Nested Example Using SPSS David A Kenny January
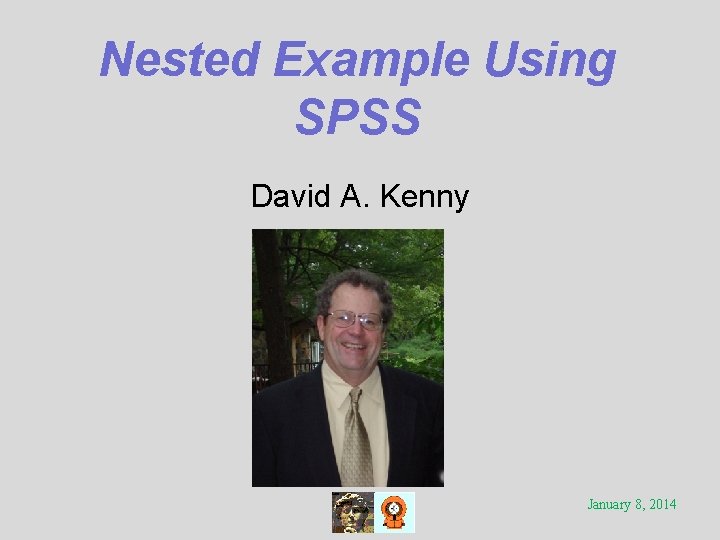
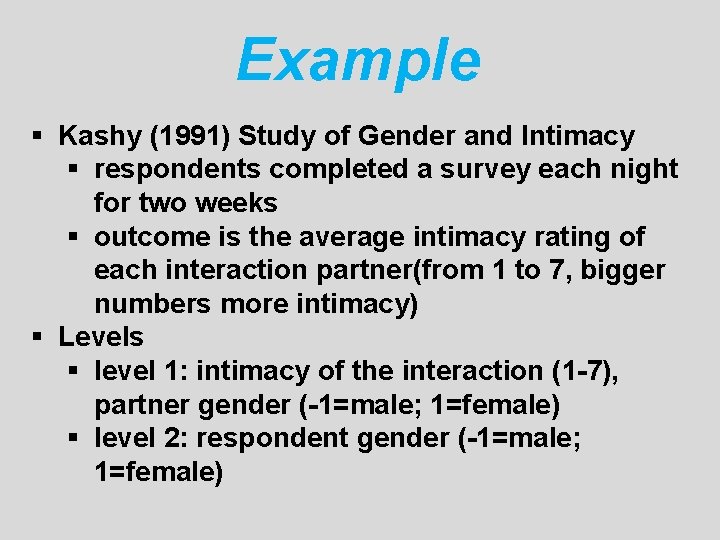
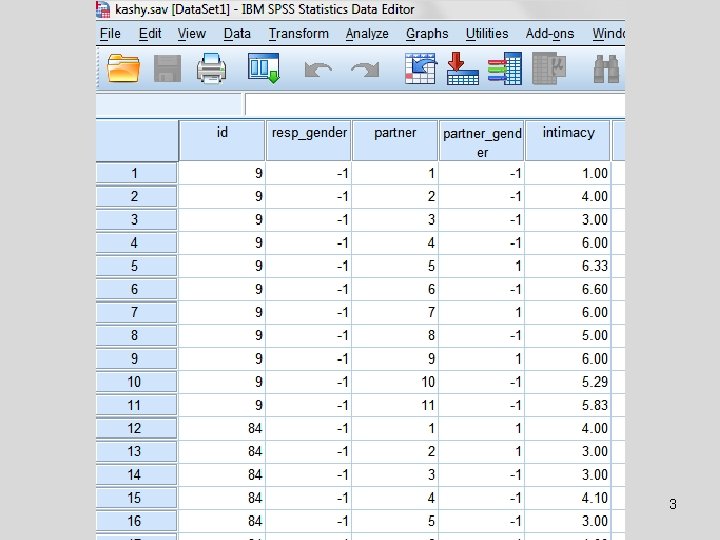
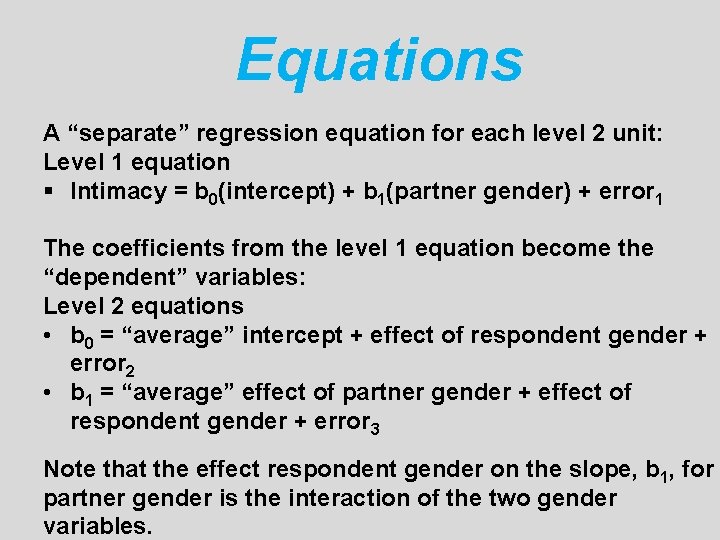
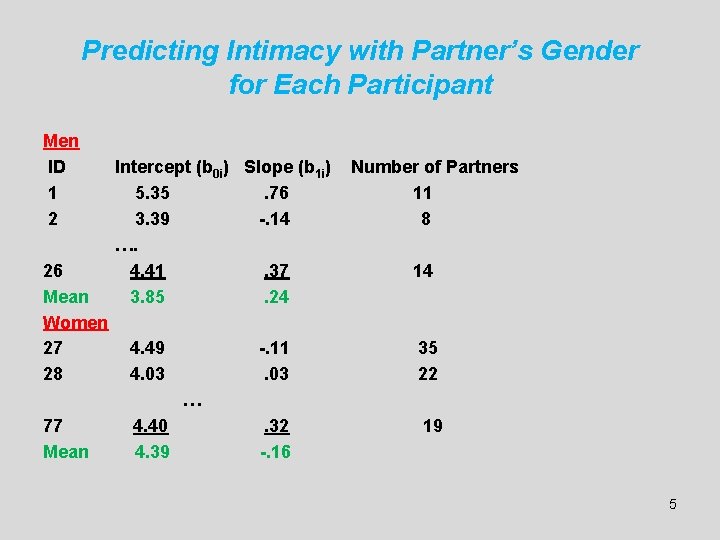
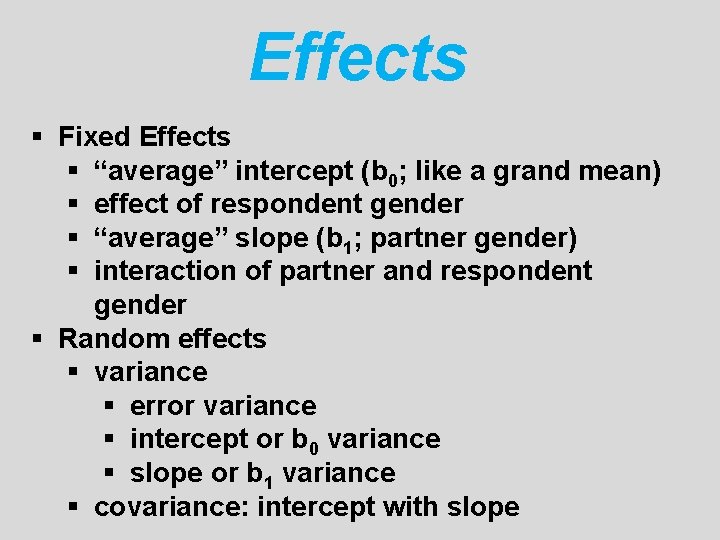
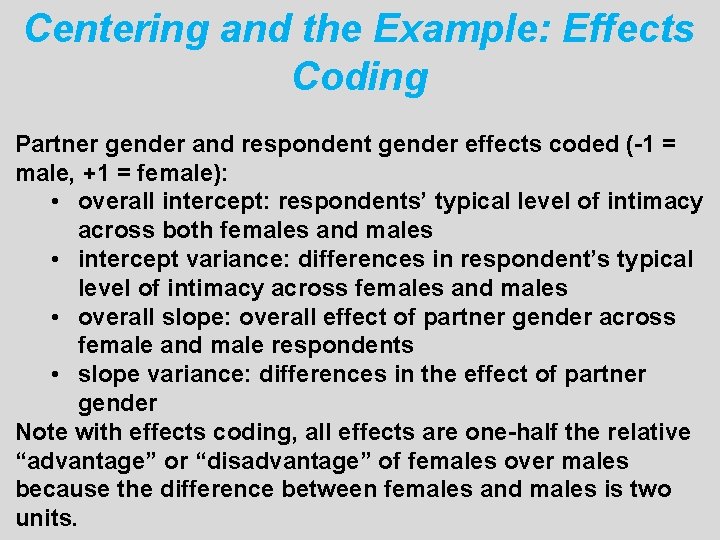
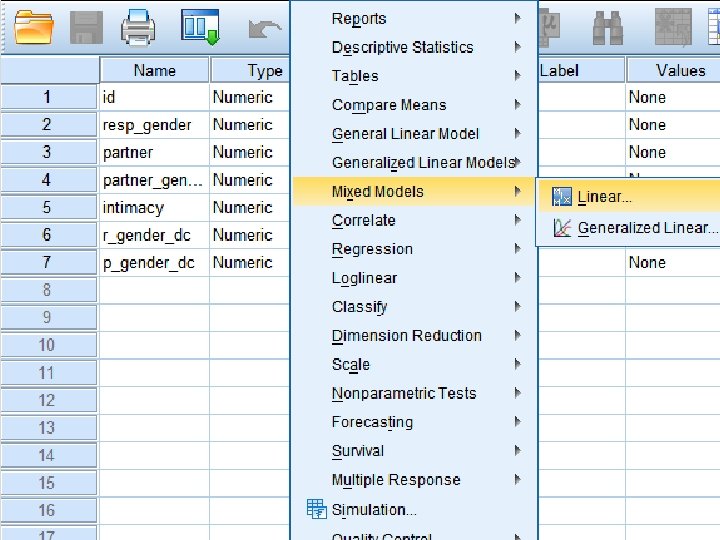
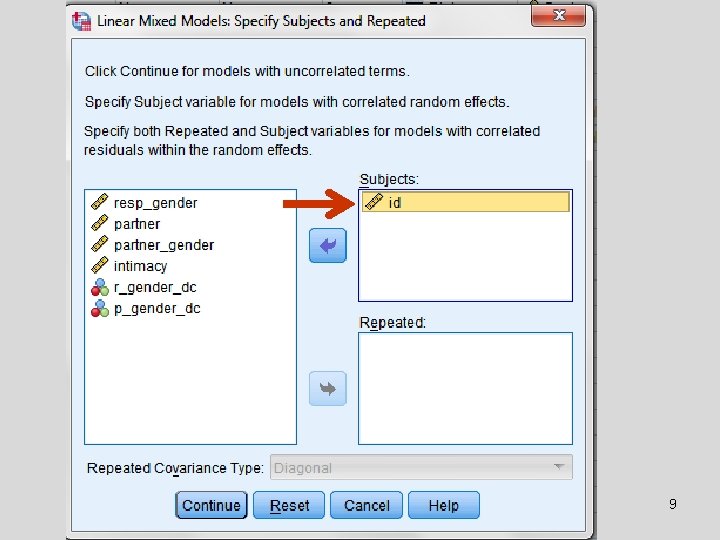
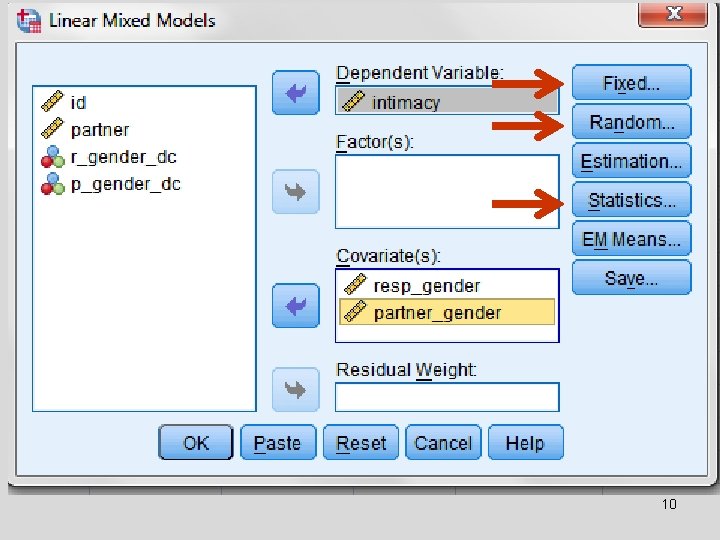
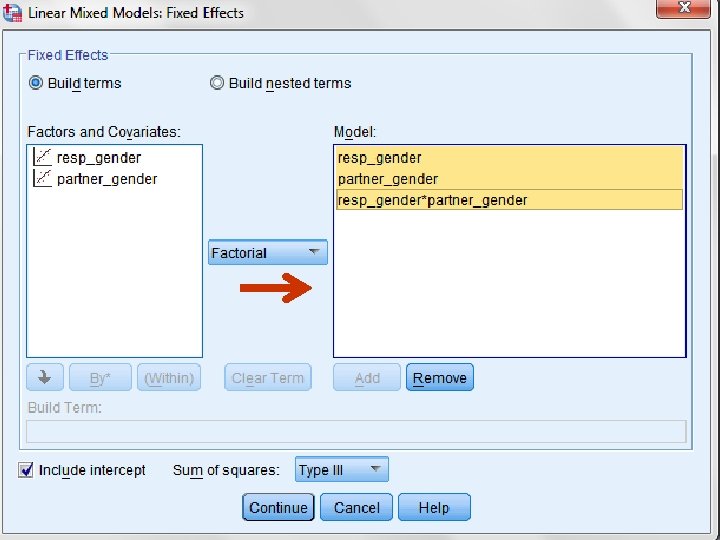
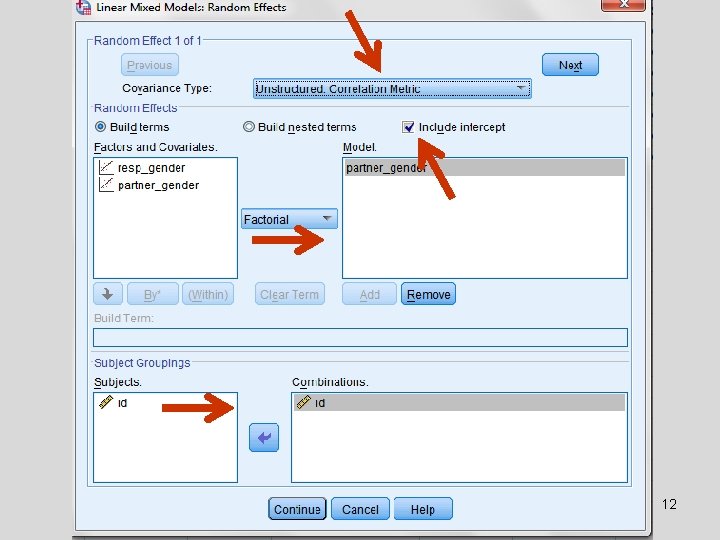
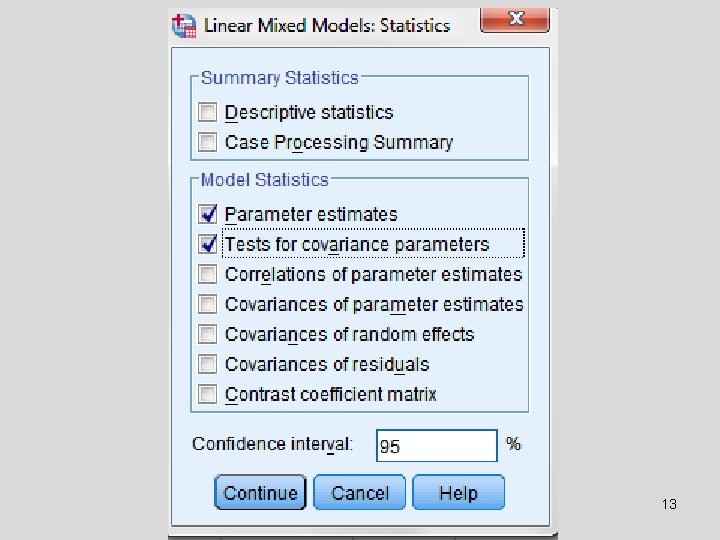
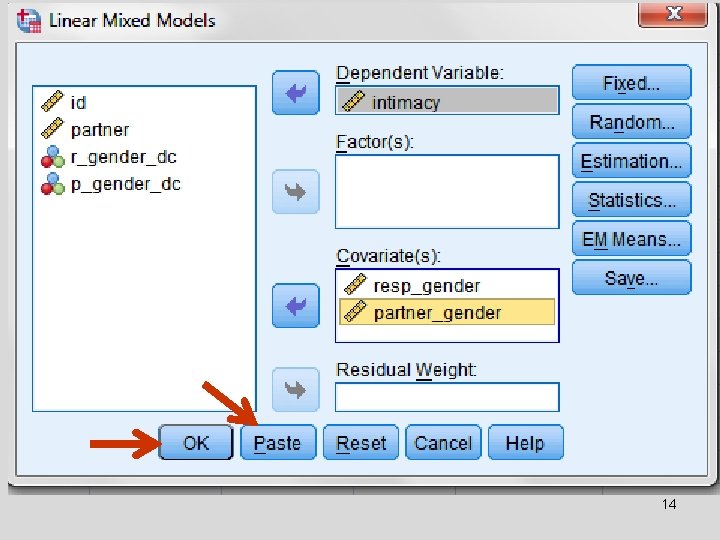
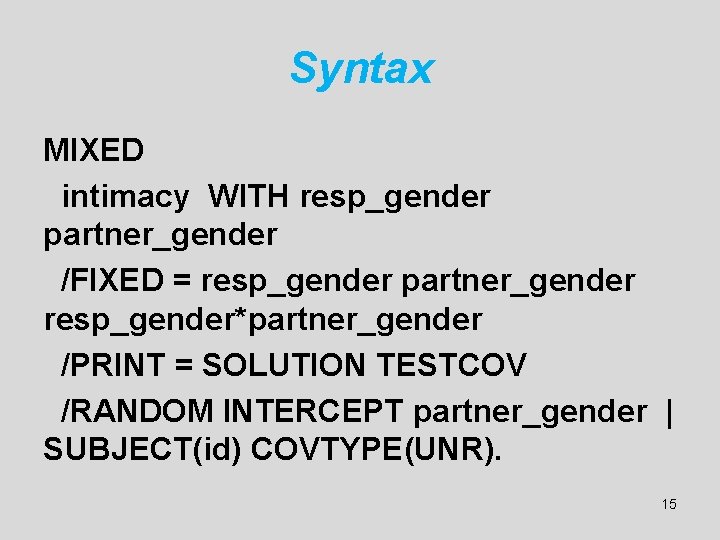
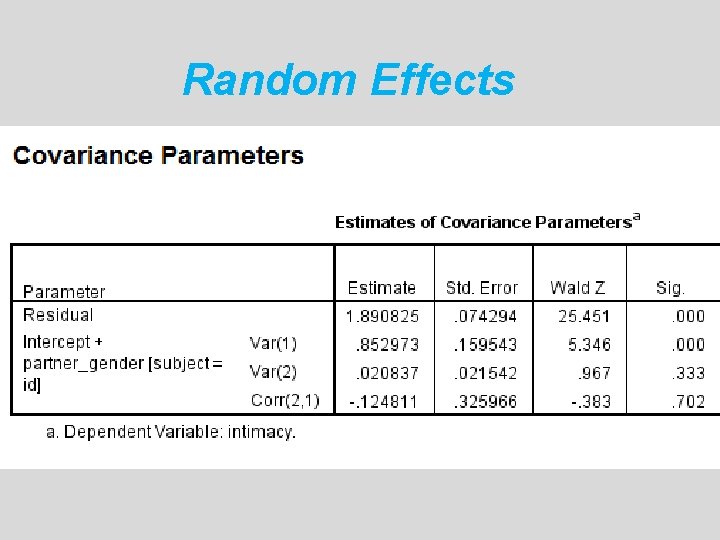
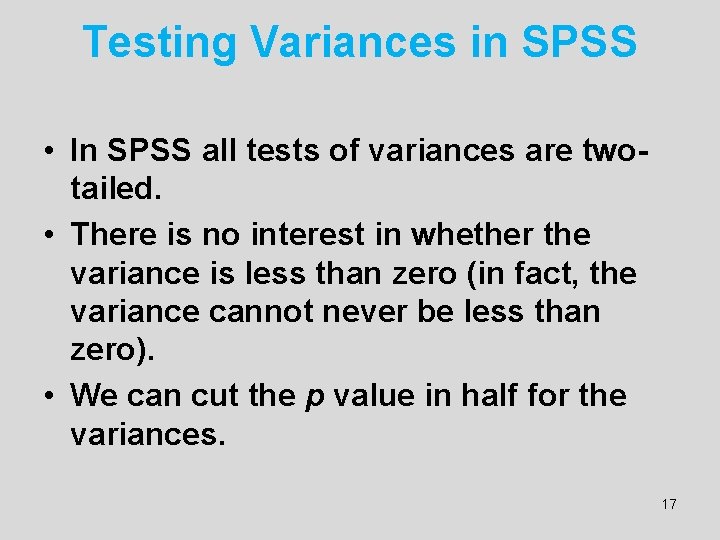
