Social Network Analysis Christopher Mc Carty University of
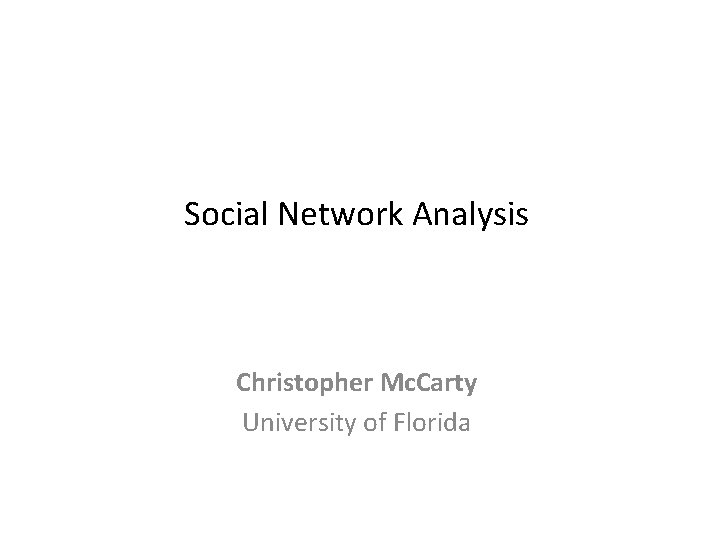
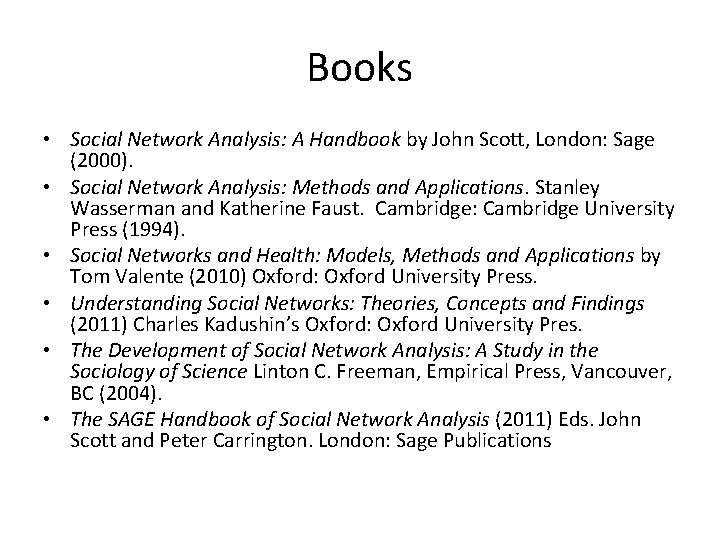
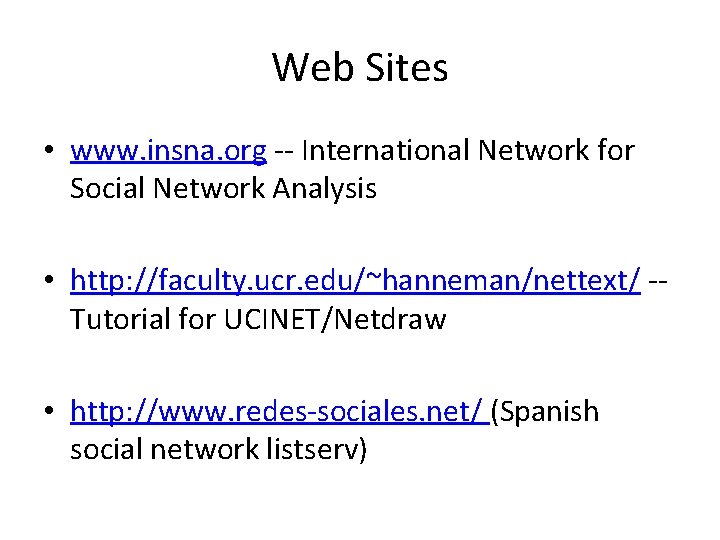
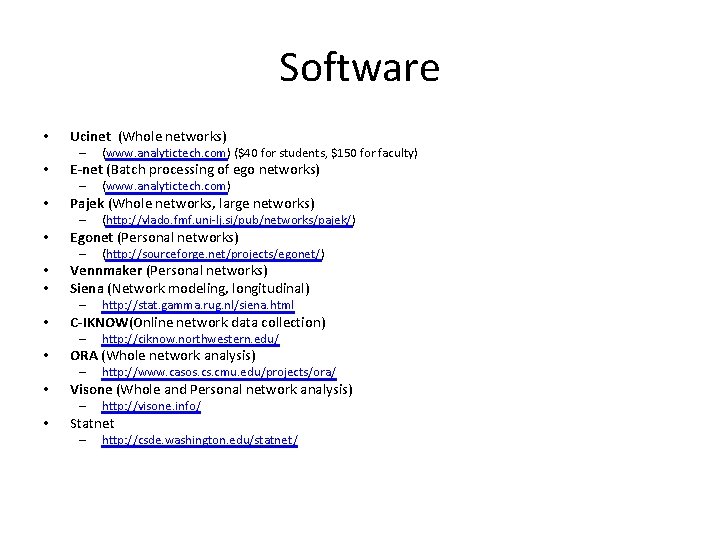
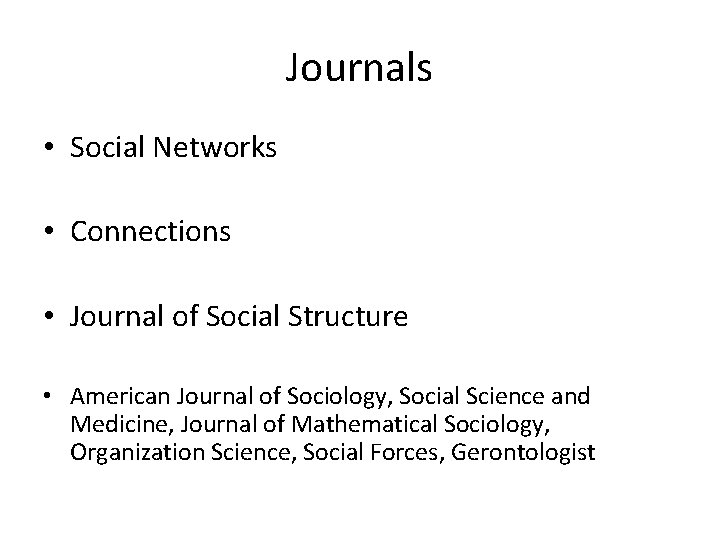
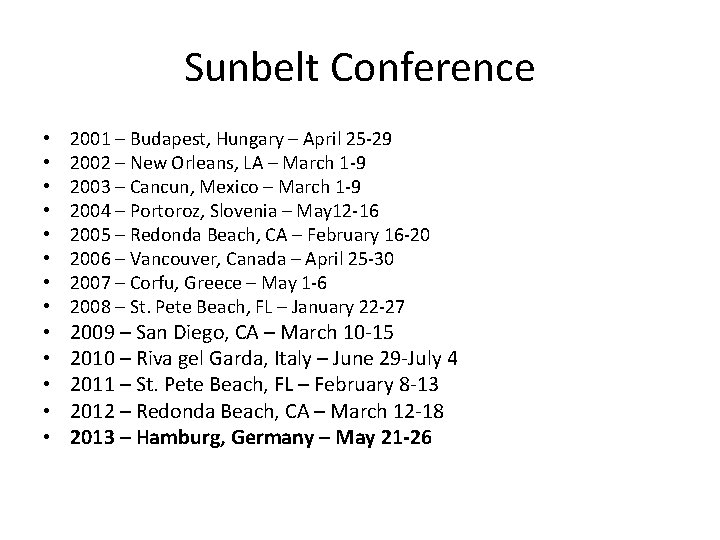
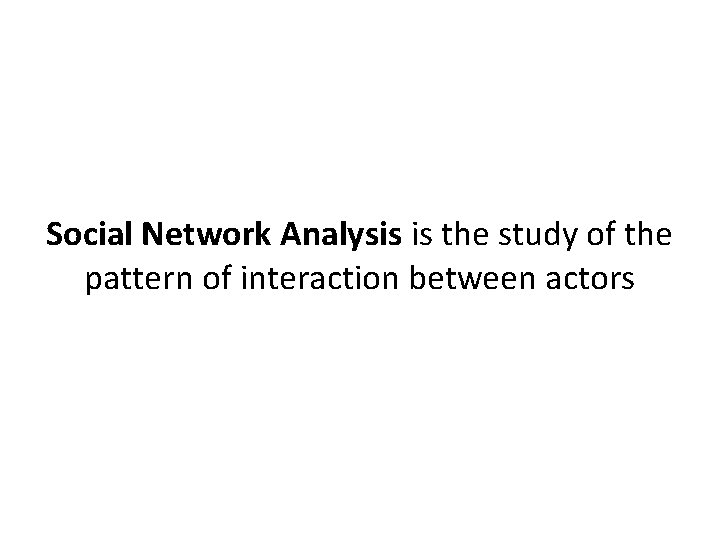
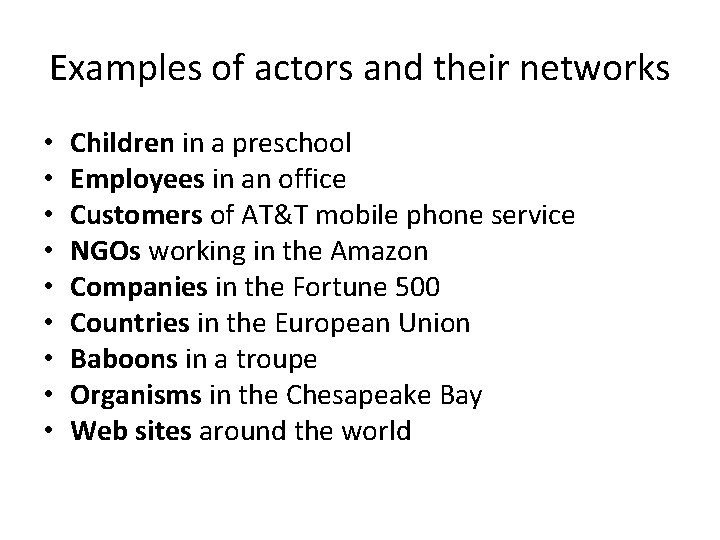
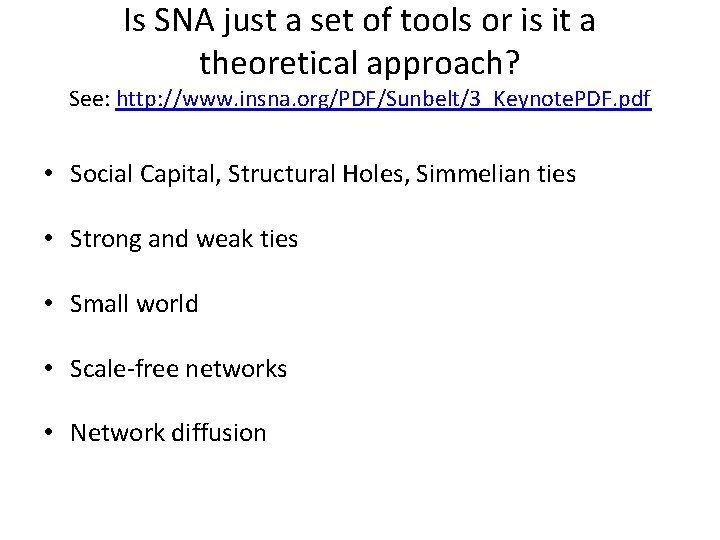
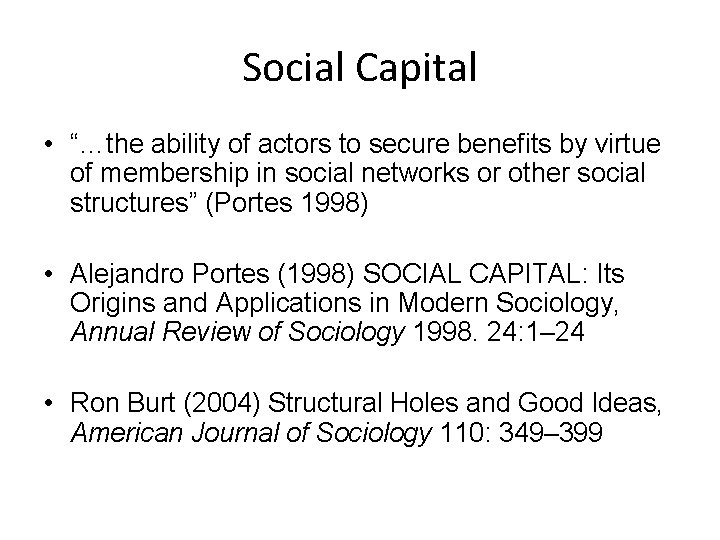
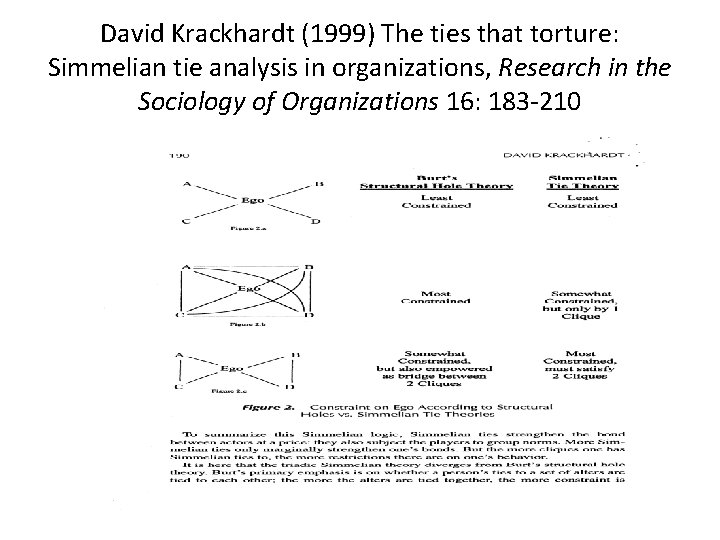
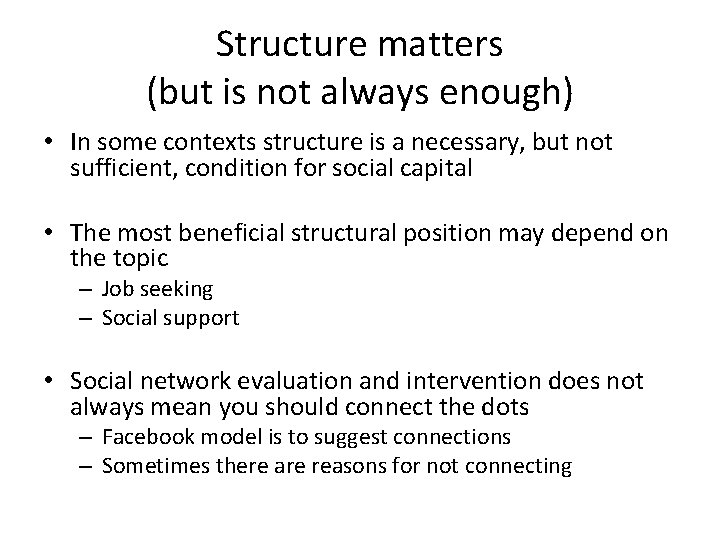
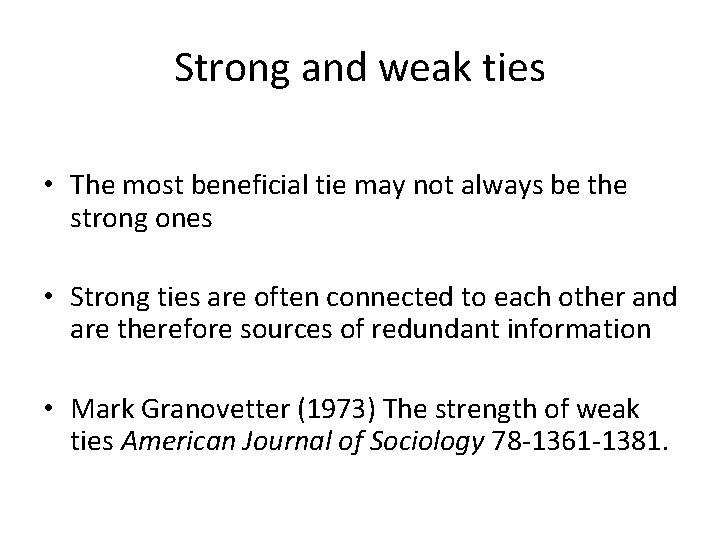
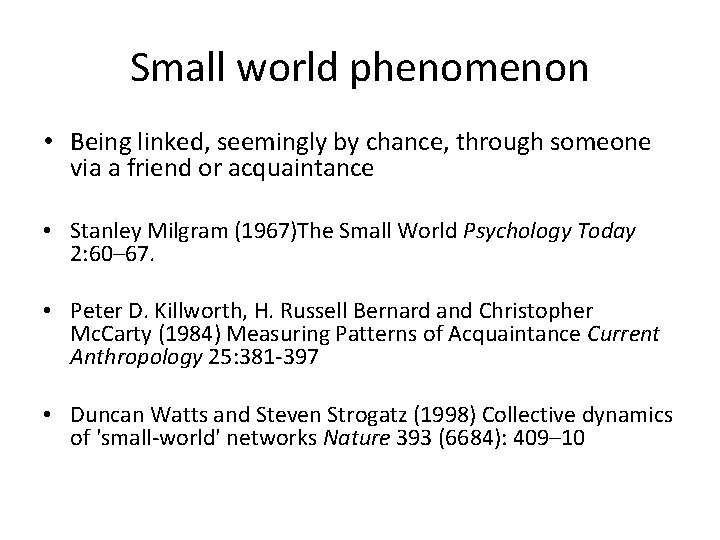
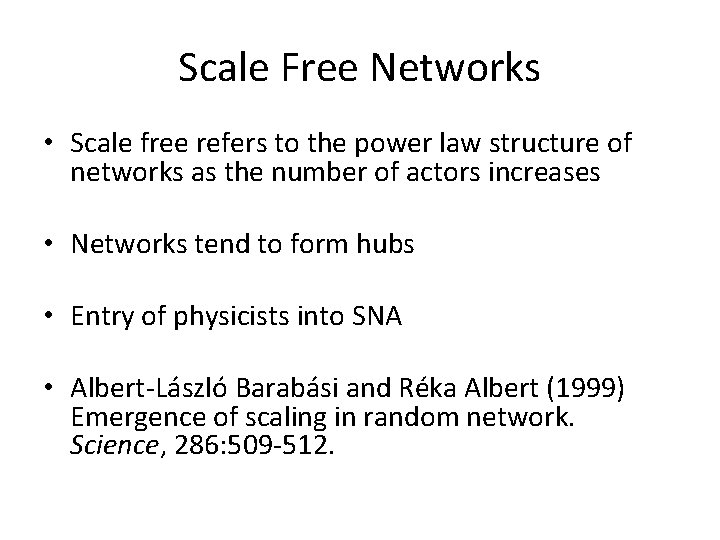
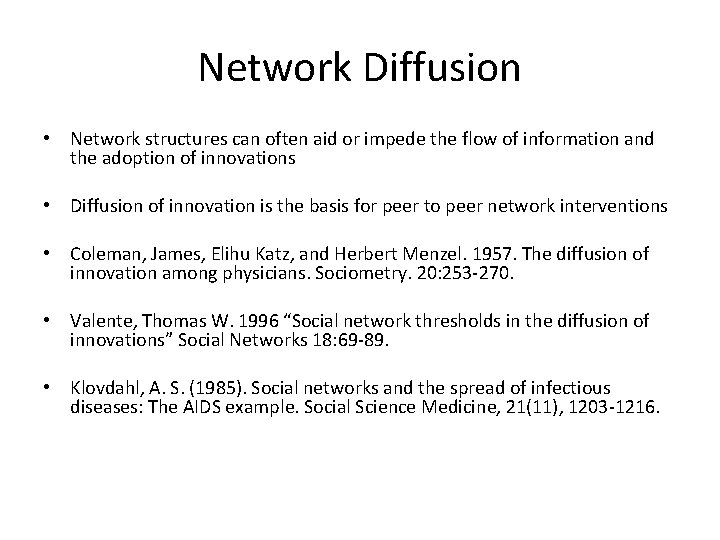
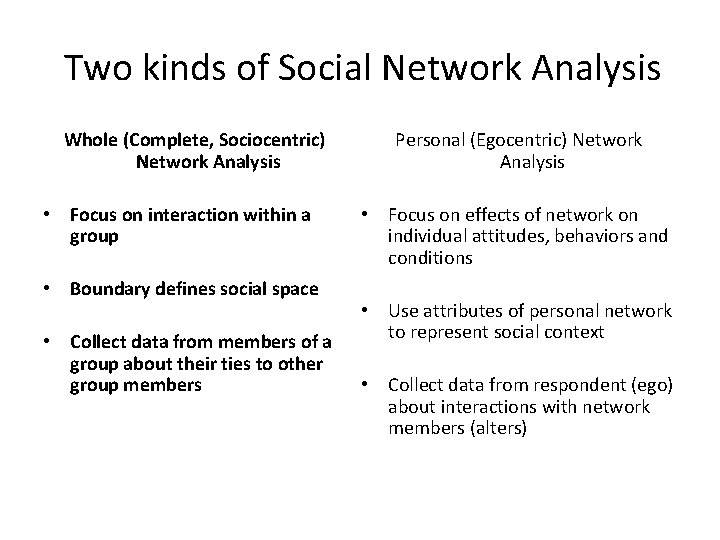
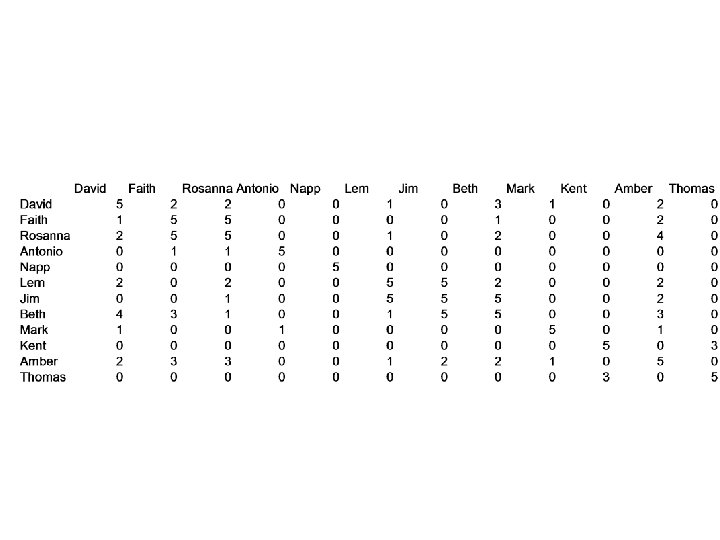
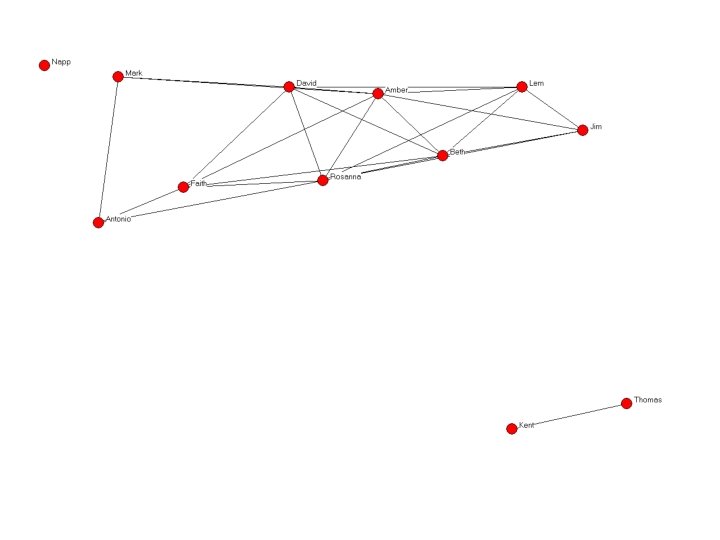
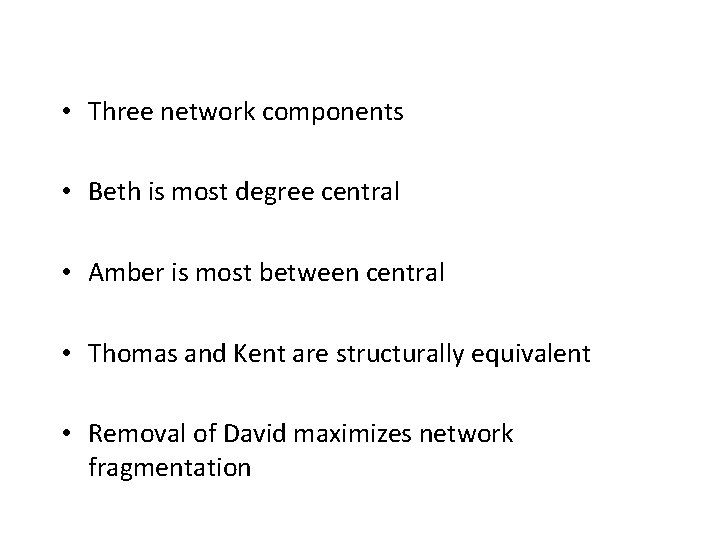
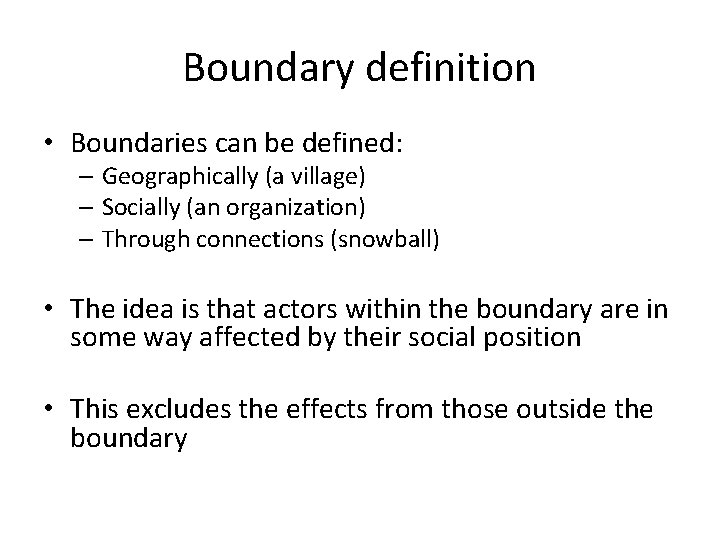
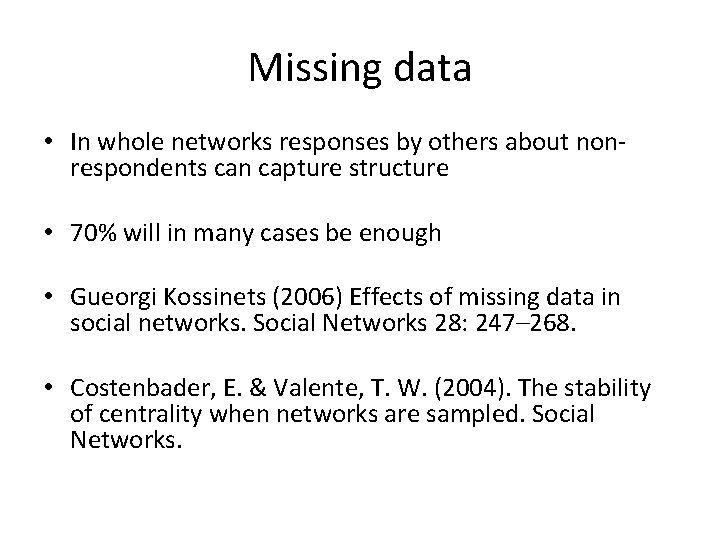
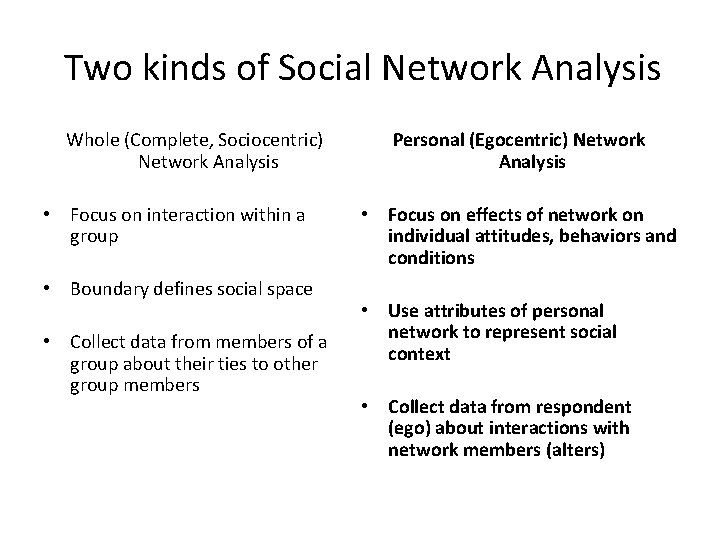
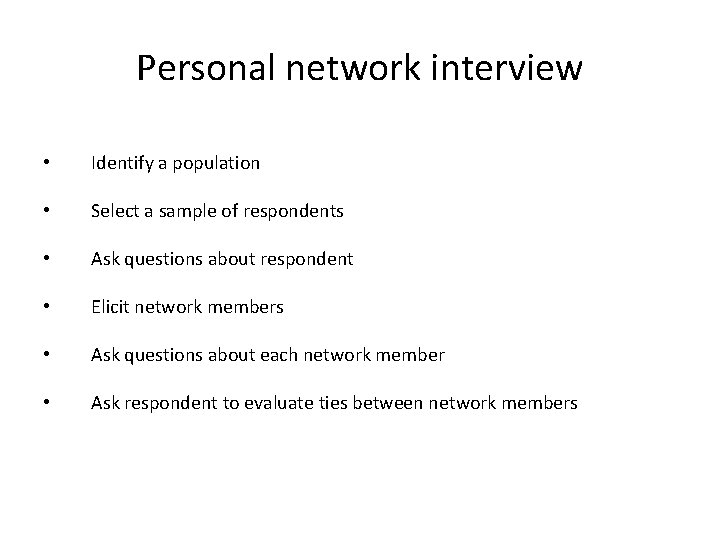
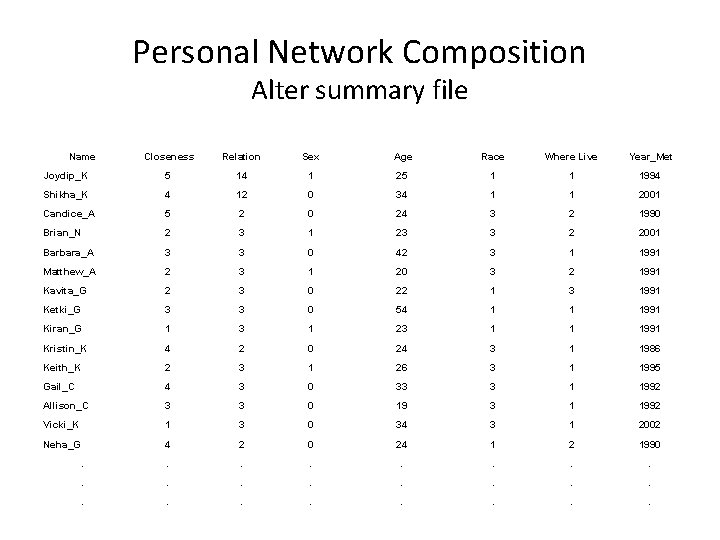
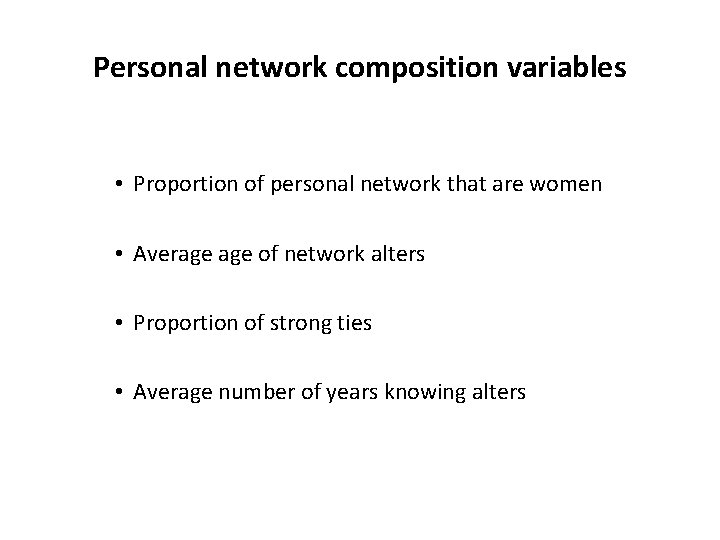
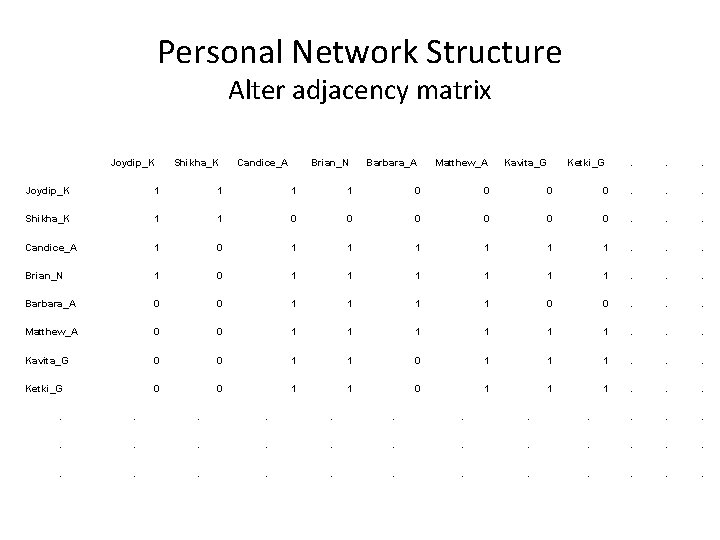
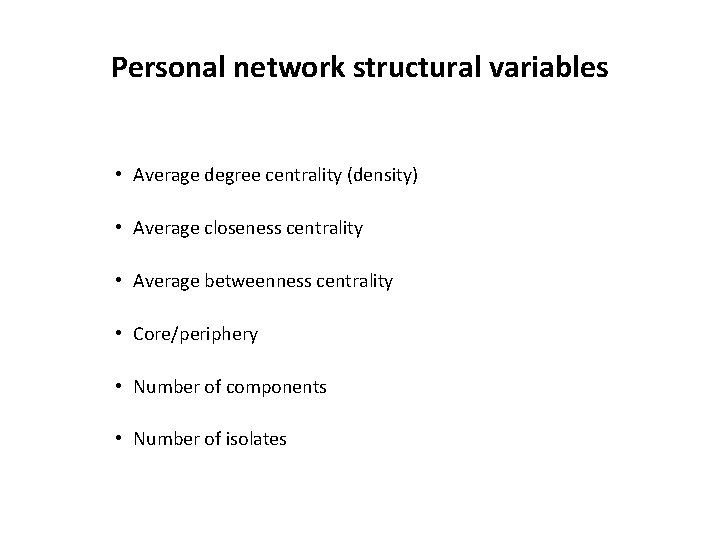
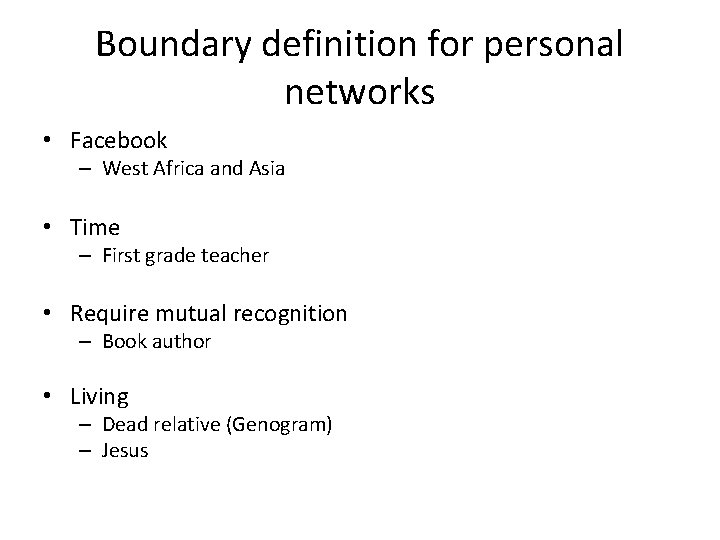
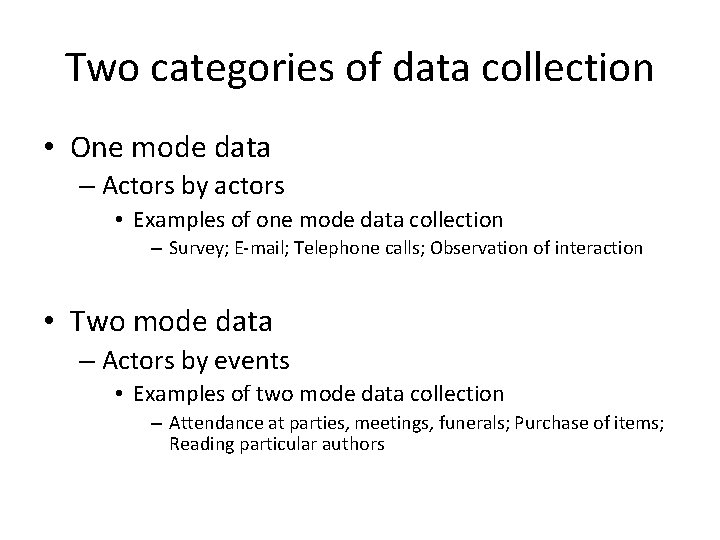
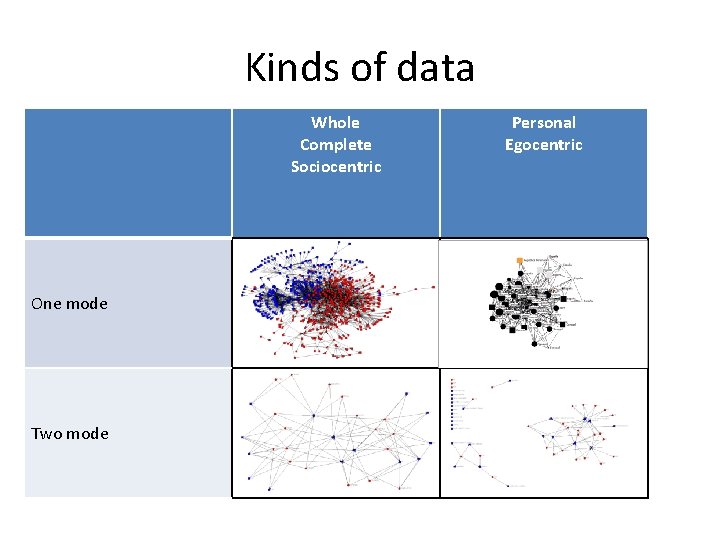
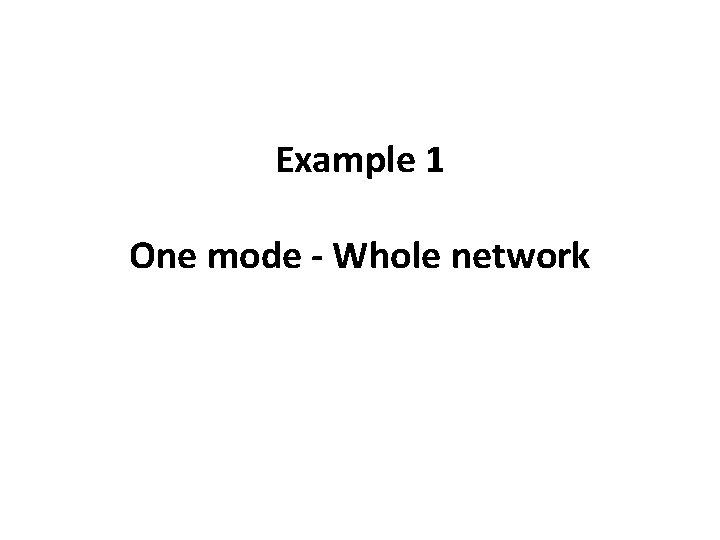
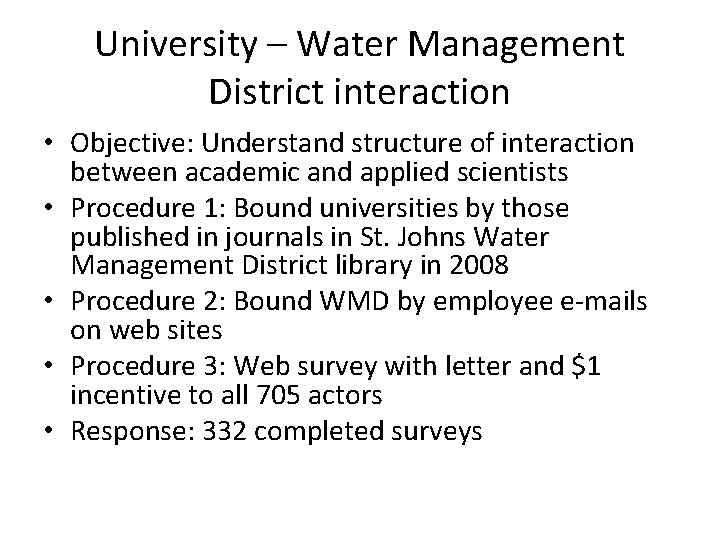
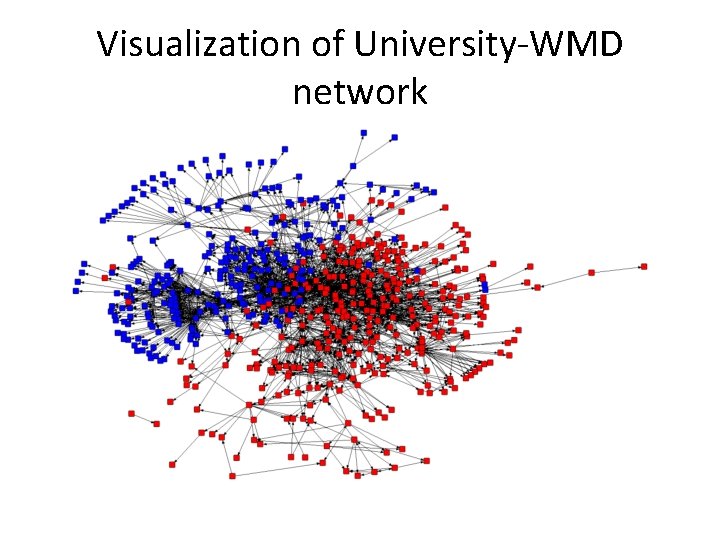
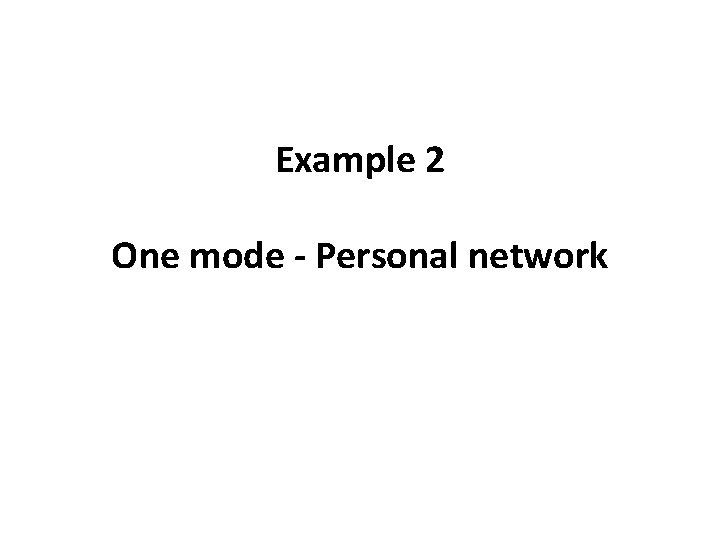
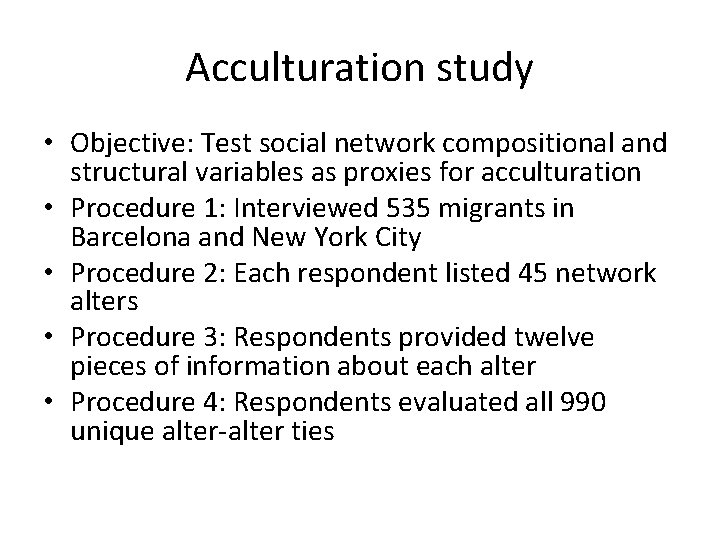
