Overview of Adaptive Treatment Regimes Sachiko Miyahara Dr
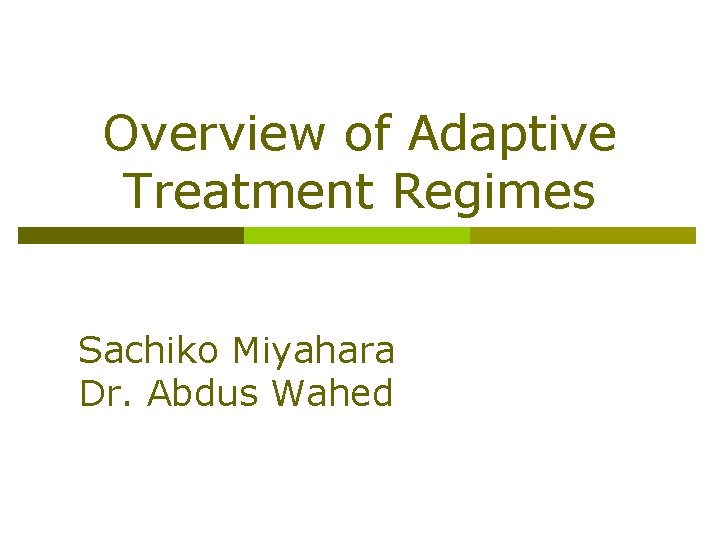
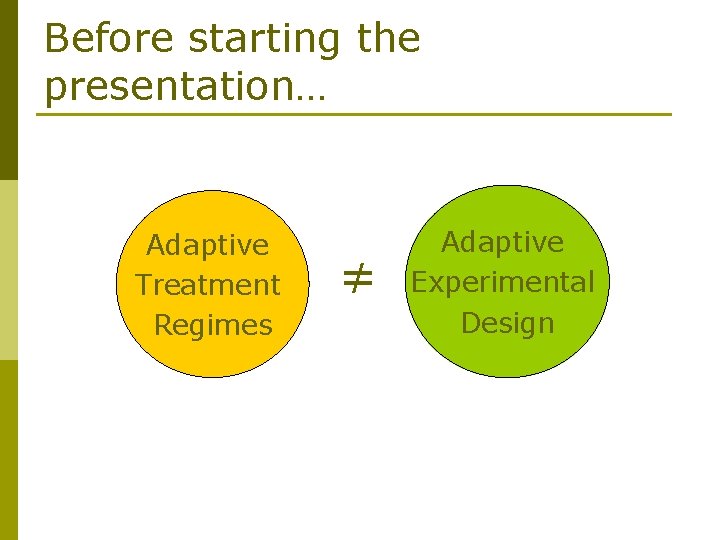
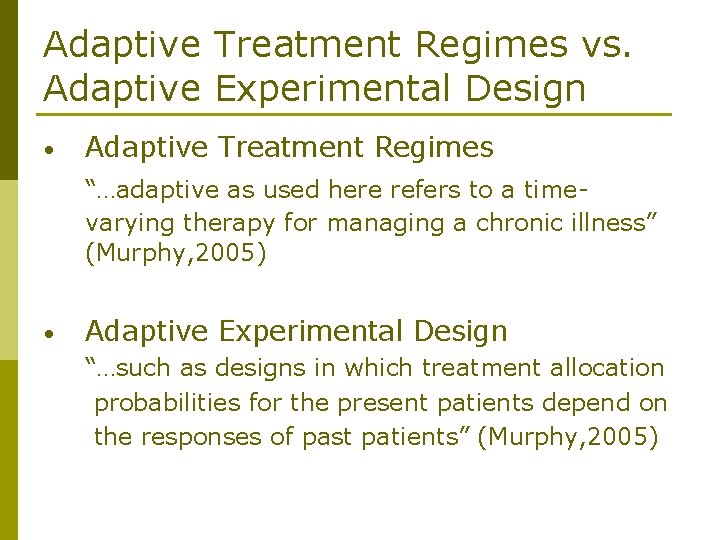
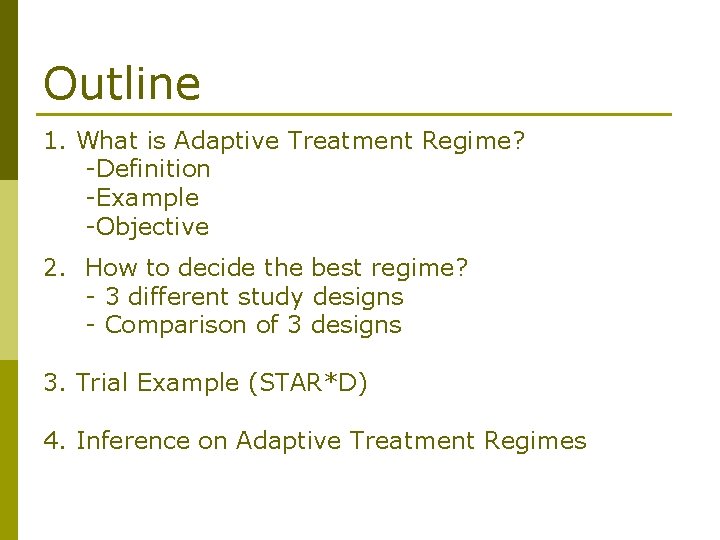
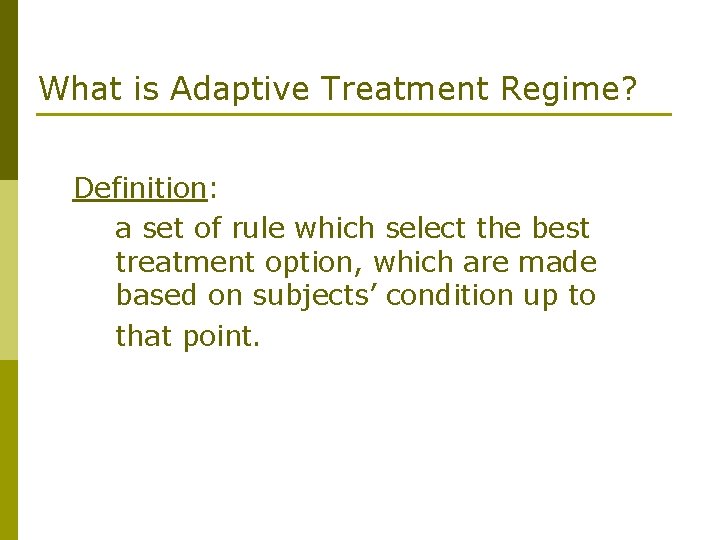
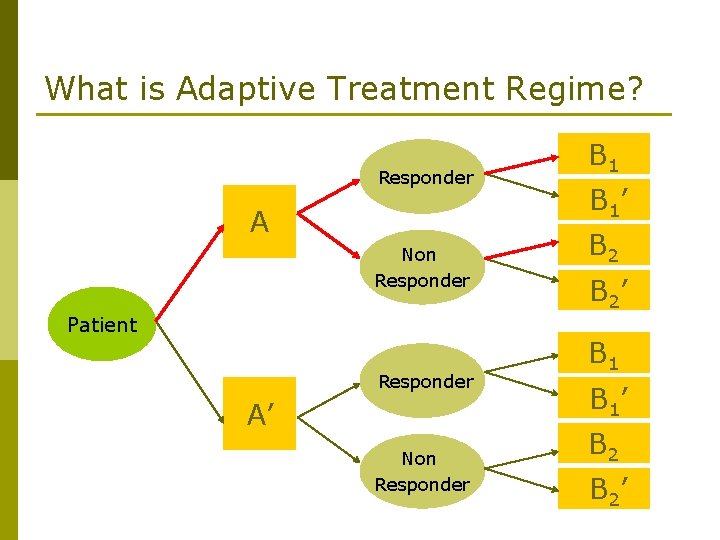
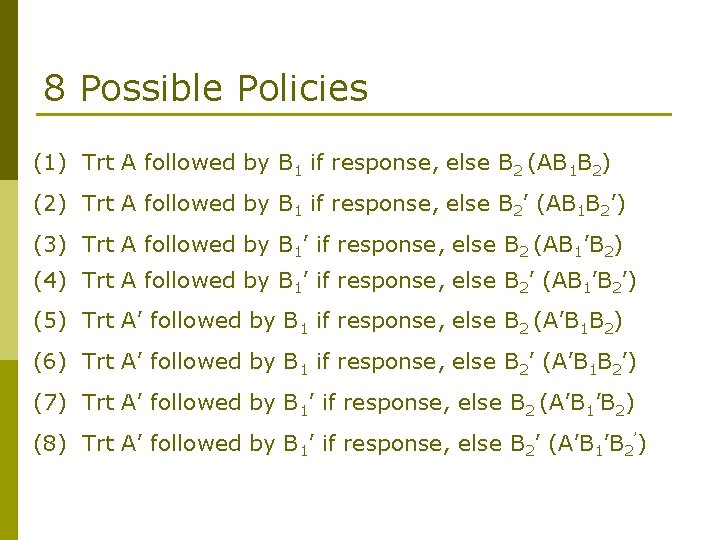
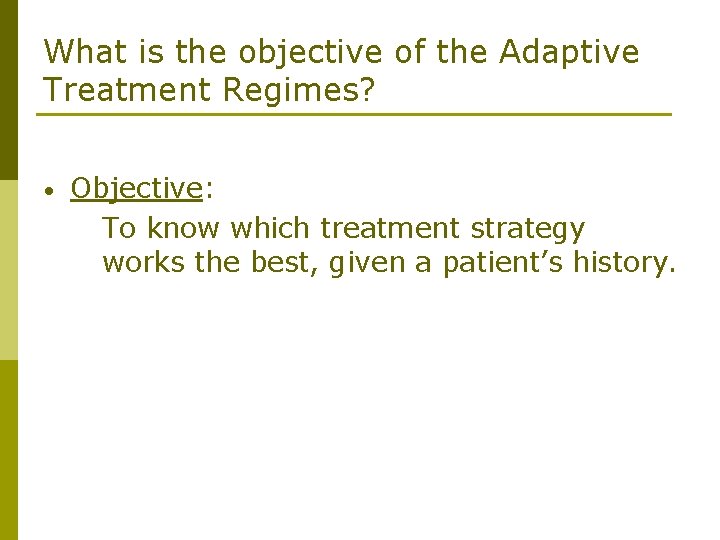
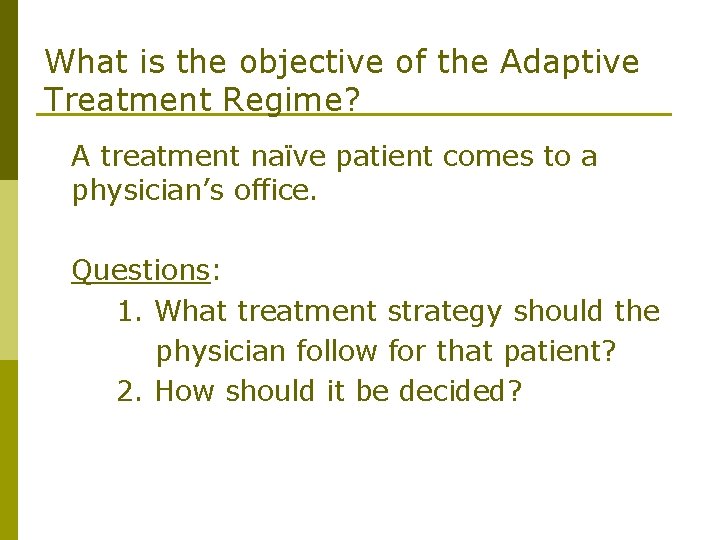
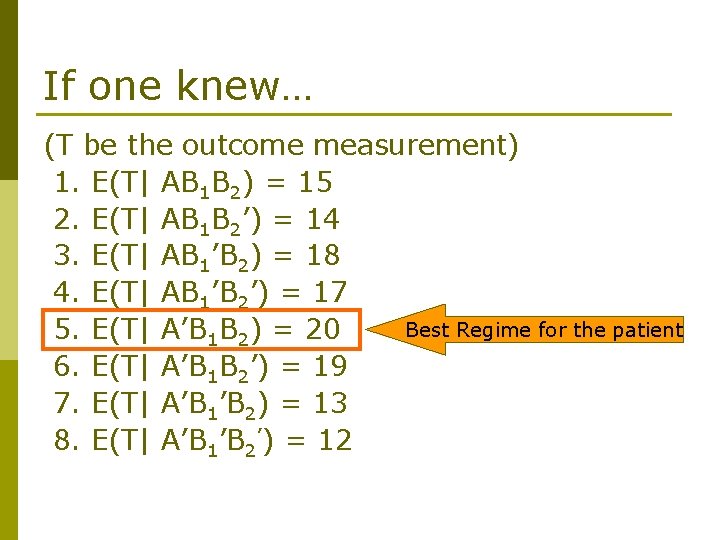
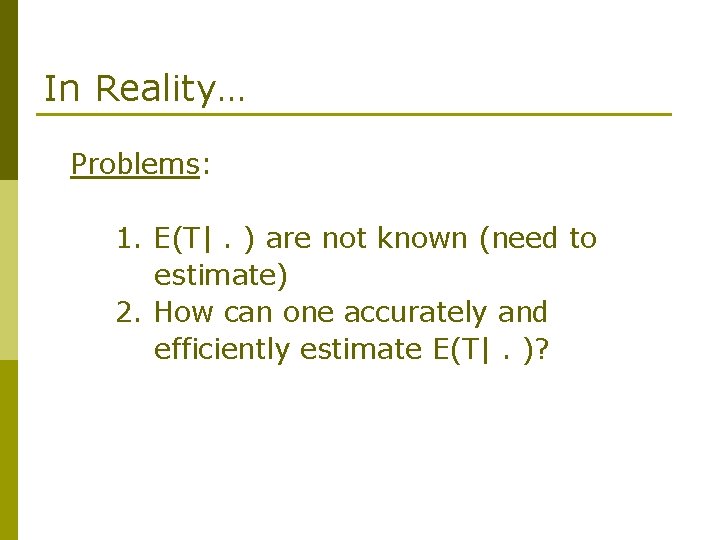
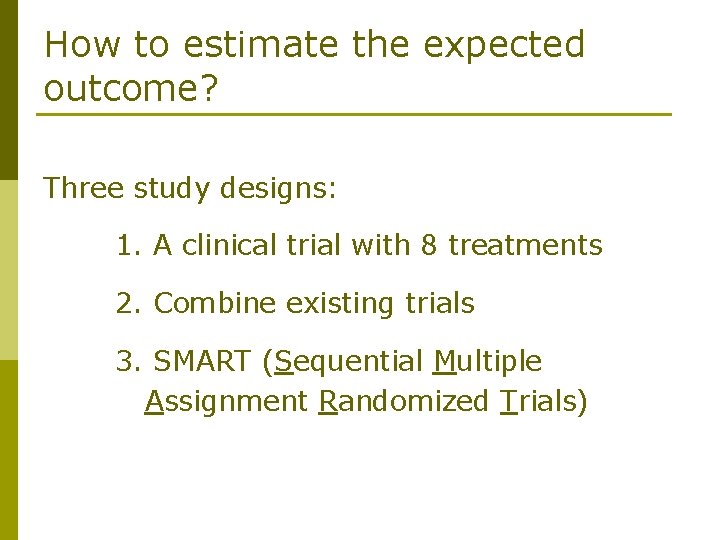
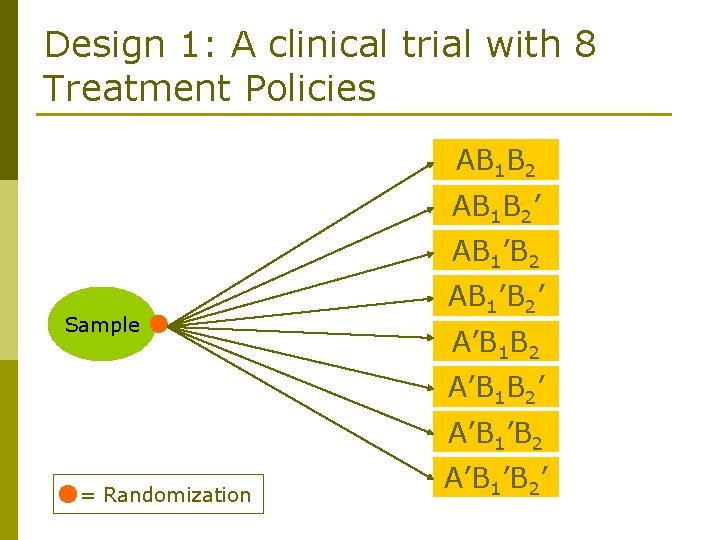
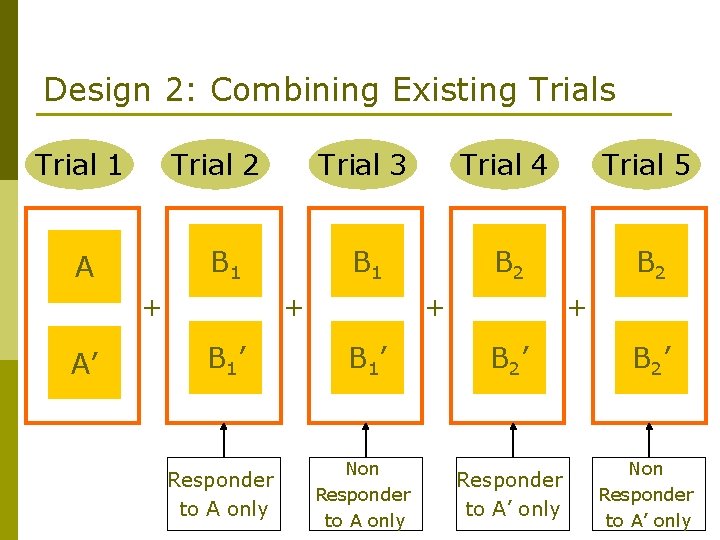
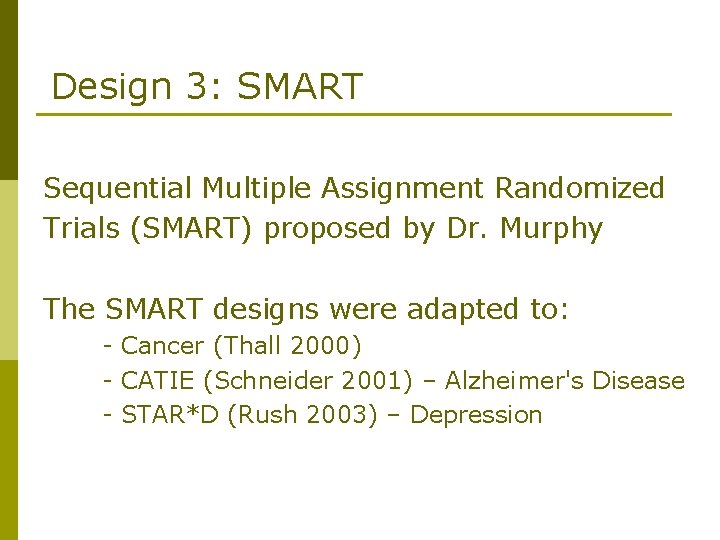
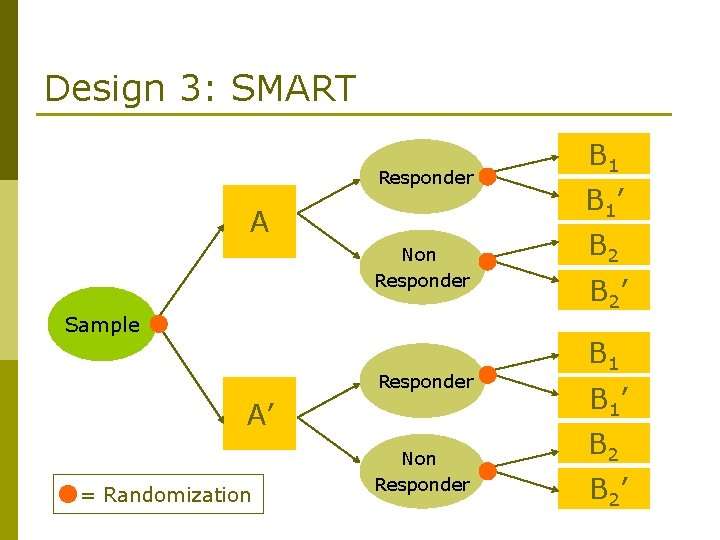
