Social Research Methods Experiments 1 Experiments l One
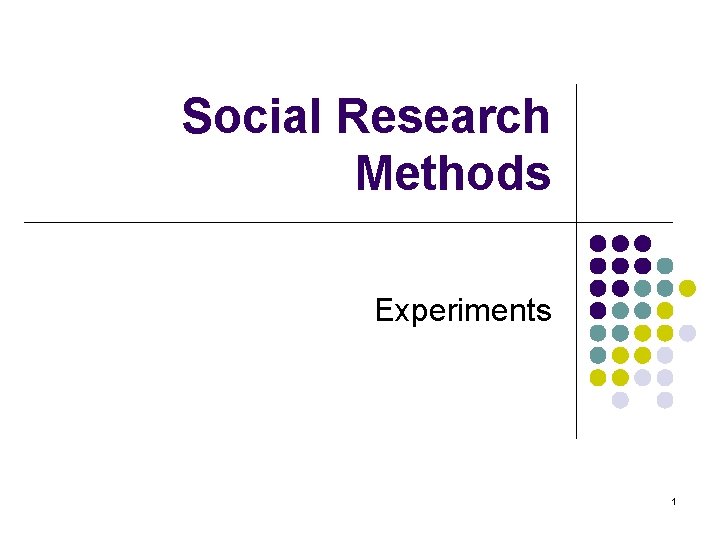
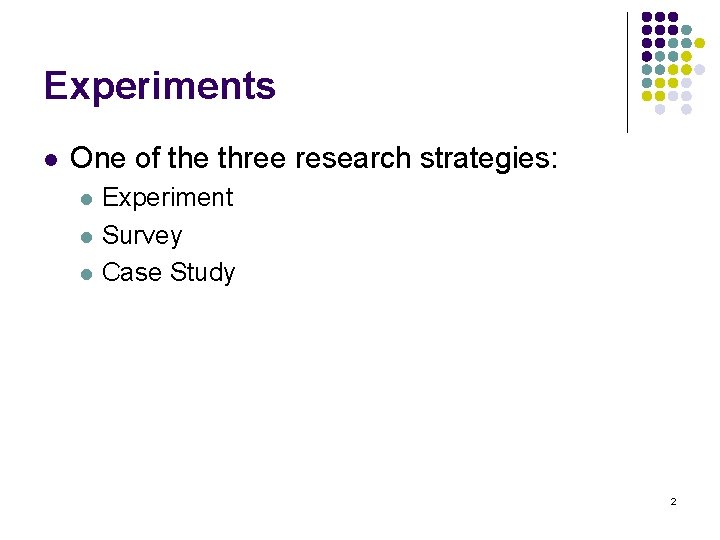
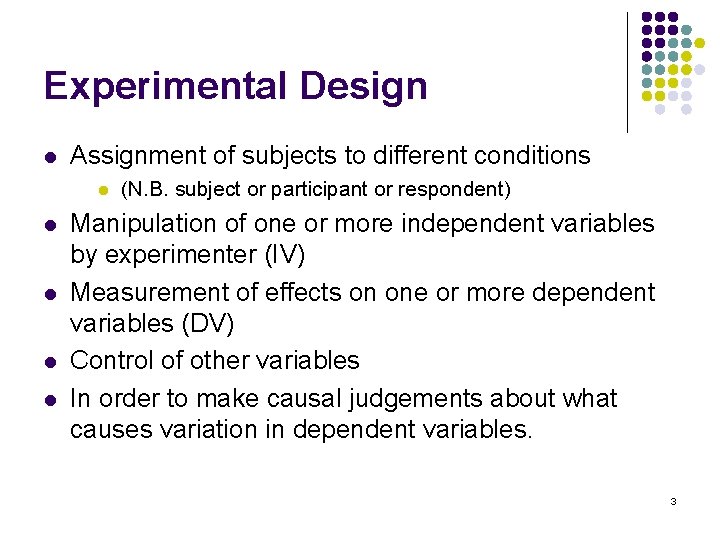
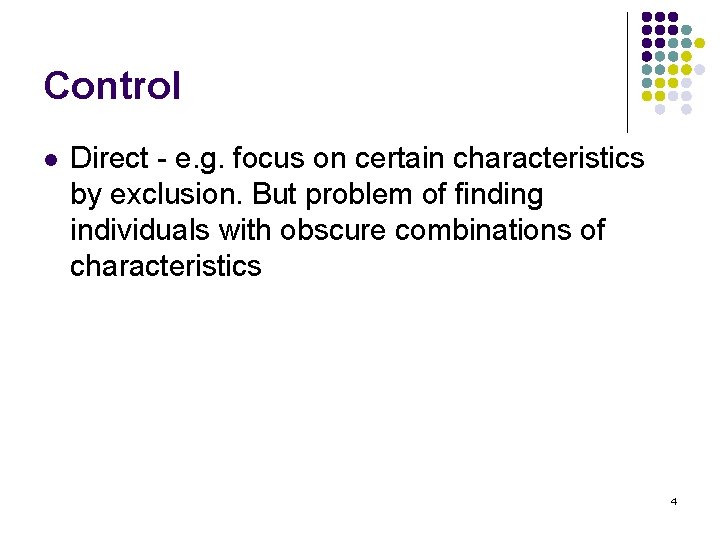
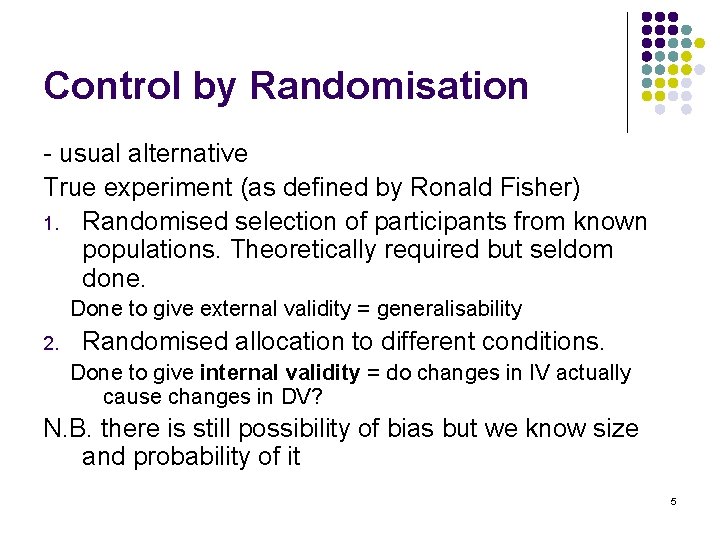
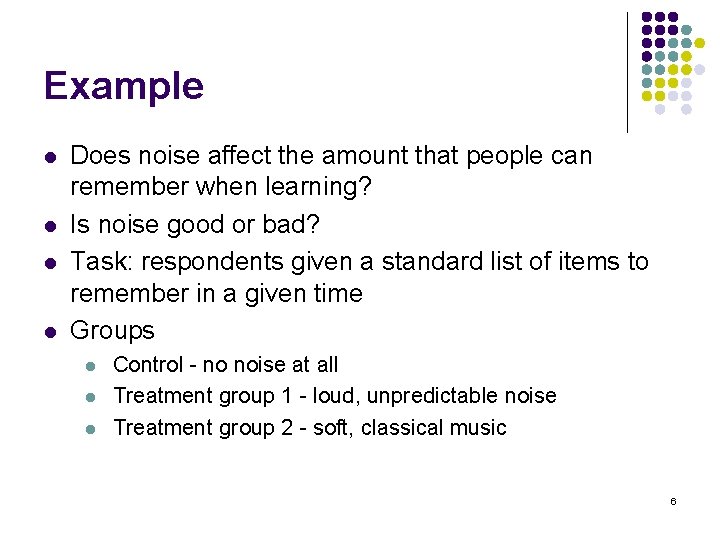
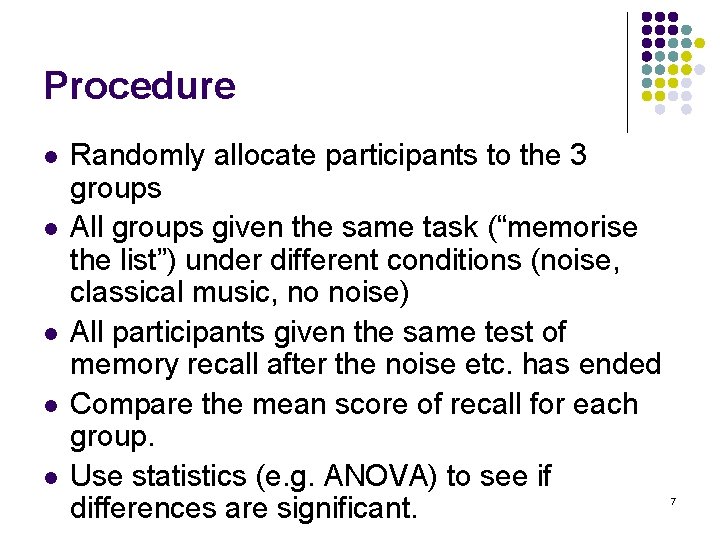
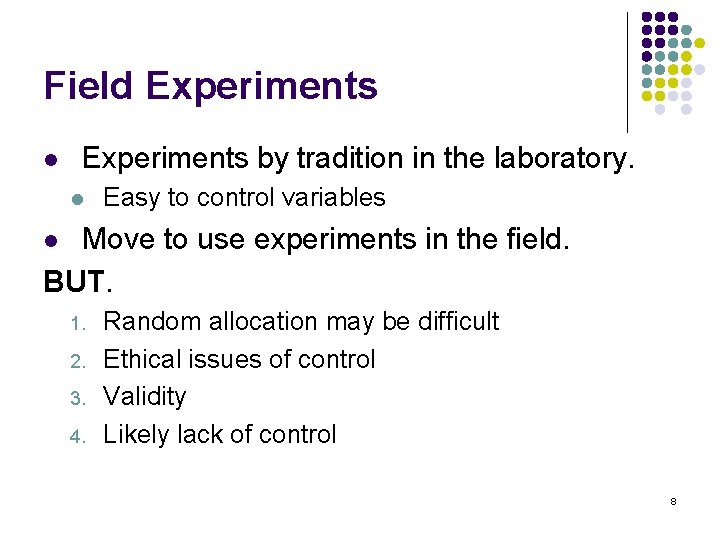
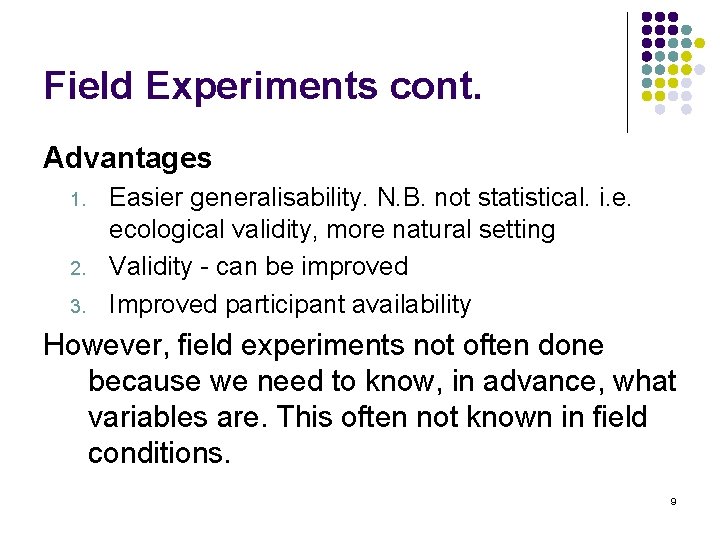
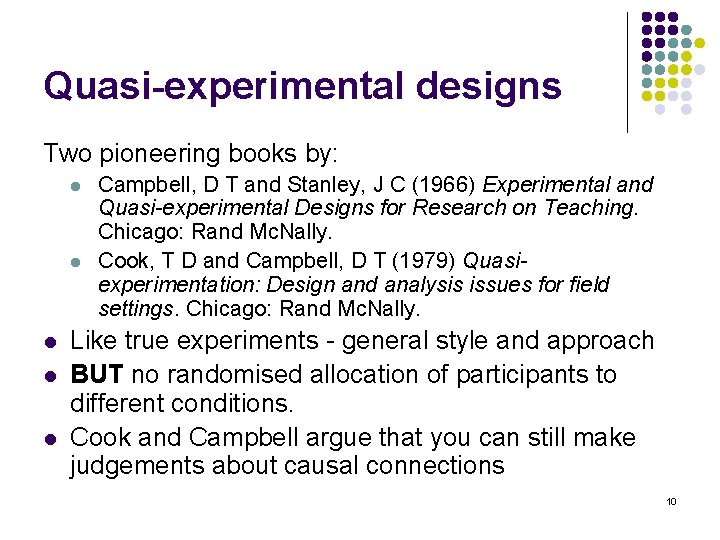
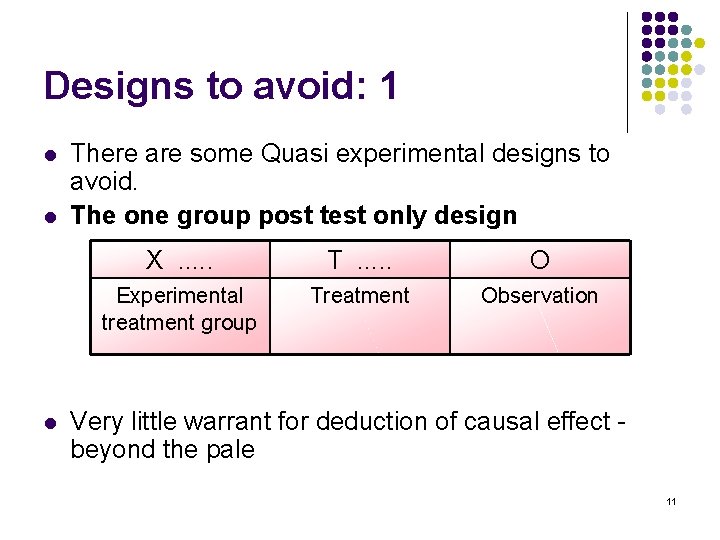
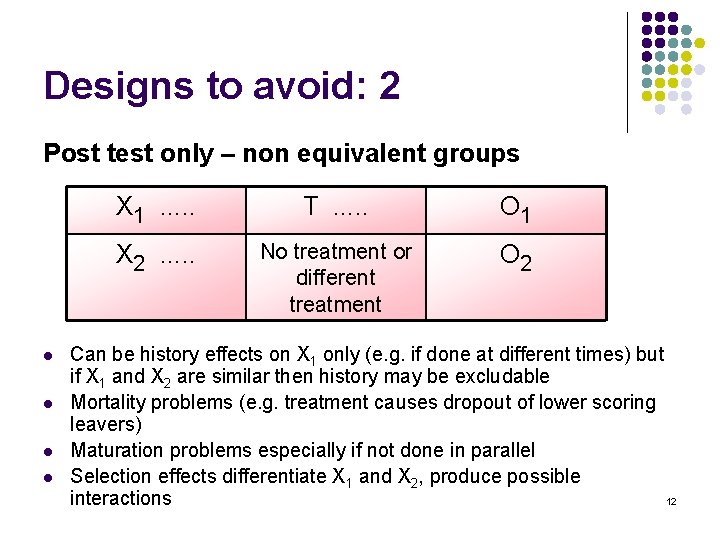
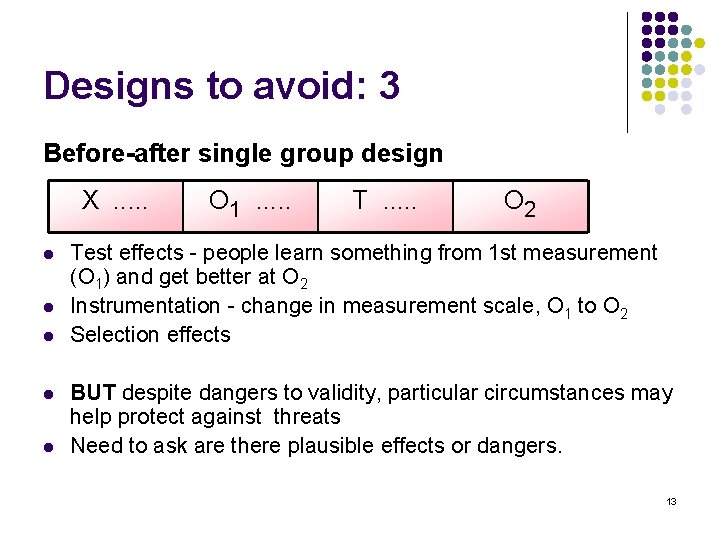
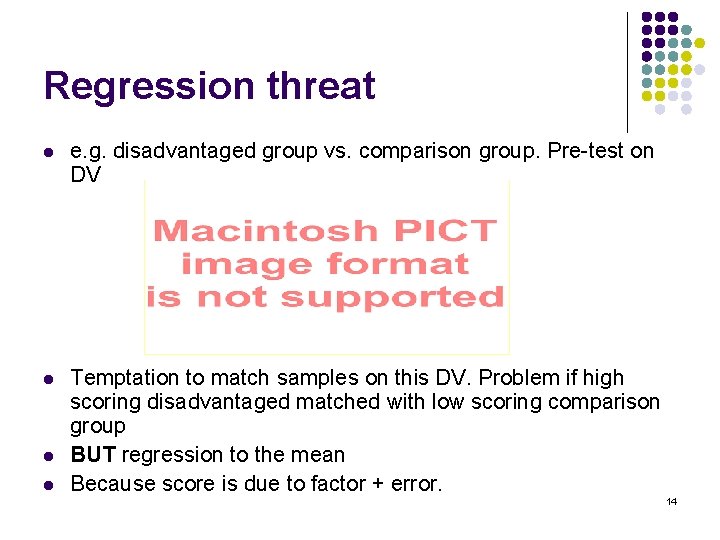
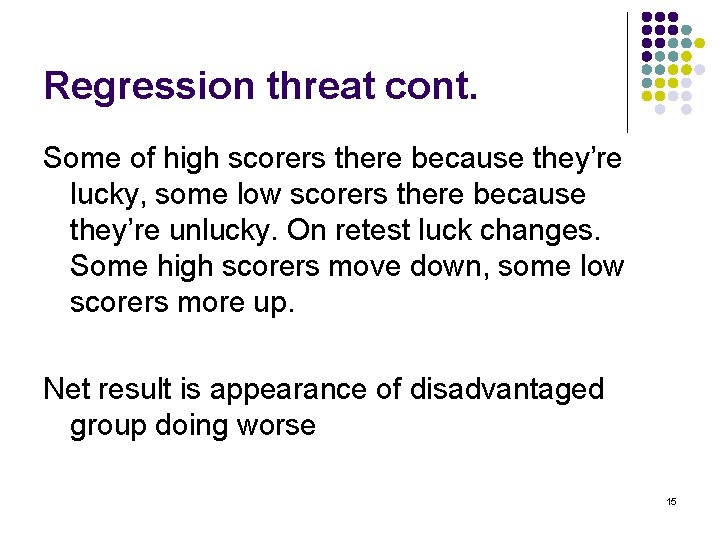
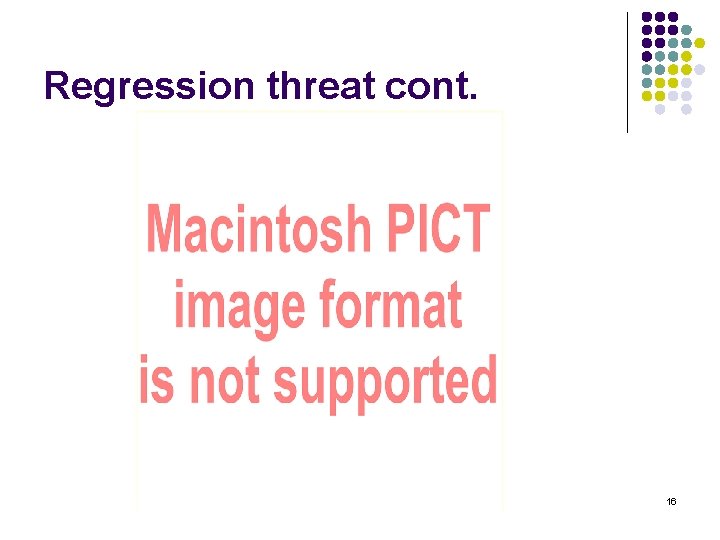
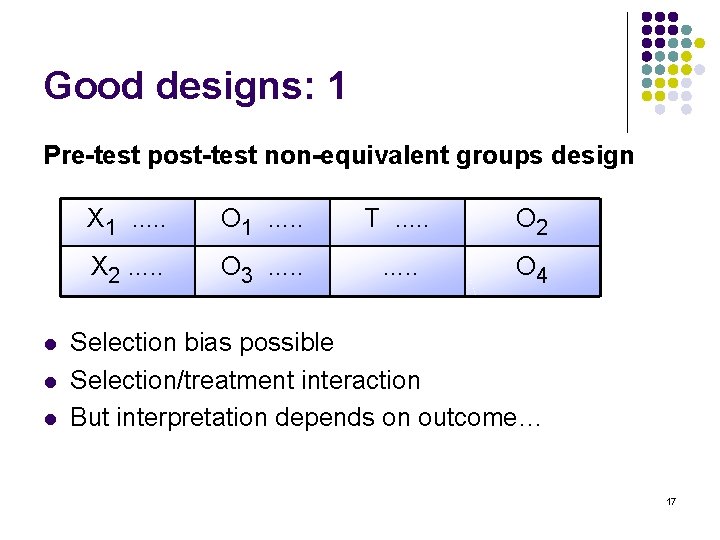
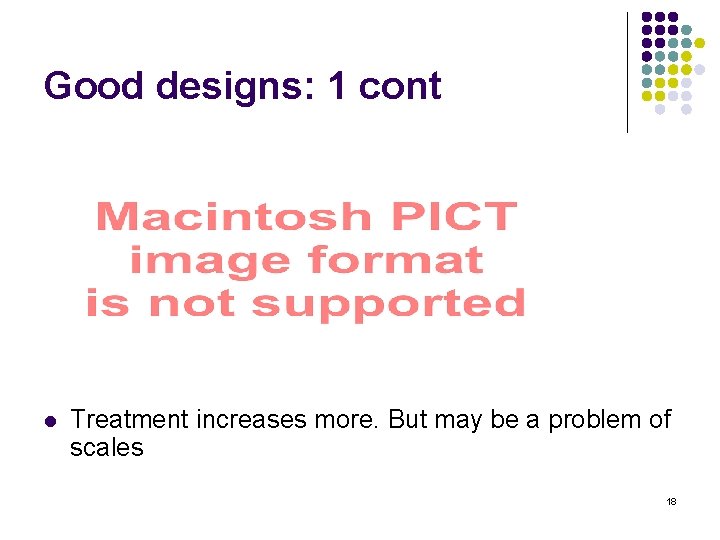
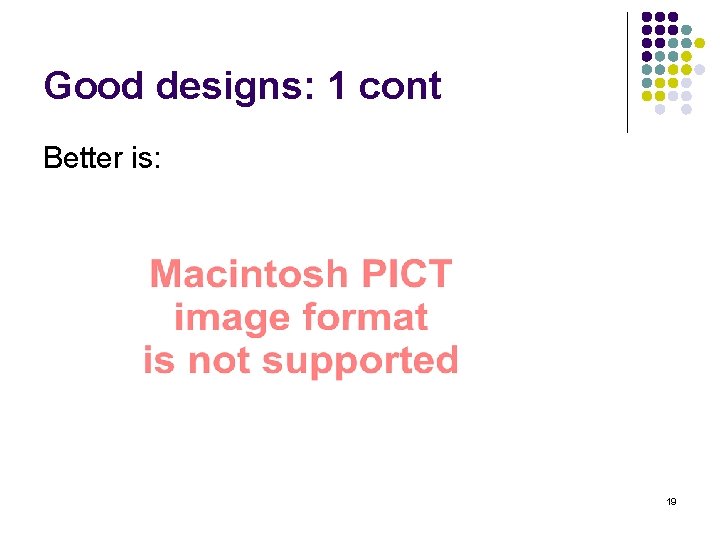
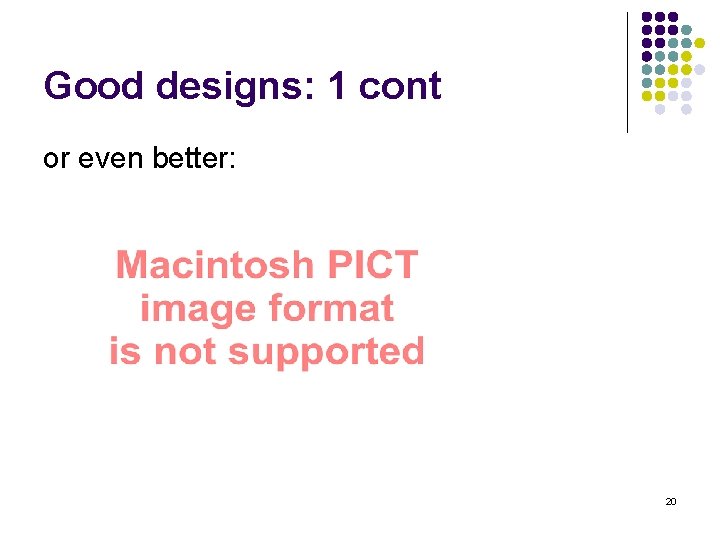
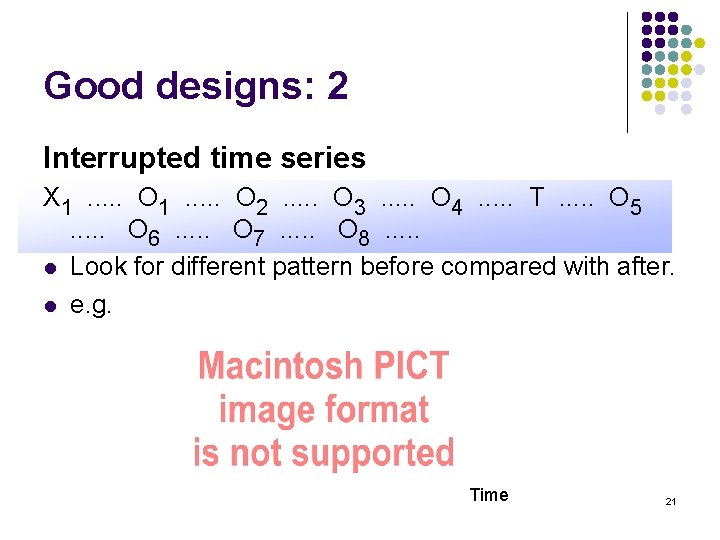
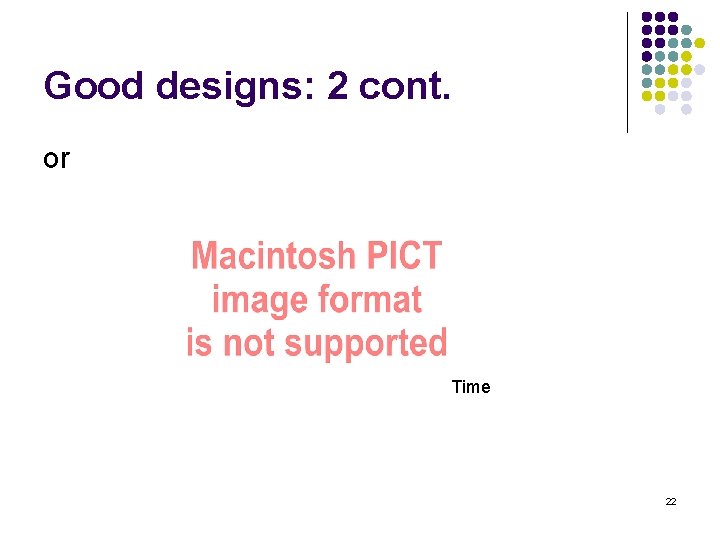
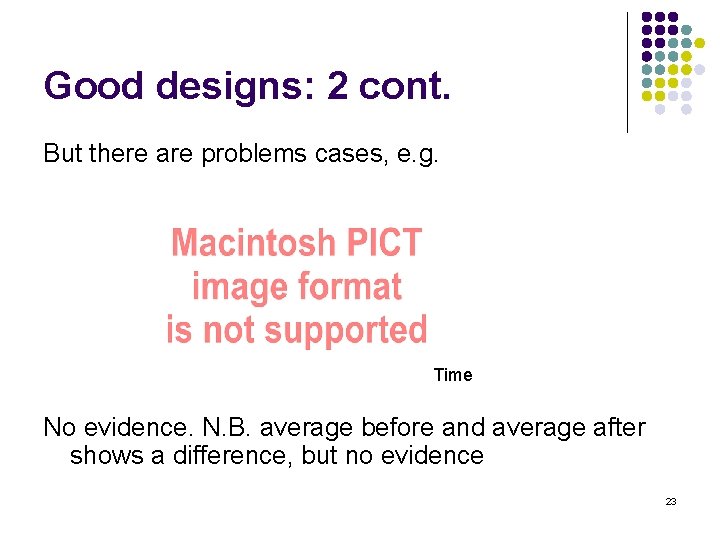
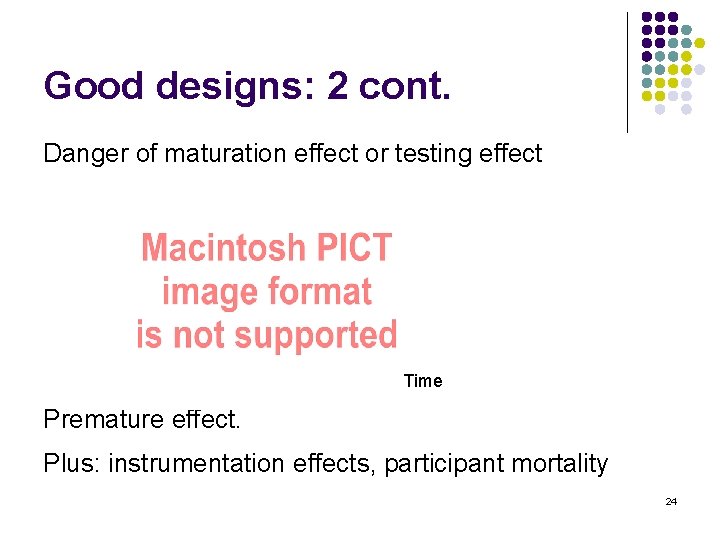
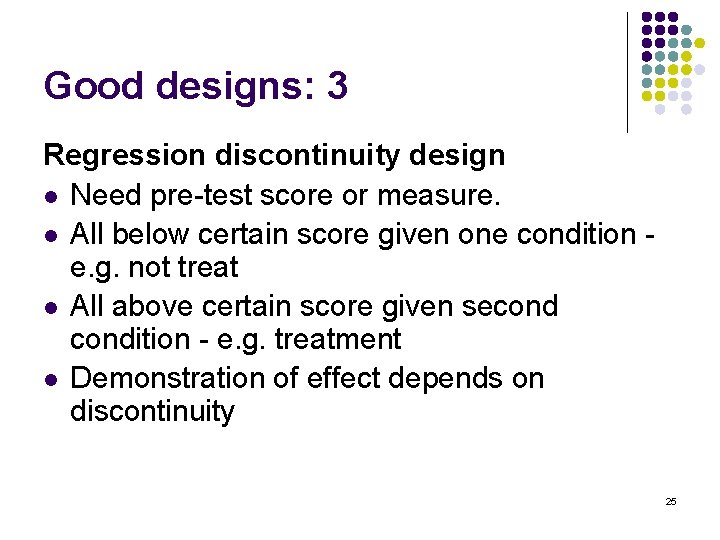
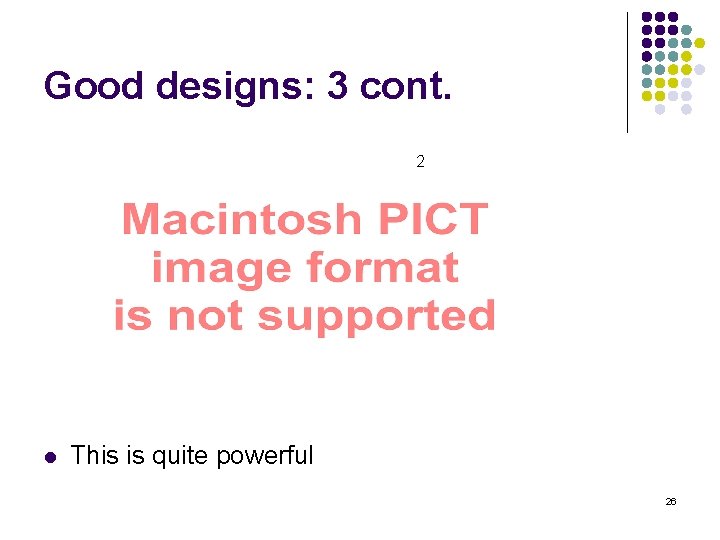
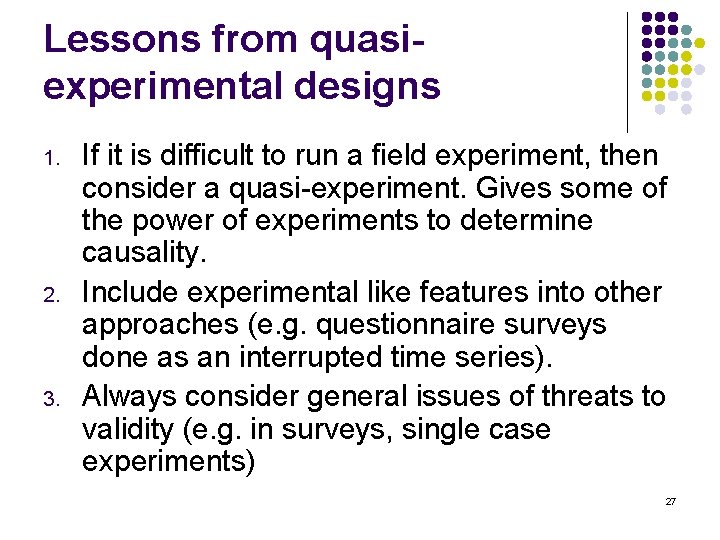
- Slides: 27