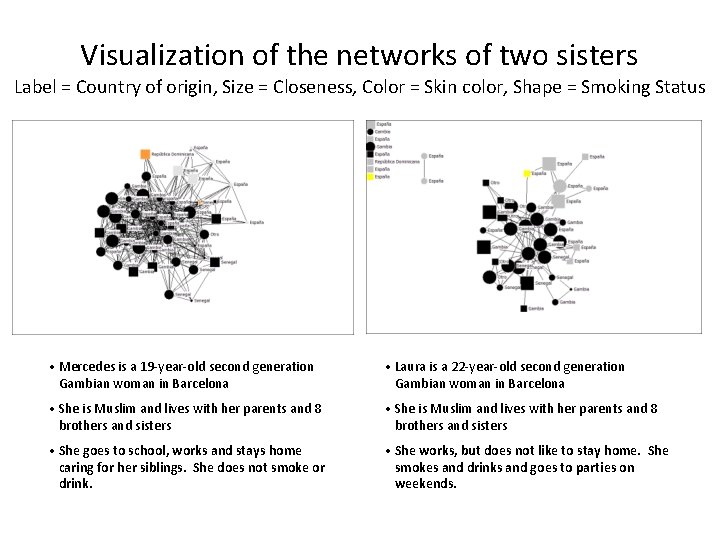
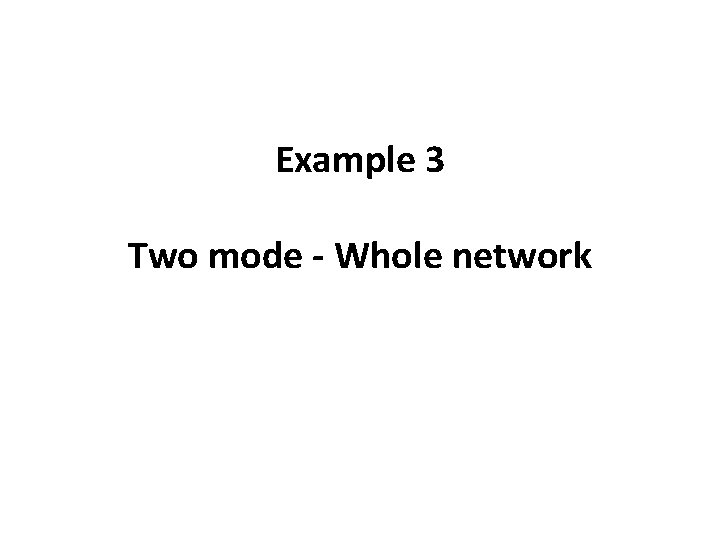
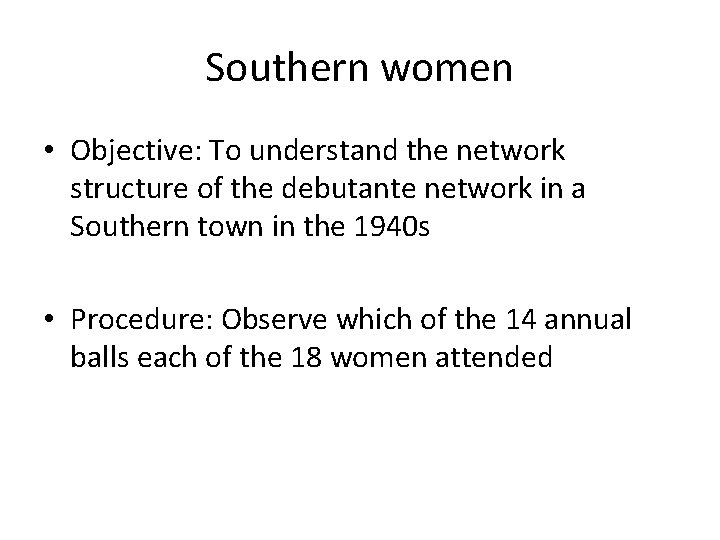
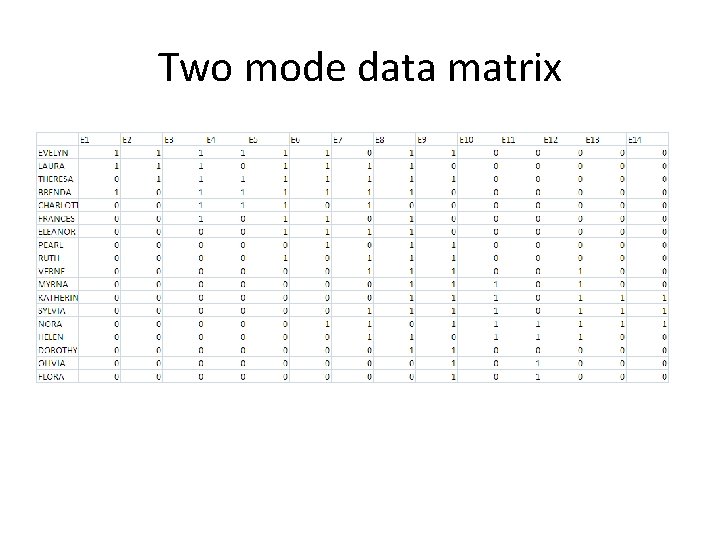
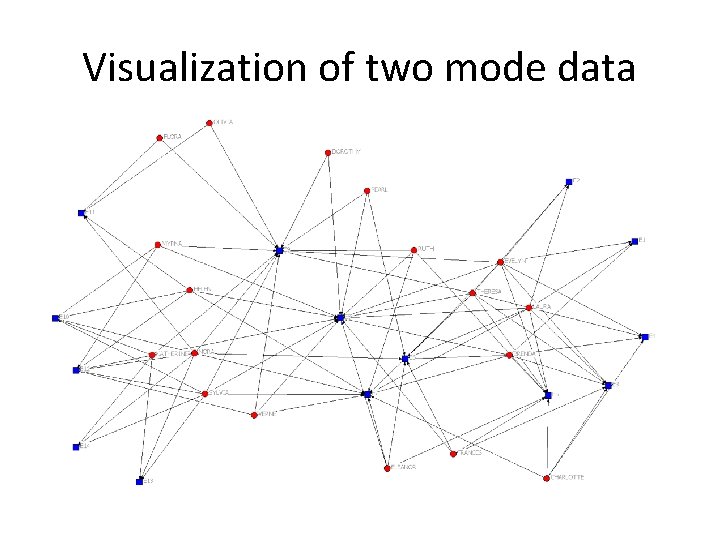
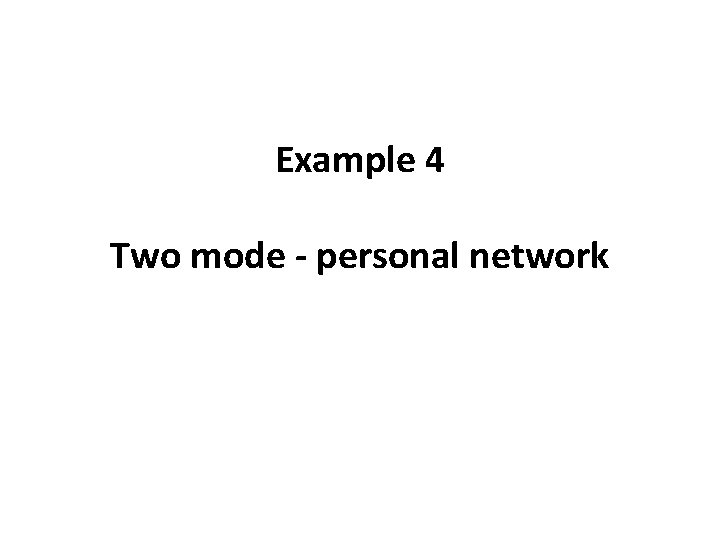
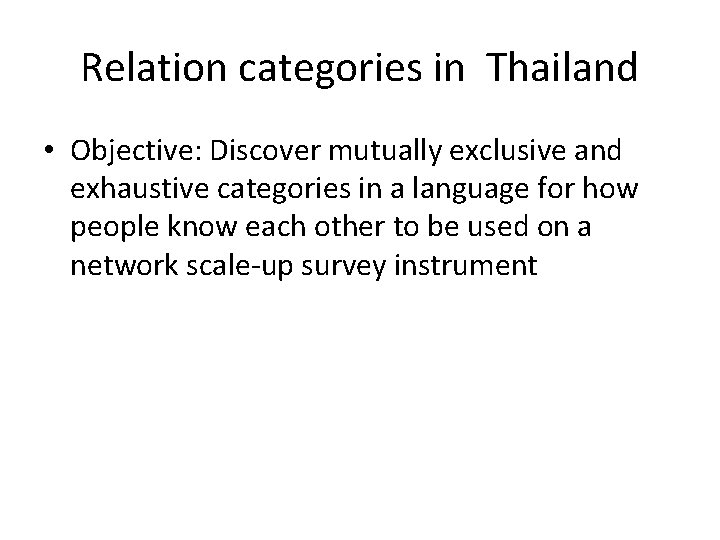
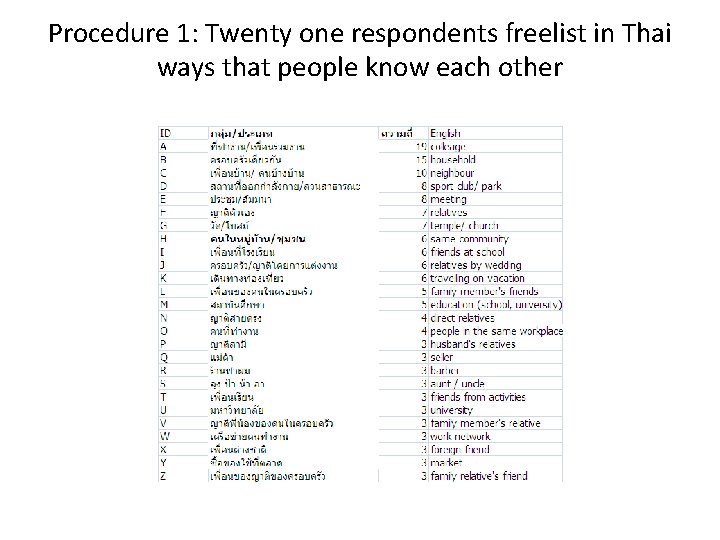
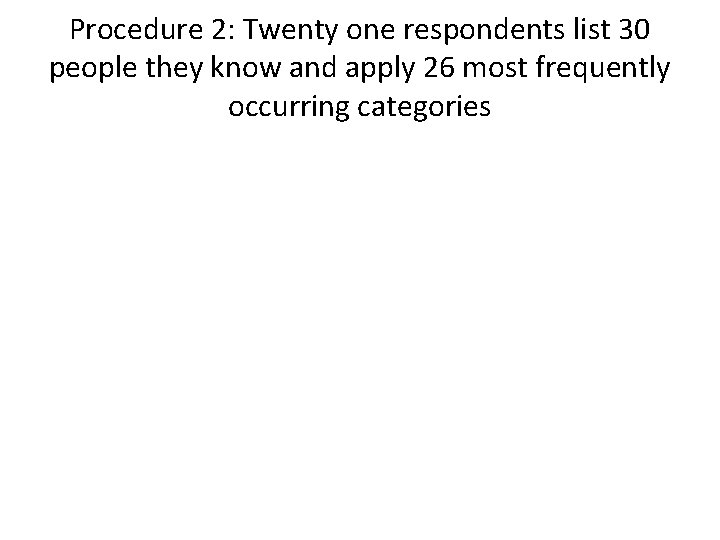
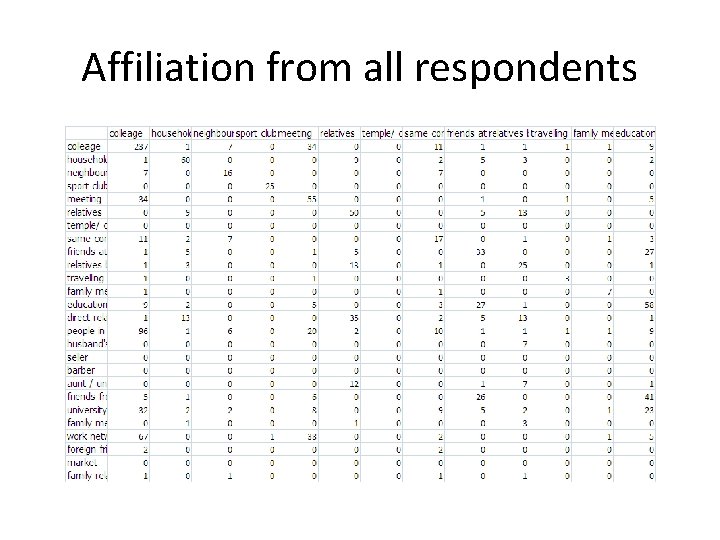
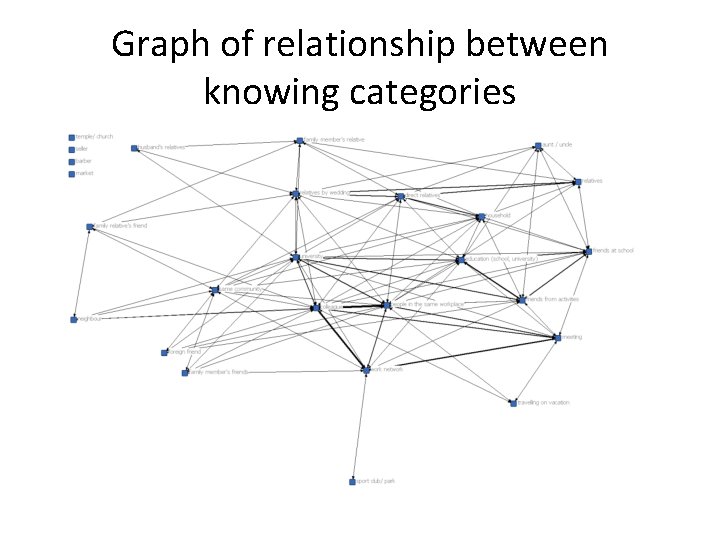
- Slides: 47
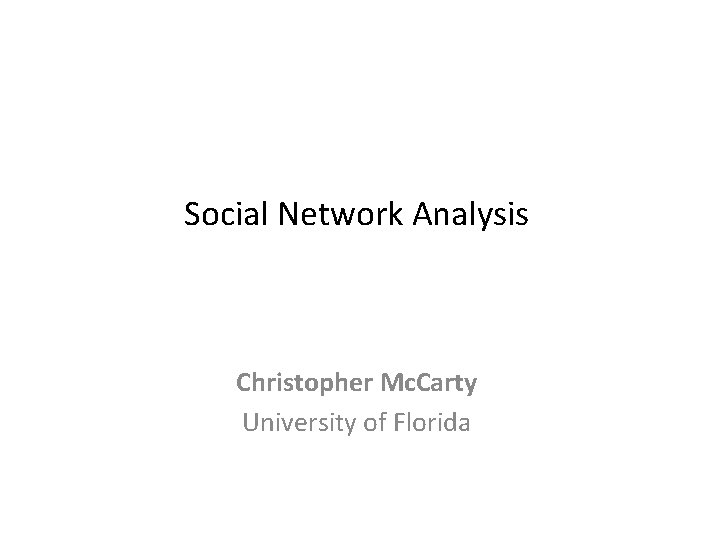
Social Network Analysis Christopher Mc. Carty University of Florida
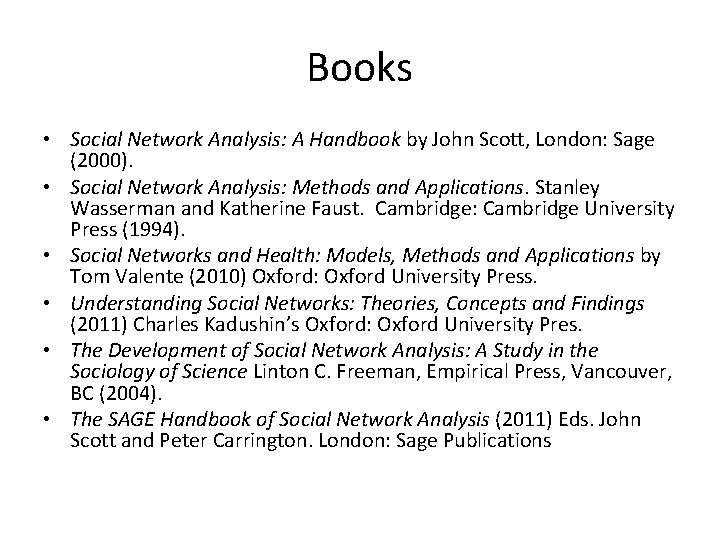
Books • Social Network Analysis: A Handbook by John Scott, London: Sage (2000). • Social Network Analysis: Methods and Applications. Stanley Wasserman and Katherine Faust. Cambridge: Cambridge University Press (1994). • Social Networks and Health: Models, Methods and Applications by Tom Valente (2010) Oxford: Oxford University Press. • Understanding Social Networks: Theories, Concepts and Findings (2011) Charles Kadushin’s Oxford: Oxford University Pres. • The Development of Social Network Analysis: A Study in the Sociology of Science Linton C. Freeman, Empirical Press, Vancouver, BC (2004). • The SAGE Handbook of Social Network Analysis (2011) Eds. John Scott and Peter Carrington. London: Sage Publications
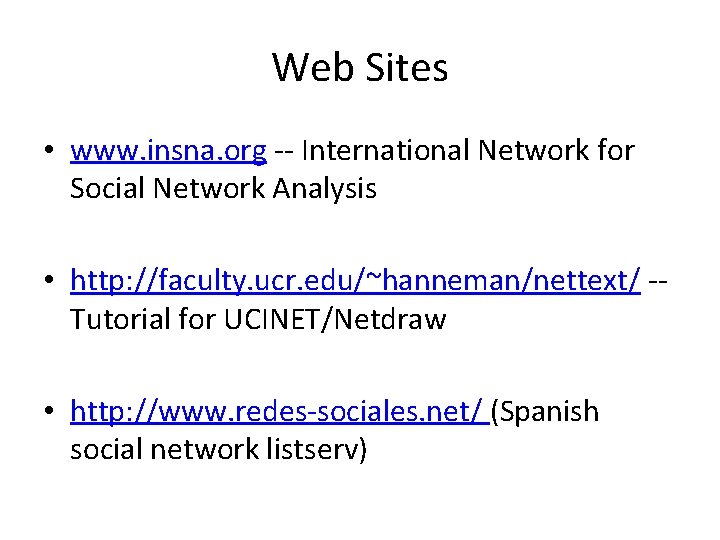
Web Sites • www. insna. org -- International Network for Social Network Analysis • http: //faculty. ucr. edu/~hanneman/nettext/ -Tutorial for UCINET/Netdraw • http: //www. redes-sociales. net/ (Spanish social network listserv)
