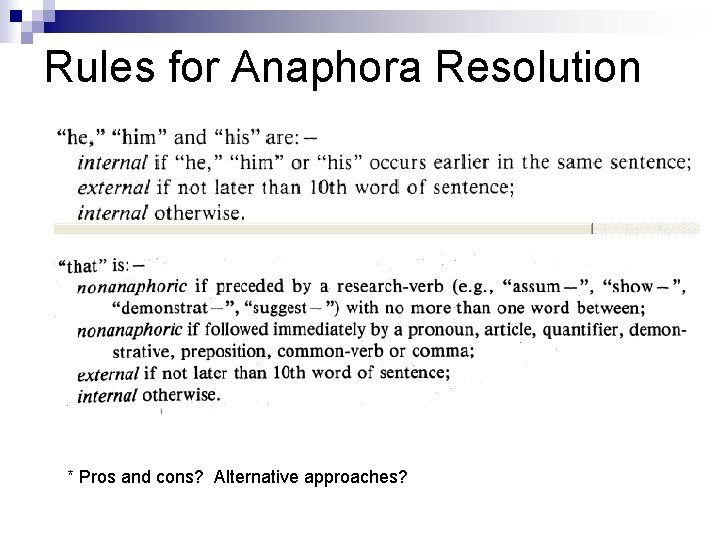
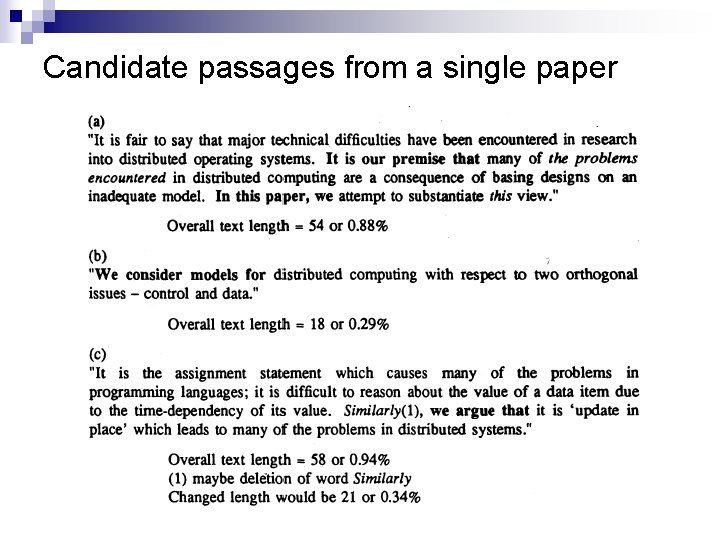
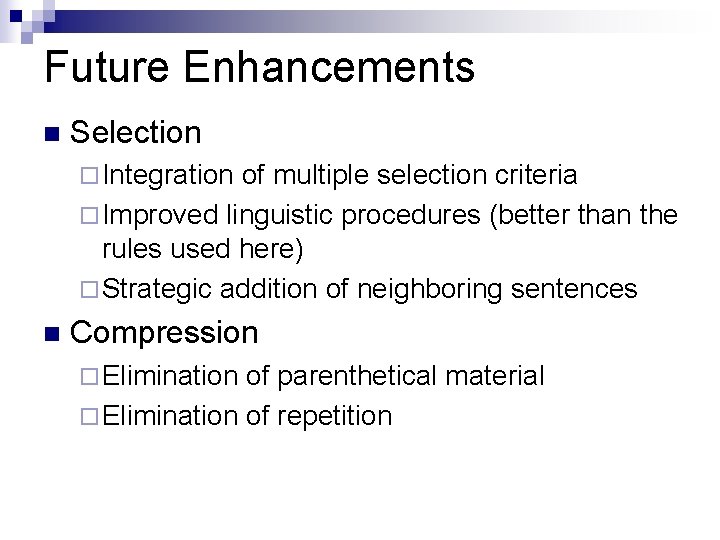
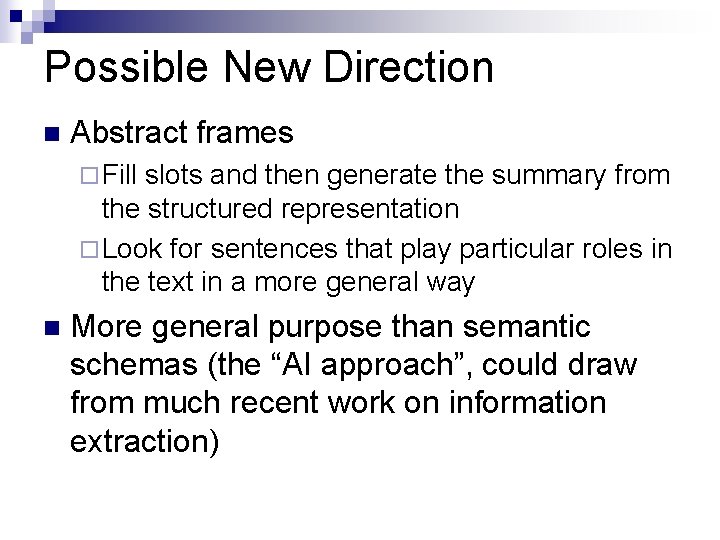
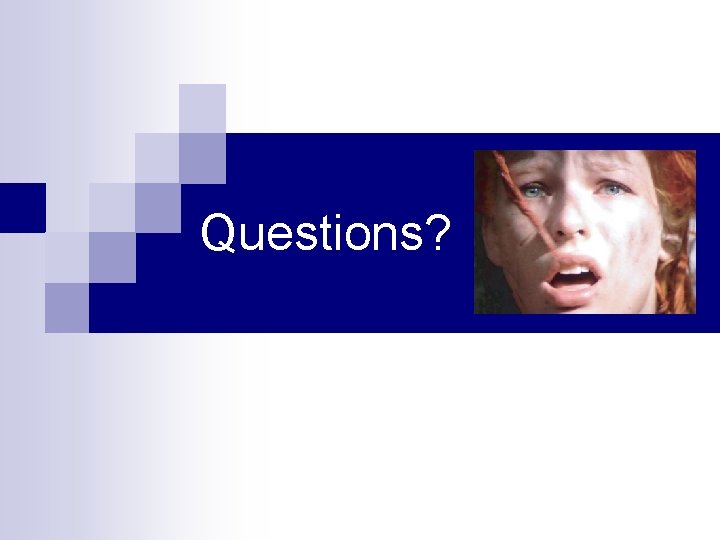
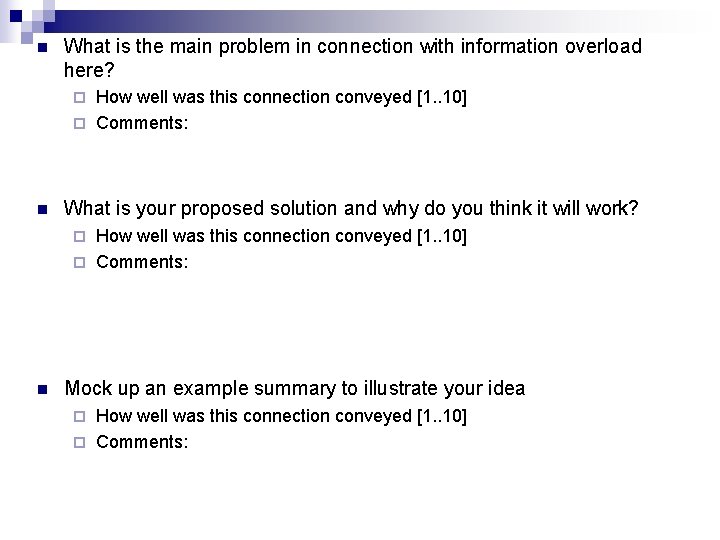
- Slides: 29
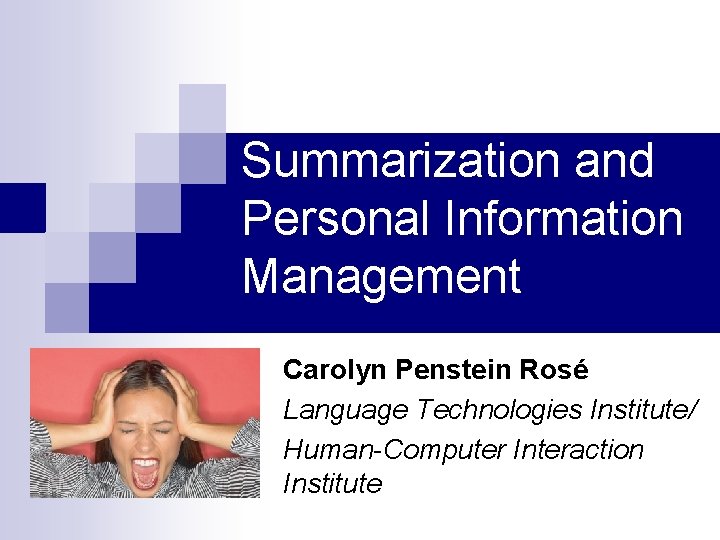
Summarization and Personal Information Management Carolyn Penstein Rosé Language Technologies Institute/ Human-Computer Interaction Institute