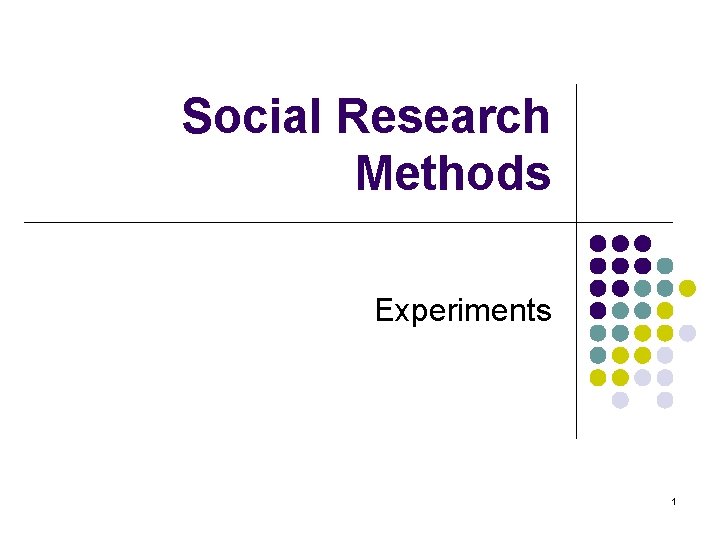
Social Research Methods Experiments 1
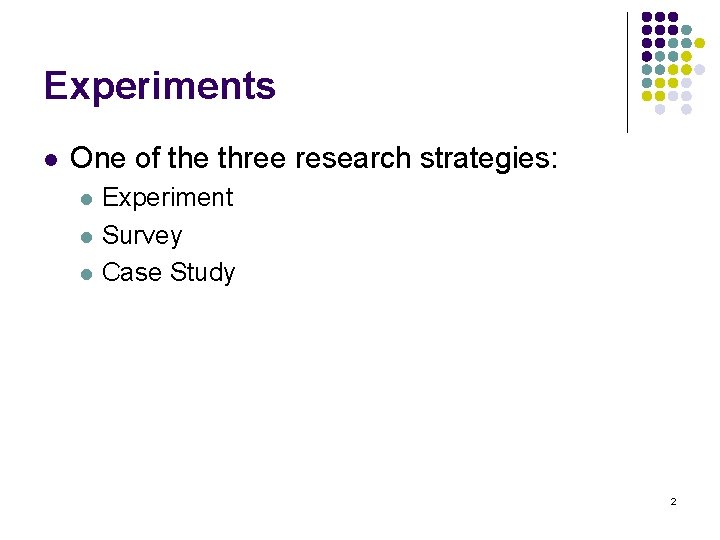
Experiments l One of the three research strategies: l l l Experiment Survey Case Study 2
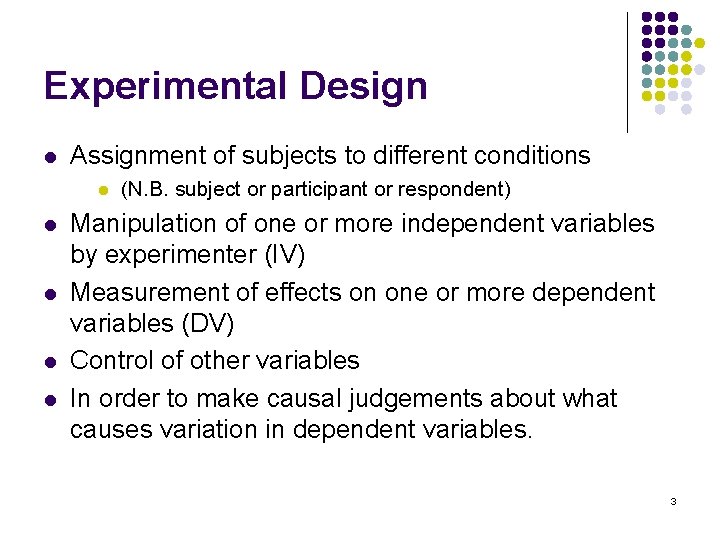
Experimental Design l Assignment of subjects to different conditions l l l (N. B. subject or participant or respondent) Manipulation of one or more independent variables by experimenter (IV) Measurement of effects on one or more dependent variables (DV) Control of other variables In order to make causal judgements about what causes variation in dependent variables. 3
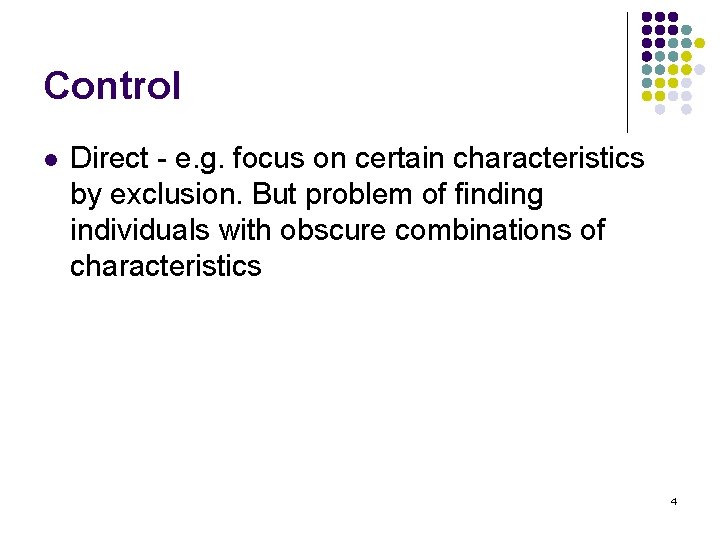
Control l Direct - e. g. focus on certain characteristics by exclusion. But problem of finding individuals with obscure combinations of characteristics 4
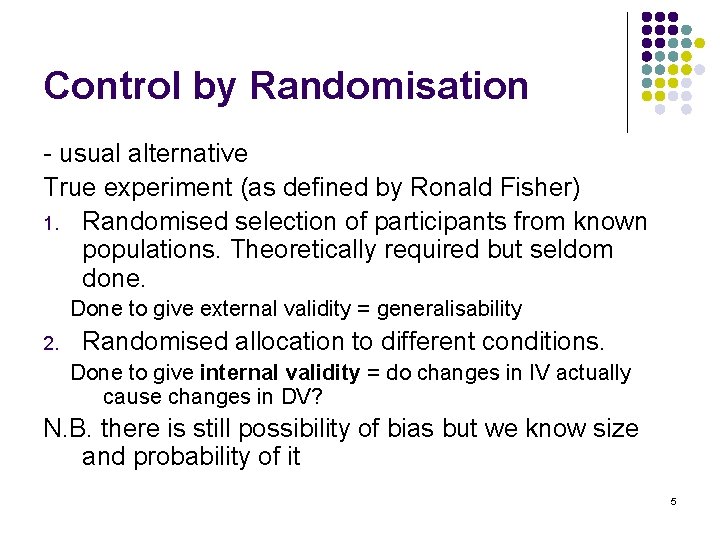
Control by Randomisation - usual alternative True experiment (as defined by Ronald Fisher) 1. Randomised selection of participants from known populations. Theoretically required but seldom done. Done to give external validity = generalisability 2. Randomised allocation to different conditions. Done to give internal validity = do changes in IV actually cause changes in DV? N. B. there is still possibility of bias but we know size and probability of it 5
