Statistical Fundamentals Using Microsoft Excel for Univariate and
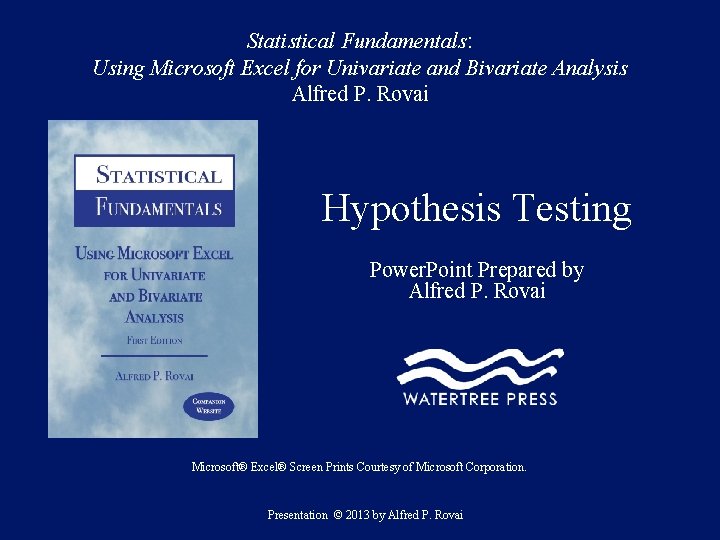
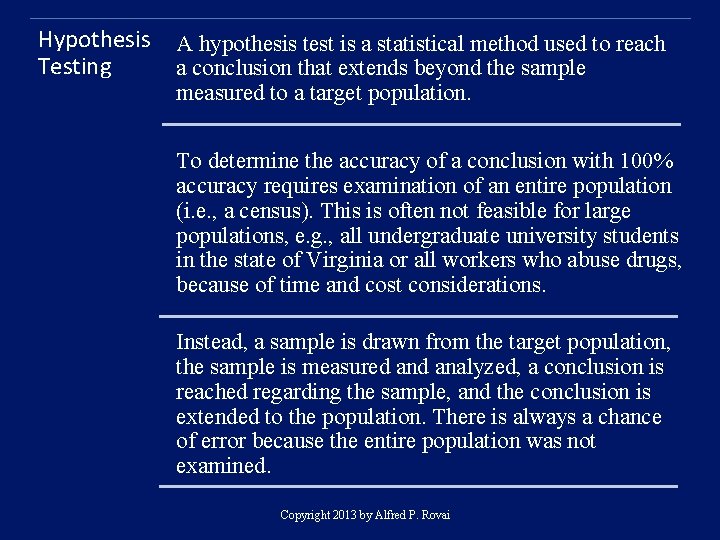
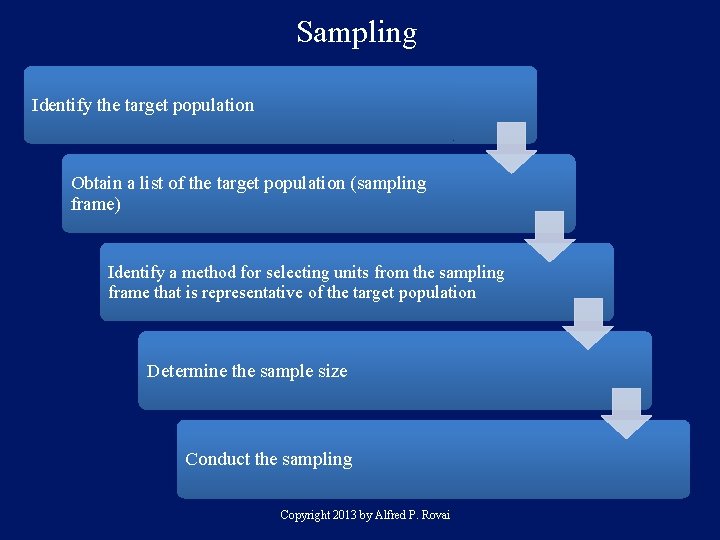
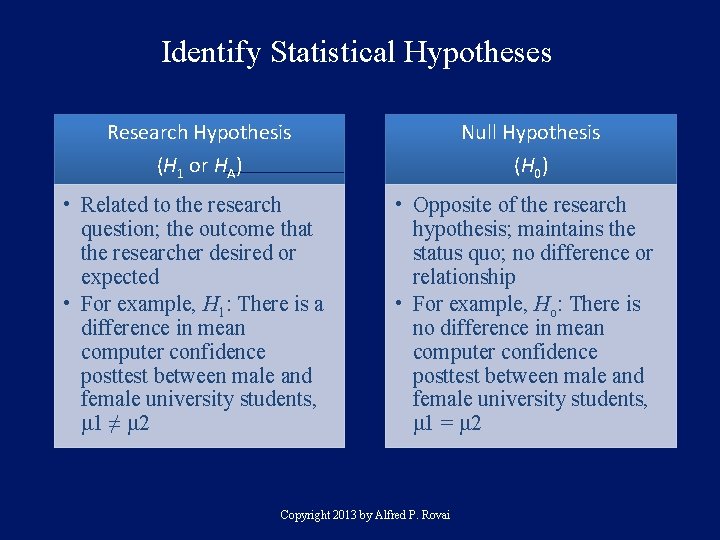
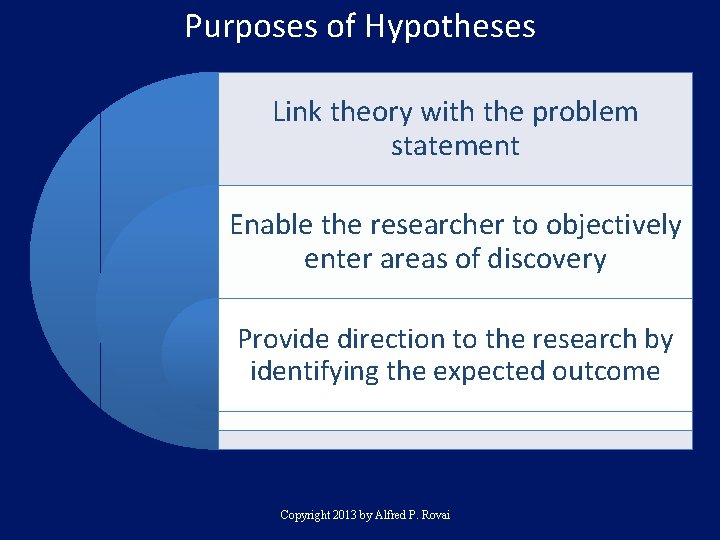
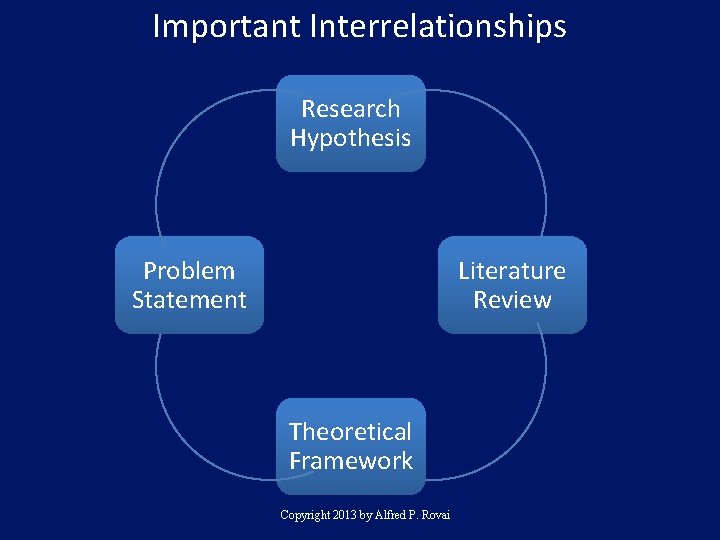
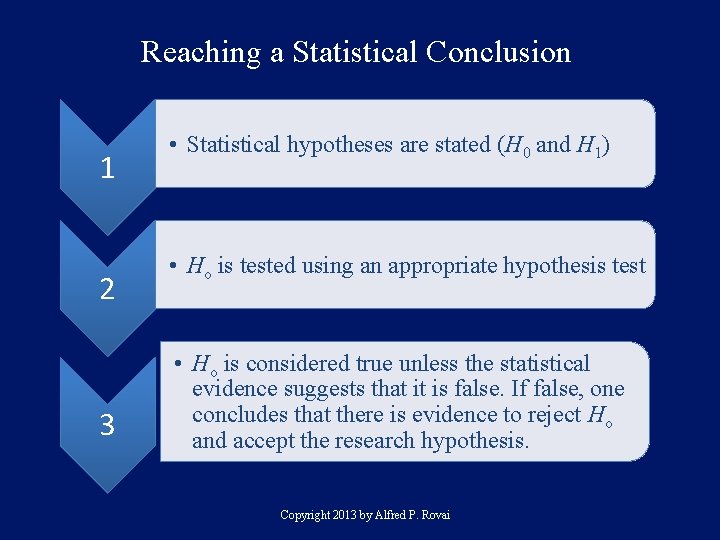
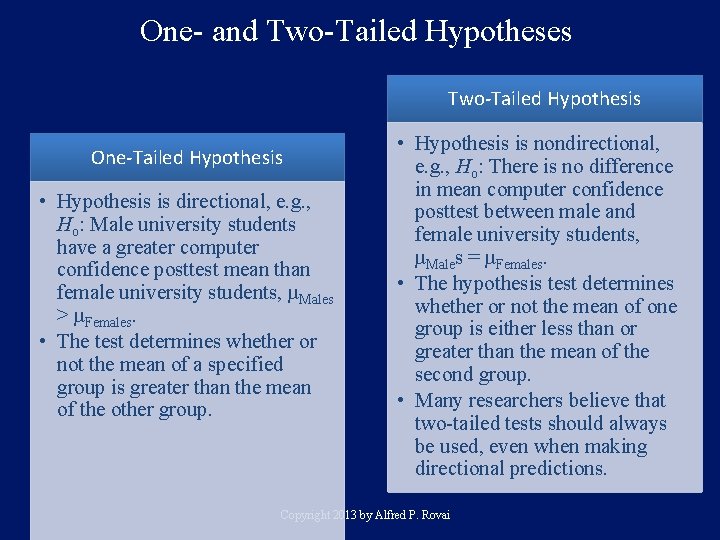
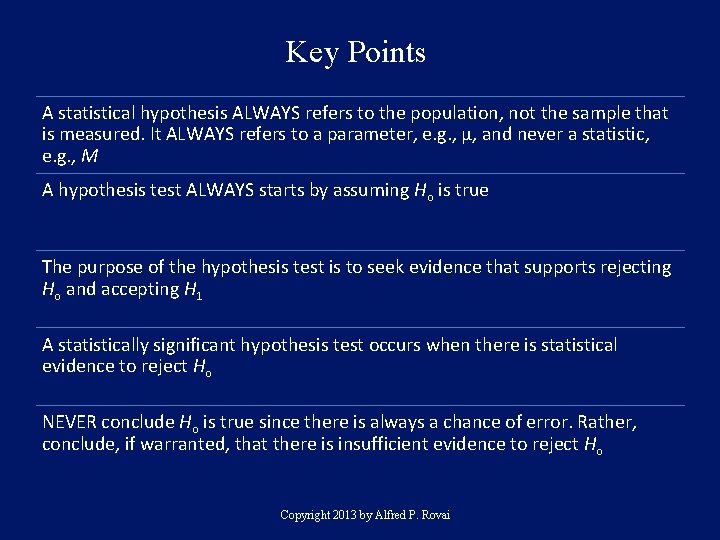
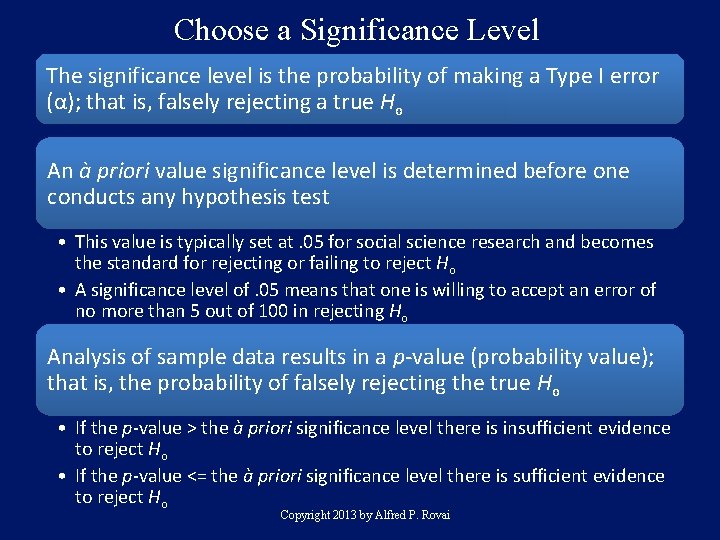
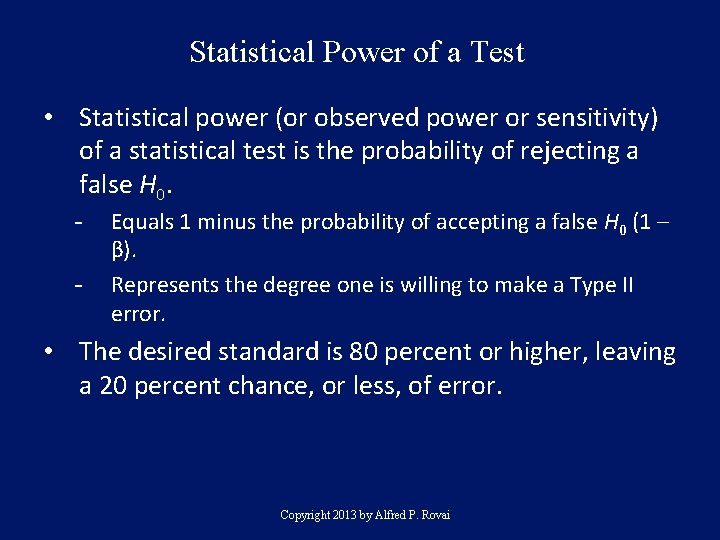
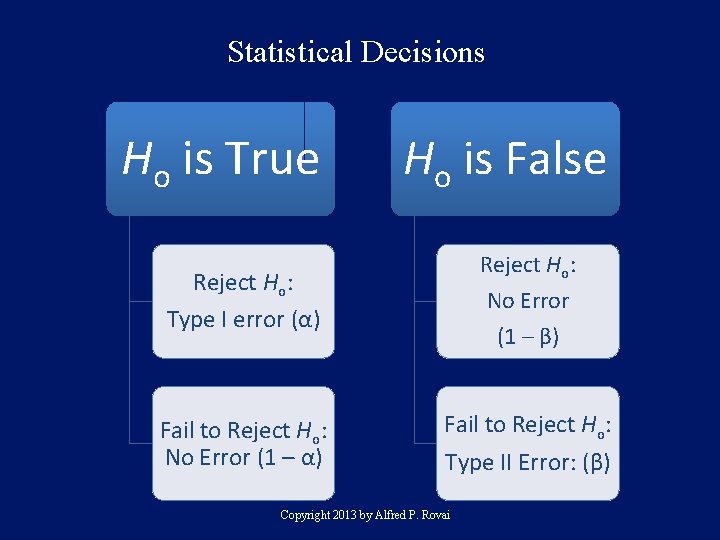
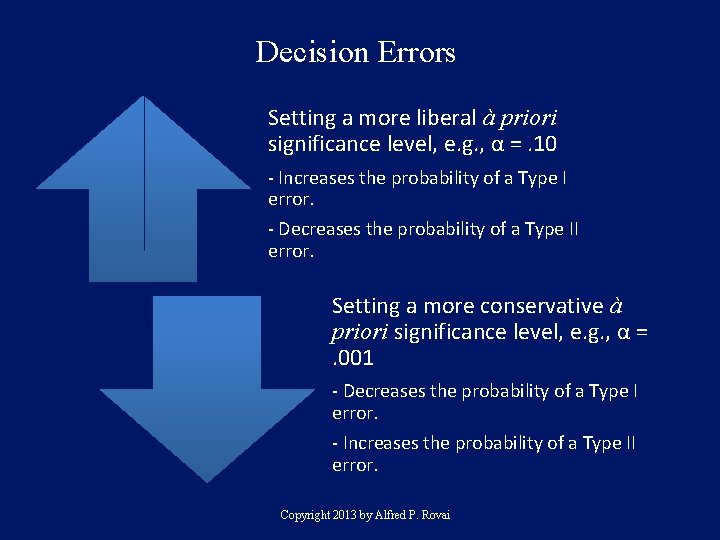
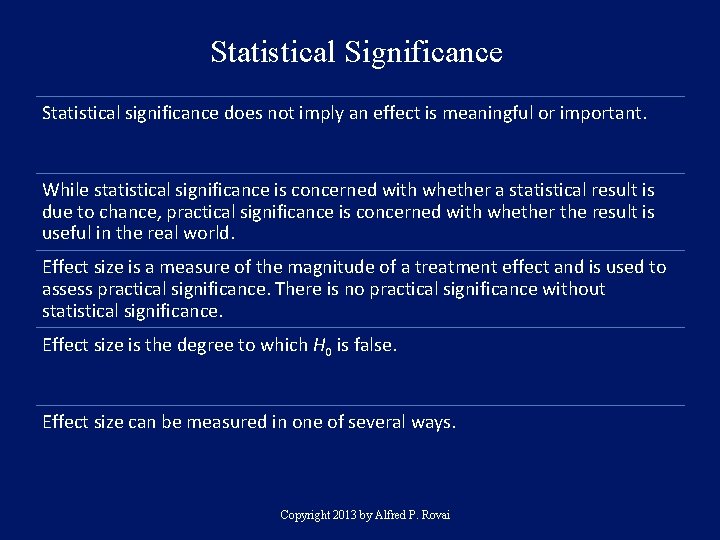
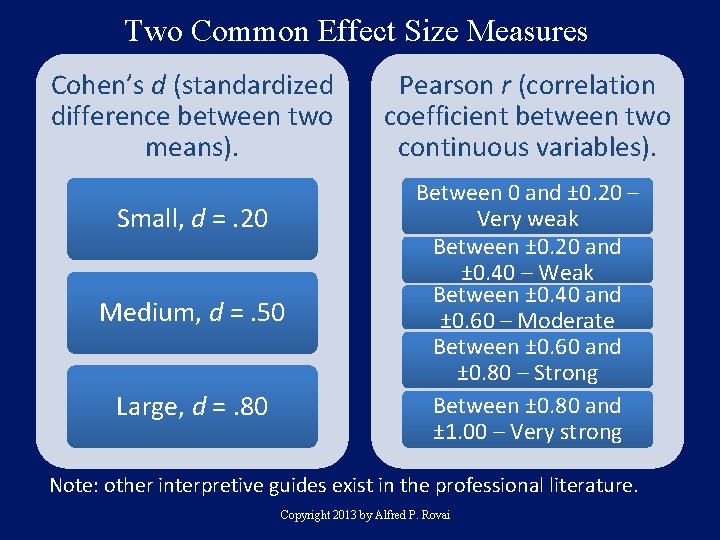
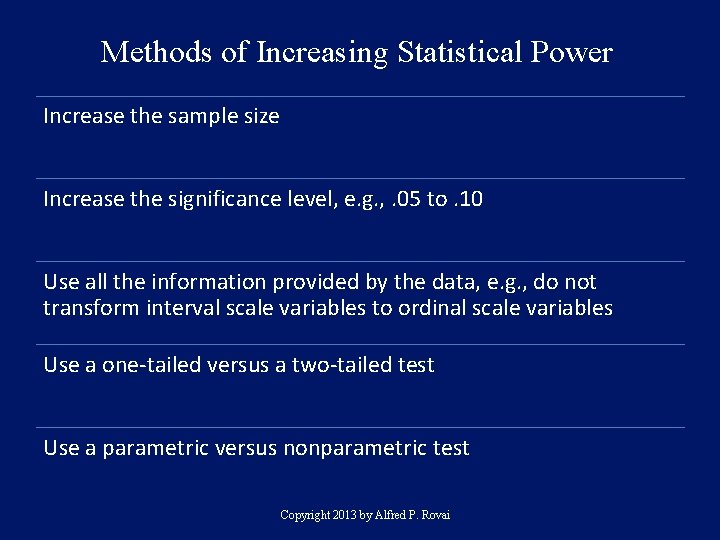
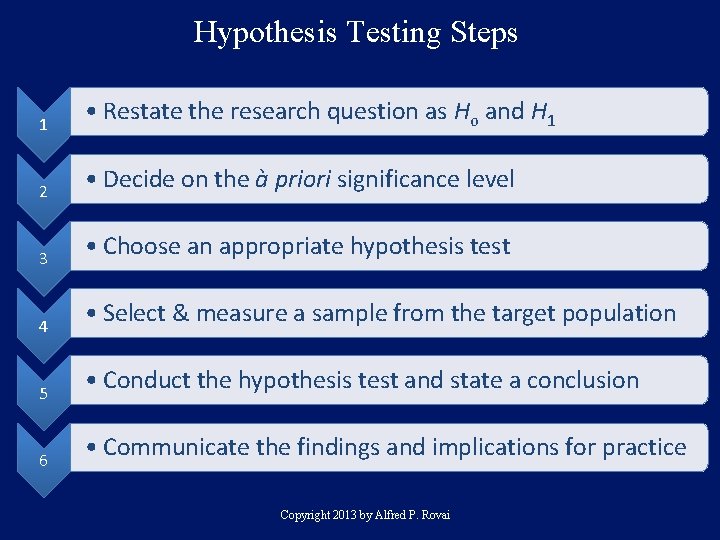
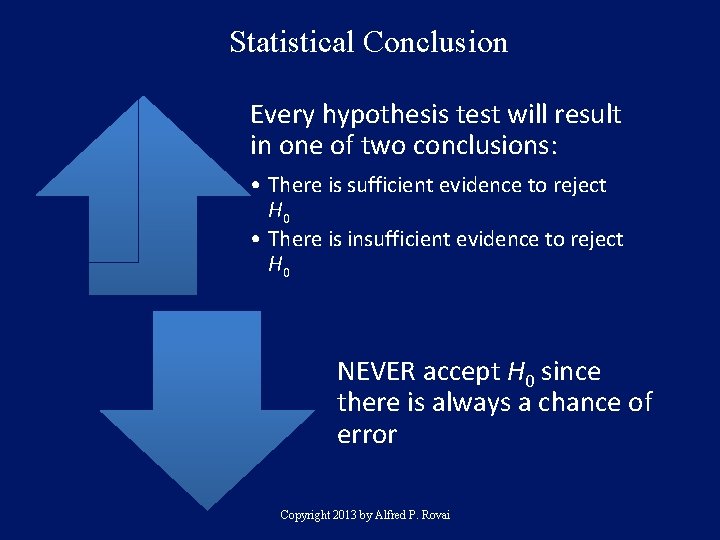
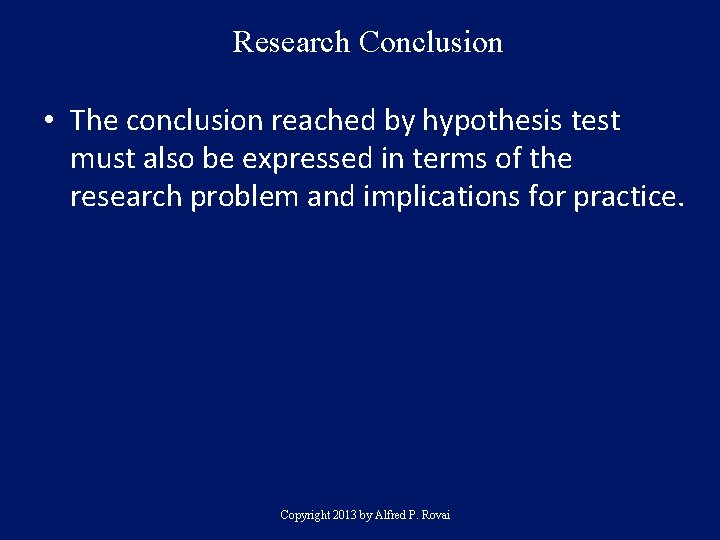
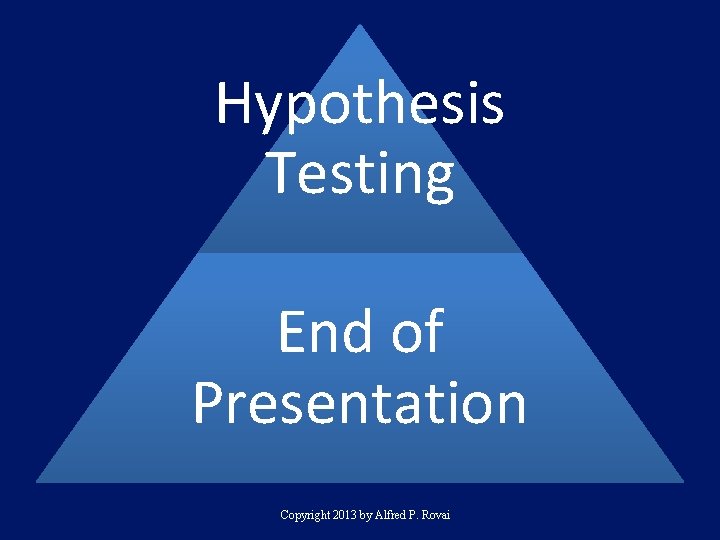
- Slides: 20
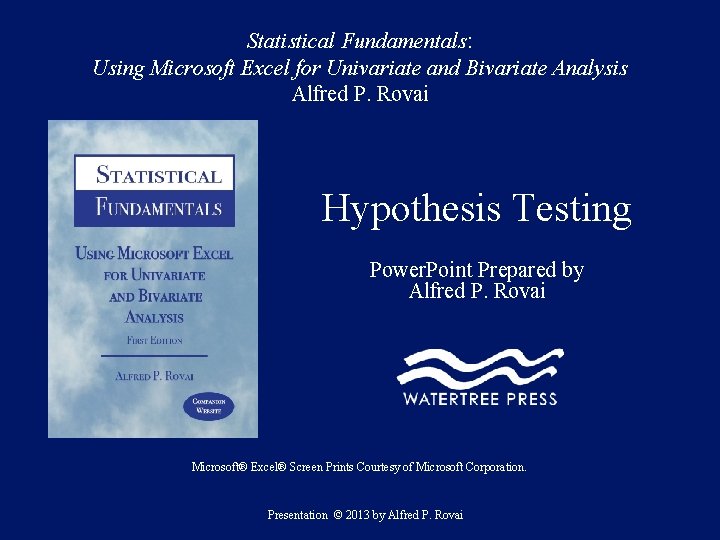
Statistical Fundamentals: Using Microsoft Excel for Univariate and Bivariate Analysis Alfred P. Rovai Hypothesis Testing Power. Point Prepared by Alfred P. Rovai Microsoft® Excel® Screen Prints Courtesy of Microsoft Corporation. Presentation © 2013 by Alfred P. Rovai
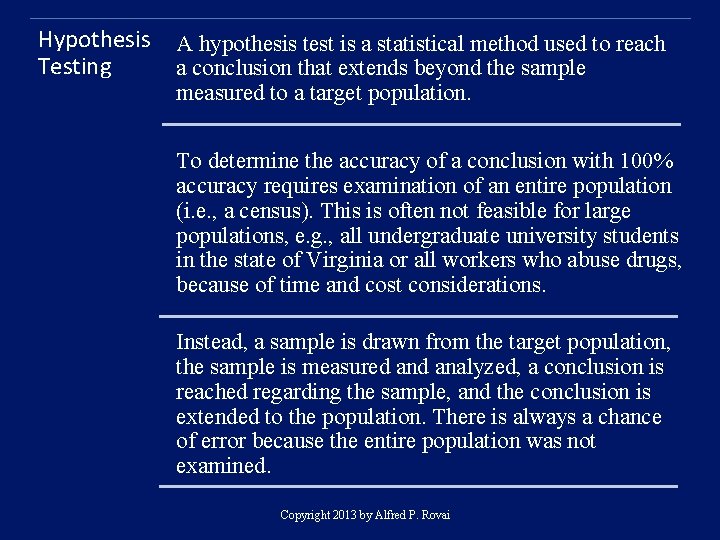
