Knowlywood Mining Activity Knowledge from Hollywood Narratives Niket
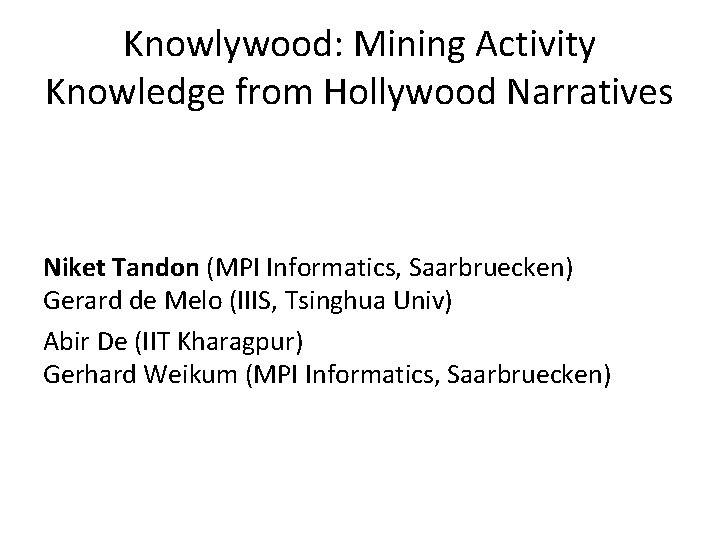
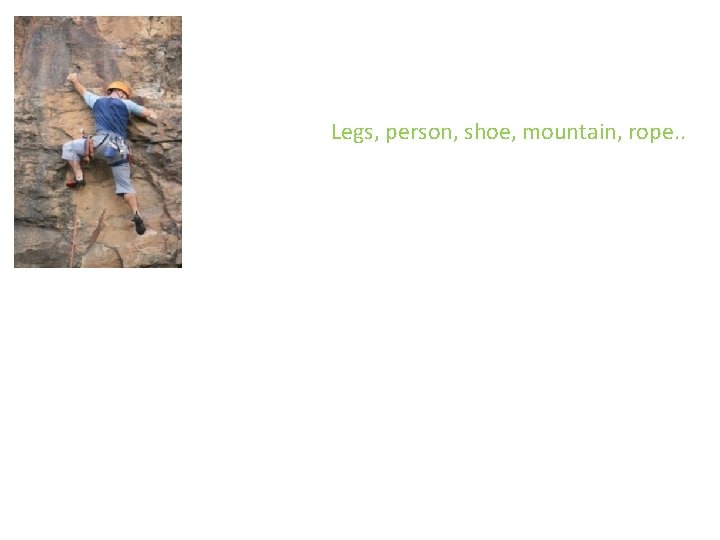
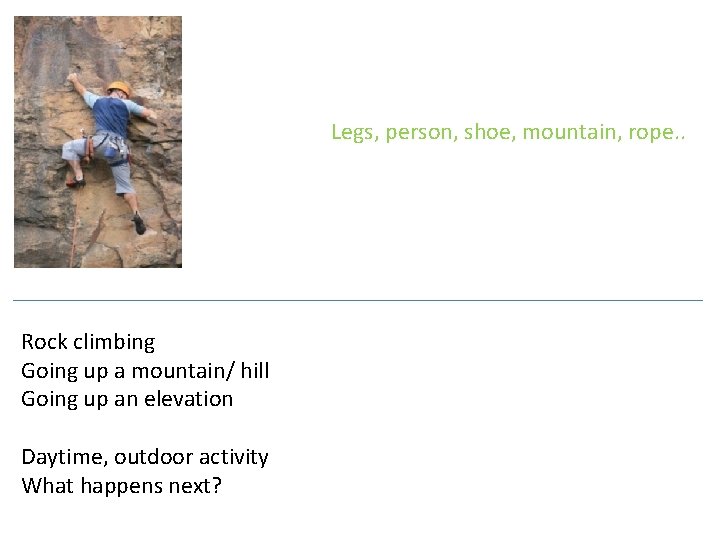
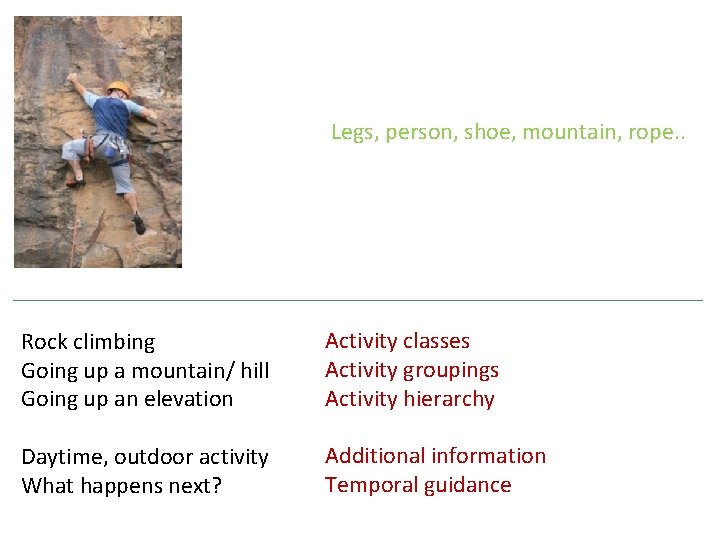
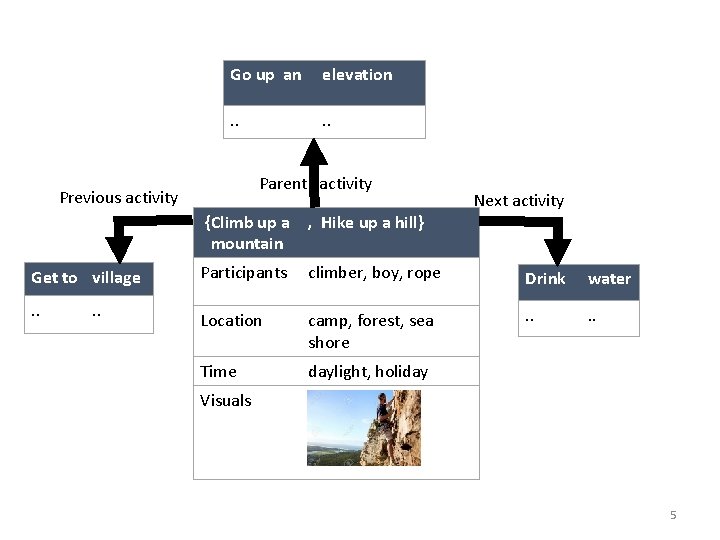
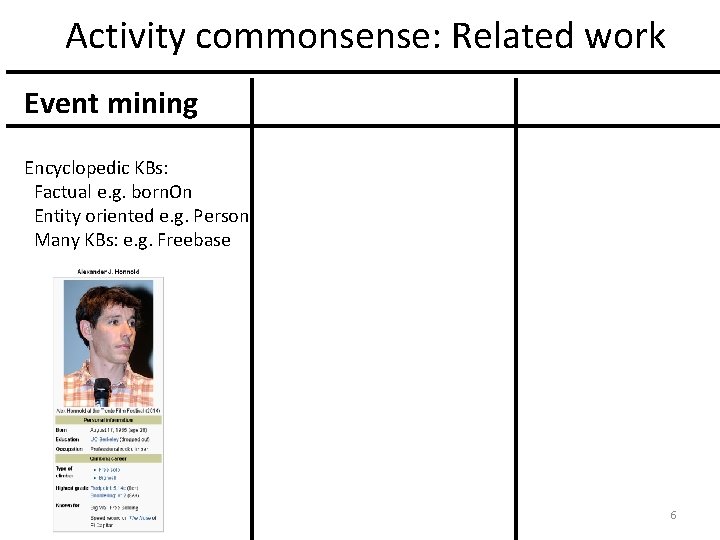
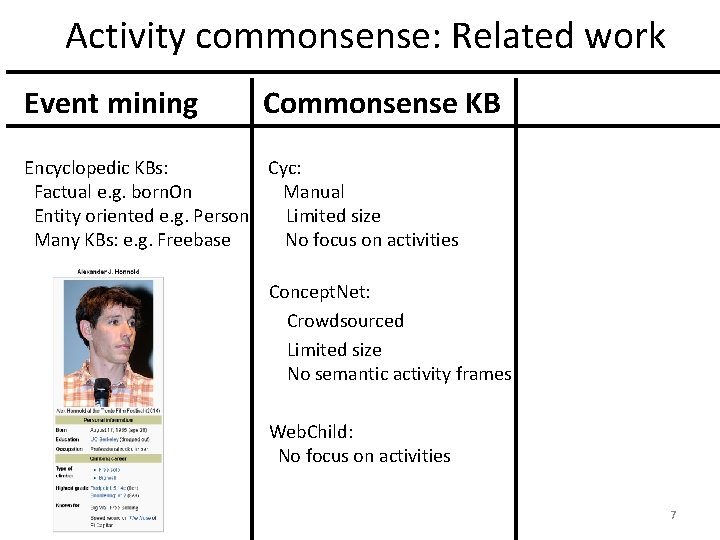
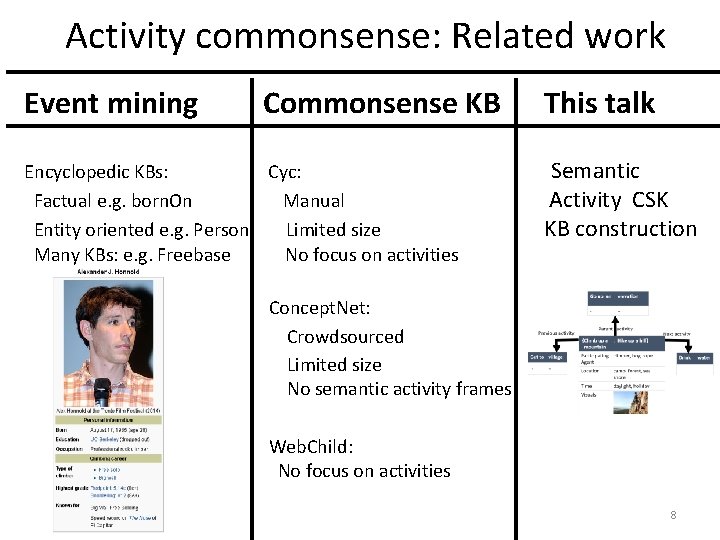
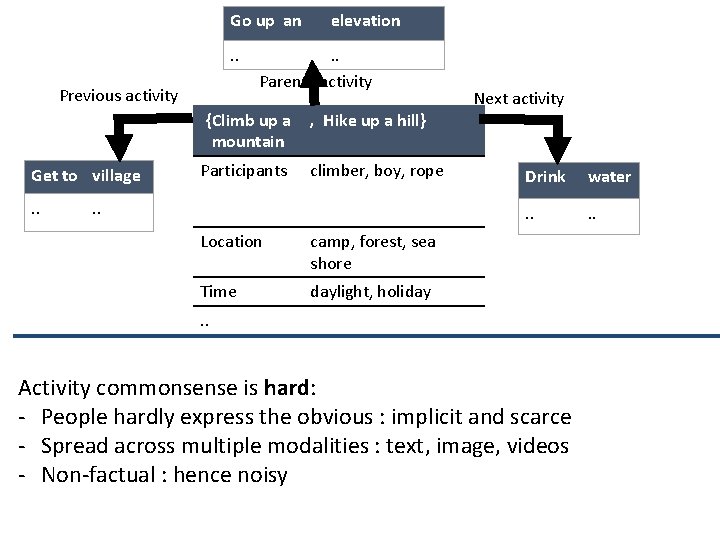
