Statistics cont Psych 231 Research Methods in Psychology
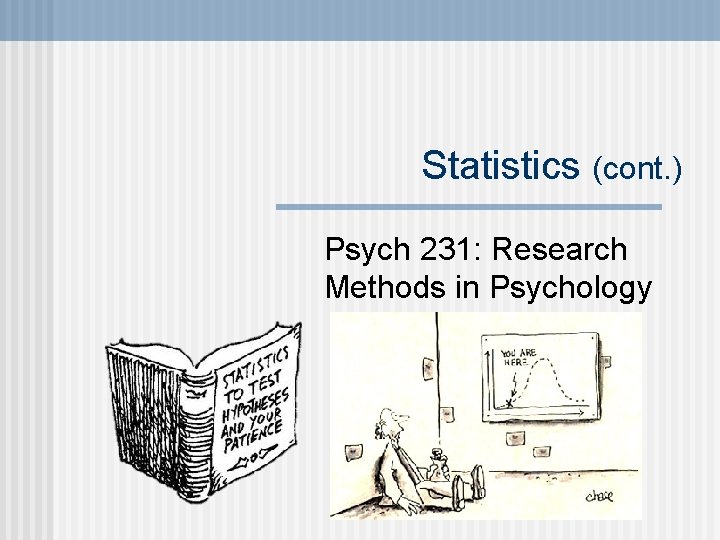
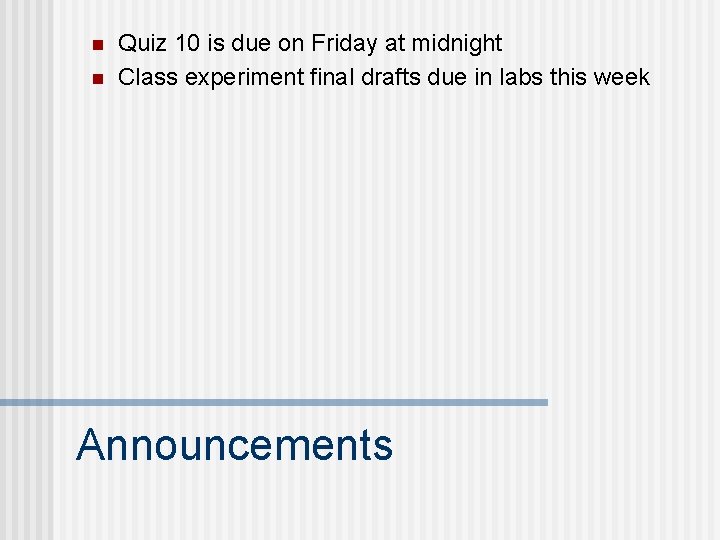
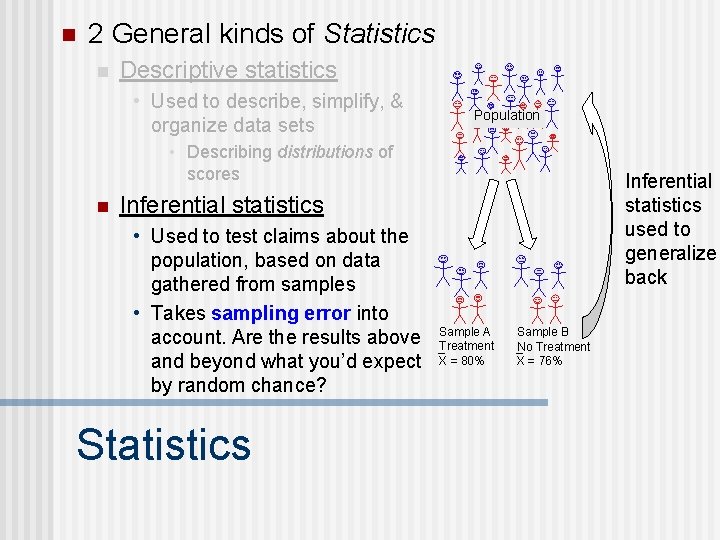
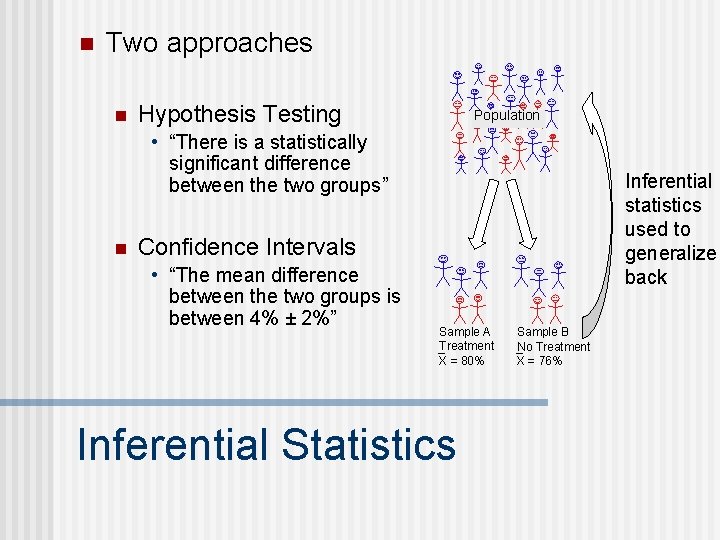
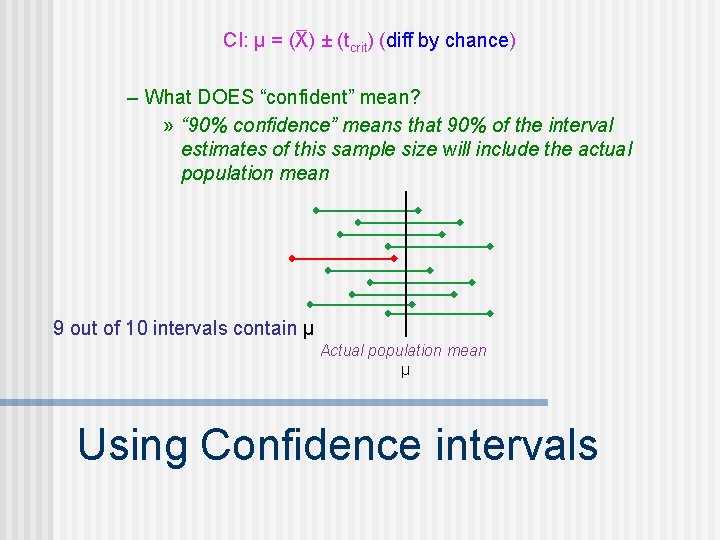
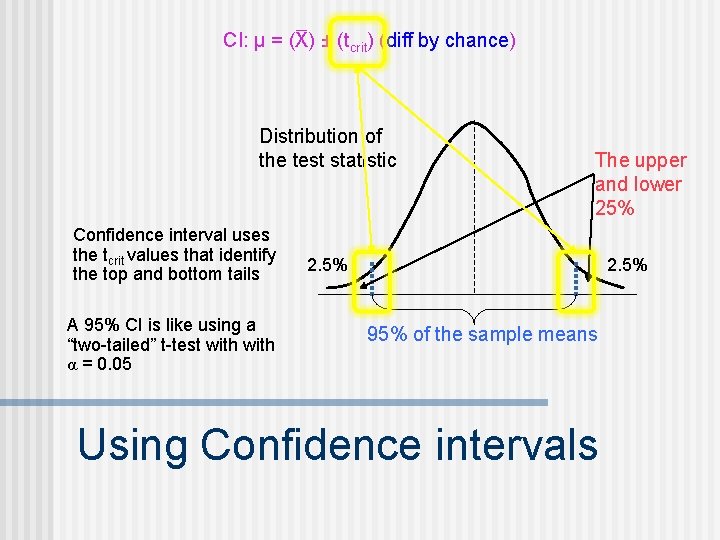
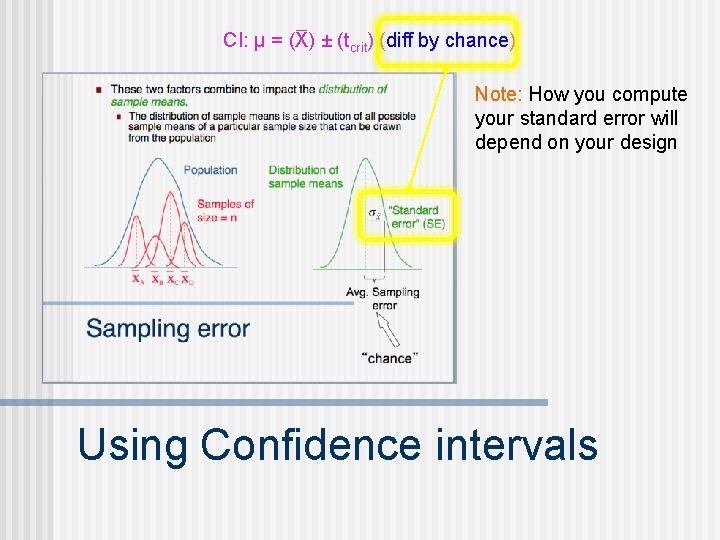
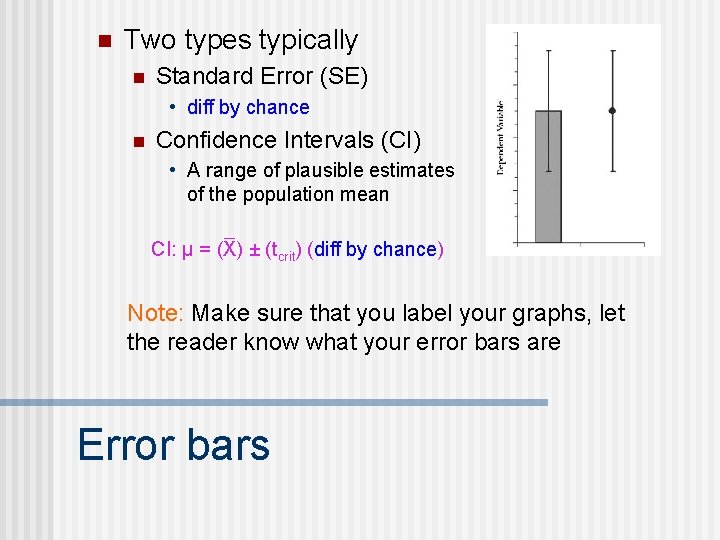
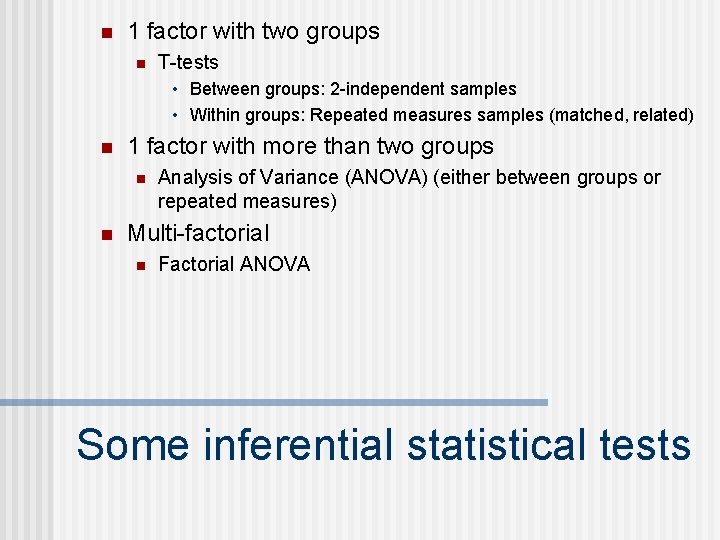
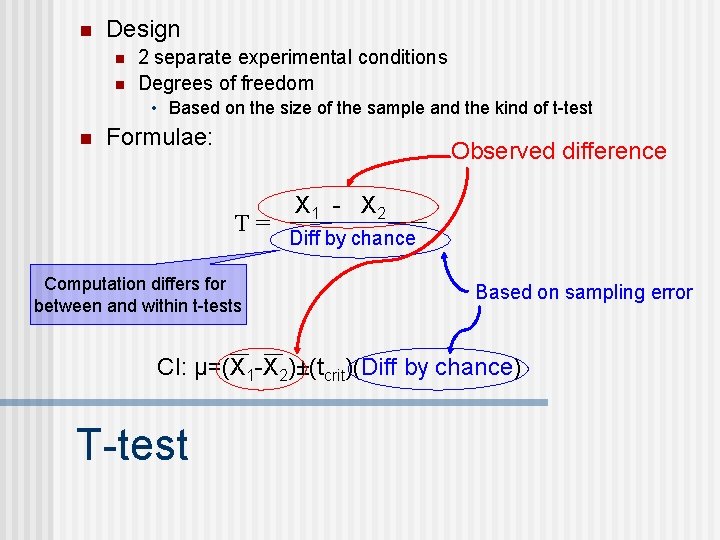
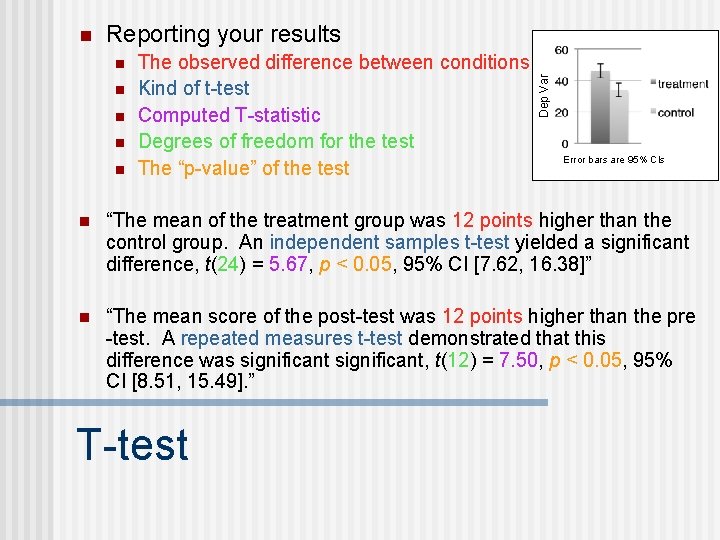
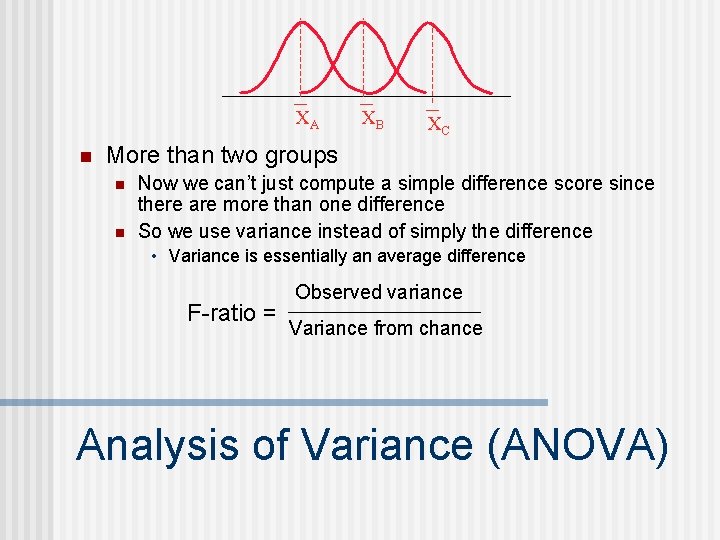
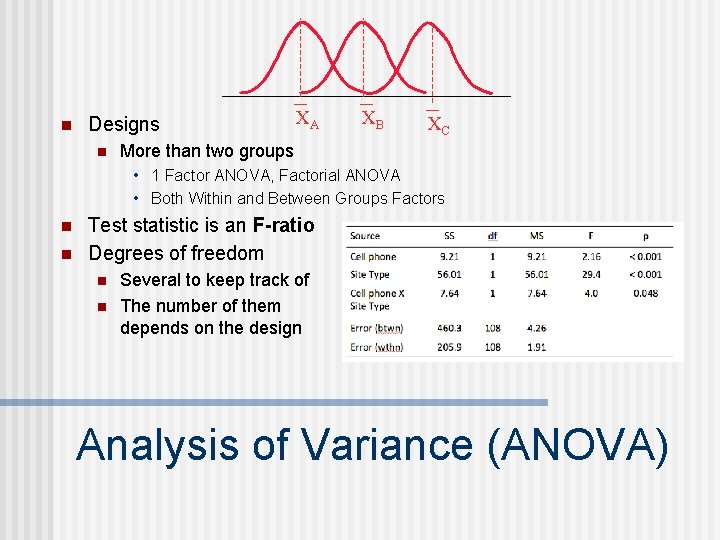
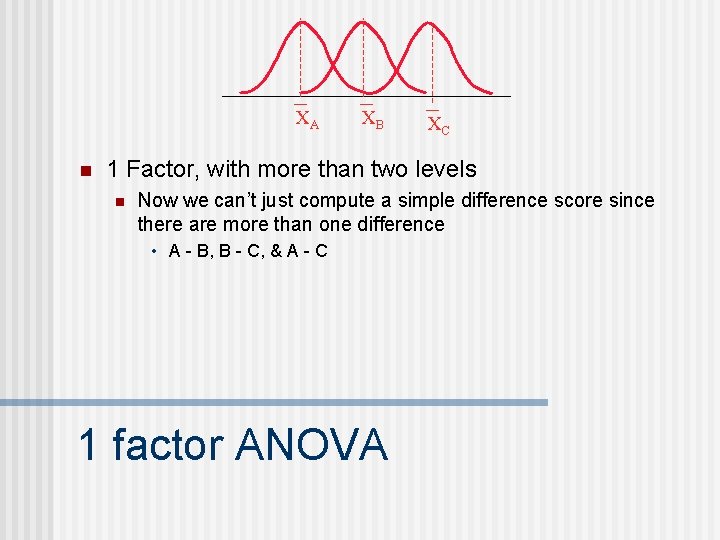
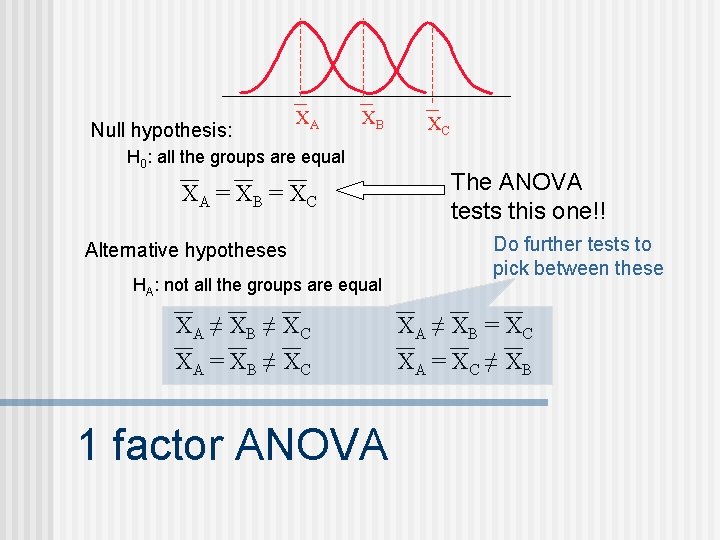
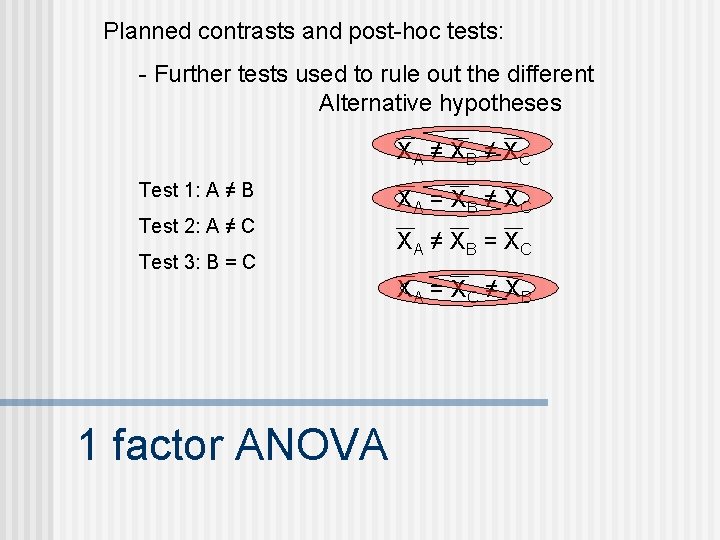
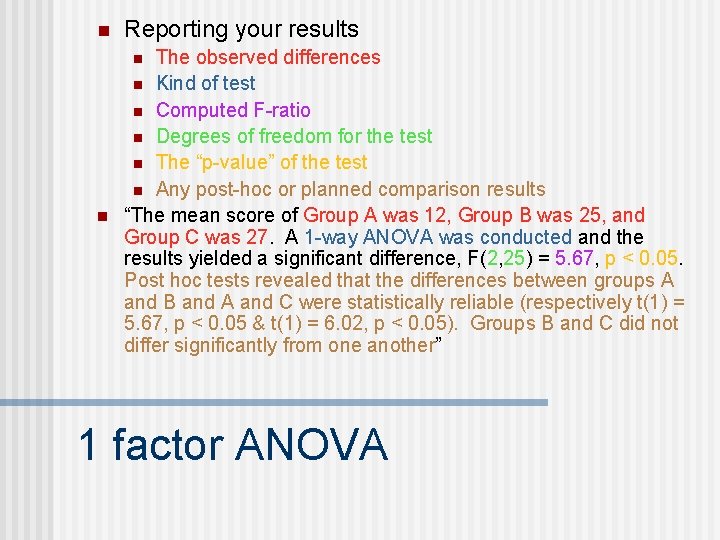
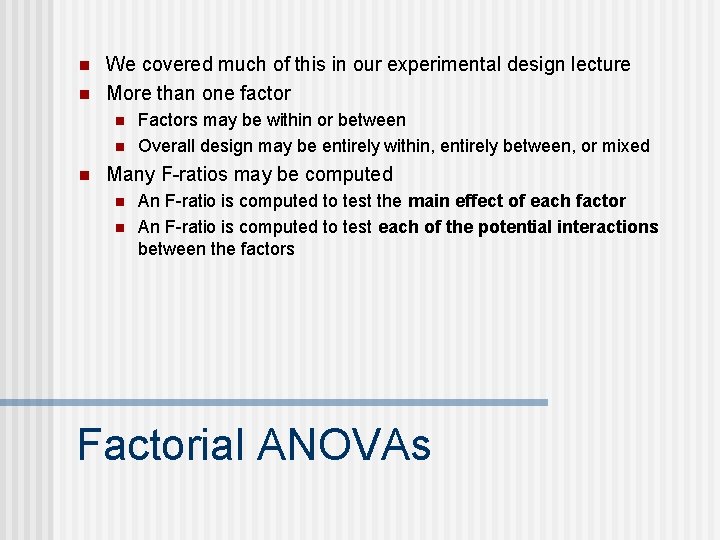
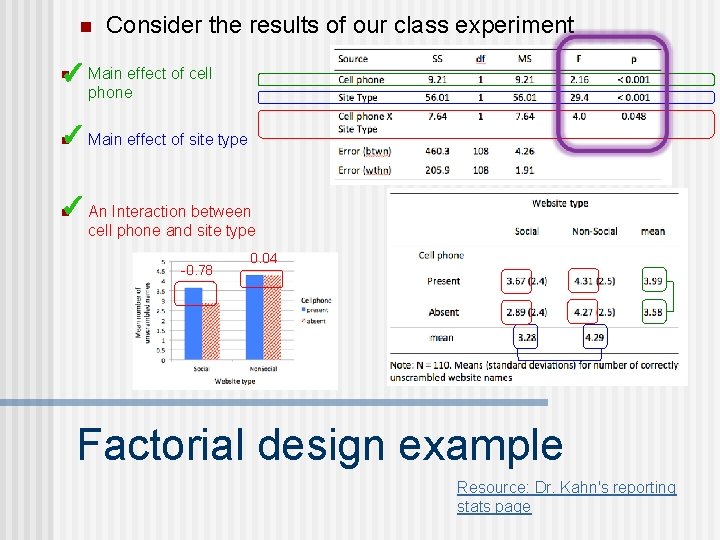
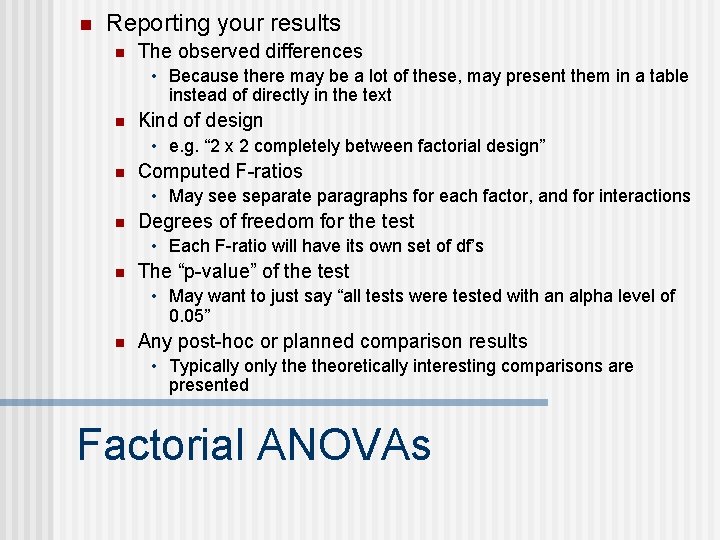
- Slides: 20
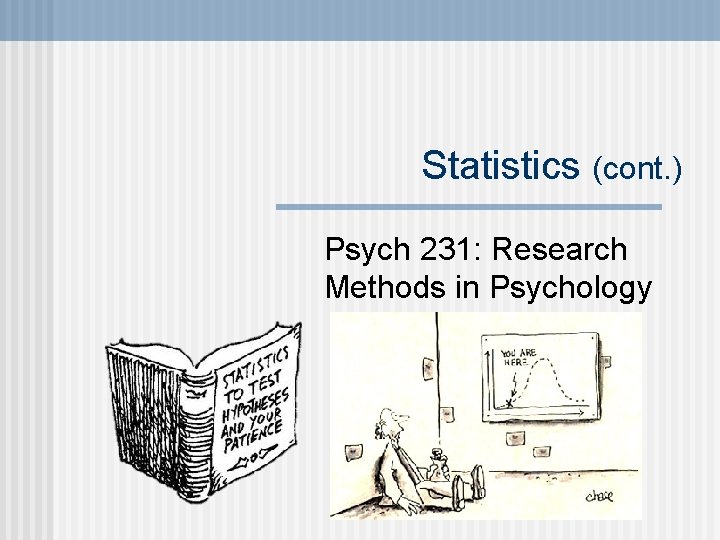
Statistics (cont. ) Psych 231: Research Methods in Psychology
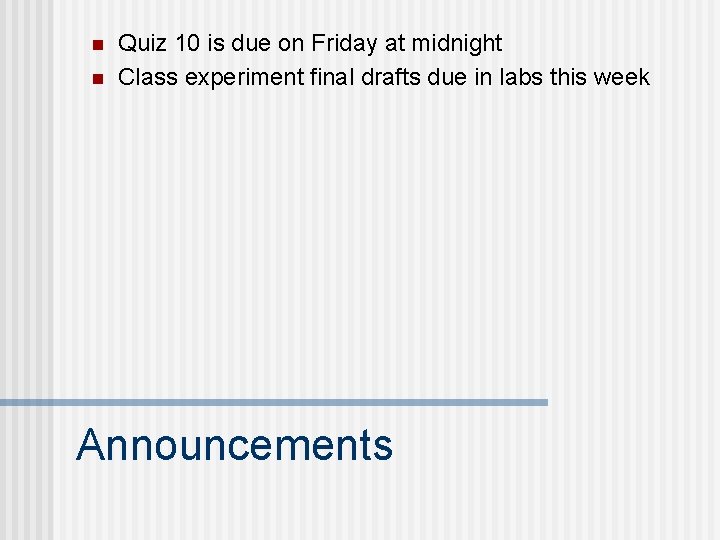