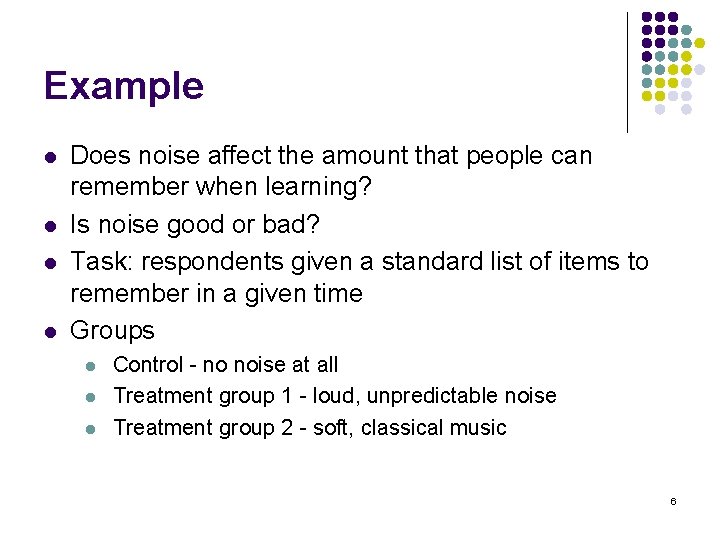
Example l l Does noise affect the amount that people can remember when learning? Is noise good or bad? Task: respondents given a standard list of items to remember in a given time Groups l l l Control - no noise at all Treatment group 1 - loud, unpredictable noise Treatment group 2 - soft, classical music 6
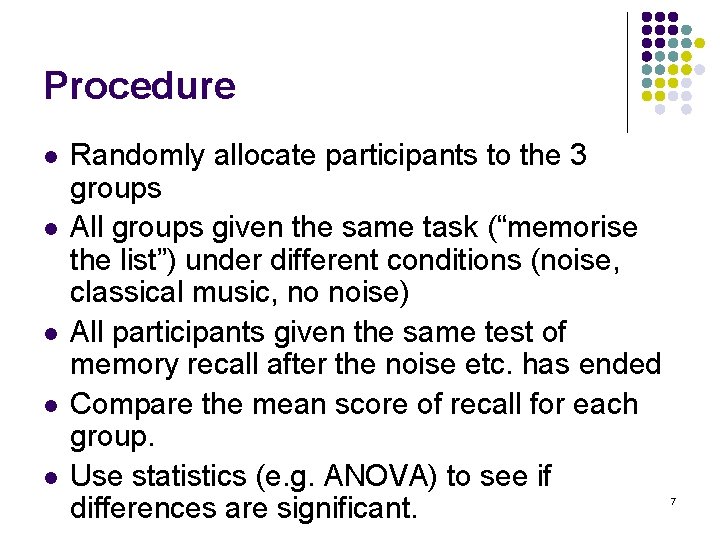
Procedure l l l Randomly allocate participants to the 3 groups All groups given the same task (“memorise the list”) under different conditions (noise, classical music, no noise) All participants given the same test of memory recall after the noise etc. has ended Compare the mean score of recall for each group. Use statistics (e. g. ANOVA) to see if differences are significant. 7
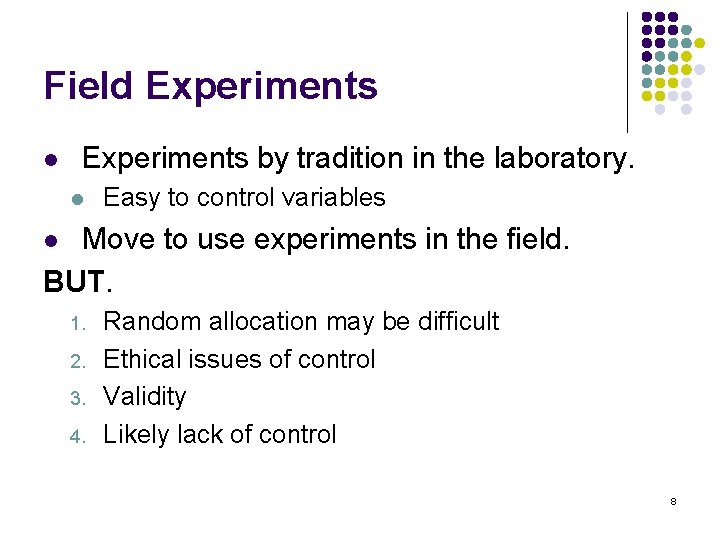
Field Experiments l Experiments by tradition in the laboratory. l Easy to control variables Move to use experiments in the field. BUT. l 1. 2. 3. 4. Random allocation may be difficult Ethical issues of control Validity Likely lack of control 8
