Multiple Regression Example David A Kenny January 31
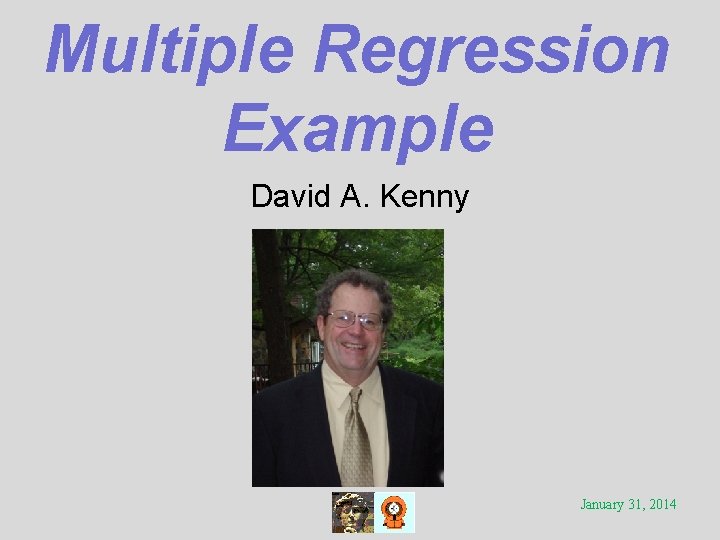
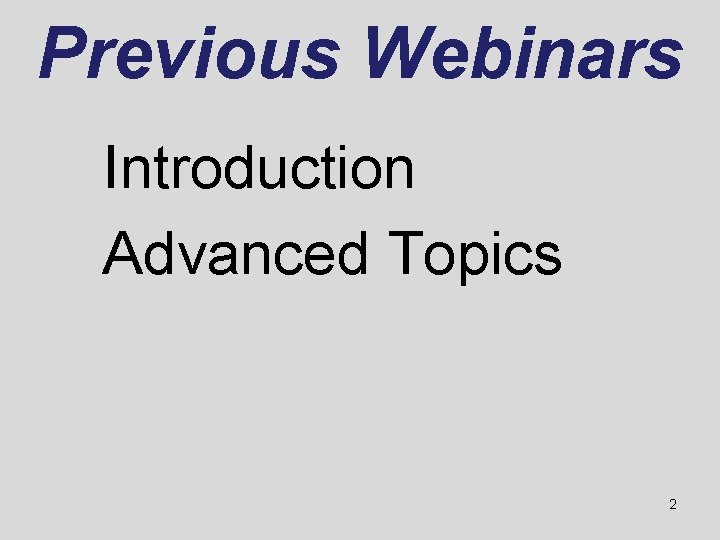
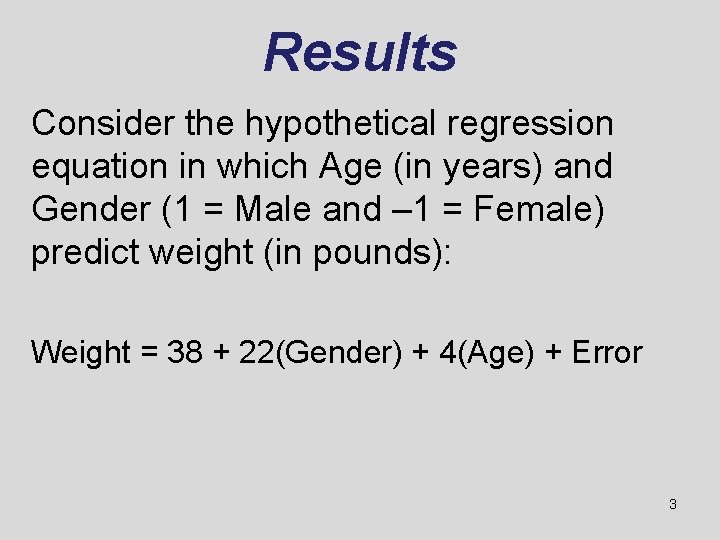
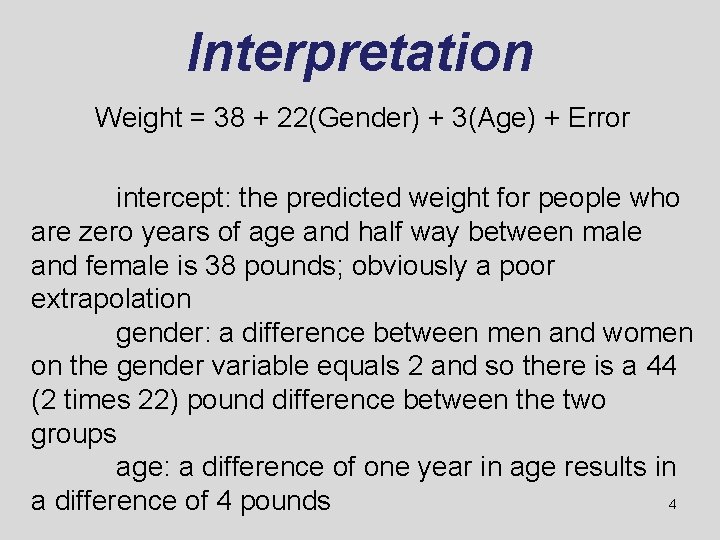
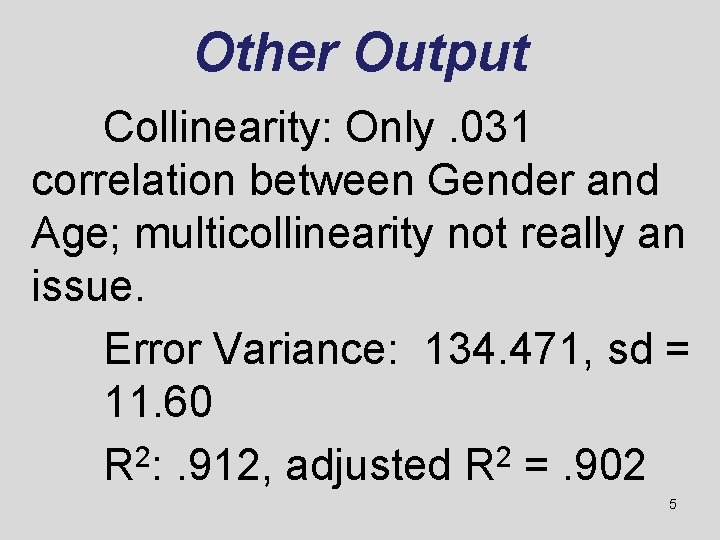
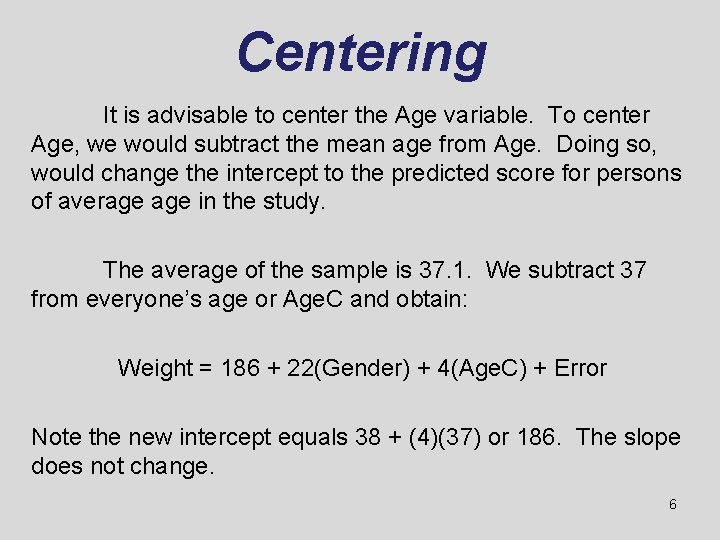
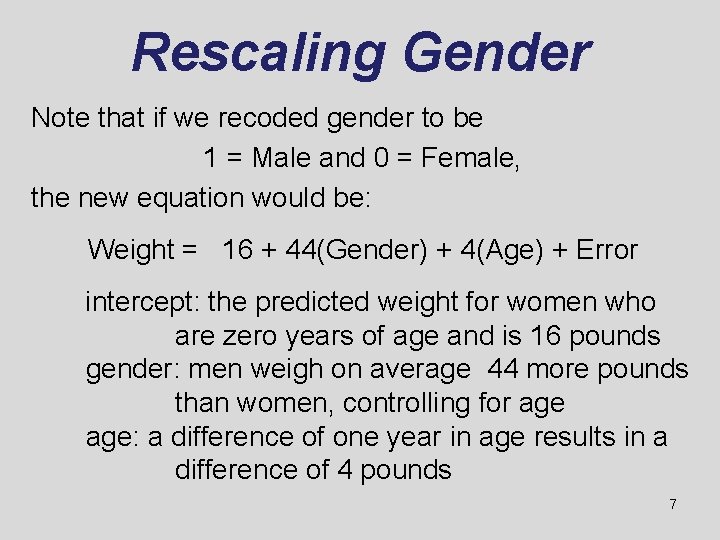
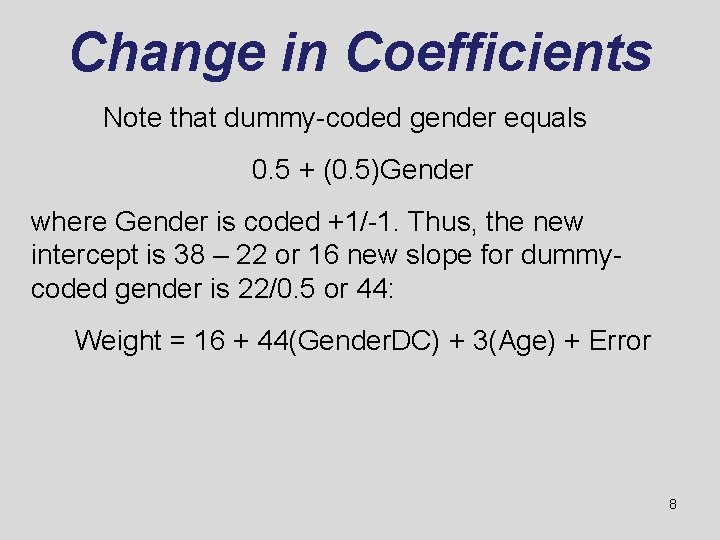
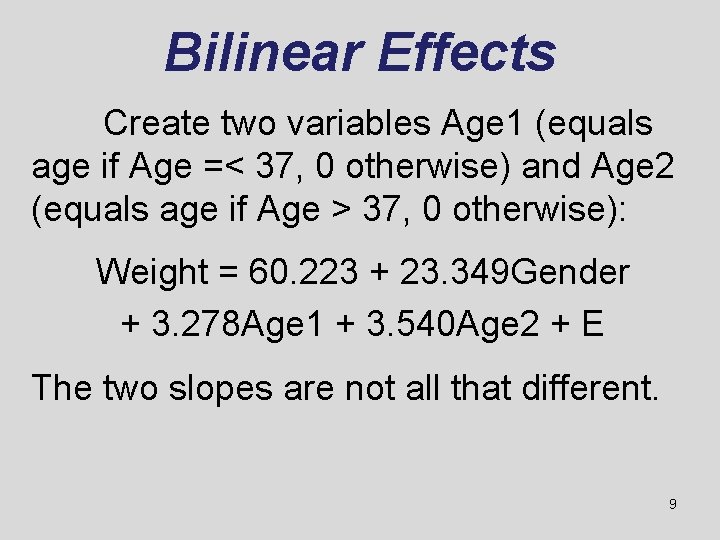
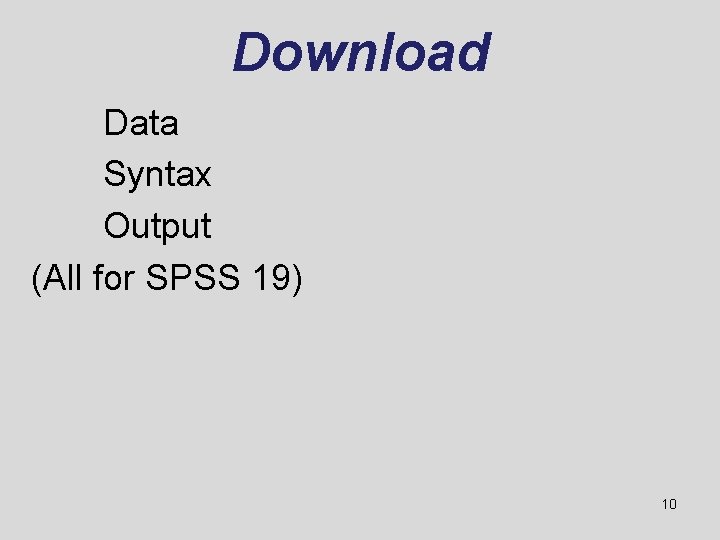
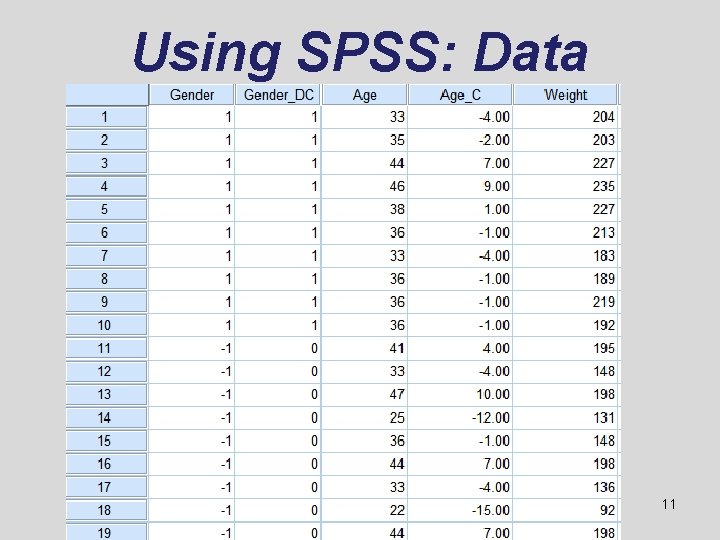