n n Quiz 10 is due on Friday at midnight Class experiment final drafts due in labs this week Announcements
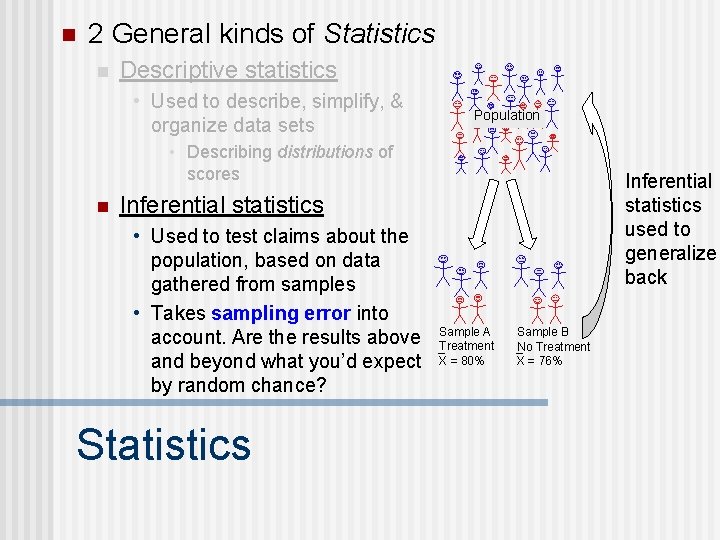
n 2 General kinds of Statistics n Descriptive statistics • Used to describe, simplify, & organize data sets Population • Describing distributions of scores n Inferential statistics used to generalize back Inferential statistics • Used to test claims about the population, based on data gathered from samples • Takes sampling error into account. Are the results above and beyond what you’d expect by random chance? Statistics Sample A Treatment X = 80% Sample B No Treatment X = 76%
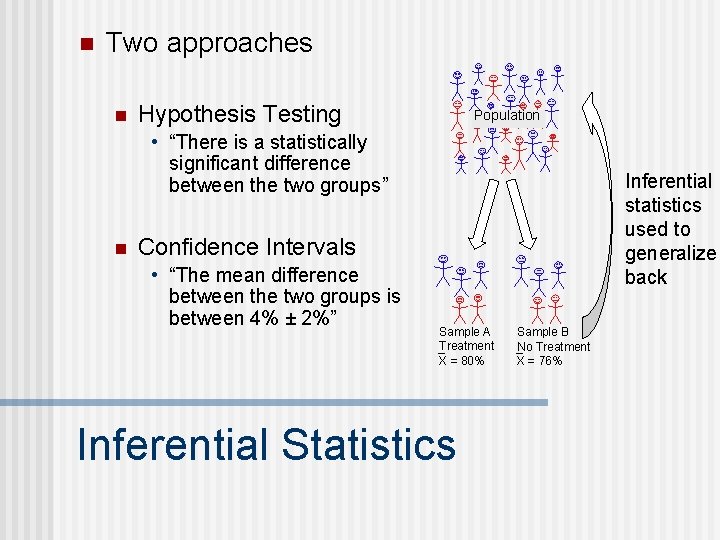
n Two approaches n Hypothesis Testing Population • “There is a statistically significant difference between the two groups” n Inferential statistics used to generalize back Confidence Intervals • “The mean difference between the two groups is between 4% ± 2%” Sample A Treatment X = 80% Inferential Statistics Sample B No Treatment X = 76%
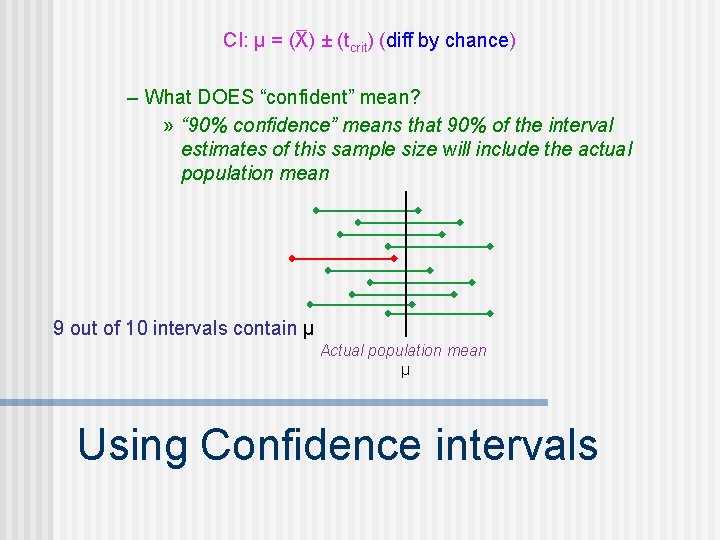