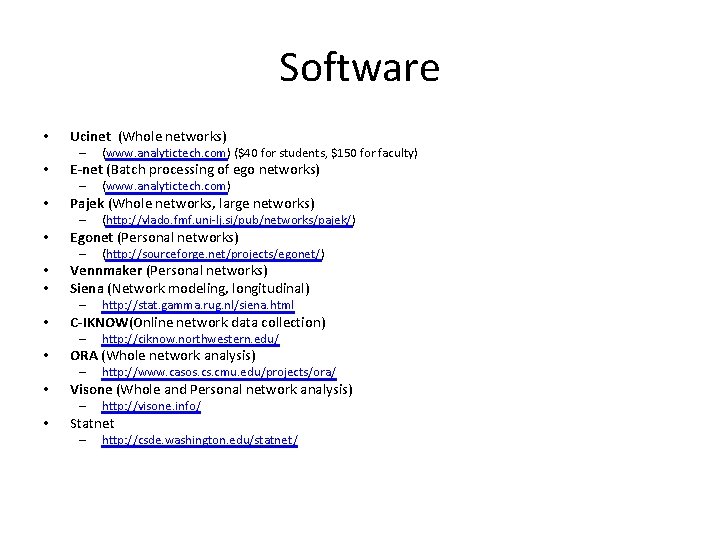
Software • Ucinet (Whole networks) – • E-net (Batch processing of ego networks) – • http: //www. casos. cmu. edu/projects/ora/ Visone (Whole and Personal network analysis) – • http: //ciknow. northwestern. edu/ ORA (Whole network analysis) – • http: //stat. gamma. rug. nl/siena. html C-IKNOW(Online network data collection) – • (http: //sourceforge. net/projects/egonet/) Vennmaker (Personal networks) Siena (Network modeling, longitudinal) – • (http: //vlado. fmf. uni-lj. si/pub/networks/pajek/) Egonet (Personal networks) – • • (www. analytictech. com) Pajek (Whole networks, large networks) – • (www. analytictech. com) ($40 for students, $150 for faculty) http: //visone. info/ Statnet – http: //csde. washington. edu/statnet/
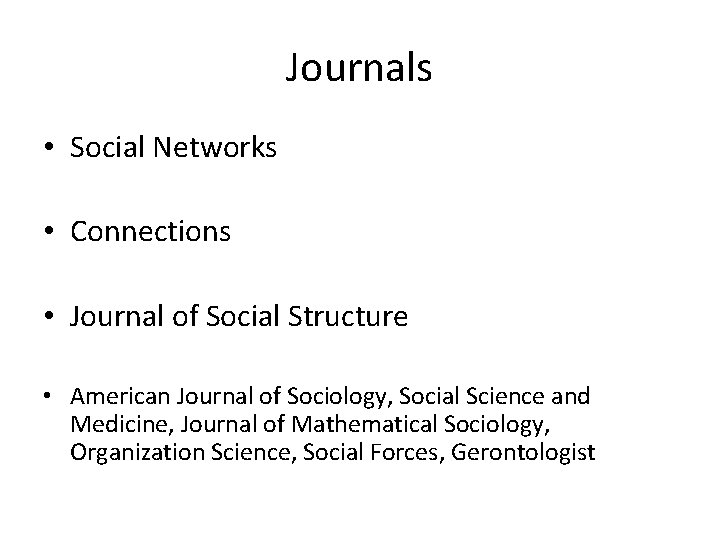
Journals • Social Networks • Connections • Journal of Social Structure • American Journal of Sociology, Social Science and Medicine, Journal of Mathematical Sociology, Organization Science, Social Forces, Gerontologist
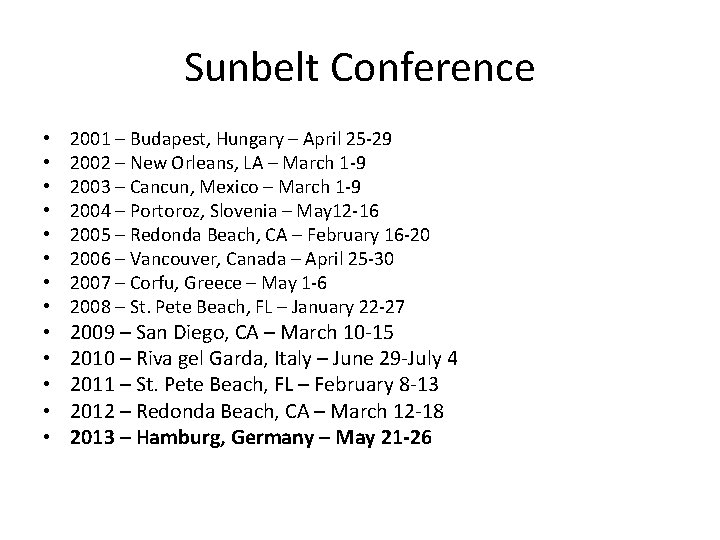
Sunbelt Conference • • • • 2001 – Budapest, Hungary – April 25 -29 2002 – New Orleans, LA – March 1 -9 2003 – Cancun, Mexico – March 1 -9 2004 – Portoroz, Slovenia – May 12 -16 2005 – Redonda Beach, CA – February 16 -20 2006 – Vancouver, Canada – April 25 -30 2007 – Corfu, Greece – May 1 -6 2008 – St. Pete Beach, FL – January 22 -27 2009 – San Diego, CA – March 10 -15 2010 – Riva gel Garda, Italy – June 29 -July 4 2011 – St. Pete Beach, FL – February 8 -13 2012 – Redonda Beach, CA – March 12 -18 2013 – Hamburg, Germany – May 21 -26
