IRI Seasonal Forecasting Update Models Run at IRI
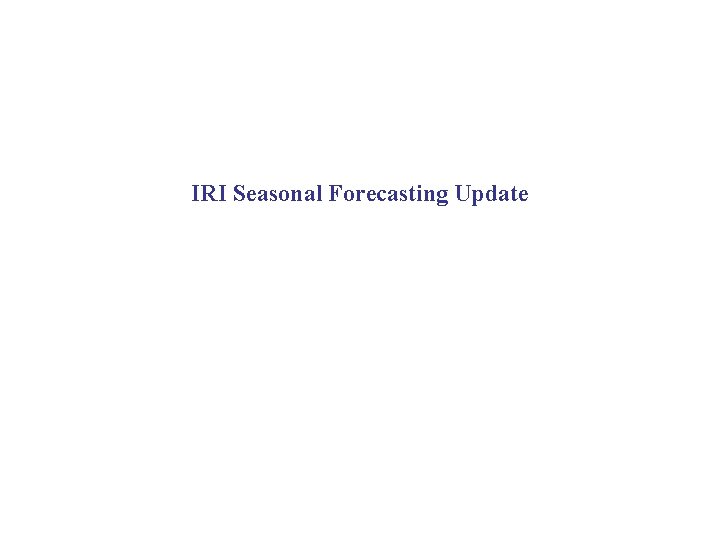
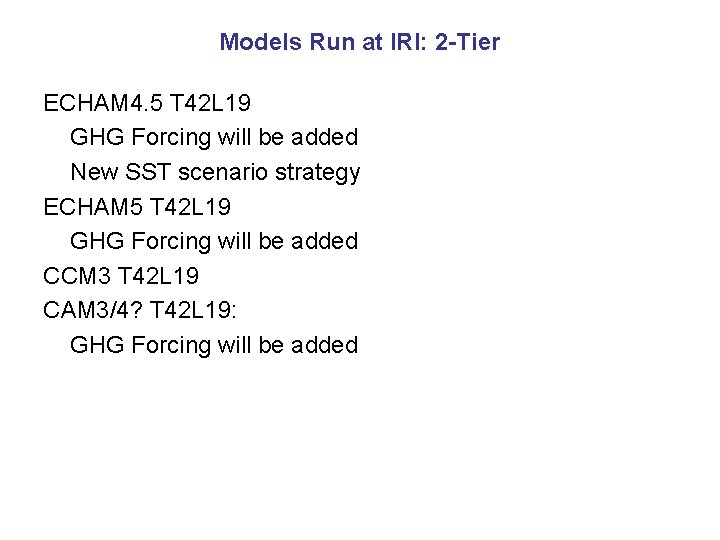
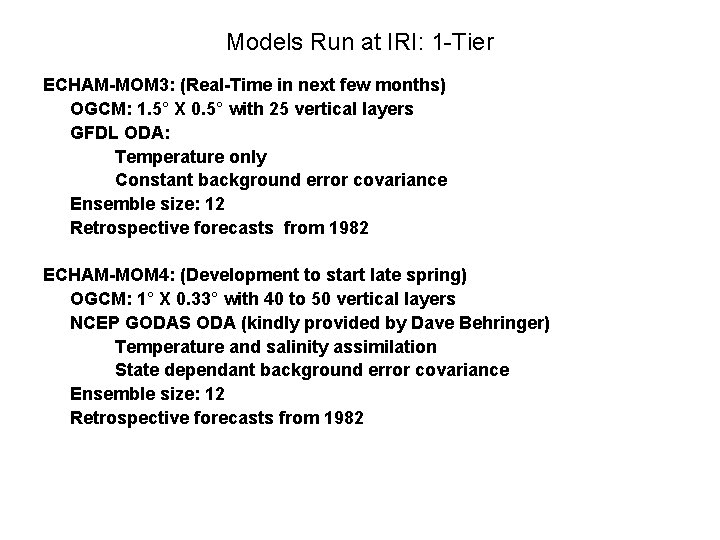
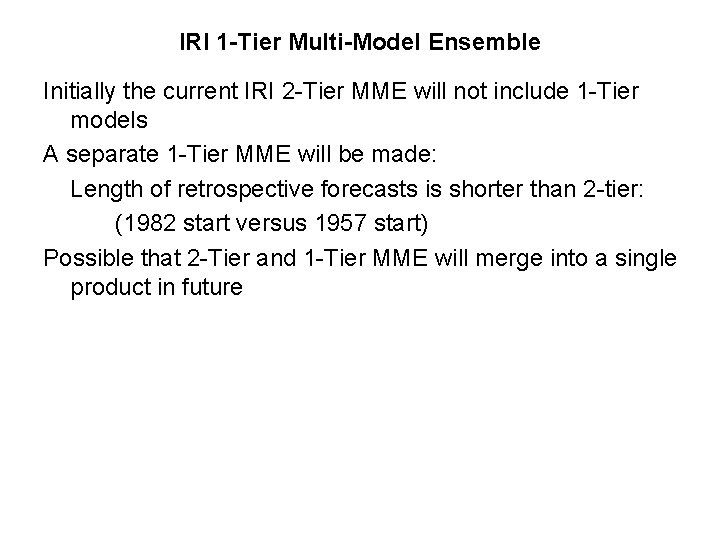
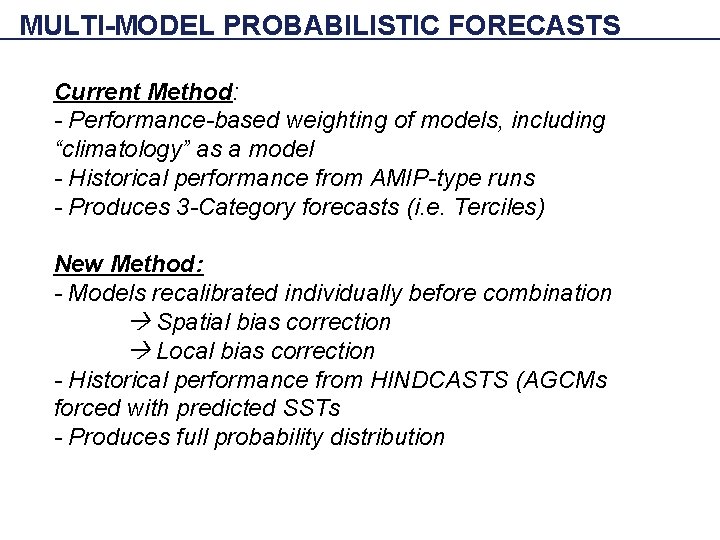
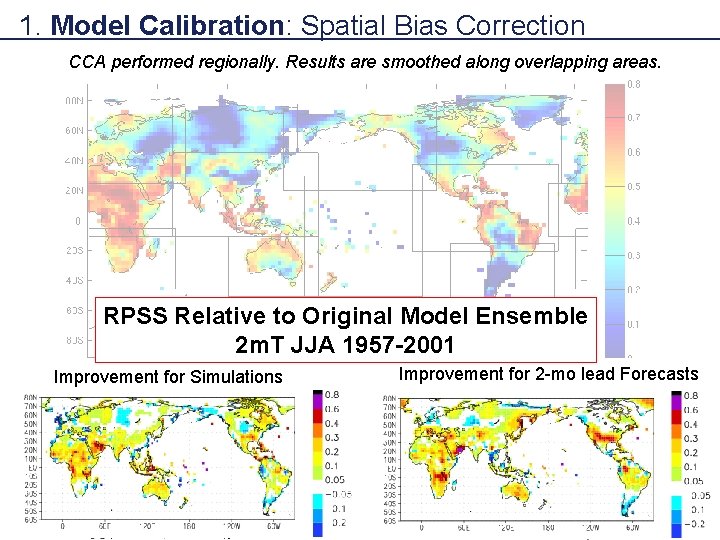
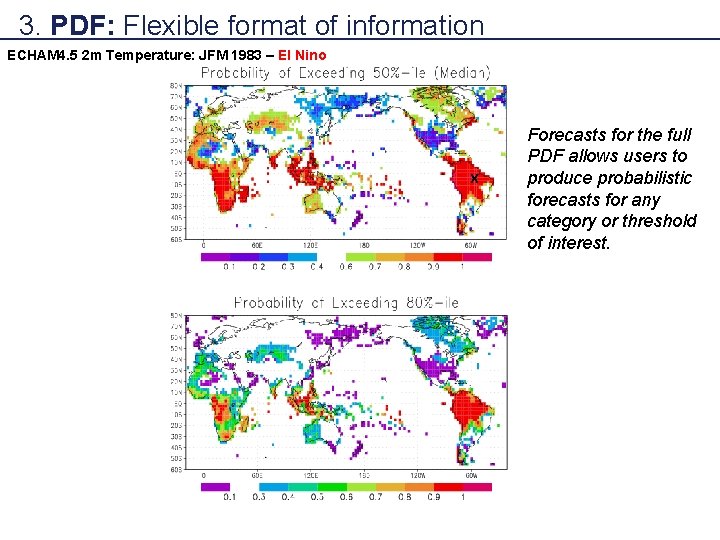
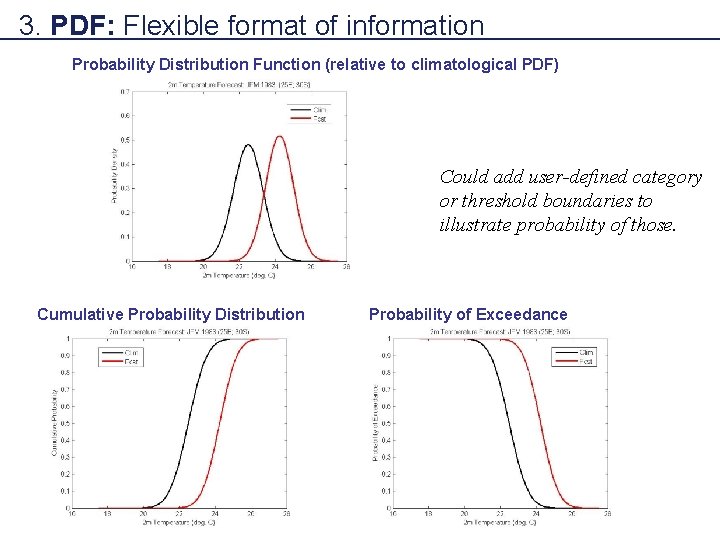
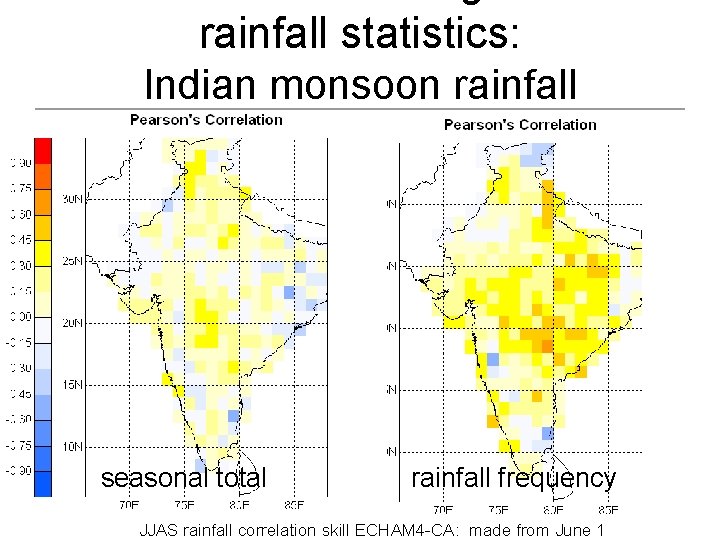
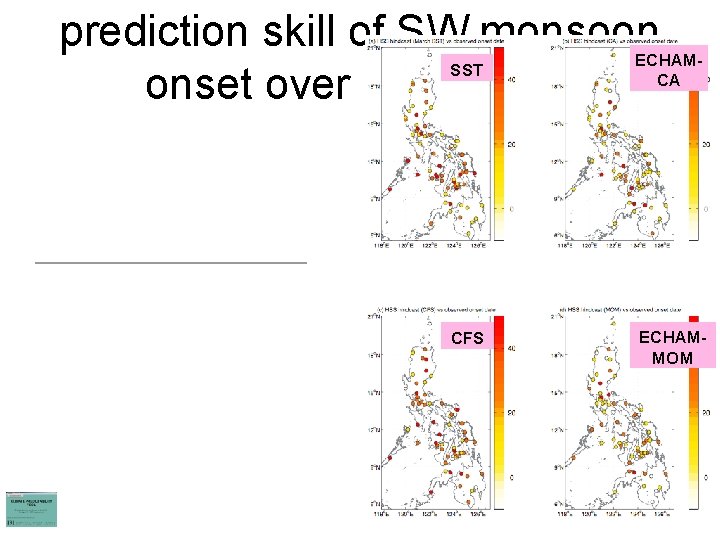
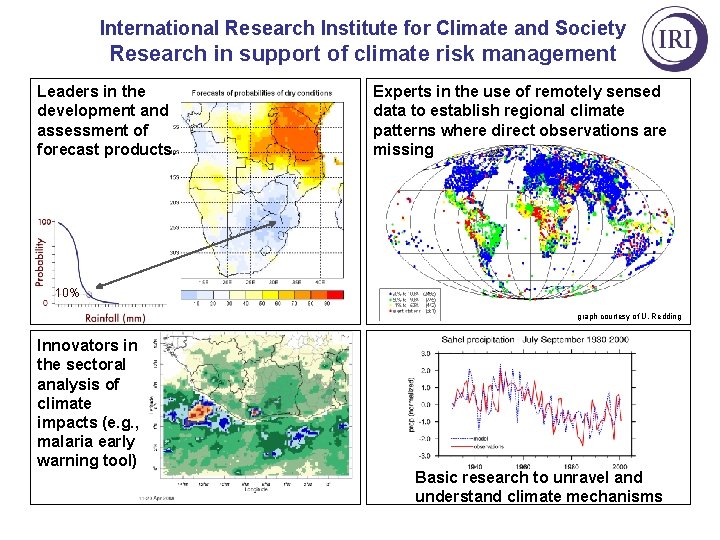
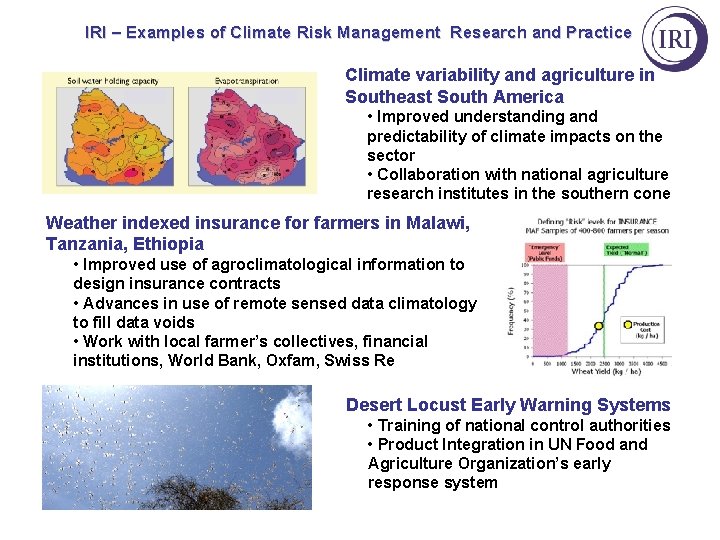
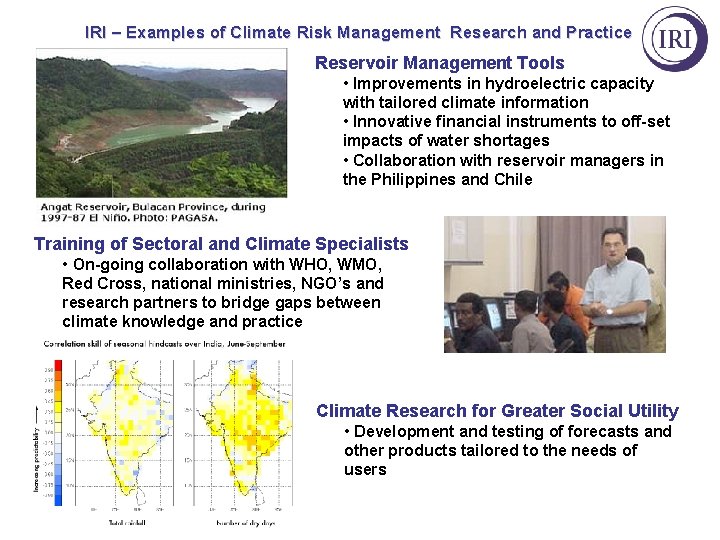
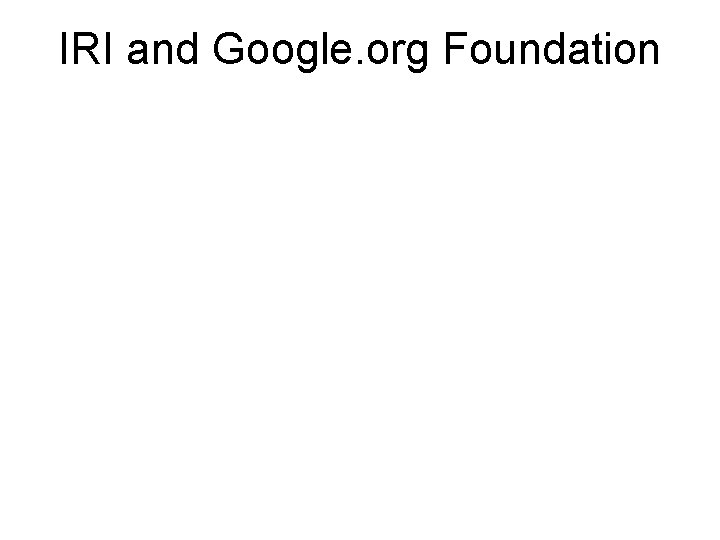