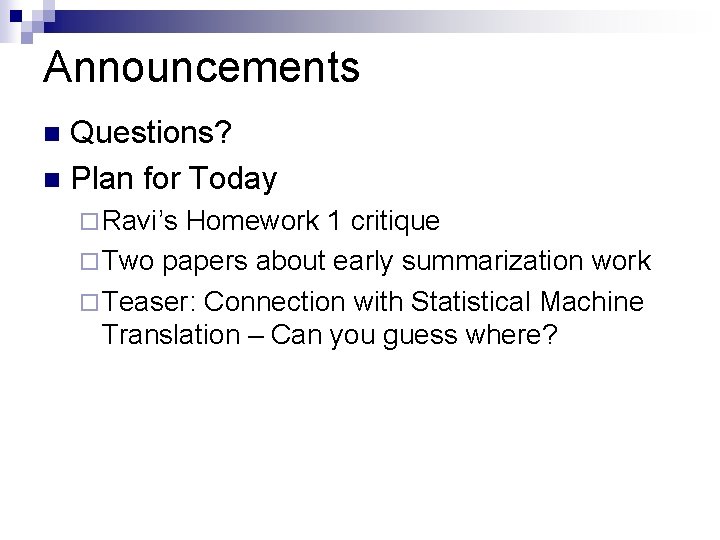
Announcements Questions? n Plan for Today n ¨ Ravi’s Homework 1 critique ¨ Two papers about early summarization work ¨ Teaser: Connection with Statistical Machine Translation – Can you guess where?
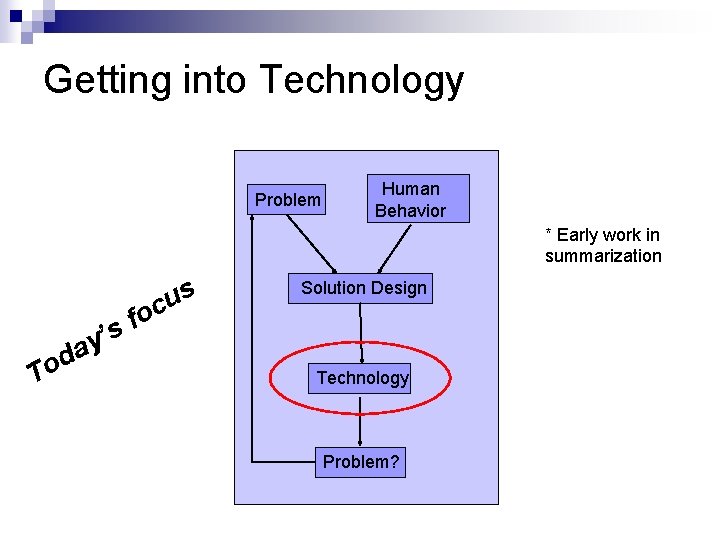
Getting into Technology Problem Human Behavior * Early work in summarization s u c Solution Design o f s To y’ a d Technology Problem?