Hypothesis Testing A hypothesis test is a statistical method used to reach a conclusion that extends beyond the sample measured to a target population. To determine the accuracy of a conclusion with 100% accuracy requires examination of an entire population (i. e. , a census). This is often not feasible for large populations, e. g. , all undergraduate university students in the state of Virginia or all workers who abuse drugs, because of time and cost considerations. Instead, a sample is drawn from the target population, the sample is measured analyzed, a conclusion is reached regarding the sample, and the conclusion is extended to the population. There is always a chance of error because the entire population was not examined. Copyright 2013 by Alfred P. Rovai
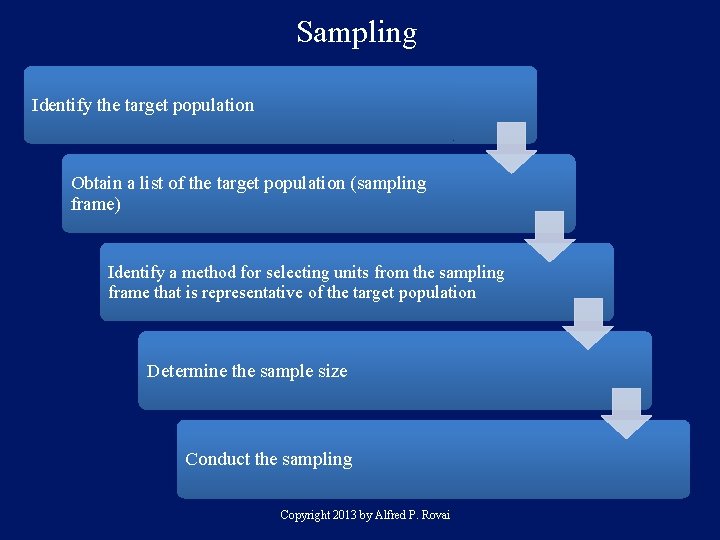
Sampling Identify the target population Obtain a list of the target population (sampling frame) Identify a method for selecting units from the sampling frame that is representative of the target population Determine the sample size Conduct the sampling Copyright 2013 by Alfred P. Rovai
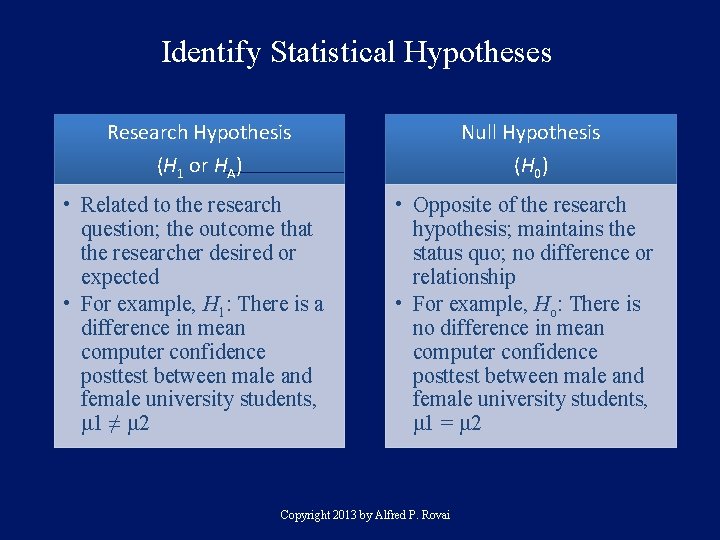
Identify Statistical Hypotheses Research Hypothesis (H 1 or HA) • Related to the research question; the outcome that the researcher desired or expected • For example, H 1: There is a difference in mean computer confidence posttest between male and