CI: μ = (X) ± (tcrit) (diff by chance) – What DOES “confident” mean? » “ 90% confidence” means that 90% of the interval estimates of this sample size will include the actual population mean 9 out of 10 intervals contain μ Actual population mean μ Using Confidence intervals
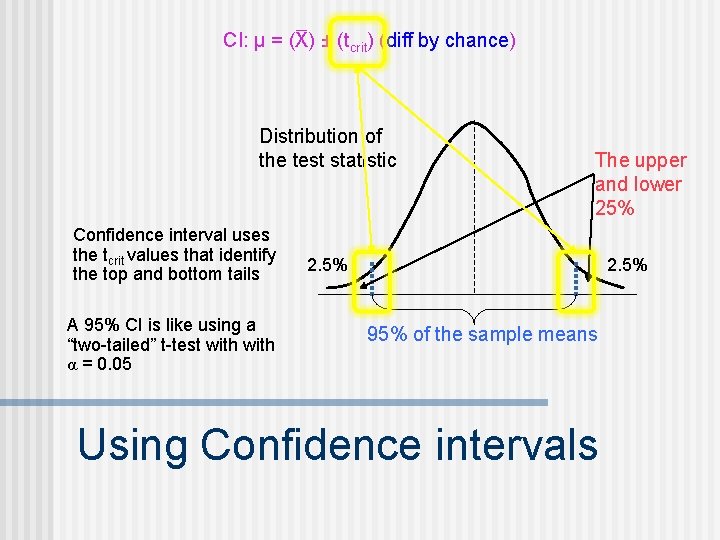
CI: μ = (X) ± (tcrit) (diff by chance) Distribution of the test statistic Confidence interval uses the tcrit values that identify the top and bottom tails A 95% CI is like using a “two-tailed” t-test with α = 0. 05 The upper and lower 25% 2. 5% 95% of the sample means Using Confidence intervals
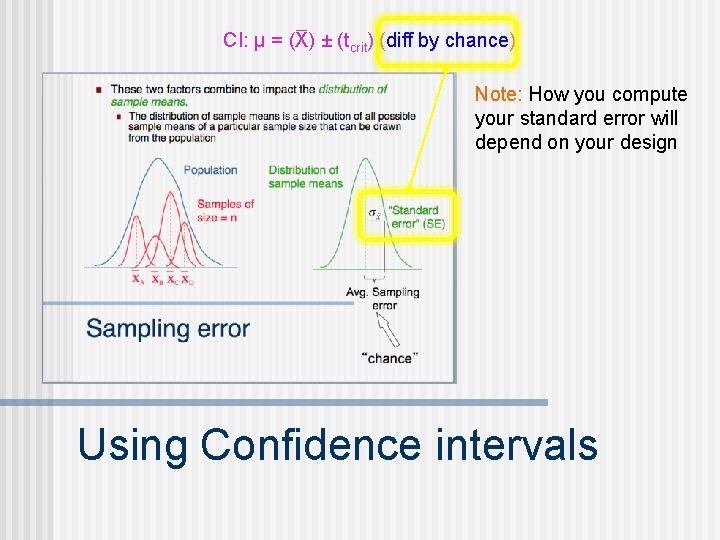
CI: μ = (X) ± (tcrit) (diff by chance) Note: How you compute your standard error will depend on your design Using Confidence intervals
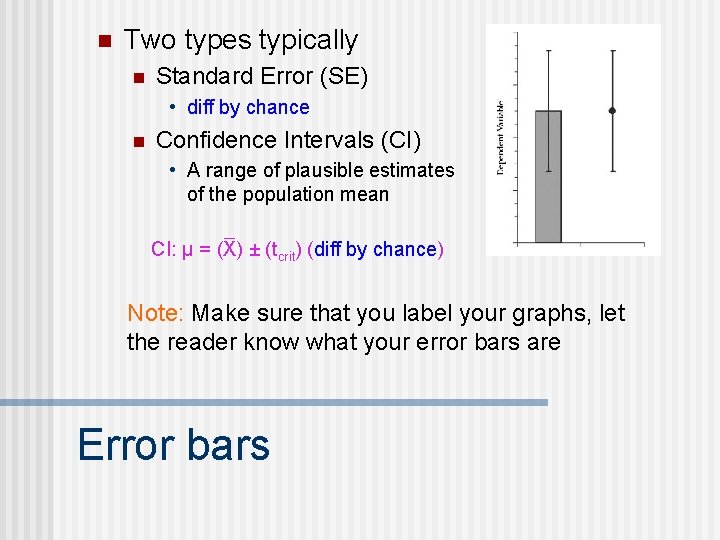
n Two types typically n Standard Error (SE) • diff by chance n Confidence Intervals (CI) • A range of plausible estimates of the population mean CI: μ = (X) ± (tcrit) (diff by chance) Note: Make sure that you label your graphs, let the reader know what your error bars are Error bars
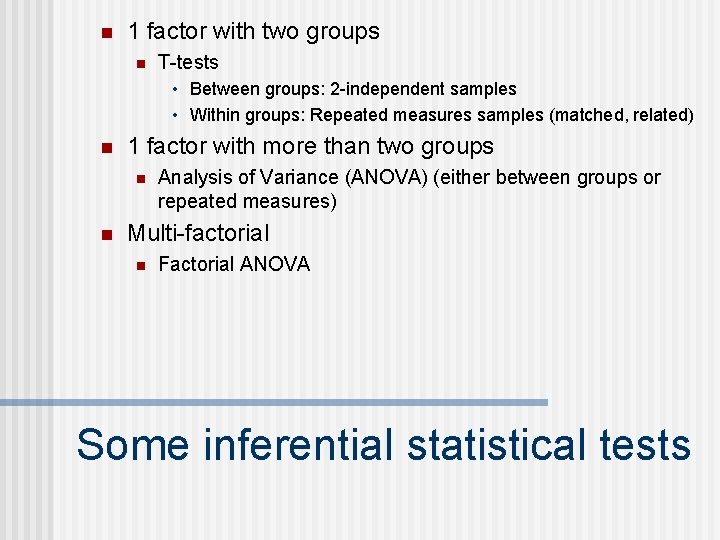