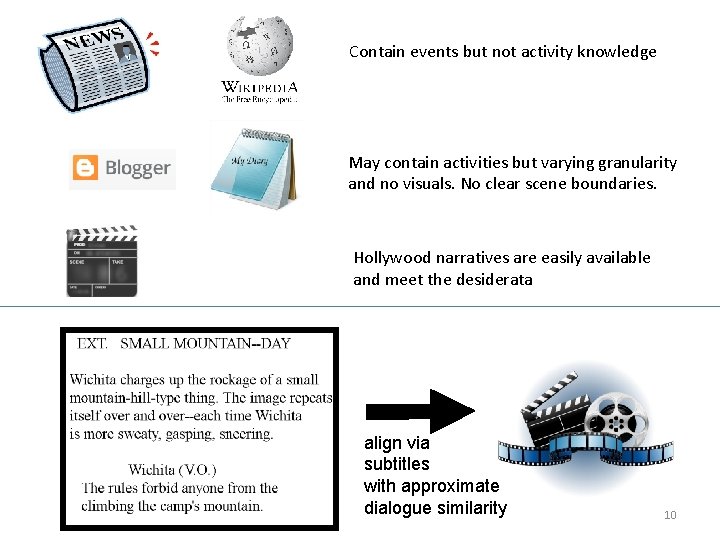
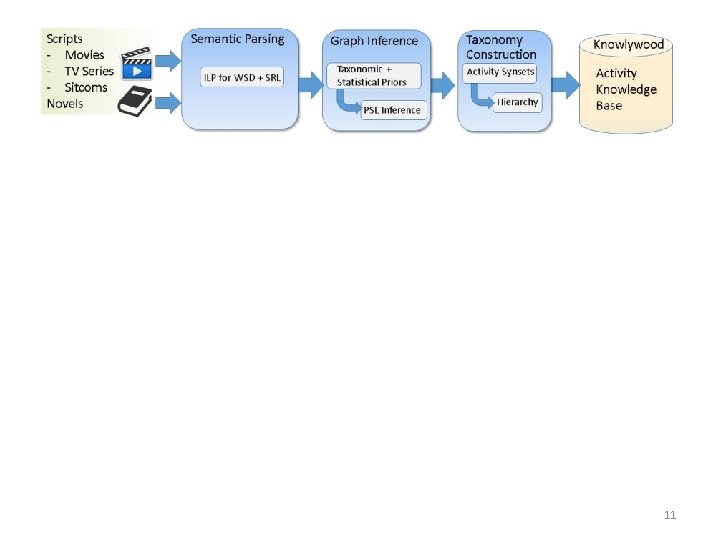
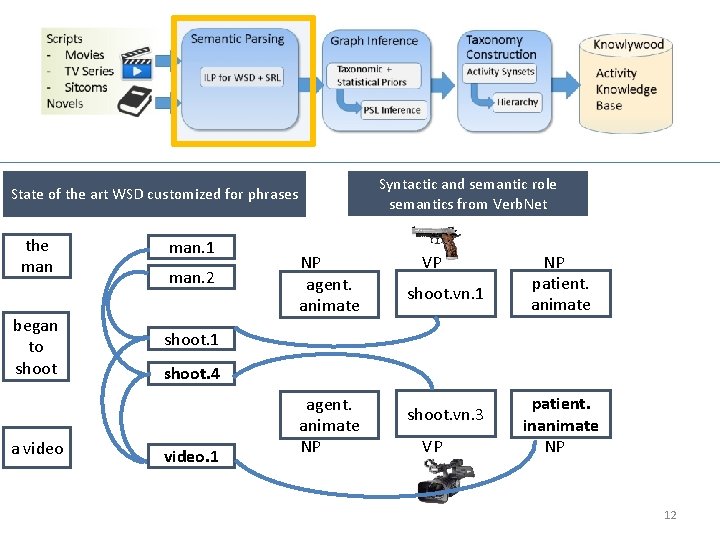
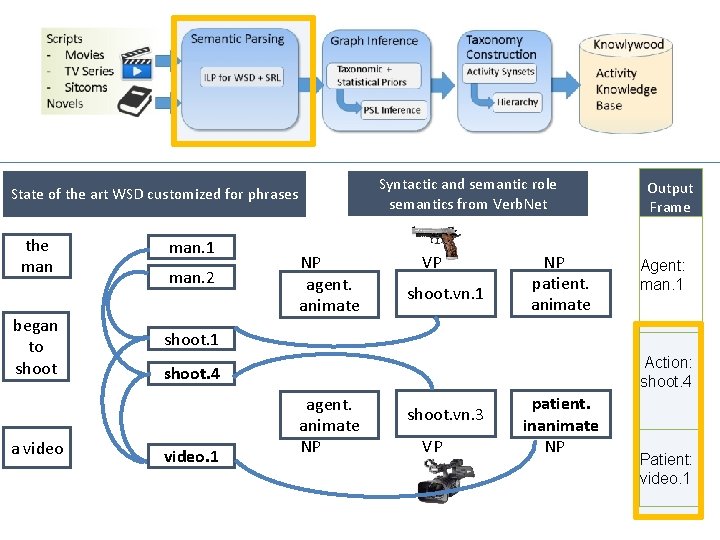
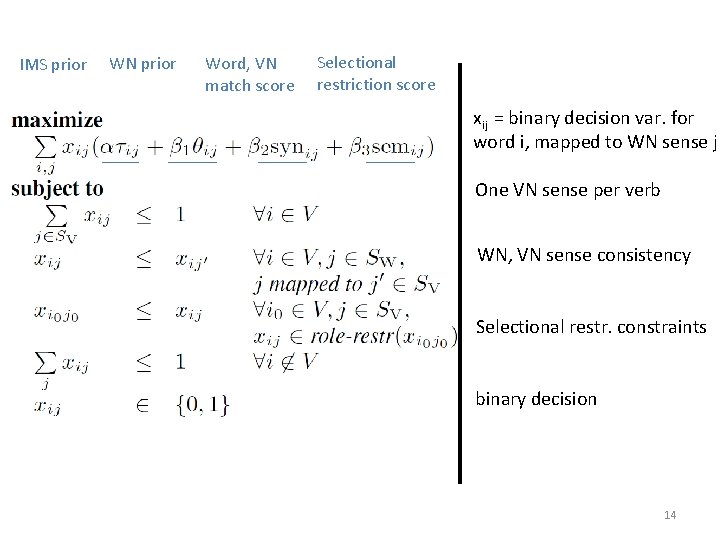
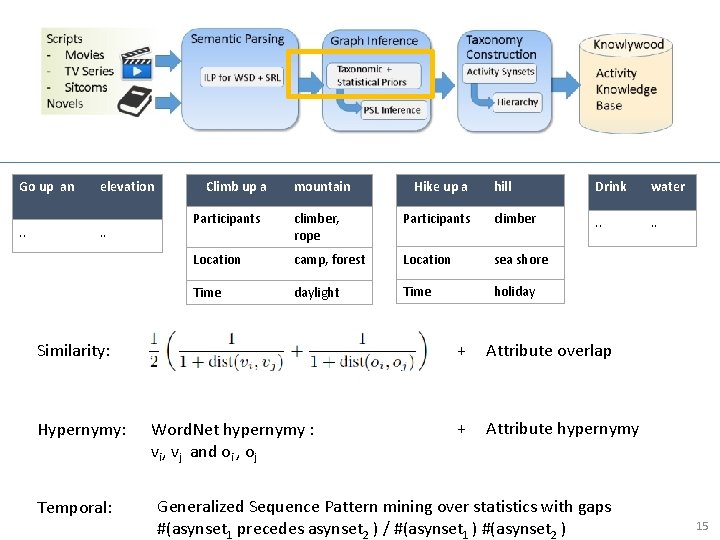
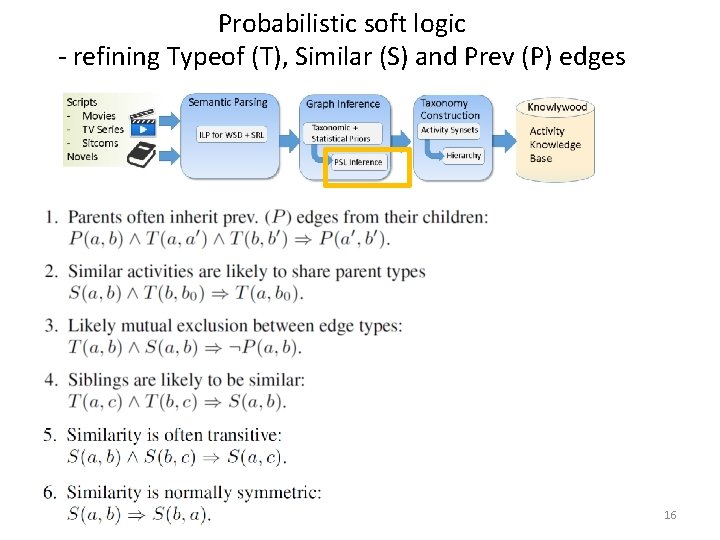
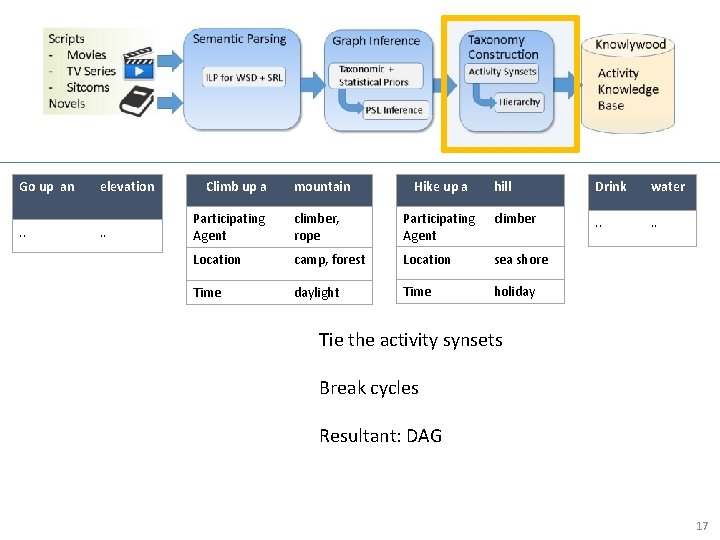
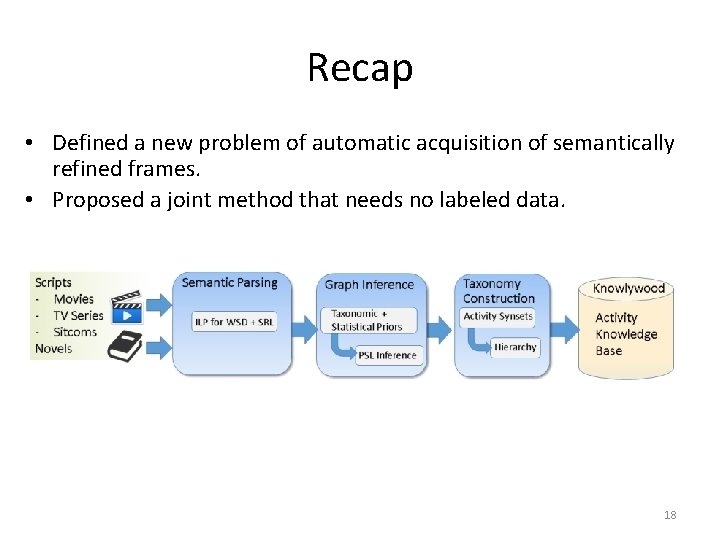
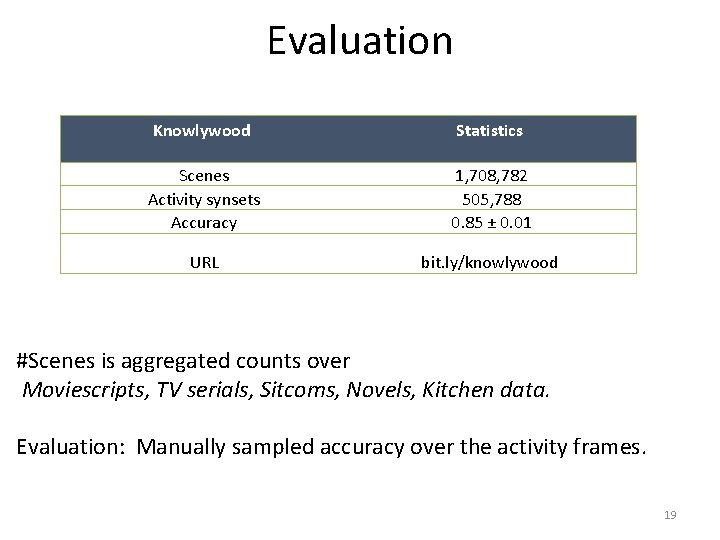
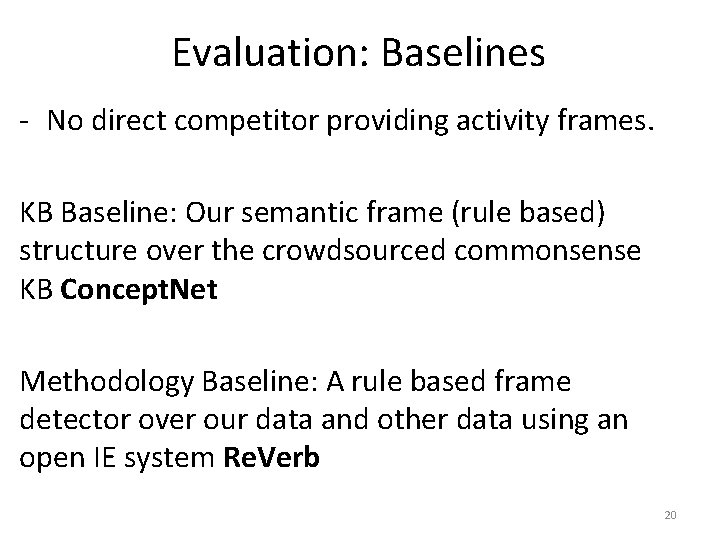