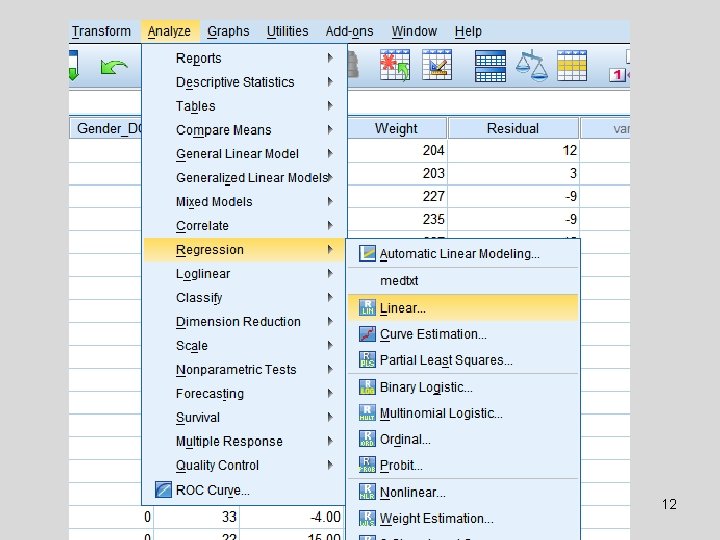
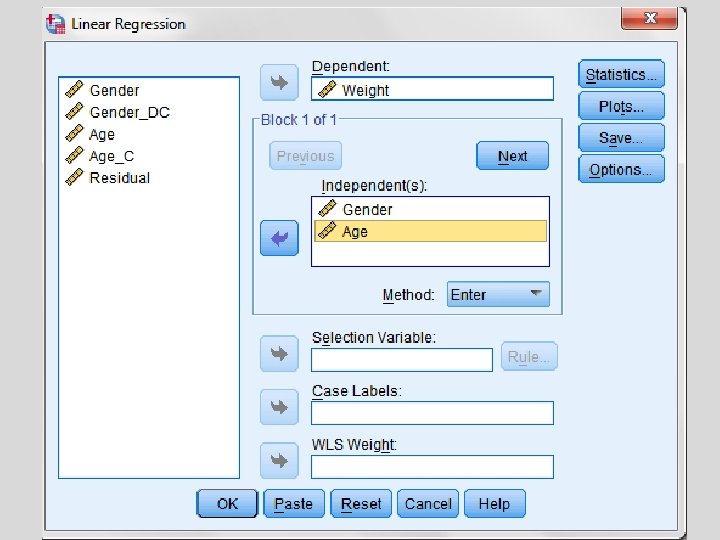
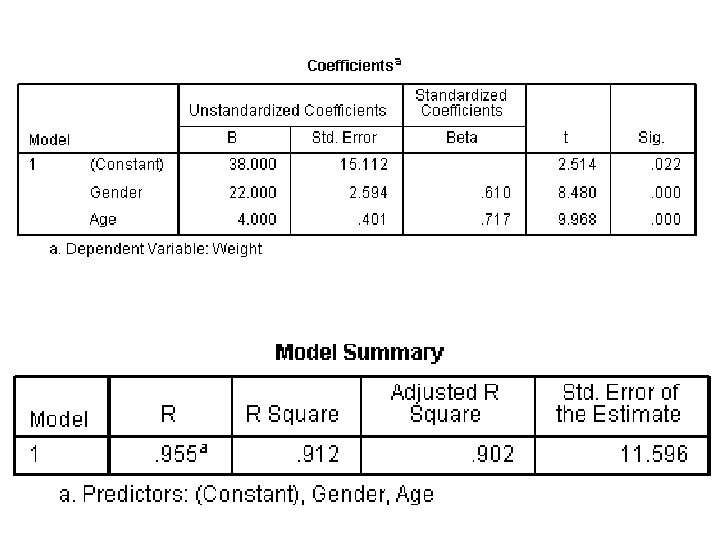
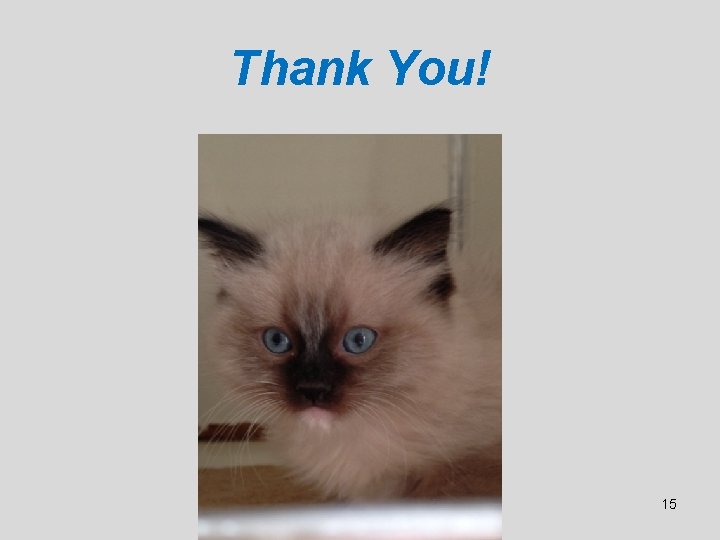
- Slides: 15
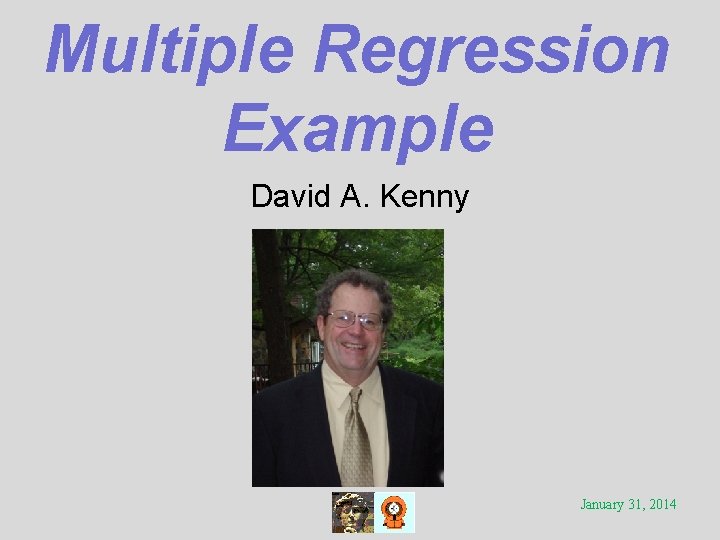
Multiple Regression Example David A. Kenny January 31, 2014
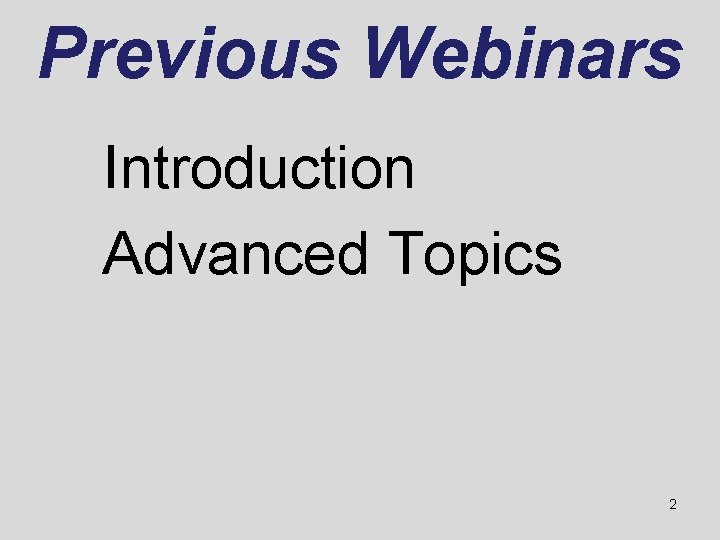
Previous Webinars Introduction Advanced Topics 2
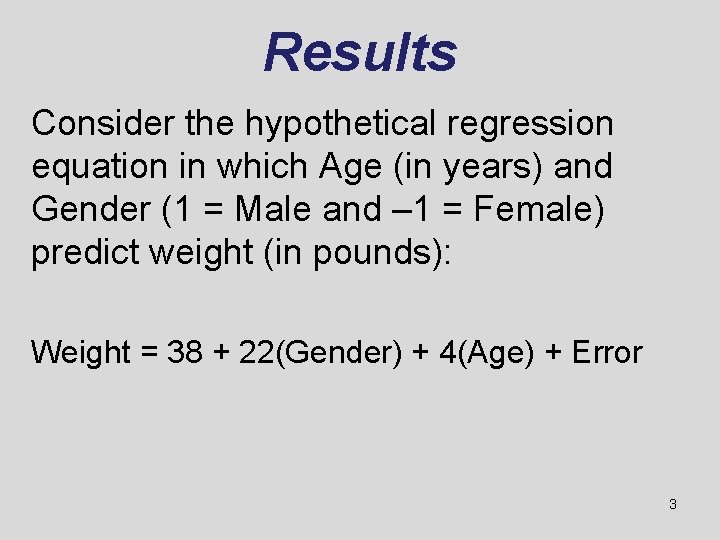
Results Consider the hypothetical regression equation in which Age (in years) and Gender (1 = Male