n 1 factor with two groups n T-tests • Between groups: 2 -independent samples • Within groups: Repeated measures samples (matched, related) n 1 factor with more than two groups n n Analysis of Variance (ANOVA) (either between groups or repeated measures) Multi-factorial n Factorial ANOVA Some inferential statistical tests
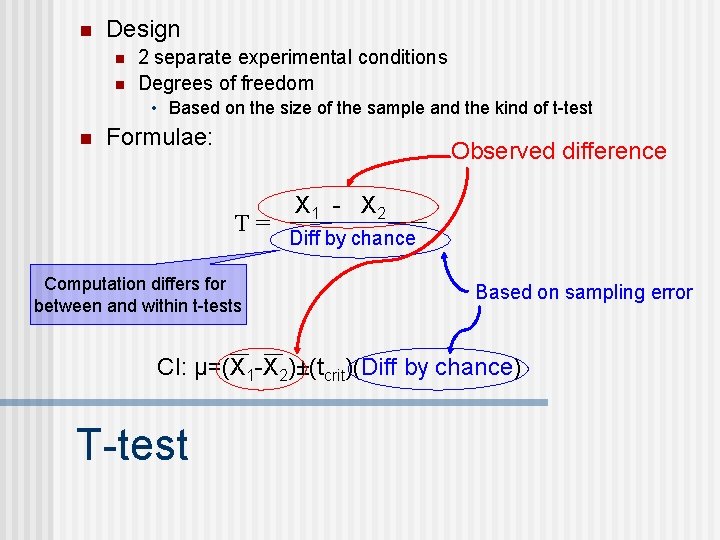
n Design n n 2 separate experimental conditions Degrees of freedom • Based on the size of the sample and the kind of t-test n Formulae: Observed difference T= Computation differs for between and within t-tests X 1 - X 2 Diff by chance Based on sampling error CI: μ=(X 1 -X 2)±(tcrit)(Diff by chance) T-test
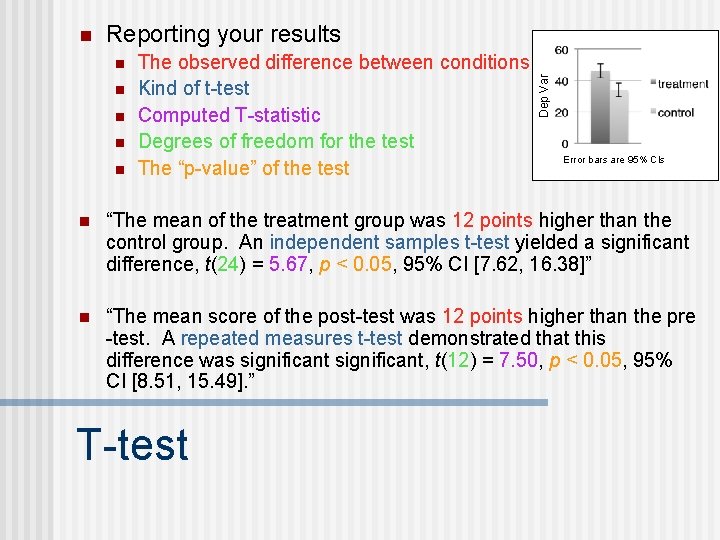
Reporting your results n n n The observed difference between conditions Kind of t-test Computed T-statistic Degrees of freedom for the test The “p-value” of the test Dep Var n Error bars are 95% CIs n “The mean of the treatment group was 12 points higher than the control group. An independent samples t-test yielded a significant difference, t(24) = 5. 67, p < 0. 05, 95% CI [7. 