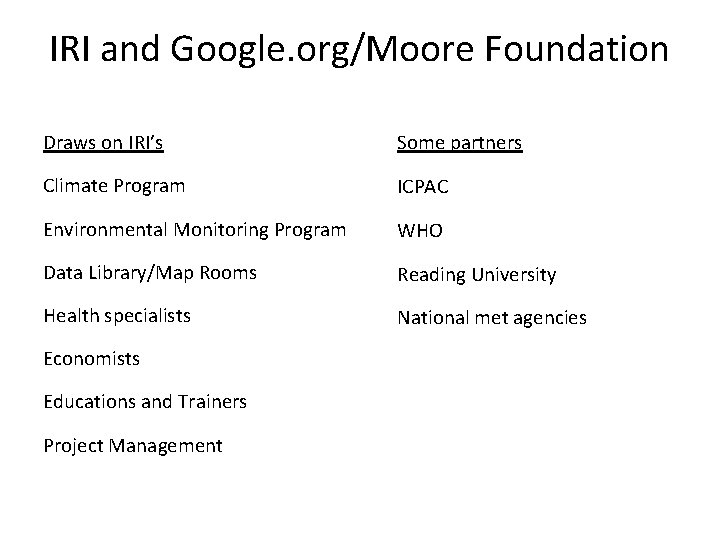
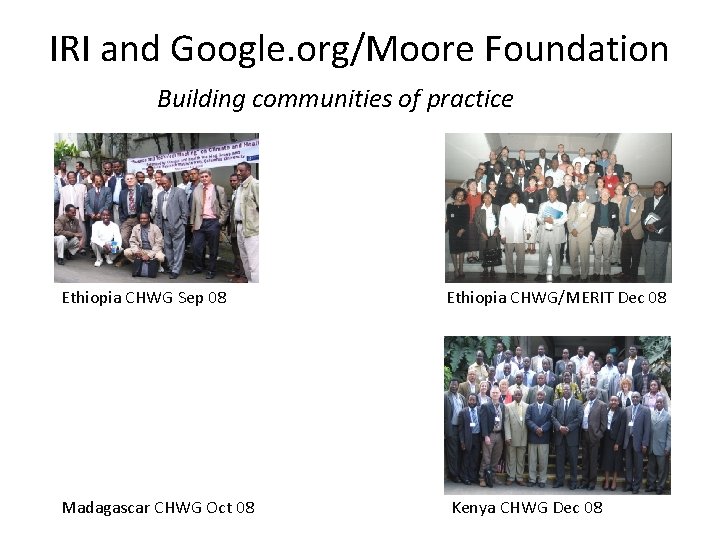
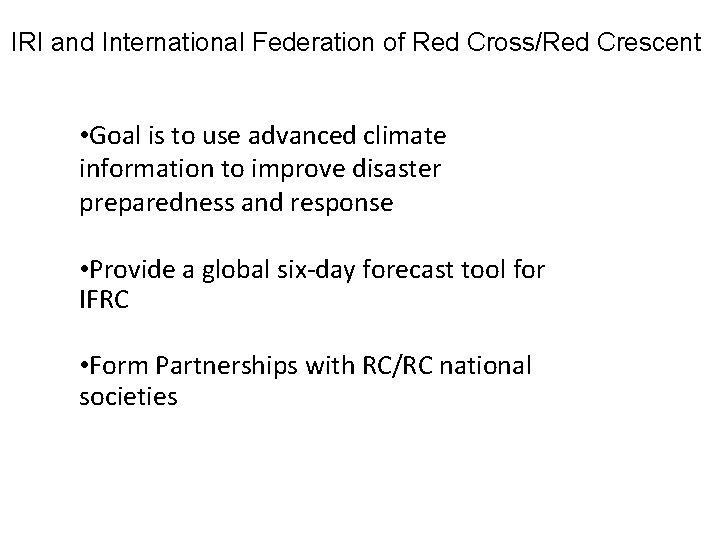
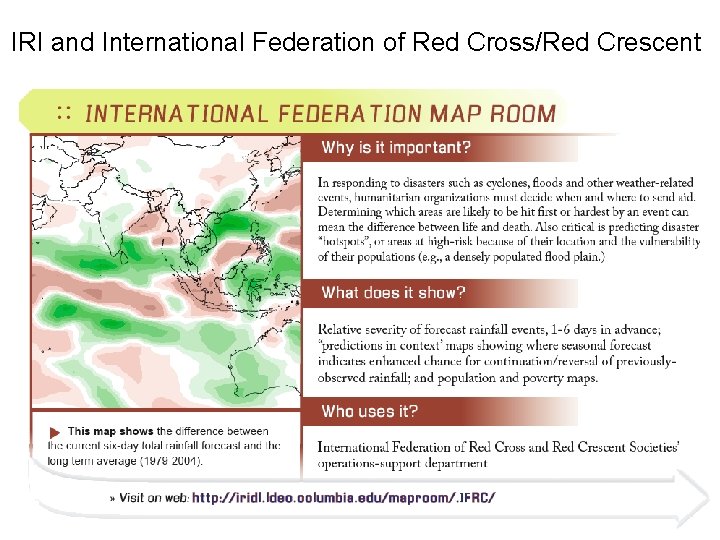
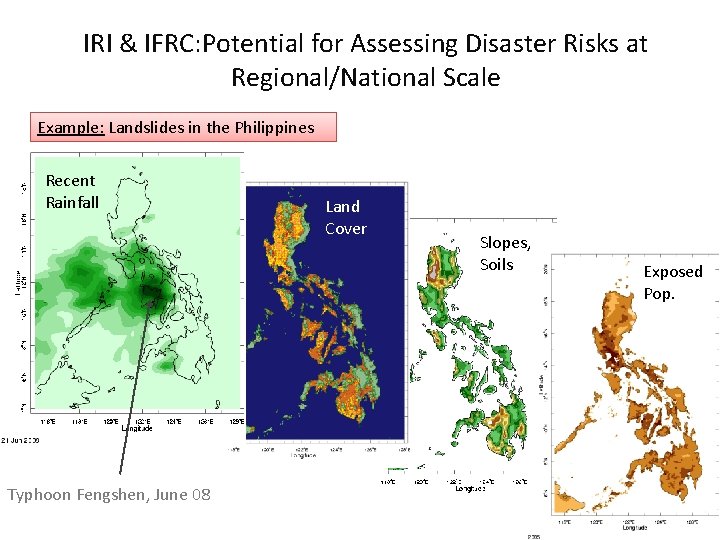
- Slides: 19
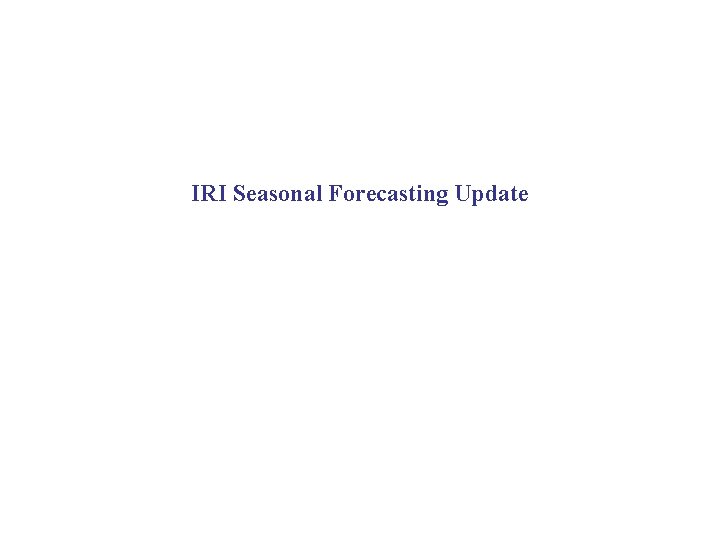
IRI Seasonal Forecasting Update
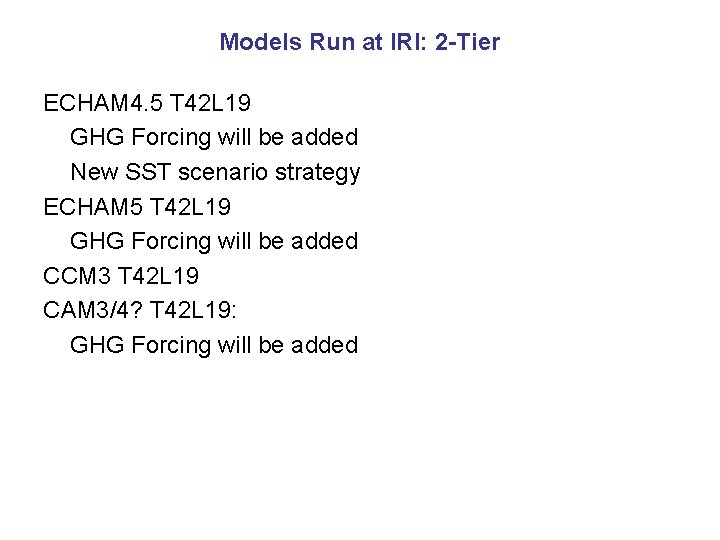
Models Run at IRI: 2 -Tier ECHAM 4. 5 T 42 L 19 GHG Forcing will be added New SST scenario strategy ECHAM 5 T 42 L 19 GHG Forcing will be added CCM 3 T 42 L 19 CAM 3/4? T 42 L 19: GHG Forcing will be added
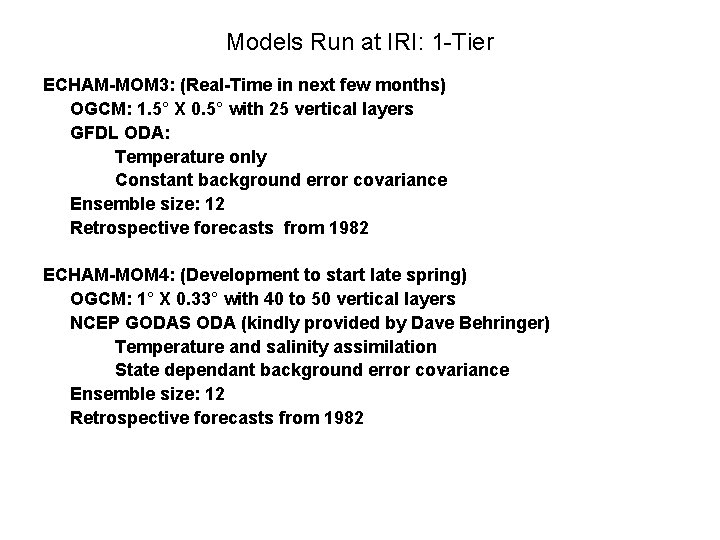