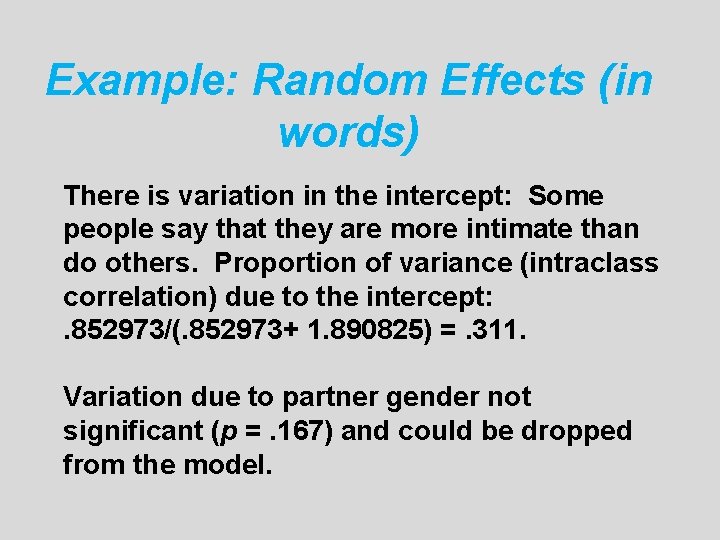
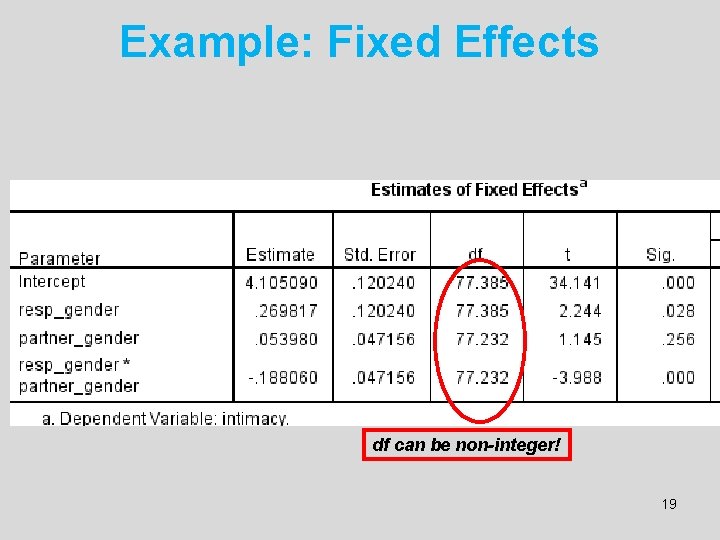
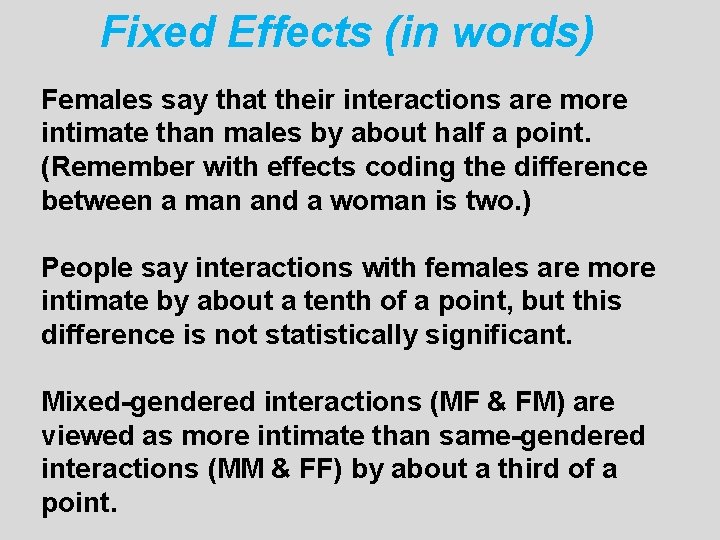
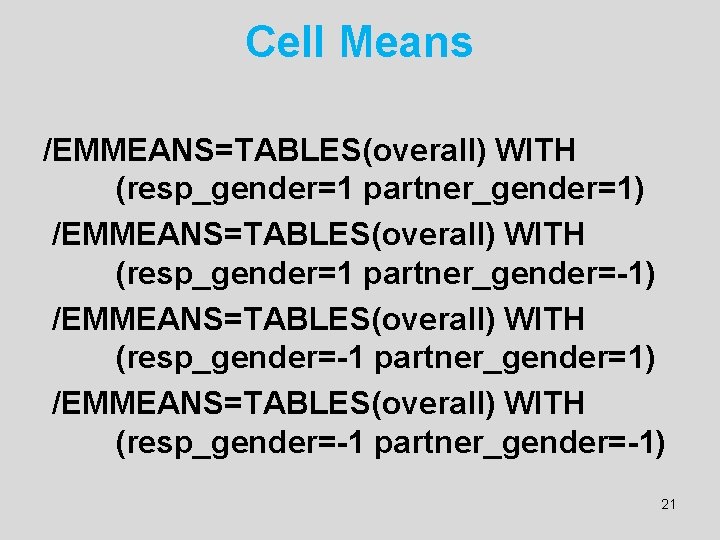
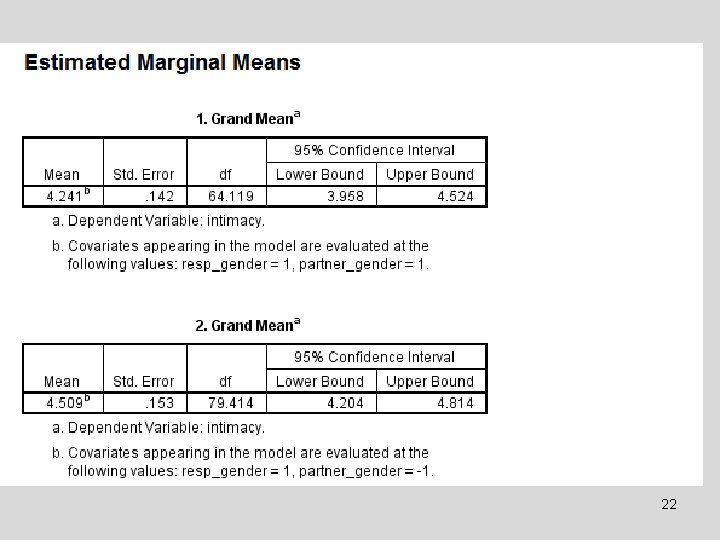
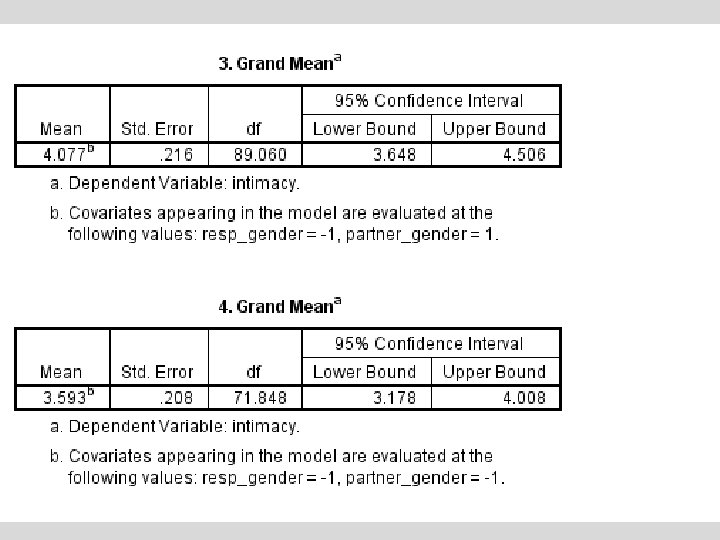
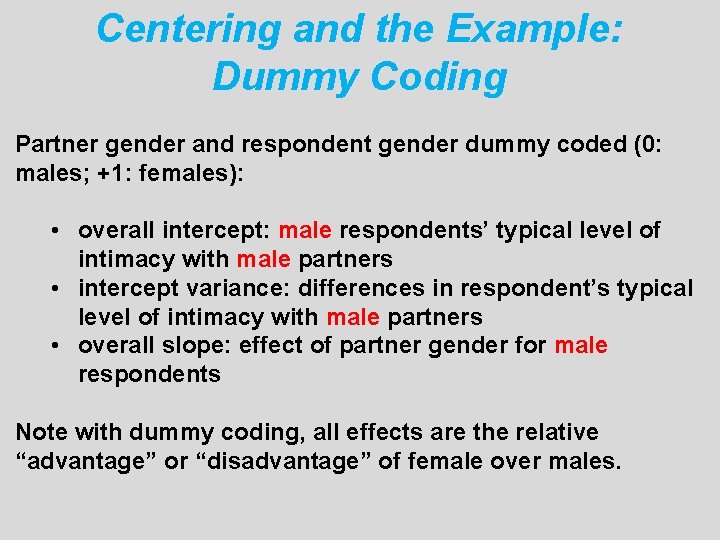
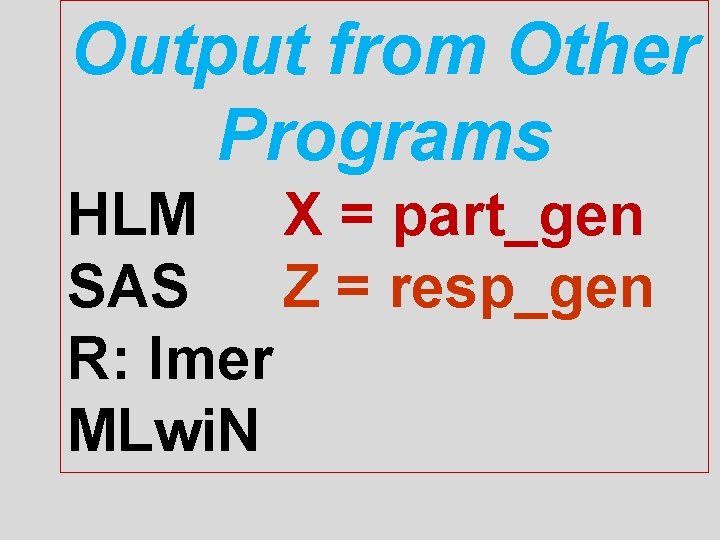
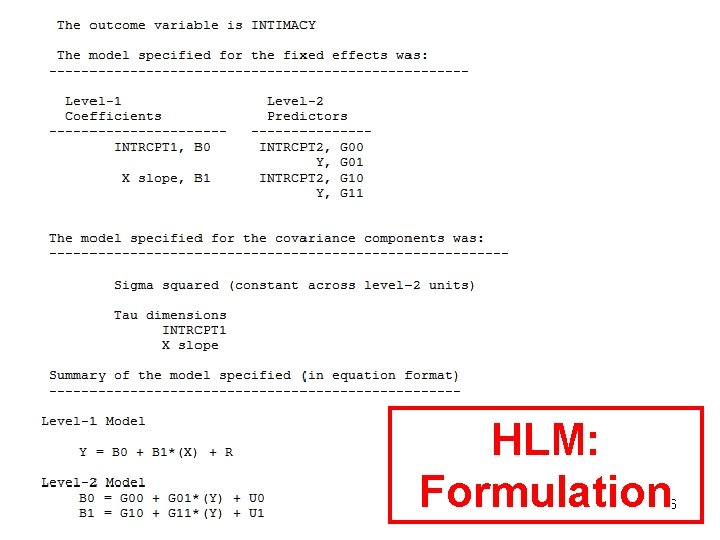
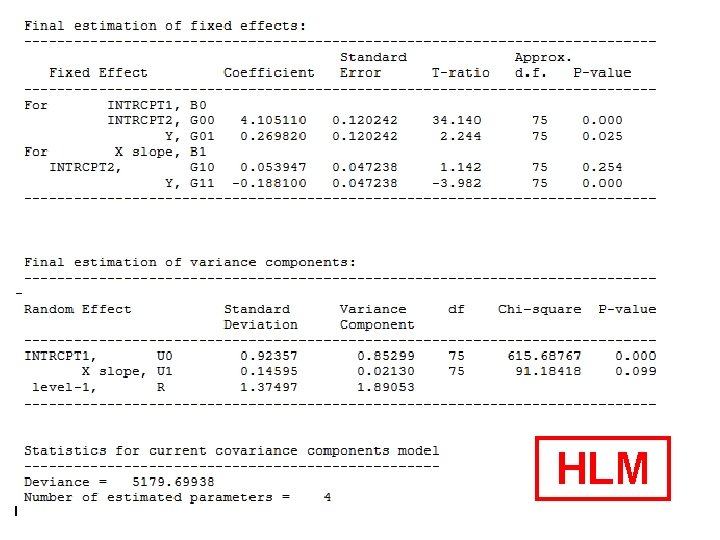
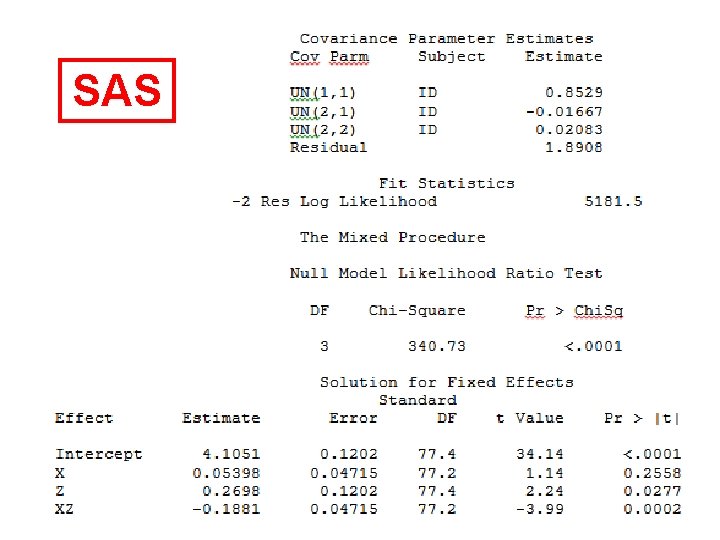
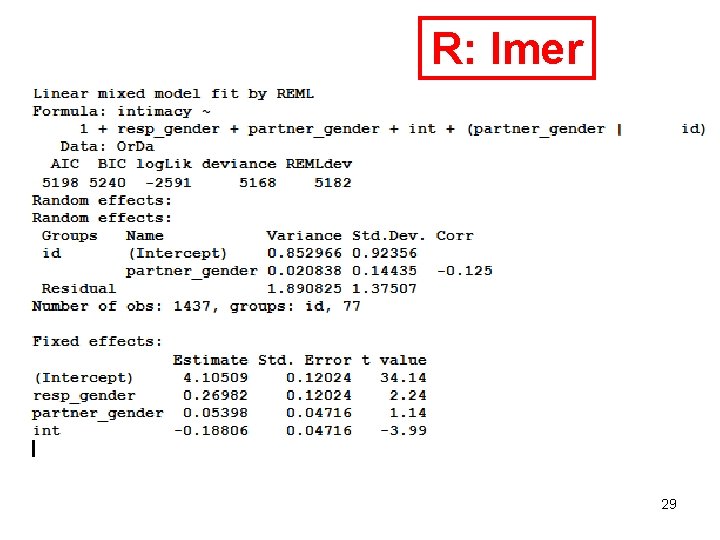
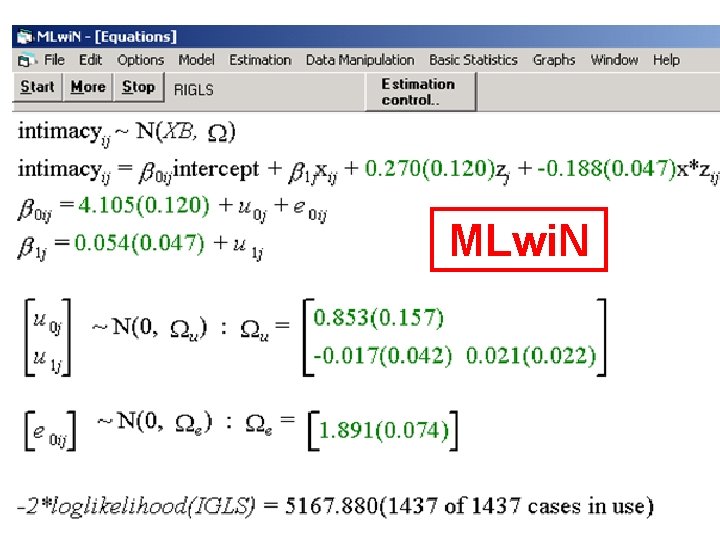
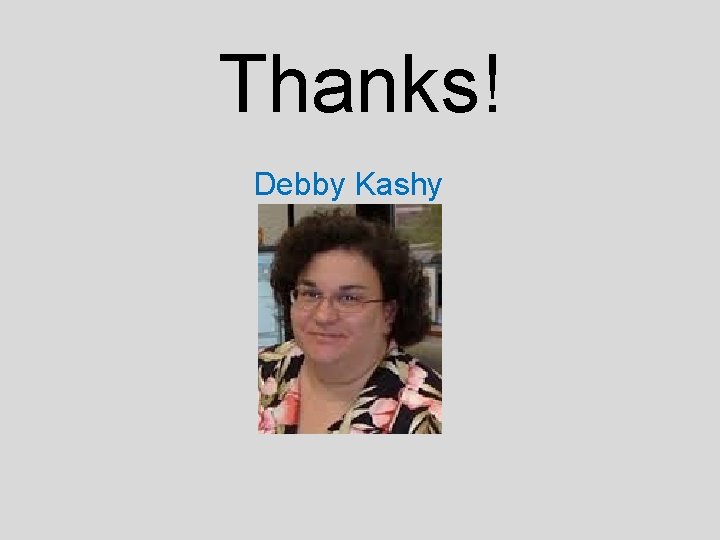
- Slides: 31
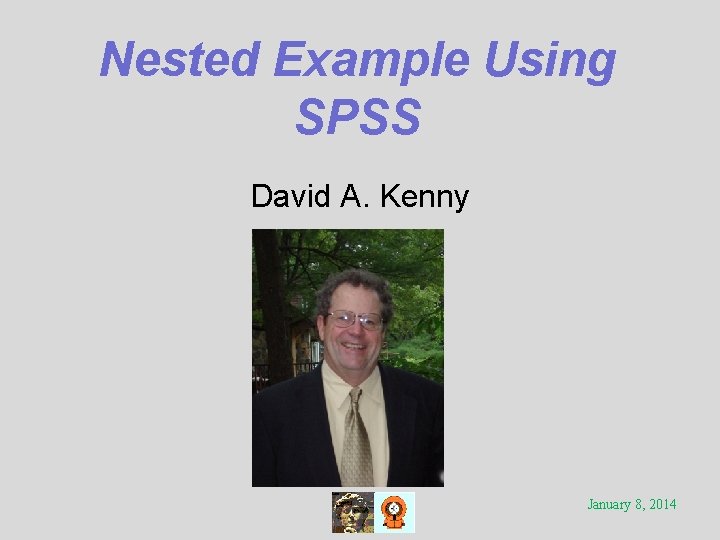
Nested Example Using SPSS David A. Kenny January 8, 2014
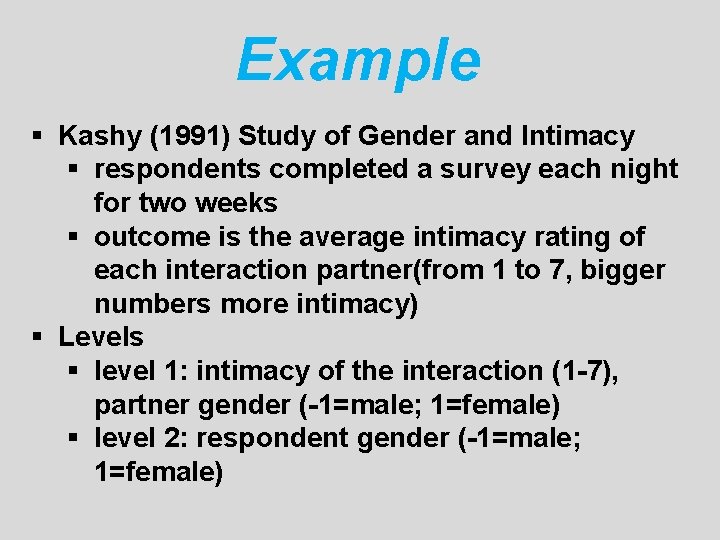