62, 16. 38]” n “The mean score of the post-test was 12 points higher than the pre -test. A repeated measures t-test demonstrated that this difference was significant, t(12) = 7. 50, p < 0. 05, 95% CI [8. 51, 15. 49]. ” T-test
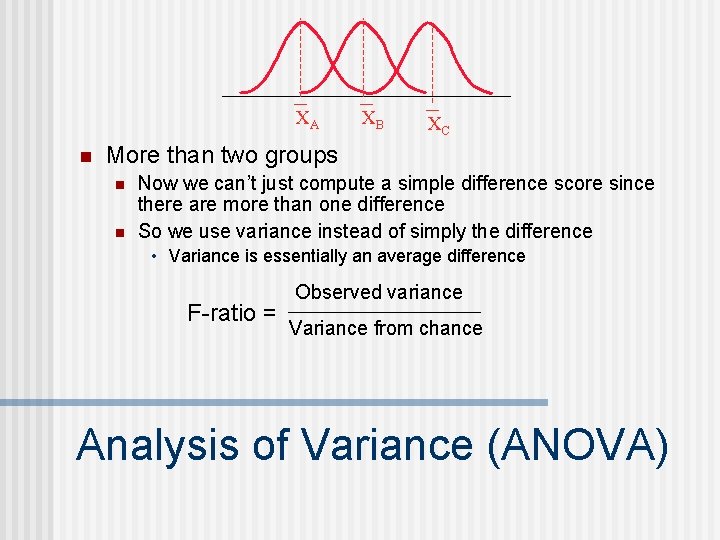
XA n XB XC More than two groups n n Now we can’t just compute a simple difference score since there are more than one difference So we use variance instead of simply the difference • Variance is essentially an average difference F-ratio = Observed variance Variance from chance Analysis of Variance (ANOVA)
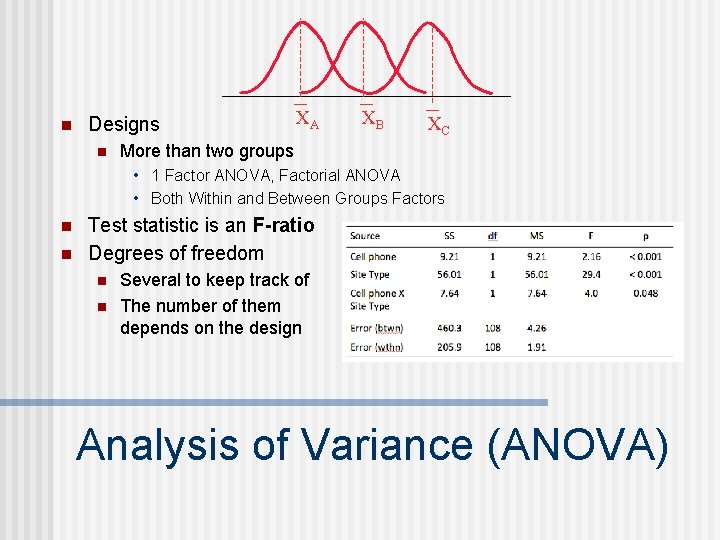
n Designs n XA XB XC More than two groups • 1 Factor ANOVA, Factorial ANOVA • Both Within and Between Groups Factors n n Test statistic is an F-ratio Degrees of freedom n n Several to keep track of The number of them depends on the design Analysis of Variance (ANOVA)
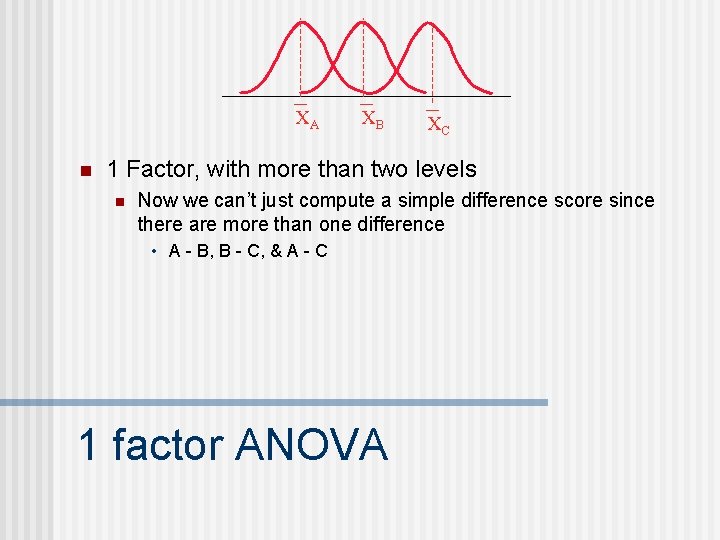
XA n XB XC 1 Factor, with more than two levels n Now we can’t just compute a simple difference score since there are more than one difference • A - B, B - C, & A - C 1 factor ANOVA