and – 1 = Female) predict weight (in pounds): Weight = 38 + 22(Gender) + 4(Age) + Error 3
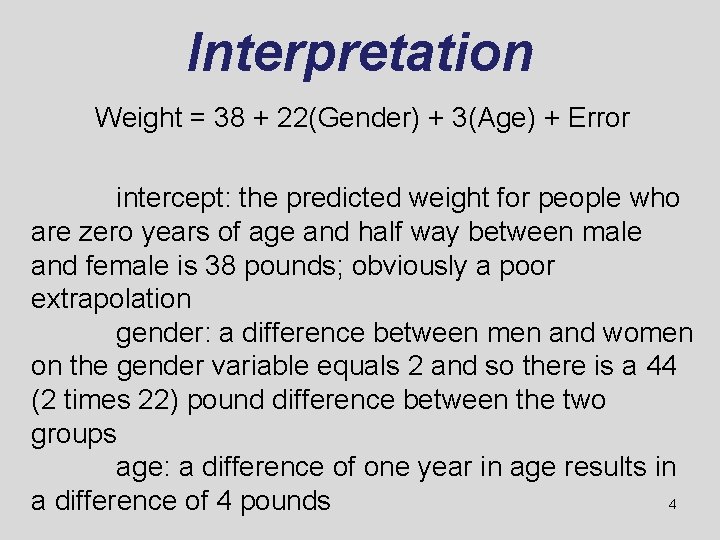
Interpretation Weight = 38 + 22(Gender) + 3(Age) + Error intercept: the predicted weight for people who are zero years of age and half way between male and female is 38 pounds; obviously a poor extrapolation gender: a difference between men and women on the gender variable equals 2 and so there is a 44 (2 times 22) pound difference between the two groups age: a difference of one year in age results in 4 a difference of 4 pounds