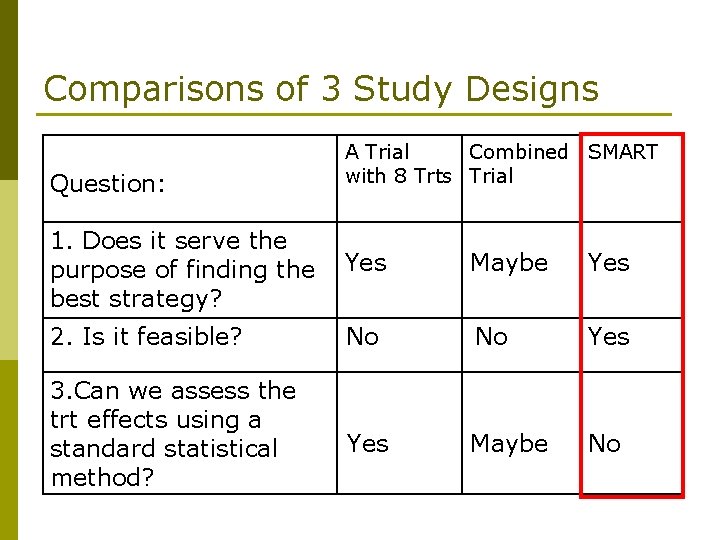
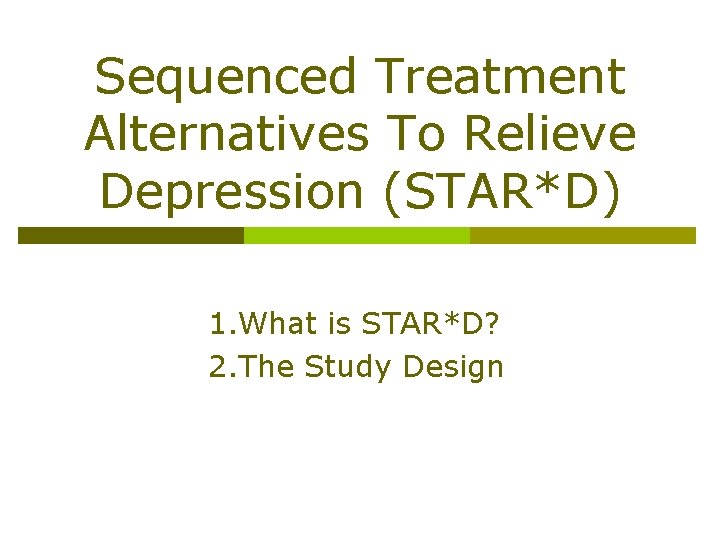
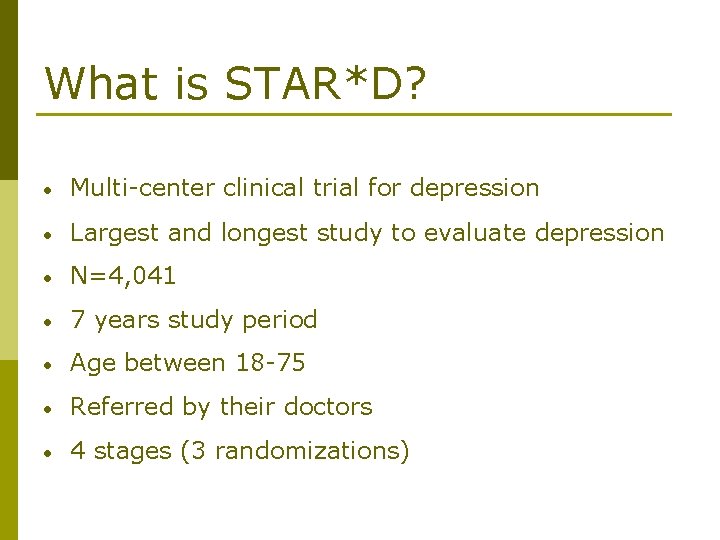
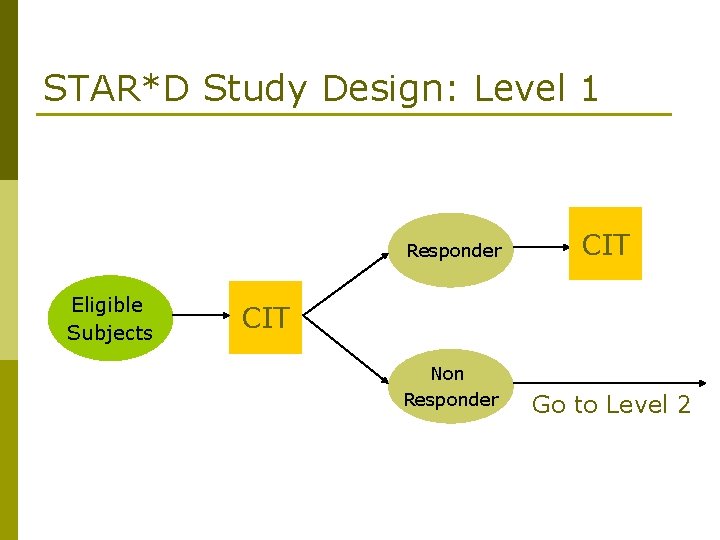
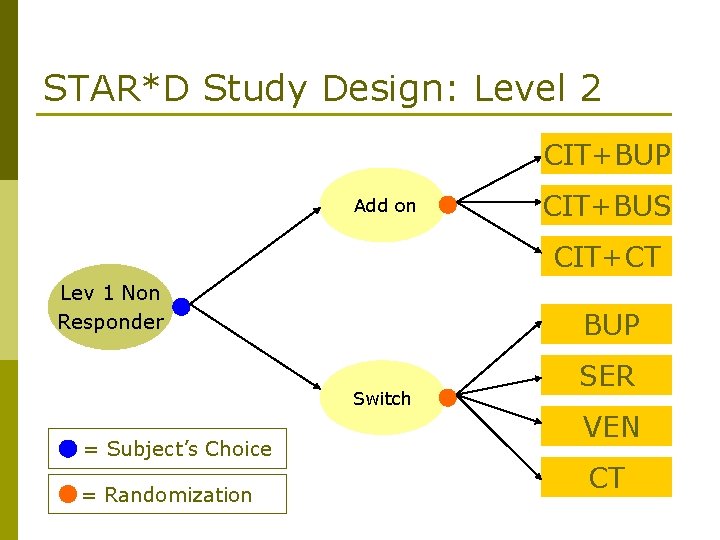
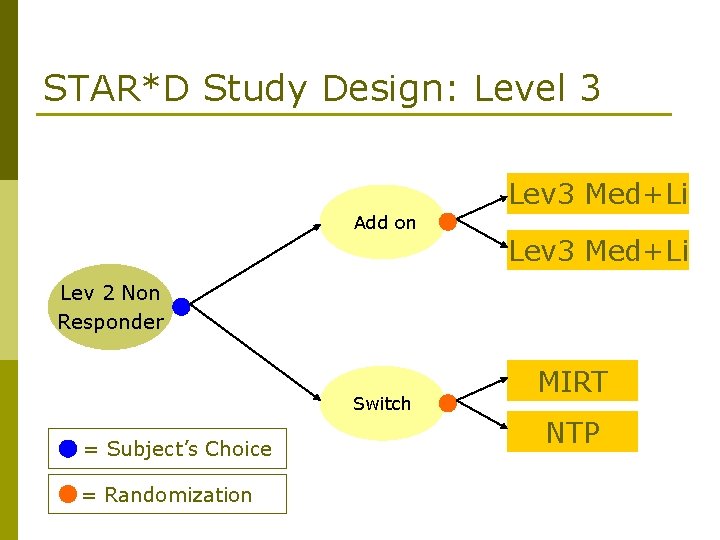
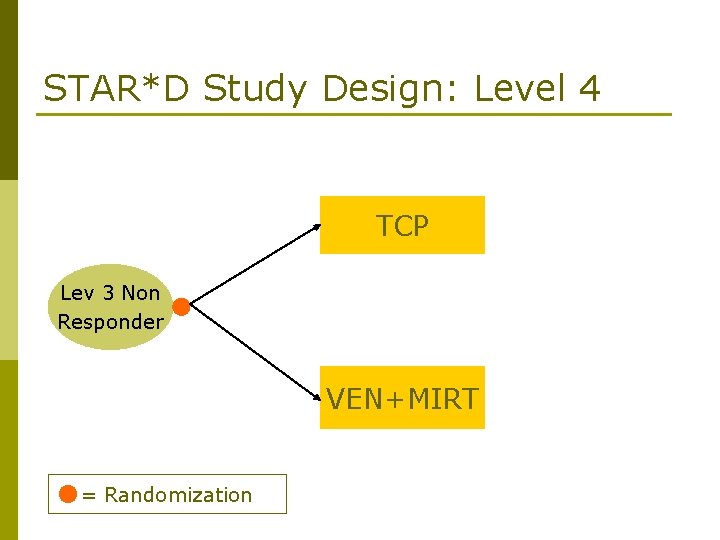
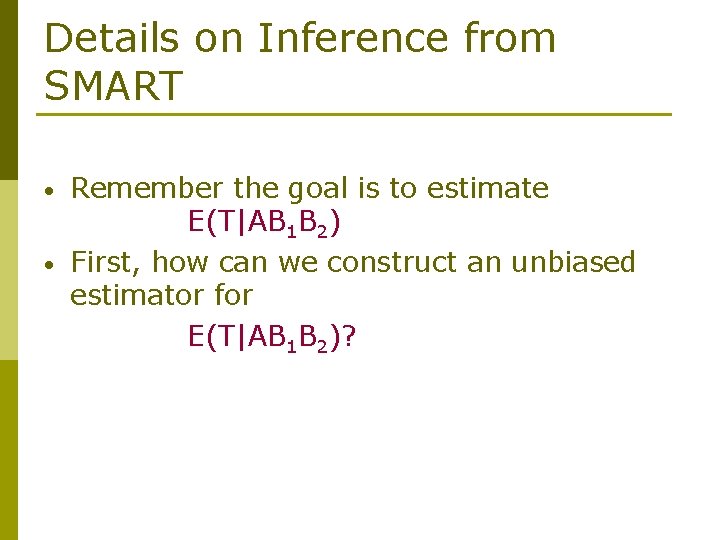
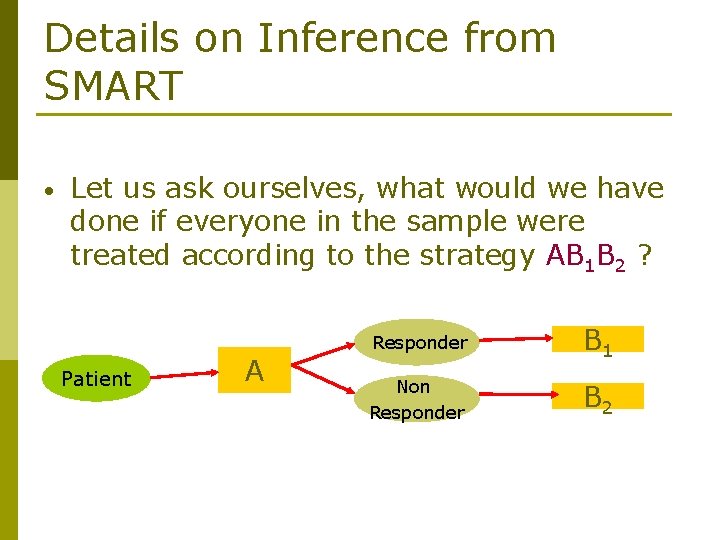
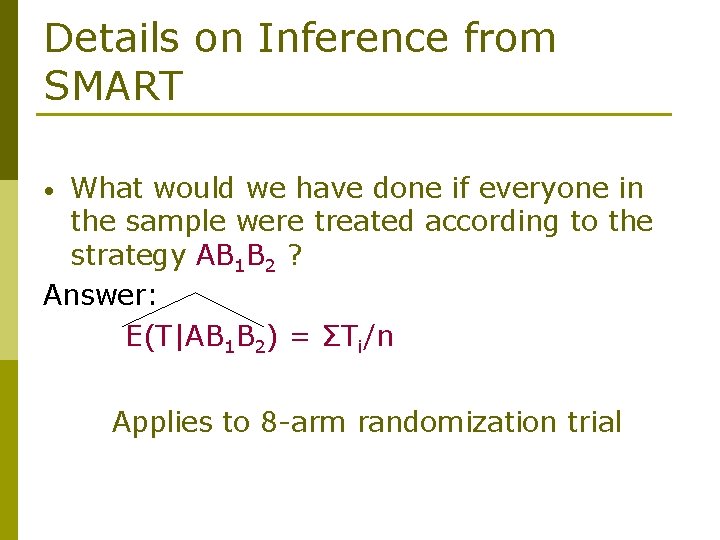
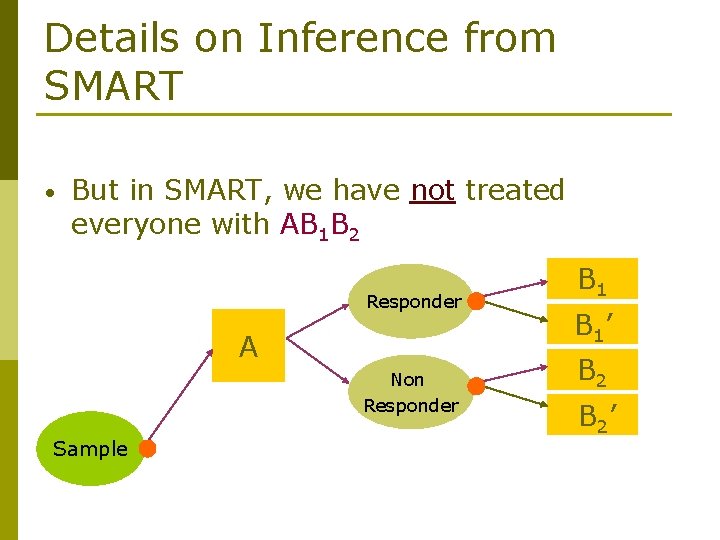
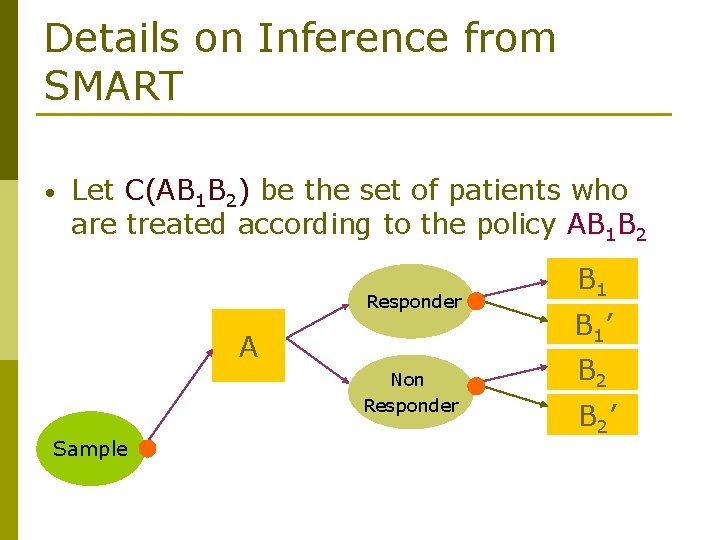
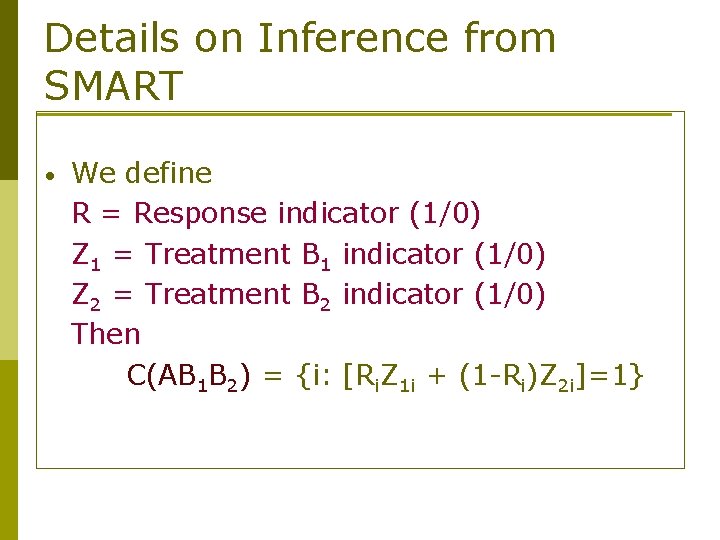
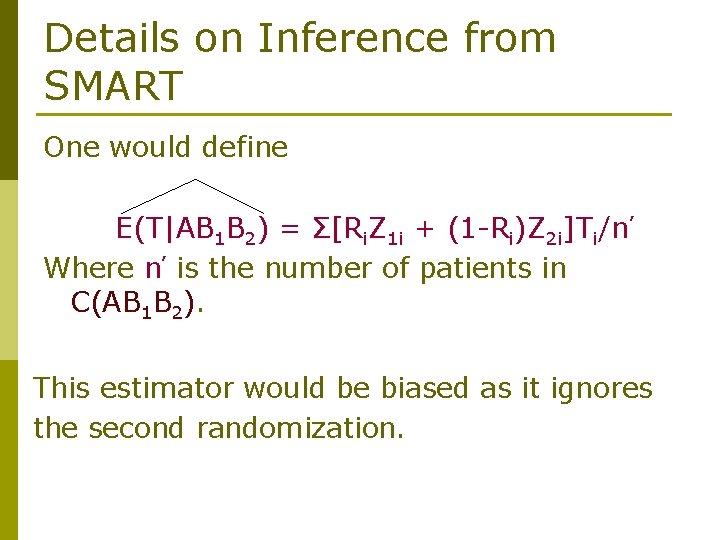
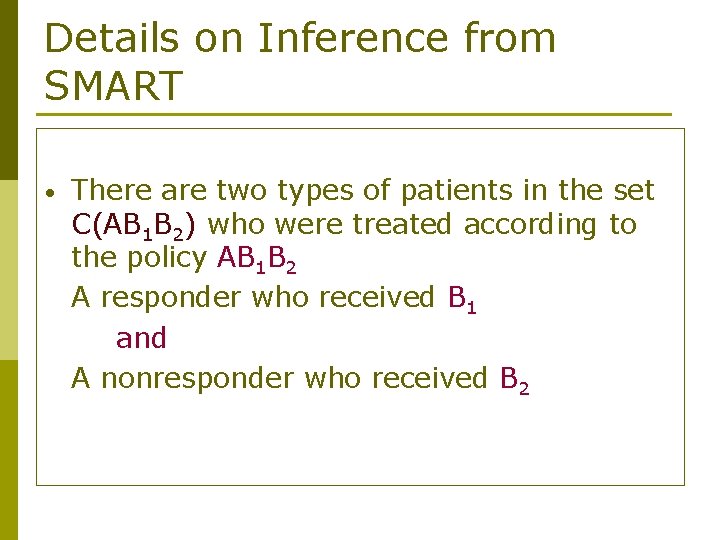
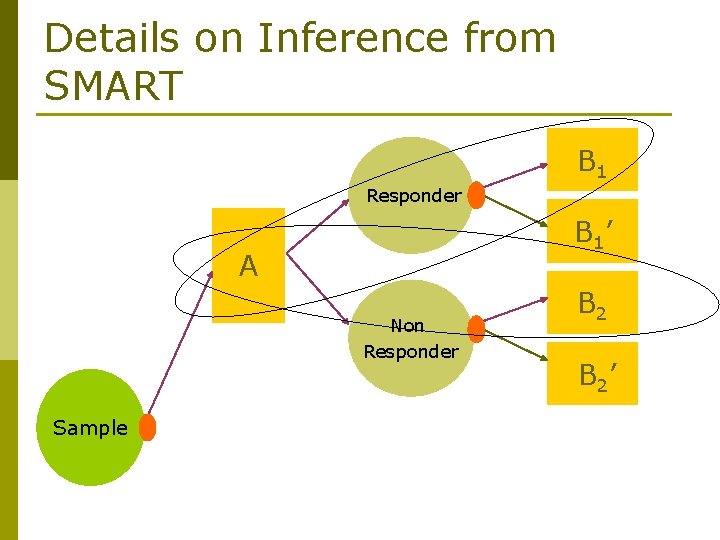
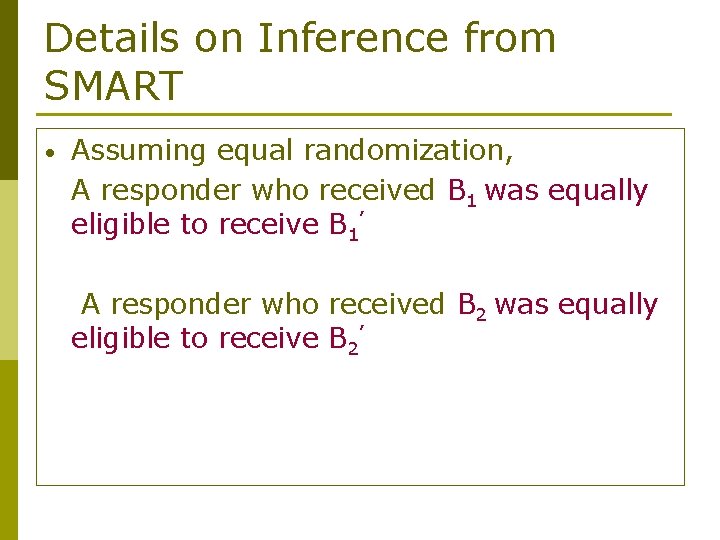