Models Run at IRI: 1 -Tier ECHAM-MOM 3: (Real-Time in next few months) OGCM: 1. 5° X 0. 5° with 25 vertical layers GFDL ODA: Temperature only Constant background error covariance Ensemble size: 12 Retrospective forecasts from 1982 ECHAM-MOM 4: (Development to start late spring) OGCM: 1° X 0. 33° with 40 to 50 vertical layers NCEP GODAS ODA (kindly provided by Dave Behringer) Temperature and salinity assimilation State dependant background error covariance Ensemble size: 12 Retrospective forecasts from 1982
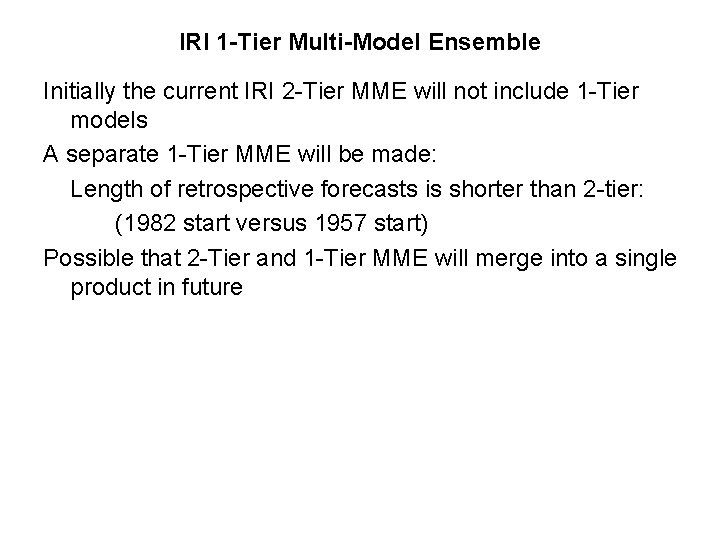
IRI 1 -Tier Multi-Model Ensemble Initially the current IRI 2 -Tier MME will not include 1 -Tier