female university students, μ 1 ≠ μ 2 Null Hypothesis (H 0) • Opposite of the research hypothesis; maintains the status quo; no difference or relationship • For example, Ho: There is no difference in mean computer confidence posttest between male and female university students, μ 1 = μ 2 Copyright 2013 by Alfred P. Rovai
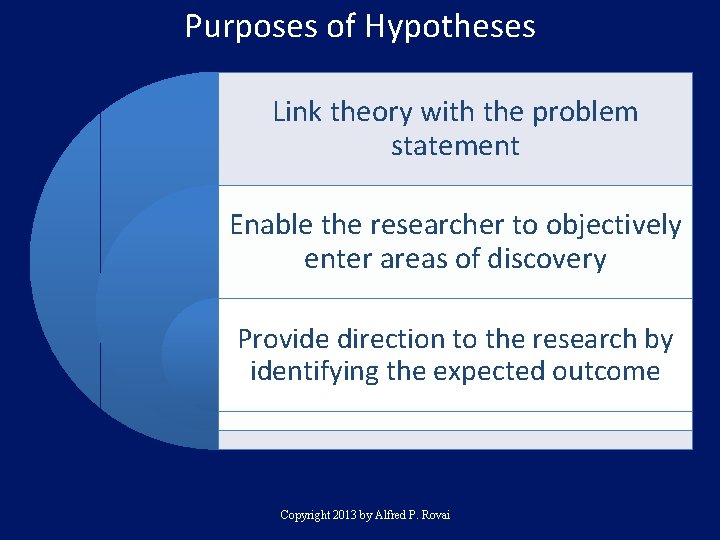
Purposes of Hypotheses Link theory with the problem statement Enable the researcher to objectively enter areas of discovery Provide direction to the research by identifying the expected outcome Copyright 2013 by Alfred P. Rovai
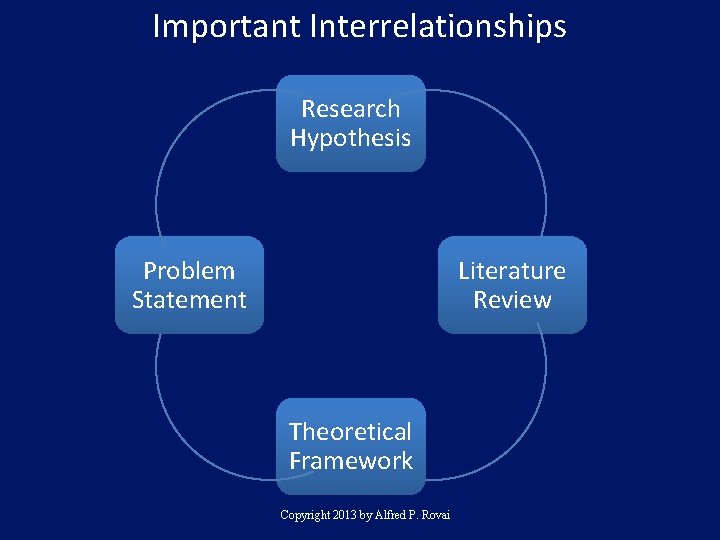
Important Interrelationships Research Hypothesis Problem Statement Literature Review Theoretical Framework Copyright 2013 by Alfred P. Rovai
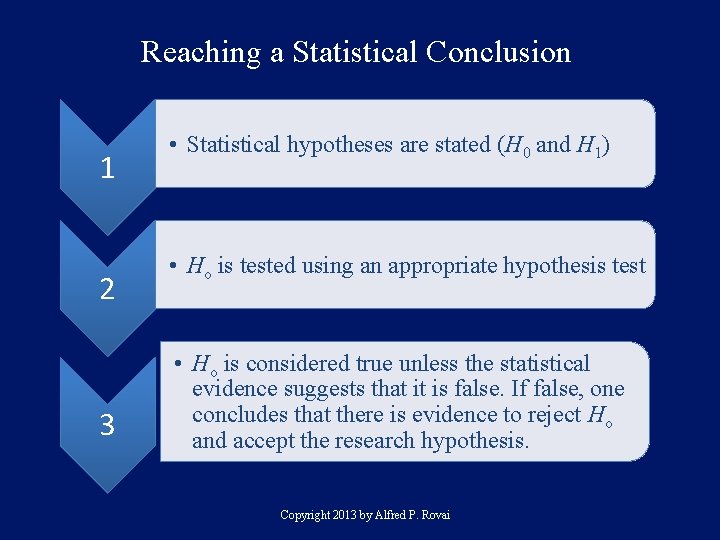
Reaching a Statistical Conclusion 1 2 3 • Statistical hypotheses are stated (H 0 and H 1) • Ho is tested using an appropriate hypothesis test • Ho is considered true unless the statistical evidence suggests that it is false. If false, one concludes that there is evidence to reject Ho and accept the research hypothesis. Copyright 2013 by Alfred P. Rovai
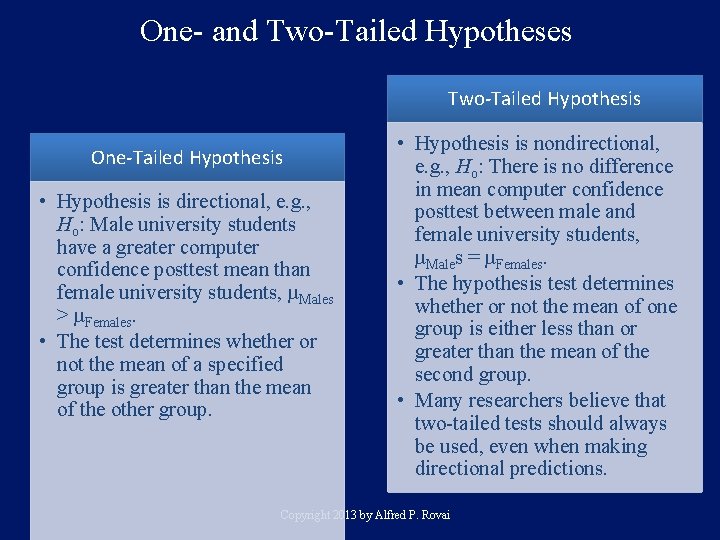