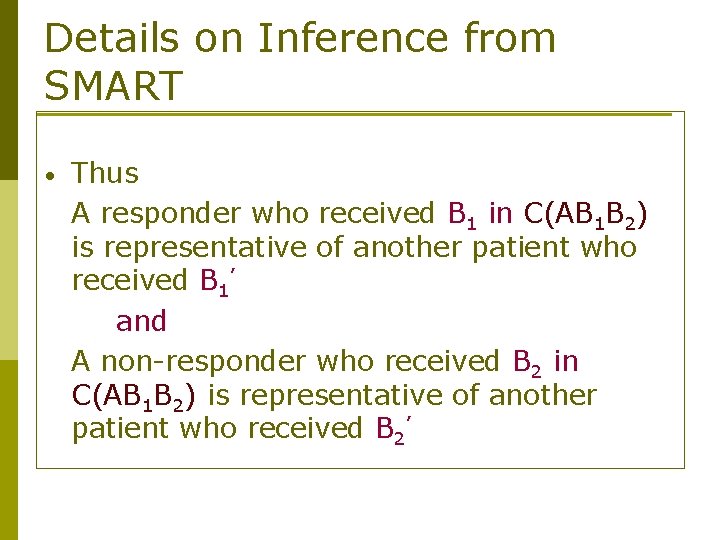
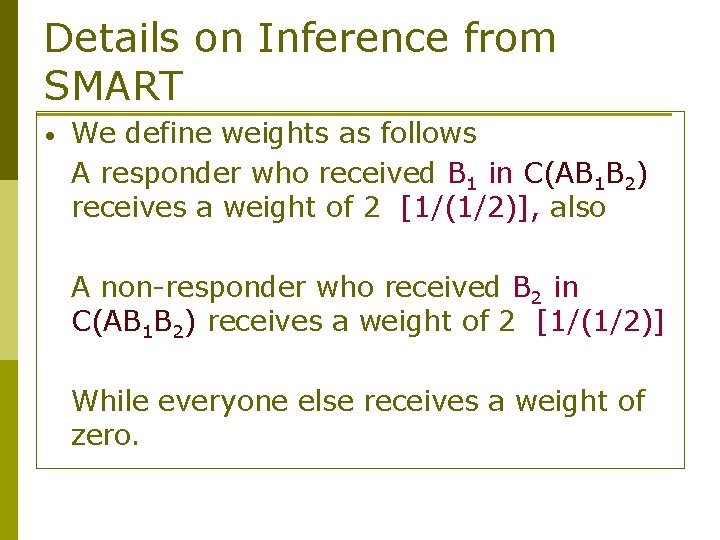
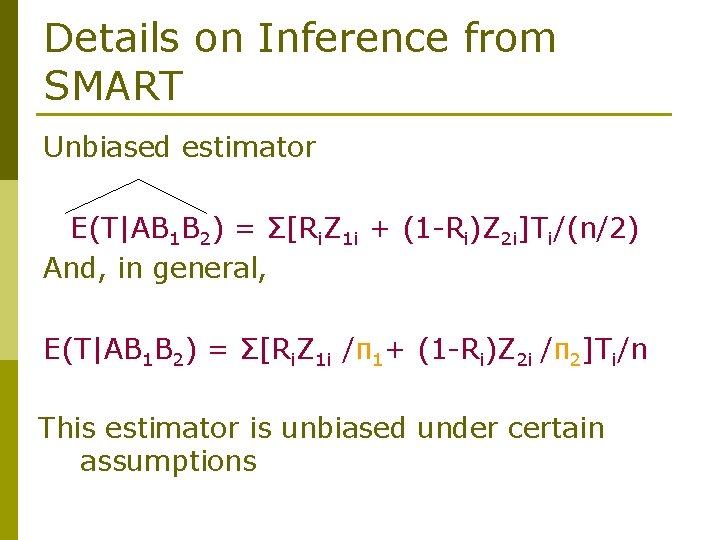
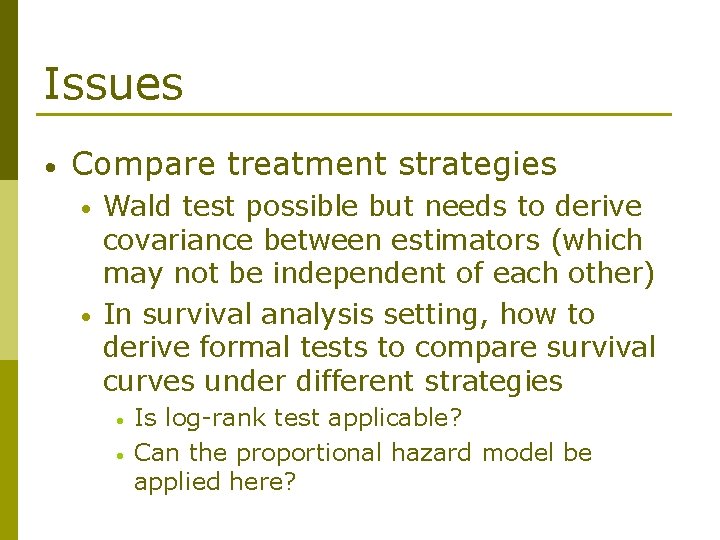
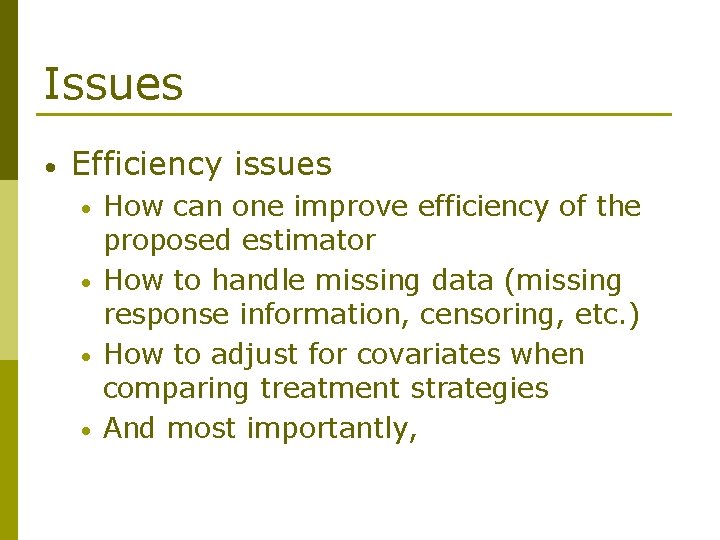
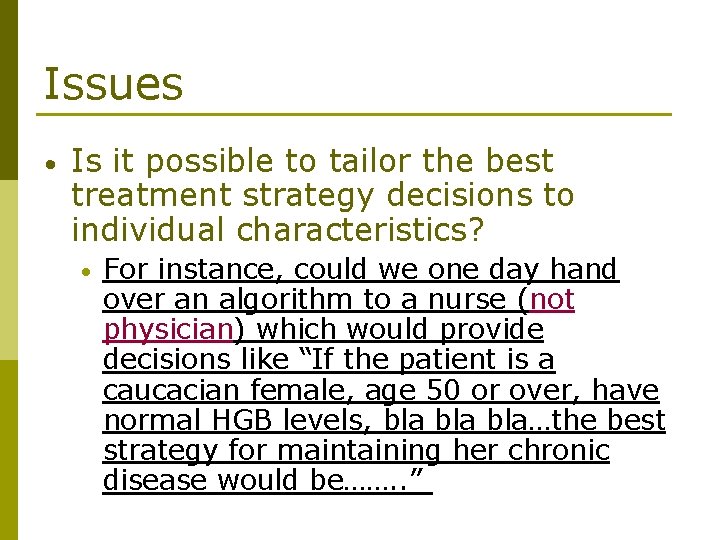
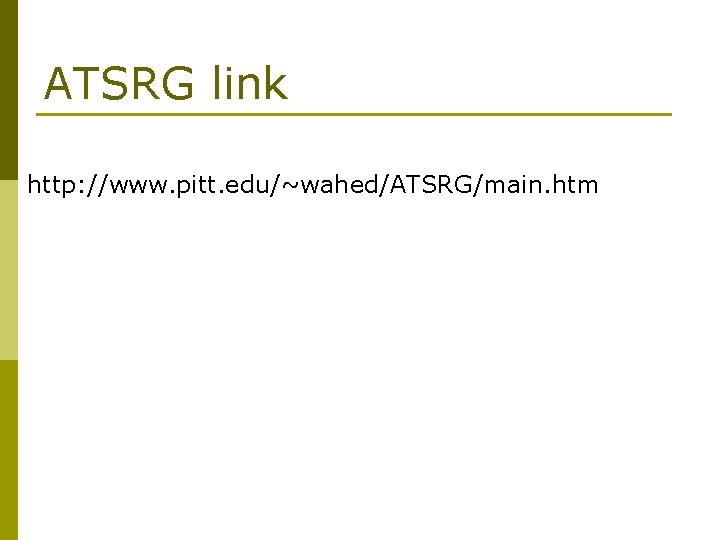
- Slides: 40
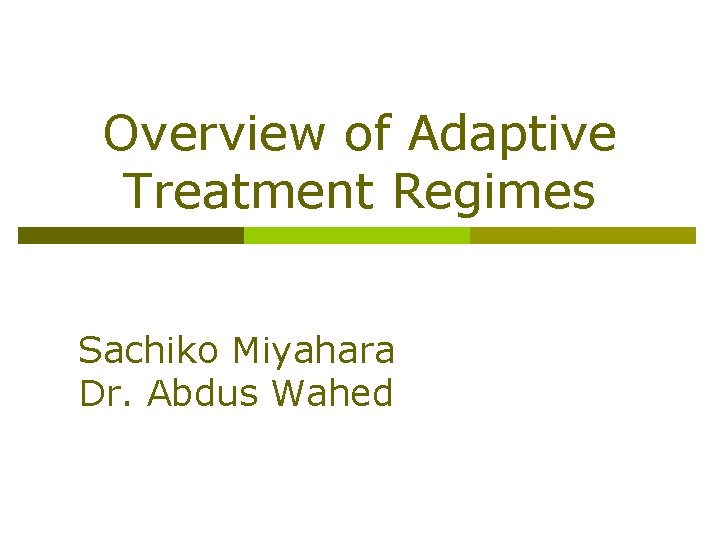
Overview of Adaptive Treatment Regimes Sachiko Miyahara Dr. Abdus Wahed
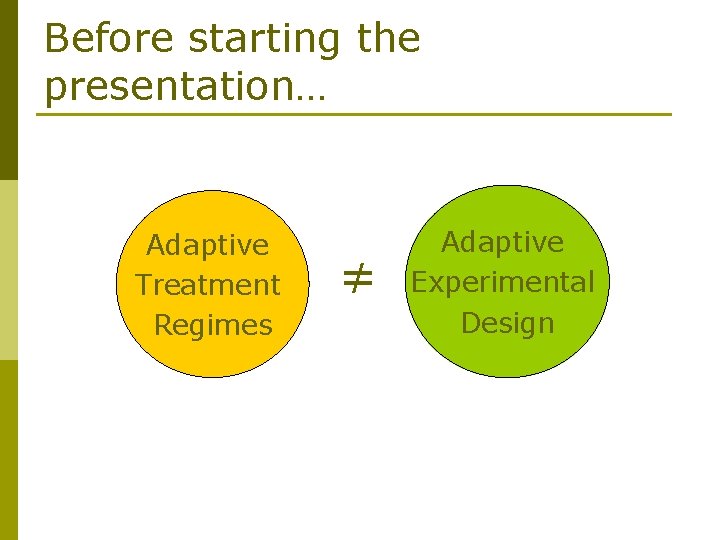
Before starting the presentation… Adaptive Treatment Regimes ≠ Adaptive Experimental Design
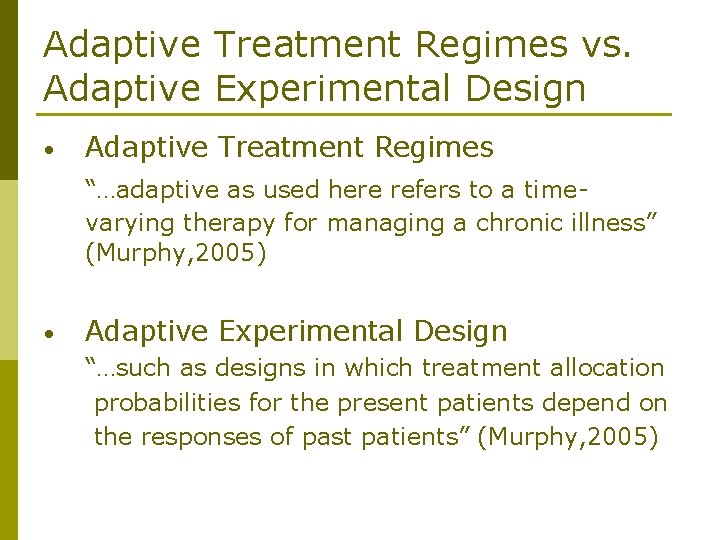
Adaptive Treatment Regimes vs. Adaptive Experimental Design • Adaptive Treatment Regimes “…adaptive as used here refers to a timevarying