Statistics cont Psych 231 Research Methods in Psychology
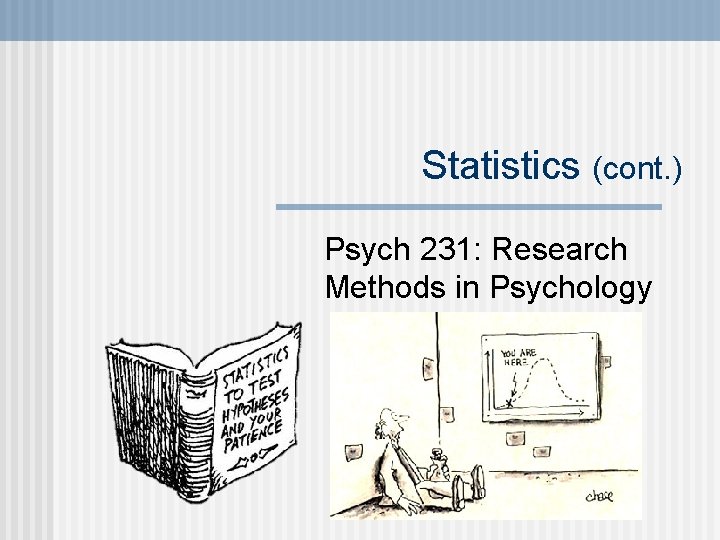
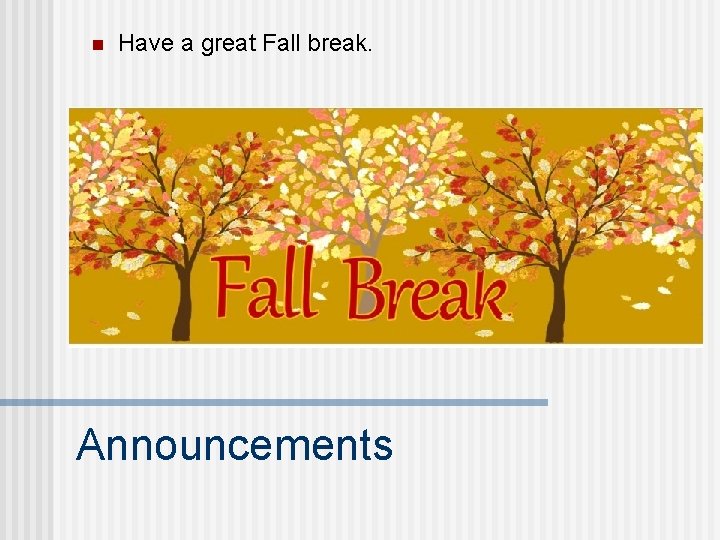
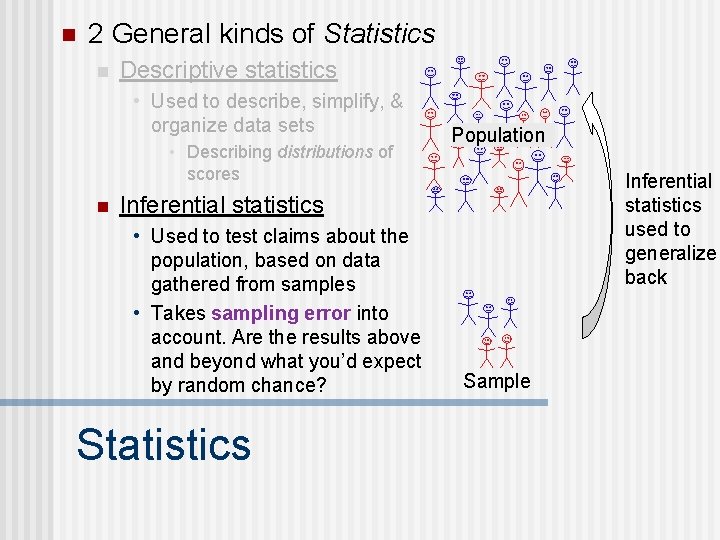
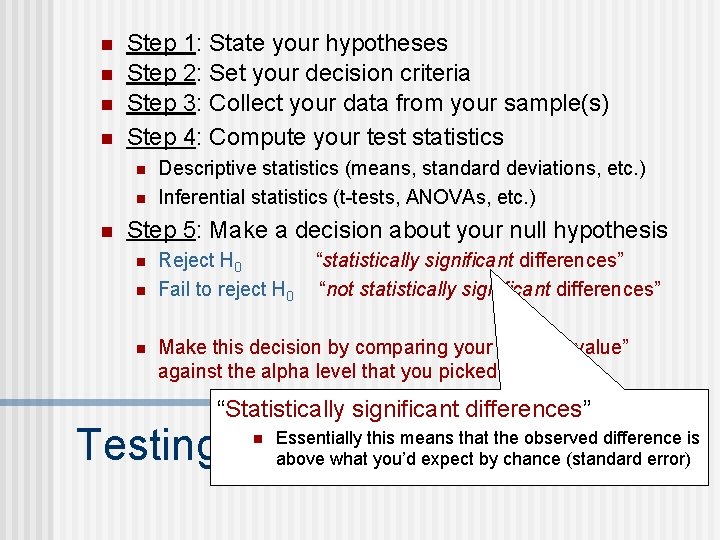
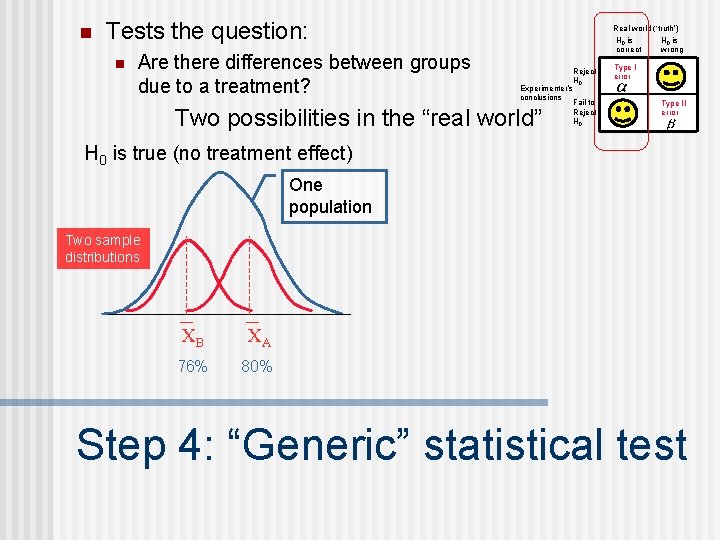
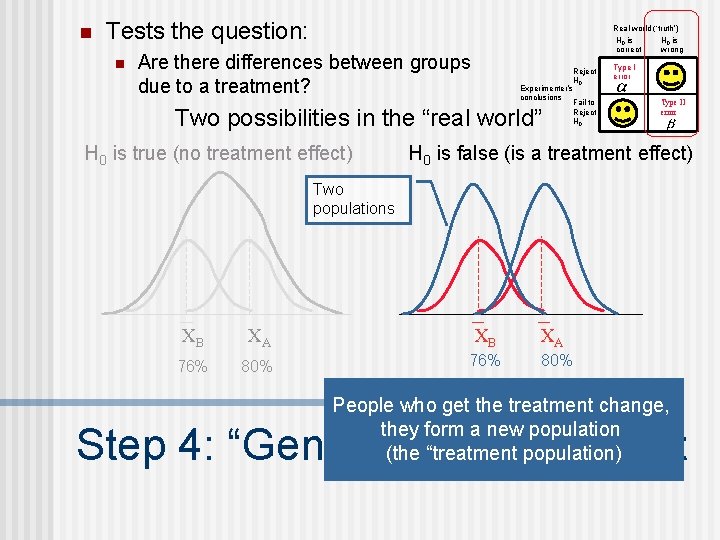
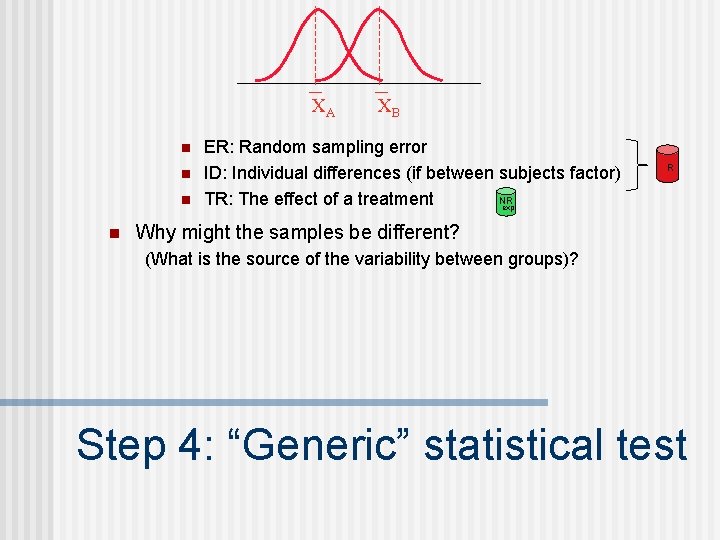
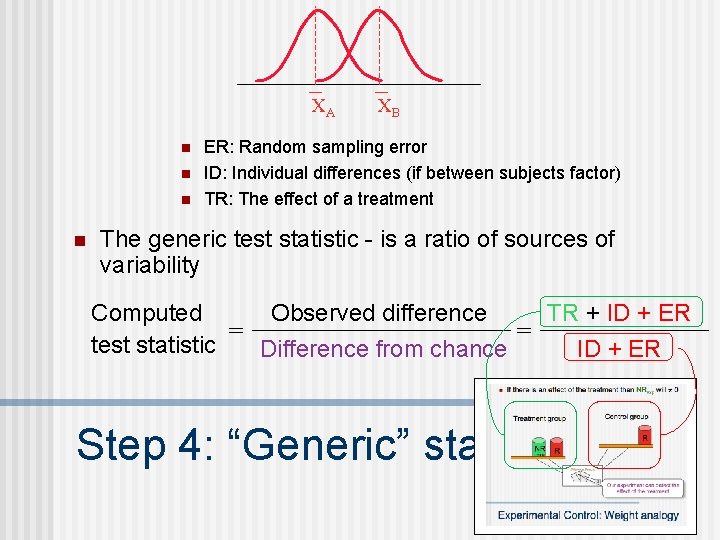
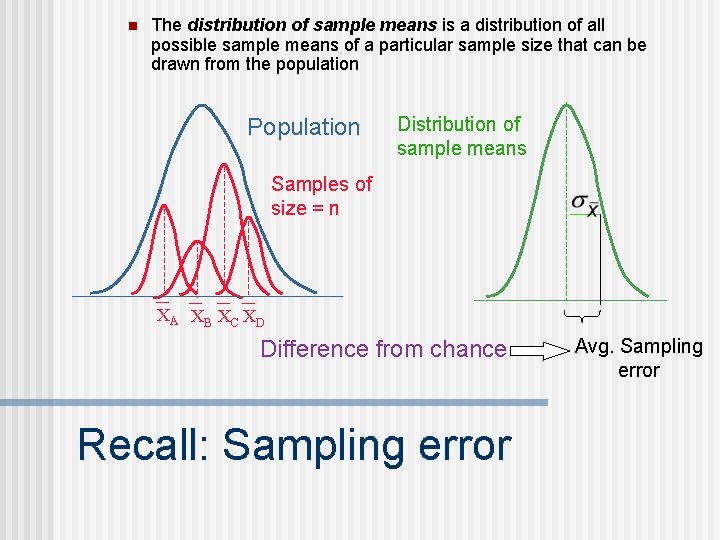
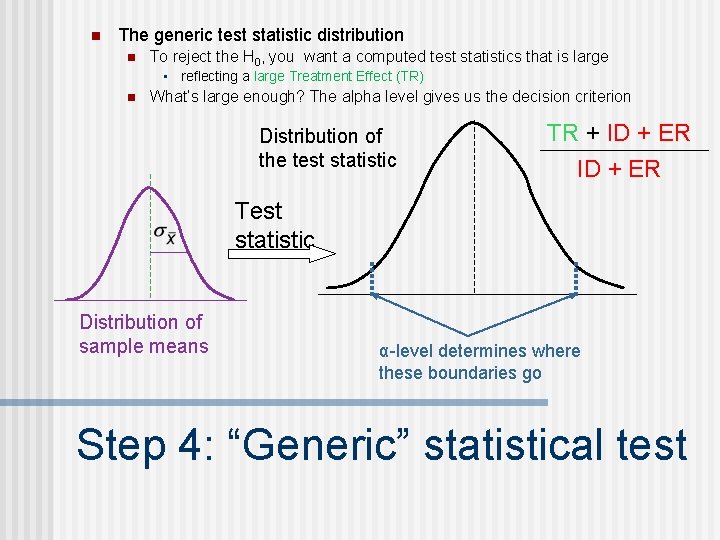
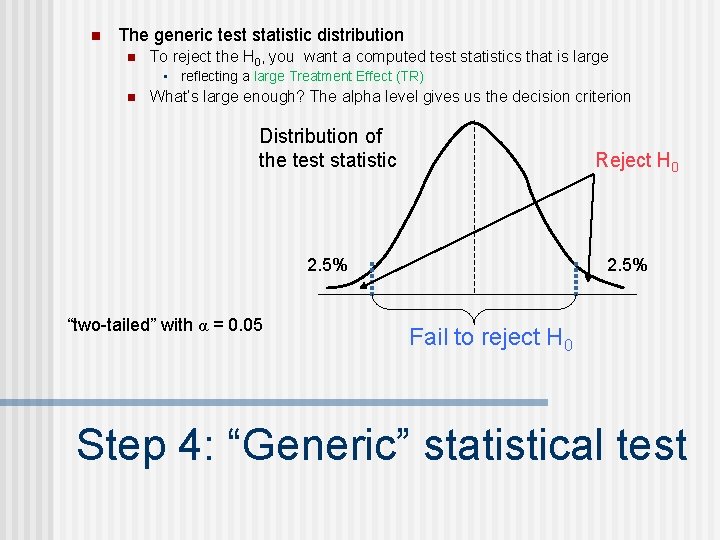
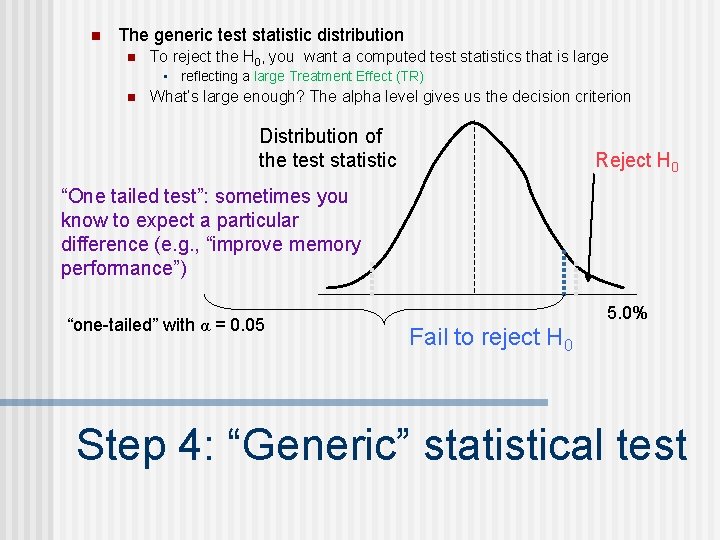
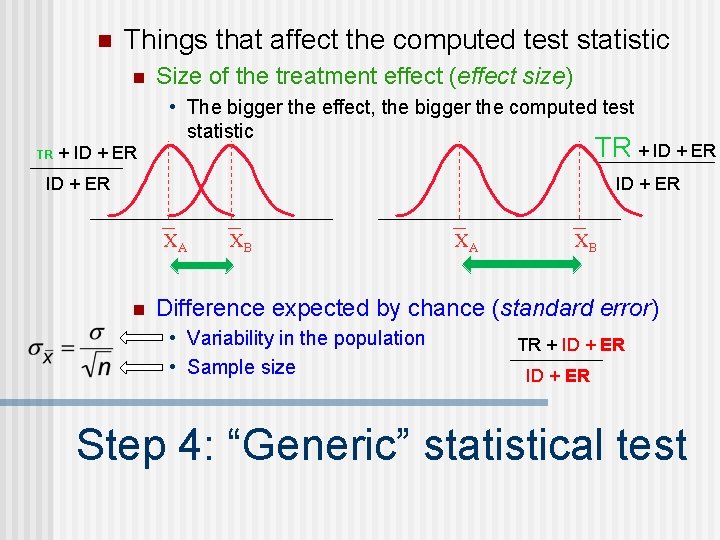
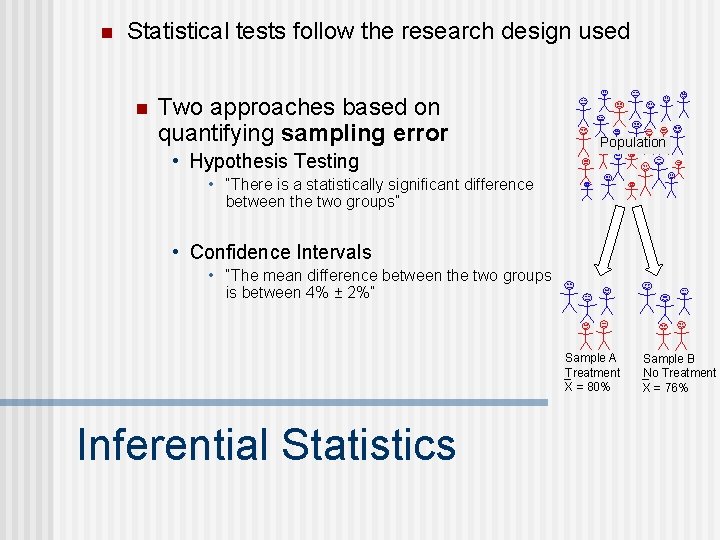
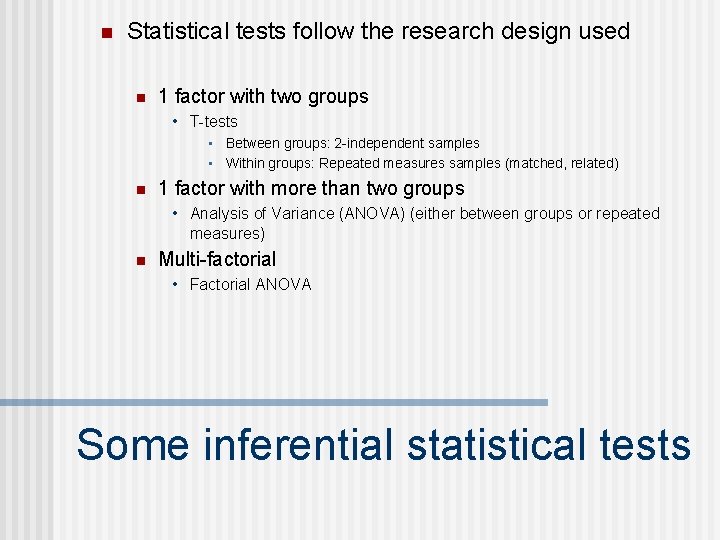
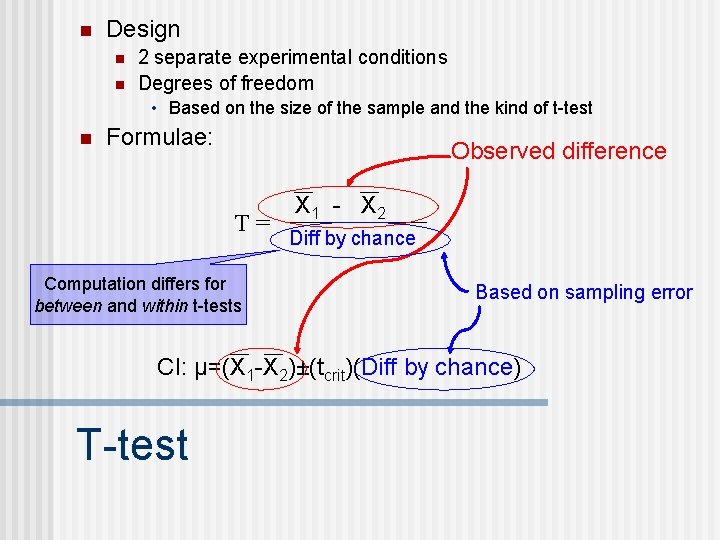
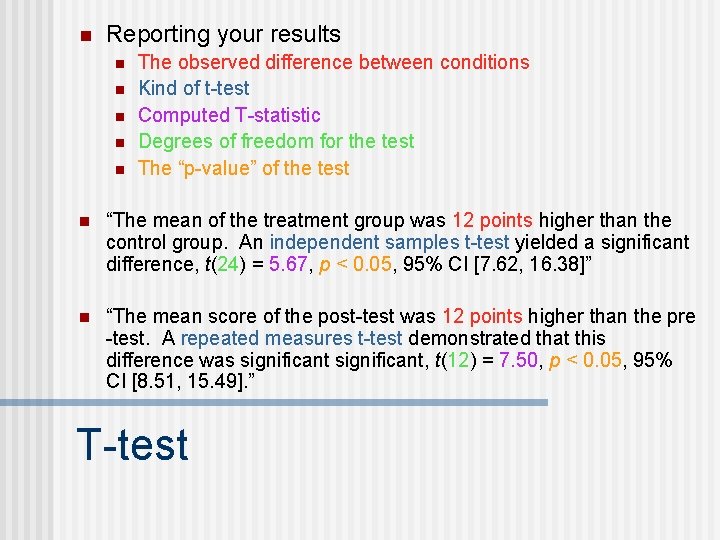
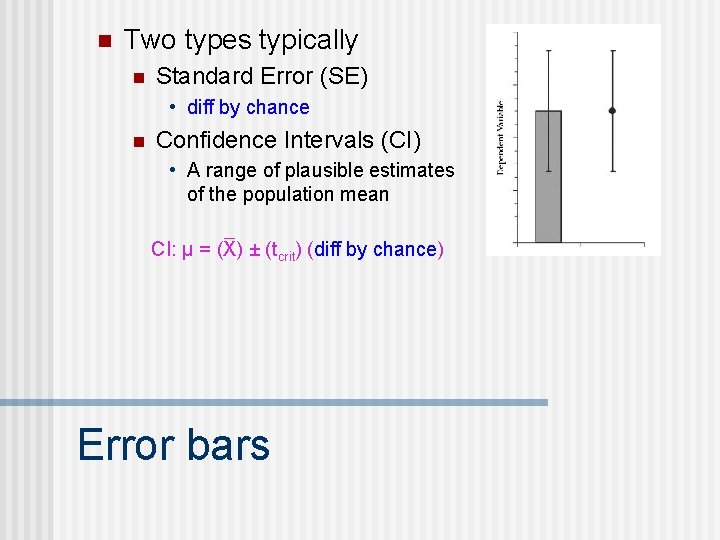
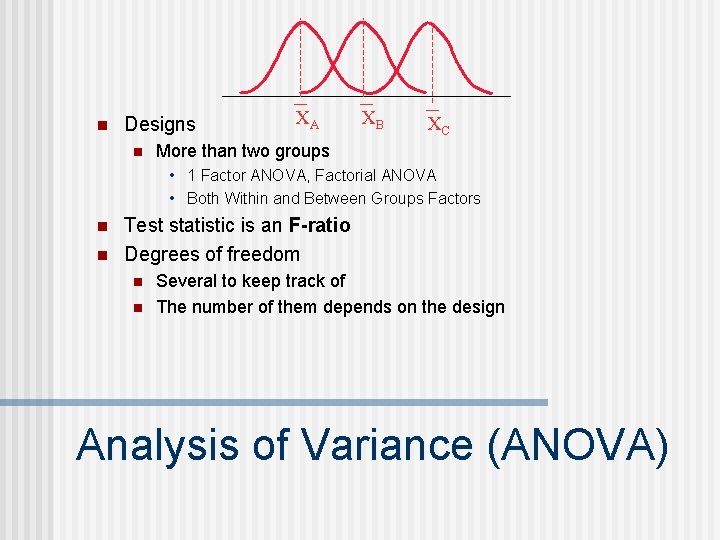
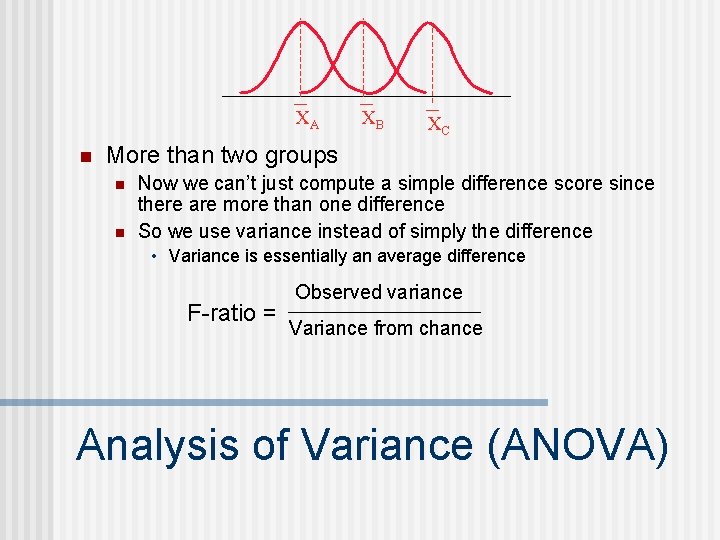
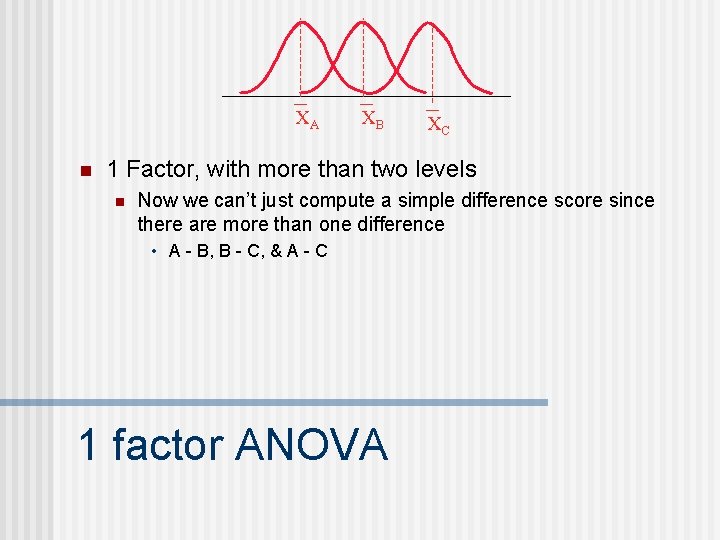
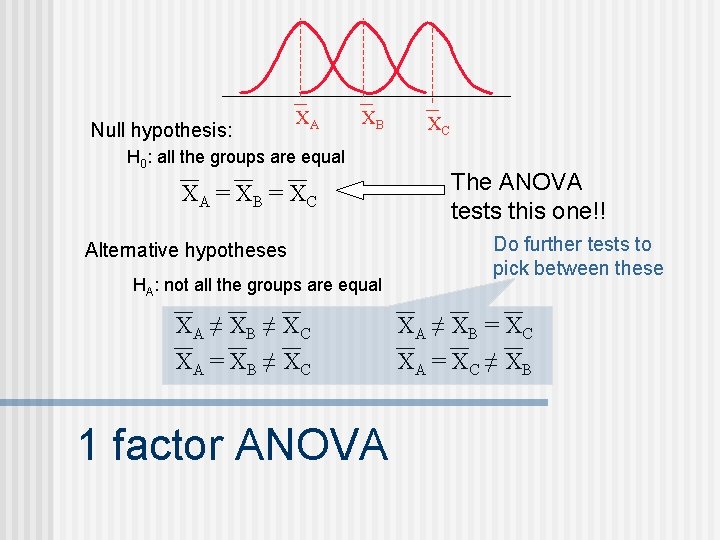
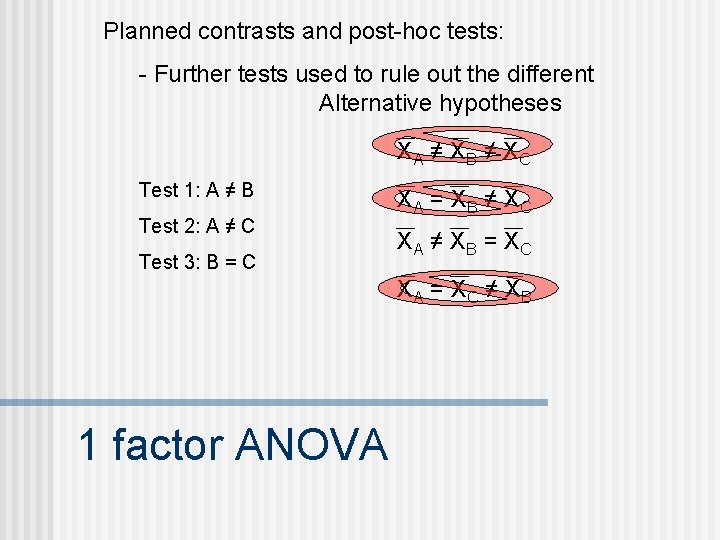
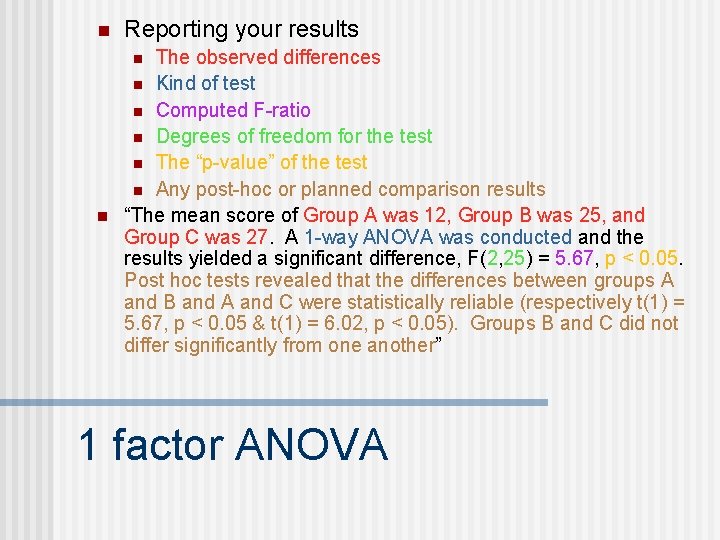
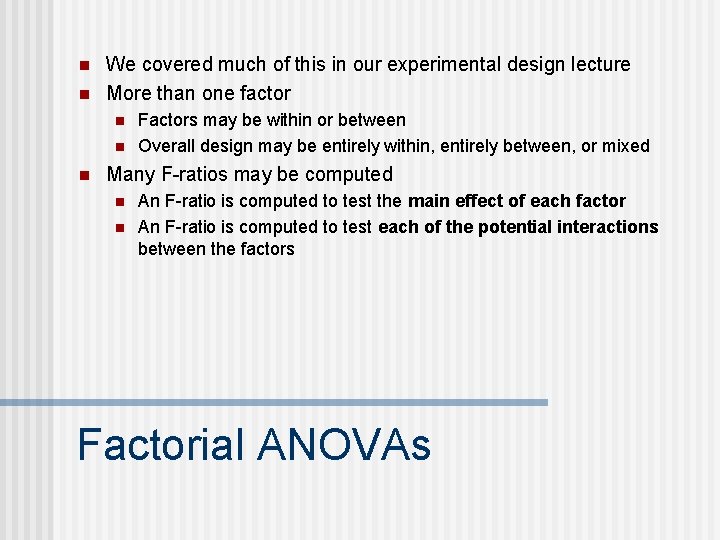
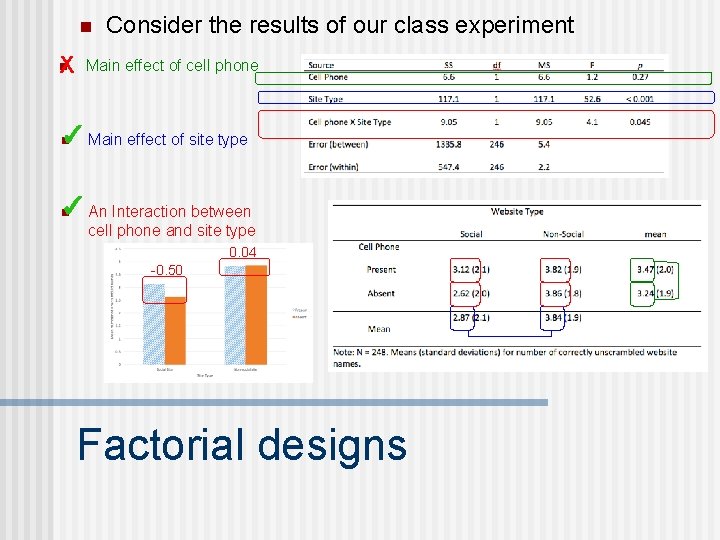
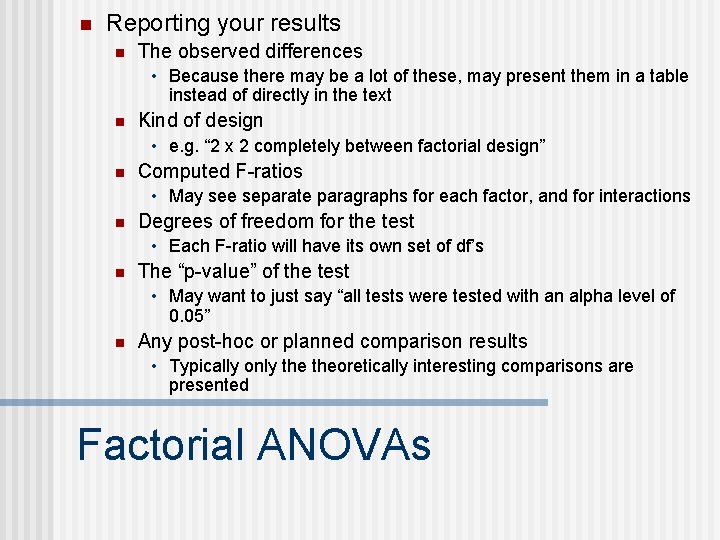
- Slides: 27
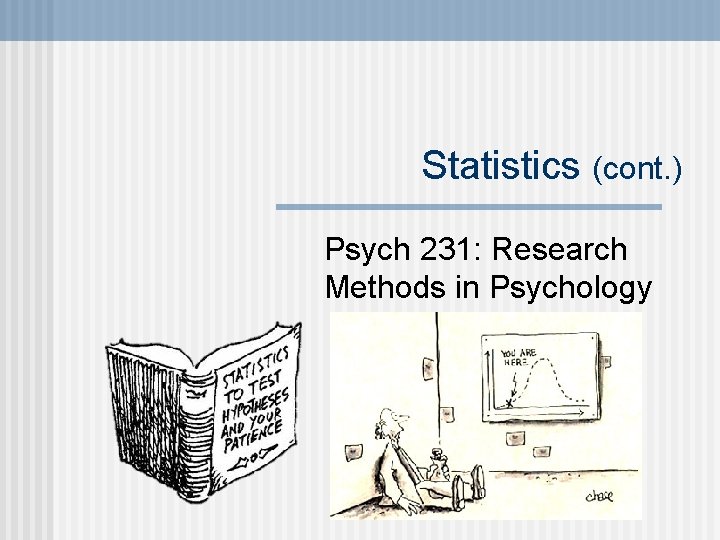
Statistics (cont. ) Psych 231: Research Methods in Psychology
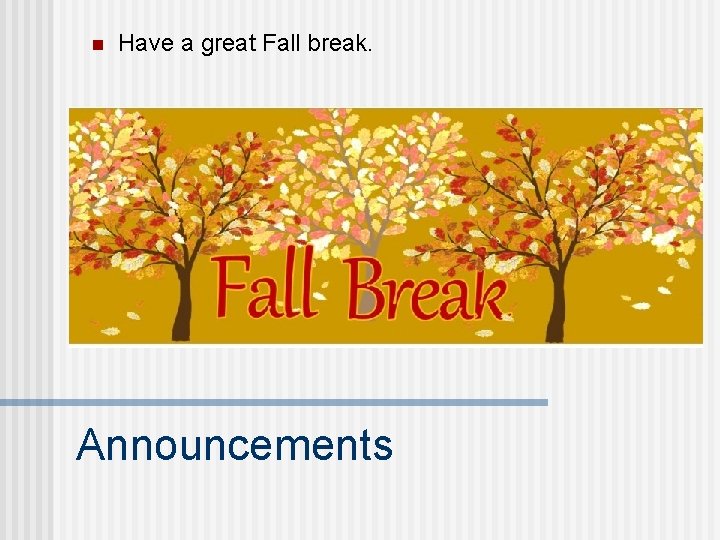
n Have a great Fall break. Announcements
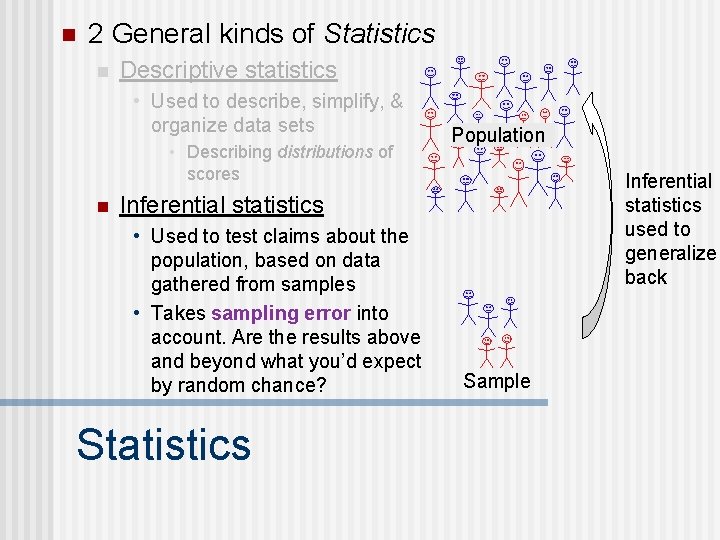