One- and Two-Tailed Hypotheses Two-Tailed Hypothesis One-Tailed Hypothesis • Hypothesis is directional, e. g. , Ho: Male university students have a greater computer confidence posttest mean than female university students, μMales > μFemales. • The test determines whether or not the mean of a specified group is greater than the mean of the other group. • Hypothesis is nondirectional, e. g. , Ho: There is no difference in mean computer confidence posttest between male and female university students, μMales = μFemales. • The hypothesis test determines whether or not the mean of one group is either less than or greater than the mean of the second group. • Many researchers believe that two-tailed tests should always be used, even when making directional predictions. Copyright 2013 by Alfred P. Rovai
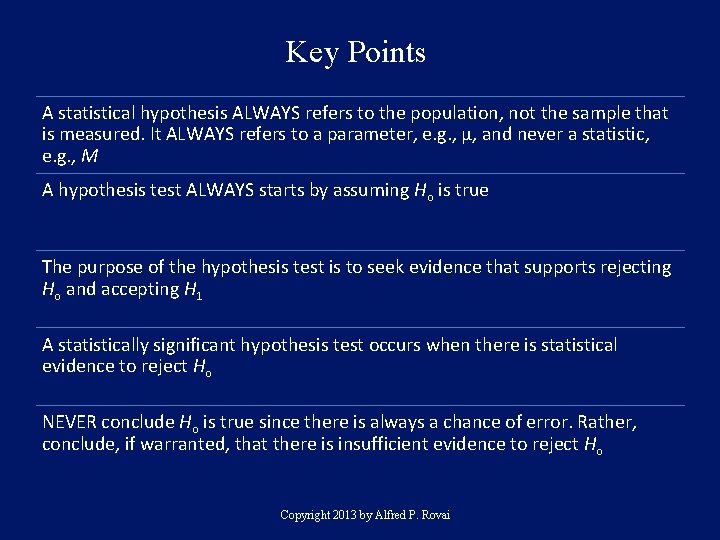
Key Points A statistical hypothesis ALWAYS refers to the population, not the sample that is measured. It ALWAYS refers to a parameter, e. g. , μ, and never a statistic, e. g. , M A hypothesis test ALWAYS starts by assuming Ho is true The purpose of the hypothesis test is to seek evidence that supports rejecting Ho and accepting H 1 A statistically significant hypothesis test occurs when there is statistical evidence to reject Ho NEVER conclude Ho is true since there is always a chance of error. Rather, conclude, if warranted, that there is insufficient evidence to reject Ho Copyright 2013 by Alfred P. Rovai