therapy for managing a chronic illness” (Murphy, 2005) • Adaptive Experimental Design “…such as designs in which treatment allocation probabilities for the present patients depend on the responses of past patients” (Murphy, 2005)
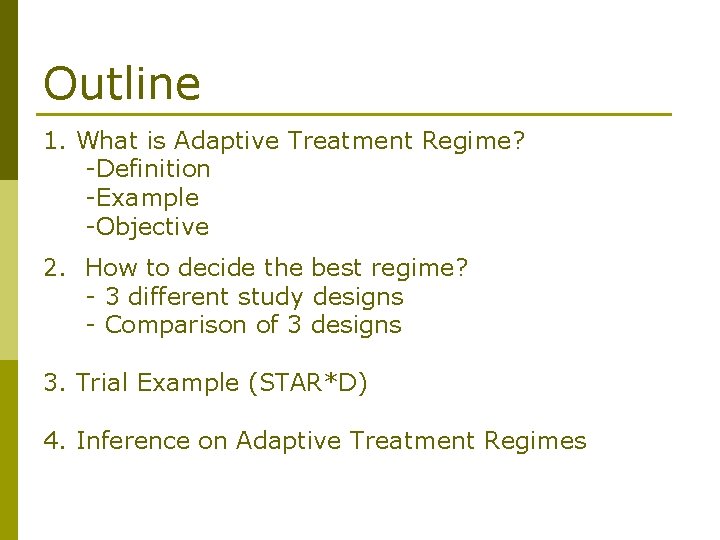
Outline 1. What is Adaptive Treatment Regime? -Definition -Example -Objective 2. How to decide the best regime? - 3 different study designs - Comparison of 3 designs 3. Trial Example (STAR*D) 4. Inference on Adaptive Treatment Regimes
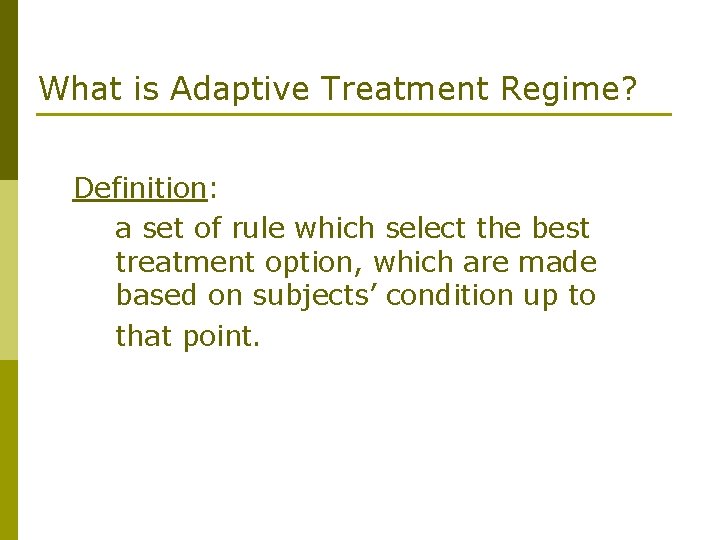
What is Adaptive Treatment Regime? Definition: a set of rule which select the best treatment option, which are made based on subjects’ condition up to that point.