n 2 General kinds of Statistics n Descriptive statistics • Used to describe, simplify, & organize data sets • Describing distributions of scores n Population Inferential statistics used to generalize back Inferential statistics • Used to test claims about the population, based on data gathered from samples • Takes sampling error into account. Are the results above and beyond what you’d expect by random chance? Statistics Sample
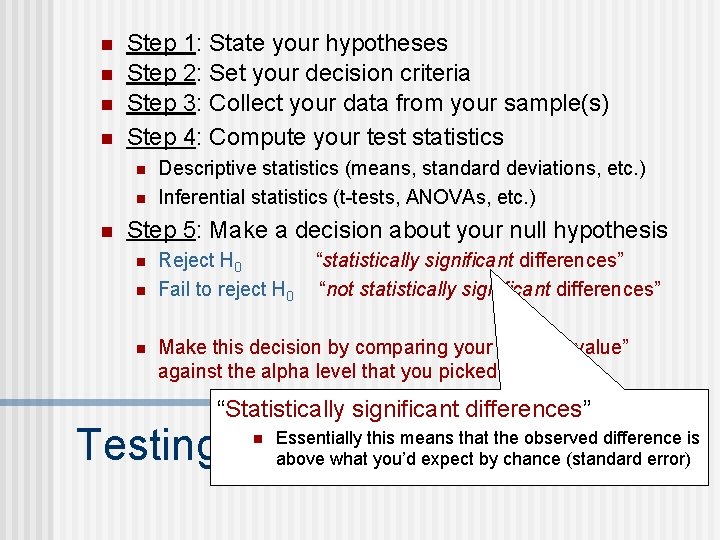
n n Step 1: State your hypotheses Step 2: Set your decision criteria Step 3: Collect your data from your sample(s) Step 4: Compute your test statistics n n n Descriptive statistics (means, standard deviations, etc. ) Inferential statistics (t-tests, ANOVAs, etc. ) Step 5: Make a decision about your null hypothesis n n n Reject H 0 Fail to reject H 0 “statistically significant differences” “not statistically significant differences” Make this decision by comparing your test’s “p-value” against the alpha level that you picked in Step 2. “Statistically significant differences” Testing Hypotheses n Essentially this means that the observed difference is above what you’d expect by chance (standard error)
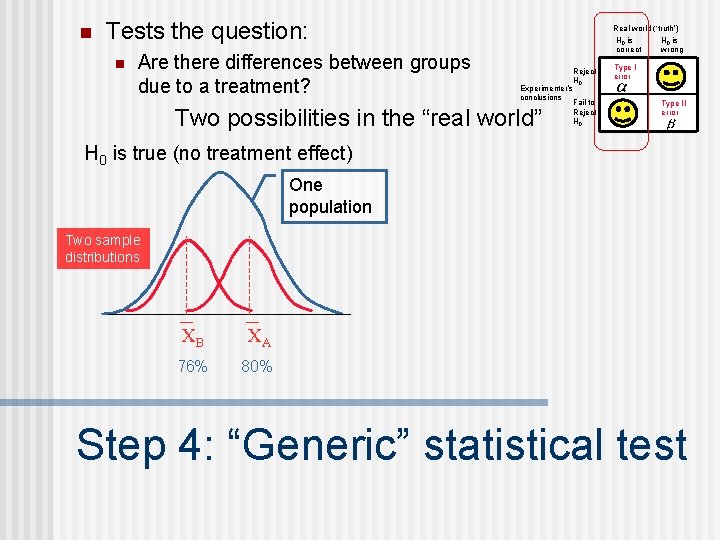
n Tests the question: n Are there differences between groups due to a treatment? Real world (‘truth’) H 0 is correct Reject H 0 Experimenter’s conclusions Fail to Reject H 0 Two possibilities in the “real world” H 0 is wrong Type I error Type II error H 0 is true (no treatment effect) One population Two sample distributions XB XA 76% 80% Step 4: “Generic” statistical test
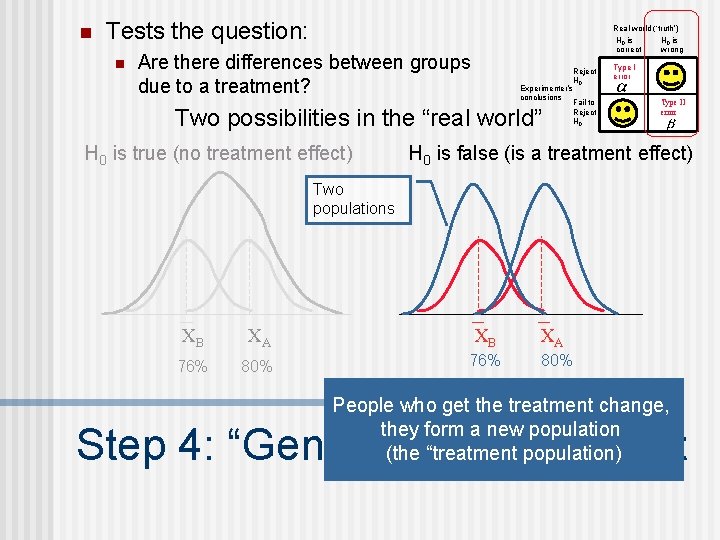