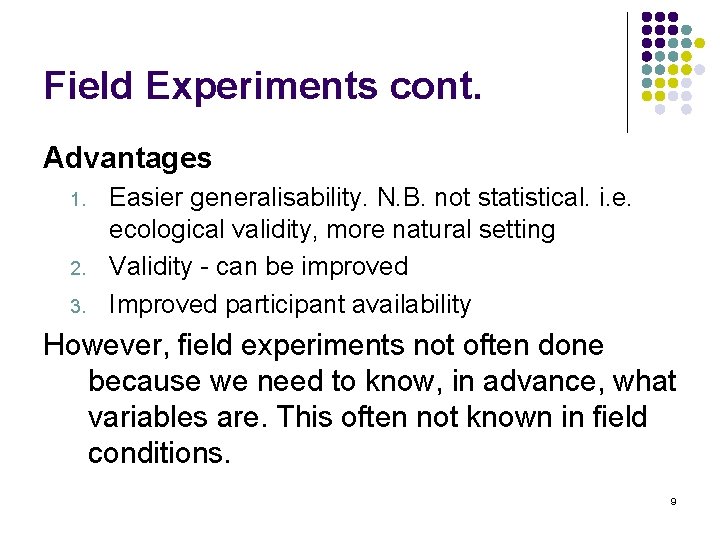
Field Experiments cont. Advantages 1. 2. 3. Easier generalisability. N. B. not statistical. i. e. ecological validity, more natural setting Validity - can be improved Improved participant availability However, field experiments not often done because we need to know, in advance, what variables are. This often not known in field conditions. 9
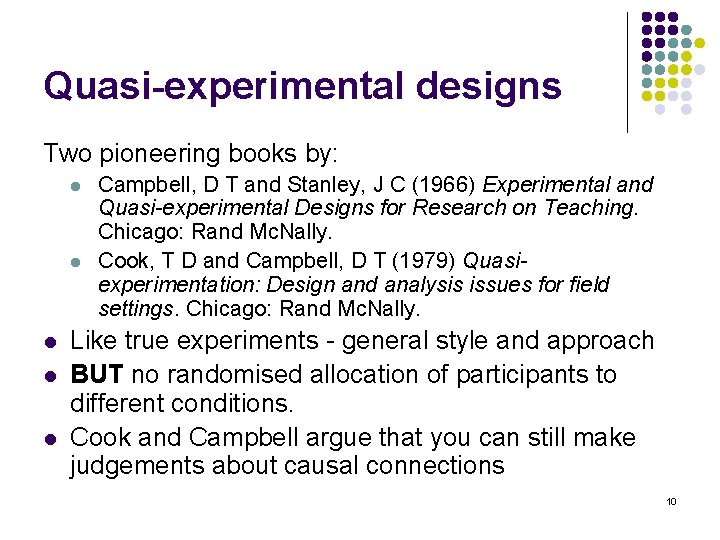
Quasi-experimental designs Two pioneering books by: l l l Campbell, D T and Stanley, J C (1966) Experimental and Quasi-experimental Designs for Research on Teaching. Chicago: Rand Mc. Nally. Cook, T D and Campbell, D T (1979) Quasiexperimentation: Design and analysis issues for field settings. Chicago: Rand Mc. Nally. Like true experiments - general style and approach BUT no randomised allocation of participants to different conditions. Cook and Campbell argue that you can still make judgements about causal connections 10
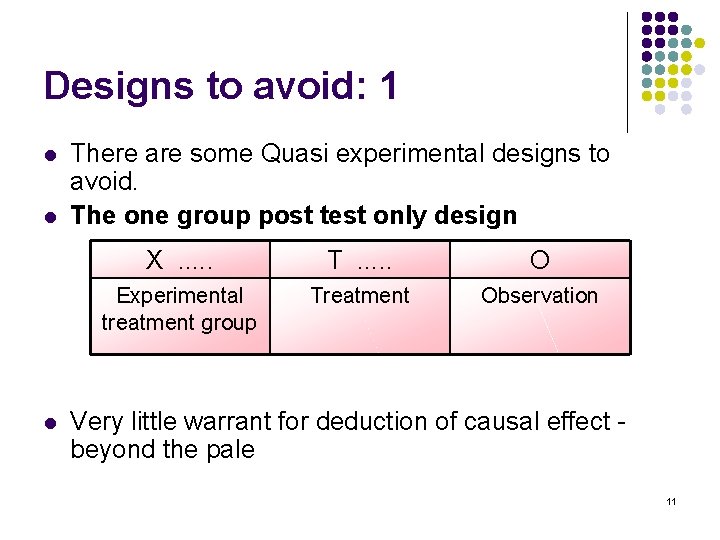
Designs to avoid: 1 l l l There are some Quasi experimental designs to avoid. The one group post test only design X. . . T. . . O Experimental treatment group Treatment Observation Very little warrant for deduction of causal effect beyond the pale 11
