Hypothesis testing Hypothesis testing Want to know something
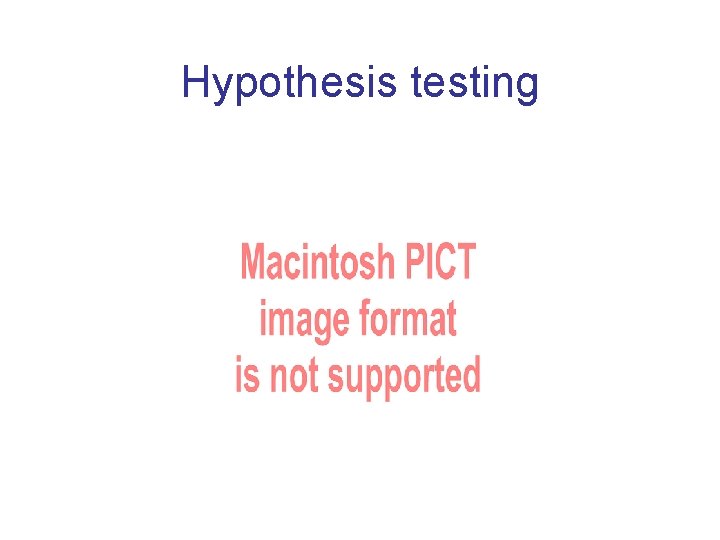
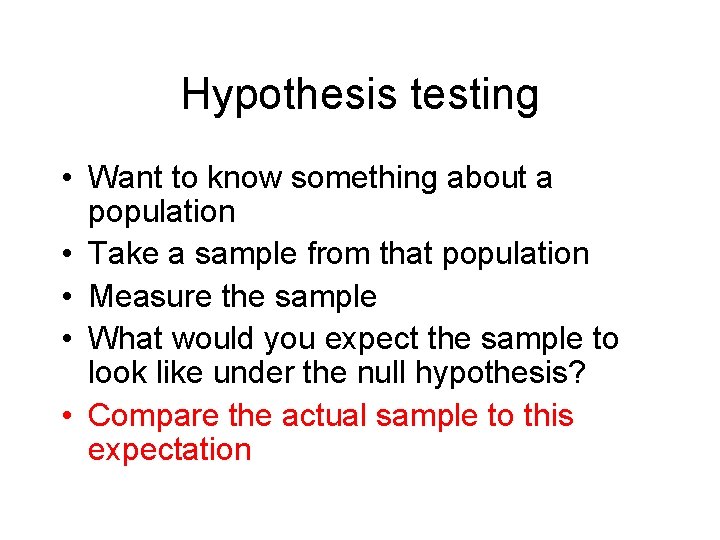