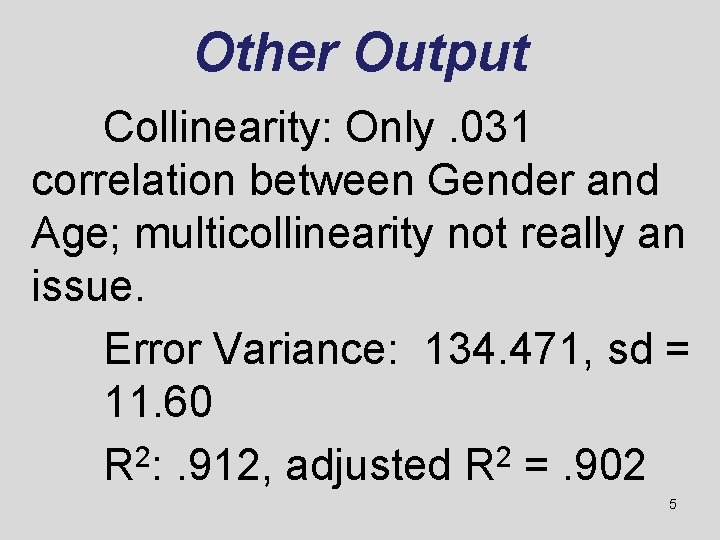
Other Output Collinearity: Only. 031 correlation between Gender and Age; multicollinearity not really an issue. Error Variance: 134. 471, sd = 11. 60 2 2 R : . 912, adjusted R =. 902 5
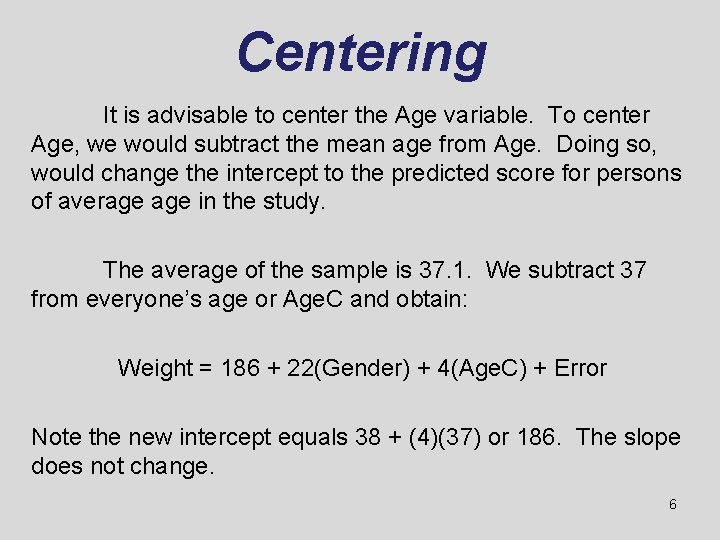
Centering It is advisable to center the Age variable. To center Age, we would subtract the mean age from Age. Doing so, would change the intercept to the predicted score for persons of average in the study. The average of the sample is 37. 