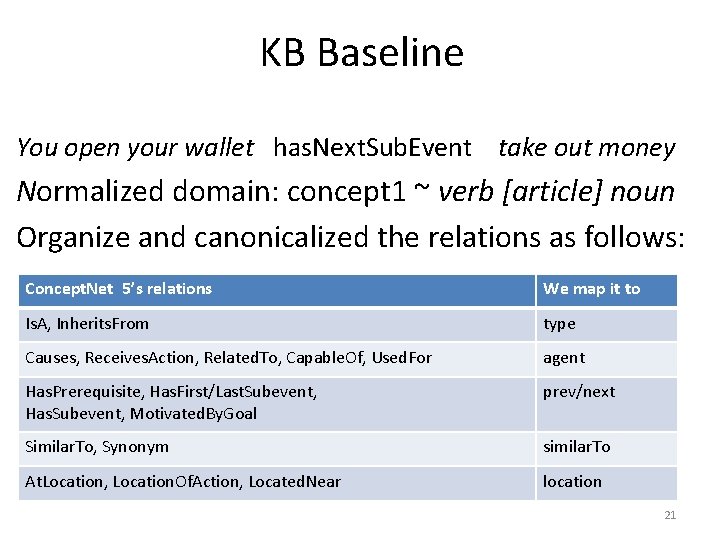
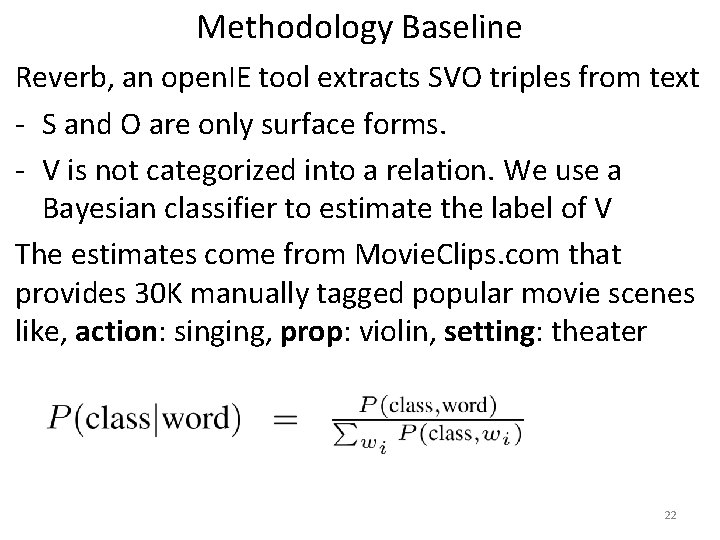
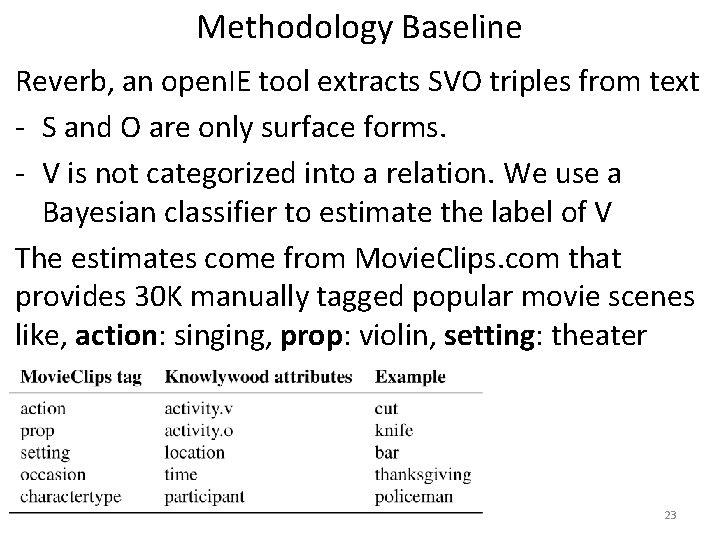
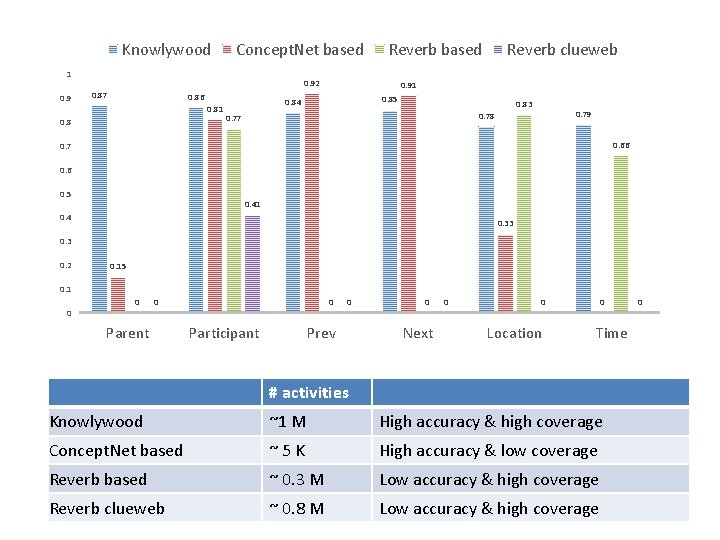
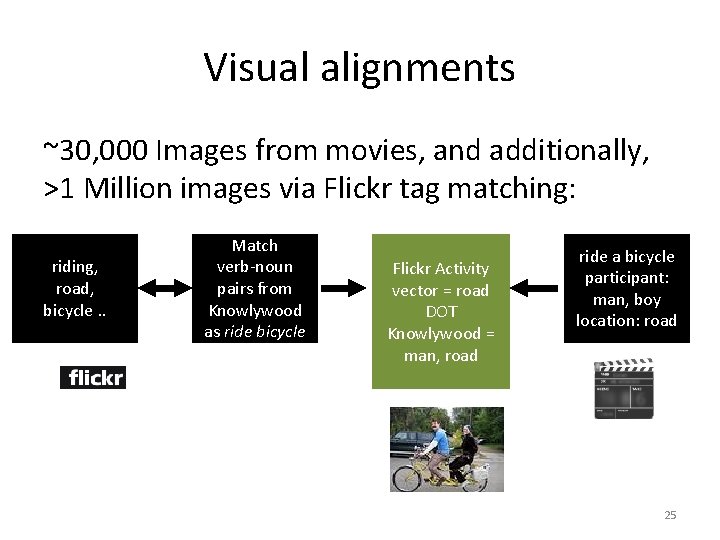
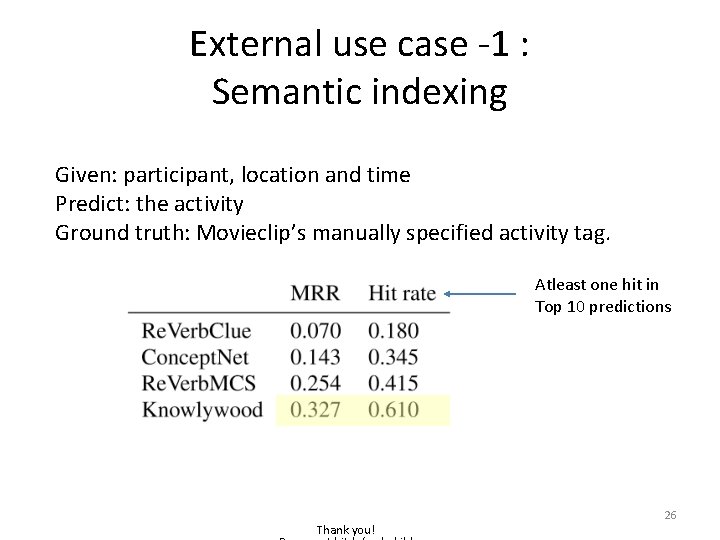
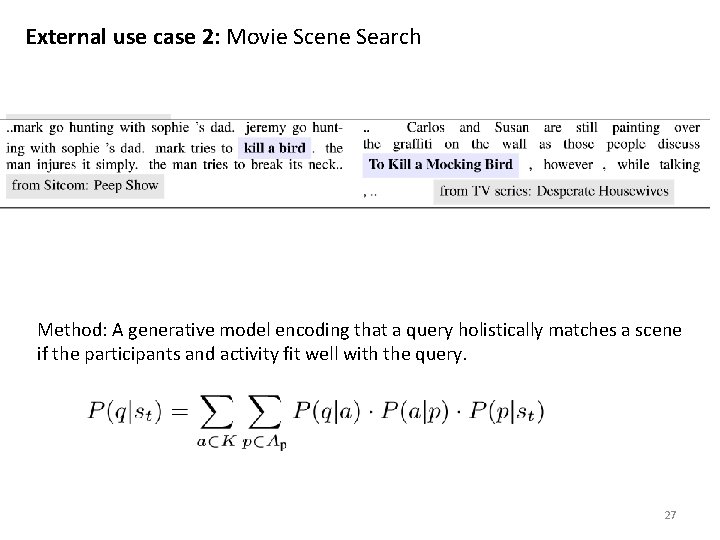
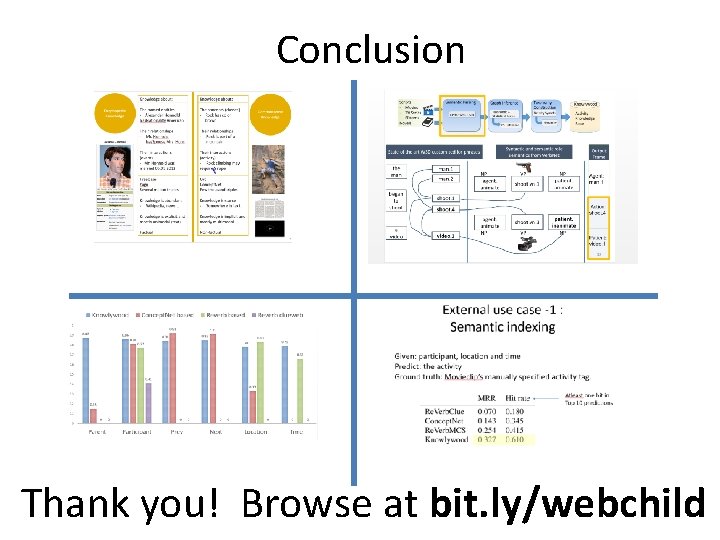
- Slides: 28
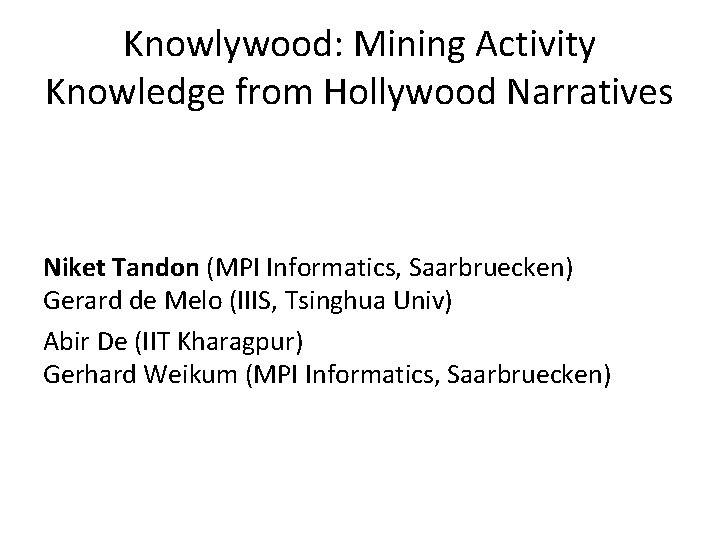