models A separate 1 -Tier MME will be made: Length of retrospective forecasts is shorter than 2 -tier: (1982 start versus 1957 start) Possible that 2 -Tier and 1 -Tier MME will merge into a single product in future
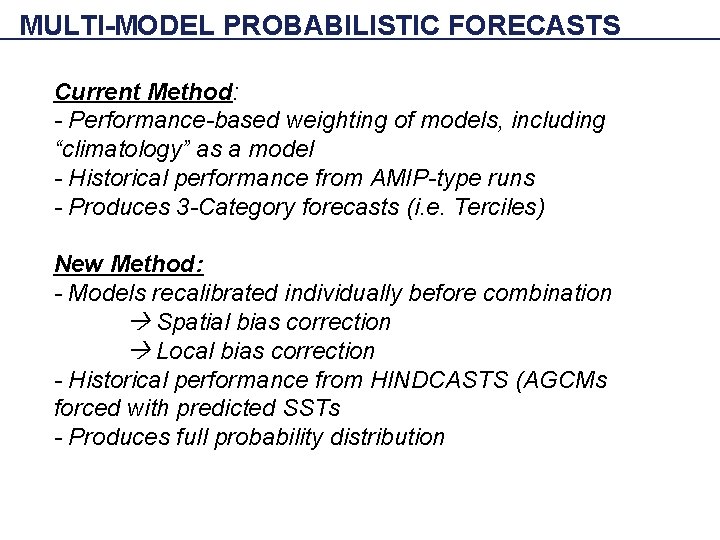
MULTI-MODEL PROBABILISTIC FORECASTS Current Method: - Performance-based weighting of models, including “climatology” as a model - Historical performance from AMIP-type runs - Produces 3 -Category forecasts (i. e. Terciles) New Method: - Models recalibrated individually before combination Spatial bias correction Local bias correction - Historical performance from HINDCASTS (AGCMs forced with predicted SSTs - Produces full probability distribution