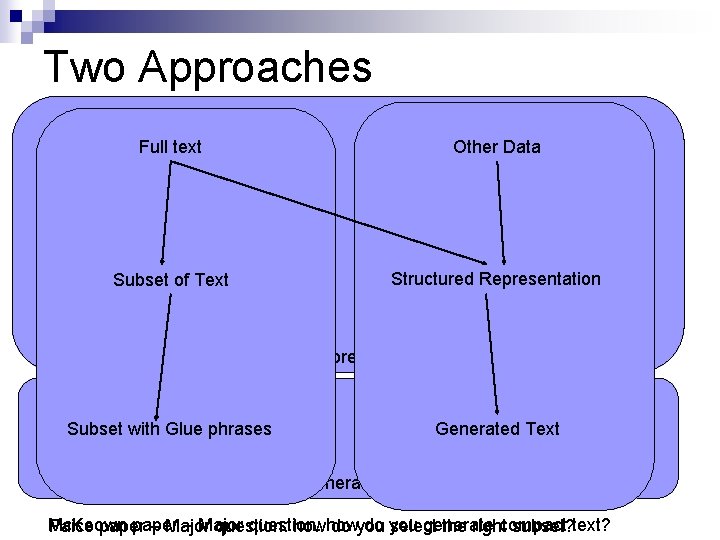
Two Approaches Full text Other Data Subset of Text Structured Representation Interpretation Subset with Glue phrases Generated Text Generation Mc. Keown paper – Major question: do you generate Paice paper – Major question: howhow do you select the rightcompact subset? text?
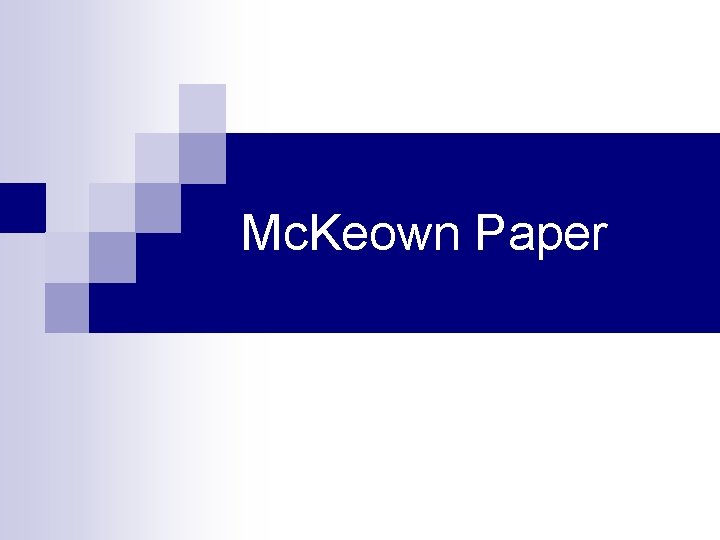
Mc. Keown Paper
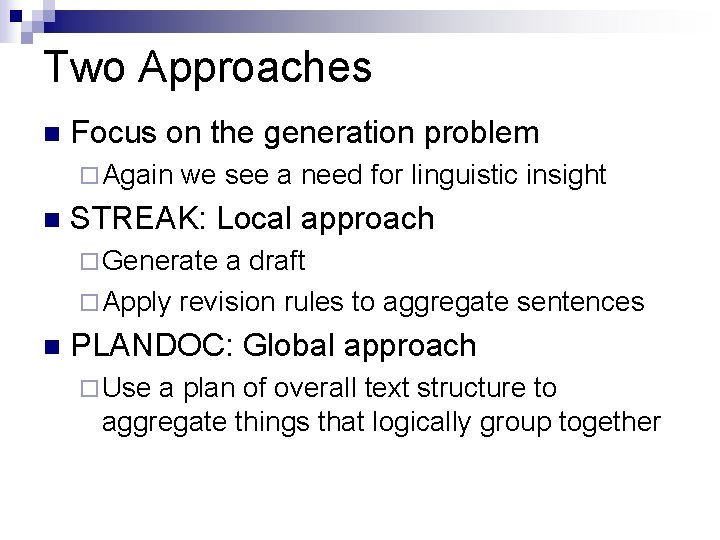
Two Approaches n Focus on the generation problem ¨ Again n we see a need for linguistic insight STREAK: Local approach ¨ Generate a draft ¨ Apply revision rules to aggregate sentences n PLANDOC: Global approach ¨ Use a plan of overall text structure to aggregate things that logically group together
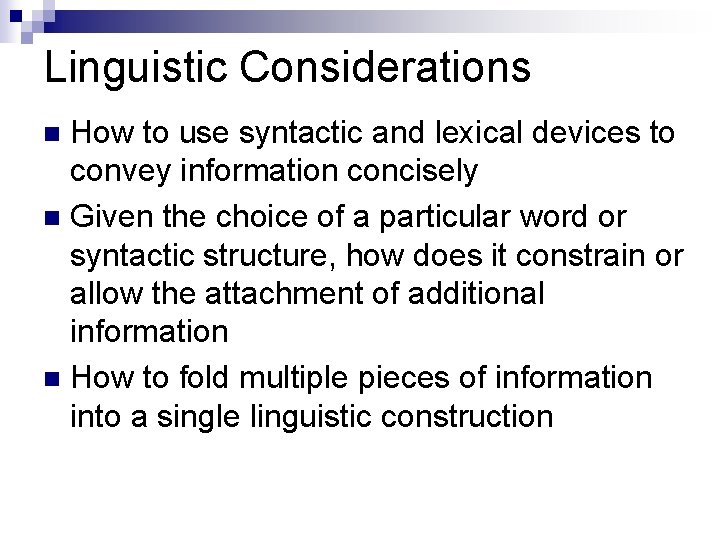