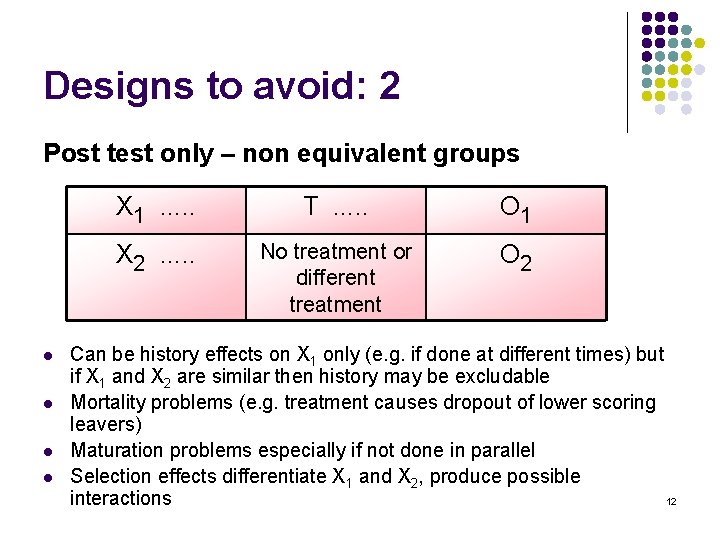
Designs to avoid: 2 Post test only – non equivalent groups l l X 1. . . T. . . O 1 X 2. . . No treatment or different treatment O 2 Can be history effects on X 1 only (e. g. if done at different times) but if X 1 and X 2 are similar then history may be excludable Mortality problems (e. g. treatment causes dropout of lower scoring leavers) Maturation problems especially if not done in parallel Selection effects differentiate X 1 and X 2, produce possible interactions 12
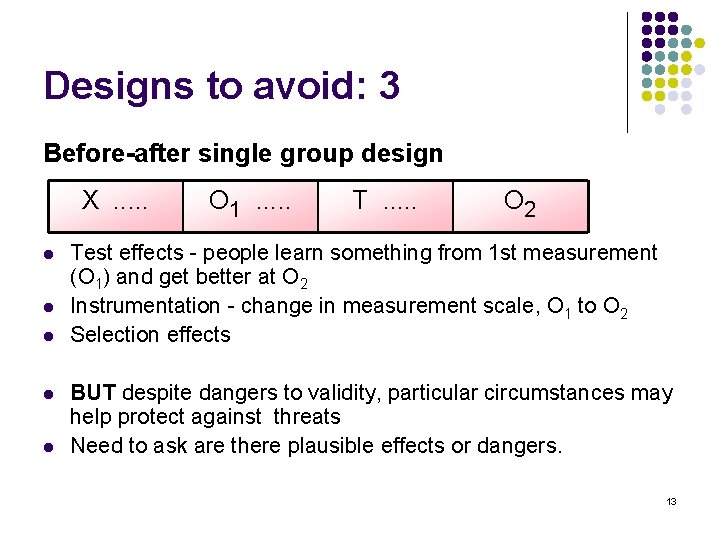
Designs to avoid: 3 Before-after single group design X. . . l l l O 1. . . T. . . O 2 Test effects - people learn something from 1 st measurement (O 1) and get better at O 2 Instrumentation - change in measurement scale, O 1 to O 2 Selection effects BUT despite dangers to validity, particular circumstances may help protect against threats Need to ask are there plausible effects or dangers. 13
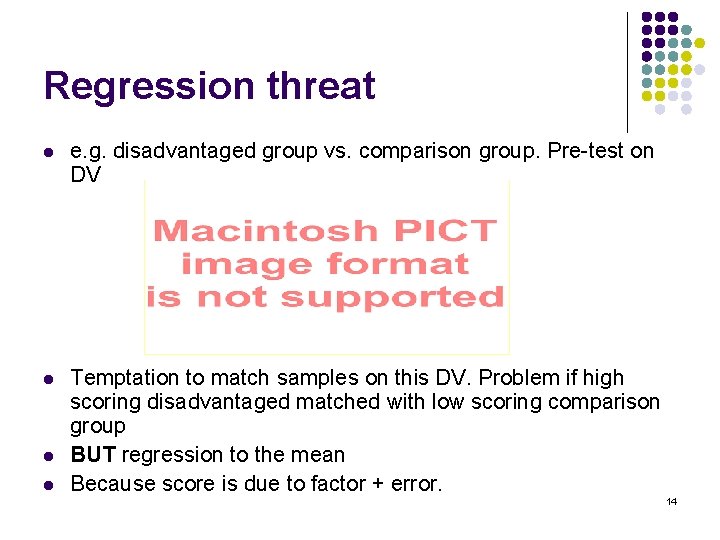