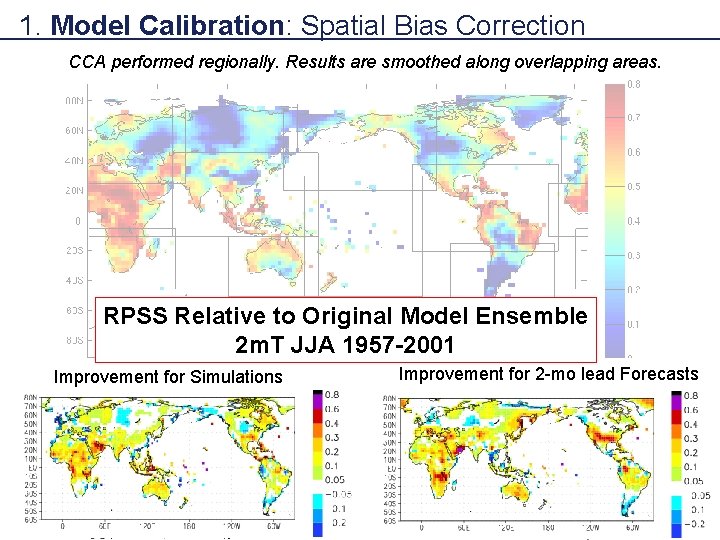
1. Model Calibration: Spatial Bias Correction CCA performed regionally. Results are smoothed along overlapping areas. RPSS Relative to Original Model Ensemble 2 m. T JJA 1957 -2001 Improvement for Simulations Improvement for 2 -mo lead Forecasts
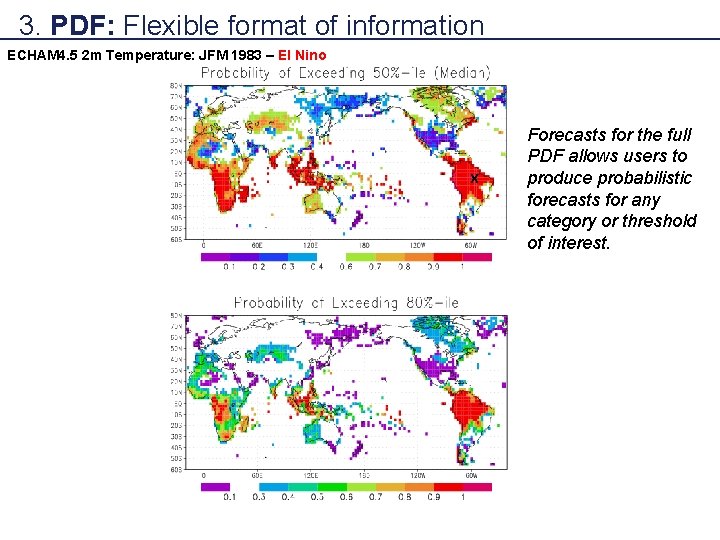
3. PDF: Flexible format of information ECHAM 4. 5 2 m Temperature: JFM 1983 – El Nino X Forecasts for the full PDF allows users to produce probabilistic forecasts for any category or threshold of interest.