Linguistic Considerations How to use syntactic and lexical devices to convey information concisely n Given the choice of a particular word or syntactic structure, how does it constrain or allow the attachment of additional information n How to fold multiple pieces of information into a single linguistic construction n
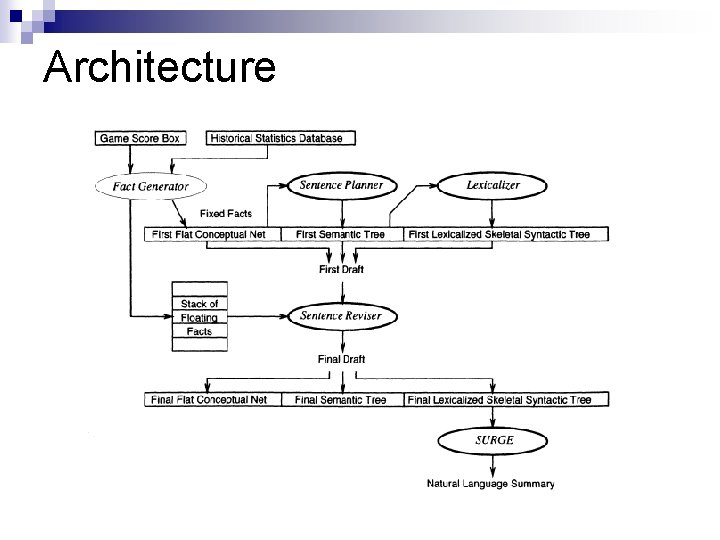
Architecture
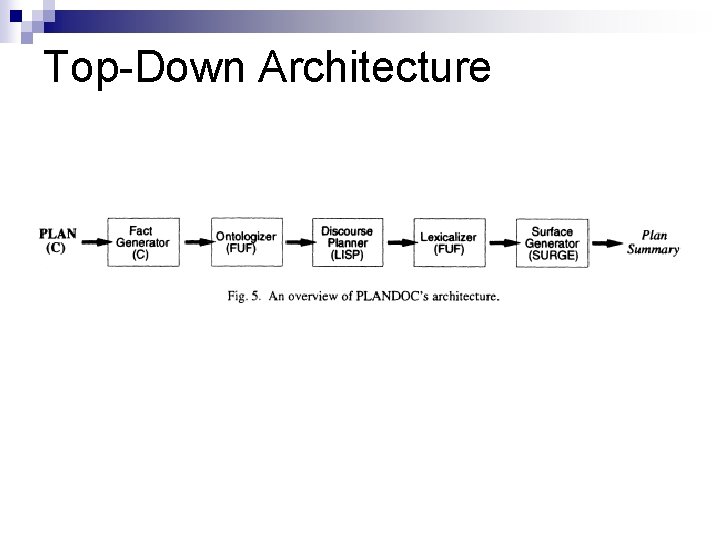
Top-Down Architecture
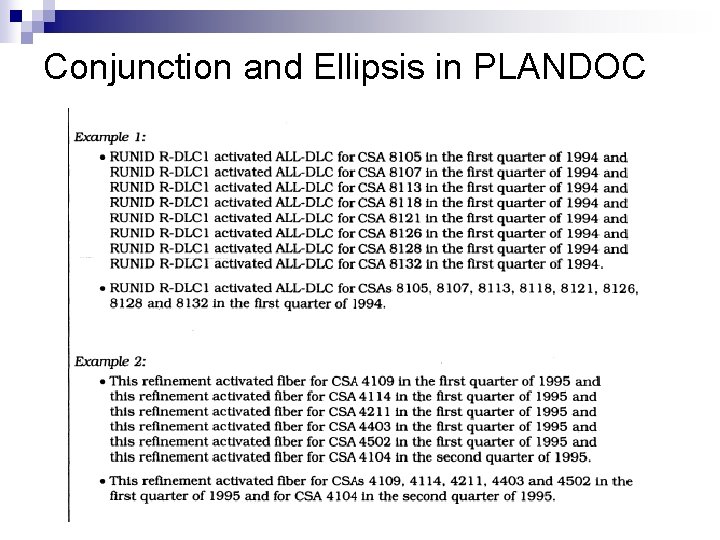
Conjunction and Ellipsis in PLANDOC
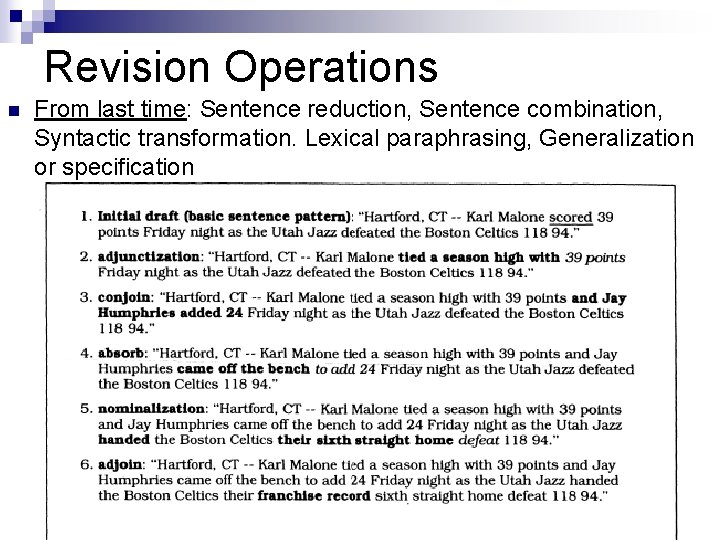
Revision Operations n From last time: Sentence reduction, Sentence combination, Syntactic transformation. Lexical paraphrasing, Generalization or specification
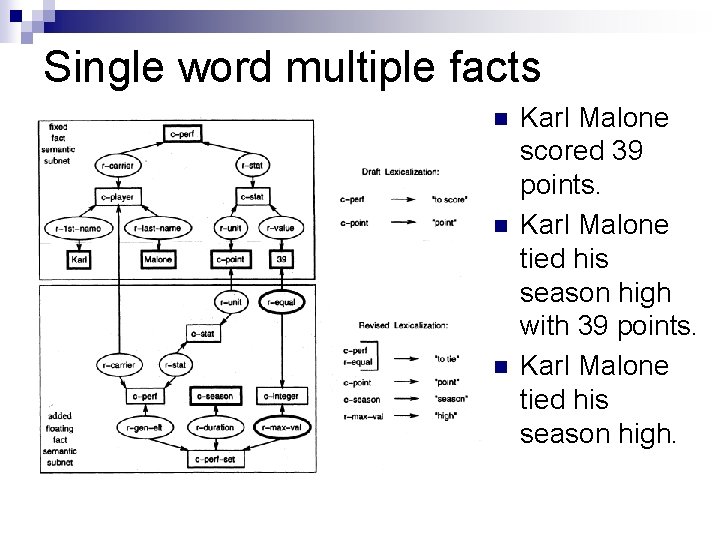
Single word multiple facts n n n Karl Malone scored 39 points. Karl Malone tied his season high with 39 points. Karl Malone tied his season high.