1. We subtract 37 from everyone’s age or Age. C and obtain: Weight = 186 + 22(Gender) + 4(Age. C) + Error Note the new intercept equals 38 + (4)(37) or 186. The slope does not change. 6
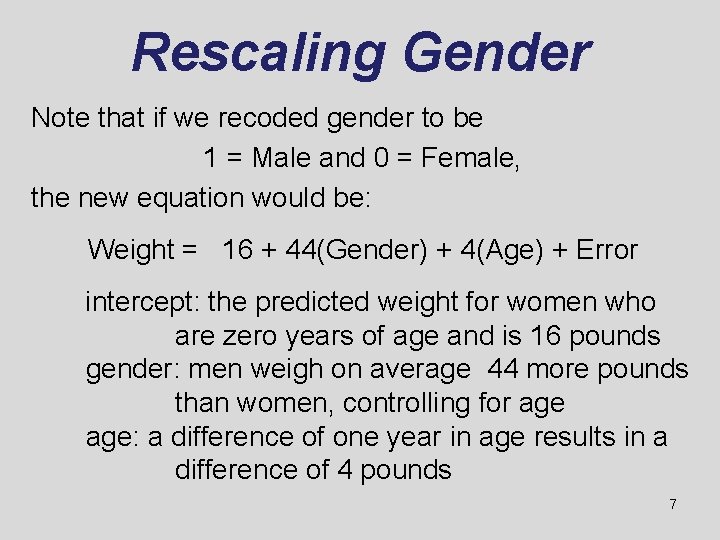
Rescaling Gender Note that if we recoded gender to be 1 = Male and 0 = Female, the new equation would be: Weight = 16 + 44(Gender) + 4(Age) + Error intercept: the predicted weight for women who are zero years of age and is 16 