n Tests the question: n Real world (‘truth’) H 0 is correct Are there differences between groups due to a treatment? Reject H 0 Experimenter’s conclusions Fail to Reject H 0 Two possibilities in the “real world” H 0 is true (no treatment effect) H 0 is wrong Type I error Type II error H 0 is false (is a treatment effect) Two populations XB XA 76% 80% People who get the treatment change, they form a new population (the “treatment population) Step 4: “Generic” statistical test
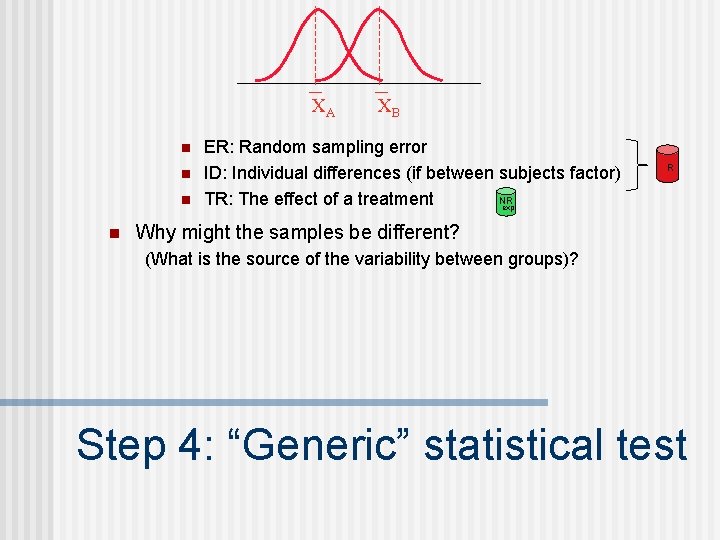
XA n n XB ER: Random sampling error ID: Individual differences (if between subjects factor) NR TR: The effect of a treatment exp R Why might the samples be different? (What is the source of the variability between groups)? Step 4: “Generic” statistical test
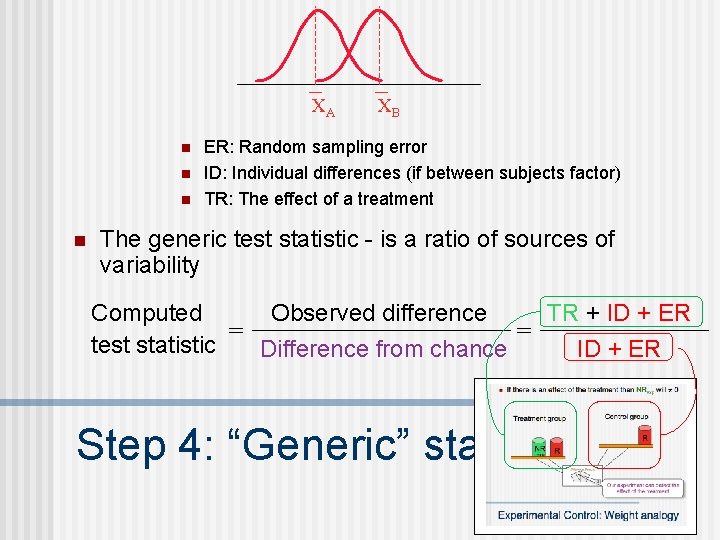
XA n n XB ER: Random sampling error ID: Individual differences (if between subjects factor) TR: The effect of a treatment The generic test statistic - is a ratio of sources of variability TR + ID + ER Observed difference Computed = = test statistic Difference from chance ID + ER Step 4: “Generic” statistical test
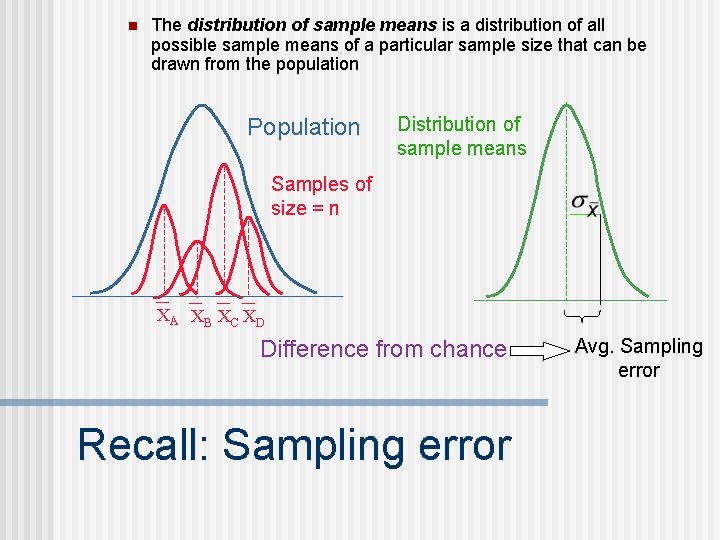
n The distribution of sample means is a distribution of all possible sample means of a particular sample size that can be drawn from the population Population Distribution of sample means Samples of size = n XA XB XC XD Difference from chance Recall: Sampling error Avg. Sampling error
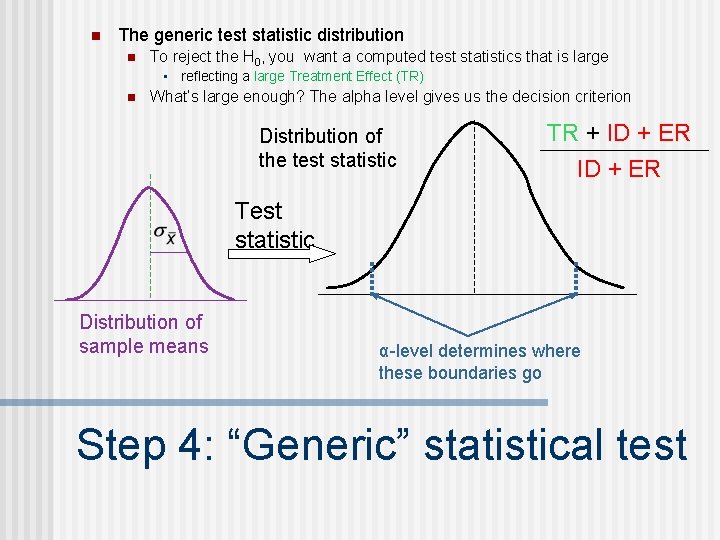