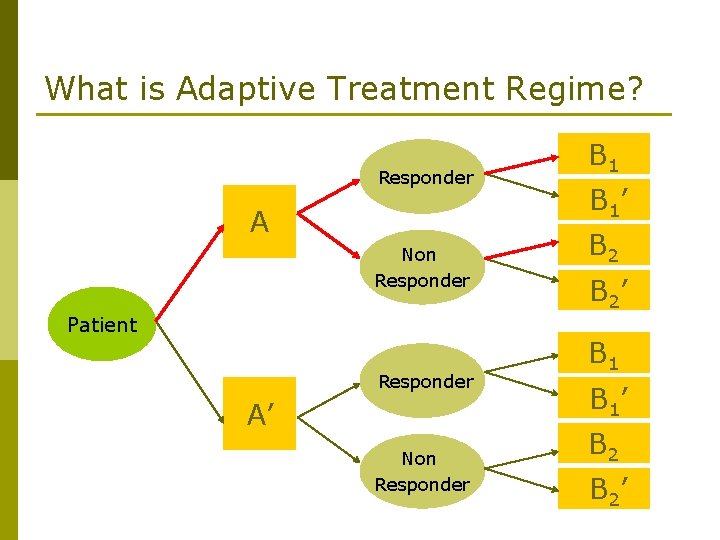
What is Adaptive Treatment Regime? Responder A Non Responder Patient Responder A’ Non Responder B 1 B 1’ B 2 B 2’
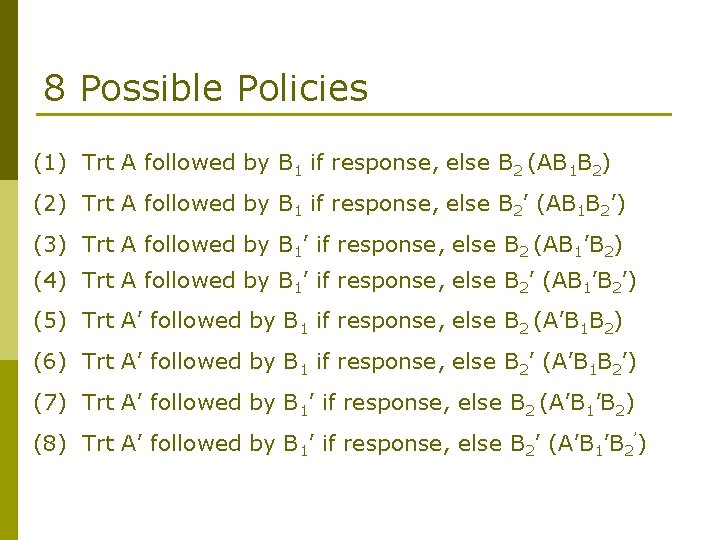
8 Possible Policies (1) Trt A followed by B 1 if response, else B 2 (AB 1 B 2) (2) Trt A followed by B 1 if response, else B 2’ (AB 1 B 2’) (3) Trt A followed by B 1’ if response, else B 2 (AB 1’B 2) (4) Trt A followed by B 1’ if response, else B 2’ (AB 1’B 2’) (5) Trt A’ followed by B 1 if response, else B 2 (A’B 1 B 2) (6) Trt A’ followed by B 1 if response, else B 2’ (A’B 1 B 2’) (7) Trt A’ followed by B 1’ if response, else B 2 (A’B 1’B 2) (8) Trt A’ followed by B 1’ if response, else B 2’ (A’B 1’B 2’)
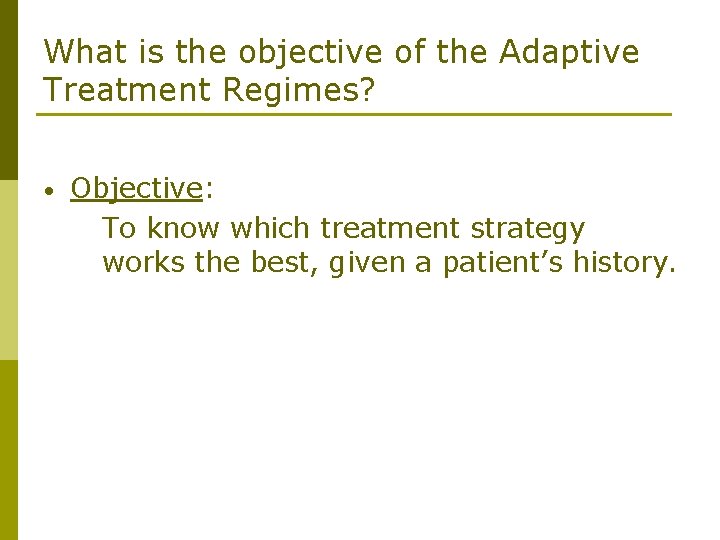
What is the objective of the Adaptive Treatment Regimes? • Objective: To know which treatment strategy works the best, given a patient’s history.
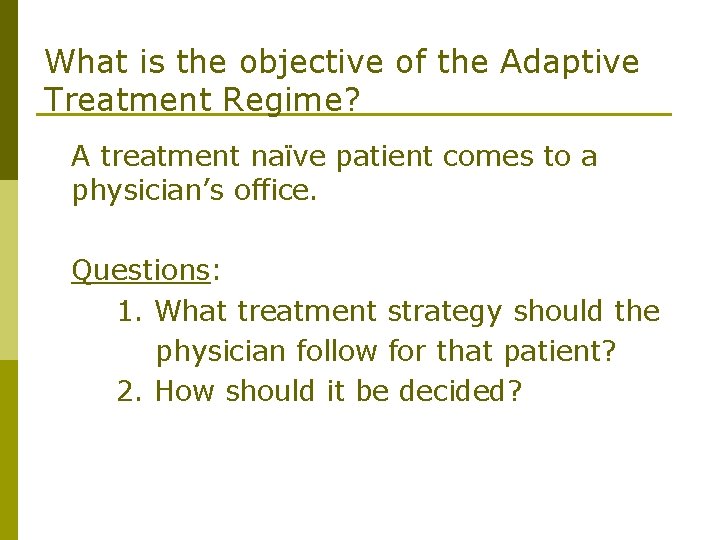
What is the objective of the Adaptive Treatment Regime? A treatment naïve patient comes to a physician’s office. Questions: 1. What treatment strategy should the physician follow for that patient? 2. How should it be decided?
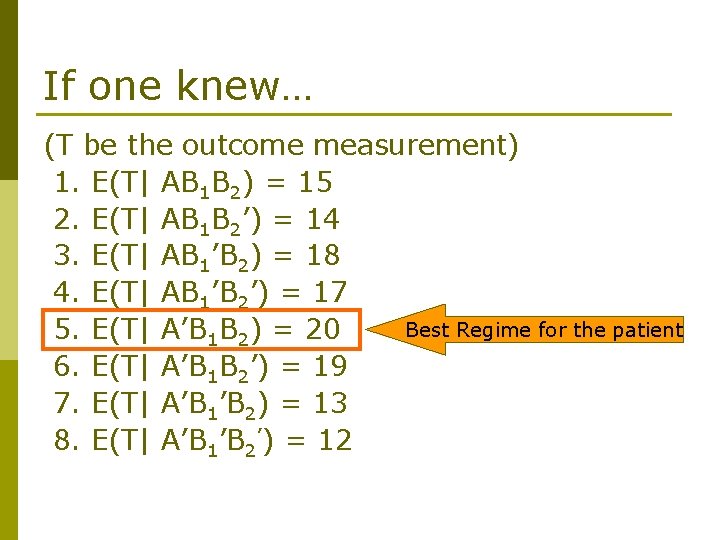
If one knew… (T be the outcome measurement) 1. E(T| AB 1 B 2) = 15 2. E(T| AB 1 B 2’) = 14 3. E(T| AB 1’B 2) = 18 4. E(T| AB 1’B 2’) = 17 Best Regime for the patient 5. E(T| A’B 1 B 2) = 20 6. E(T| A’B 1 B 2’) = 19 7. E(T| A’B 1’B 2) = 13 8. E(T| A’B 1’B 2’) = 12
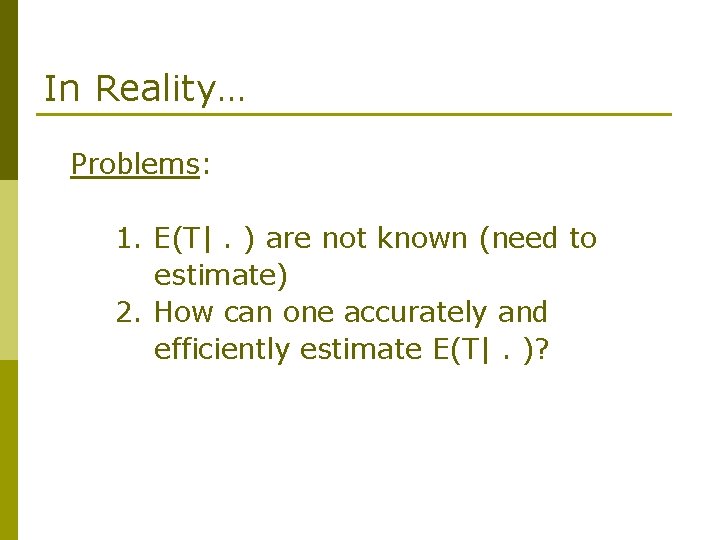
In Reality… Problems: 1. E(T|. ) are not known (need to estimate) 2. How can one accurately and efficiently estimate E(T|. )?