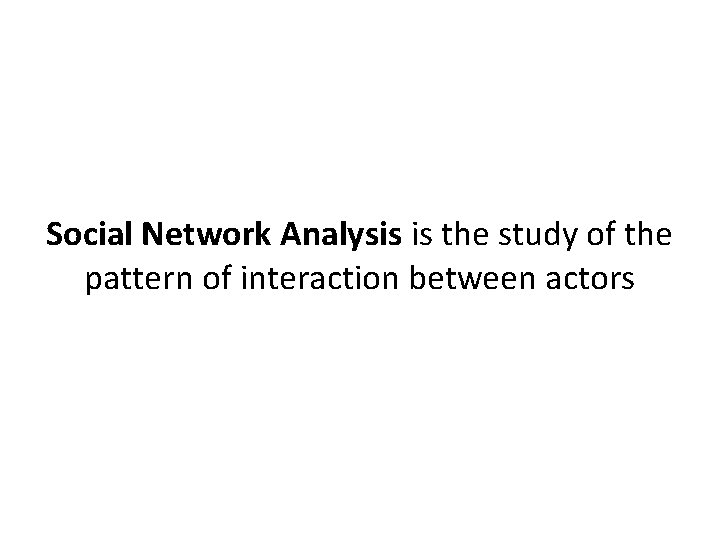
Social Network Analysis is the study of the pattern of interaction between actors
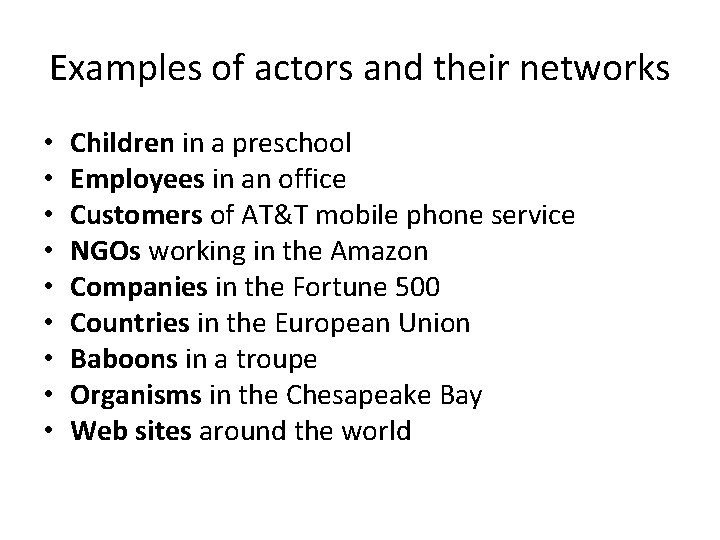
Examples of actors and their networks • • • Children in a preschool Employees in an office Customers of AT&T mobile phone service NGOs working in the Amazon Companies in the Fortune 500 Countries in the European Union Baboons in a troupe Organisms in the Chesapeake Bay Web sites around the world
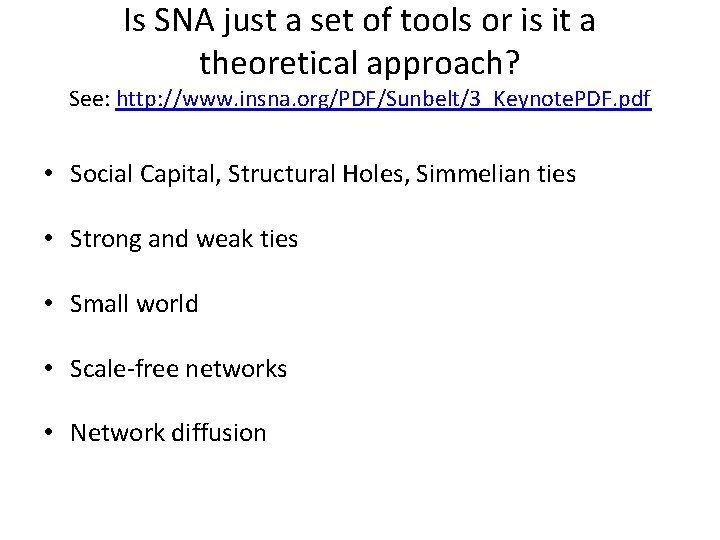
Is SNA just a set of tools or is it a theoretical approach? See: http: //www. insna. org/PDF/Sunbelt/3_Keynote. PDF. pdf • Social Capital, Structural Holes, Simmelian ties • Strong and weak ties • Small world • Scale-free networks • Network diffusion
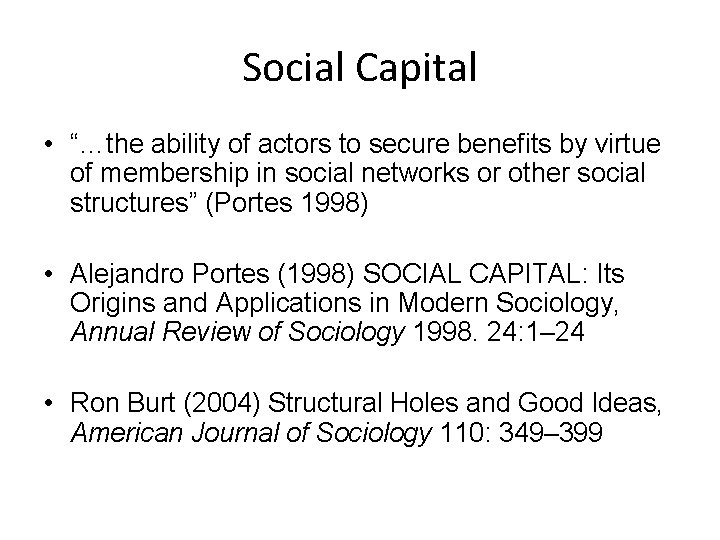
Social Capital • “…the ability of actors to secure benefits by virtue of membership in social networks or other social structures” (Portes 1998) • Alejandro Portes (1998) SOCIAL CAPITAL: Its Origins and Applications in Modern Sociology, Annual Review of Sociology 1998. 24: 1– 24 • Ron Burt (2004) Structural Holes and Good Ideas, American Journal of Sociology 110: 349– 399
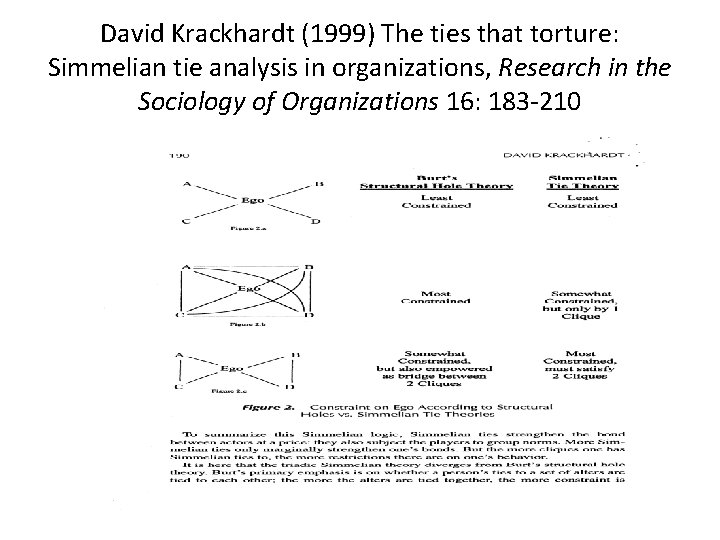
David Krackhardt (1999) The ties that torture: Simmelian tie analysis in organizations, Research in the Sociology of Organizations 16: 183 -210
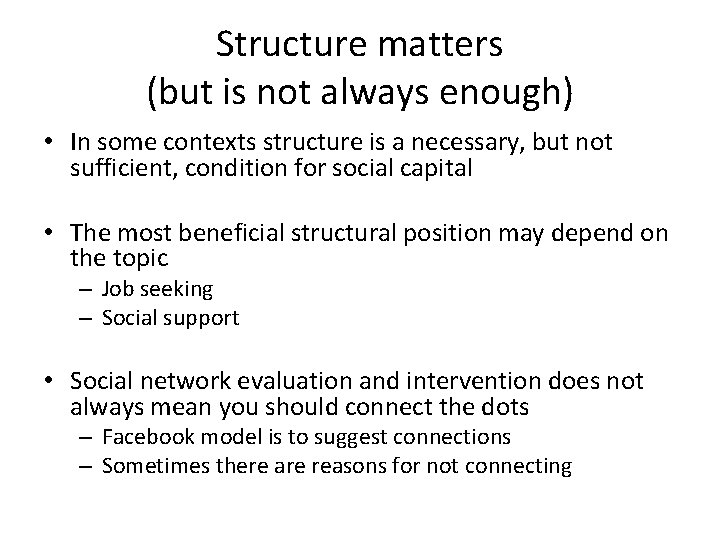