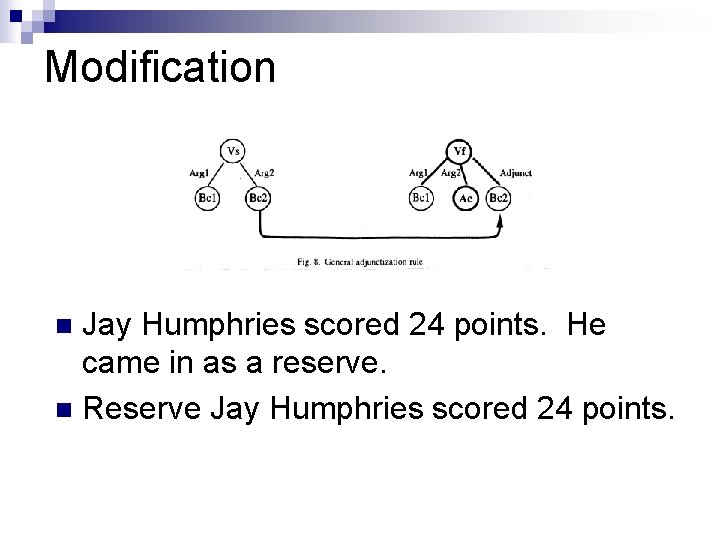
Modification Jay Humphries scored 24 points. He came in as a reserve. n Reserve Jay Humphries scored 24 points. n
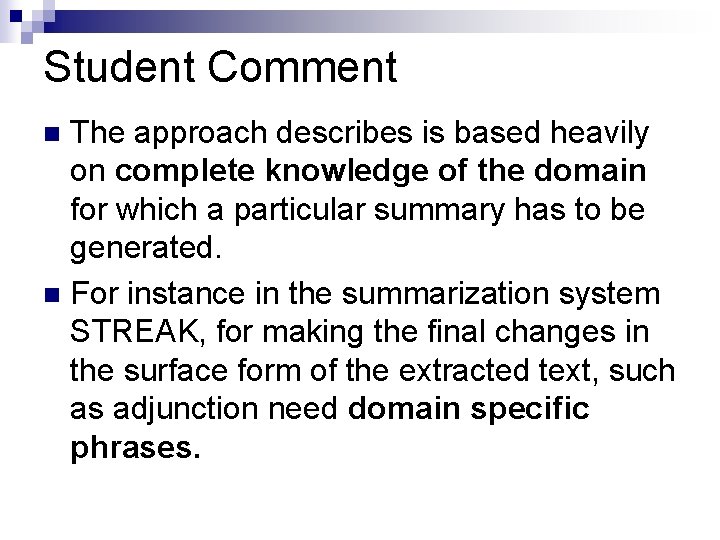
Student Comment The approach describes is based heavily on complete knowledge of the domain for which a particular summary has to be generated. n For instance in the summarization system STREAK, for making the final changes in the surface form of the extracted text, such as adjunction need domain specific phrases. n
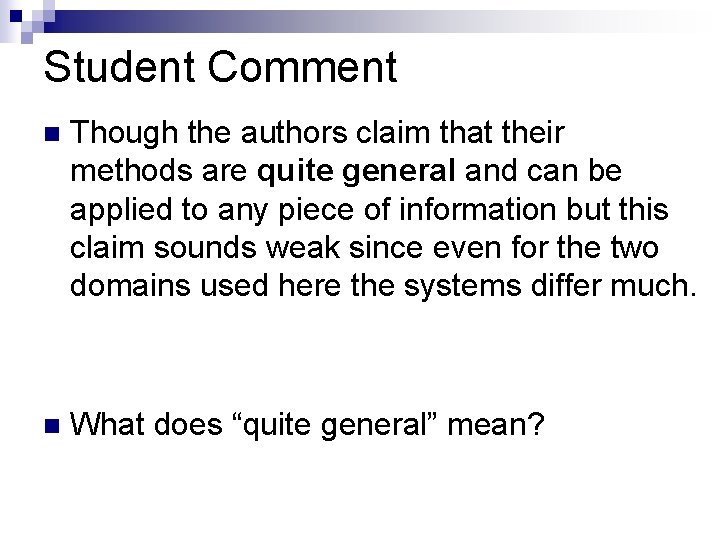
Student Comment n Though the authors claim that their methods are quite general and can be applied to any piece of information but this claim sounds weak since even for the two domains used here the systems differ much. n What does “quite general” mean?
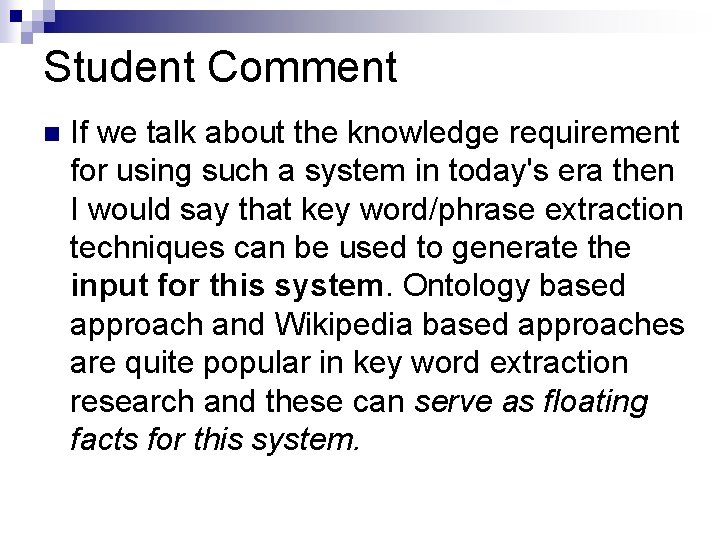
Student Comment n If we talk about the knowledge requirement for using such a system in today's era then I would say that key word/phrase extraction techniques can be used to generate the input for this system. Ontology based approach and Wikipedia based approaches are quite popular in key word extraction research and these can serve as floating facts for this system.