Knowlywood: Mining Activity Knowledge from Hollywood Narratives Niket Tandon (MPI Informatics, Saarbruecken) Gerard de Melo (IIIS, Tsinghua Univ) Abir De (IIT Kharagpur) Gerhard Weikum (MPI Informatics, Saarbruecken)
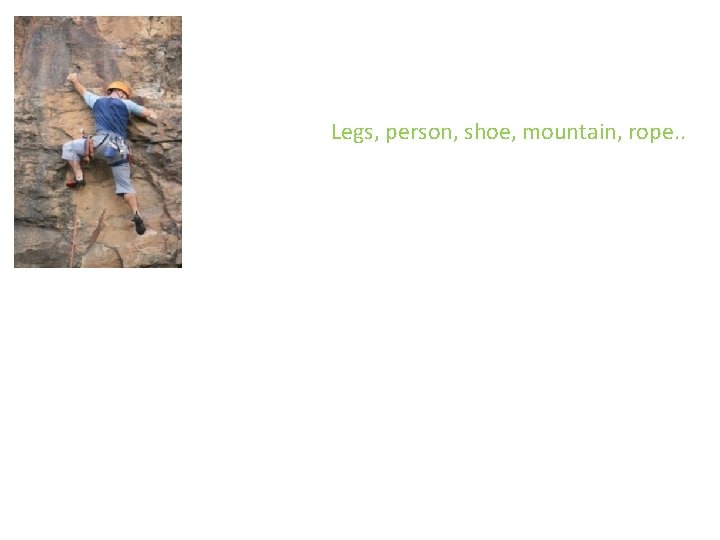
Legs, person, shoe, mountain, rope. .
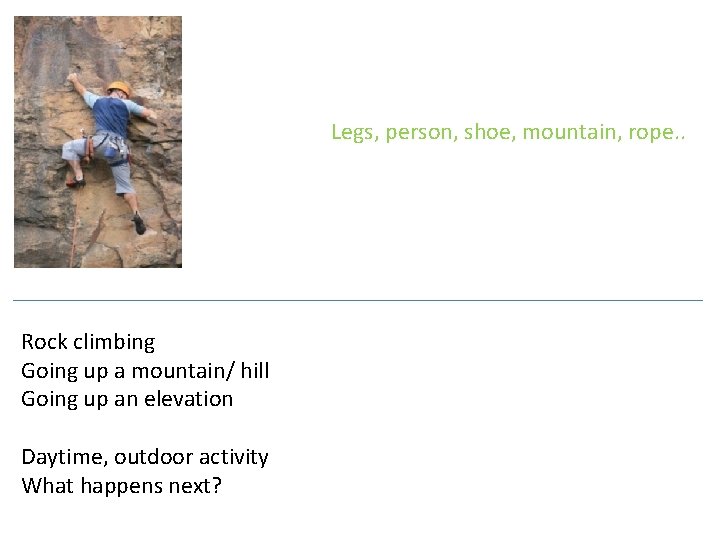
Legs, person, shoe, mountain, rope. . Rock climbing Going up a mountain/ hill Going up an elevation Daytime, outdoor activity What happens next?