pounds gender: men weigh on average 44 more pounds than women, controlling for age: a difference of one year in age results in a difference of 4 pounds 7
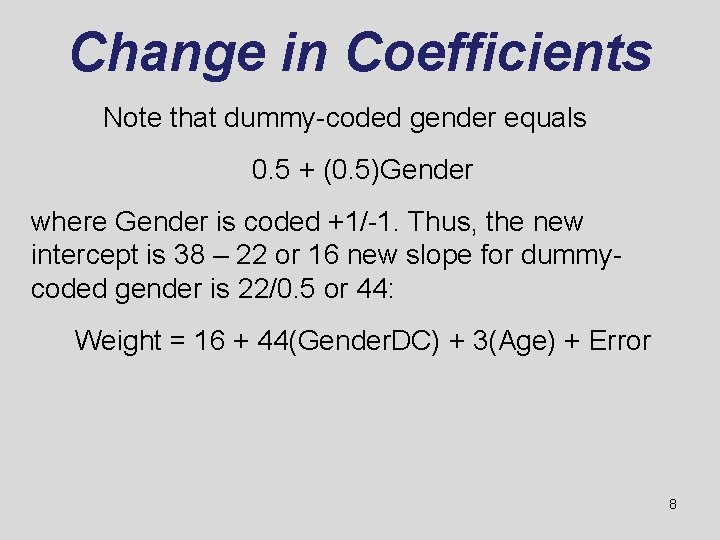
Change in Coefficients Note that dummy-coded gender equals 0. 5 + (0. 5)Gender where Gender is coded +1/-1. Thus, the new intercept is 38 – 22 or 16 new slope for dummycoded gender is 22/0. 5 or 44: Weight = 16 + 44(Gender. DC) + 3(Age) + Error 8
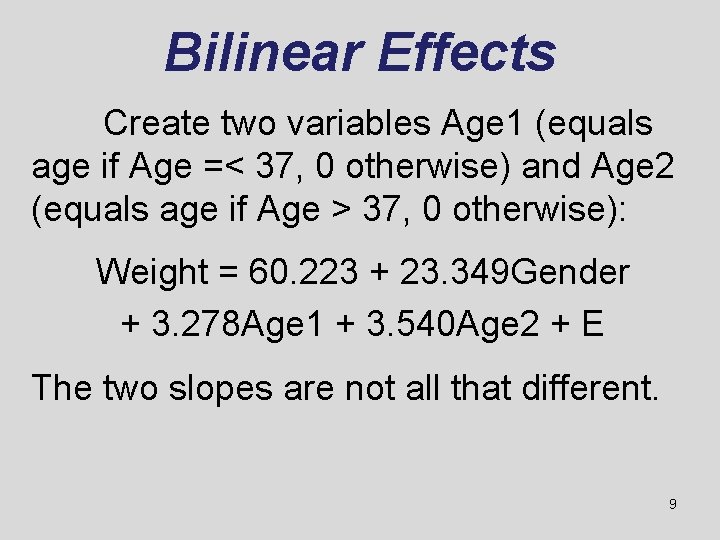
