Informing Ethical Evaluations of Adaptive Clinical Trials through
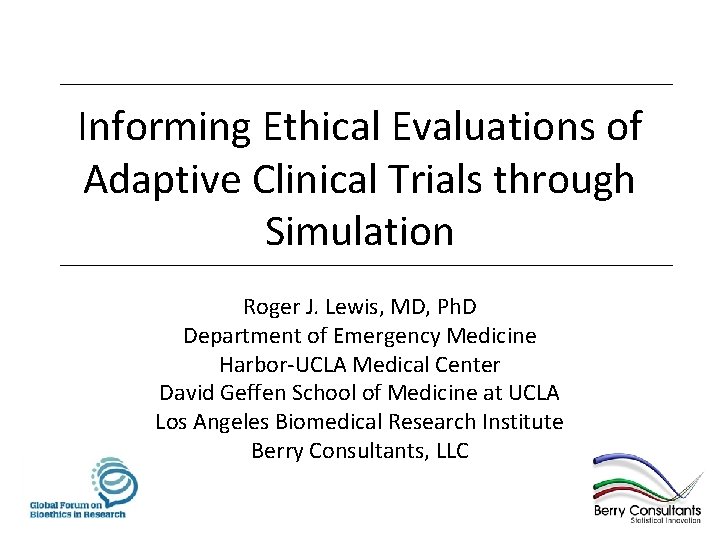
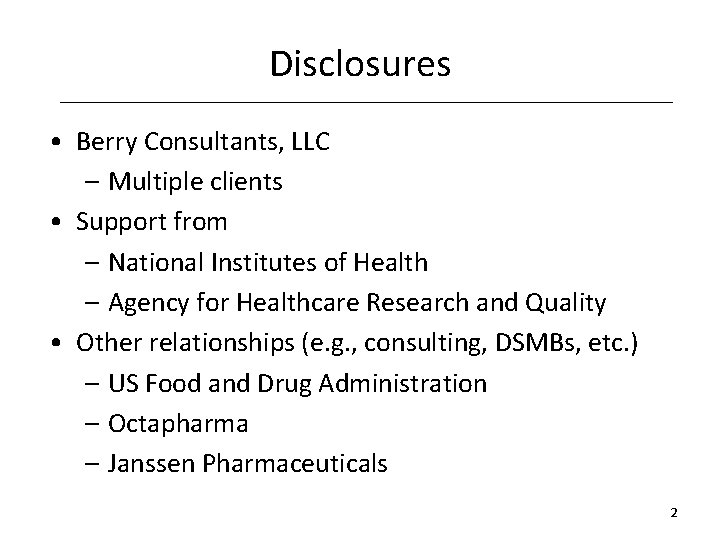
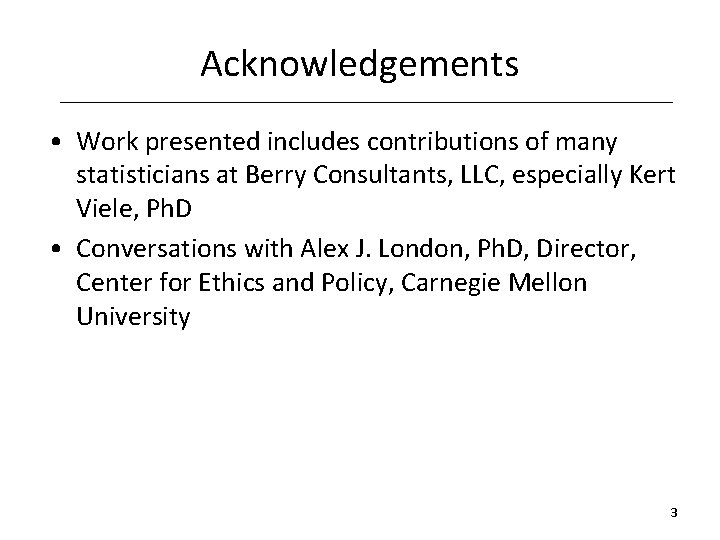
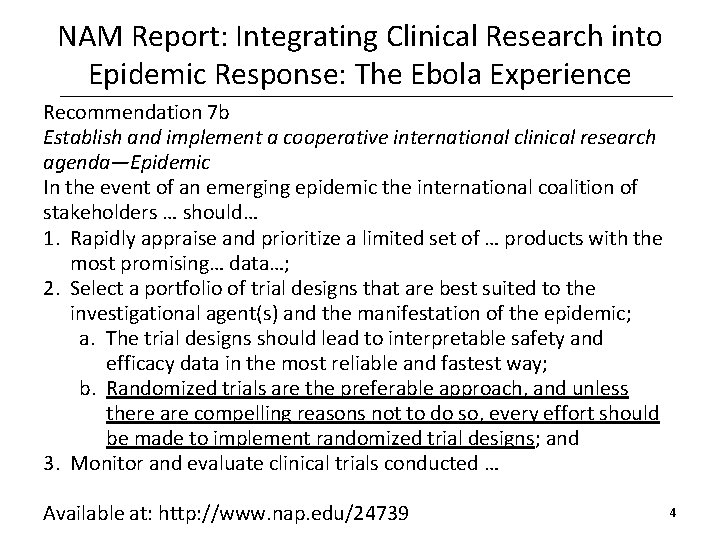
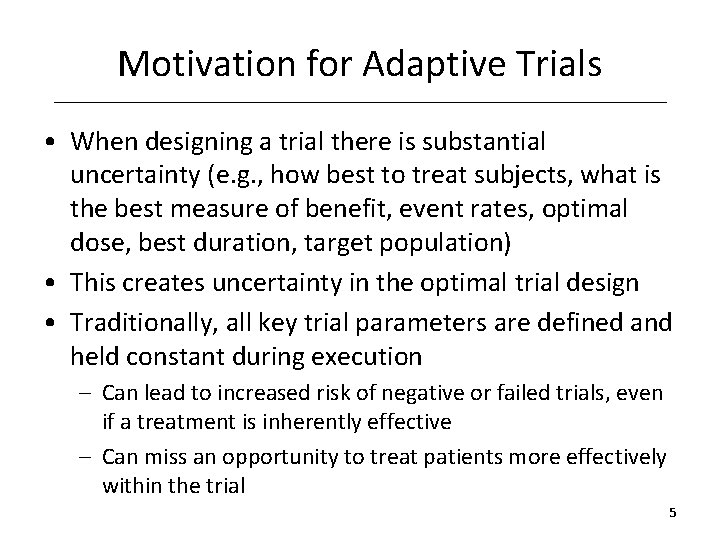
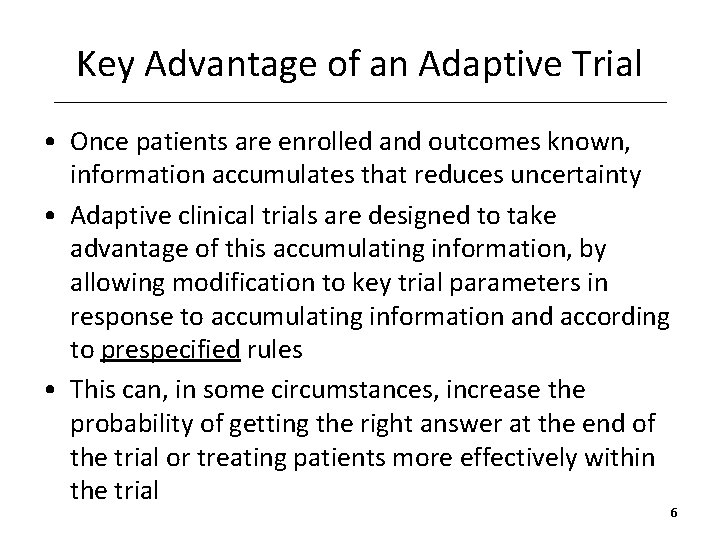
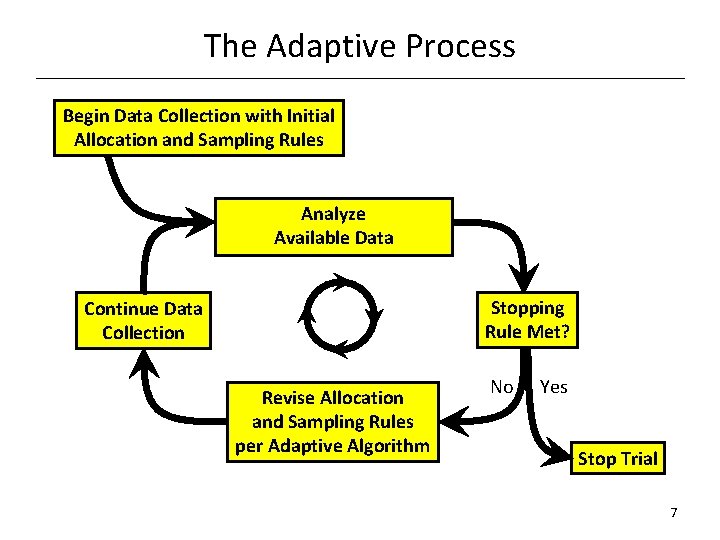
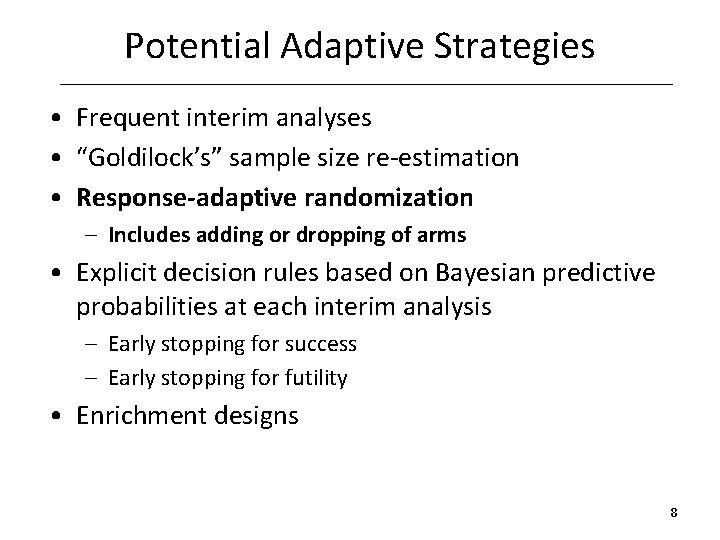
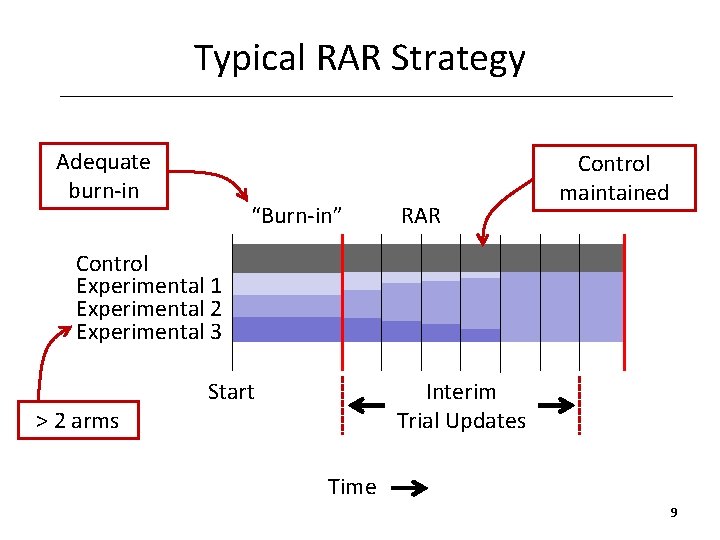
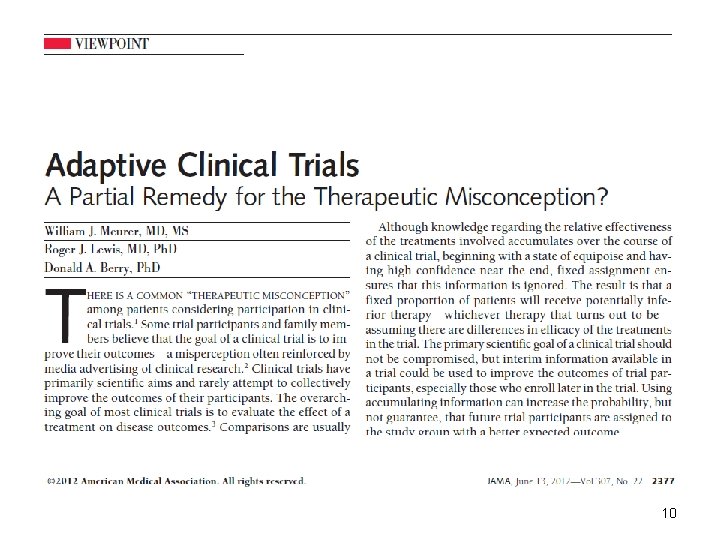
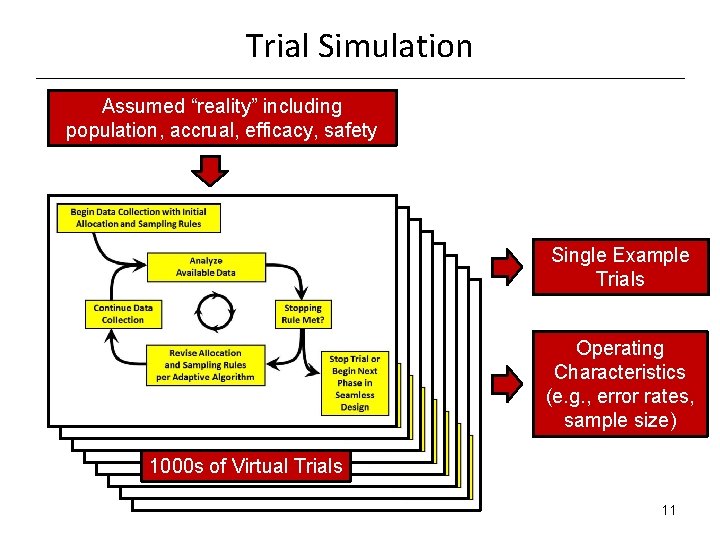
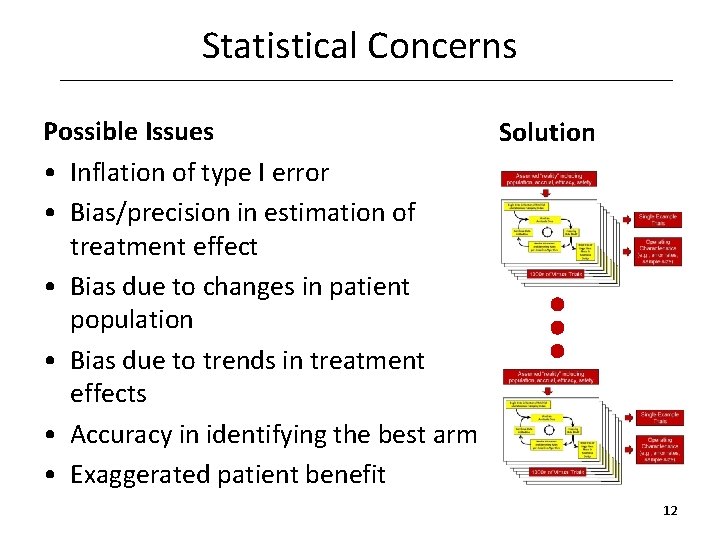
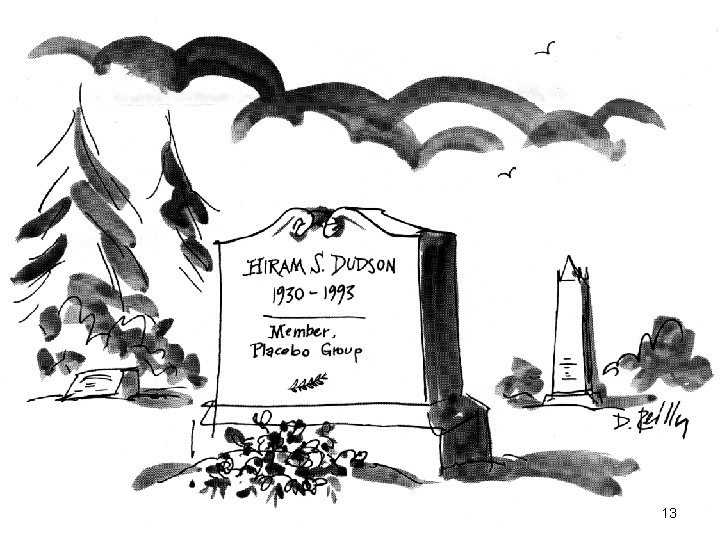
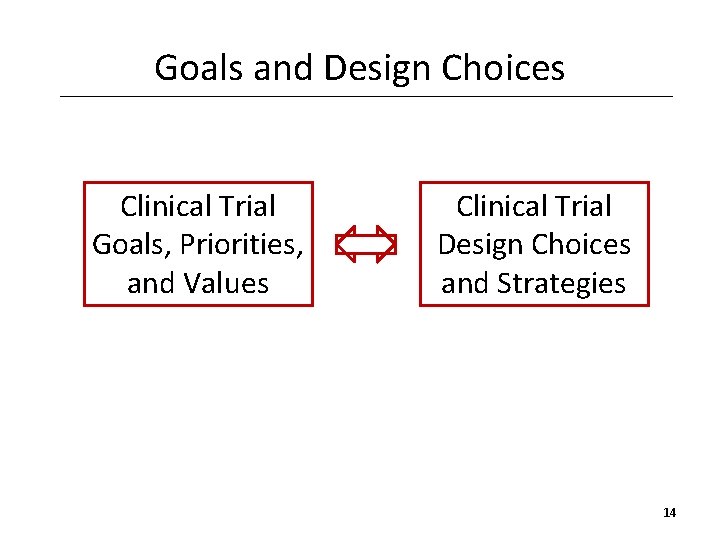
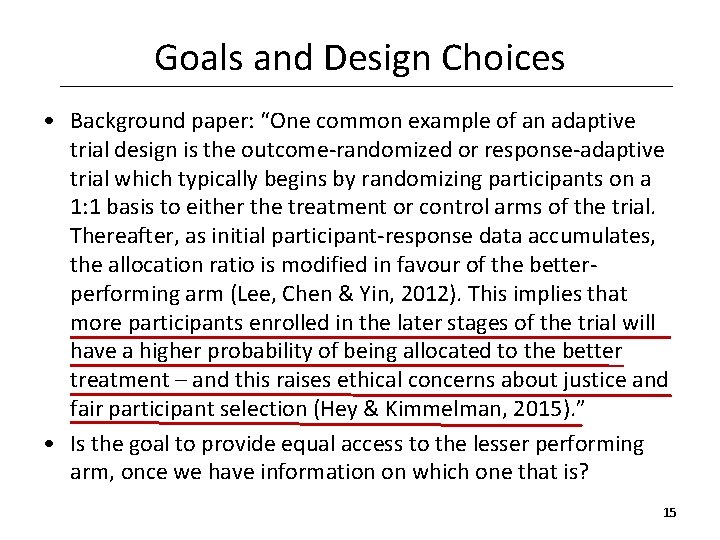
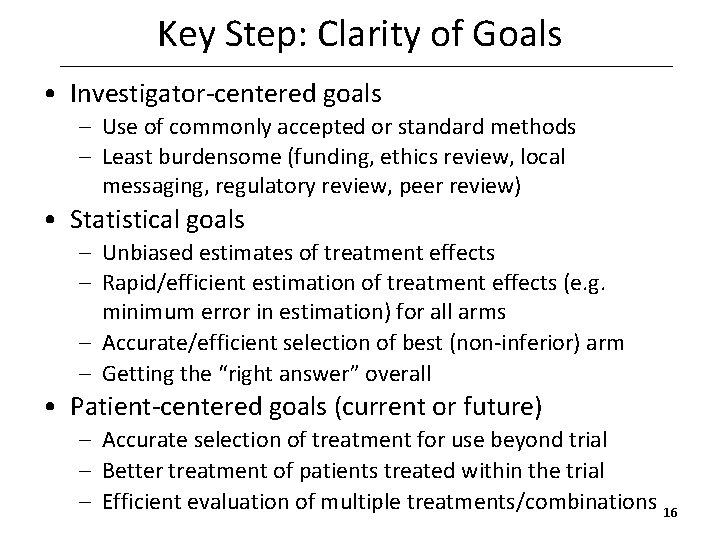
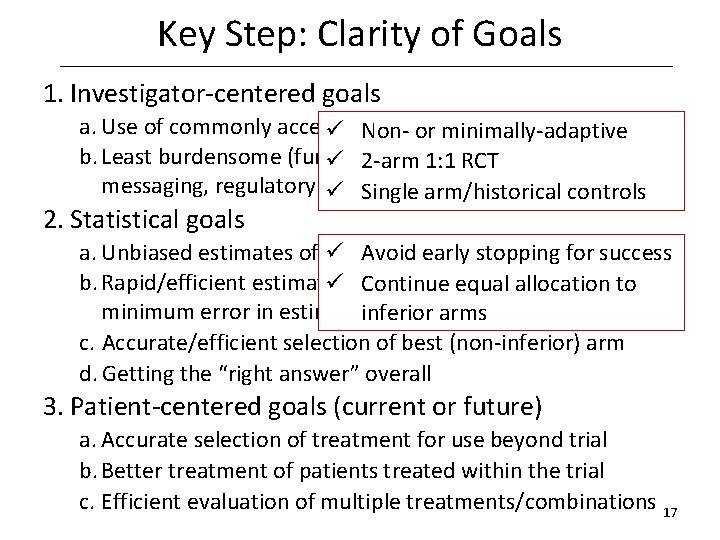
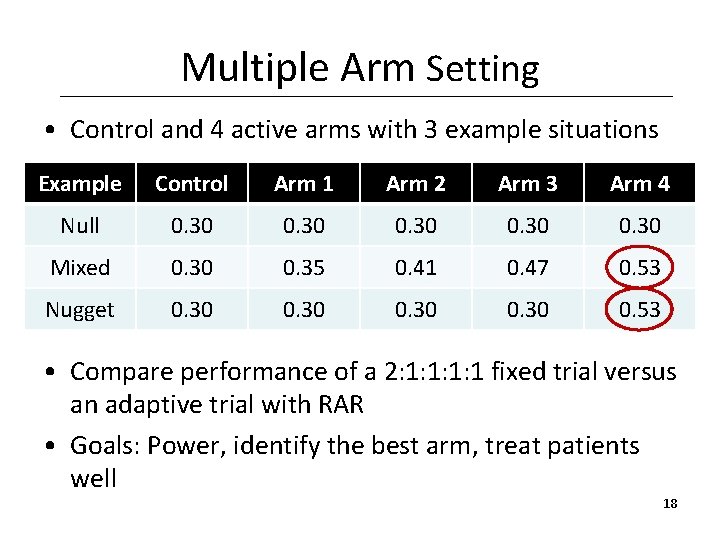
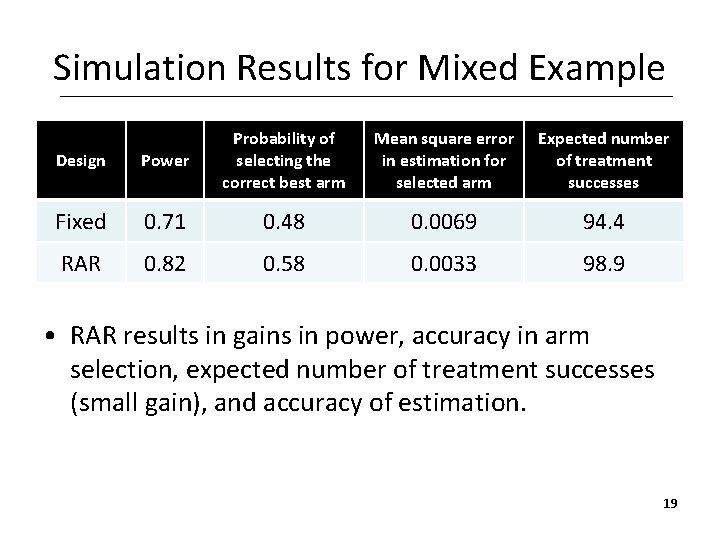
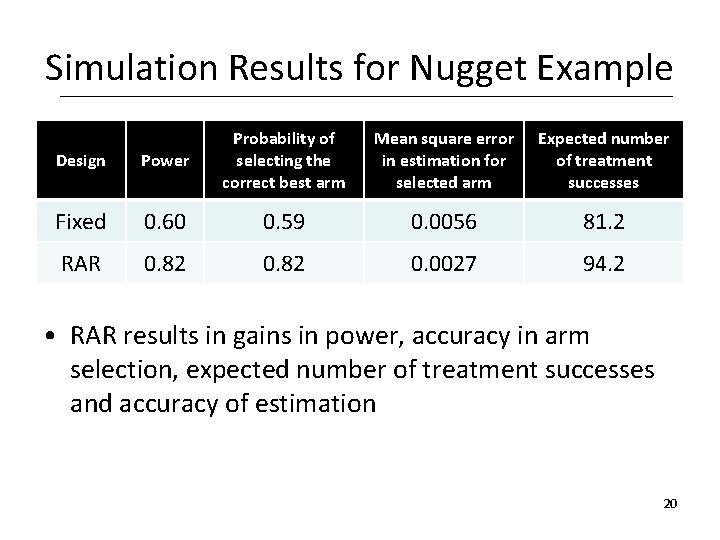
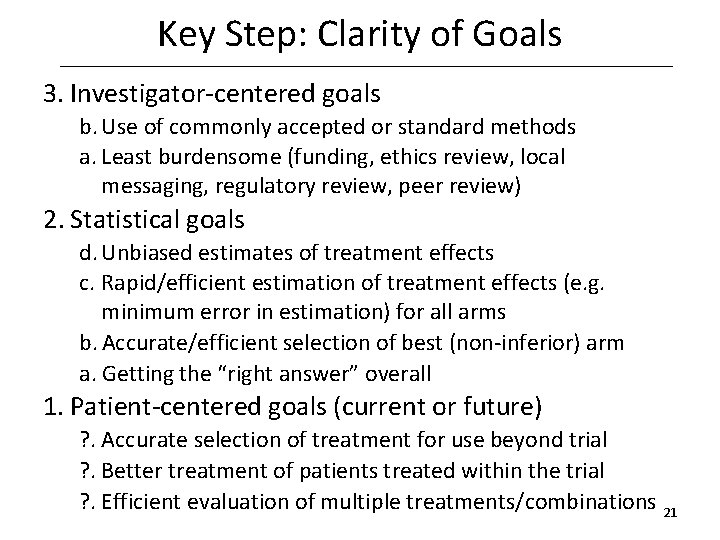
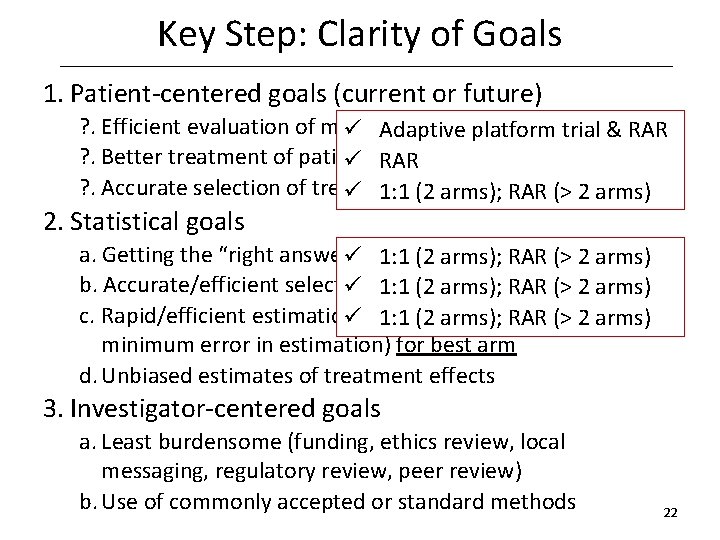
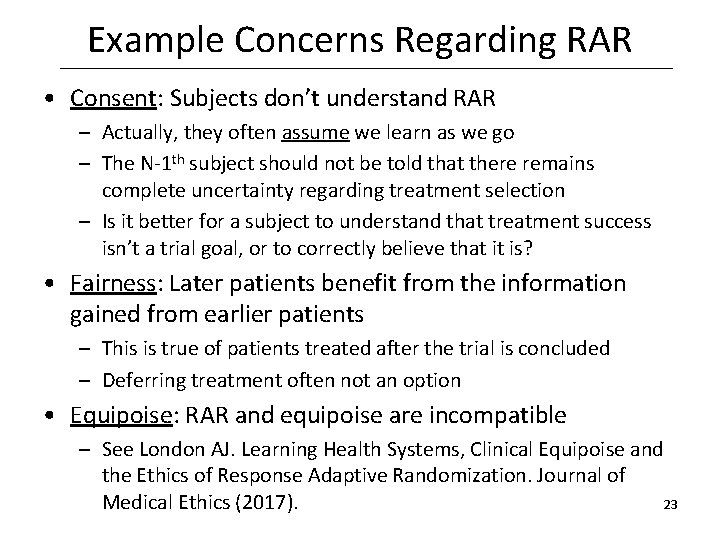
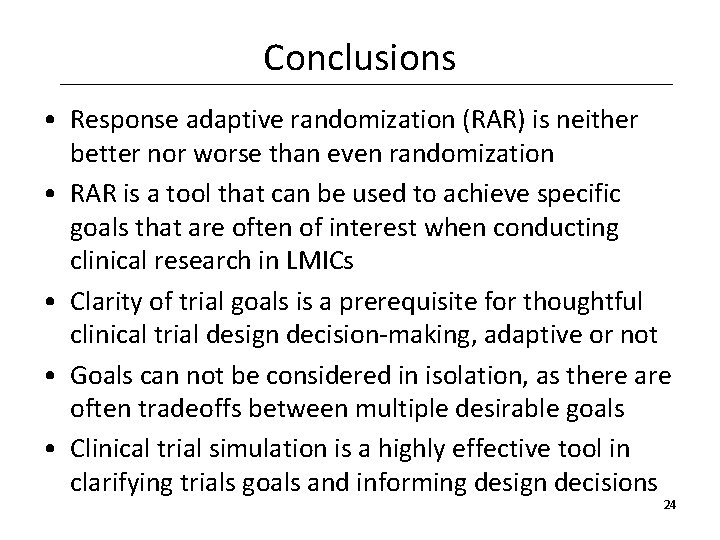
- Slides: 24
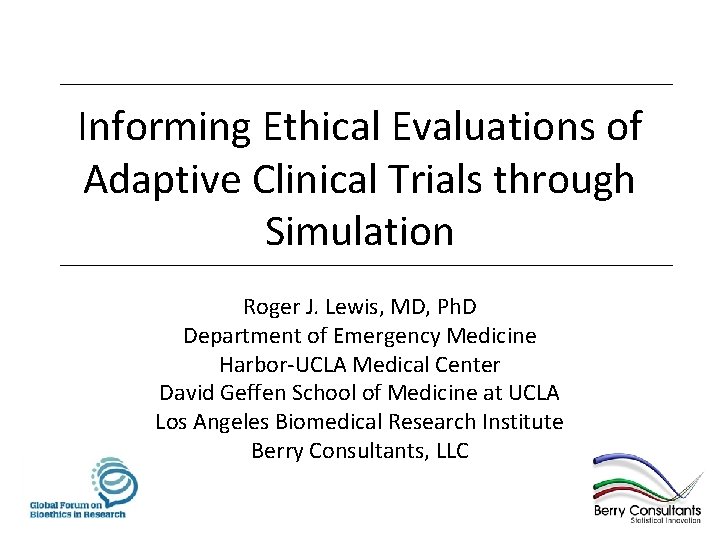
Informing Ethical Evaluations of Adaptive Clinical Trials through Simulation Roger J. Lewis, MD, Ph. D Department of Emergency Medicine Harbor-UCLA Medical Center David Geffen School of Medicine at UCLA Los Angeles Biomedical Research Institute Berry Consultants, LLC
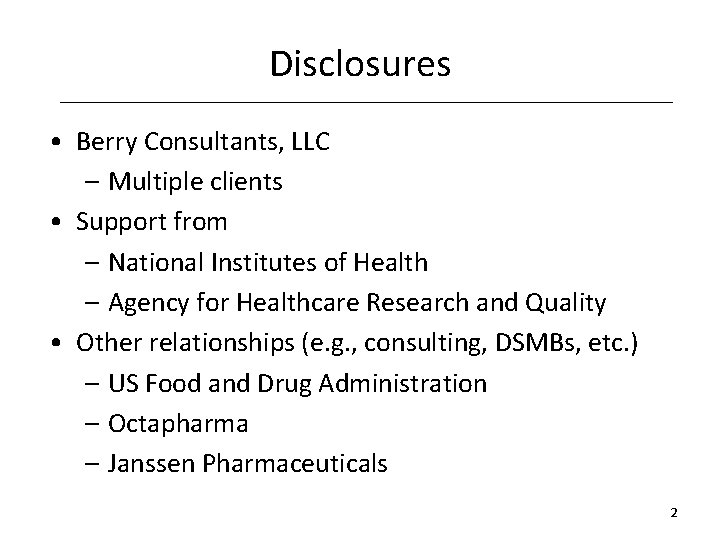
Disclosures • Berry Consultants, LLC – Multiple clients • Support from – National Institutes of Health – Agency for Healthcare Research and Quality • Other relationships (e. g. , consulting, DSMBs, etc. ) – US Food and Drug Administration – Octapharma – Janssen Pharmaceuticals 2