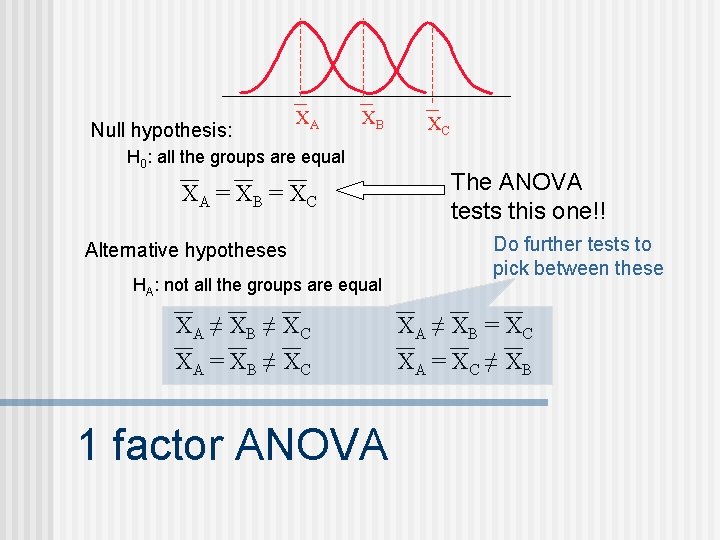
Null hypothesis: XA XB H 0: all the groups are equal XA = X B = X C Alternative hypotheses HA: not all the groups are equal XA ≠ X B ≠ X C XA = X B ≠ X C 1 factor ANOVA XC The ANOVA tests this one!! Do further tests to pick between these XA ≠ X B = X C XA = X C ≠ X B
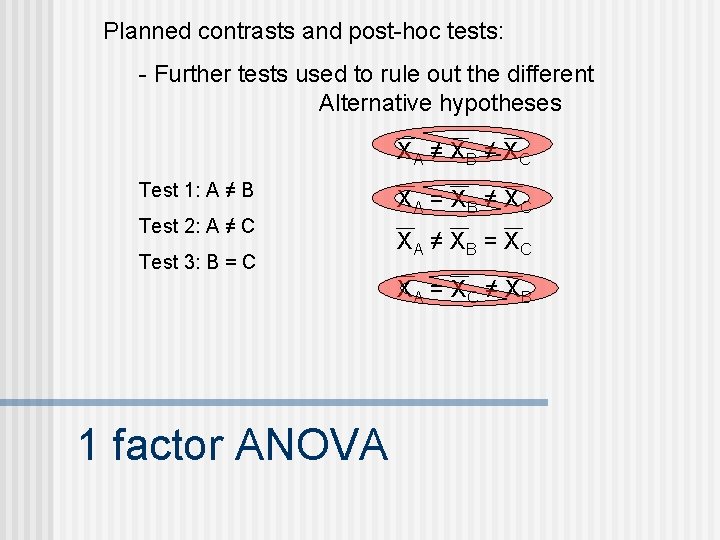
Planned contrasts and post-hoc tests: - Further tests used to rule out the different Alternative hypotheses XA ≠ X B ≠ X C Test 1: A ≠ B Test 2: A ≠ C Test 3: B = C XA = X B ≠ X C XA ≠ X B = X C XA = X C ≠ X B 1 factor ANOVA
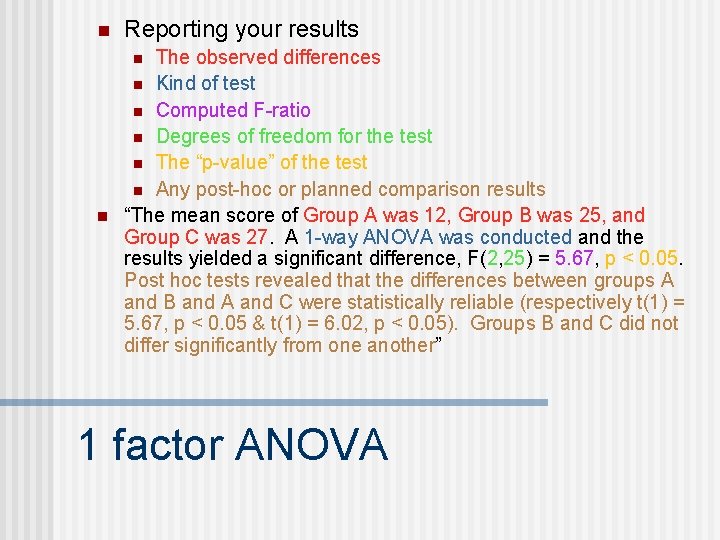
n Reporting your results The observed differences n Kind of test n Computed F-ratio n Degrees of freedom for the test n The “p-value” of the test n Any post-hoc or planned comparison results “The mean score of Group A was 12, Group B was 25, and Group C was 27. A 1 -way ANOVA was conducted and the results yielded a significant difference, F(2, 25) = 5. 67, p < 0. 05. Post hoc tests revealed that the differences between groups A and B and A and C were statistically reliable (respectively t(1) = 5. 