Example § Kashy (1991) Study of Gender and Intimacy § respondents completed a survey each night for two weeks § outcome is the average intimacy rating of each interaction partner(from 1 to 7, bigger numbers more intimacy) § Levels § level 1: intimacy of the interaction (1 -7), partner gender (-1=male; 1=female) § level 2: respondent gender (-1=male; 1=female)
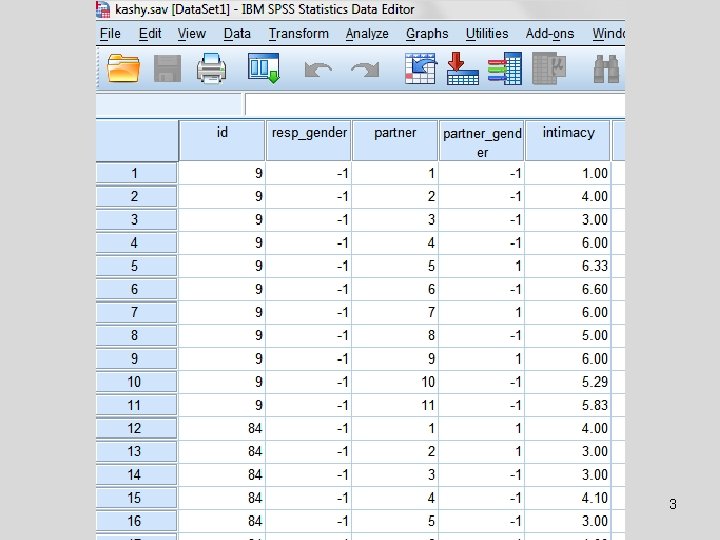
3
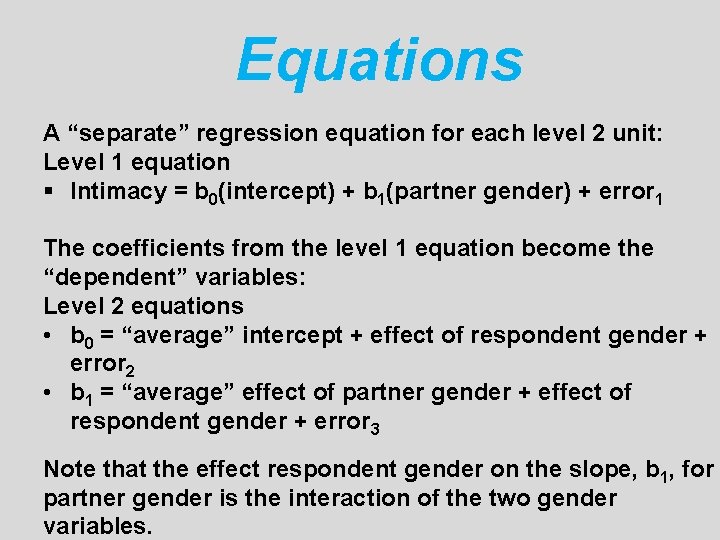
Equations A “separate” regression equation for each level 2 unit: Level 1 equation § Intimacy = b 0(intercept) + b 1(partner gender) + error 1 The coefficients from the level 1 equation become the “dependent” variables: Level 2 equations • b 0 = “average” intercept + effect of respondent gender + error 2 • b 1 = “average” effect of partner gender + effect of respondent gender + error 3 Note that the effect respondent gender on the slope, b 1, for partner gender is the interaction of the two gender variables.
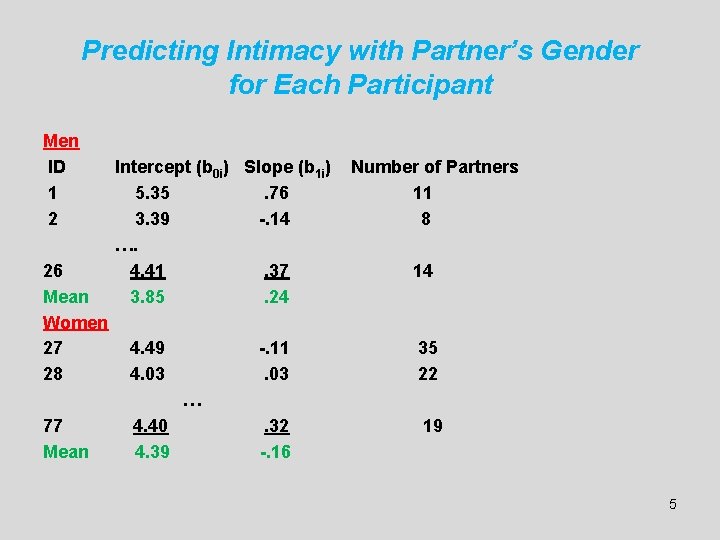
Predicting Intimacy with Partner’s Gender for Each Participant Men ID 1 2 Intercept (b 0 i) Slope (b 1 i) Number of Partners 5. 35 . 76 11 3. 39 -. 14 8 …. 4. 41 . 37 14 3. 85 . 24 26 Mean Women 27 4. 49 -. 11 28 4. 03 … 77 4. 40 . 32 Mean 4. 39 -. 16 35 22 19 5
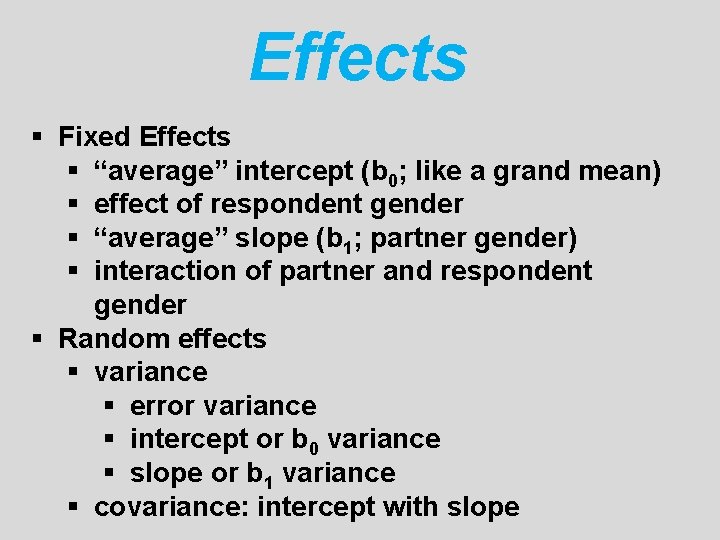
Effects § Fixed Effects § “average” intercept (b 0; like a grand mean) § effect of respondent gender § “average” slope (b 1; partner gender) § interaction of partner and respondent gender § Random effects § variance § error variance § intercept or b 0 variance § slope or b 1 variance § covariance: intercept with slope