Bilinear Effects Create two variables Age 1 (equals age if Age =< 37, 0 otherwise) and Age 2 (equals age if Age > 37, 0 otherwise): Weight = 60. 223 + 23. 349 Gender + 3. 278 Age 1 + 3. 540 Age 2 + E The two slopes are not all that different. 9
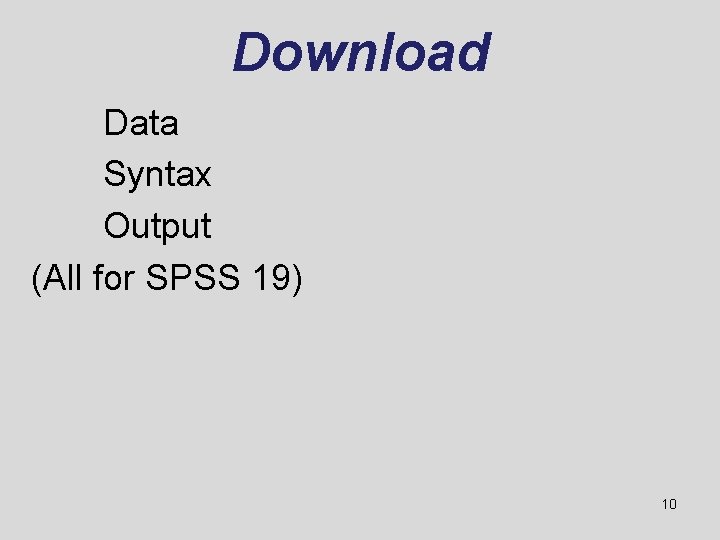
Download Data Syntax Output (All for SPSS 19) 10
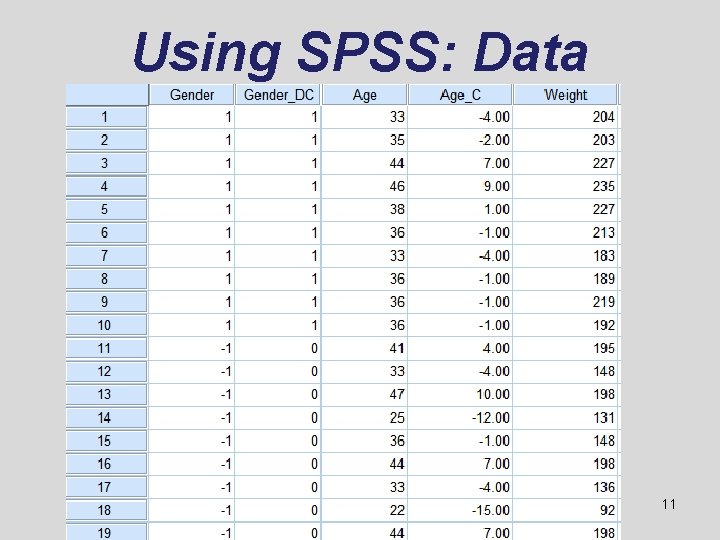
Using SPSS: Data 11
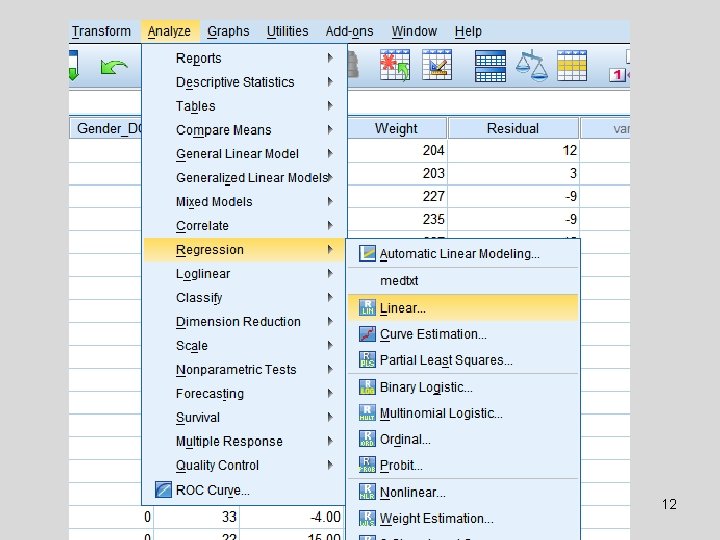
12
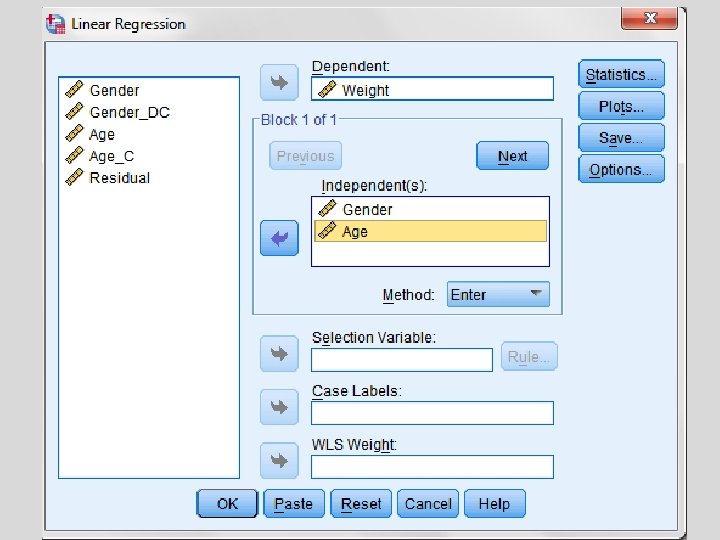
13
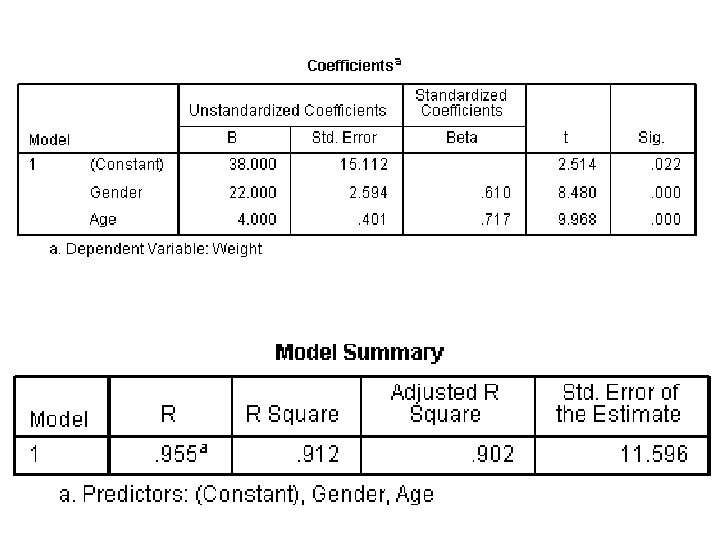
14