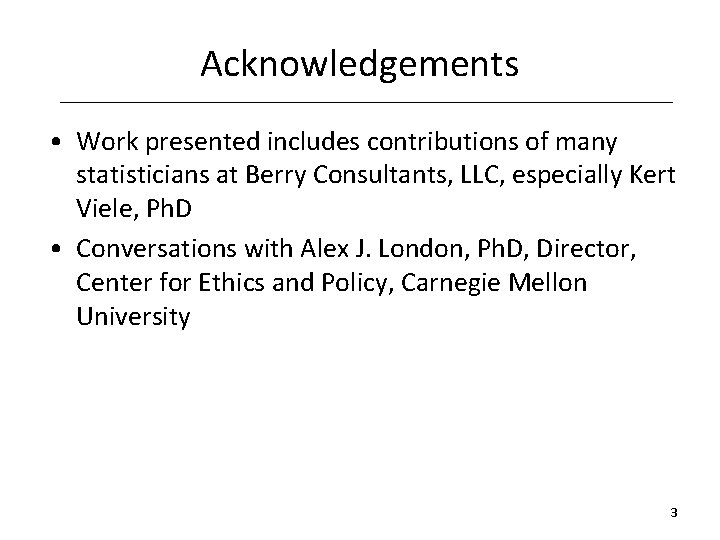
Acknowledgements • Work presented includes contributions of many statisticians at Berry Consultants, LLC, especially Kert Viele, Ph. D • Conversations with Alex J. London, Ph. D, Director, Center for Ethics and Policy, Carnegie Mellon University 3
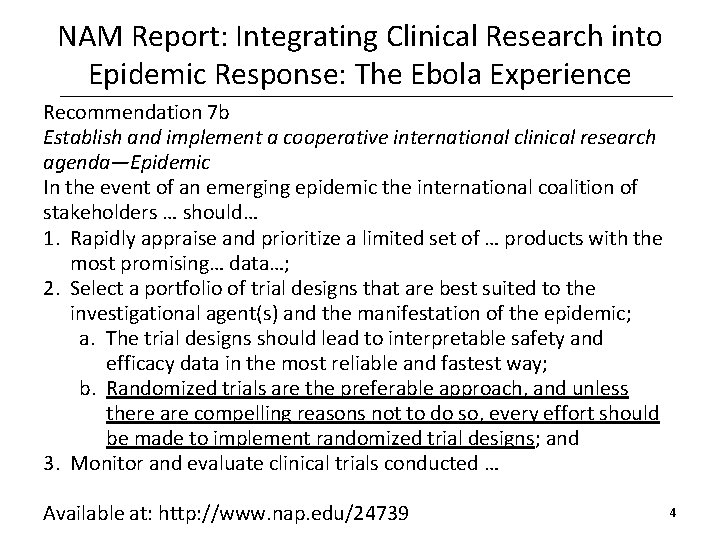
NAM Report: Integrating Clinical Research into Epidemic Response: The Ebola Experience Recommendation 7 b Establish and implement a cooperative international clinical research agenda—Epidemic In the event of an emerging epidemic the international coalition of stakeholders … should… 1. Rapidly appraise and prioritize a limited set of … products with the most promising… data…; 2. Select a portfolio of trial designs that are best suited to the investigational agent(s) and the manifestation of the epidemic; a. The trial designs should lead to interpretable safety and efficacy data in the most reliable and fastest way; b. Randomized trials are the preferable approach, and unless there are compelling reasons not to do so, every effort should be made to implement randomized trial designs; and 3. Monitor and evaluate clinical trials conducted … Available at: http: //www. nap. edu/24739 4
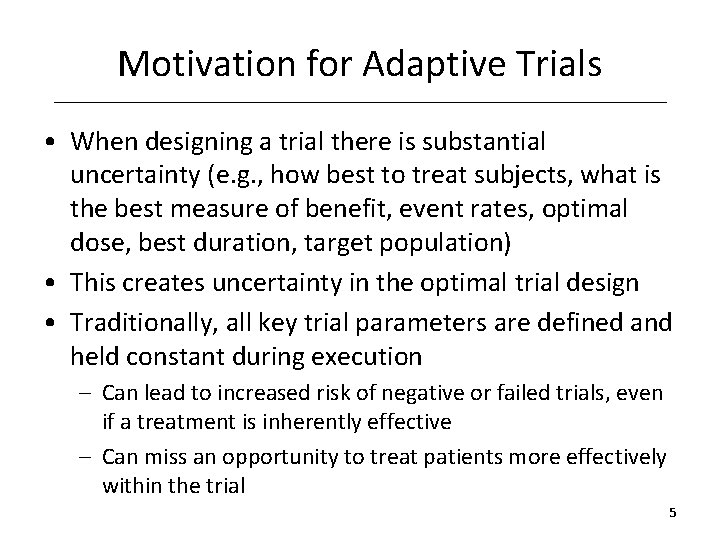
Motivation for Adaptive Trials • When designing a trial there is substantial uncertainty (e. g. , how best to treat subjects, what is the best measure of benefit, event rates, optimal dose, best duration, target population) • This creates uncertainty in the optimal trial design • Traditionally, all key trial parameters are defined and held constant during execution – Can lead to increased risk of negative or failed trials, even if a treatment is inherently effective – Can miss an opportunity to treat patients more effectively within the trial 5