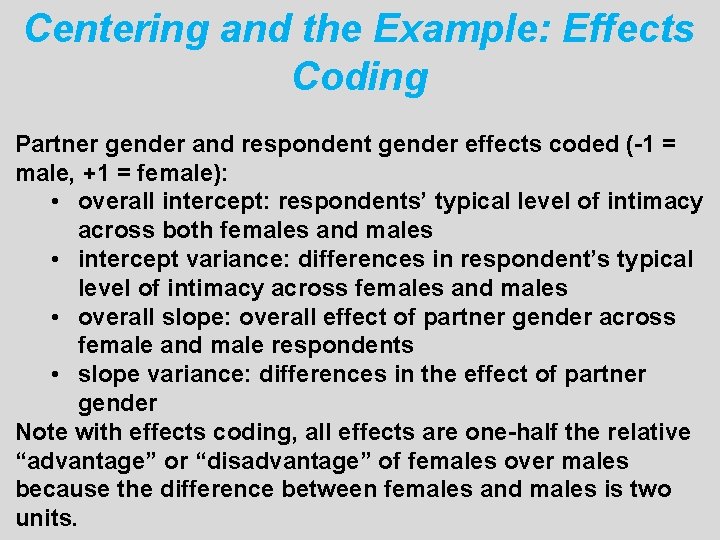
Centering and the Example: Effects Coding Partner gender and respondent gender effects coded (-1 = male, +1 = female): • overall intercept: respondents’ typical level of intimacy across both females and males • intercept variance: differences in respondent’s typical level of intimacy across females and males • overall slope: overall effect of partner gender across female and male respondents • slope variance: differences in the effect of partner gender Note with effects coding, all effects are one-half the relative “advantage” or “disadvantage” of females over males because the difference between females and males is two units.