n The generic test statistic distribution n To reject the H 0, you want a computed test statistics that is large • reflecting a large Treatment Effect (TR) n What’s large enough? The alpha level gives us the decision criterion Distribution of the test statistic TR + ID + ER Test statistic Distribution of sample means α-level determines where these boundaries go Step 4: “Generic” statistical test
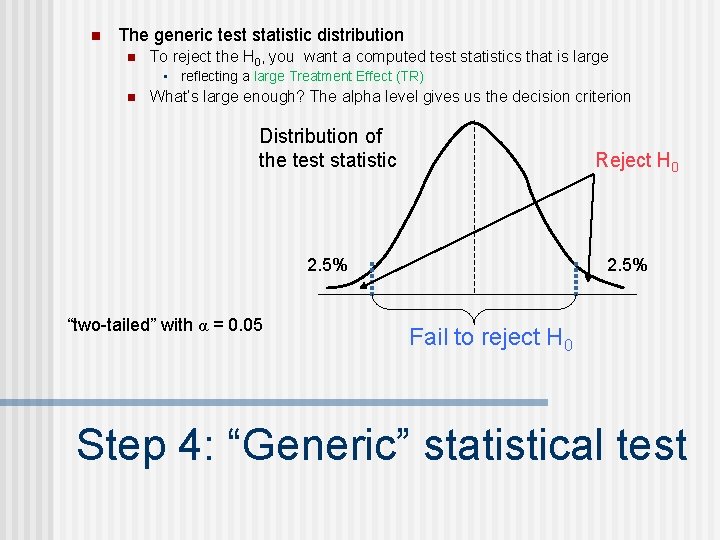
n The generic test statistic distribution n To reject the H 0, you want a computed test statistics that is large • reflecting a large Treatment Effect (TR) n What’s large enough? The alpha level gives us the decision criterion Distribution of the test statistic Reject H 0 2. 5% “two-tailed” with α = 0. 05 2. 5% Fail to reject H 0 Step 4: “Generic” statistical test
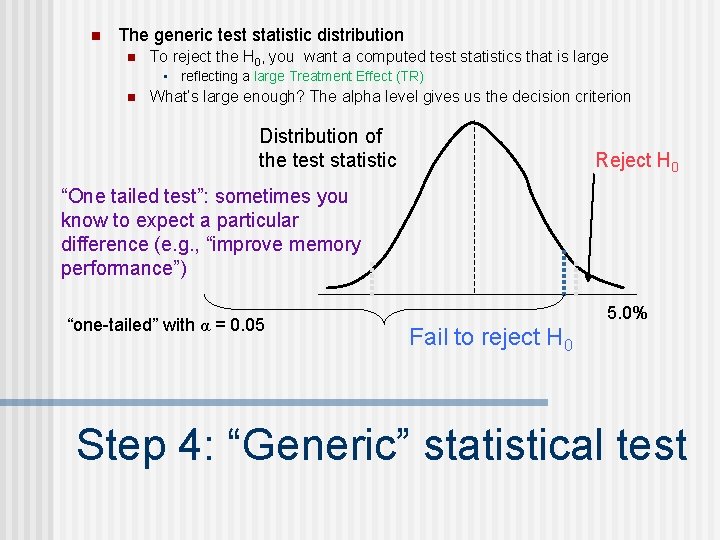
n The generic test statistic distribution n To reject the H 0, you want a computed test statistics that is large • reflecting a large Treatment Effect (TR) n What’s large enough? The alpha level gives us the decision criterion Distribution of the test statistic Reject H 0 “One tailed test”: sometimes you know to expect a particular difference (e. g. , “improve memory performance”) “one-tailed” with α = 0. 05 5. 0% Fail to reject H 0 Step 4: “Generic” statistical test
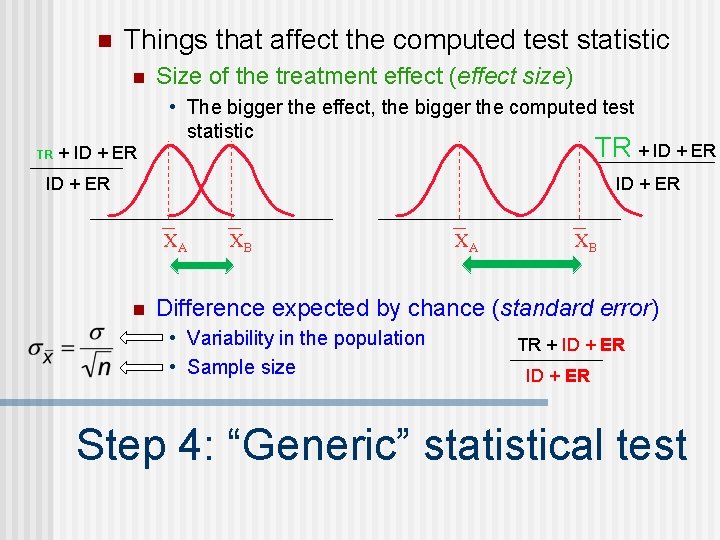
n Things that affect the computed test statistic n Size of the treatment effect (effect size) • The bigger the effect, the bigger the computed test statistic TR TR + ID + ER XA n XB XA XB Difference expected by chance (standard error) • Variability in the population • Sample size TR + ID + ER Step 4: “Generic” statistical test