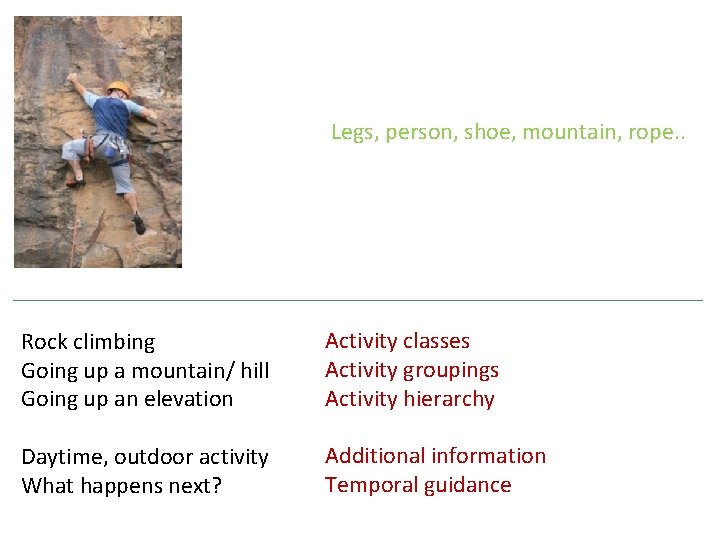
Legs, person, shoe, mountain, rope. . Rock climbing Going up a mountain/ hill Going up an elevation Activity classes Activity groupings Activity hierarchy Daytime, outdoor activity What happens next? Additional information Temporal guidance
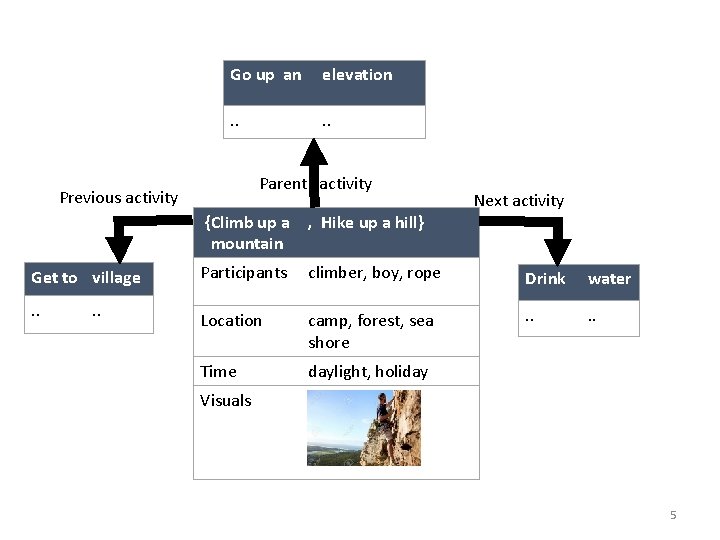
Go up an elevation . . Parent activity Previous activity Get to village. . Next activity {Climb up a mountain , Hike up a hill} Participants climber, boy, rope Drink water Location camp, forest, sea shore . . Time daylight, holiday Visuals 5
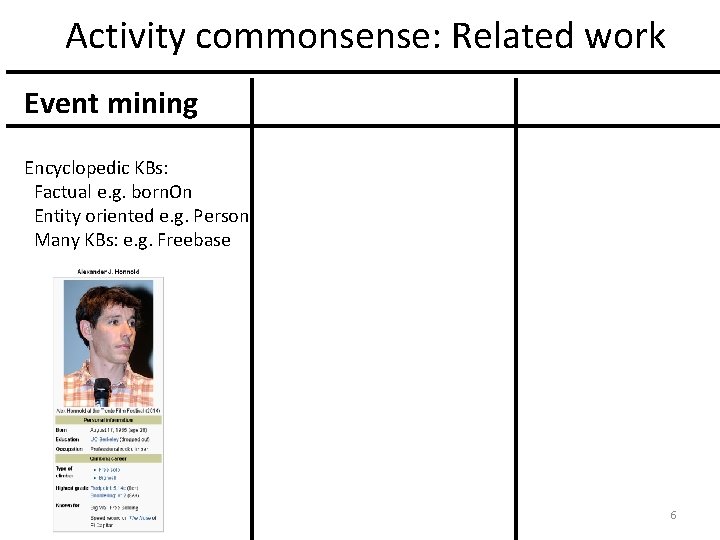
Activity commonsense: Related work Event mining Encyclopedic KBs: Factual e. g. born. On Entity oriented e. g. Person Many KBs: e. g. Freebase 6
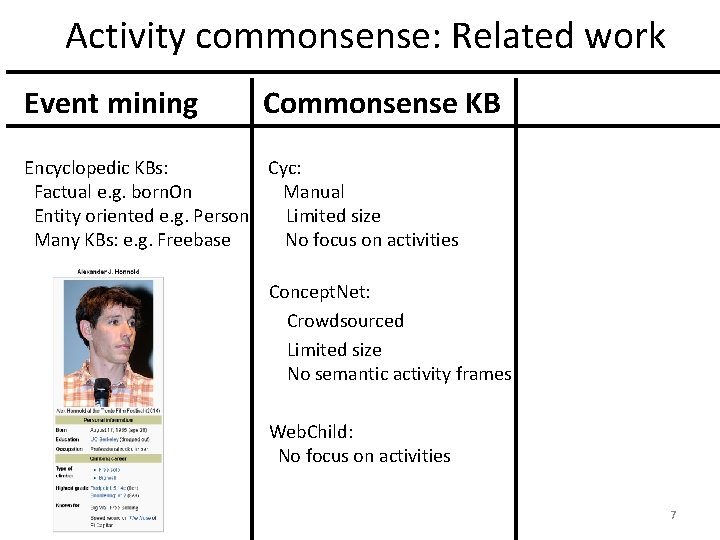
Activity commonsense: Related work Event mining Commonsense KB Encyclopedic KBs: Cyc: Factual e. g. born. On Manual Entity oriented e. g. Person Limited size Many KBs: e. g. Freebase No focus on activities Concept. Net: Crowdsourced Limited size No semantic activity frames Web. Child: No focus on activities 7
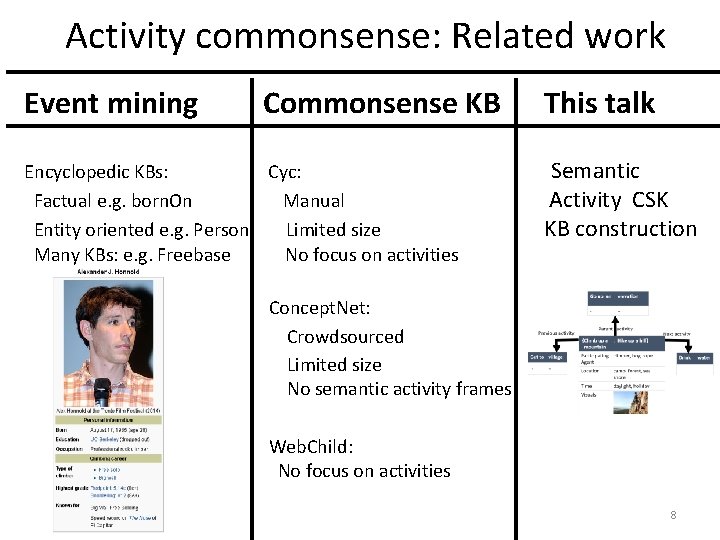
Activity commonsense: Related work Event mining Commonsense KB Encyclopedic KBs: Cyc: Factual e. g. born. On Manual Entity oriented e. g. Person Limited size Many KBs: e. g. Freebase No focus on activities This talk Semantic Activity CSK KB construction Concept. Net: Crowdsourced Limited size No semantic activity frames Web. Child: No focus on activities 8
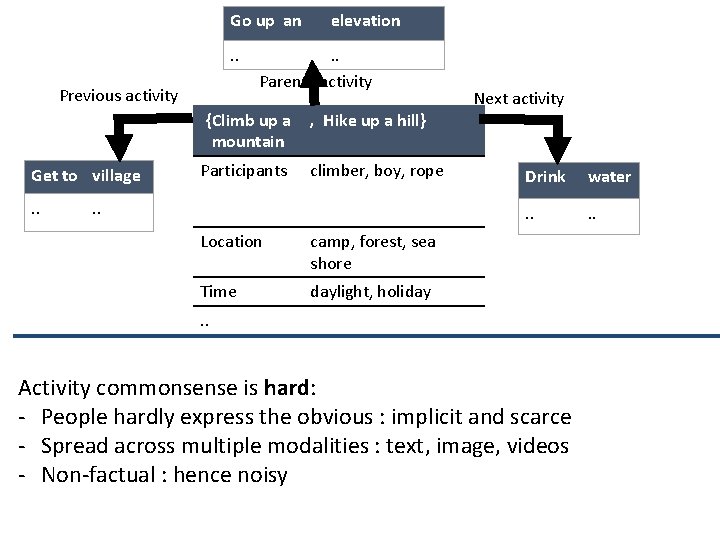
Go up an. . Previous activity Get to village. . elevation . . Parent activity {Climb up a mountain , Hike up a hill} Participants climber, boy, rope . . Location camp, forest, sea shore Time daylight, holiday Next activity Drink water . . . Activity commonsense is hard: - People hardly express the obvious : implicit and scarce - Spread across multiple modalities : text, image, videos - Non-factual : hence noisy
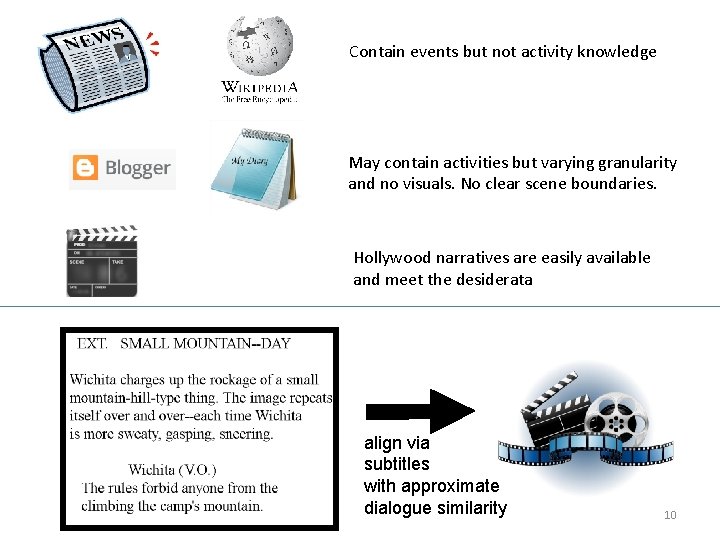
Contain events but not activity knowledge May contain activities but varying granularity and no visuals. No clear scene boundaries. Hollywood narratives are easily available and meet the desiderata align via subtitles with approximate dialogue similarity 10