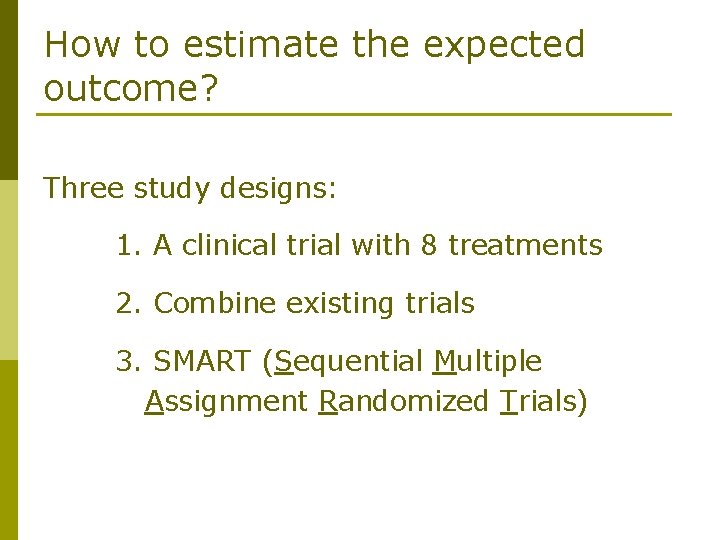
How to estimate the expected outcome? Three study designs: 1. A clinical trial with 8 treatments 2. Combine existing trials 3. SMART (Sequential Multiple Assignment Randomized Trials)
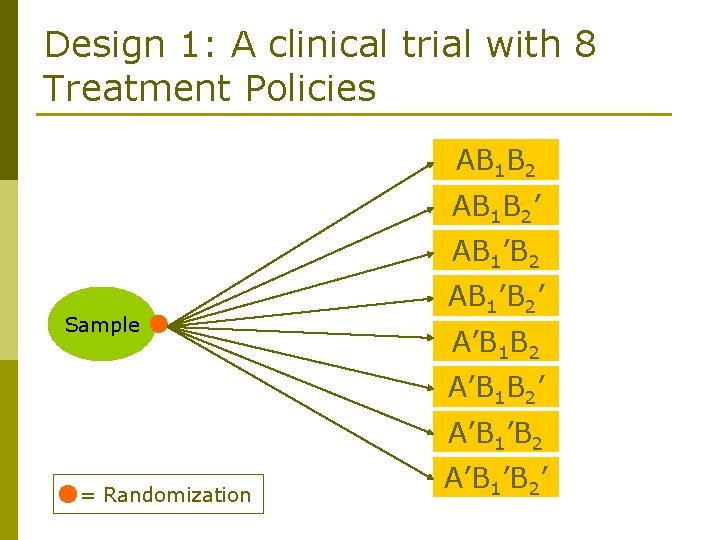
Design 1: A clinical trial with 8 Treatment Policies AB 1 B 2’ AB 1’B 2 Sample AB 1’B 2’ A’B 1 B 2’ A’B 1’B 2 = Randomization A’B 1’B 2’
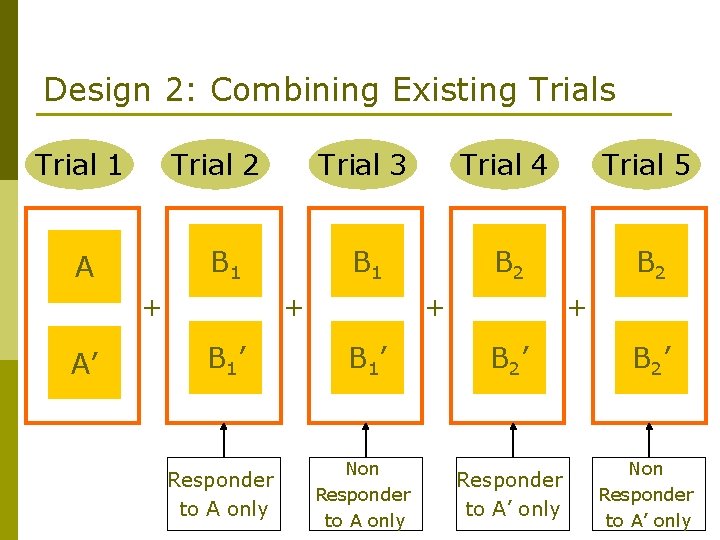
Design 2: Combining Existing Trials Trial 1 Trial 2 B 1 A + A’ Trial 3 Trial 4 Trial 5 B 1 B 2 + B 1’ Responder to A only + + B 1’ B 2’ Non Responder to A only Responder to A’ only Non Responder to A’ only
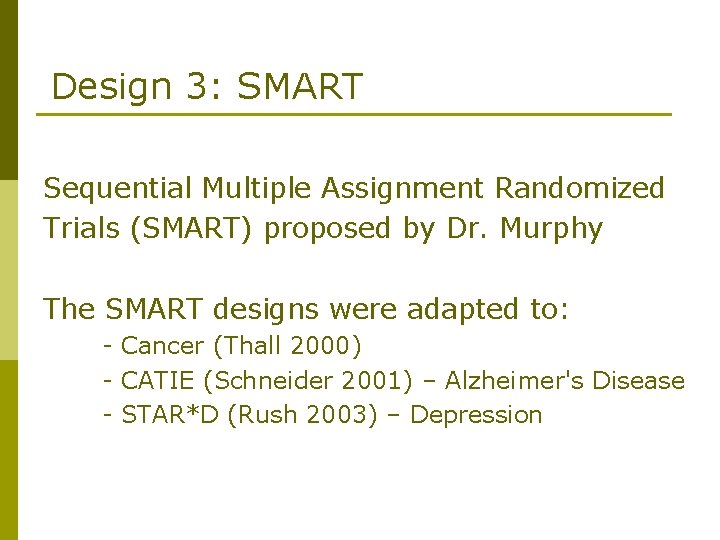
Design 3: SMART Sequential Multiple Assignment Randomized Trials (SMART) proposed by Dr. Murphy The SMART designs were adapted to: - Cancer (Thall 2000) - CATIE (Schneider 2001) – Alzheimer's Disease - STAR*D (Rush 2003) – Depression
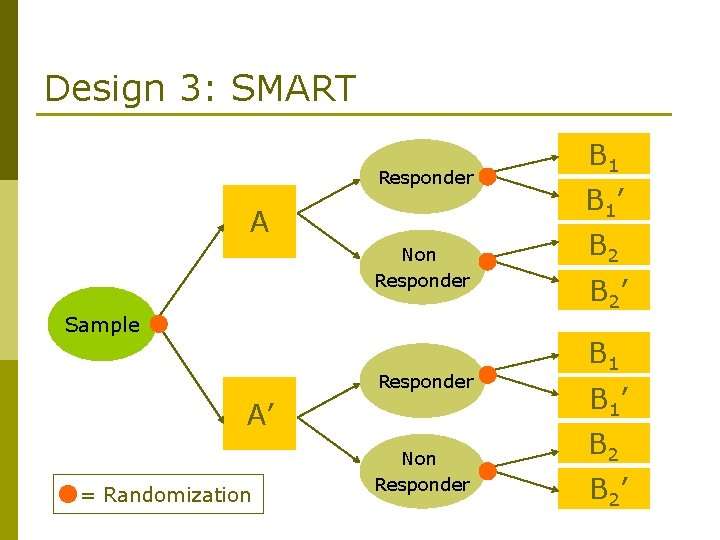
Design 3: SMART Responder A Non Responder Sample Responder A’ = Randomization Non Responder B 1 B 1’ B 2 B 2’
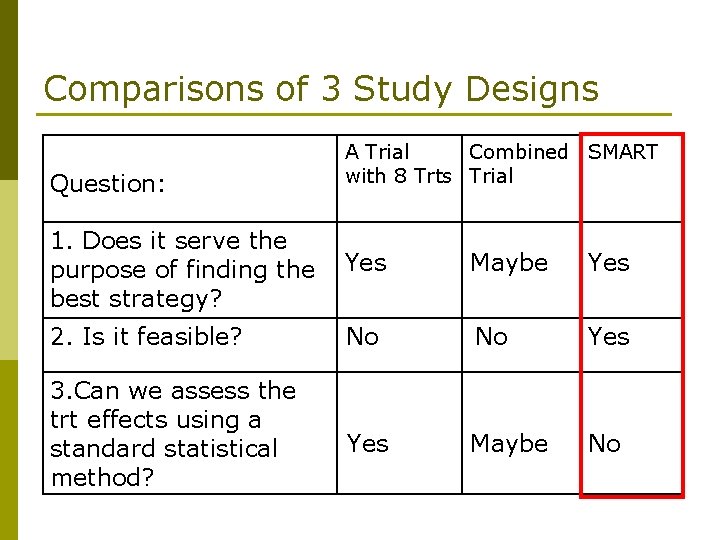
Comparisons of 3 Study Designs Question: A Trial Combined SMART with 8 Trts Trial 1. Does it serve the purpose of finding the best strategy? Yes Maybe Yes 2. Is it feasible? No No Yes 3. Can we assess the trt effects using a standard statistical method? Yes Maybe No
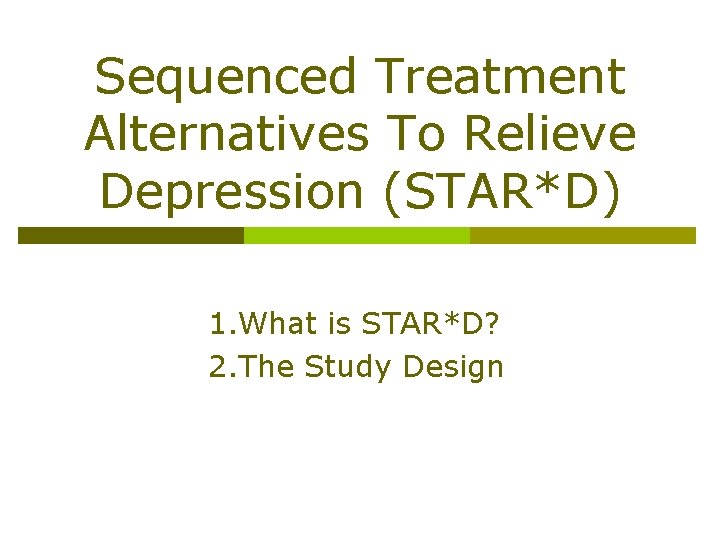
Sequenced Treatment Alternatives To Relieve Depression (STAR*D) 1. What is STAR*D? 2. The Study Design
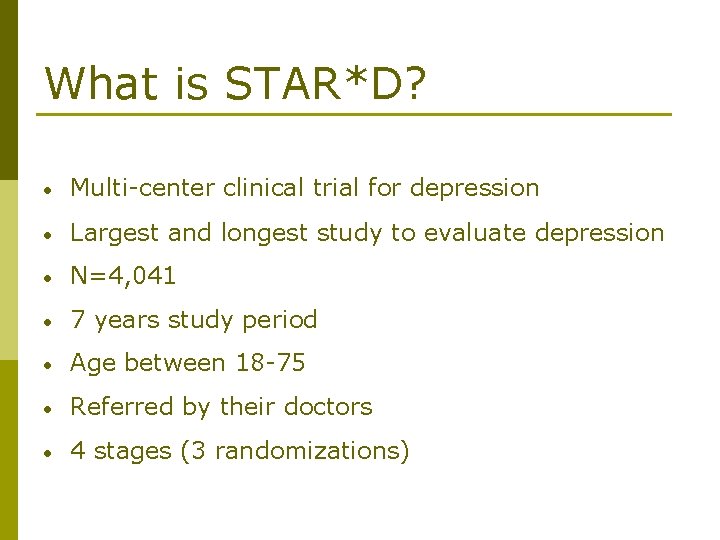
What is STAR*D? • Multi-center clinical trial for depression • Largest and longest study to evaluate depression • N=4, 041 • 7 years study period • Age between 18 -75 • Referred by their doctors • 4 stages (3 randomizations)
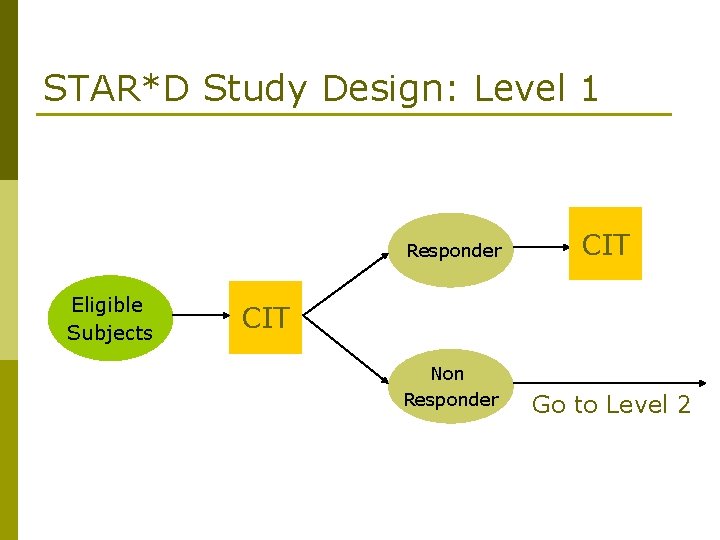
STAR*D Study Design: Level 1 Responder Eligible Subjects CIT Non Responder Go to Level 2
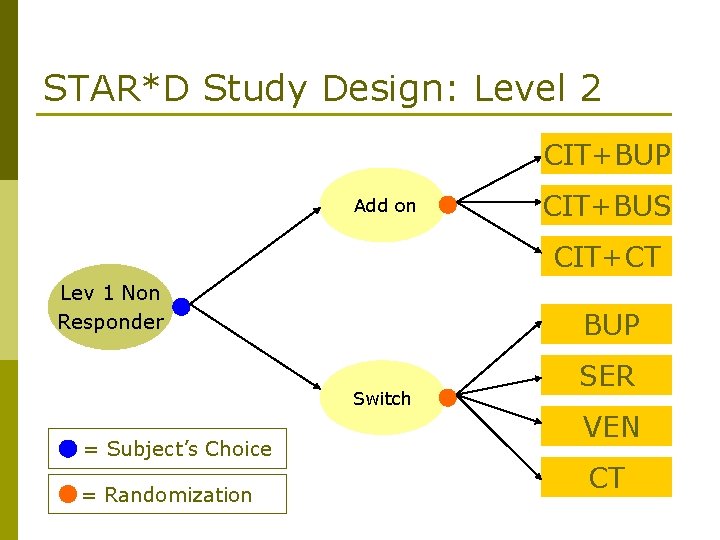
STAR*D Study Design: Level 2 CIT+BUP Add on CIT+BUS CIT+CT Lev 1 Non Responder BUP Switch = Subject’s Choice = Randomization SER VEN CT
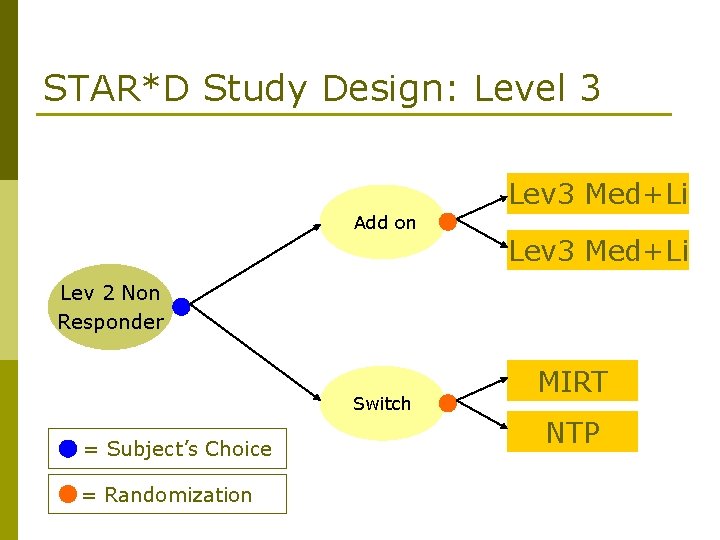
STAR*D Study Design: Level 3 Lev 3 Med+Li Add on Lev 3 Med+Li Lev 2 Non Responder Switch = Subject’s Choice = Randomization MIRT NTP
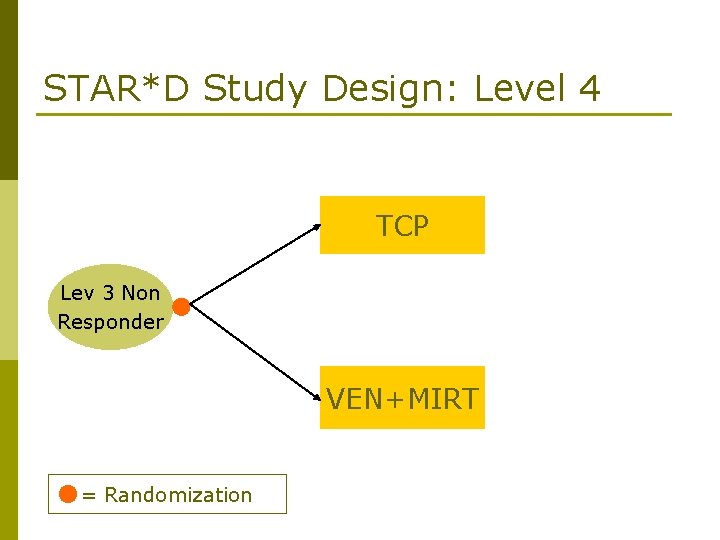
STAR*D Study Design: Level 4 TCP Lev 3 Non Responder VEN+MIRT = Randomization
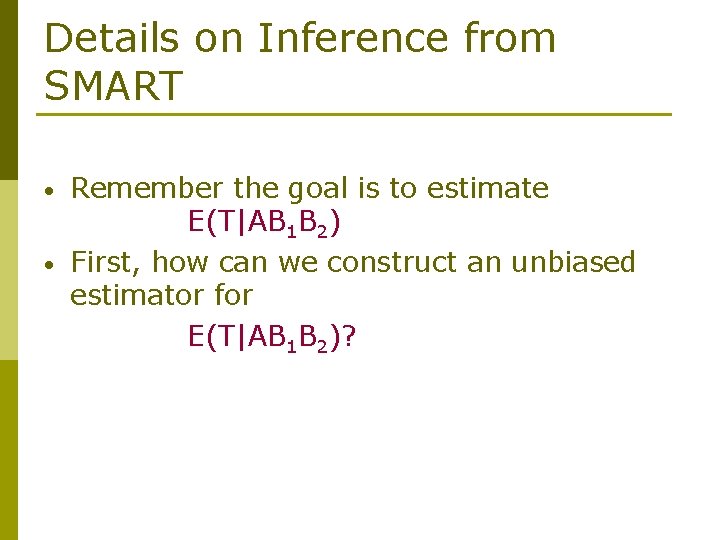
Details on Inference from SMART • • Remember the goal is to estimate E(T|AB 1 B 2) First, how can we construct an unbiased estimator for E(T|AB 1 B 2)?