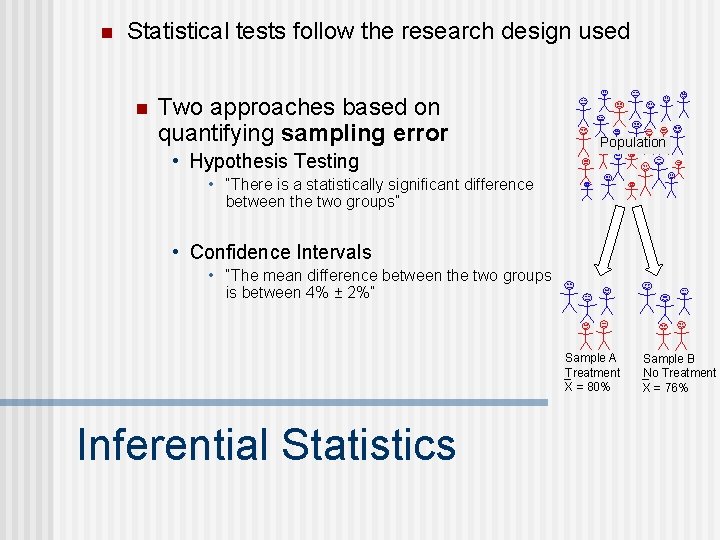
n Statistical tests follow the research design used n Two approaches based on quantifying sampling error • Hypothesis Testing Population • “There is a statistically significant difference between the two groups” • Confidence Intervals • “The mean difference between the two groups is between 4% ± 2%” Sample A Treatment X = 80% Inferential Statistics Sample B No Treatment X = 76%
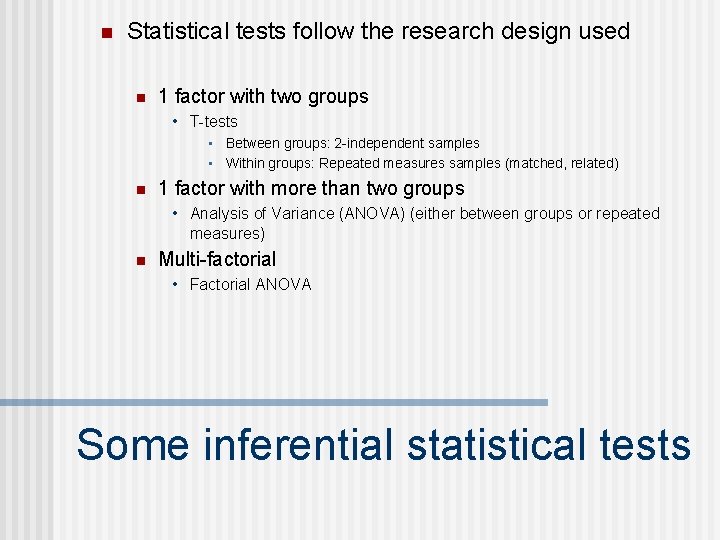
n Statistical tests follow the research design used n 1 factor with two groups • T-tests • Between groups: 2 -independent samples • Within groups: Repeated measures samples (matched, related) n 1 factor with more than two groups • Analysis of Variance (ANOVA) (either between groups or repeated measures) n Multi-factorial • Factorial ANOVA Some inferential statistical tests
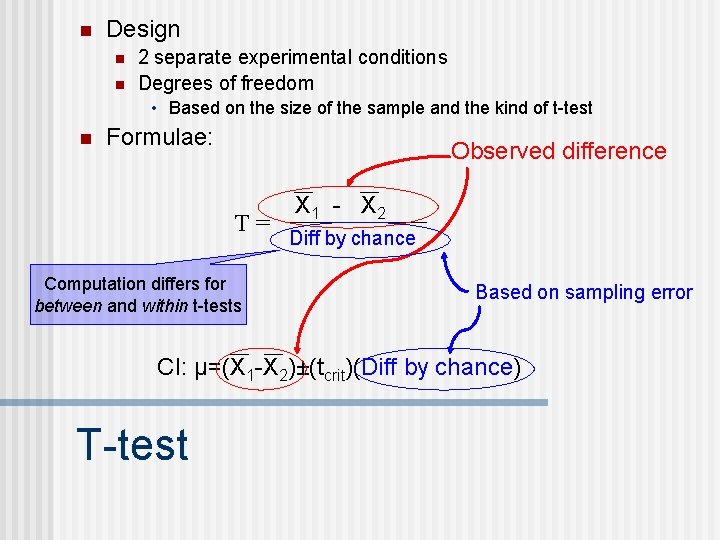
n Design n n 2 separate experimental conditions Degrees of freedom • Based on the size of the sample and the kind of t-test n Formulae: Observed difference T= Computation differs for between and within t-tests X 1 - X 2 Diff by chance Based on sampling error CI: μ=(X 1 -X 2)±(tcrit)(Diff by chance) T-test
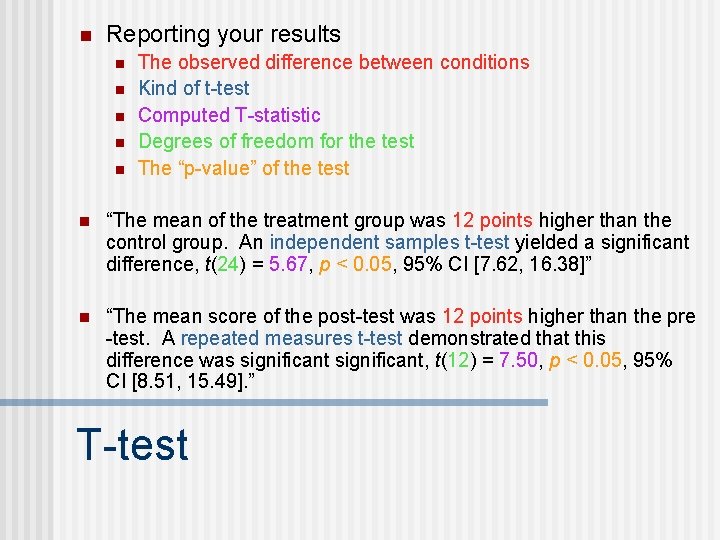
n Reporting your results n n n The observed difference between conditions Kind of t-test Computed T-statistic Degrees of freedom for the test The “p-value” of the test n “The mean of the treatment group was 12 points higher than the control group. An independent samples t-test yielded a significant difference, t(24) = 5. 