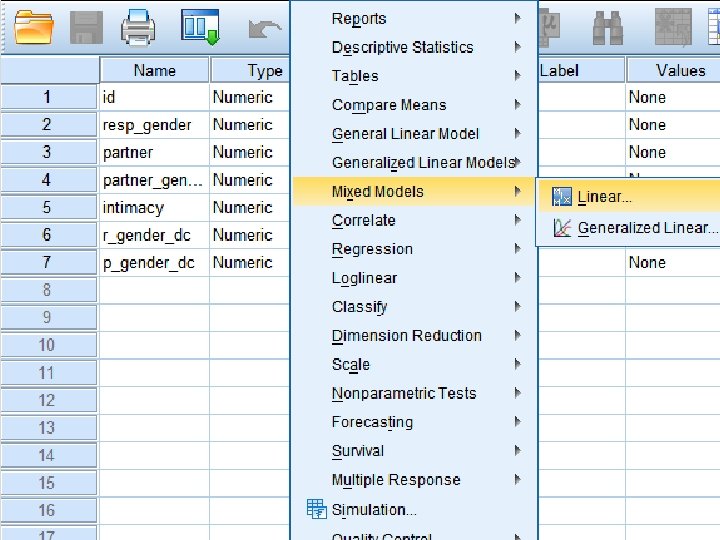
8
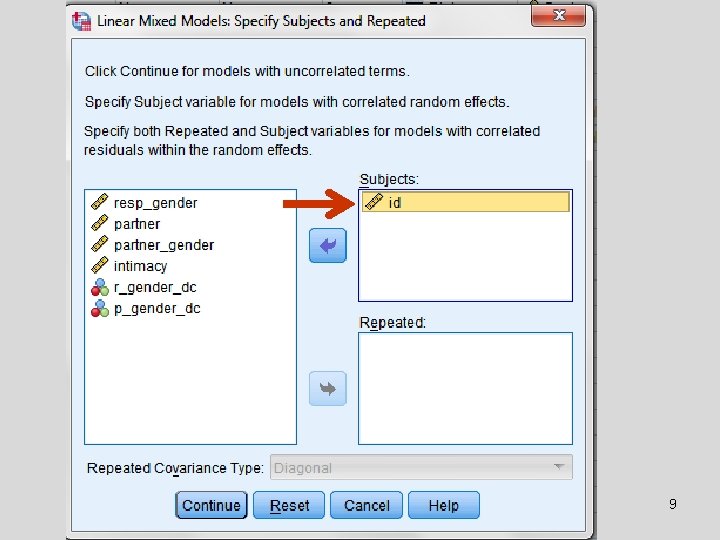
9
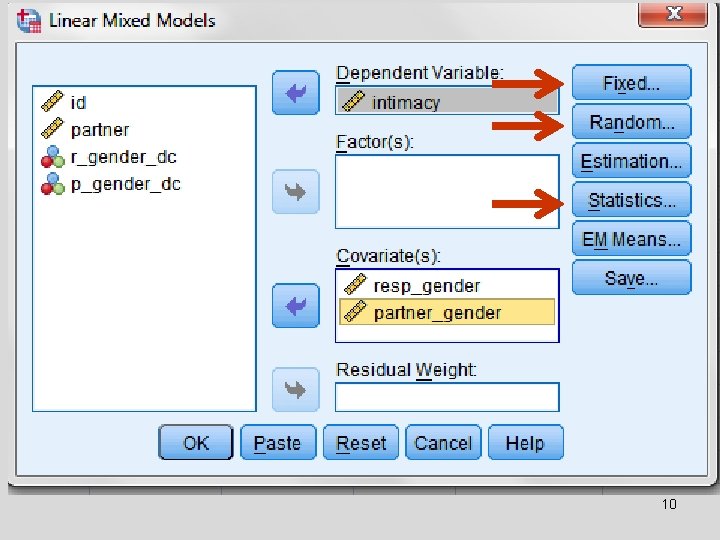
10
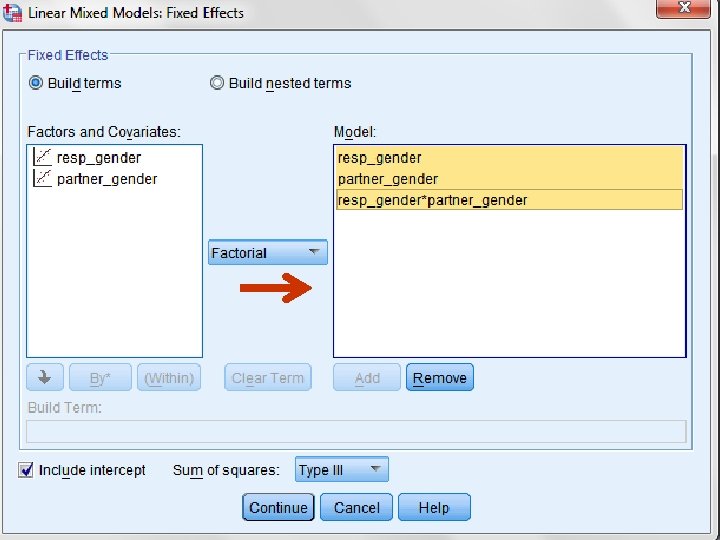
11
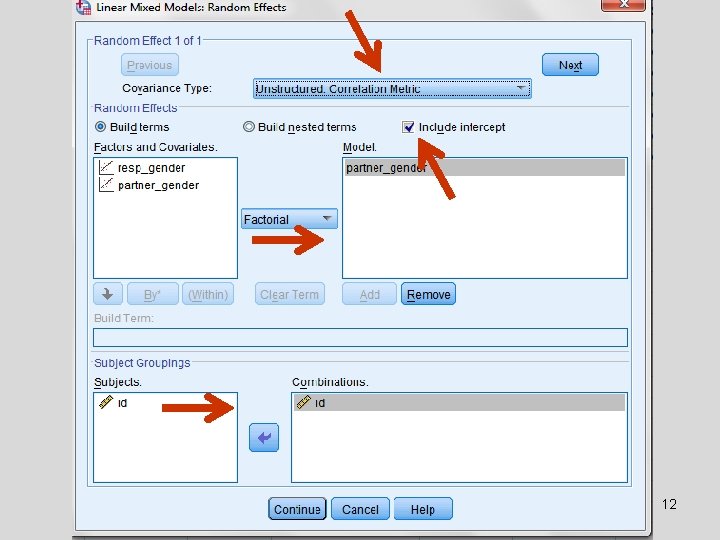
12
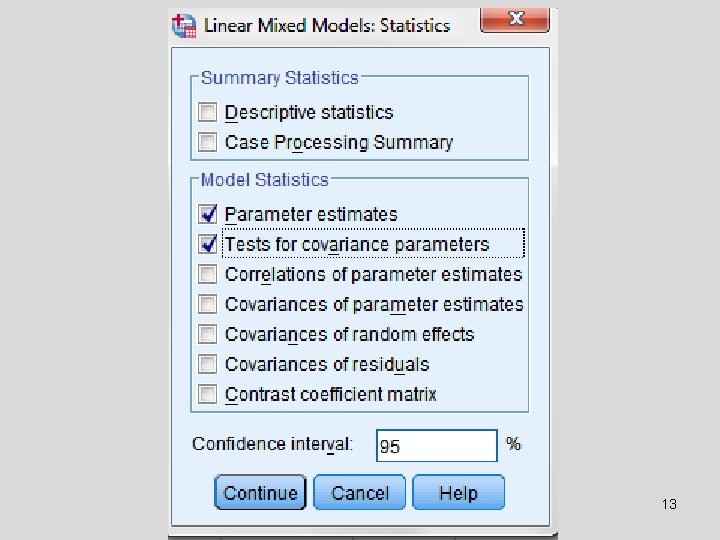
13
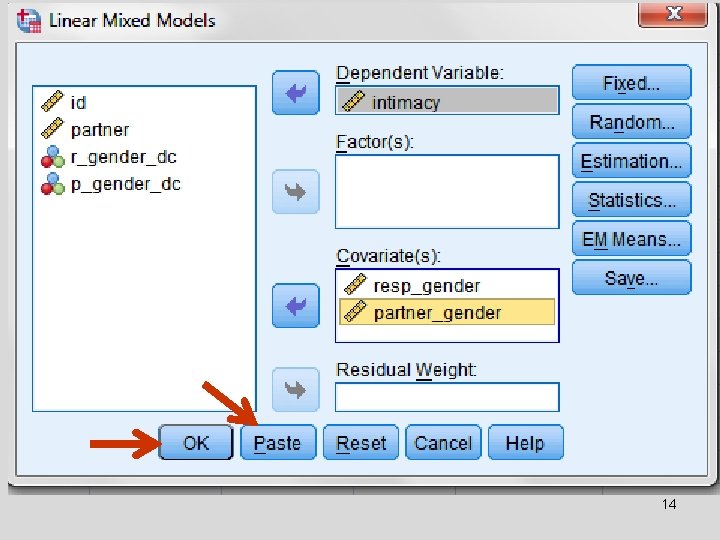
14
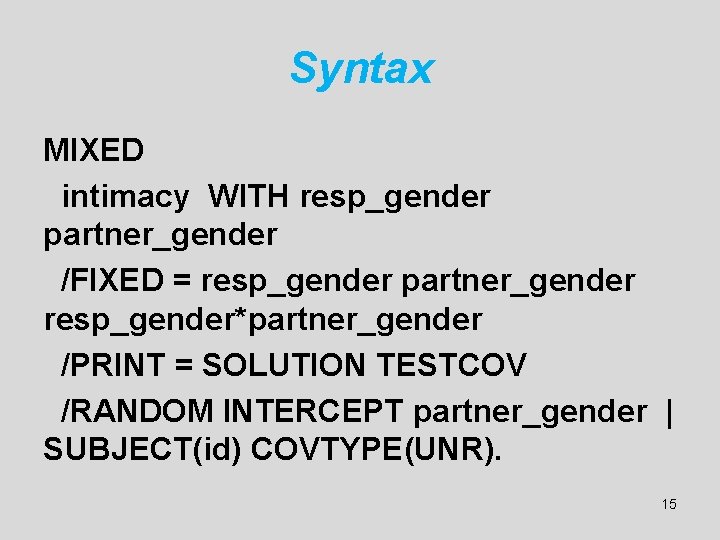
Syntax MIXED intimacy WITH resp_gender partner_gender /FIXED = resp_gender partner_gender resp_gender*partner_gender /PRINT = SOLUTION TESTCOV /RANDOM INTERCEPT partner_gender | SUBJECT(id) COVTYPE(UNR). 15
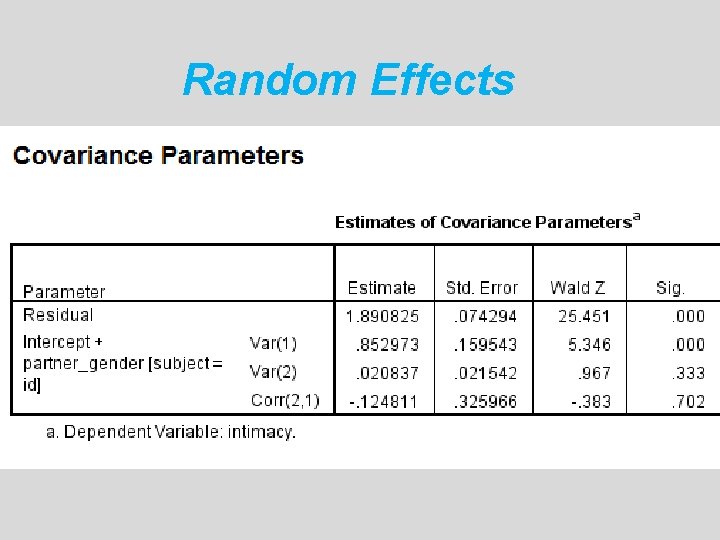
Random Effects
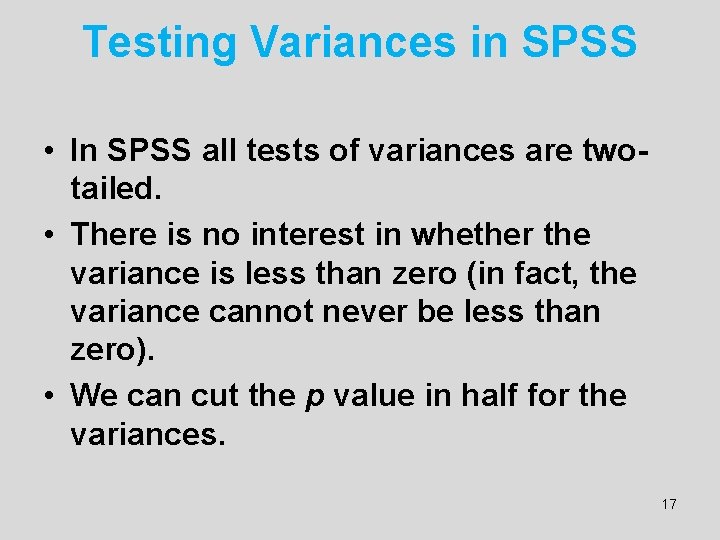
Testing Variances in SPSS • In SPSS all tests of variances are twotailed. • There is no interest in whether the variance is less than zero (in fact, the variance cannot never be less than zero). • We can cut the p value in half for the variances. 17
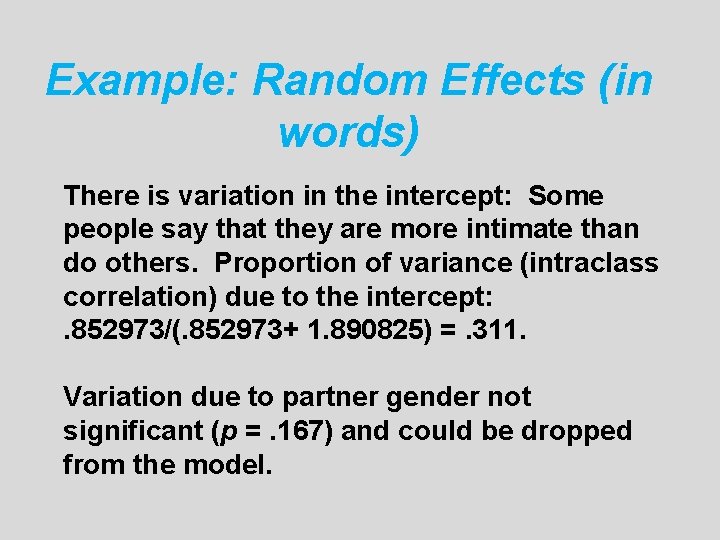
Example: Random Effects (in words) There is variation in the intercept: Some people say that they are more intimate than do others. Proportion of variance (intraclass correlation) due to the intercept: . 852973/(. 852973+ 1. 890825) =. 311. Variation due to partner gender not significant (p =. 167) and could be dropped from the model.