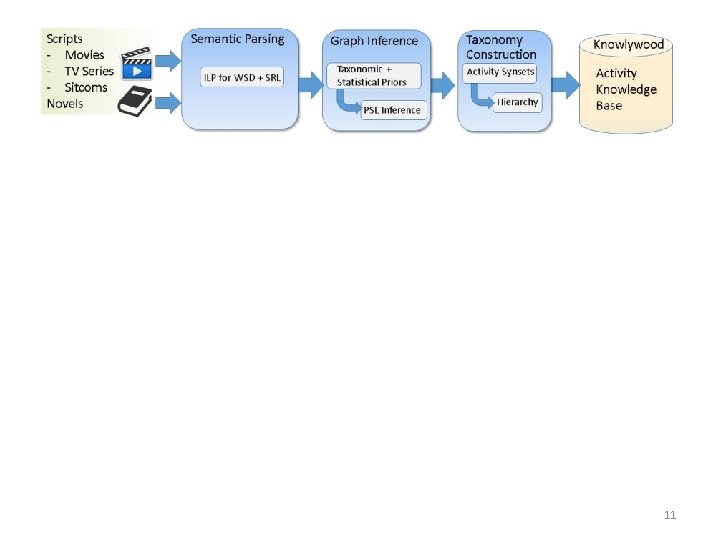
11
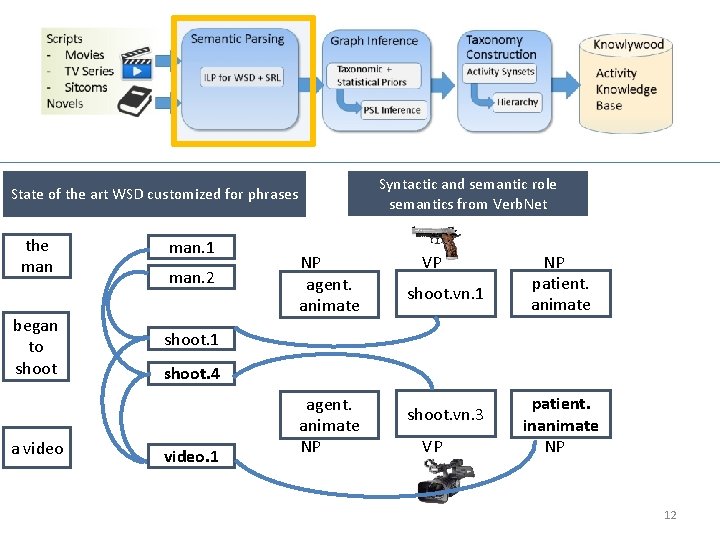
Syntactic and semantic role semantics from Verb. Net State of the art WSD customized for phrases the man began to shoot a video man. 1 man. 2 NP agent. animate VP shoot. vn. 1 NP patient. animate shoot. 1 shoot. 4 video. 1 agent. animate NP shoot. vn. 3 VP patient. inanimate NP 12
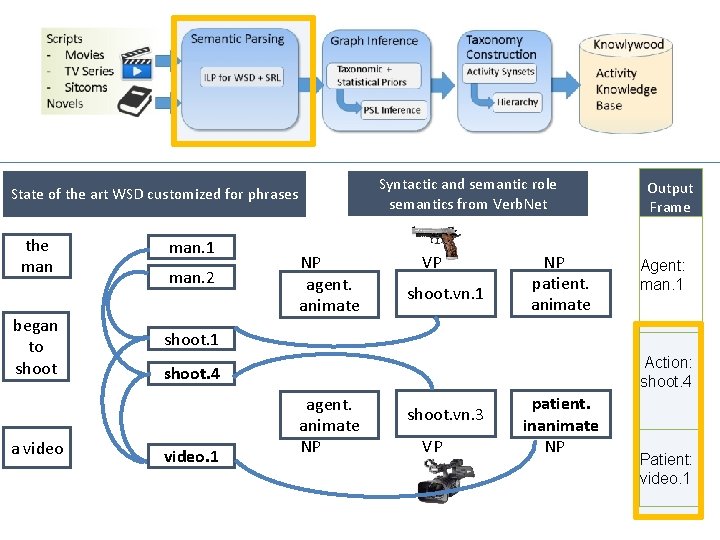
Syntactic and semantic role semantics from Verb. Net State of the art WSD customized for phrases the man began to shoot a video man. 1 man. 2 NP agent. animate VP shoot. vn. 1 NP patient. animate Output Frame Agent: man. 1 shoot. 1 Action: shoot. 4 video. 1 agent. animate NP shoot. vn. 3 VP patient. inanimate NP Patient: video. 1
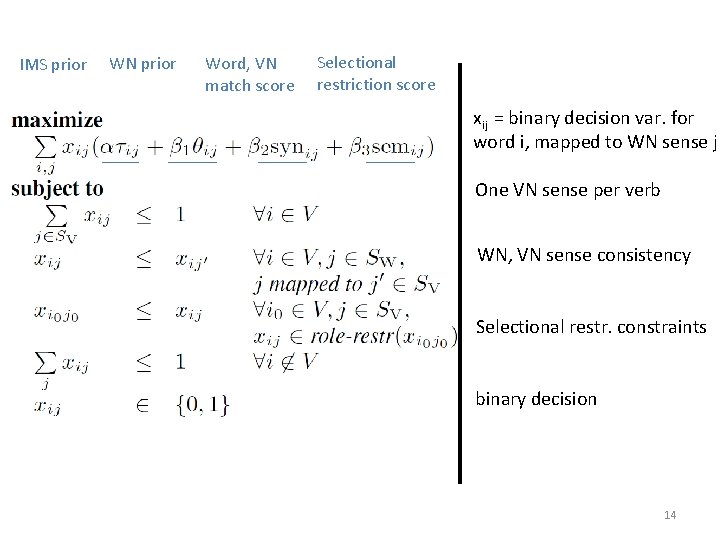
IMS prior WN prior Word, VN match score Selectional restriction score xij = binary decision var. for word i, mapped to WN sense j One VN sense per verb WN, VN sense consistency Selectional restr. constraints binary decision 14
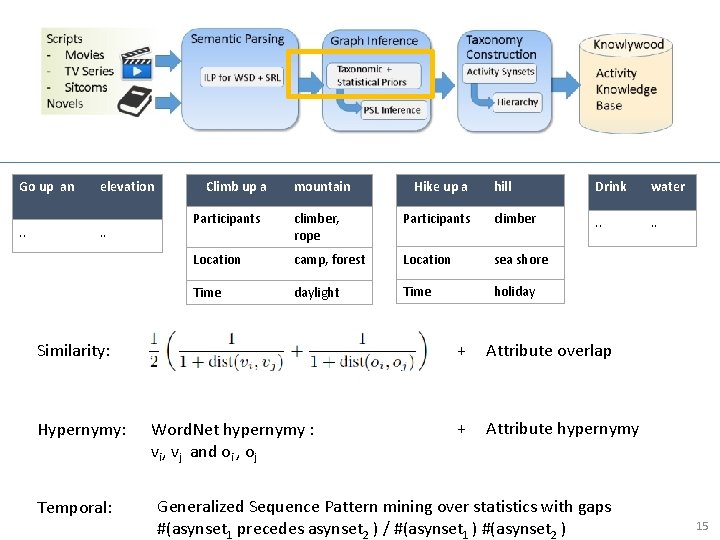
Go up an. . elevation. . Climb up a mountain Temporal: hill Drink water . . Participants climber, rope Participants climber Location camp, forest Location sea shore Time daylight Time holiday Similarity: Hypernymy: Hike up a Word. Net hypernymy : vi, vj and oi , oj + Attribute overlap + Attribute hypernymy Generalized Sequence Pattern mining over statistics with gaps #(asynset 1 precedes asynset 2 ) / #(asynset 1 ) #(asynset 2 ) 15
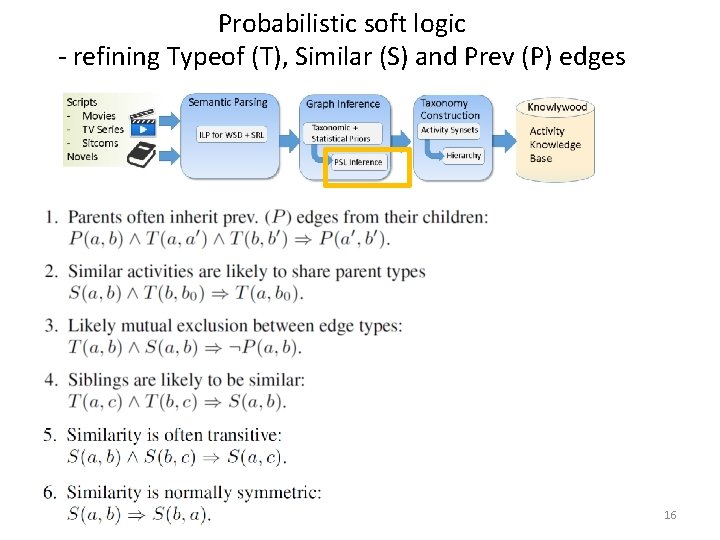
Probabilistic soft logic - refining Typeof (T), Similar (S) and Prev (P) edges 16
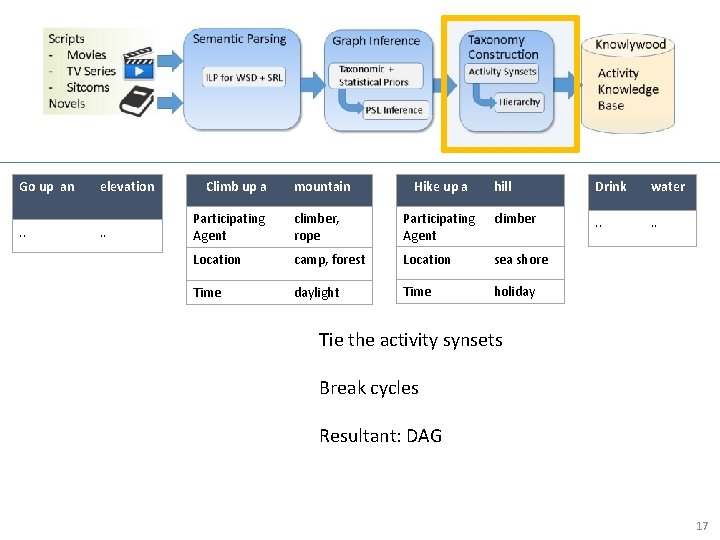
Go up an. . elevation. . Climb up a mountain Hike up a hill Drink water . . Participating Agent climber, rope Participating Agent climber Location camp, forest Location sea shore Time daylight Time holiday Tie the activity synsets Break cycles Resultant: DAG 17
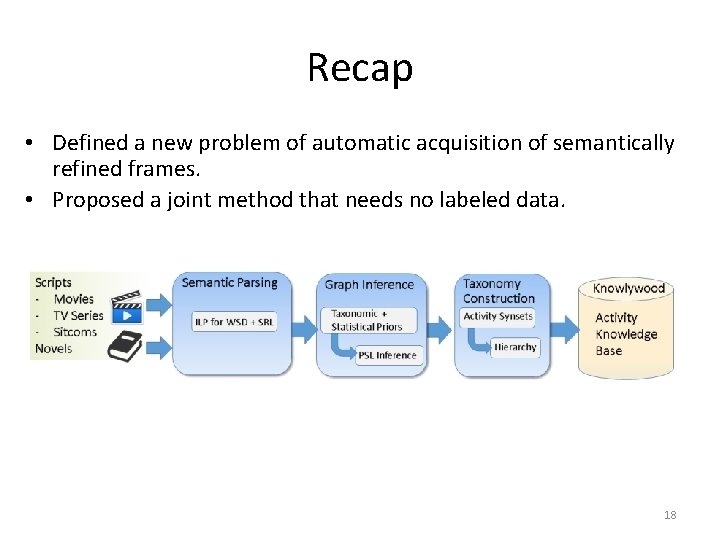
Recap • Defined a new problem of automatic acquisition of semantically refined frames. • Proposed a joint method that needs no labeled data. 18