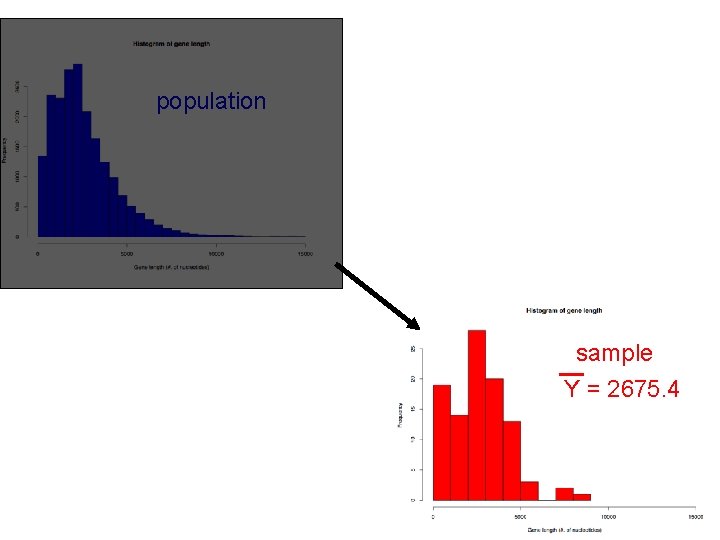
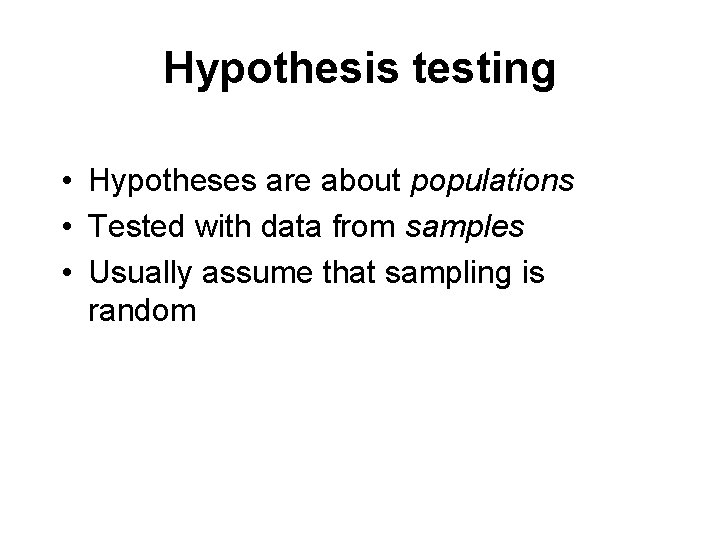
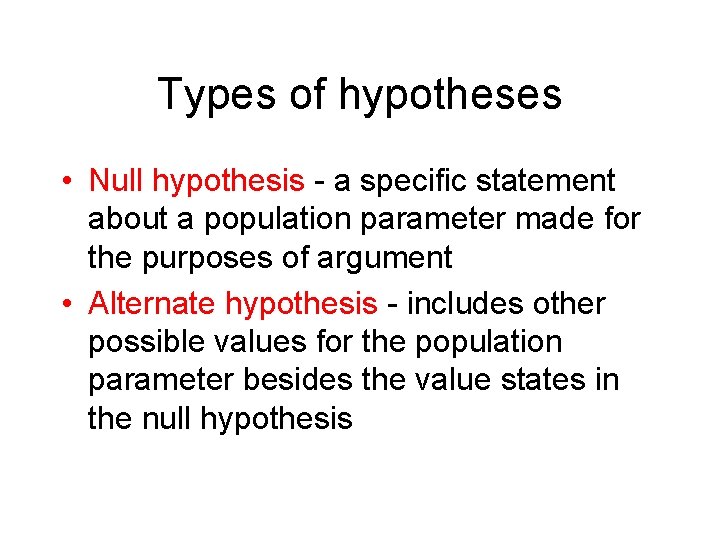
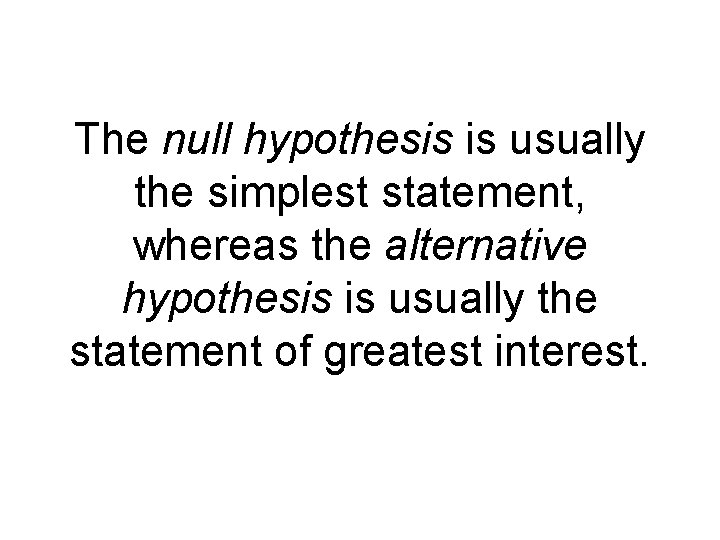