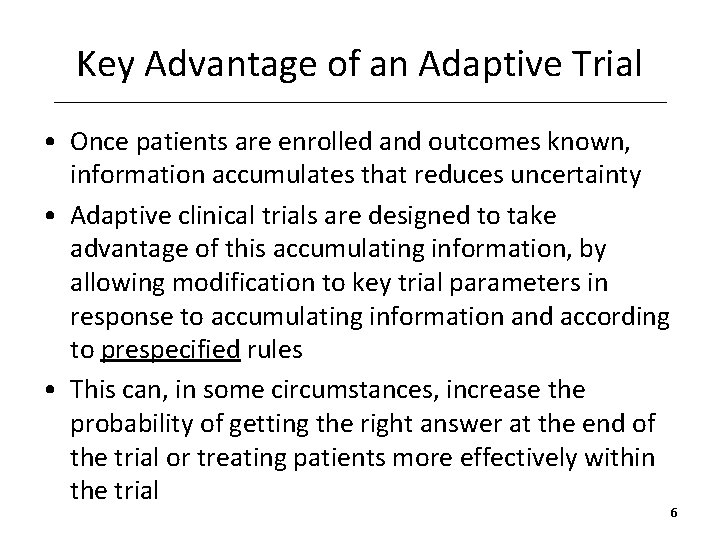
Key Advantage of an Adaptive Trial • Once patients are enrolled and outcomes known, information accumulates that reduces uncertainty • Adaptive clinical trials are designed to take advantage of this accumulating information, by allowing modification to key trial parameters in response to accumulating information and according to prespecified rules • This can, in some circumstances, increase the probability of getting the right answer at the end of the trial or treating patients more effectively within the trial 6
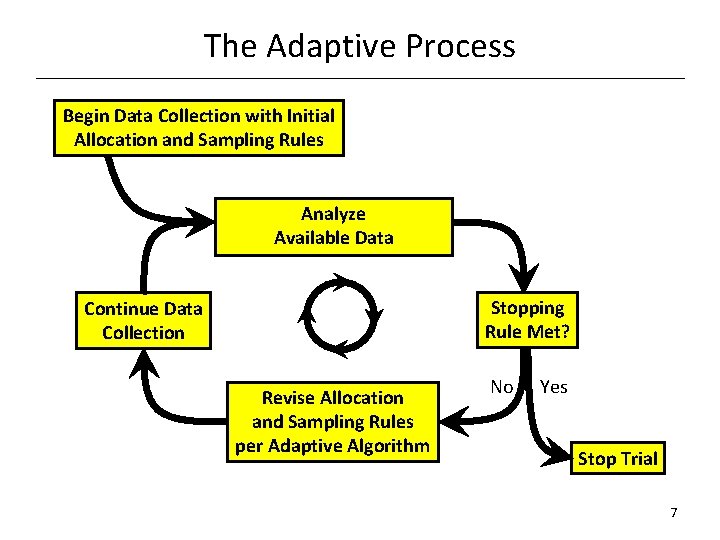
The Adaptive Process Begin Data Collection with Initial Allocation and Sampling Rules Analyze Available Data Stopping Rule Met? Continue Data Collection Revise Allocation and Sampling Rules per Adaptive Algorithm No Yes Stop Trial 7
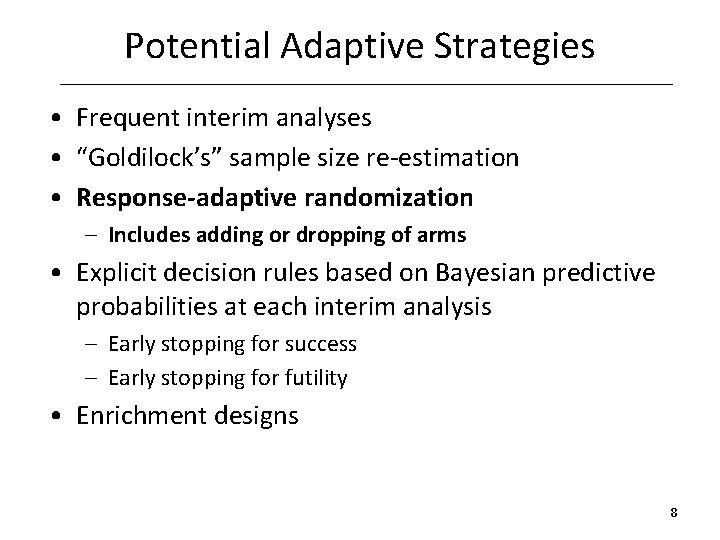
Potential Adaptive Strategies • Frequent interim analyses • “Goldilock’s” sample size re-estimation • Response-adaptive randomization – Includes adding or dropping of arms • Explicit decision rules based on Bayesian predictive probabilities at each interim analysis – Early stopping for success – Early stopping for futility • Enrichment designs 8
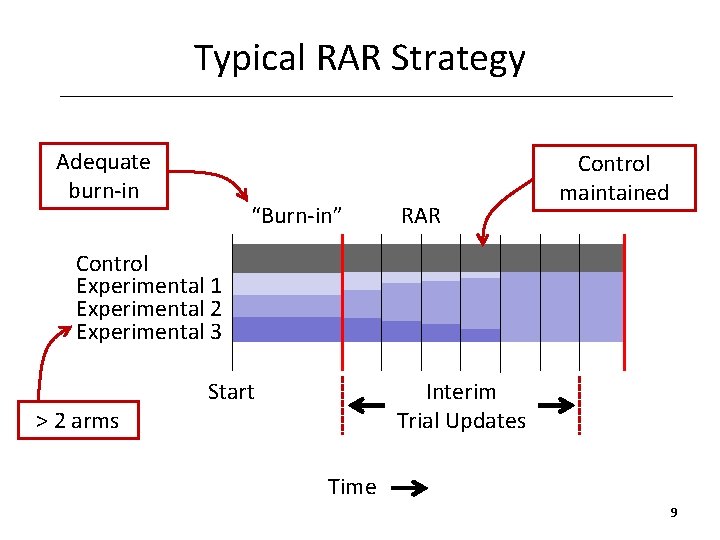
Typical RAR Strategy Adequate burn-in “Burn-in” RAR Control maintained Control Experimental 1 Experimental 2 Experimental 3 > 2 arms Start Interim Trial Updates Time 9