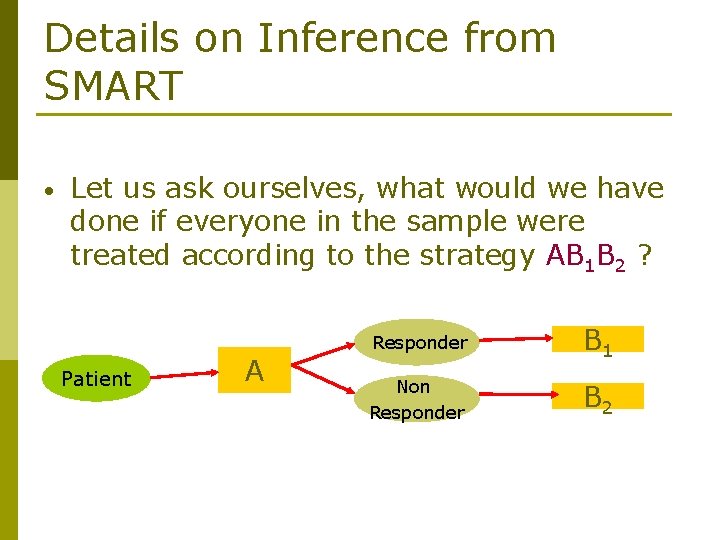
Details on Inference from SMART • Let us ask ourselves, what would we have done if everyone in the sample were treated according to the strategy AB 1 B 2 ? Patient A Responder B 1 Non Responder B 2
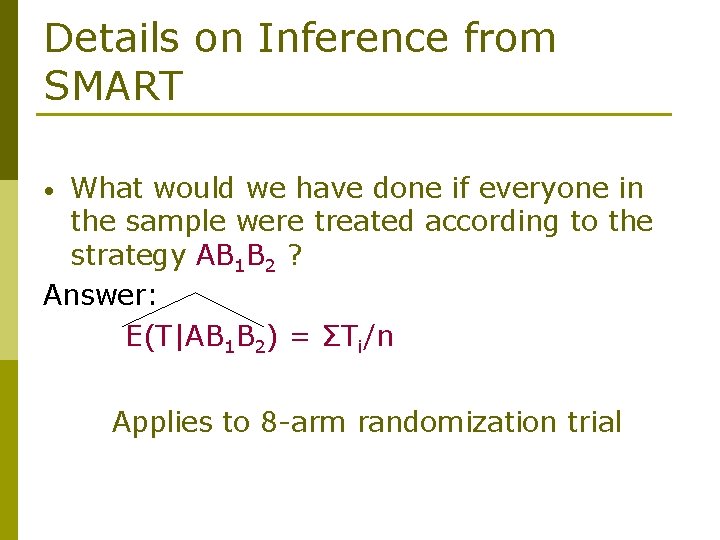
Details on Inference from SMART What would we have done if everyone in the sample were treated according to the strategy AB 1 B 2 ? Answer: E(T|AB 1 B 2) = ΣTi/n • Applies to 8 -arm randomization trial
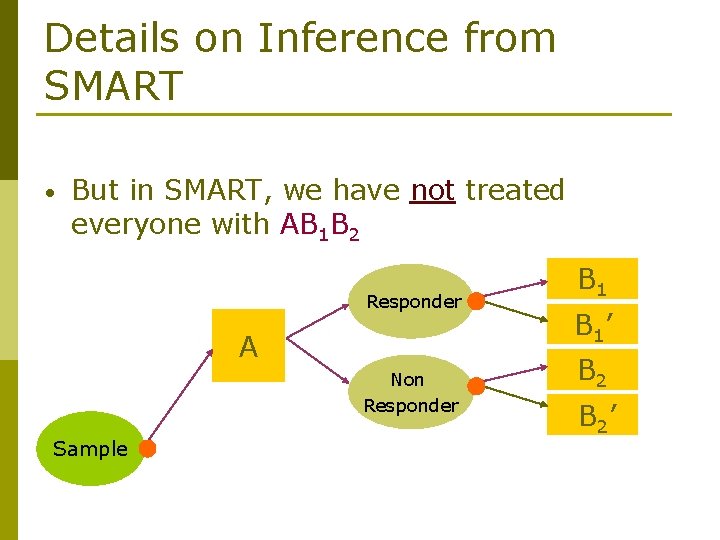
Details on Inference from SMART • But in SMART, we have not treated everyone with AB 1 B 2 Responder A Non Responder Sample B 1’ B 2’
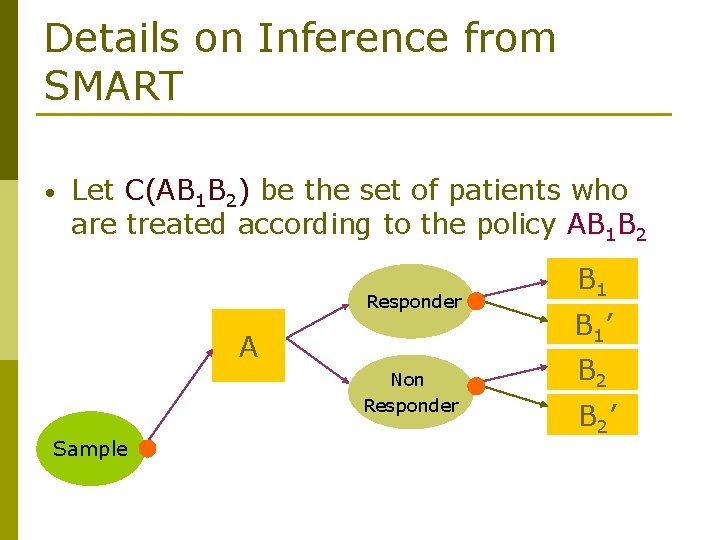
Details on Inference from SMART • Let C(AB 1 B 2) be the set of patients who are treated according to the policy AB 1 B 2 Responder A Non Responder Sample B 1’ B 2’
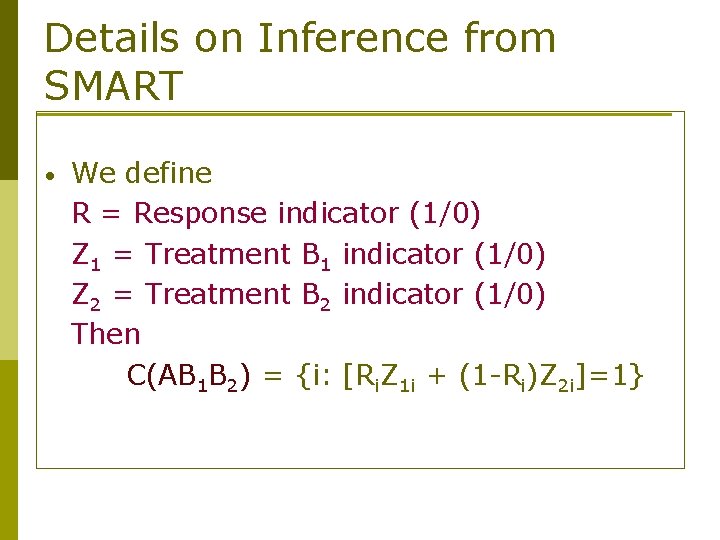
Details on Inference from SMART • We define R = Response indicator (1/0) Z 1 = Treatment B 1 indicator (1/0) Z 2 = Treatment B 2 indicator (1/0) Then C(AB 1 B 2) = {i: [Ri. Z 1 i + (1 -Ri)Z 2 i]=1}
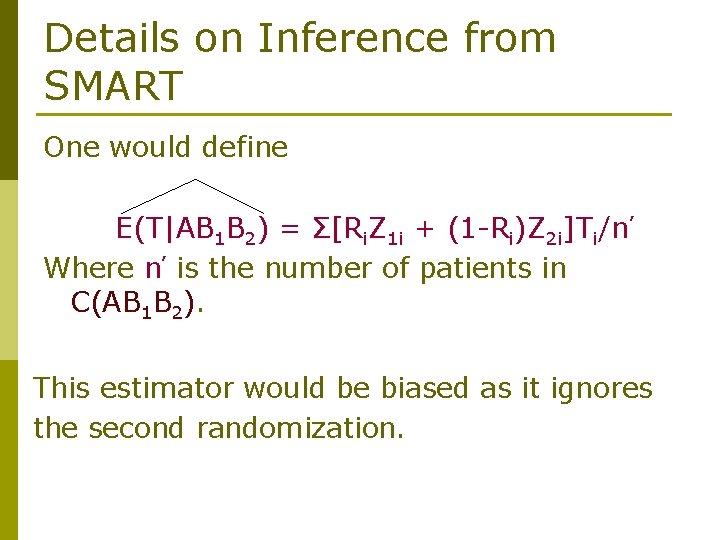
Details on Inference from SMART One would define E(T|AB 1 B 2) = Σ[Ri. Z 1 i + (1 -Ri)Z 2 i]Ti/n’ Where n’ is the number of patients in C(AB 1 B 2). This estimator would be biased as it ignores the second randomization.