67, p < 0. 05, 95% CI [7. 62, 16. 38]” n “The mean score of the post-test was 12 points higher than the pre -test. A repeated measures t-test demonstrated that this difference was significant, t(12) = 7. 50, p < 0. 05, 95% CI [8. 51, 15. 49]. ” T-test
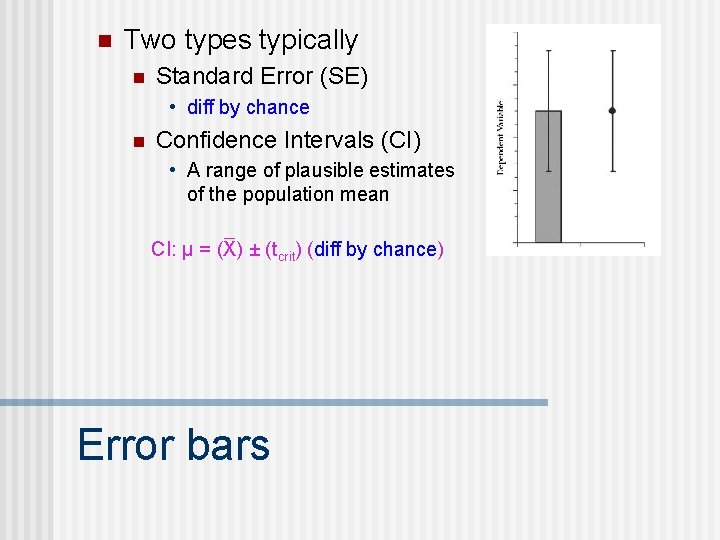
n Two types typically n Standard Error (SE) • diff by chance n Confidence Intervals (CI) • A range of plausible estimates of the population mean CI: μ = (X) ± (tcrit) (diff by chance) Error bars
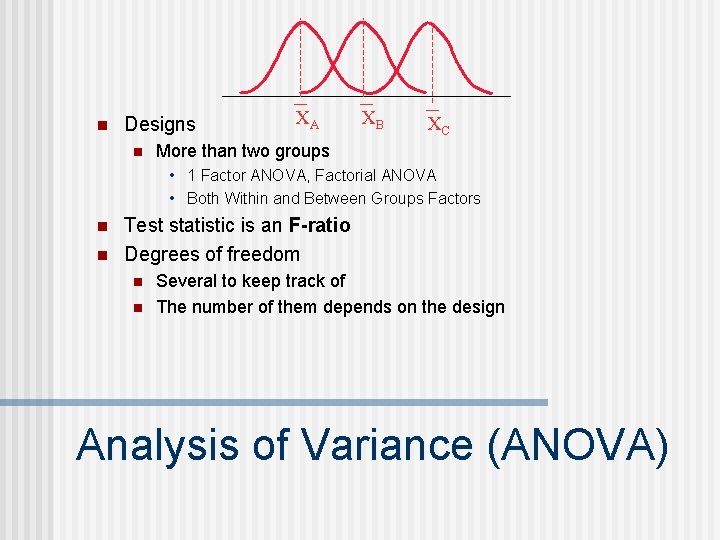
n Designs n XA XB XC More than two groups • 1 Factor ANOVA, Factorial ANOVA • Both Within and Between Groups Factors n n Test statistic is an F-ratio Degrees of freedom n n Several to keep track of The number of them depends on the design Analysis of Variance (ANOVA)
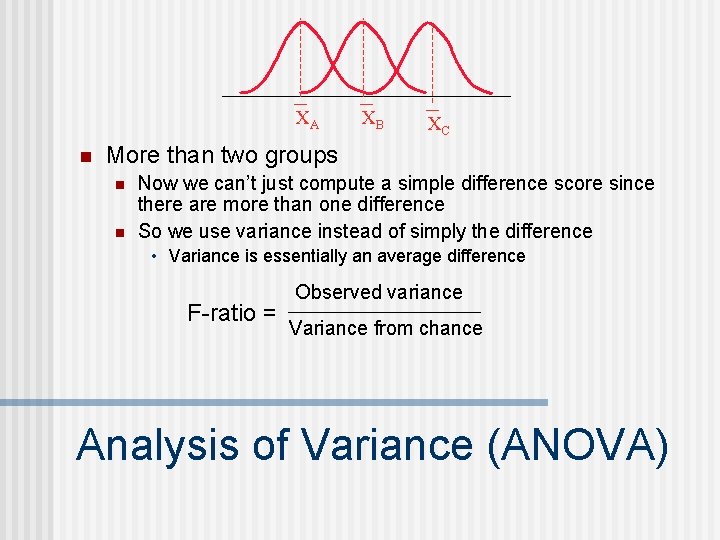
XA n XB XC More than two groups n n Now we can’t just compute a simple difference score since there are more than one difference So we use variance instead of simply the difference • Variance is essentially an average difference F-ratio = Observed variance Variance from chance Analysis of Variance (ANOVA)
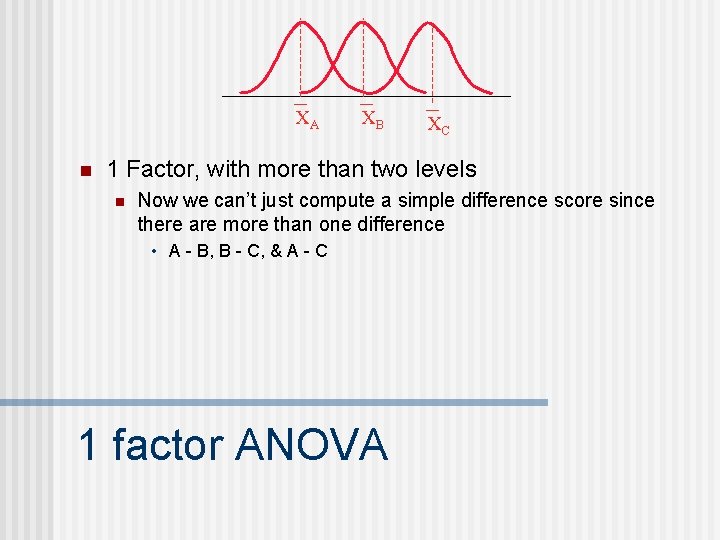
XA n XB XC 1 Factor, with more than two levels n Now we can’t just compute a simple difference score since there are more than one difference • A - B, B - C, & A - C 1 factor ANOVA
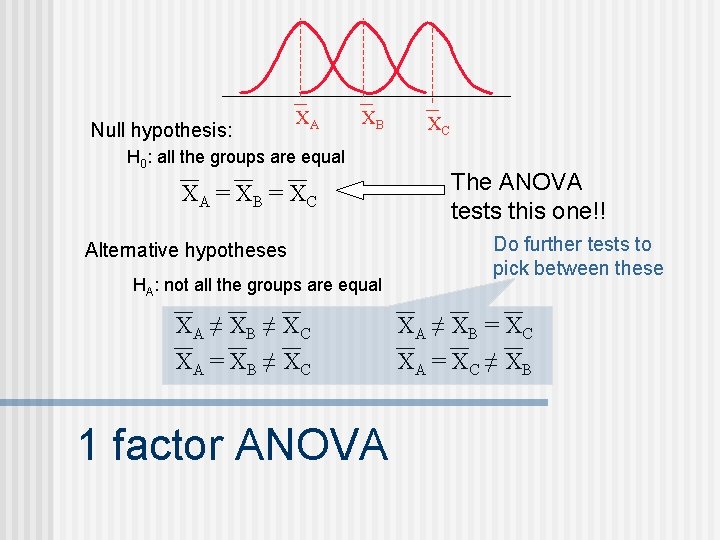