Structure matters (but is not always enough) • In some contexts structure is a necessary, but not sufficient, condition for social capital • The most beneficial structural position may depend on the topic – Job seeking – Social support • Social network evaluation and intervention does not always mean you should connect the dots – Facebook model is to suggest connections – Sometimes there are reasons for not connecting
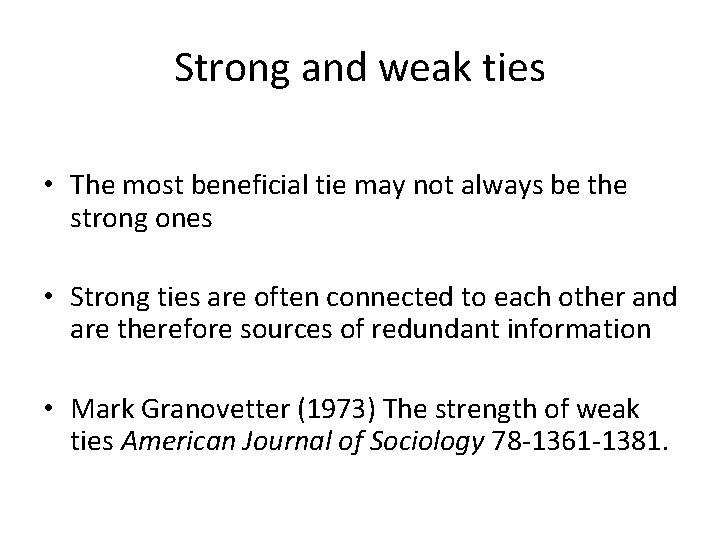
Strong and weak ties • The most beneficial tie may not always be the strong ones • Strong ties are often connected to each other and are therefore sources of redundant information • Mark Granovetter (1973) The strength of weak ties American Journal of Sociology 78 -1361 -1381.
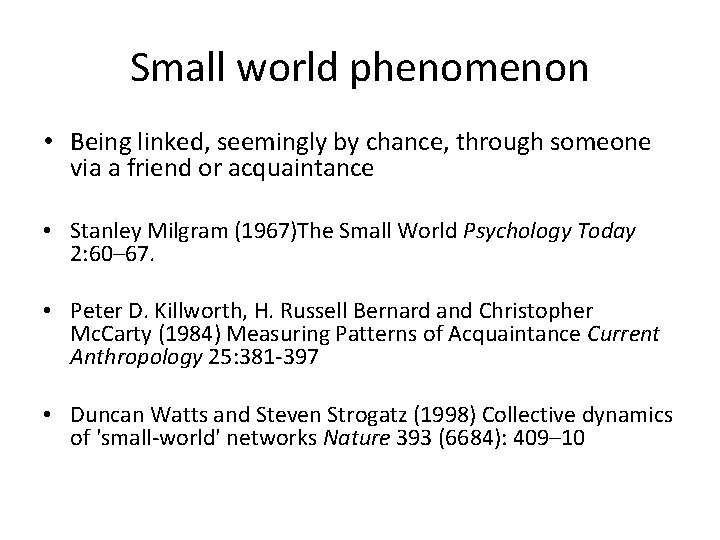
Small world phenomenon • Being linked, seemingly by chance, through someone via a friend or acquaintance • Stanley Milgram (1967)The Small World Psychology Today 2: 60– 67. • Peter D. Killworth, H. Russell Bernard and Christopher Mc. Carty (1984) Measuring Patterns of Acquaintance Current Anthropology 25: 381 -397 • Duncan Watts and Steven Strogatz (1998) Collective dynamics of 'small-world' networks Nature 393 (6684): 409– 10
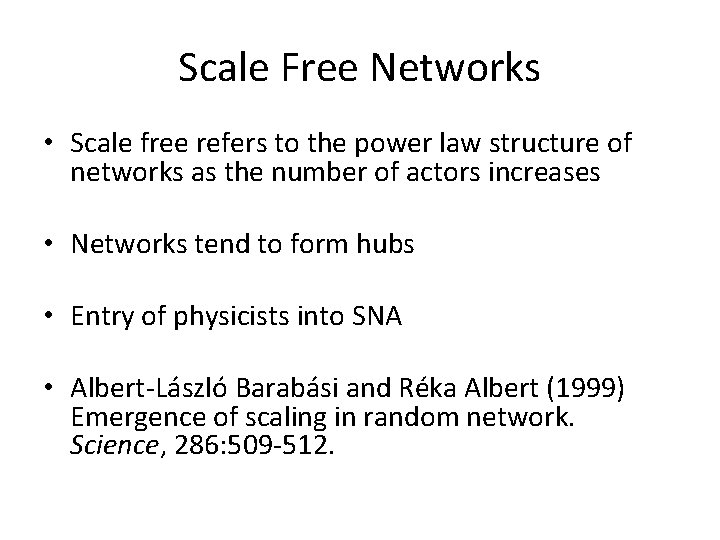
Scale Free Networks • Scale free refers to the power law structure of networks as the number of actors increases • Networks tend to form hubs • Entry of physicists into SNA • Albert-László Barabási and Réka Albert (1999) Emergence of scaling in random network. Science, 286: 509 -512.