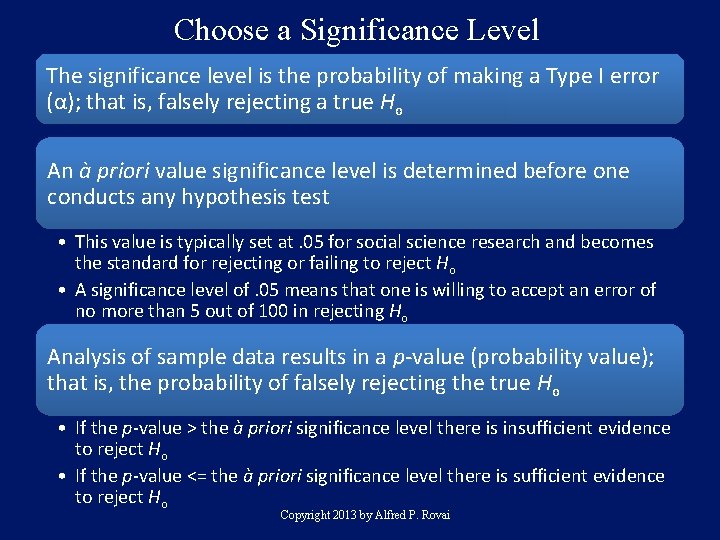
Choose a Significance Level The significance level is the probability of making a Type I error (α); that is, falsely rejecting a true Ho An à priori value significance level is determined before one conducts any hypothesis test • This value is typically set at. 05 for social science research and becomes the standard for rejecting or failing to reject Ho • A significance level of. 05 means that one is willing to accept an error of no more than 5 out of 100 in rejecting Ho Analysis of sample data results in a p-value (probability value); that is, the probability of falsely rejecting the true Ho • If the p-value > the à priori significance level there is insufficient evidence to reject Ho • If the p-value <= the à priori significance level there is sufficient evidence to reject Ho Copyright 2013 by Alfred P. Rovai
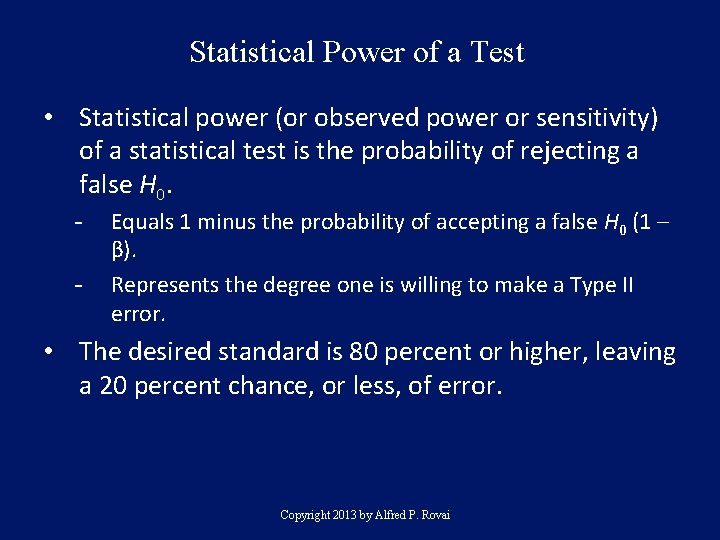
Statistical Power of a Test • Statistical power (or observed power or sensitivity) of a statistical test is the probability of rejecting a false H 0. - Equals 1 minus the probability of accepting a false H 0 (1 – β). Represents the degree one is willing to make a Type II error. • The desired standard is 80 percent or higher, leaving a 20 percent chance, or less, of error. Copyright 2013 by Alfred P. Rovai