Regression threat l e. g. disadvantaged group vs. comparison group. Pre-test on DV l Temptation to match samples on this DV. Problem if high scoring disadvantaged matched with low scoring comparison group BUT regression to the mean Because score is due to factor + error. l l 14
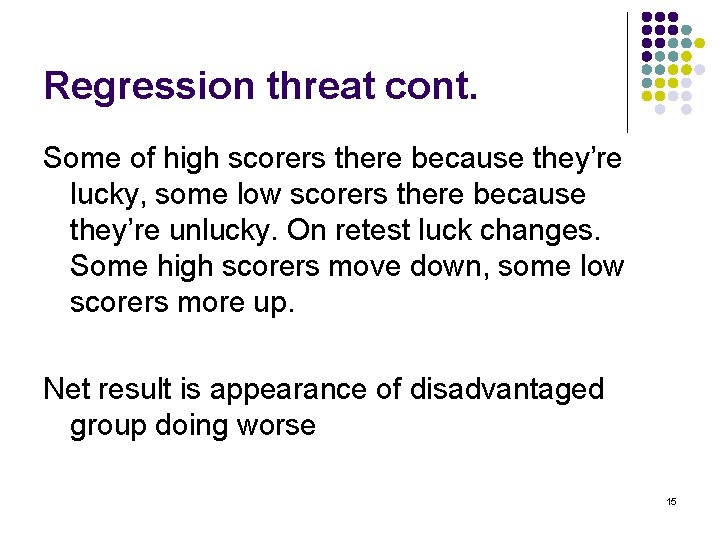
Regression threat cont. Some of high scorers there because they’re lucky, some low scorers there because they’re unlucky. On retest luck changes. Some high scorers move down, some low scorers more up. Net result is appearance of disadvantaged group doing worse 15
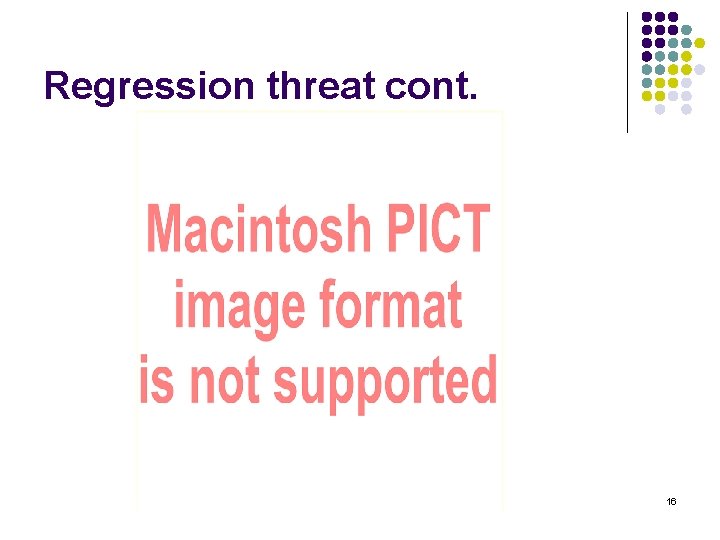
Regression threat cont. 16
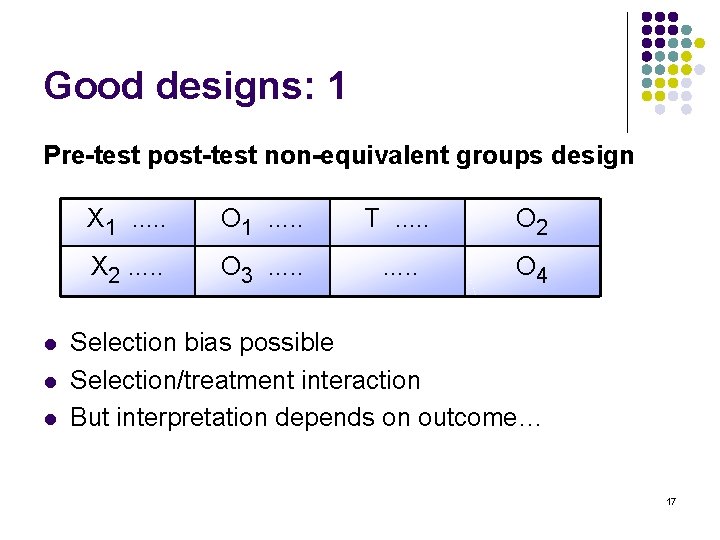
Good designs: 1 Pre-test post-test non-equivalent groups design l l l X 1. . . O 1. . . T. . . O 2 X 2. . . O 3. . O 4 Selection bias possible Selection/treatment interaction But interpretation depends on outcome… 17
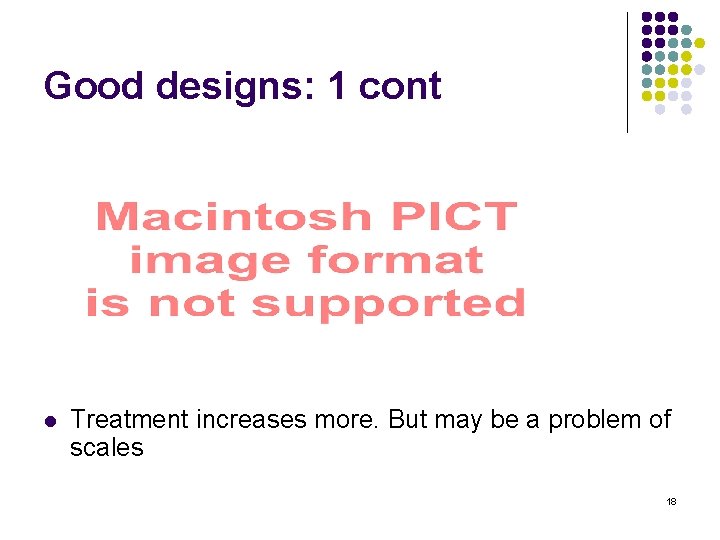
Good designs: 1 cont l Treatment increases more. But may be a problem of scales 18
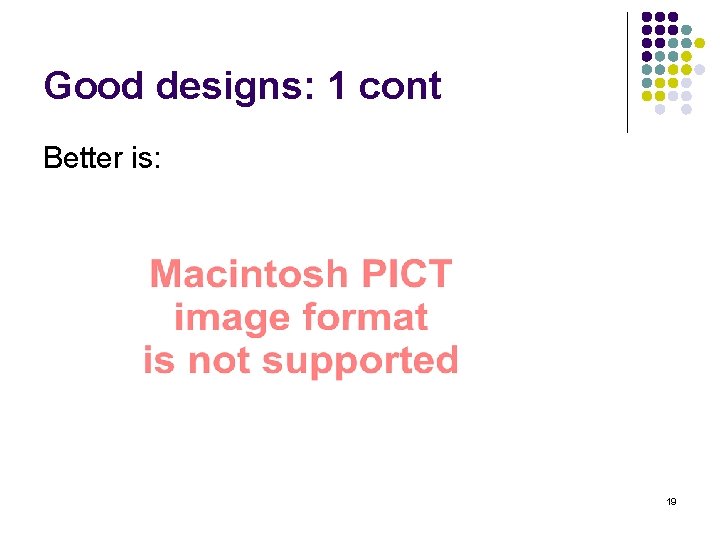
Good designs: 1 cont Better is: 19
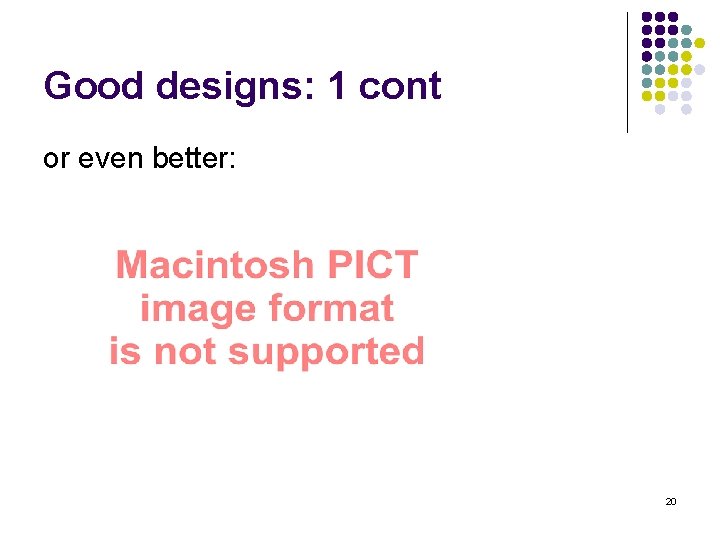
Good designs: 1 cont or even better: 20
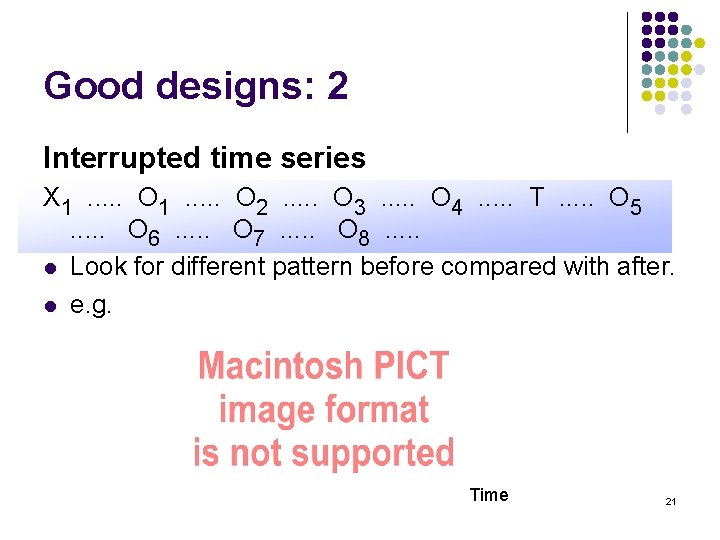