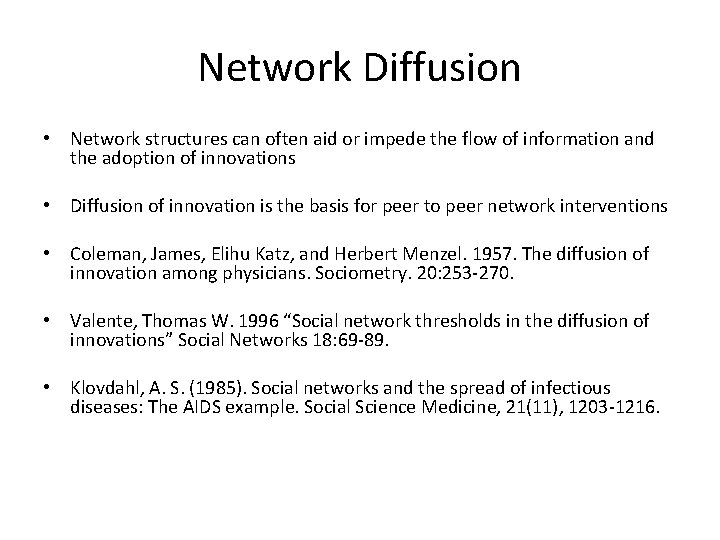
Network Diffusion • Network structures can often aid or impede the flow of information and the adoption of innovations • Diffusion of innovation is the basis for peer to peer network interventions • Coleman, James, Elihu Katz, and Herbert Menzel. 1957. The diffusion of innovation among physicians. Sociometry. 20: 253 -270. • Valente, Thomas W. 1996 “Social network thresholds in the diffusion of innovations” Social Networks 18: 69 -89. • Klovdahl, A. S. (1985). Social networks and the spread of infectious diseases: The AIDS example. Social Science Medicine, 21(11), 1203 -1216.
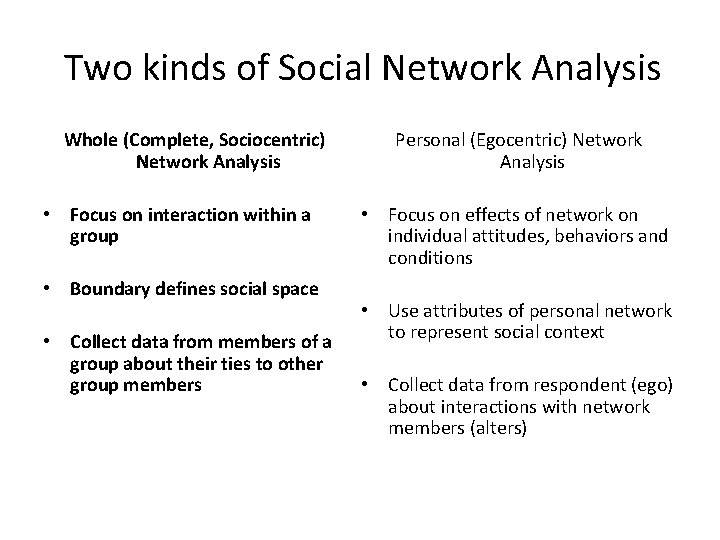
Two kinds of Social Network Analysis Whole (Complete, Sociocentric) Network Analysis • Focus on interaction within a group • Boundary defines social space • Collect data from members of a group about their ties to other group members Personal (Egocentric) Network Analysis • Focus on effects of network on individual attitudes, behaviors and conditions • Use attributes of personal network to represent social context • Collect data from respondent (ego) about interactions with network members (alters)
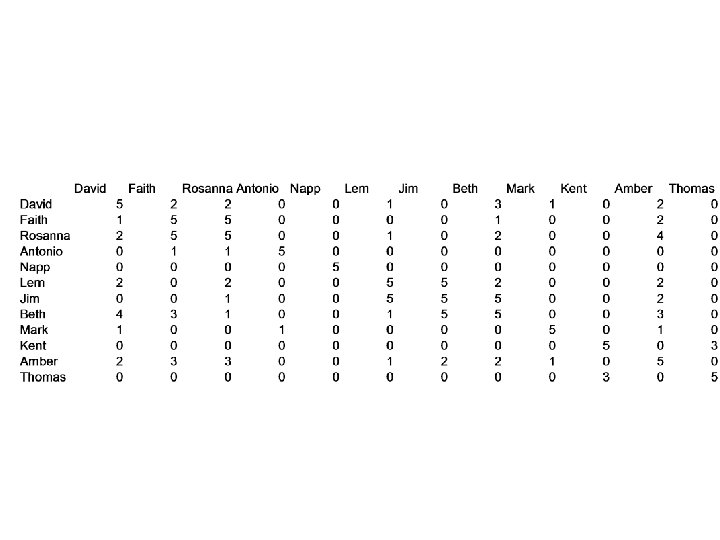
Sociocentric Network Data From Graduate Anthropology Course
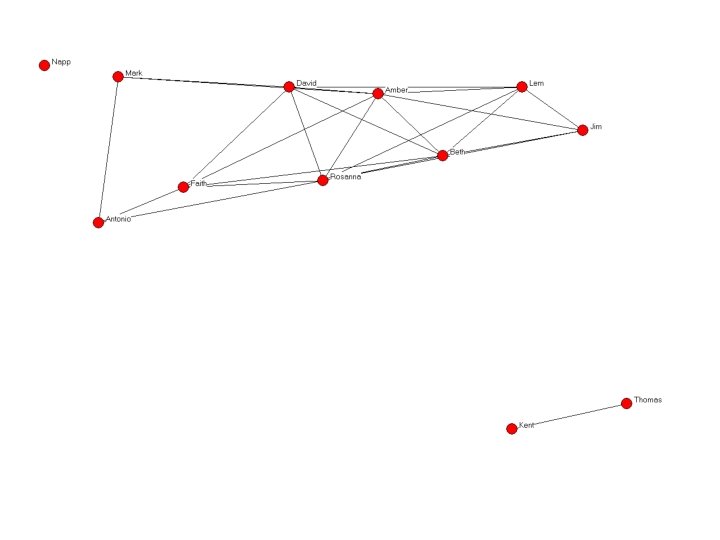
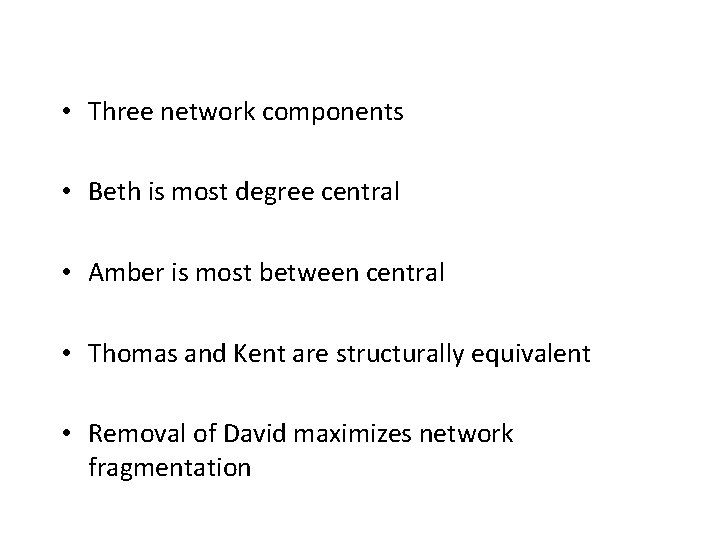
• Three network components • Beth is most degree central • Amber is most between central • Thomas and Kent are structurally equivalent • Removal of David maximizes network fragmentation
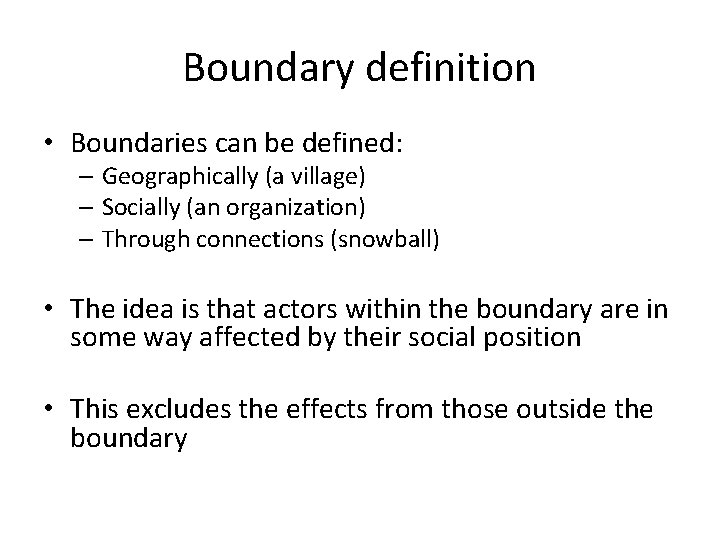
Boundary definition • Boundaries can be defined: – Geographically (a village) – Socially (an organization) – Through connections (snowball) • The idea is that actors within the boundary are in some way affected by their social position • This excludes the effects from those outside the boundary
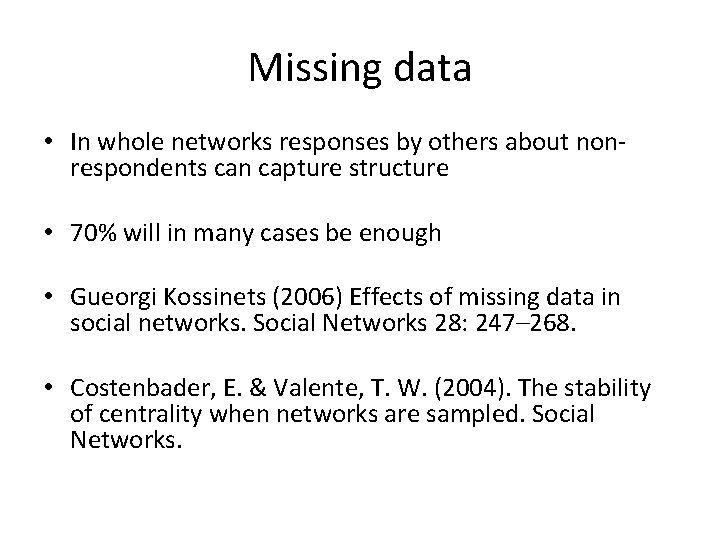
Missing data • In whole networks responses by others about nonrespondents can capture structure • 70% will in many cases be enough • Gueorgi Kossinets (2006) Effects of missing data in social networks. Social Networks 28: 247– 268. • Costenbader, E. & Valente, T. W. (2004). The stability of centrality when networks are sampled. Social Networks.
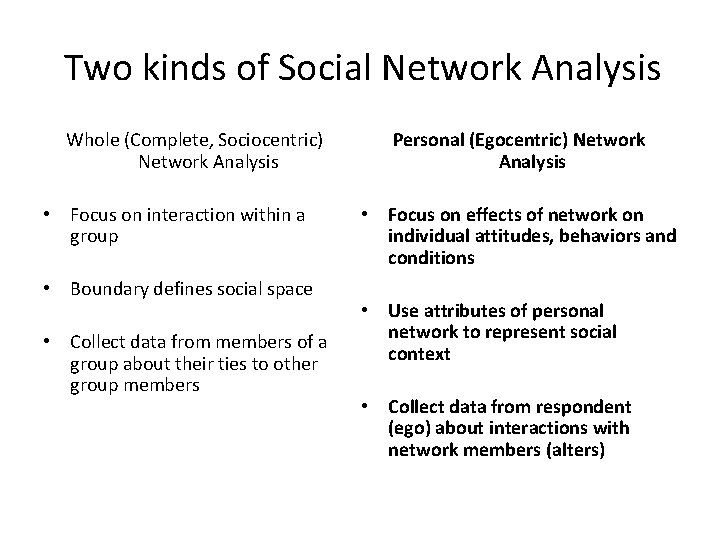
Two kinds of Social Network Analysis Whole (Complete, Sociocentric) Network Analysis • Focus on interaction within a group • Boundary defines social space • Collect data from members of a group about their ties to other group members Personal (Egocentric) Network Analysis • Focus on effects of network on individual attitudes, behaviors and conditions • Use attributes of personal network to represent social context • Collect data from respondent (ego) about interactions with network members (alters)
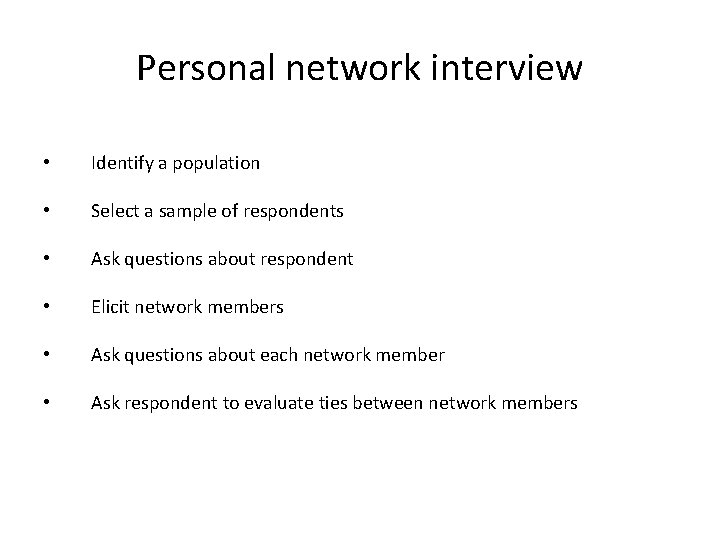
Personal network interview • Identify a population • Select a sample of respondents • Ask questions about respondent • Elicit network members • Ask questions about each network member • Ask respondent to evaluate ties between network members
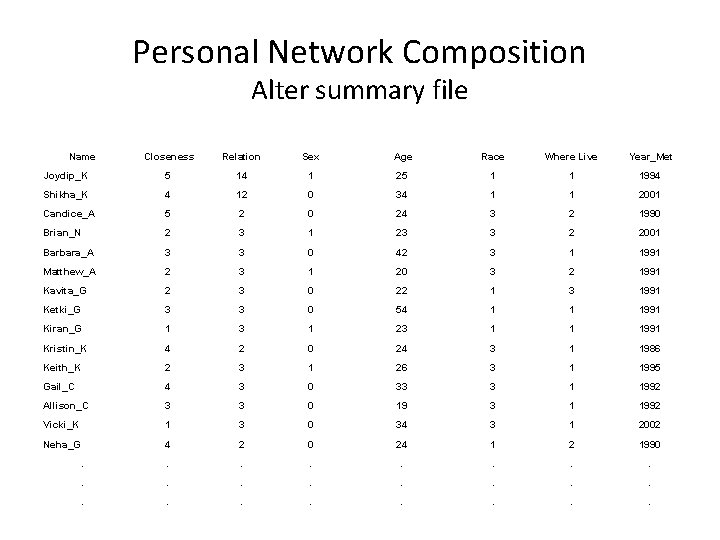
Personal Network Composition Alter summary file Name Closeness Relation Sex Age Race Where Live Year_Met Joydip_K 5 14 1 25 1 1 1994 Shikha_K 4 12 0 34 1 1 2001 Candice_A 5 2 0 24 3 2 1990 Brian_N 2 3 1 23 3 2 2001 Barbara_A 3 3 0 42 3 1 1991 Matthew_A 2 3 1 20 3 2 1991 Kavita_G 2 3 0 22 1 3 1991 Ketki_G 3 3 0 54 1 1 1991 Kiran_G 1 3 1 23 1 1 1991 Kristin_K 4 2 0 24 3 1 1986 Keith_K 2 3 1 26 3 1 1995 Gail_C 4 3 0 33 3 1 1992 Allison_C 3 3 0 19 3 1 1992 Vicki_K 1 3 0 34 3 1 2002 Neha_G 4 2 0 24 1 2 1990 . . .