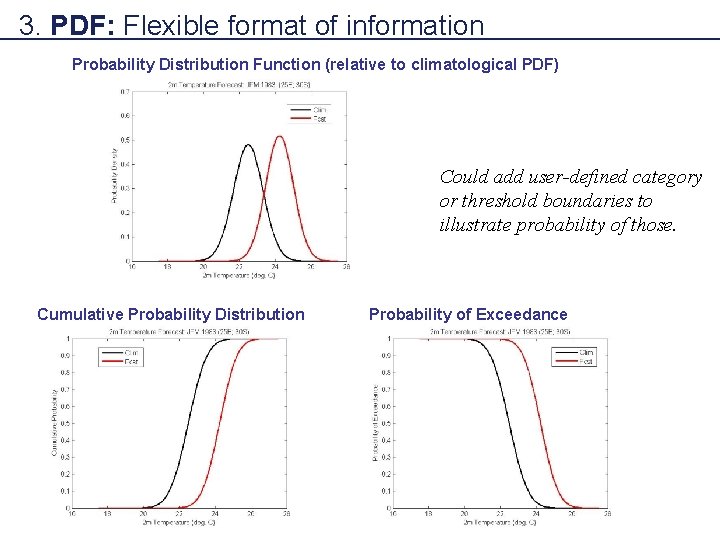
3. PDF: Flexible format of information Probability Distribution Function (relative to climatological PDF) Could add user-defined category or threshold boundaries to illustrate probability of those. Cumulative Probability Distribution Probability of Exceedance
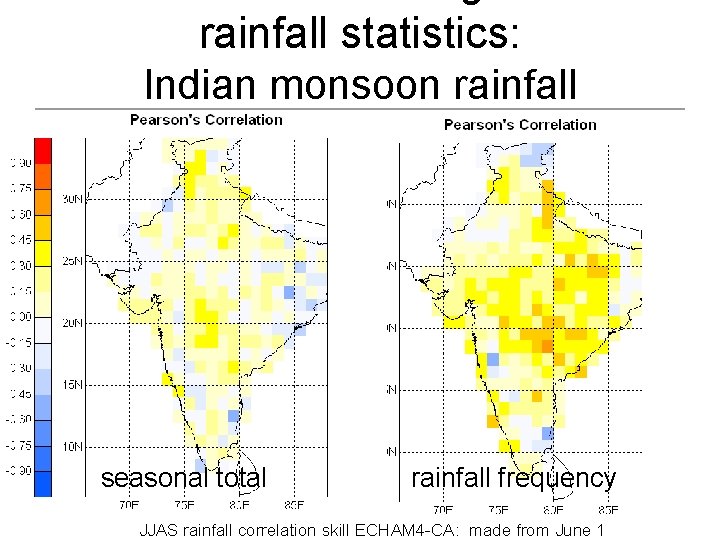
rainfall statistics: Indian monsoon rainfall seasonal total rainfall frequency JJAS rainfall correlation skill ECHAM 4 -CA: made from June 1
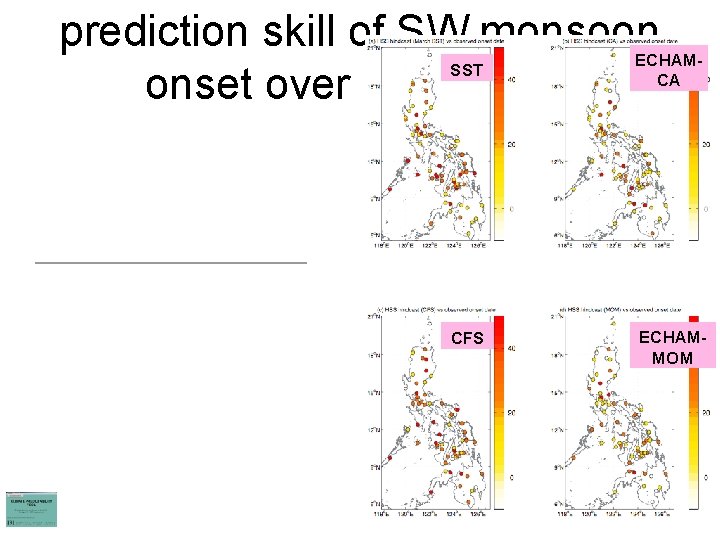
prediction skill of SW monsoon onset over Philippines SST CFS ECHAMCA ECHAMMOM
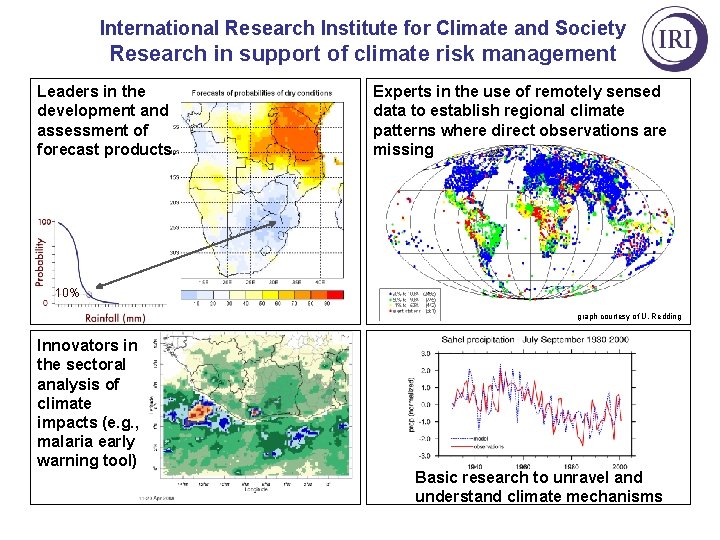
International Research Institute for Climate and Society Research in support of climate risk management Leaders in the development and assessment of forecast products. Experts in the use of remotely sensed data to establish regional climate patterns where direct observations are missing 10% graph courtesy of U. Redding Innovators in the sectoral analysis of climate impacts (e. g. , malaria early warning tool) Basic research to unravel and understand climate mechanisms
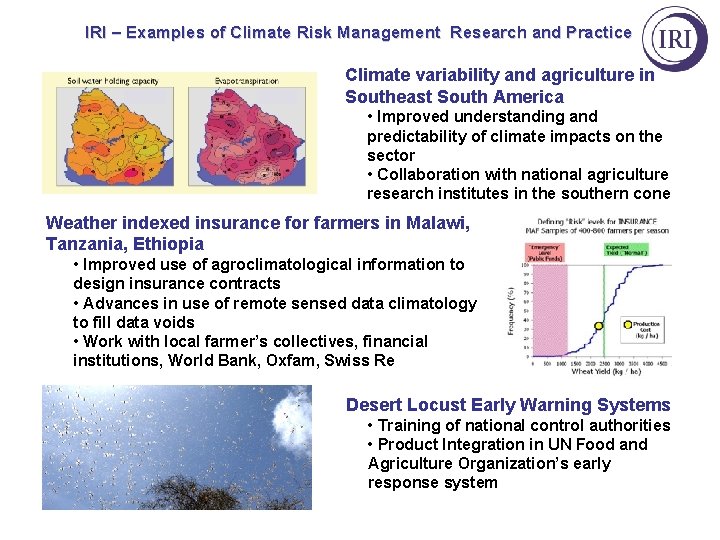
IRI – Examples of Climate Risk Management Research and Practice Climate variability and agriculture in Southeast South America • Improved understanding and predictability of climate impacts on the sector • Collaboration with national agriculture research institutes in the southern cone Weather indexed insurance for farmers in Malawi, Tanzania, Ethiopia • Improved use of agroclimatological information to design insurance