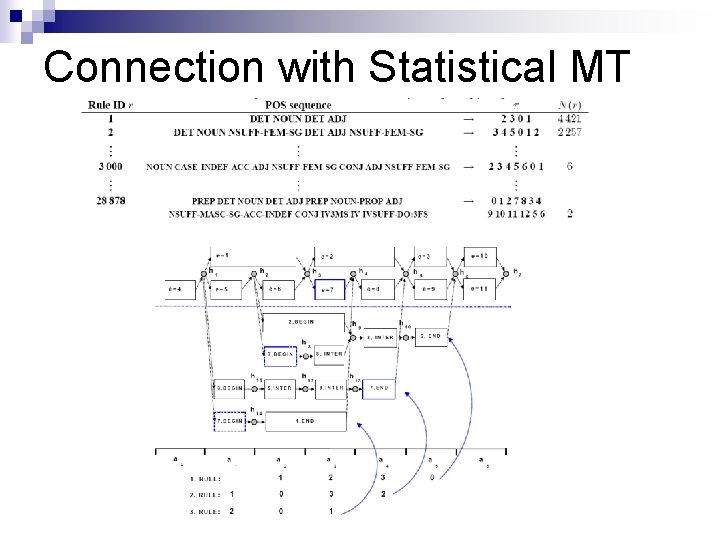
Connection with Statistical MT
![Tracing the History of the Idea n AI Planning Chapman 1987 Partial Order Causal Tracing the History of the Idea n [AI Planning] Chapman 1987: Partial Order Causal](https://slidetodoc.com/presentation_image/871600129c11e575e377c2864b8b7c1a/image-18.jpg)
Tracing the History of the Idea n [AI Planning] Chapman 1987: Partial Order Causal Link Planning (TWEAK) ¨ Plans are partially ordered graphs ¨ Threats mark weaknesses in the plan ¨ Tweak rules operate on plans to remove threats n n n [Summarization] Mc. Keown et al. 1995: Modification rules for generation in a summarization system [Statistical MT] Och & Ney 2004: Distortion rules for statistical MT [Compression] You 2010? ? ? : application of Mc. Keown’s edit rules to statistical compression
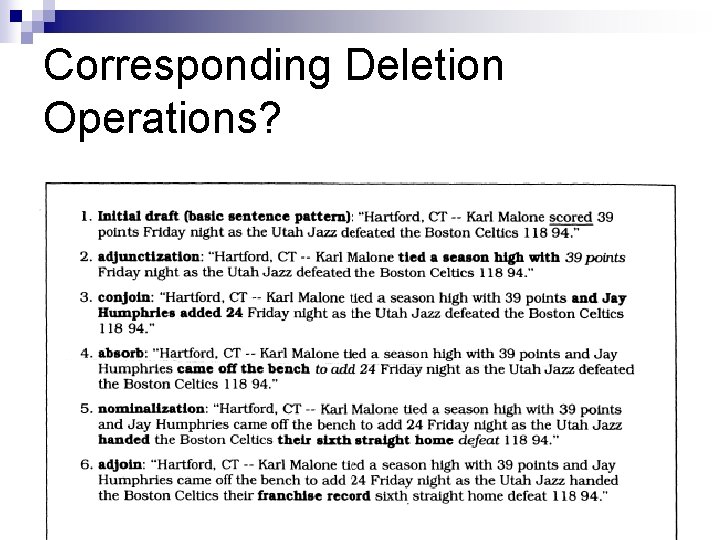
Corresponding Deletion Operations?
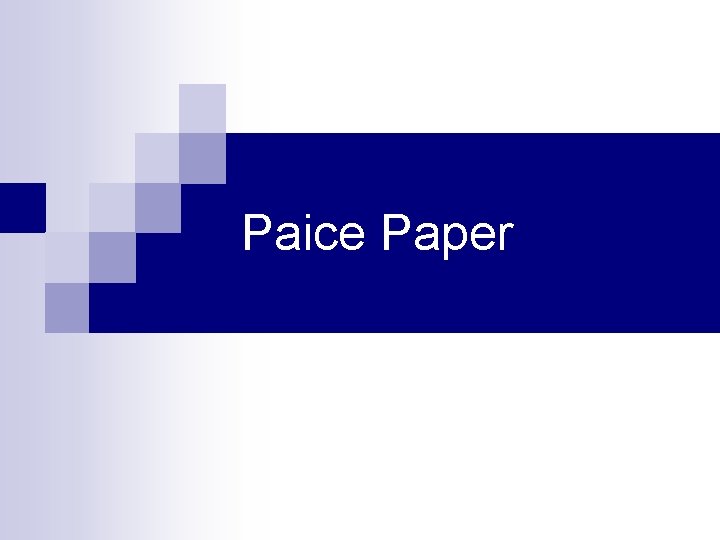
Paice Paper
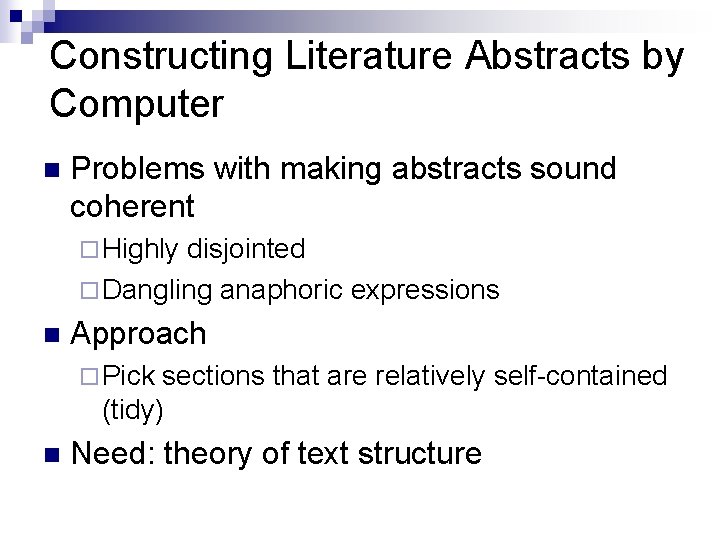