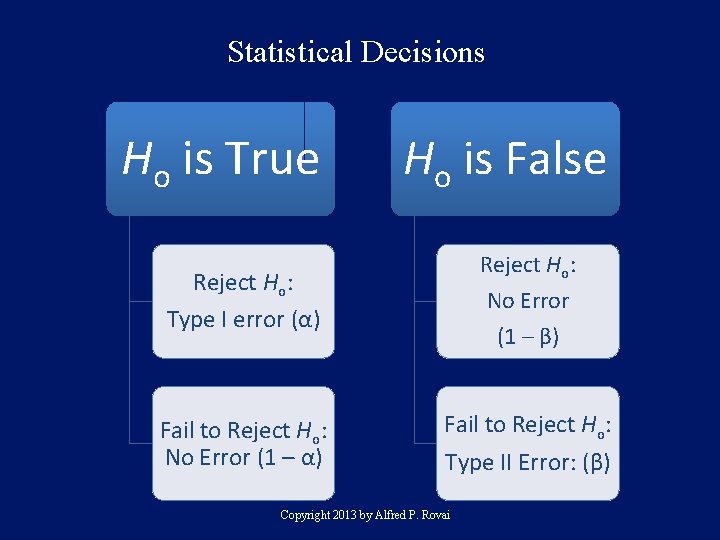
Statistical Decisions Ho is True Ho is False Reject Ho: Type I error (α) Reject Ho: No Error (1 – β) Fail to Reject Ho: No Error (1 – α) Fail to Reject Ho: Type II Error: (β) Copyright 2013 by Alfred P. Rovai
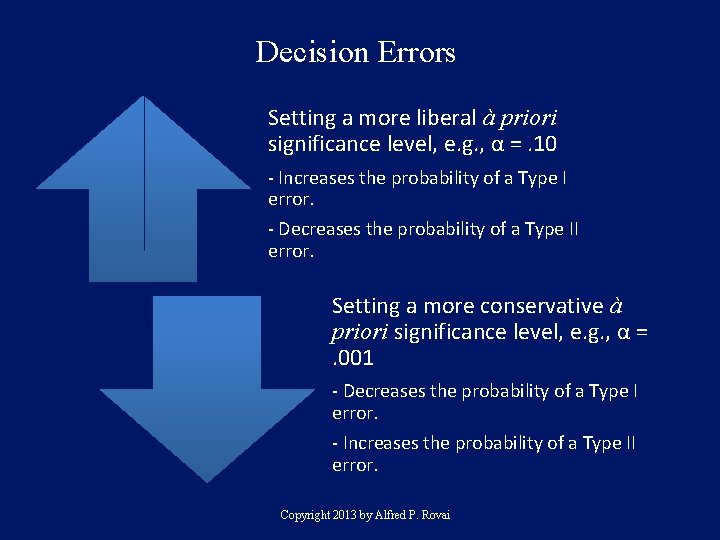
Decision Errors Setting a more liberal à priori significance level, e. g. , α =. 10 - Increases the probability of a Type I error. - Decreases the probability of a Type II error. Setting a more conservative à priori significance level, e. g. , α =. 001 - Decreases the probability of a Type I error. - Increases the probability of a Type II error. Copyright 2013 by Alfred P. Rovai
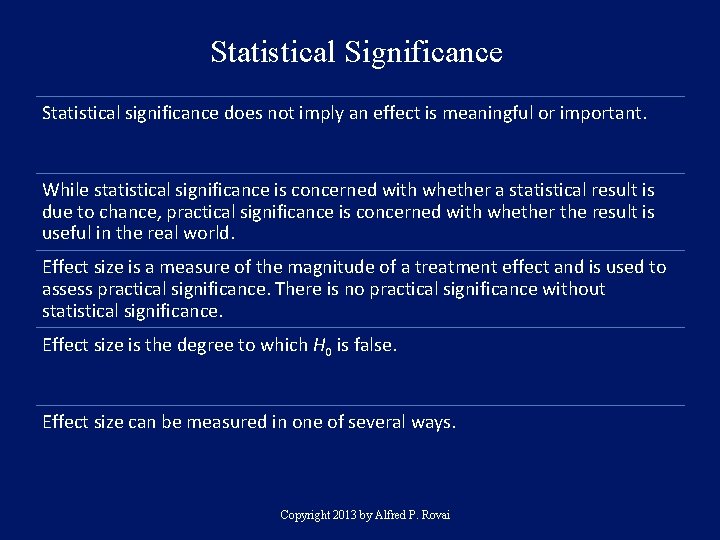
Statistical Significance Statistical significance does not imply an effect is meaningful or important. While statistical significance is concerned with whether a statistical result is due to chance, practical significance is concerned with whether the result is useful in the real world. Effect size is a measure of the magnitude of a treatment effect and is used to assess practical significance. There is no practical significance without statistical significance. Effect size is the degree to which H 0 is false. Effect size can be measured in one of several ways. Copyright 2013 by Alfred P. Rovai