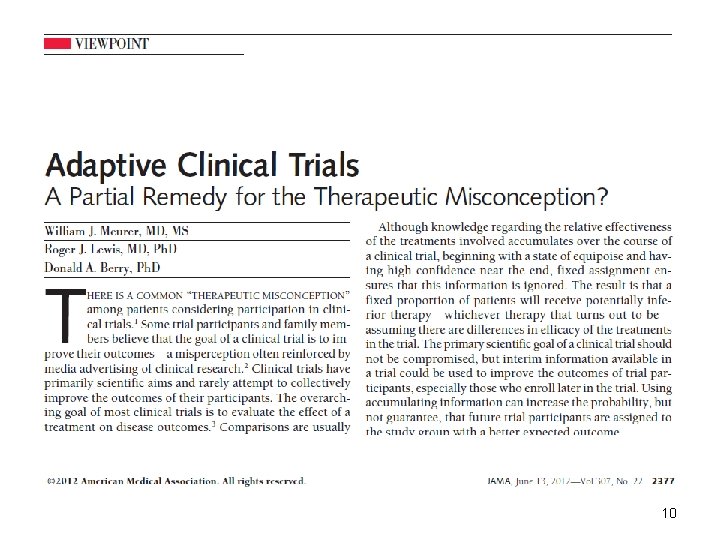
10
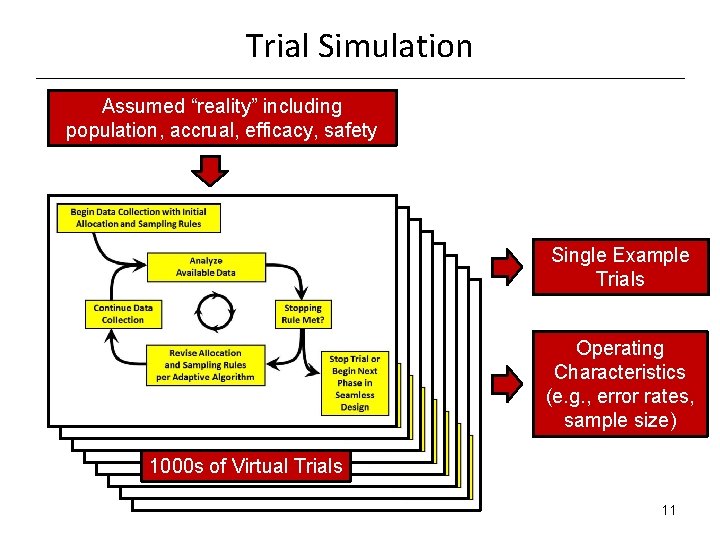
Trial Simulation Assumed “reality” including population, accrual, efficacy, safety Single Example Trials Operating Characteristics (e. g. , error rates, sample size) 1000 s of Virtual Trials 11
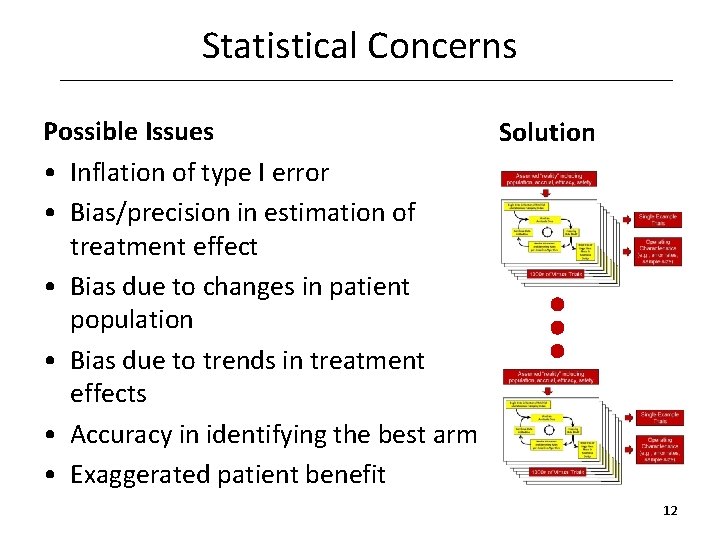
Statistical Concerns Possible Issues Solution • Inflation of type I error • Bias/precision in estimation of treatment effect • Bias due to changes in patient population • Bias due to trends in treatment effects • Accuracy in identifying the best arm • Exaggerated patient benefit 12
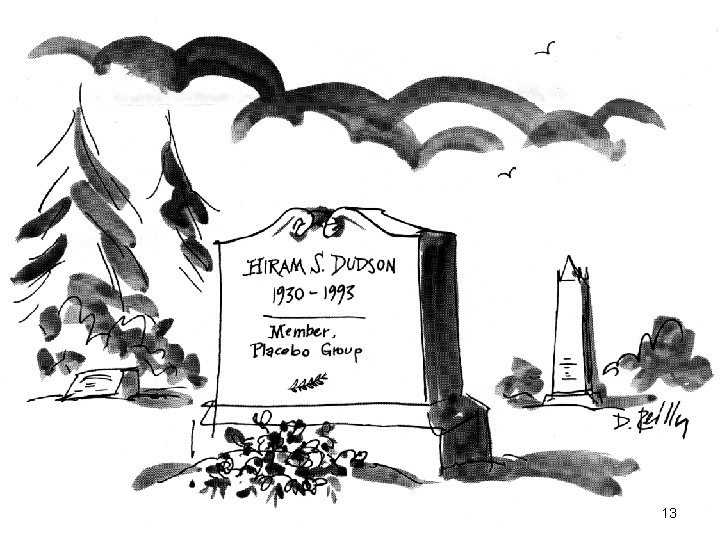
13
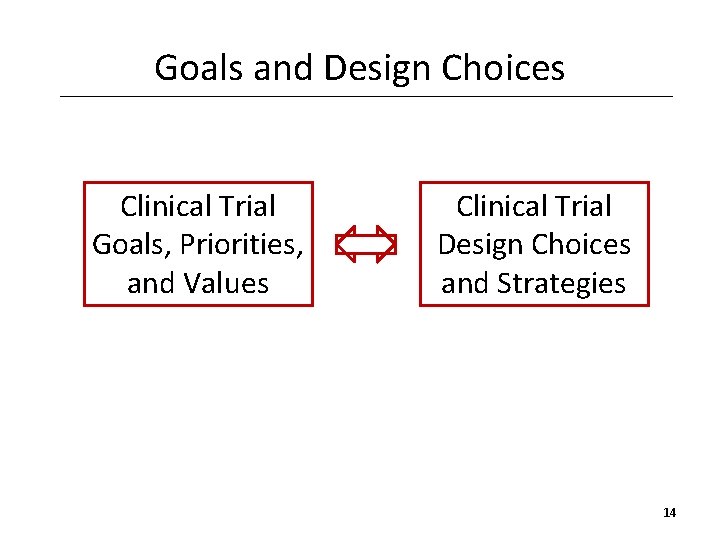
Goals and Design Choices Clinical Trial Goals, Priorities, and Values Clinical Trial Design Choices and Strategies 14
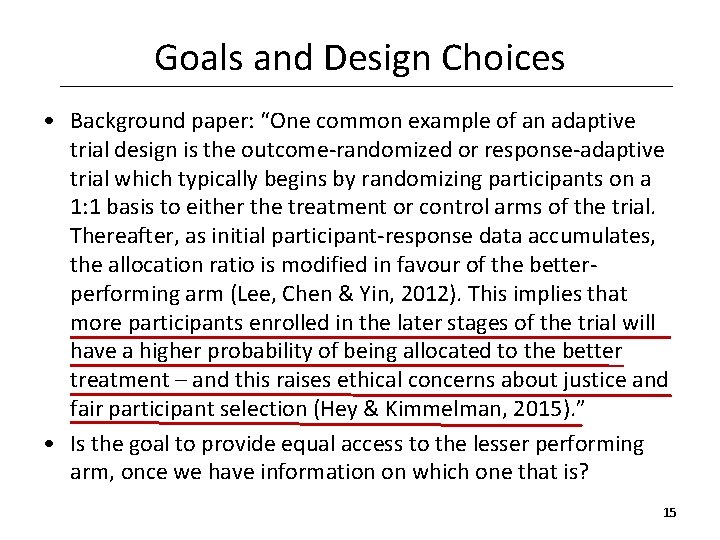
Goals and Design Choices • Background paper: “One common example of an adaptive trial design is the outcome-randomized or response-adaptive trial which typically begins by randomizing participants on a 1: 1 basis to either the treatment or control arms of the trial. Thereafter, as initial participant-response data accumulates, the allocation ratio is modified in favour of the betterperforming arm (Lee, Chen & Yin, 2012). This implies that more participants enrolled in the later stages of the trial will have a higher probability of being allocated to the better treatment – and this raises ethical concerns about justice and fair participant selection (Hey & Kimmelman, 2015). ” • Is the goal to provide equal access to the lesser performing arm, once we have information on which one that is? 15