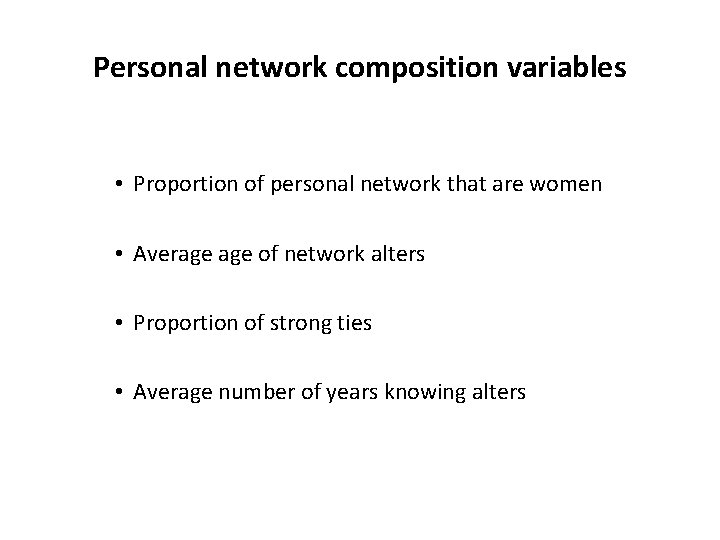
Personal network composition variables • Proportion of personal network that are women • Average of network alters • Proportion of strong ties • Average number of years knowing alters
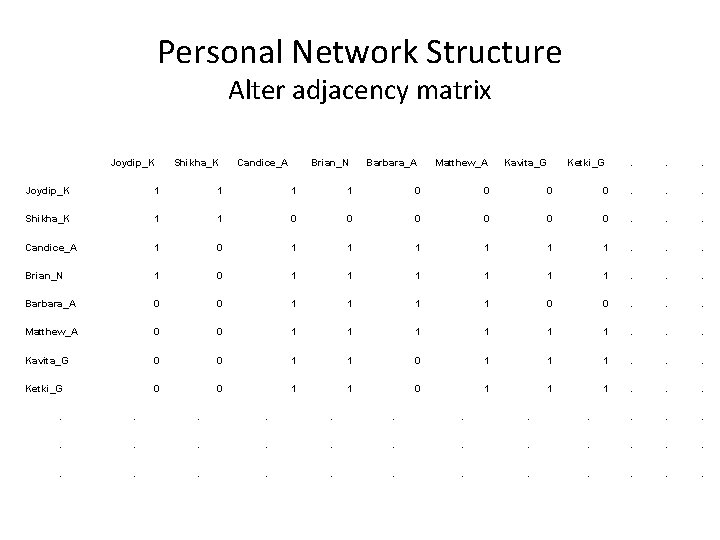
Personal Network Structure Alter adjacency matrix Joydip_K Shikha_K Candice_A Brian_N Barbara_A Matthew_A Kavita_G Ketki_G . . . Joydip_K 1 1 0 0 . . . Shikha_K 1 1 0 0 0 . . . Candice_A 1 0 1 1 1 . . . Brian_N 1 0 1 1 1 . . . Barbara_A 0 0 1 1 0 0 . . . Matthew_A 0 0 1 1 1 . . . Kavita_G 0 0 1 1 1 . . . Ketki_G 0 0 1 1 1 . .
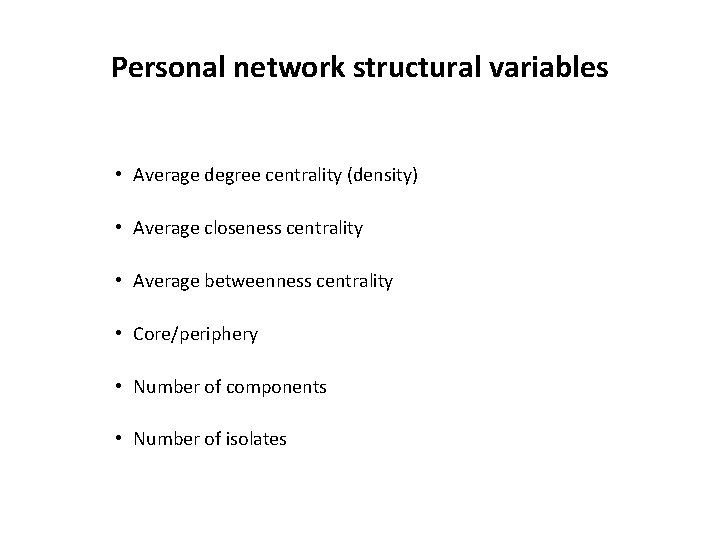
Personal network structural variables • Average degree centrality (density) • Average closeness centrality • Average betweenness centrality • Core/periphery • Number of components • Number of isolates
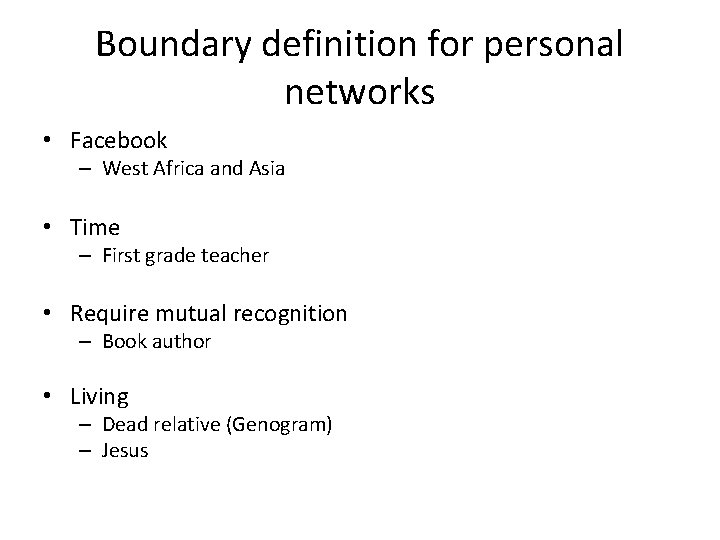
Boundary definition for personal networks • Facebook – West Africa and Asia • Time – First grade teacher • Require mutual recognition – Book author • Living – Dead relative (Genogram) – Jesus
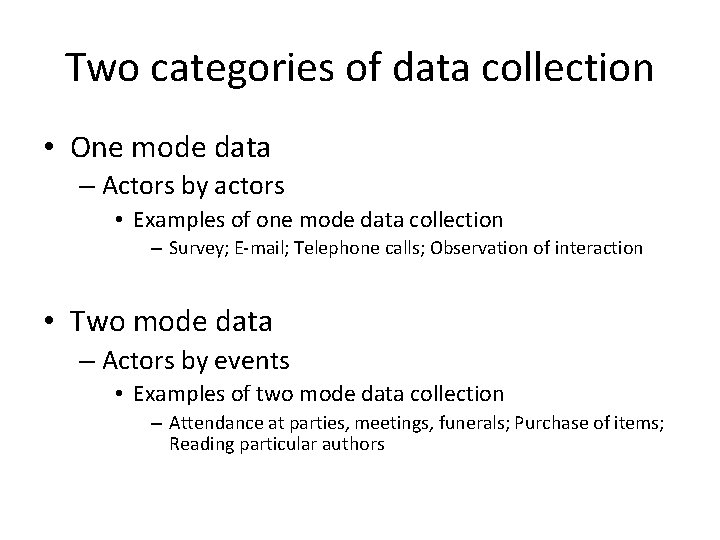
Two categories of data collection • One mode data – Actors by actors • Examples of one mode data collection – Survey; E-mail; Telephone calls; Observation of interaction • Two mode data – Actors by events • Examples of two mode data collection – Attendance at parties, meetings, funerals; Purchase of items; Reading particular authors
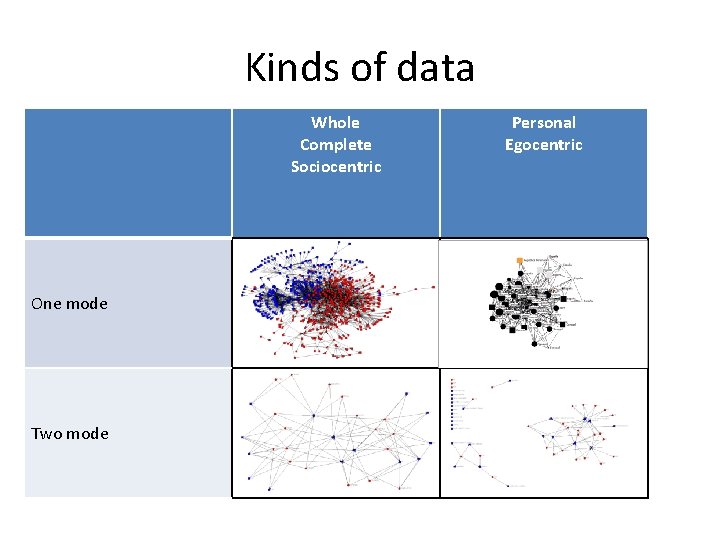
Kinds of data Whole Complete Sociocentric One mode Two mode Personal Egocentric
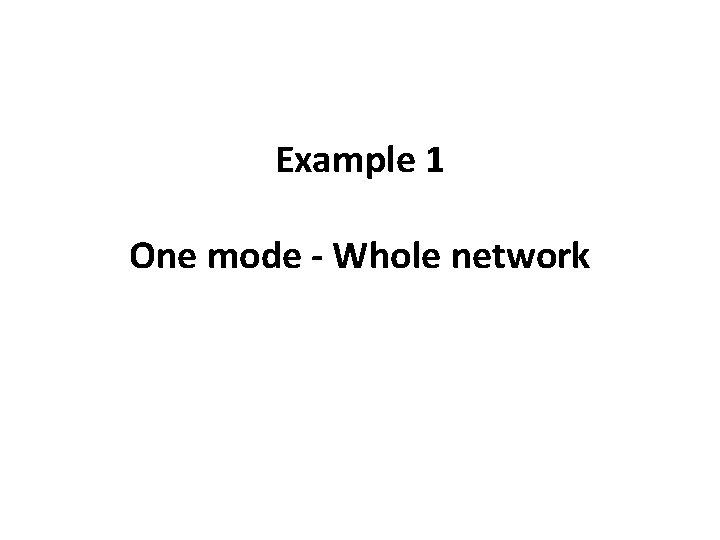
Example 1 One mode - Whole network
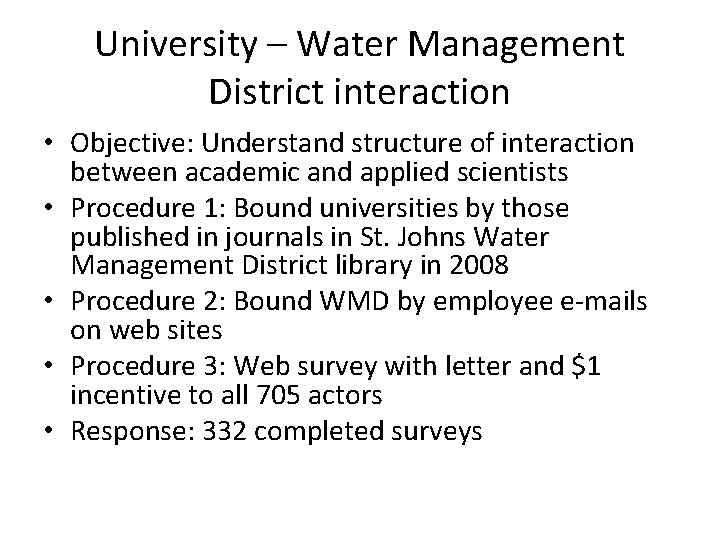
University – Water Management District interaction • Objective: Understand structure of interaction between academic and applied scientists • Procedure 1: Bound universities by those published in journals in St. Johns Water Management District library in 2008 • Procedure 2: Bound WMD by employee e-mails on web sites • Procedure 3: Web survey with letter and $1 incentive to all 705 actors • Response: 332 completed surveys
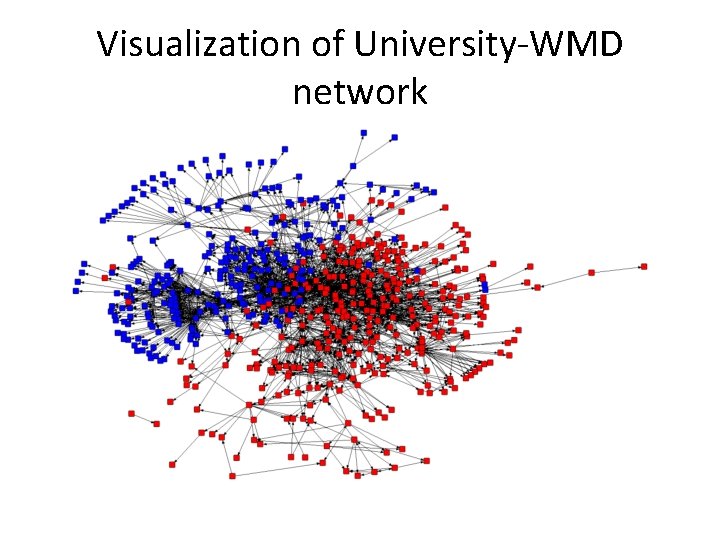
Visualization of University-WMD network
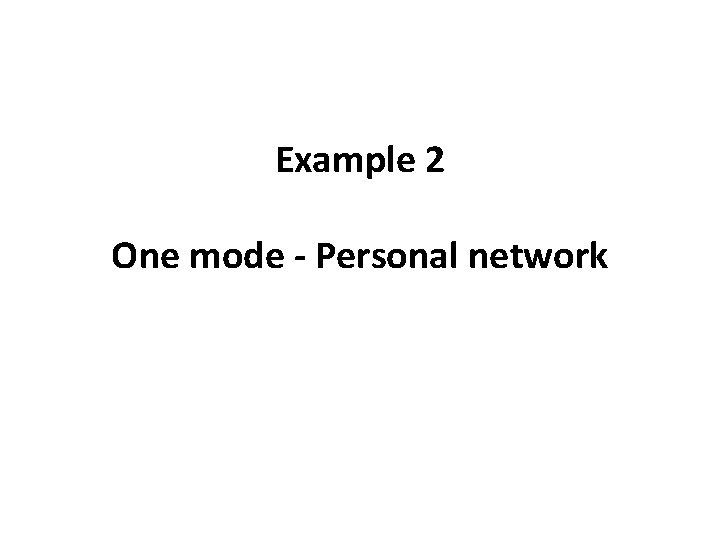
Example 2 One mode - Personal network
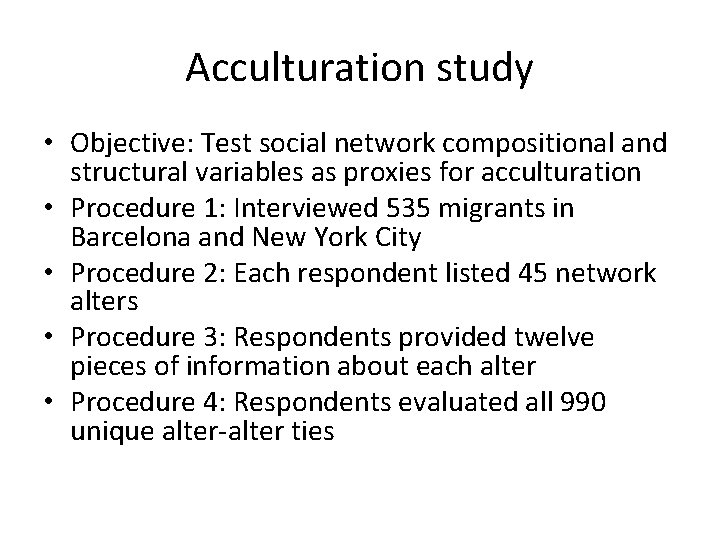
Acculturation study • Objective: Test social network compositional and structural variables as proxies for acculturation • Procedure 1: Interviewed 535 migrants in Barcelona and New York City • Procedure 2: Each respondent listed 45 network alters • Procedure 3: Respondents provided twelve pieces of information about each alter • Procedure 4: Respondents evaluated all 990 unique alter-alter ties
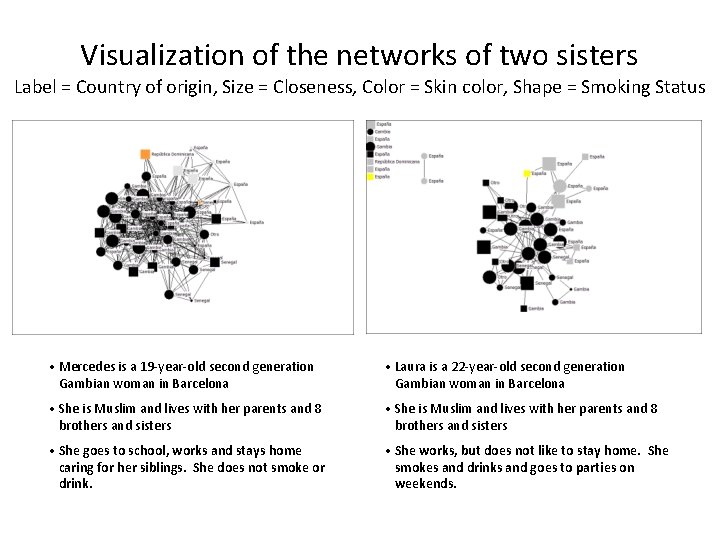
Visualization of the networks of two sisters Label = Country of origin, Size = Closeness, Color = Skin color, Shape = Smoking Status • Mercedes is a 19 -year-old second generation Gambian woman in Barcelona • Laura is a 22 -year-old second generation Gambian woman in Barcelona • She is Muslim and lives with her parents and 8 brothers and sisters • She goes to school, works and stays home caring for her siblings. She does not smoke or drink. • She works, but does not like to stay home. She smokes and drinks and goes to parties on weekends.