Null hypothesis: XA XB H 0: all the groups are equal XA = X B = X C Alternative hypotheses HA: not all the groups are equal XA ≠ X B ≠ X C XA = X B ≠ X C 1 factor ANOVA XC The ANOVA tests this one!! Do further tests to pick between these XA ≠ X B = X C XA = X C ≠ X B
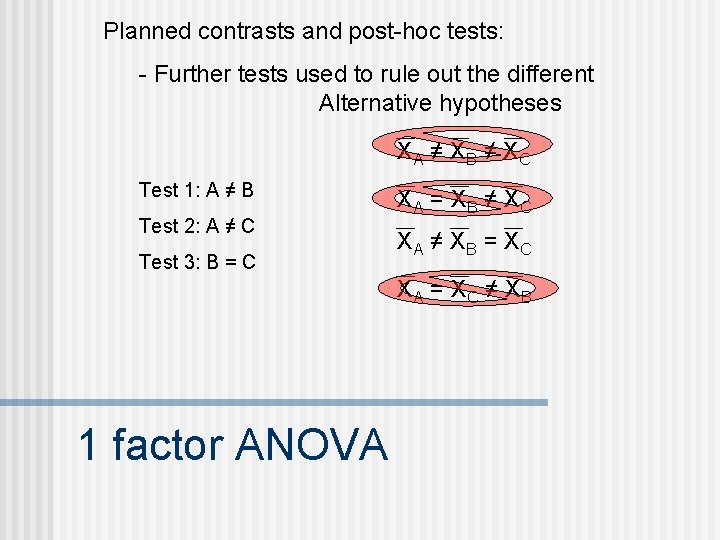
Planned contrasts and post-hoc tests: - Further tests used to rule out the different Alternative hypotheses XA ≠ X B ≠ X C Test 1: A ≠ B Test 2: A ≠ C Test 3: B = C XA = X B ≠ X C XA ≠ X B = X C XA = X C ≠ X B 1 factor ANOVA
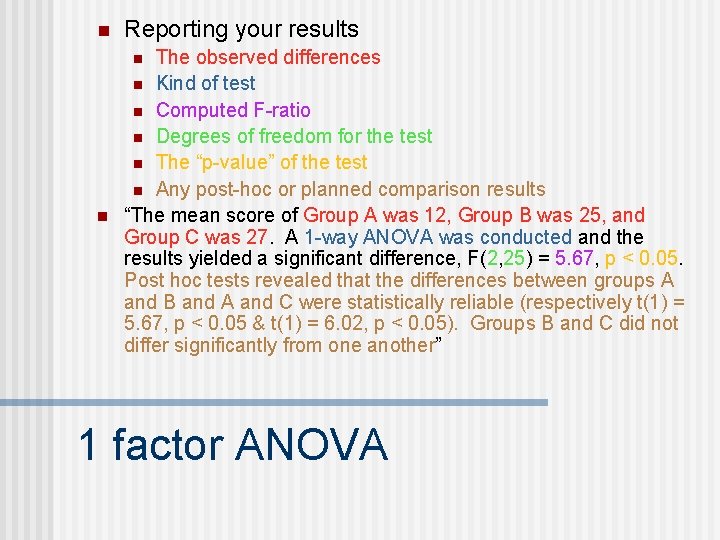
n Reporting your results The observed differences n Kind of test n Computed F-ratio n Degrees of freedom for the test n The “p-value” of the test n Any post-hoc or planned comparison results “The mean score of Group A was 12, Group B was 25, and Group C was 27. A 1 -way ANOVA was conducted and the results yielded a significant difference, F(2, 25) = 5. 67, p < 0. 05. Post hoc tests revealed that the differences between groups A and B and A and C were statistically reliable (respectively t(1) = 5. 67, p < 0. 05 & t(1) = 6. 02, p < 0. 05). Groups B and C did not differ significantly from one another” n n 1 factor ANOVA
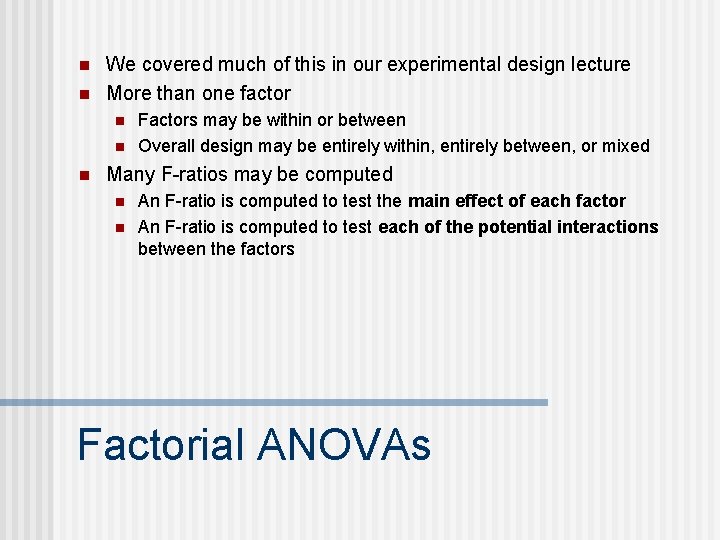
n n We covered much of this in our experimental design lecture More than one factor n n n Factors may be within or between Overall design may be entirely within, entirely between, or mixed Many F-ratios may be computed n n An F-ratio is computed to test the main effect of each factor An F-ratio is computed to test each of the potential interactions between the factors Factorial ANOVAs
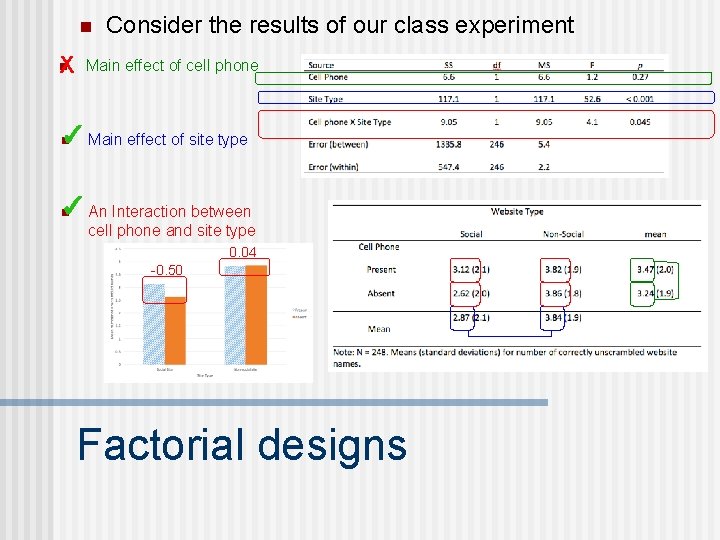