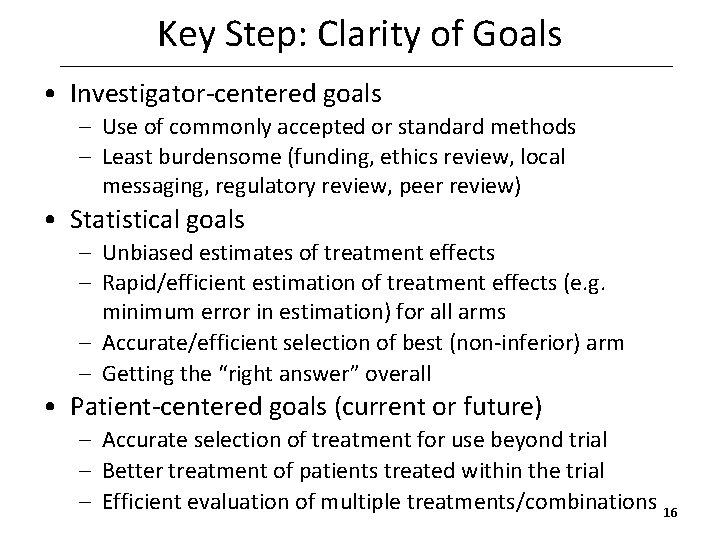
Key Step: Clarity of Goals • Investigator-centered goals – Use of commonly accepted or standard methods – Least burdensome (funding, ethics review, local messaging, regulatory review, peer review) • Statistical goals – Unbiased estimates of treatment effects – Rapid/efficient estimation of treatment effects (e. g. minimum error in estimation) for all arms – Accurate/efficient selection of best (non-inferior) arm – Getting the “right answer” overall • Patient-centered goals (current or future) – Accurate selection of treatment for use beyond trial – Better treatment of patients treated within the trial – Efficient evaluation of multiple treatments/combinations 16
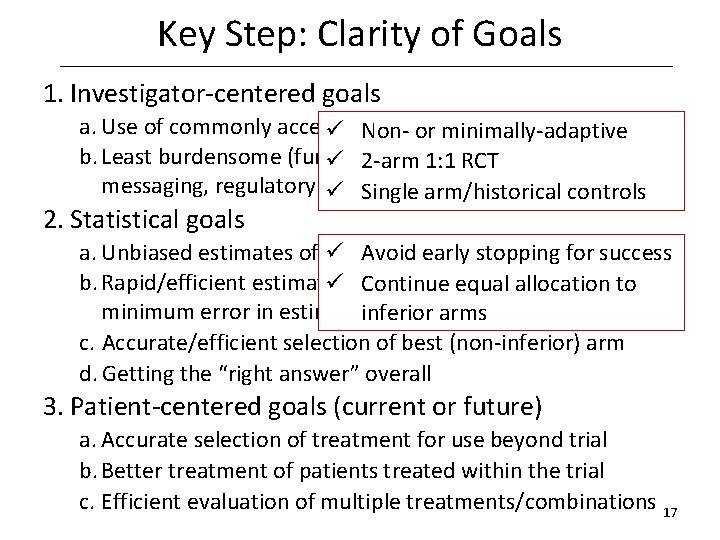
Key Step: Clarity of Goals 1. Investigator-centered goals a. Use of commonly accepted or standard methods ü Nonor minimally-adaptive b. Least burdensome (funding, ethics review, ü 2 -arm 1: 1 RCT local messaging, regulatory review, peer review) ü Single arm/historical controls 2. Statistical goals a. Unbiased estimates of treatment effects ü Avoid early stopping for success b. Rapid/efficient estimation of treatment effects (e. g. to ü Continue equal allocation minimum error in estimation) for all arms inferior arms c. Accurate/efficient selection of best (non-inferior) arm d. Getting the “right answer” overall 3. Patient-centered goals (current or future) a. Accurate selection of treatment for use beyond trial b. Better treatment of patients treated within the trial c. Efficient evaluation of multiple treatments/combinations 17