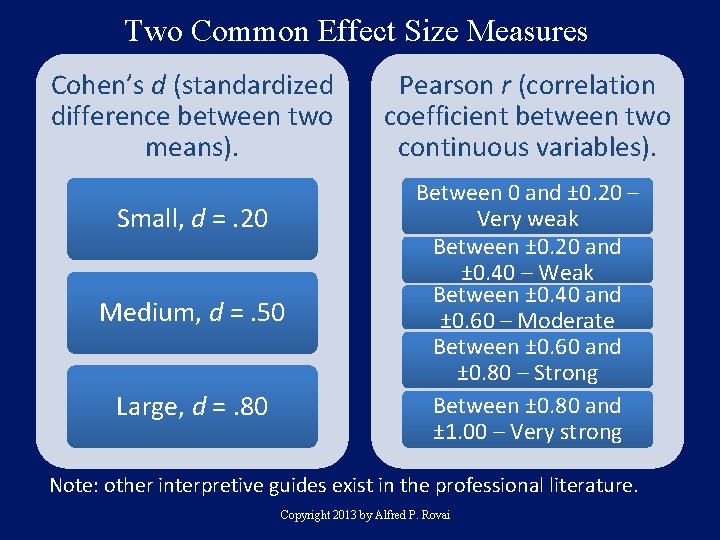
Two Common Effect Size Measures Cohen’s d (standardized difference between two means). Small, d =. 20 Medium, d =. 50 Large, d =. 80 Pearson r (correlation coefficient between two continuous variables). Between 0 and ± 0. 20 – Very weak Between ± 0. 20 and ± 0. 40 – Weak Between ± 0. 40 and ± 0. 60 – Moderate Between ± 0. 60 and ± 0. 80 – Strong Between ± 0. 80 and ± 1. 00 – Very strong Note: other interpretive guides exist in the professional literature. Copyright 2013 by Alfred P. Rovai
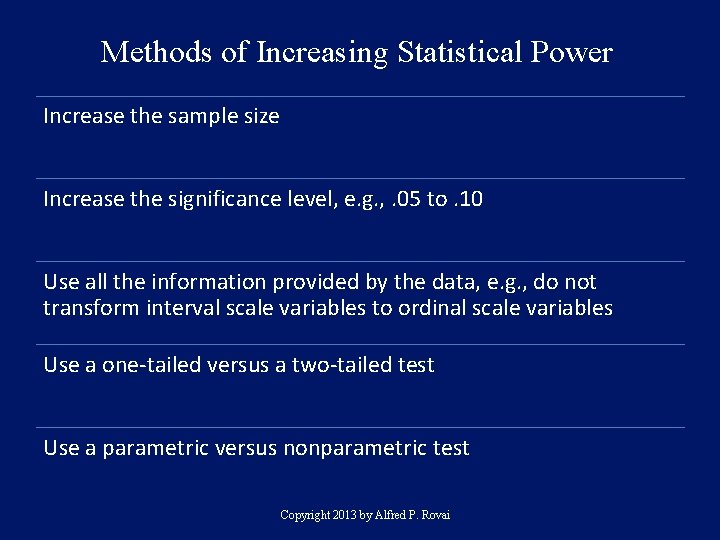
Methods of Increasing Statistical Power Increase the sample size Increase the significance level, e. g. , . 05 to. 10 Use all the information provided by the data, e. g. , do not transform interval scale variables to ordinal scale variables Use a one-tailed versus a two-tailed test Use a parametric versus nonparametric test Copyright 2013 by Alfred P. Rovai
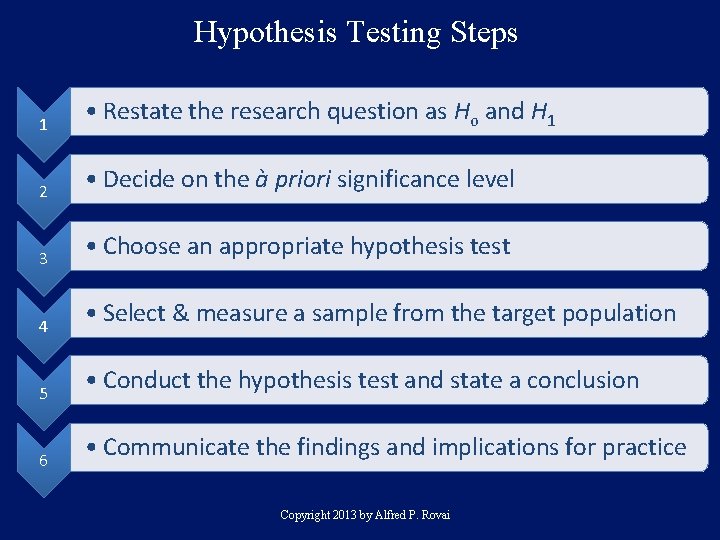
Hypothesis Testing Steps 1 • Restate the research question as Ho and H 1 2 • Decide on the à priori significance level 3 • Choose an appropriate hypothesis test 4 • Select & measure a sample from the target population 5 • Conduct the hypothesis test and state a conclusion 6 • Communicate the findings and implications for practice Copyright 2013 by Alfred P. Rovai