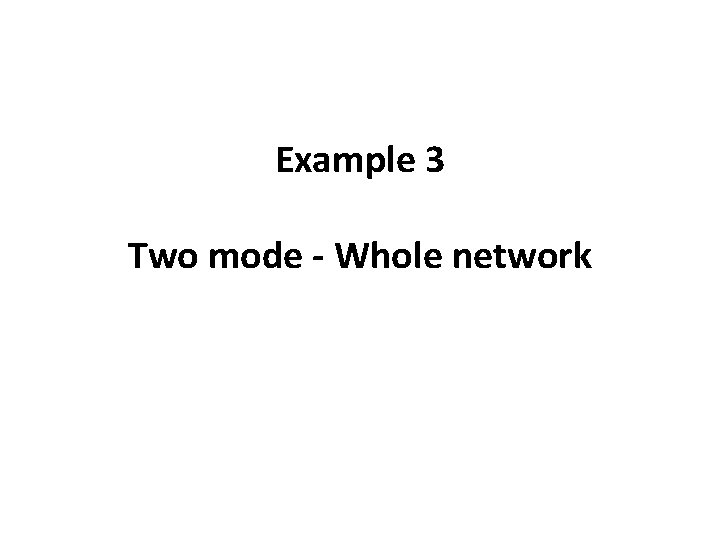
Example 3 Two mode - Whole network
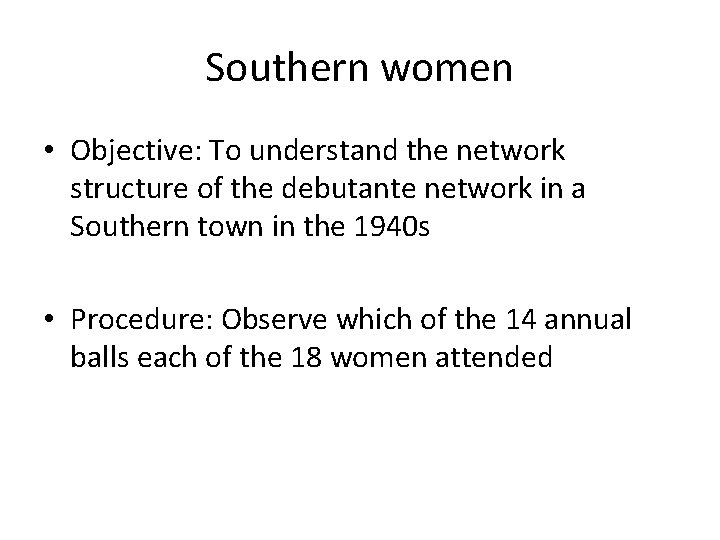
Southern women • Objective: To understand the network structure of the debutante network in a Southern town in the 1940 s • Procedure: Observe which of the 14 annual balls each of the 18 women attended
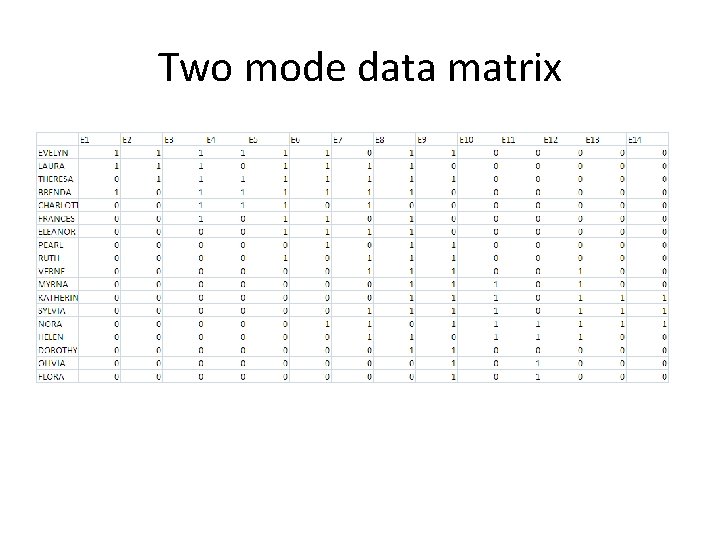
Two mode data matrix
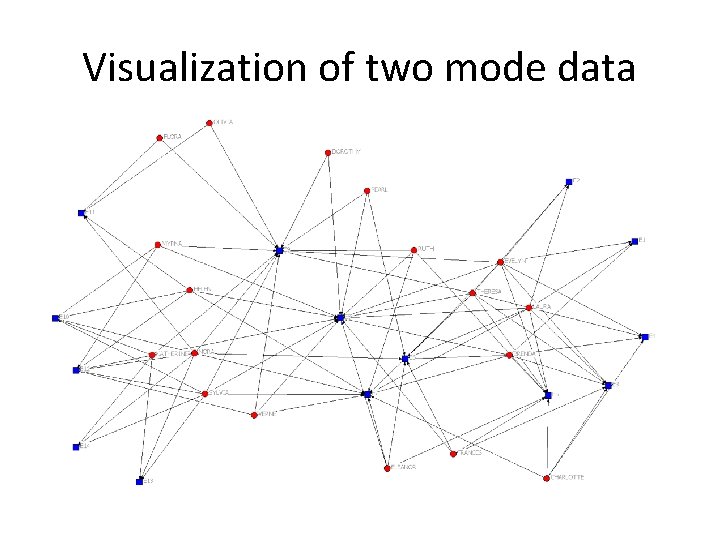
Visualization of two mode data
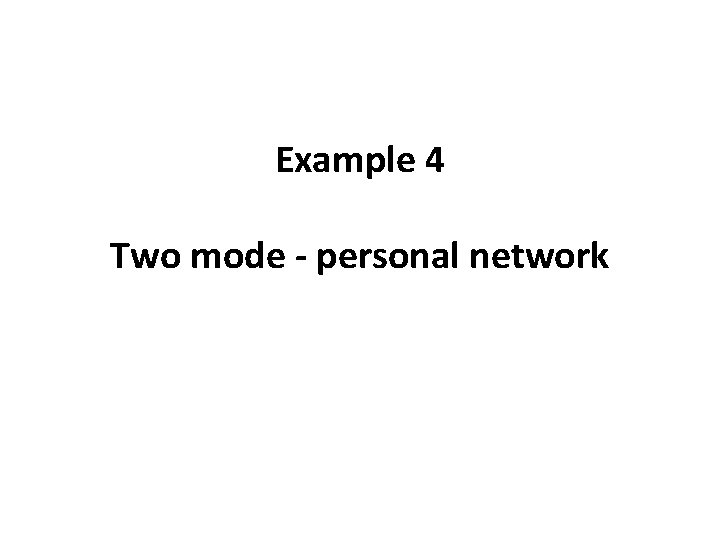
Example 4 Two mode - personal network
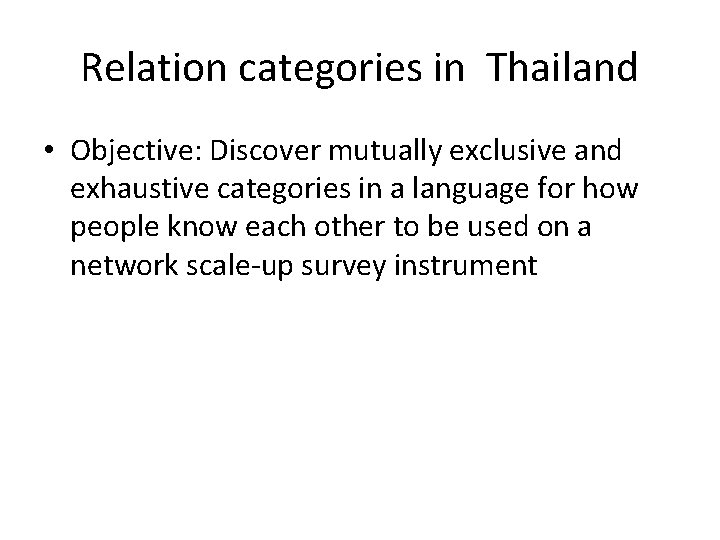
Relation categories in Thailand • Objective: Discover mutually exclusive and exhaustive categories in a language for how people know each other to be used on a network scale-up survey instrument
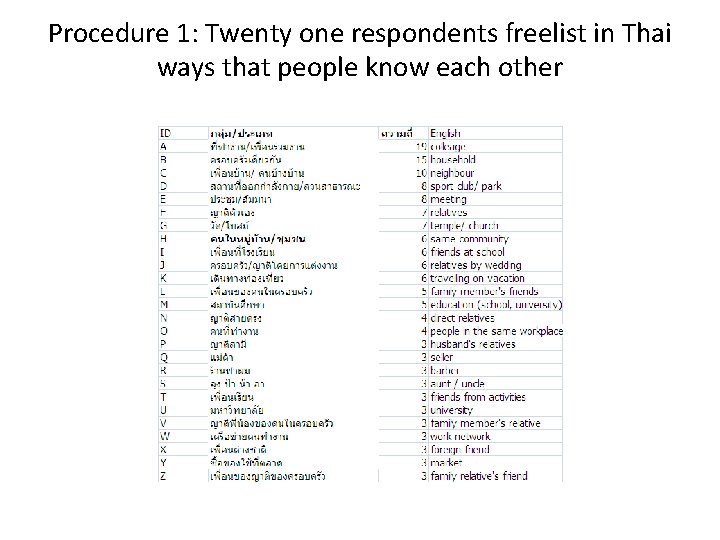
Procedure 1: Twenty one respondents freelist in Thai ways that people know each other
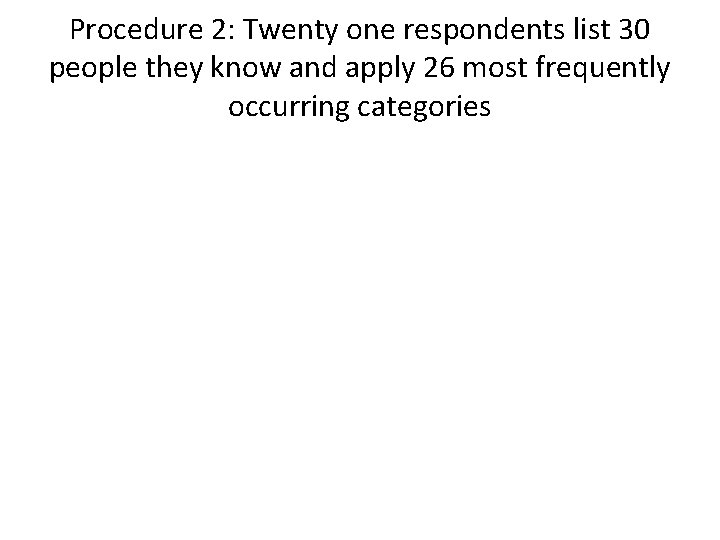
Procedure 2: Twenty one respondents list 30 people they know and apply 26 most frequently occurring categories
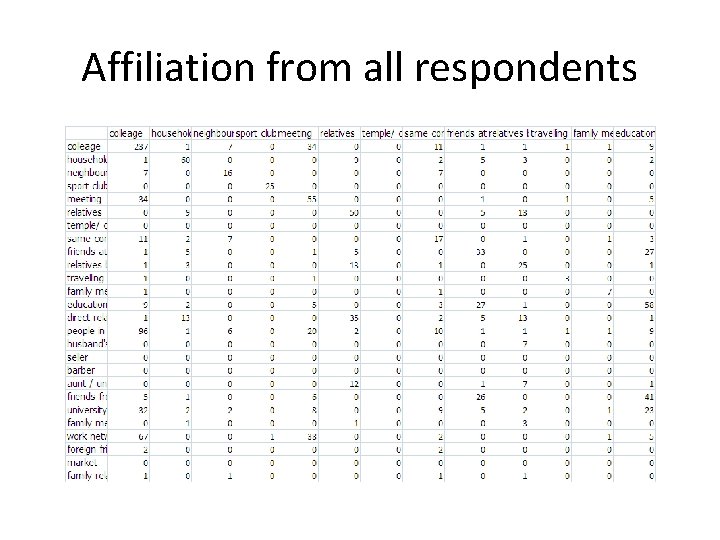
Affiliation from all respondents
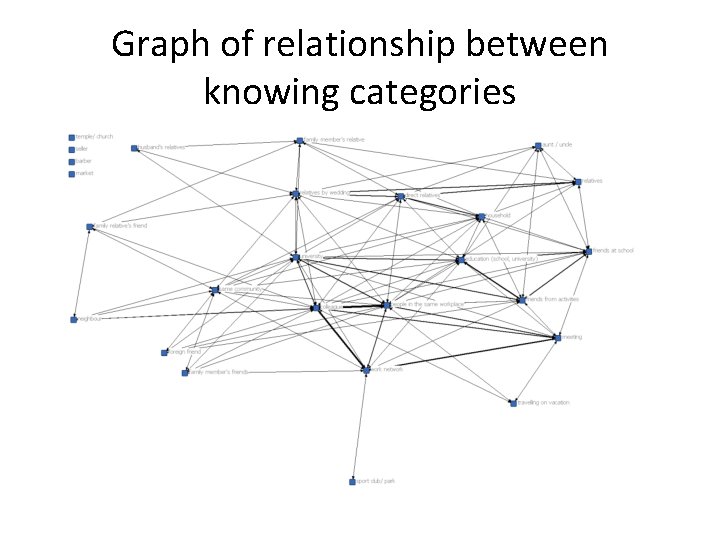
Graph of relationship between knowing categories
Jodi baker faa
Direct and indirect worksheet
Social network analysis tutorial
Network analysis for dummies
Social network analysis stata
Mitchell centre for social network analysis
Machine learning social network analysis
Pajek download
Social thinking and social influence in psychology
Social thinking social influence social relations
Difference between datagram and virtual circuit
Network topology in computer network
Features of peer to peer network and client server network
Network systems design using network processors
Network centric computing
Advantage and disadvantages of packet switching
Felicia reed watt
"university of maryland university college"
Christopher ding
Michael buehler
Christopher hirata
Cma cgm christopher columbus
Notorious big thomas
Charlene gallego victims
Devion christopher brooks
Christopher p cannon
When did christopher columbus discover america
Christopher dalley
Macbeth connections
We finally arrived at your destination
Christopher columbus sailed the ocean blue in 1492
Christopher hirata
Ghost dances costumes
Christopher tingwell
Over it all quotes
Christopher janietz
Scott kyle
Edward christopher sheeran
Doctor faustus summary act 1
Christopher geyer
Christopher s. bretherton
Christopher w tyler
Dr christopher morrissey
Christopher reeve spinal cord injury level
Facts about christopher paul curtis
Christopher marlowe biography
Where did columbus land
Columbus voyage map