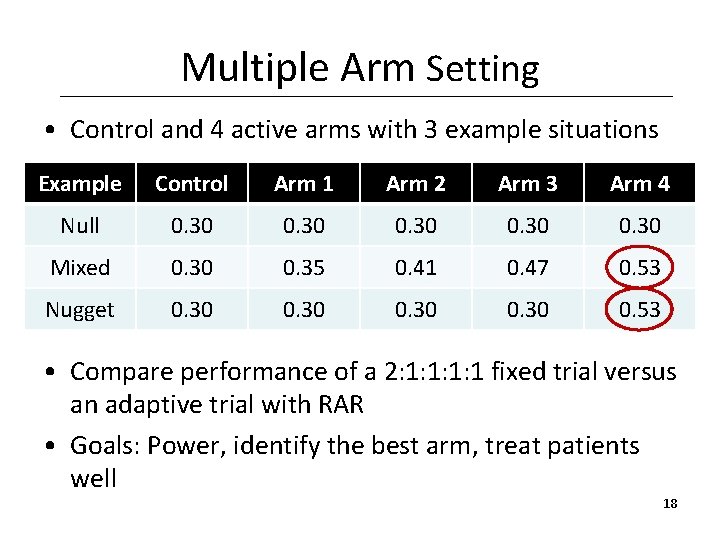
Multiple Arm Setting • Control and 4 active arms with 3 example situations Example Control Arm 1 Arm 2 Arm 3 Arm 4 Null 0. 30 Mixed 0. 30 0. 35 0. 41 0. 47 0. 53 Nugget 0. 30 0. 53 • Compare performance of a 2: 1: 1 fixed trial versus an adaptive trial with RAR • Goals: Power, identify the best arm, treat patients well 18
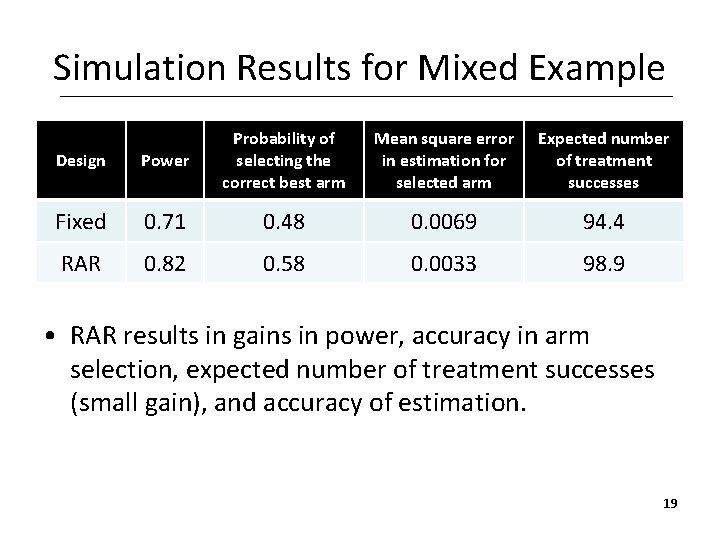
Simulation Results for Mixed Example Design Power Probability of selecting the correct best arm Mean square error in estimation for selected arm Expected number of treatment successes Fixed 0. 71 0. 48 0. 0069 94. 4 RAR 0. 82 0. 58 0. 0033 98. 9 • RAR results in gains in power, accuracy in arm selection, expected number of treatment successes (small gain), and accuracy of estimation. 19
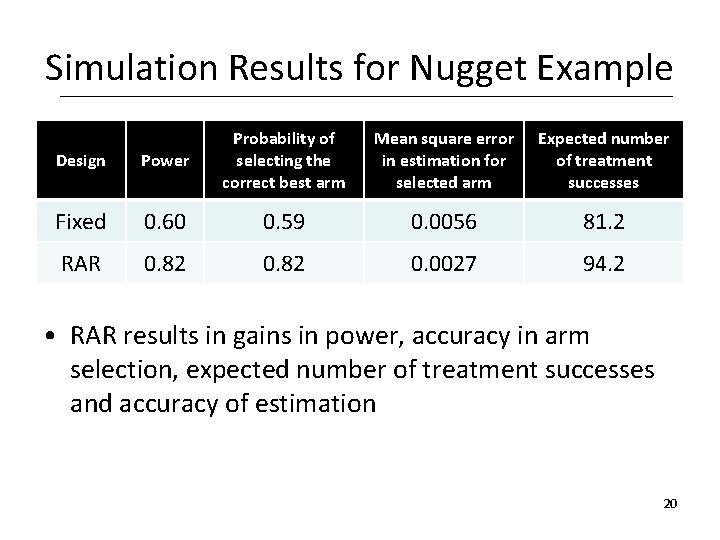
Simulation Results for Nugget Example Design Power Probability of selecting the correct best arm Mean square error in estimation for selected arm Expected number of treatment successes Fixed 0. 60 0. 59 0. 0056 81. 2 RAR 0. 82 0. 0027 94. 2 • RAR results in gains in power, accuracy in arm selection, expected number of treatment successes and accuracy of estimation 20
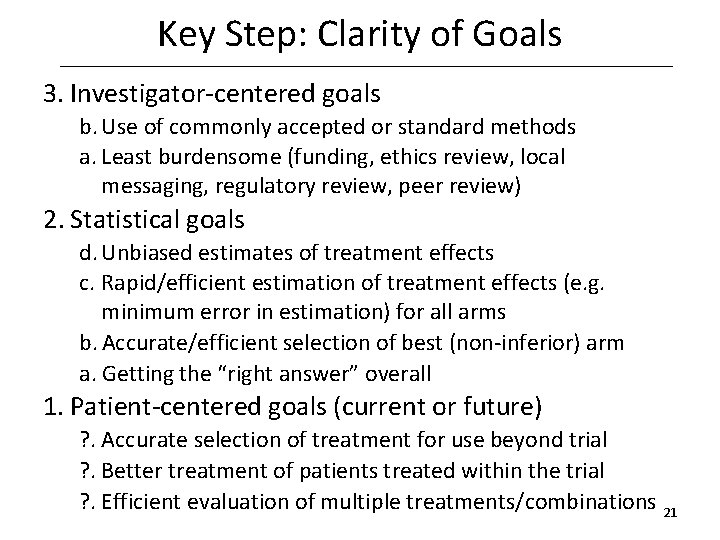
Key Step: Clarity of Goals 3. Investigator-centered goals b. Use of commonly accepted or standard methods a. Least burdensome (funding, ethics review, local messaging, regulatory review, peer review) 2. Statistical goals d. Unbiased estimates of treatment effects c. Rapid/efficient estimation of treatment effects (e. g. minimum error in estimation) for all arms b. Accurate/efficient selection of best (non-inferior) arm a. Getting the “right answer” overall 1. Patient-centered goals (current or future) ? . Accurate selection of treatment for use beyond trial ? . Better treatment of patients treated within the trial ? . Efficient evaluation of multiple treatments/combinations 21
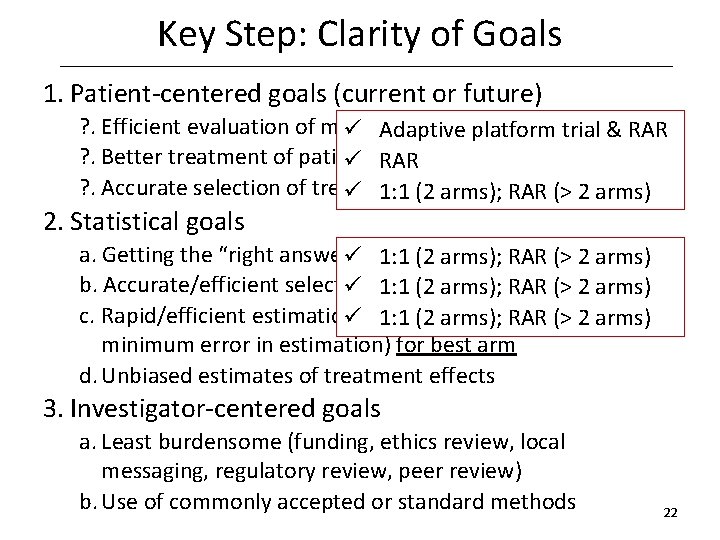
Key Step: Clarity of Goals 1. Patient-centered goals (current or future) ? . Efficient evaluation of multiple treatments/combinations ü Adaptive platform trial & RAR ? . Better treatment of patients treated within the trial ü RAR ? . Accurate selection of treatment forarms); use beyond ü 1: 1 (2 RAR (>trial 2 arms) 2. Statistical goals a. Getting the “right answer” ü overall 1: 1 (2 arms); RAR (> 2 arms) b. Accurate/efficient selection (non-inferior) ü of 1: 1 best (2 arms); RAR (> 2 arm arms) c. Rapid/efficient estimation treatment üof 1: 1 (2 arms); effects RAR (>(e. g. 2 arms) minimum error in estimation) for best arm d. Unbiased estimates of treatment effects 3. Investigator-centered goals a. Least burdensome (funding, ethics review, local messaging, regulatory review, peer review) b. Use of commonly accepted or standard methods 22
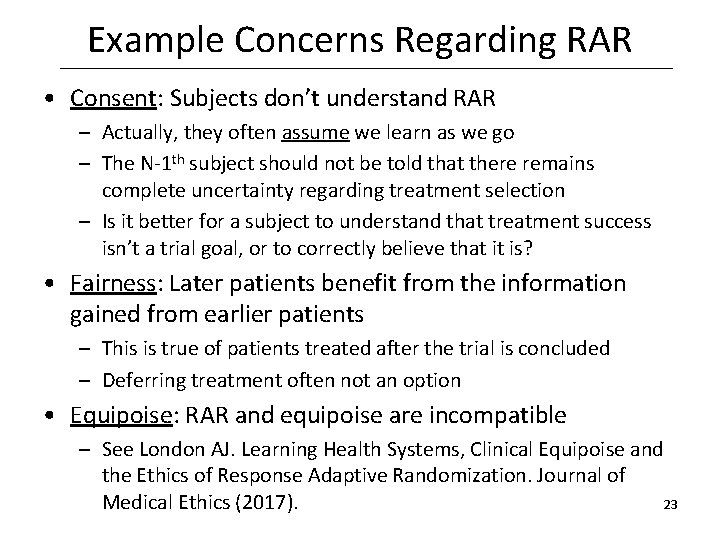
Example Concerns Regarding RAR • Consent: Subjects don’t understand RAR – Actually, they often assume we learn as we go – The N-1 th subject should not be told that there remains complete uncertainty regarding treatment selection – Is it better for a subject to understand that treatment success isn’t a trial goal, or to correctly believe that it is? • Fairness: Later patients benefit from the information gained from earlier