Constructing Literature Abstracts by Computer n Problems with making abstracts sound coherent ¨ Highly disjointed ¨ Dangling anaphoric expressions n Approach ¨ Pick sections that are relatively self-contained (tidy) n Need: theory of text structure
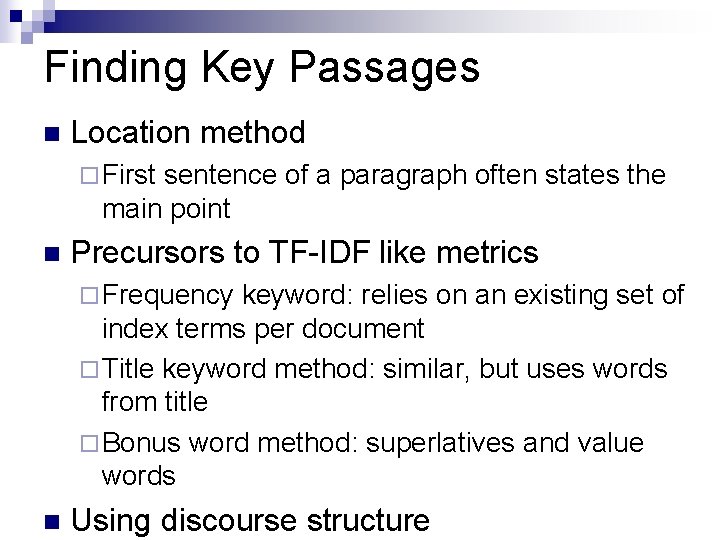
Finding Key Passages n Location method ¨ First sentence of a paragraph often states the main point n Precursors to TF-IDF like metrics ¨ Frequency keyword: relies on an existing set of index terms per document ¨ Title keyword method: similar, but uses words from title ¨ Bonus word method: superlatives and value words n Using discourse structure
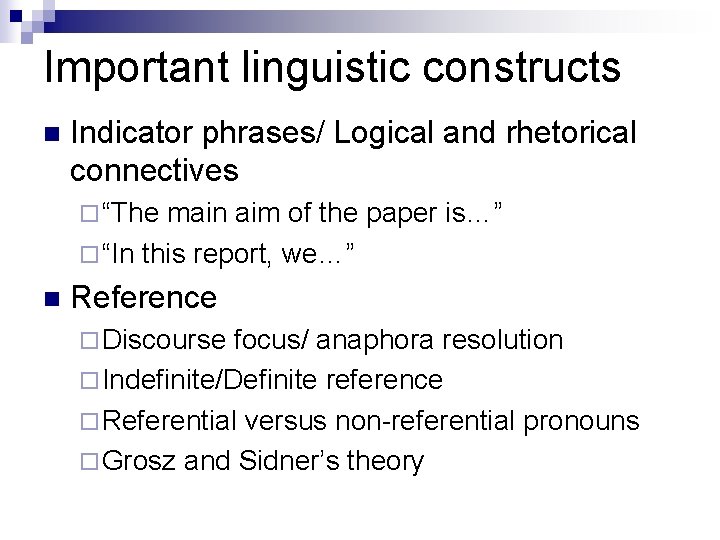
Important linguistic constructs n Indicator phrases/ Logical and rhetorical connectives ¨ “The main aim of the paper is…” ¨ “In this report, we…” n Reference ¨ Discourse focus/ anaphora resolution ¨ Indefinite/Definite reference ¨ Referential versus non-referential pronouns ¨ Grosz and Sidner’s theory
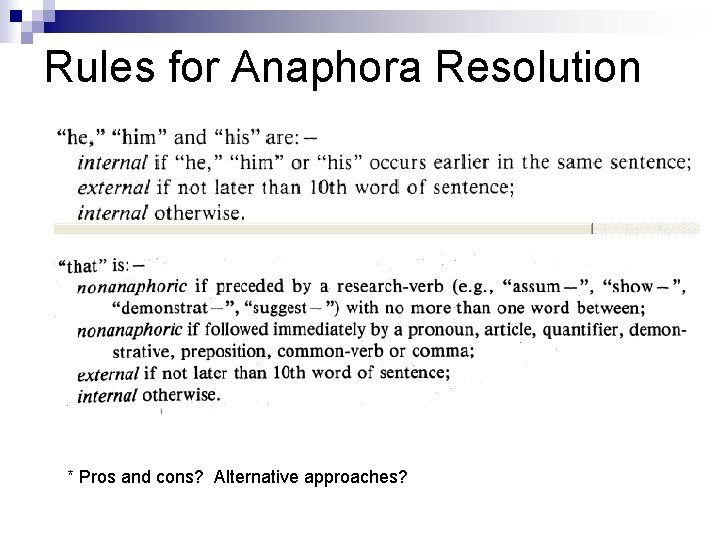
Rules for Anaphora Resolution * Pros and cons? Alternative approaches?