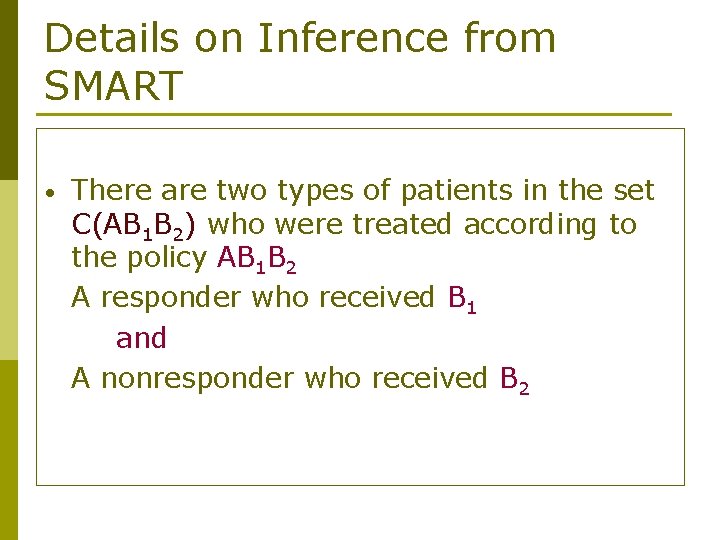
Details on Inference from SMART • There are two types of patients in the set C(AB 1 B 2) who were treated according to the policy AB 1 B 2 A responder who received B 1 and A nonresponder who received B 2
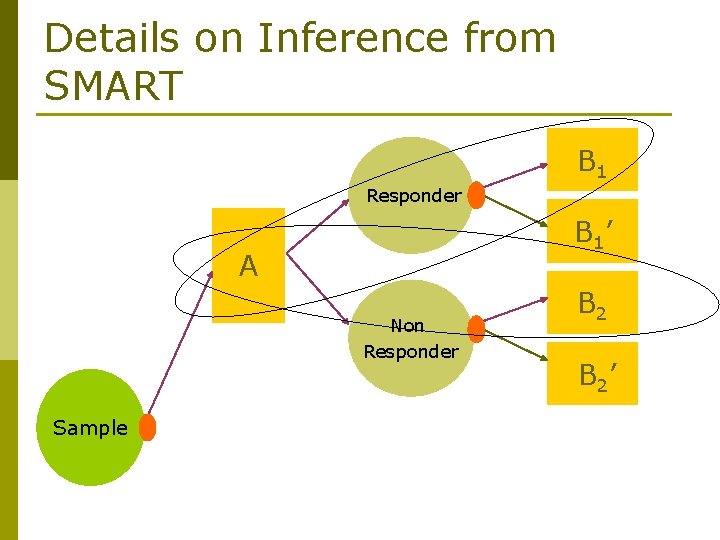
Details on Inference from SMART B 1 Responder B 1’ A Non Responder Sample B 2’
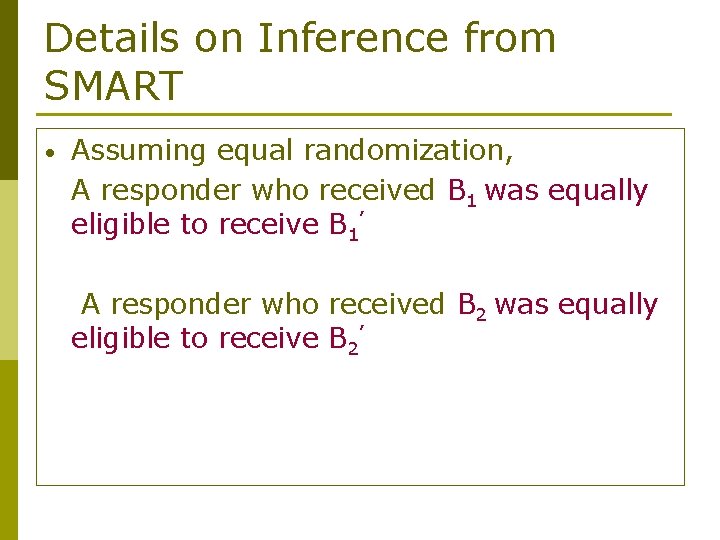
Details on Inference from SMART • Assuming equal randomization, A responder who received B 1 was equally eligible to receive B 1’ A responder who received B 2 was equally eligible to receive B 2’
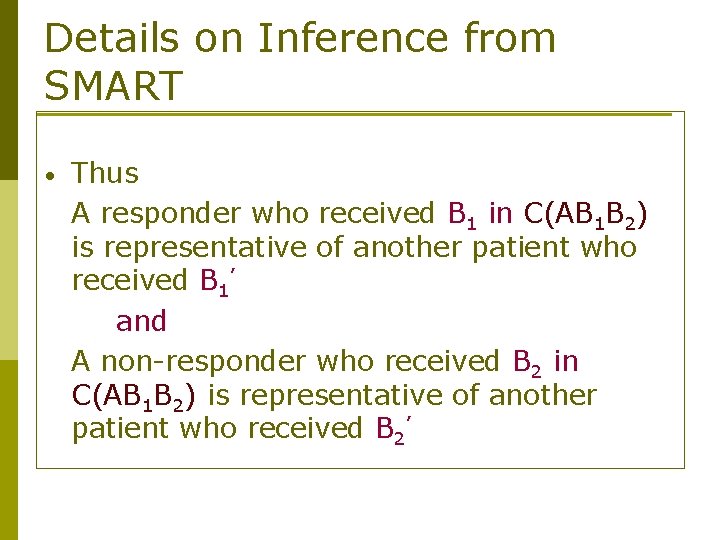
Details on Inference from SMART • Thus A responder who received B 1 in C(AB 1 B 2) is representative of another patient who received B 1’ and A non-responder who received B 2 in C(AB 1 B 2) is representative of another patient who received B 2’
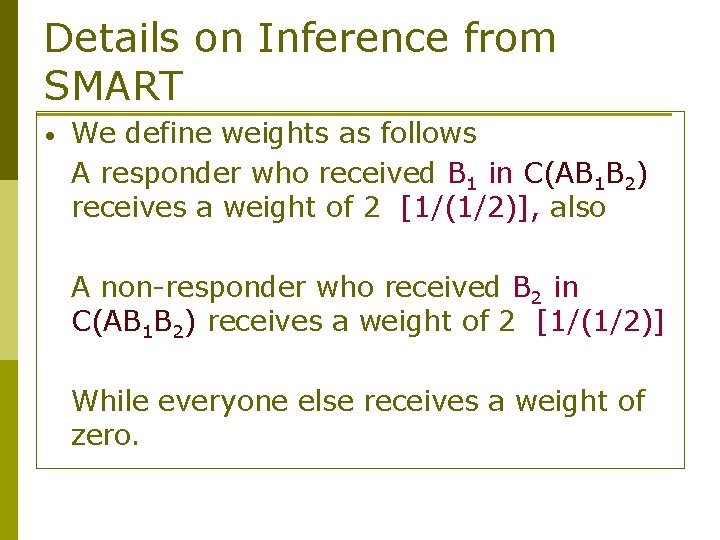
Details on Inference from SMART • We define weights as follows A responder who received B 1 in C(AB 1 B 2) receives a weight of 2 [1/(1/2)], also A non-responder who received B 2 in C(AB 1 B 2) receives a weight of 2 [1/(1/2)] While everyone else receives a weight of zero.
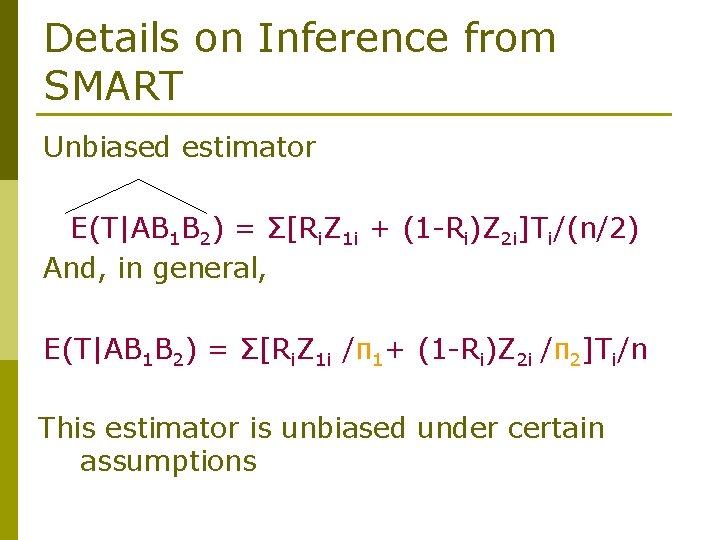
Details on Inference from SMART Unbiased estimator E(T|AB 1 B 2) = Σ[Ri. Z 1 i + (1 -Ri)Z 2 i]Ti/(n/2) And, in general, E(T|AB 1 B 2) = Σ[Ri. Z 1 i /π1+ (1 -Ri)Z 2 i /π2]Ti/n This estimator is unbiased under certain assumptions
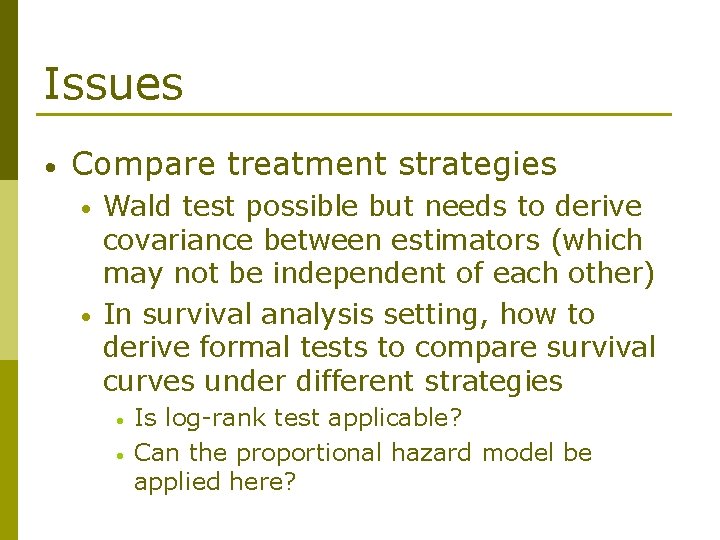
Issues • Compare treatment strategies • • Wald test possible but needs to derive covariance between estimators (which may not be independent of each other) In survival analysis setting, how to derive formal tests to compare survival curves under different strategies • • Is log-rank test applicable? Can the proportional hazard model be applied here?
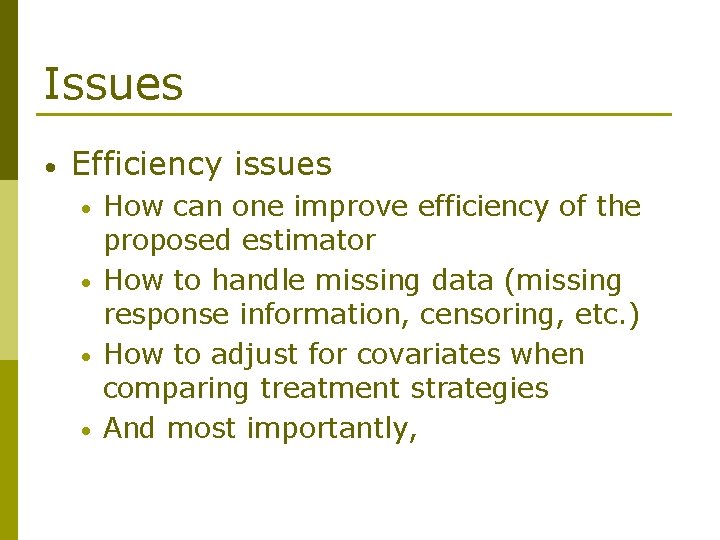
Issues • Efficiency issues • • How can one improve efficiency of the proposed estimator How to handle missing data (missing response information, censoring, etc. ) How to adjust for covariates when comparing treatment strategies And most importantly,
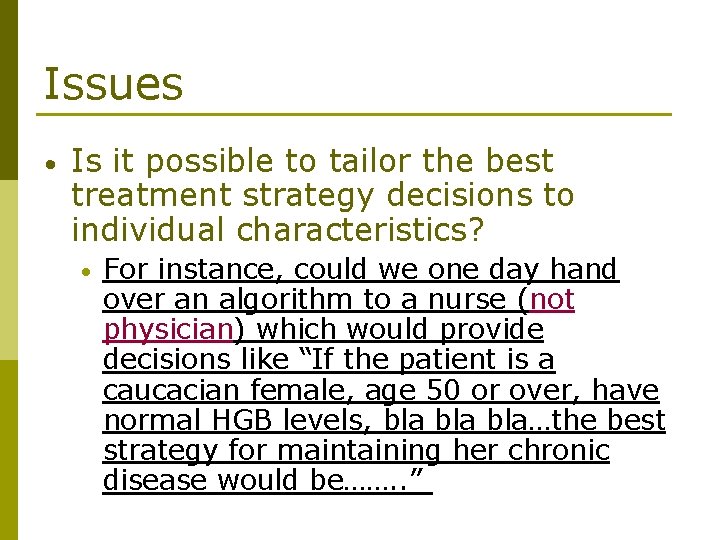
Issues • Is it possible to tailor the best treatment strategy decisions to individual characteristics? • For instance, could we one day hand over an algorithm to a nurse (not physician) which would provide decisions like “If the patient is a caucacian female, age 50 or over, have normal HGB levels, bla bla…the best strategy for maintaining her chronic disease would be……. . ”
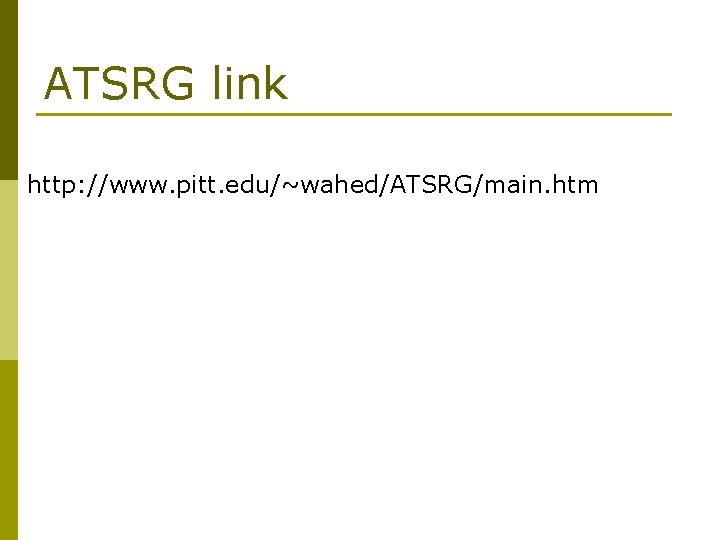
ATSRG link http: //www. pitt. edu/~wahed/ATSRG/main. htm
Régimes douaniers
The rise of dictatorial regimes
The seeds of totalitarian regimes are nurtured
The rise of dictatorial regimes lesson 2
Why were italian nationalists outraged after ww1
Data analysis
Sas customer intelligence
Inert organizational culture
Whats adaptive radiation
Preserving statistical validity in adaptive data analysis
Adaptive cruise control
Adaptive plasticity
Self catheterization adaptive equipment
Acm adaptive case management
Deseasonalized demand formula
Dolphins cheetah
5 adaptive features of camel
Adaptive maintenance in software engineering
Adaptive enrichment design
Adaptive defense system
Sitecore adaptive personalisation
Vestigial
H.264
Adaptive project life cycle
Adaptive leadership definition
Two level adaptive branch prediction
Adaptive and corrective maintenance
Adaptive
Mechanical isolatio
Types of software changes
Domain adaptive faster rcnn
Adaptive challenges examples
Linda r greene
Adaptive control
Fsa assessments org apm
Coévolution
Decreased intracranial adaptive capacity
Is adaptive radiation divergent evolution
Adaptive products
Adaptive learning neural network
Adaptive challenge example