patients – This is true of patients treated after the trial is concluded – Deferring treatment often not an option • Equipoise: RAR and equipoise are incompatible – See London AJ. Learning Health Systems, Clinical Equipoise and the Ethics of Response Adaptive Randomization. Journal of 23 Medical Ethics (2017).
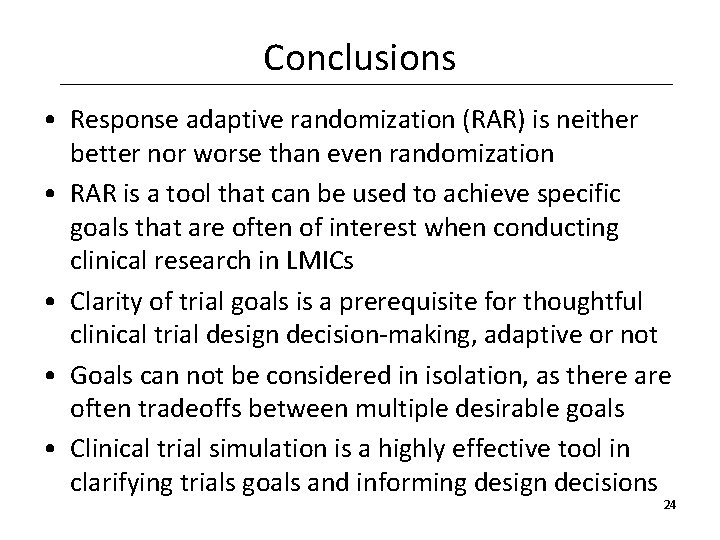
Conclusions • Response adaptive randomization (RAR) is neither better nor worse than even randomization • RAR is a tool that can be used to achieve specific goals that are often of interest when conducting clinical research in LMICs • Clarity of trial goals is a prerequisite for thoughtful clinical trial design decision-making, adaptive or not • Goals can not be considered in isolation, as there are often tradeoffs between multiple desirable goals • Clinical trial simulation is a highly effective tool in clarifying trials goals and informing design decisions 24
Audits and inspections of clinical trials
Role of statistician in clinical trials
Clinical hysteria salem witch trials
Clinical trials.gov login
Mpn clinical trials
Nida clinical trial network
Clinical trials quality by design
Mrc clinical trials unit
Iwr clinical trial
Clinicaltrials gov prs
Site initiation visit ppt
Ohsu clinical trials office
Mpn clinical trials
Stratified randomization
York trials unit
Clinical trials
Clinicaltrials.gov api
Dhl atyrau
Clinical trial prs
Phs human subjects and clinical trials information
Difference between informing and persuading
Informing science institute
Csr and business ethics
Ethical decision making and ethical leadership
Perbedaan ethical dilemma dan ethical lapse