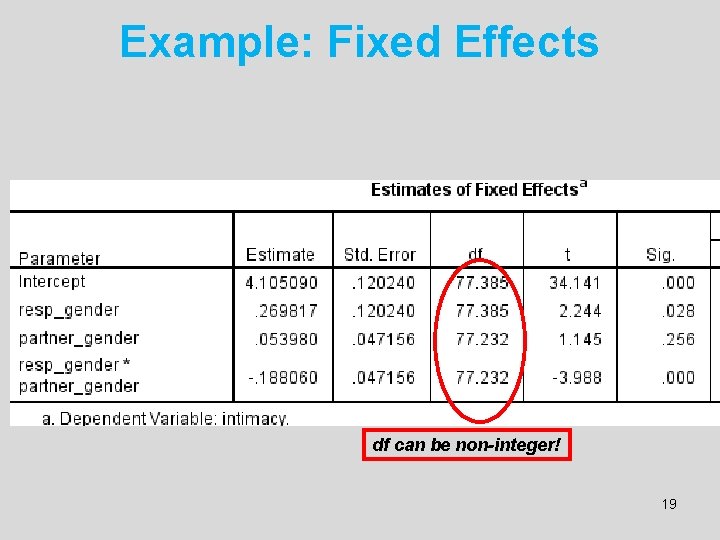
Example: Fixed Effects df can be non-integer! 19
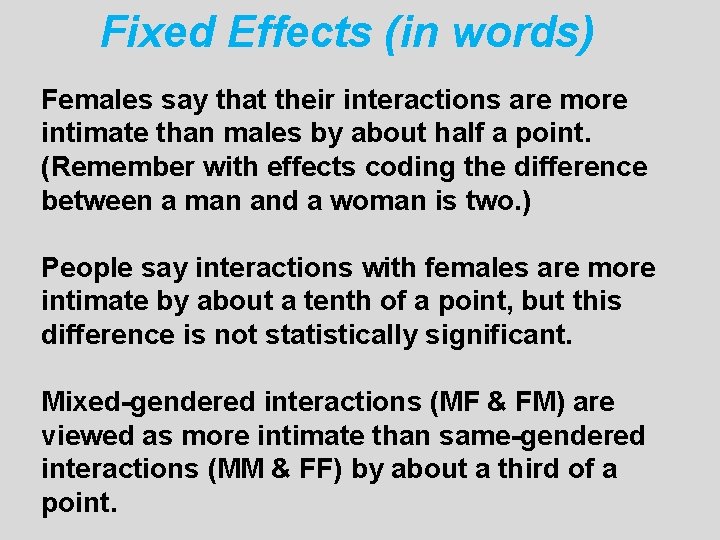
Fixed Effects (in words) Females say that their interactions are more intimate than males by about half a point. (Remember with effects coding the difference between a man and a woman is two. ) People say interactions with females are more intimate by about a tenth of a point, but this difference is not statistically significant. Mixed-gendered interactions (MF & FM) are viewed as more intimate than same-gendered interactions (MM & FF) by about a third of a point.
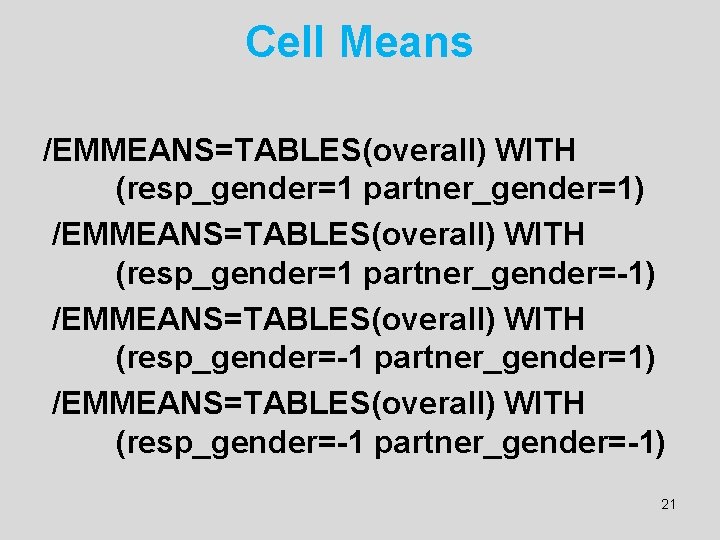
Cell Means /EMMEANS=TABLES(overall) WITH (resp_gender=1 partner_gender=1) /EMMEANS=TABLES(overall) WITH (resp_gender=1 partner_gender=-1) /EMMEANS=TABLES(overall) WITH (resp_gender=-1 partner_gender=-1) 21
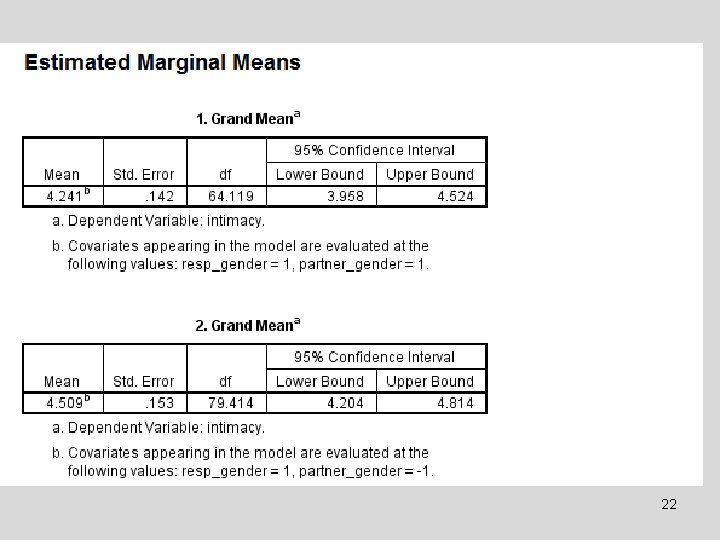
22
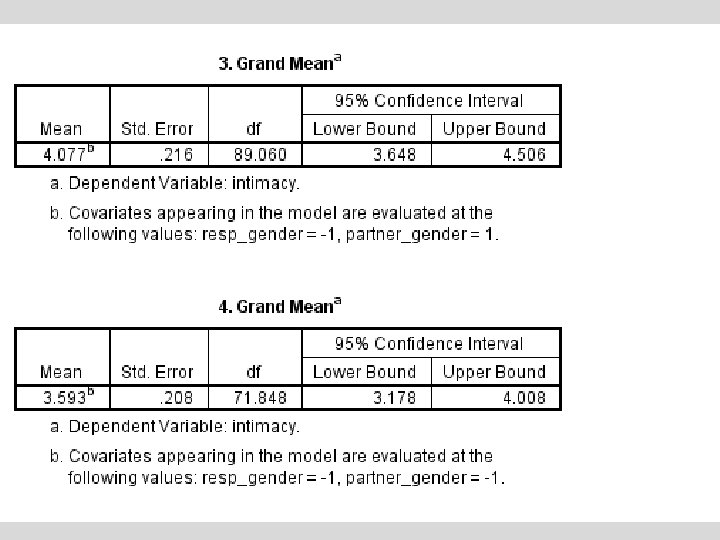
23
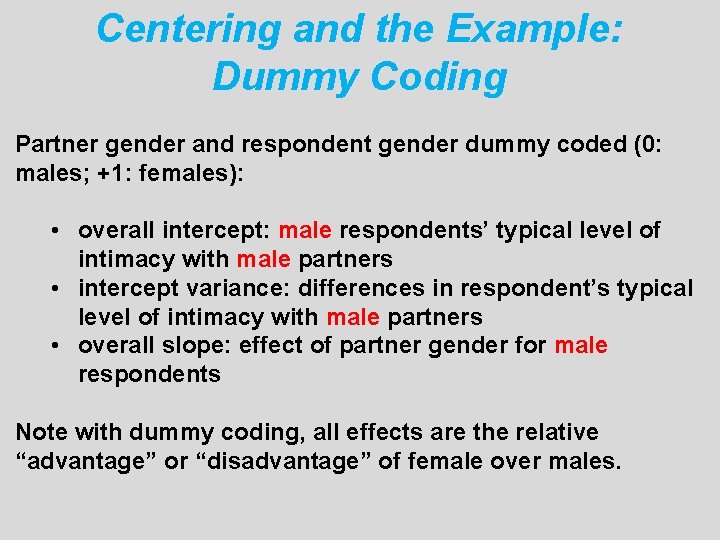
Centering and the Example: Dummy Coding Partner gender and respondent gender dummy coded (0: males; +1: females): • overall intercept: male respondents’ typical level of intimacy with male partners • intercept variance: differences in respondent’s typical level of intimacy with male partners • overall slope: effect of partner gender for male respondents Note with dummy coding, all effects are the relative “advantage” or “disadvantage” of female over males.