n n X Consider the results of our class experiment Main effect of cell phone ✓ Main effect of site type n ✓ An Interaction between n cell phone and site type 0. 04 -0. 50 Factorial designs
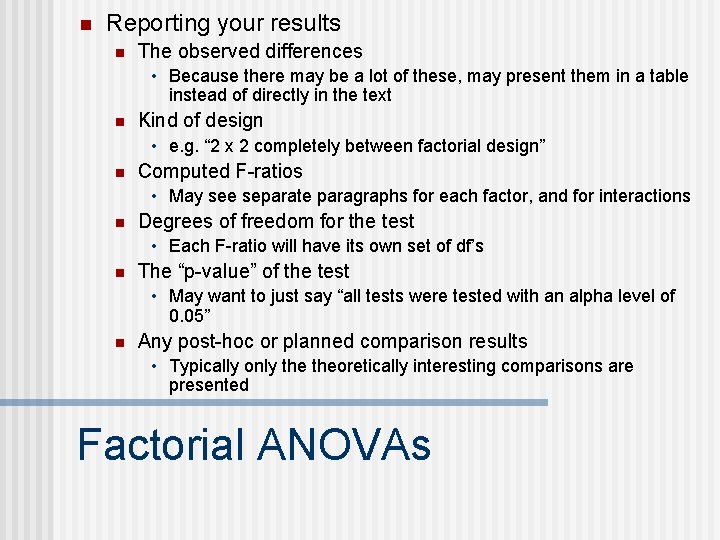
n Reporting your results n The observed differences • Because there may be a lot of these, may present them in a table instead of directly in the text n Kind of design • e. g. “ 2 x 2 completely between factorial design” n Computed F-ratios • May see separate paragraphs for each factor, and for interactions n Degrees of freedom for the test • Each F-ratio will have its own set of df’s n The “p-value” of the test • May want to just say “all tests were tested with an alpha level of 0. 05” n Any post-hoc or planned comparison results • Typically only theoretically interesting comparisons are presented Factorial ANOVAs
Debriefing report
Cont or cont'd
Positive psychology ap psych
Attribution theory ap psych
Method of social psychology
Research methods in developmental psychology
Research methods in abnormal psychology
Ocr psychology research methods
Psychology research methods worksheet answers
Introduction to statistics what is statistics
Descriptive statistics numerical measures
Sampling methods statistics
Tabular presentation of quantitative data
Wax pattern fabrication
Acf 231
Article 231 of the treaty of versailles
123 132 213 231 312 321
Pa msu
D. lgs. 231/2007
Hino 231
Gezang 231
Counter with unused states
Transitor
Walfisch ikegami model
Draw 231 with base ten blocks
040 231 3666
Mvcnn pytorch
Standford cs231