Good designs: 2 Interrupted time series X 1. . O 2. . . O 3. . . O 4. . . T. . . O 5. . . O 6. . . O 7. . . O 8. . . l Look for different pattern before compared with after. l e. g. Time 21
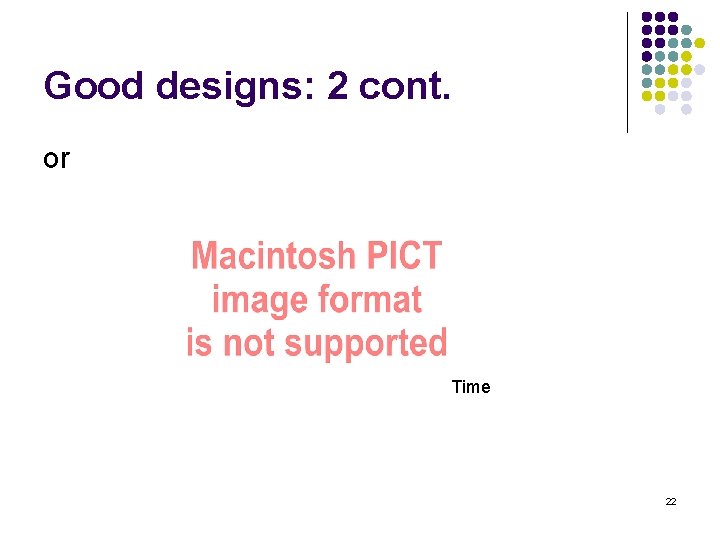
Good designs: 2 cont. or Time 22
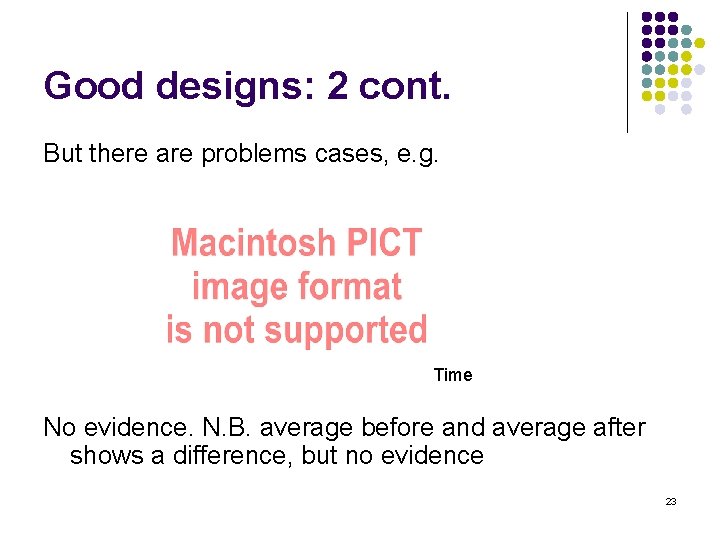
Good designs: 2 cont. But there are problems cases, e. g. Time No evidence. N. B. average before and average after shows a difference, but no evidence 23
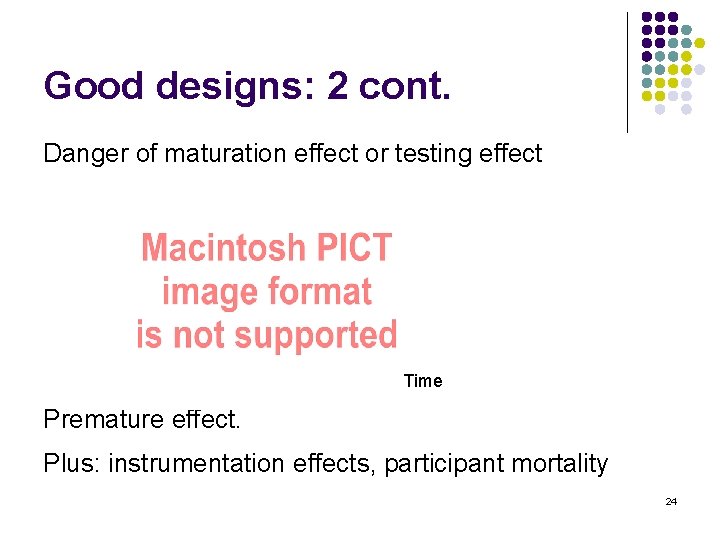
Good designs: 2 cont. Danger of maturation effect or testing effect Time Premature effect. Plus: instrumentation effects, participant mortality 24
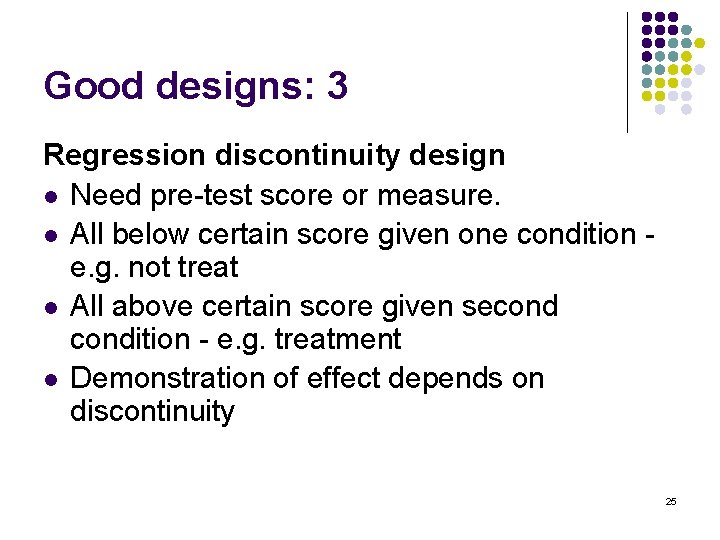
Good designs: 3 Regression discontinuity design l Need pre-test score or measure. l All below certain score given one condition e. g. not treat l All above certain score given secondition - e. g. treatment l Demonstration of effect depends on discontinuity 25
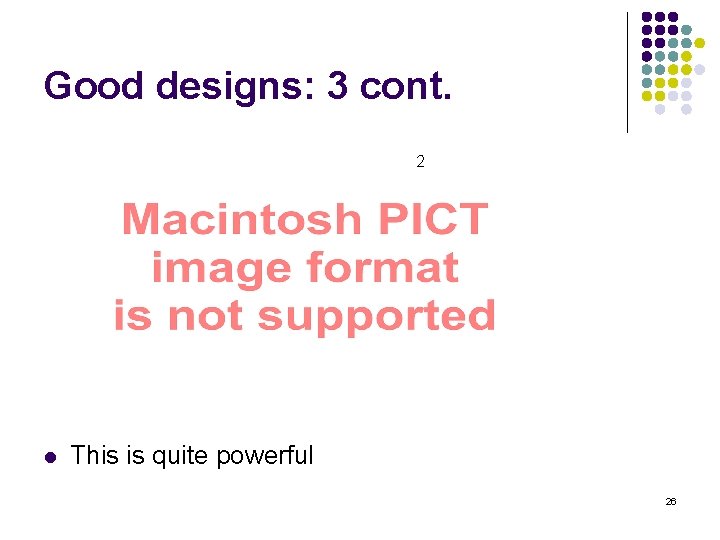
Good designs: 3 cont. 2 l This is quite powerful 26
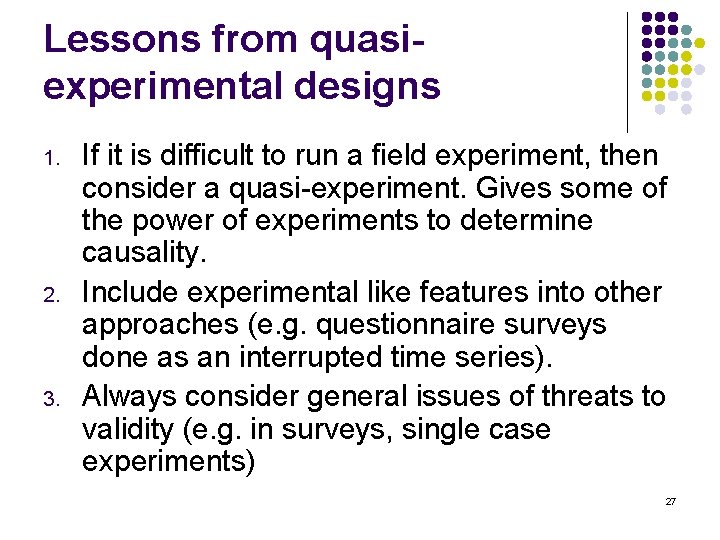
Lessons from quasiexperimental designs 1. 2. 3. If it is difficult to run a field experiment, then consider a quasi-experiment. Gives some of the power of experiments to determine causality. Include experimental like features into other approaches (e. g. questionnaire surveys done as an interrupted time series). Always consider general issues of threats to validity (e. g. in surveys, single case experiments) 27
Alan bryman social research methods
Method of social psychology
Neuman social research methods "torrent"
Direct and indirect wax pattern
One god one empire one emperor
One one one little dog run
One king one law one faith
One empire one god one emperor
One ford
See one do one teach one
One price policy
Night structure
See one do one teach one
Asean tourism strategic plan
Asean one vision one identity one community
Communication in health and social care
Social thinking and social influence
Social thinking social influence social relations
Notes on research methods
Research methods in developmental psychology
Time dimension of the study
Research design example
Pr research process
Disadvantages of primary research
Examples of quantitative research questions and hypotheses
Mixed methods research examples
Business research methods assignment
Descriptive research design