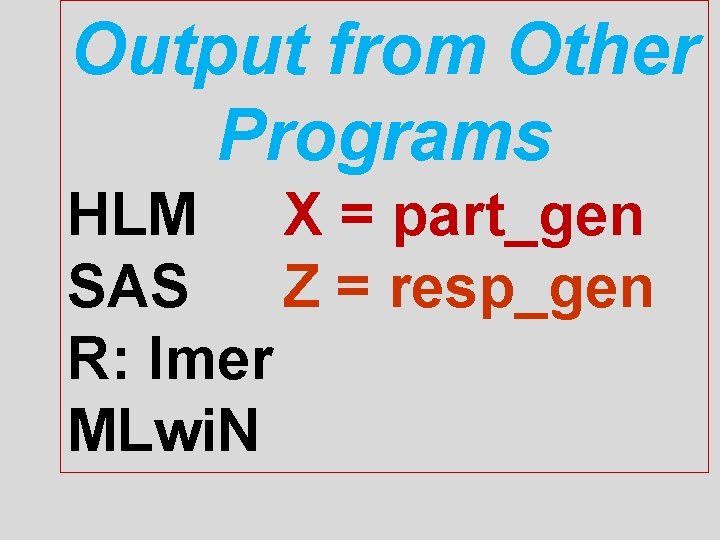
Output from Other Programs HLM X = part_gen SAS Z = resp_gen R: lmer MLwi. N
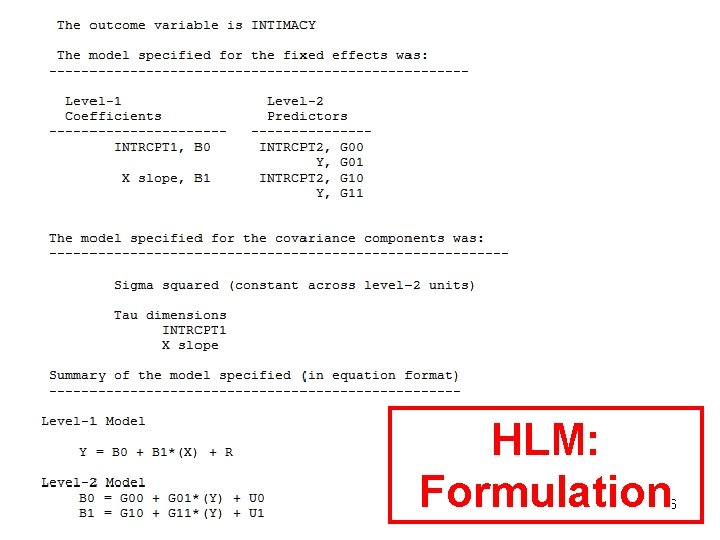
HLM: Formulation 26
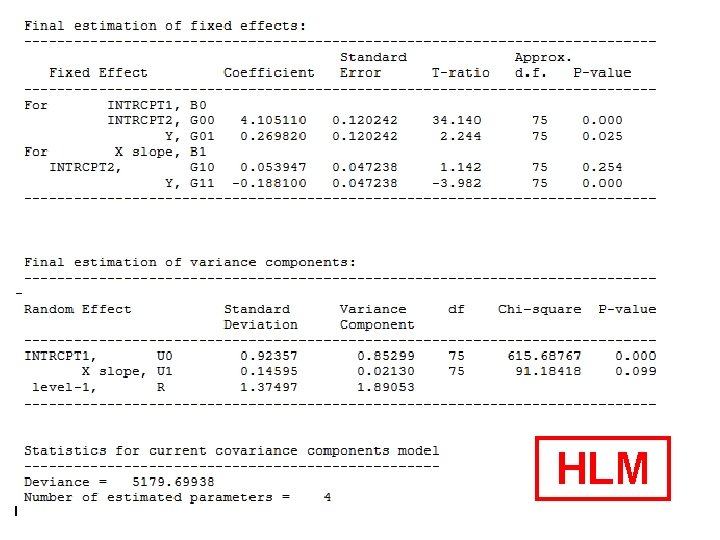
HLM 27
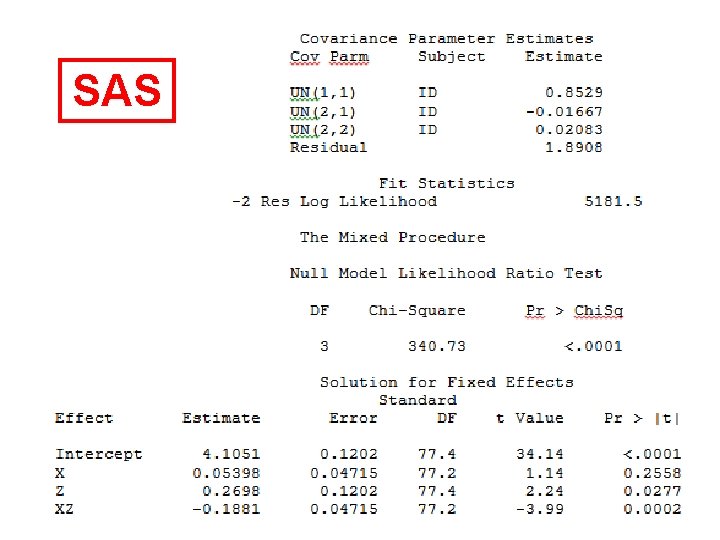
SAS 28
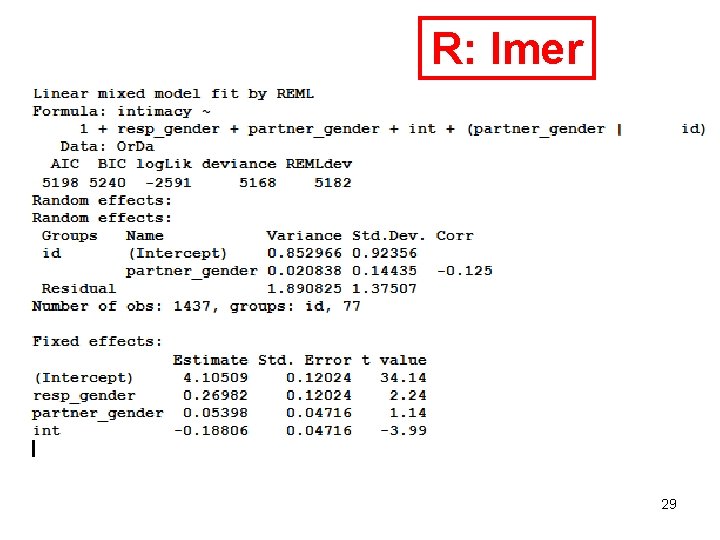
R: lmer 29
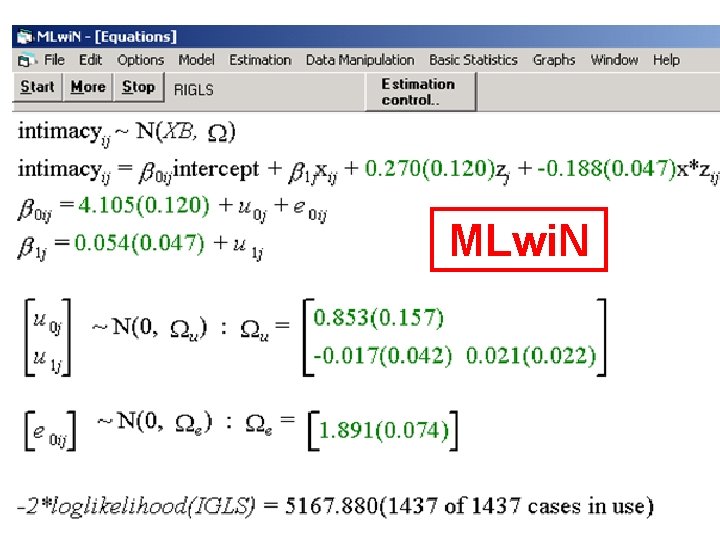
MLwi. N 30
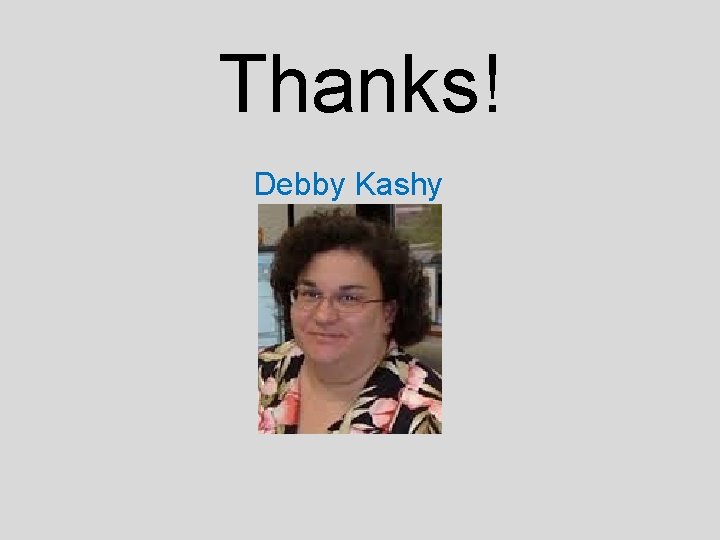
Thanks! Debby Kashy
Nested anova spss
David kenny mediation
David kenny sem
Srmr
Sem model fit
Kenny samuel david
Ounluk
Block nested-loop join
Standardized canonical discriminant function coefficients
Nested quotation example
Pseudocode for loop example
Three stage nested design
Flat transaction in distributed system
The tannery liverpool
The watsons go to birmingham kenny
Kenny rogers the gambler meaning
Make a prediction about kenny and franchesca
Kenny ray carter
Kenny chesney cleveland
Farfetch scandal
Andy field moderation
Qian hu kenny yap
Kenny clamp
Kenny g louis armstrong
Kenny colson
Kenny holmes the voice
Monotony of the road
Kenny sou
Kenny lischer
What did kenny expect grandma sands to look like?
Kenny web
Yyy6yy