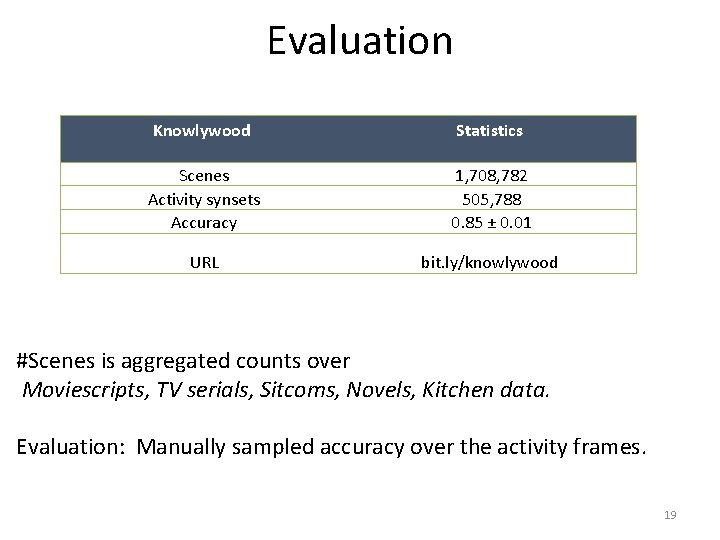
Evaluation Knowlywood Statistics Scenes Activity synsets Accuracy 1, 708, 782 505, 788 0. 85 ± 0. 01 URL bit. ly/knowlywood #Scenes is aggregated counts over Moviescripts, TV serials, Sitcoms, Novels, Kitchen data. Evaluation: Manually sampled accuracy over the activity frames. 19
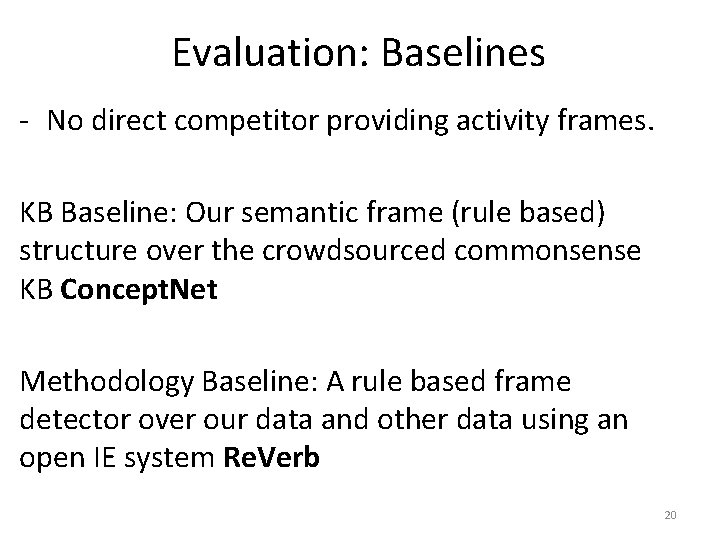
Evaluation: Baselines - No direct competitor providing activity frames. KB Baseline: Our semantic frame (rule based) structure over the crowdsourced commonsense KB Concept. Net Methodology Baseline: A rule based frame detector over our data and other data using an open IE system Re. Verb 20
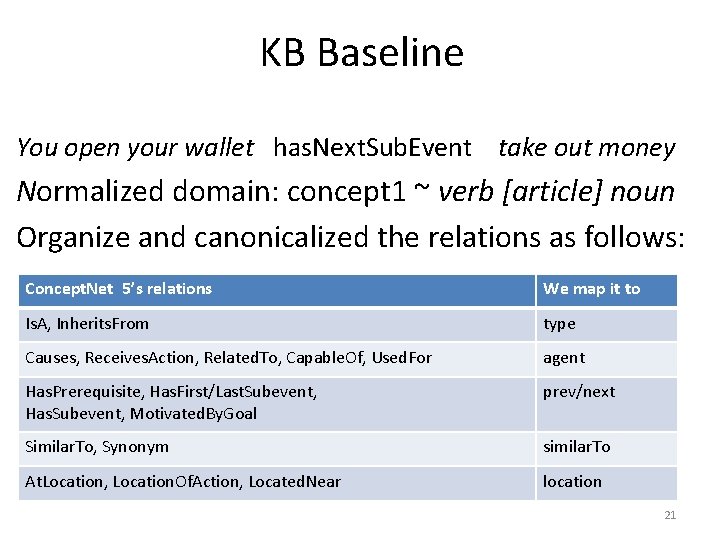
KB Baseline You open your wallet has. Next. Sub. Event take out money Normalized domain: concept 1 ~ verb [article] noun Organize and canonicalized the relations as follows: Concept. Net 5’s relations We map it to Is. A, Inherits. From type Causes, Receives. Action, Related. To, Capable. Of, Used. For agent Has. Prerequisite, Has. First/Last. Subevent, Has. Subevent, Motivated. By. Goal prev/next Similar. To, Synonym similar. To At. Location, Location. Of. Action, Located. Near location 21
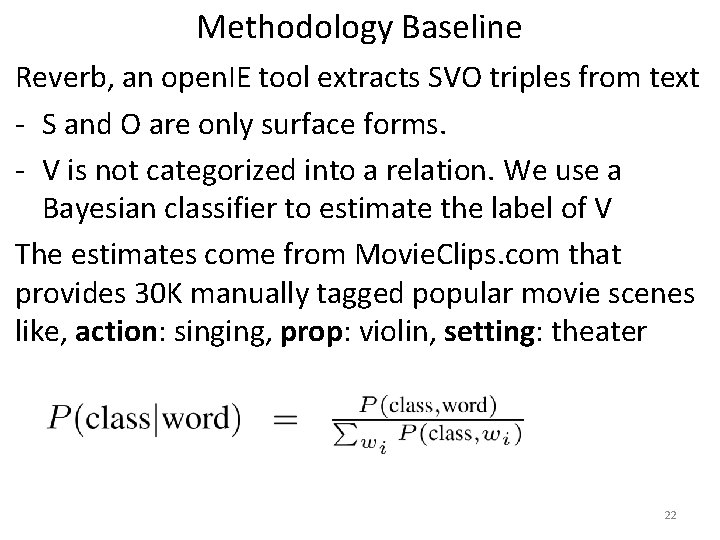
Methodology Baseline Reverb, an open. IE tool extracts SVO triples from text - S and O are only surface forms. - V is not categorized into a relation. We use a Bayesian classifier to estimate the label of V The estimates come from Movie. Clips. com that provides 30 K manually tagged popular movie scenes like, action: singing, prop: violin, setting: theater 22
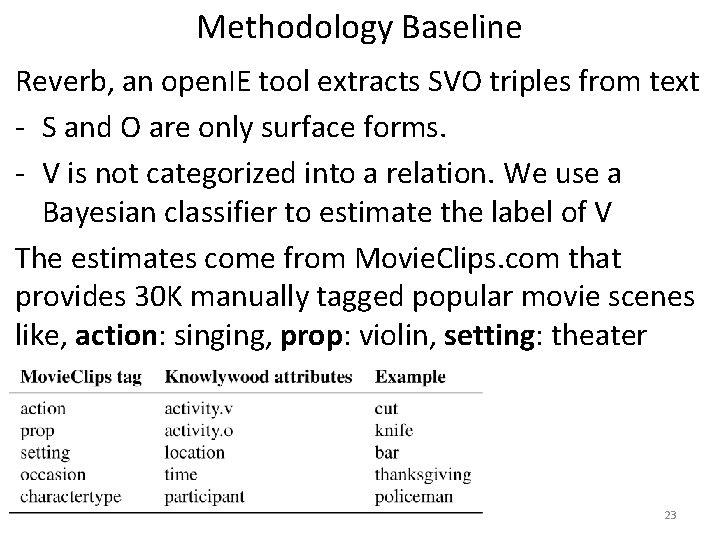
Methodology Baseline Reverb, an open. IE tool extracts SVO triples from text - S and O are only surface forms. - V is not categorized into a relation. We use a Bayesian classifier to estimate the label of V The estimates come from Movie. Clips. com that provides 30 K manually tagged popular movie scenes like, action: singing, prop: violin, setting: theater 23
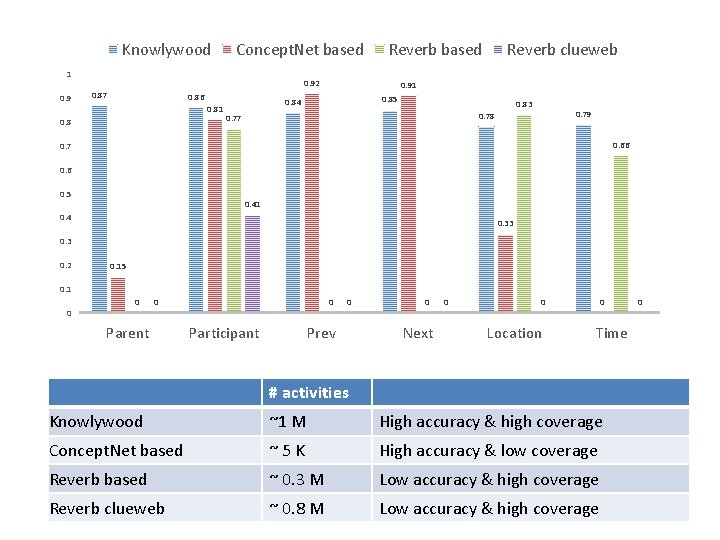