67, p < 0. 05 & t(1) = 6. 02, p < 0. 05). Groups B and C did not differ significantly from one another” n n 1 factor ANOVA
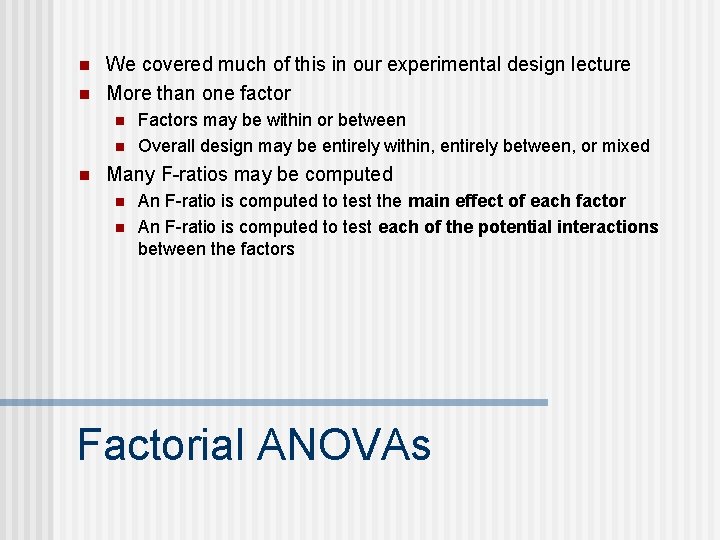
n n We covered much of this in our experimental design lecture More than one factor n n n Factors may be within or between Overall design may be entirely within, entirely between, or mixed Many F-ratios may be computed n n An F-ratio is computed to test the main effect of each factor An F-ratio is computed to test each of the potential interactions between the factors Factorial ANOVAs
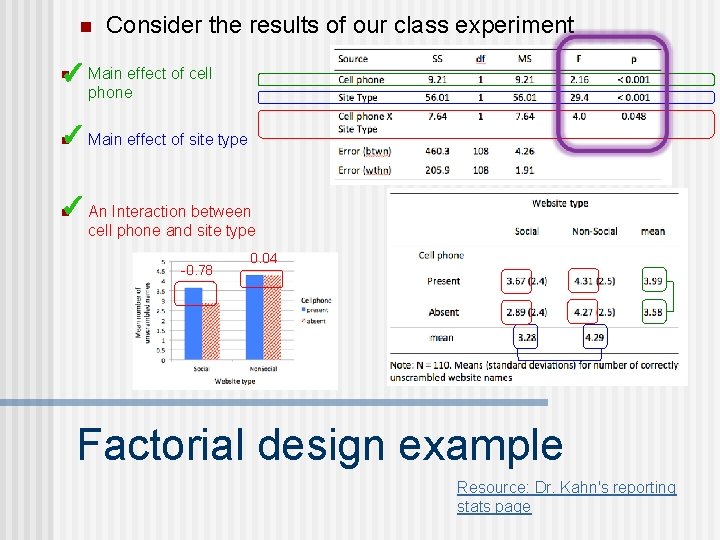
n Consider the results of our class experiment ✓ Main effect of cell n phone ✓ Main effect of site type n ✓ An Interaction between n cell phone and site type -0. 78 0. 04 Factorial design example Resource: Dr. Kahn's reporting stats page
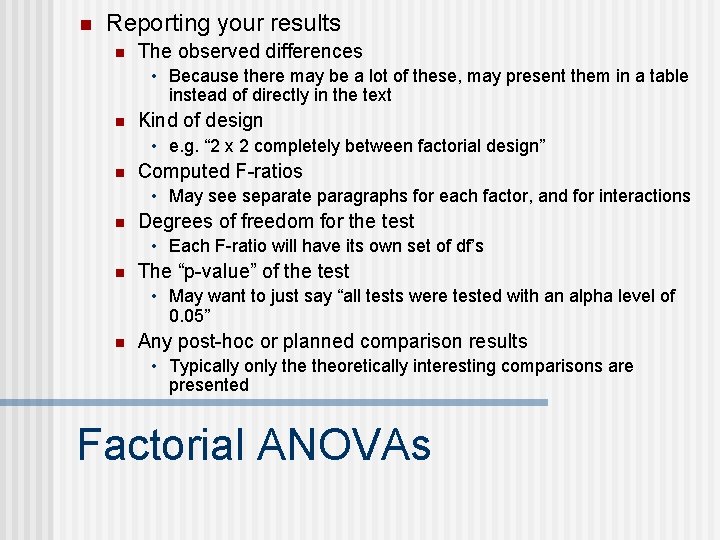
n Reporting your results n The observed differences • Because there may be a lot of these, may present them in a table instead of directly in the text n Kind of design • e. g. “ 2 x 2 completely between factorial design” n Computed F-ratios • May see separate paragraphs for each factor, and for interactions n Degrees of freedom for the test • Each F-ratio will have its own set of df’s n The “p-value” of the test • May want to just say “all tests were tested with an alpha level of 0. 05” n Any post-hoc or planned comparison results • Typically only theoretically interesting comparisons are presented Factorial ANOVAs
Debriefing report
Cont or cont'd
Positive psychology ap psych
Social loafing ap psychology definition
Method of social psychology
Research methods in developmental psychology
Research methods in abnormal psychology
Ocr psychology research methods
Psychology research methods worksheet answers
Introduction to statistics what is statistics
Descriptive statistics numerical measures
Sampling methods statistics
Tabular presentation of qualitative data
A-wax pattern recognition
Acf 231
Article 231 of the treaty of versailles
123 132 213 231 312 321
Pa msu
Decreto 231 del 2007
O primeiro natal letra
Gezang 231