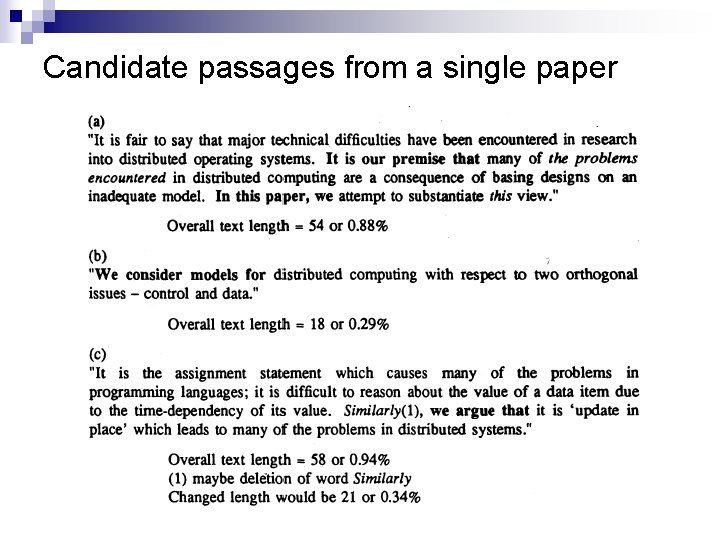
Candidate passages from a single paper
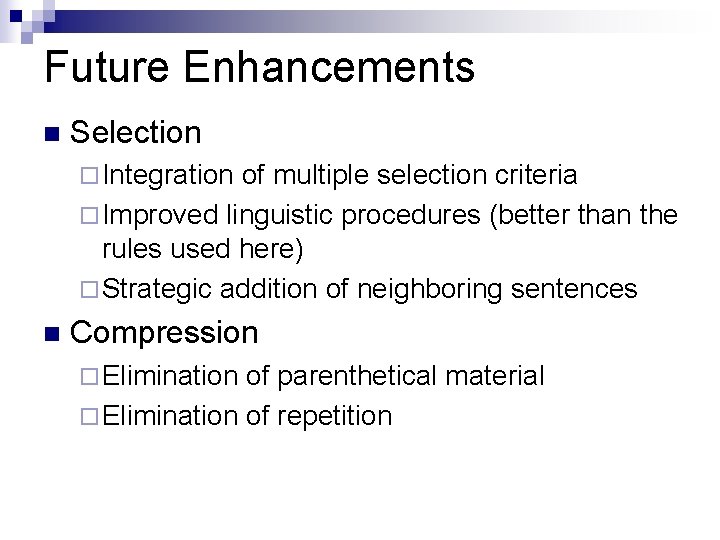
Future Enhancements n Selection ¨ Integration of multiple selection criteria ¨ Improved linguistic procedures (better than the rules used here) ¨ Strategic addition of neighboring sentences n Compression ¨ Elimination of parenthetical material ¨ Elimination of repetition
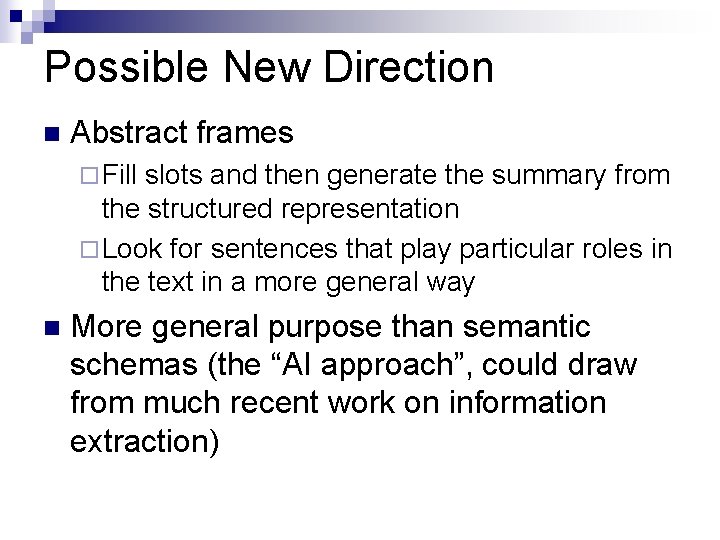
Possible New Direction n Abstract frames ¨ Fill slots and then generate the summary from the structured representation ¨ Look for sentences that play particular roles in the text in a more general way n More general purpose than semantic schemas (the “AI approach”, could draw from much recent work on information extraction)
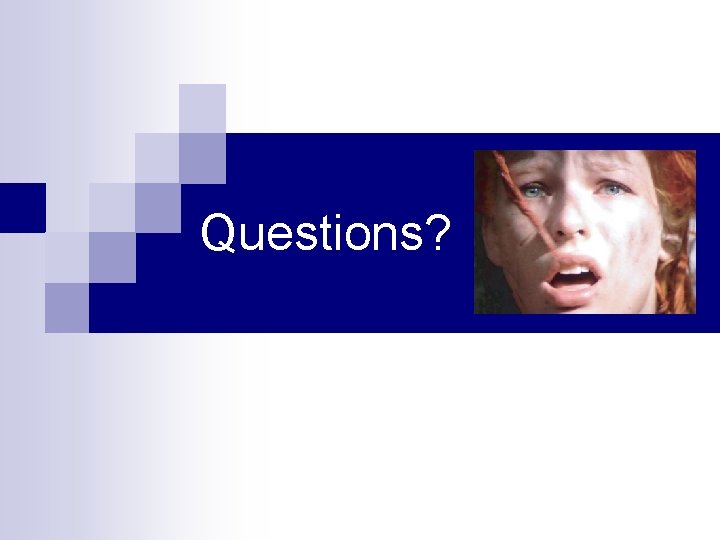
Questions?
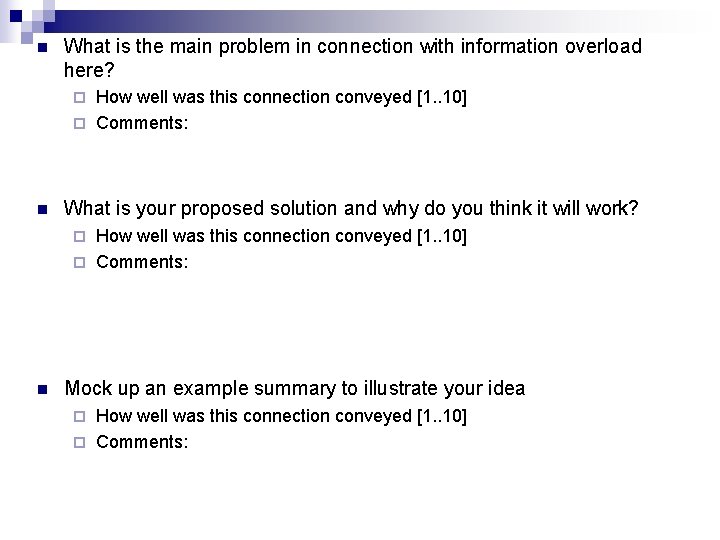
n What is the main problem in connection with information overload here? How well was this connection conveyed [1. . 10] ¨ Comments: ¨ n What is your proposed solution and why do you think it will work? How well was this connection conveyed [1. . 10] ¨ Comments: ¨ n Mock up an example summary to illustrate your idea How well was this connection conveyed [1. . 10] ¨ Comments: ¨
Newtons law review
Entity summarization
Text summarization vietnamese
Medical summaries for law firms
Text summarization vietnamese
Text summarization vietnamese
Abstractive summarization
Carolyn mendiola
Synchronicity market timing
How to tell wild animals book
Carolyn sourek
Carolyn talbot
Carolyn johnston md
Carolyn shread
Carolyn graham jazz chants
Carolyn hanesworth
Stury
Carolyn marano nj
Carolyn brownawell
Carolyn maull
Clive wants to estimate the number of bees in a beehive
Carolyn ells
Carolyn hotchkiss
Upton lake christian school
Carolyn washburn
Carolyn cherry
Carolyn knoepfler
Carolyn saxby facts
Beggs and brill correlation
Ros lidar mapping