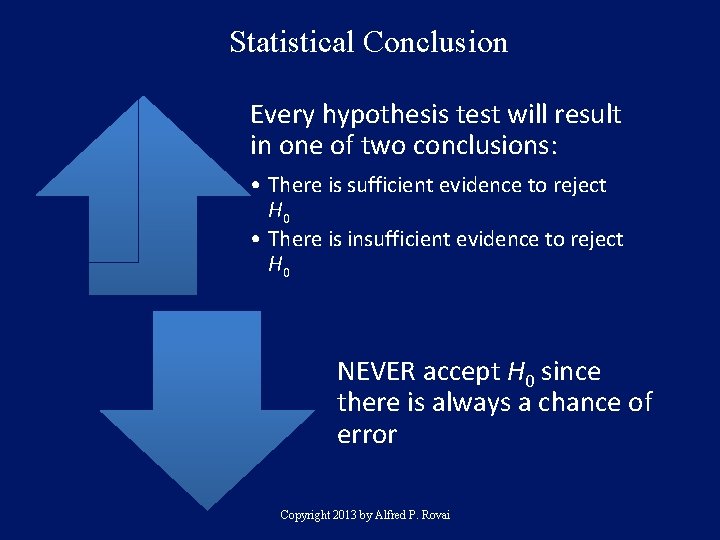
Statistical Conclusion Every hypothesis test will result in one of two conclusions: • There is sufficient evidence to reject H 0 • There is insufficient evidence to reject H 0 NEVER accept H 0 since there is always a chance of error Copyright 2013 by Alfred P. Rovai
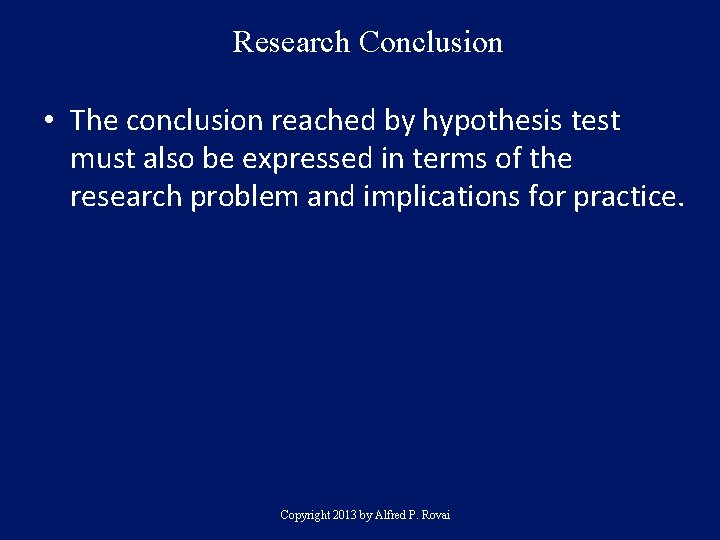
Research Conclusion • The conclusion reached by hypothesis test must also be expressed in terms of the research problem and implications for practice. Copyright 2013 by Alfred P. Rovai
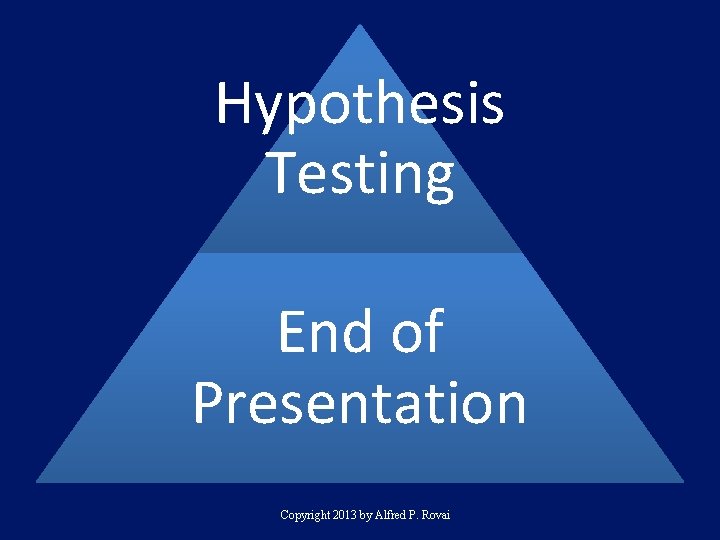
Hypothesis Testing End of Presentation Copyright 2013 by Alfred P. Rovai
Univariate analysis tests
Univariate analysis excel
Microsoft official academic course microsoft excel 2016
Microsoft excel merupakan program aplikasi….
Contoh univariat dan bivariat
Univariate verfahren
Univariate analysis spss
Univariate eda
Univariate transformation
Univariate vs multivariate logistic regression
Univariate anova
Experimental vs non experimental
Univariate vs multivariate
Statistical functions in excel
Using statistical measures to compare populations
Introduction to ms word
Microsoft sql fundamentals
Kontinuitetshantering
Typiska drag för en novell
Tack för att ni lyssnade bild
Ekologiskt fotavtryck