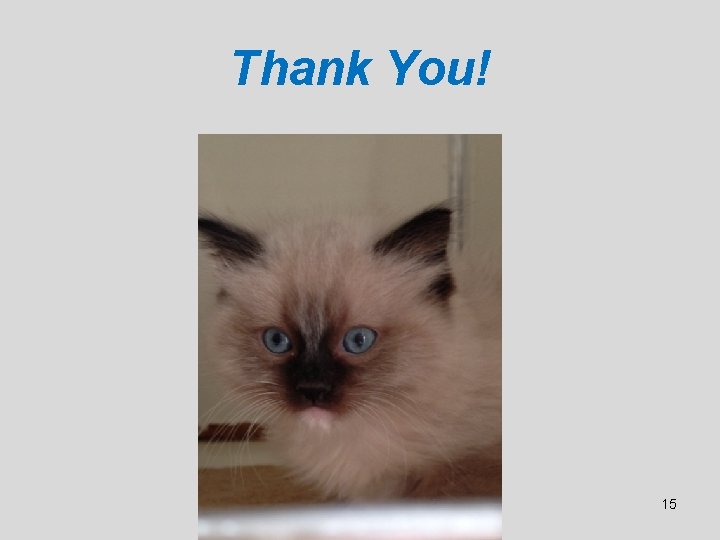
Thank You! 15
Simple and multiple linear regression
Linear model regression
David kenny mediation
David kenny sem
Srmr
Sem model fit
Moderation assumptions
David kenny sem
Survival analysis vs logistic regression
Logistic regression vs linear regression
Multiple linear regression
Anova multiple regression
Extra sum of squares multiple regression
Multiple regression analysis with qualitative information
Explanatory variable definition
Dataset for regression analysis