Knowlywood Concept. Net based 1 0. 9 Reverb based 0. 92 0. 87 0. 86 0. 81 0. 8 Reverb clueweb 0. 91 0. 85 0. 84 0. 83 0. 79 0. 78 0. 77 0. 66 0. 7 0. 6 0. 5 0. 41 0. 4 0. 33 0. 2 0. 15 0. 1 0 0 0 Parent 0 Participant 0 Prev 0 Next 0 0 Location 0 0 Time # activities Knowlywood ~1 M High accuracy & high coverage Concept. Net based ~5 K High accuracy & low coverage Reverb based ~ 0. 3 M Low accuracy & high coverage Reverb clueweb ~ 0. 8 M Low accuracy & high coverage 24
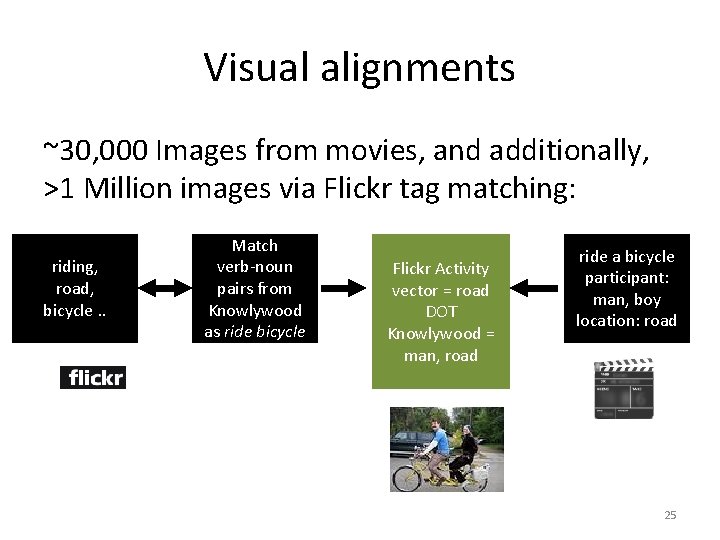
Visual alignments ~30, 000 Images from movies, and additionally, >1 Million images via Flickr tag matching: riding, road, bicycle. . Match verb-noun pairs from Knowlywood as ride bicycle Flickr Activity vector = road DOT Knowlywood = man, road ride a bicycle participant: man, boy location: road 25
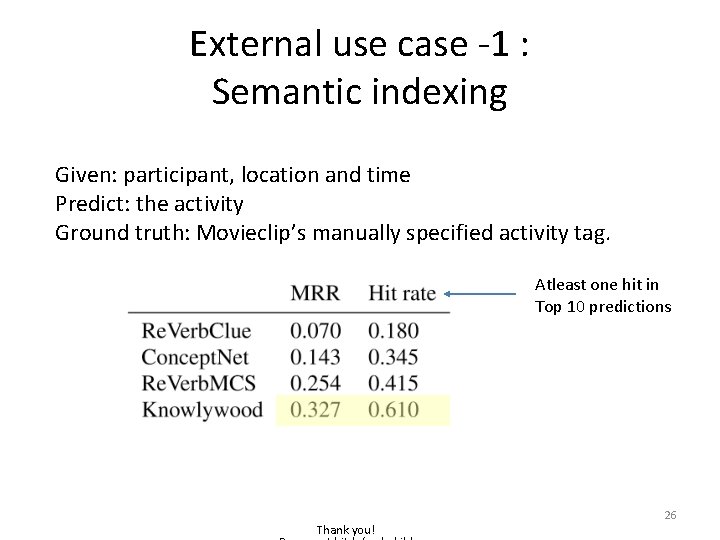
External use case -1 : Semantic indexing Given: participant, location and time Predict: the activity Ground truth: Movieclip’s manually specified activity tag. Atleast one hit in Top 10 predictions Thank you! 26
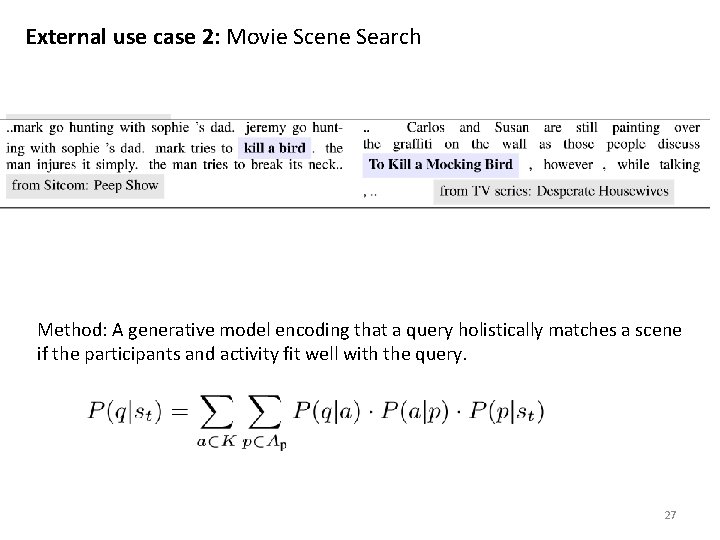
External use case 2: Movie Scene Search Method: A generative model encoding that a query holistically matches a scene if the participants and activity fit well with the query. 27
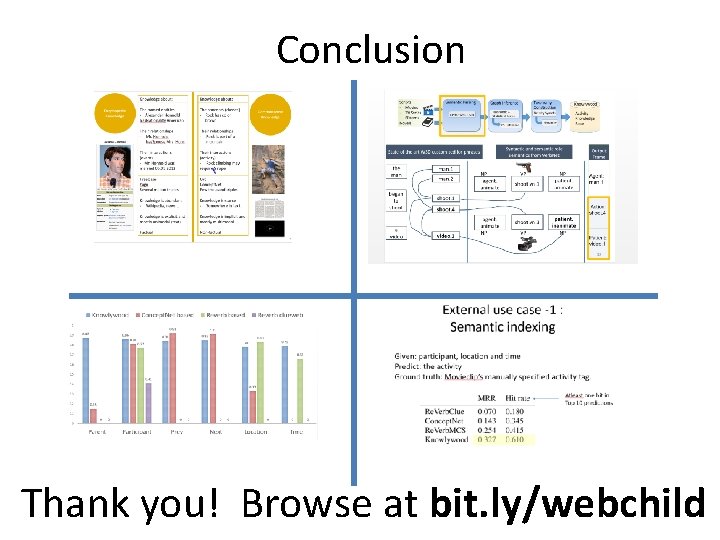
Conclusion Thank you! Browse at bit. ly/webchild
Difference between strip mining and open pit mining
Web text mining
Strip mining vs open pit mining
Mining multimedia databases
Strip mining vs open pit mining
Mining complex types of data
Features of narrative paragraph
Gre exam
Primeval narratives
Postmodern theory
Kyrene educational resources
Oral narratives
What is oral literature?
How to write a dialogue
Postmodernism definition
What is the nature of the resurrection narratives
Conclusions for personal narratives
Sondierungsfragen
The purpose of the narrative text is …
Messianic prophecies fulfilled in the infancy narratives
Narrative tenses perfect english grammar
Use case priority matrix for system
Benzedrine inhaler hollywood
Dream factory hollywood
Hollywood renaissance films
Hollywood
Hollywood africans
Hollywood 1977
Elements of classical hollywood cinema