contracts • Advances in use of remote sensed data climatology to fill data voids • Work with local farmer’s collectives, financial institutions, World Bank, Oxfam, Swiss Re Desert Locust Early Warning Systems • Training of national control authorities • Product Integration in UN Food and Agriculture Organization’s early response system
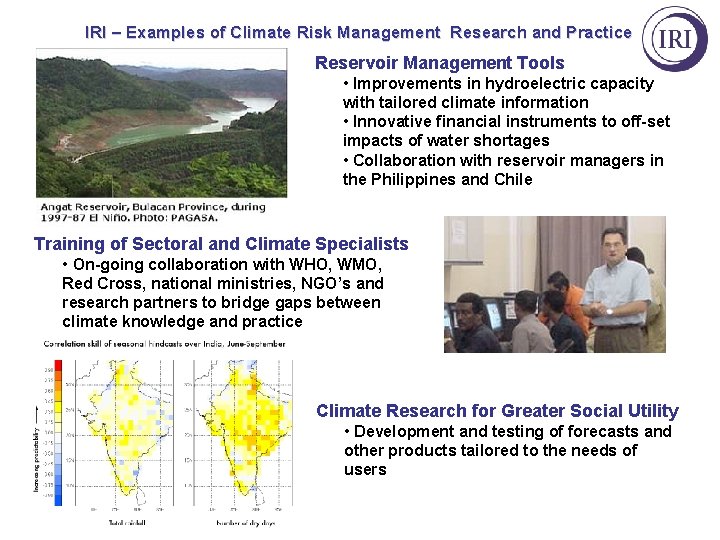
IRI – Examples of Climate Risk Management Research and Practice Reservoir Management Tools • Improvements in hydroelectric capacity with tailored climate information • Innovative financial instruments to off-set impacts of water shortages • Collaboration with reservoir managers in the Philippines and Chile Training of Sectoral and Climate Specialists • On-going collaboration with WHO, WMO, Red Cross, national ministries, NGO’s and research partners to bridge gaps between climate knowledge and practice Climate Research for Greater Social Utility • Development and testing of forecasts and other products tailored to the needs of users
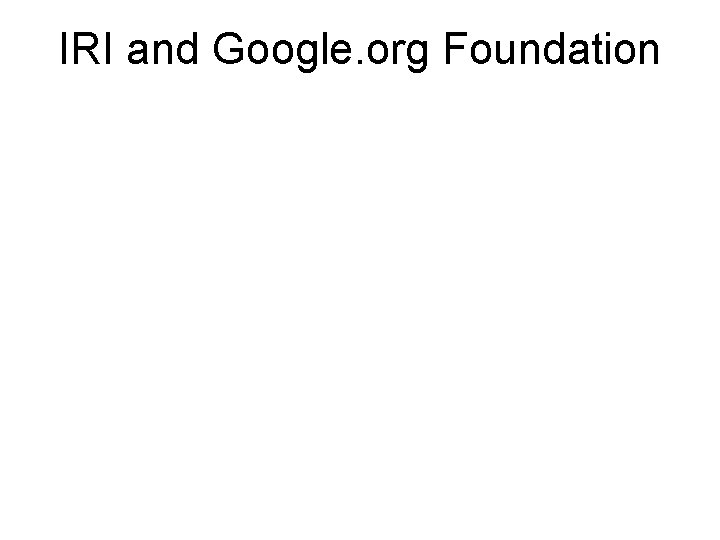
IRI and Google. org Foundation Three-year invited project from Google/Moore (March 08) Geographical Focus: Greater Horn of Africa Focal country case study: Ethiopia Climate side: increase capacity to supply useful information products to help guide health interventions Health side: increase capacity to demand use climate products for more effective interventions
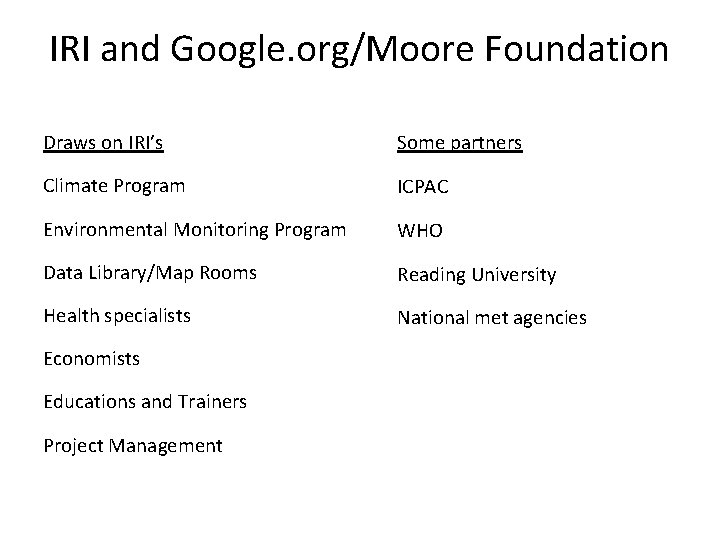
IRI and Google. org/Moore Foundation Draws on IRI’s Some partners Climate Program ICPAC Environmental Monitoring Program WHO Data Library/Map Rooms Reading University Health specialists National met agencies Economists Educations and Trainers Project Management
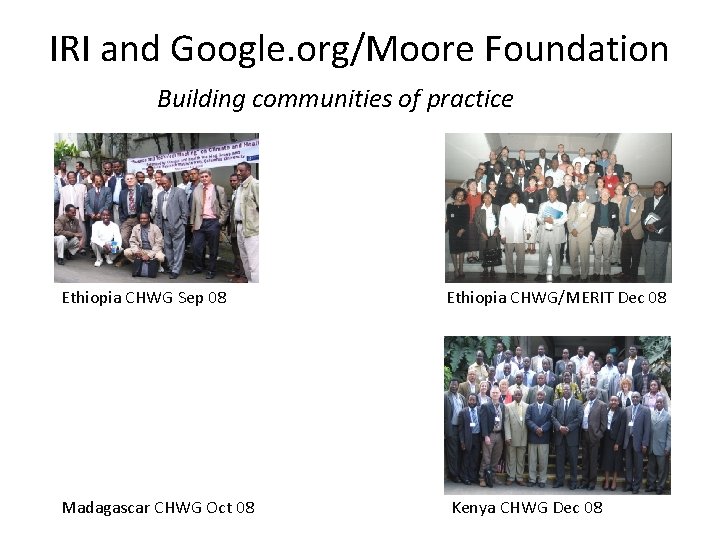
IRI and Google. org/Moore Foundation Building communities of practice Ethiopia CHWG Sep 08 Ethiopia CHWG/MERIT Dec 08 Madagascar CHWG Oct 08 Kenya CHWG Dec 08
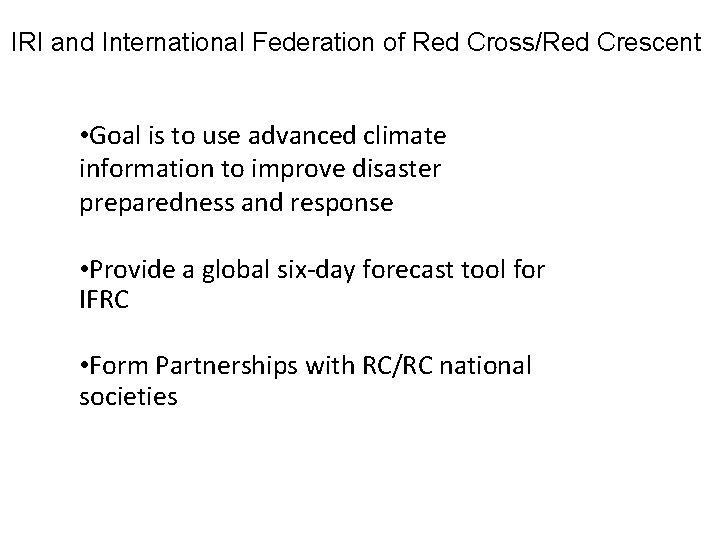
IRI and International Federation of Red Cross/Red Crescent • Goal is to use advanced climate information to improve disaster preparedness and response • Provide a global six-day forecast tool for IFRC • Form Partnerships with RC/RC national societies
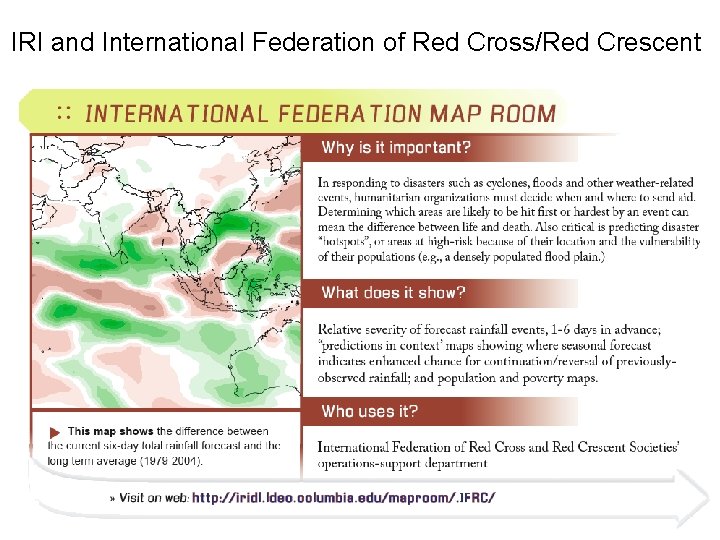
IRI and International Federation of Red Cross/Red Crescent
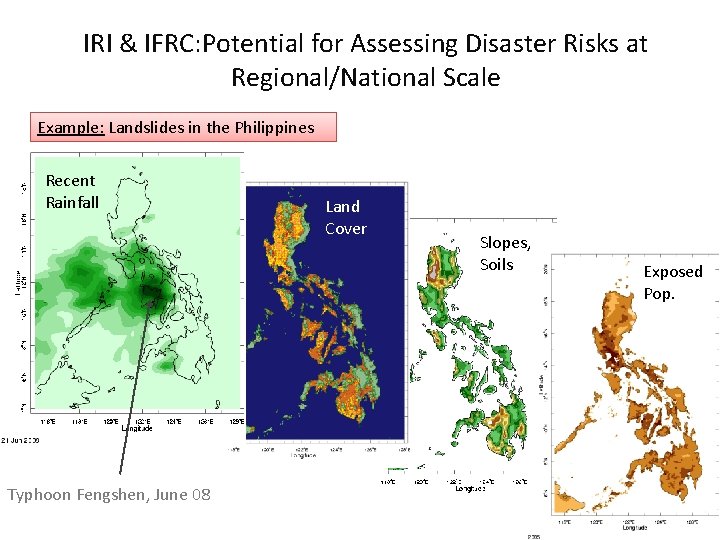
IRI & IFRC: Potential for Assessing Disaster Risks at Regional/National Scale Example: Landslides in the Philippines Recent Rainfall Typhoon Fengshen, June 08 Land Cover Slopes, Soils Exposed Pop.
Iri columbia seasonal forecast
Iri columbia seasonal forecast
Is an alternative of log based recovery.
Types of forecasting models
Statistical forecasting models
Run lola run themes
Short run equilibrium under perfect competition
Run lola run editing techniques
Lola brigitta
Short run vs long run economics
Brigitta manthey
Short run equilibrium under perfect competition
Within run and across run meaning
Difference between model and semi modal
Sundial meaning
Asia seasonal forecast
Seasonal work means
Macroeconomics
Systematic component = (level + trend) * seasonal factor
Chronic allergies icd 10