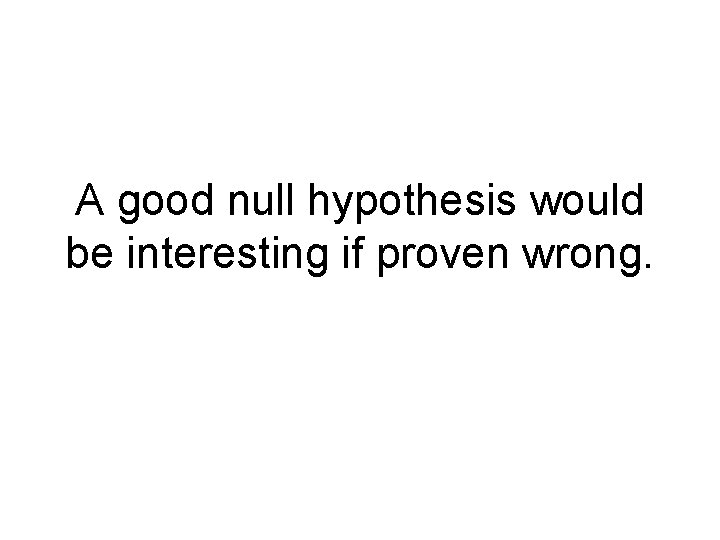
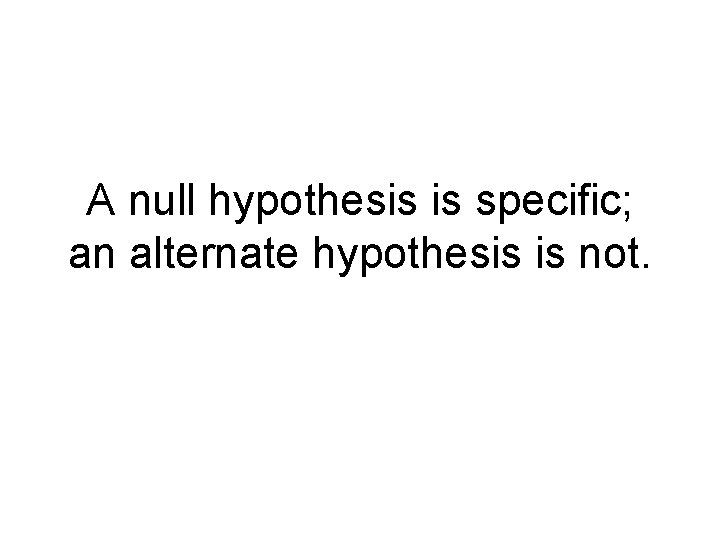
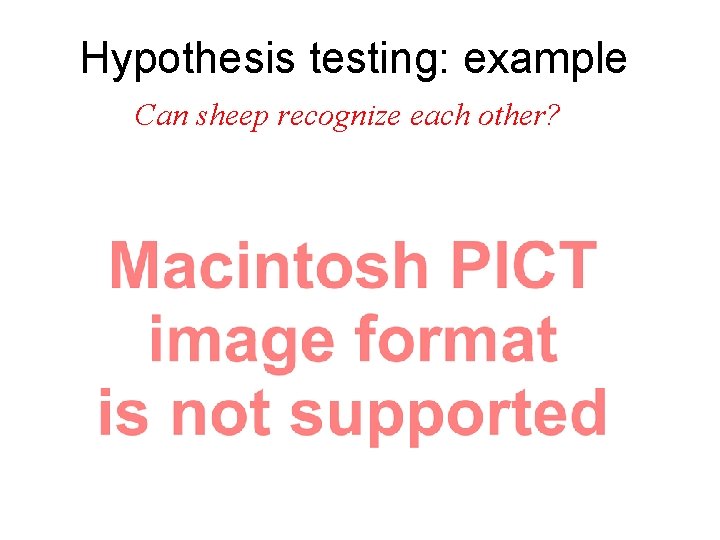
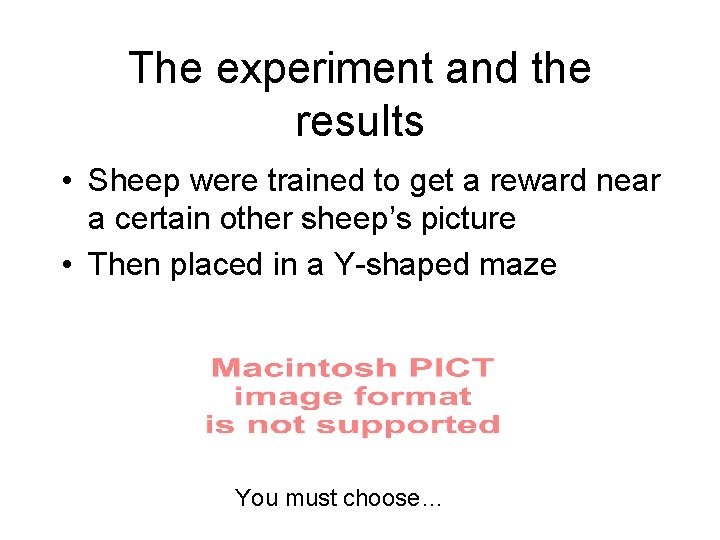
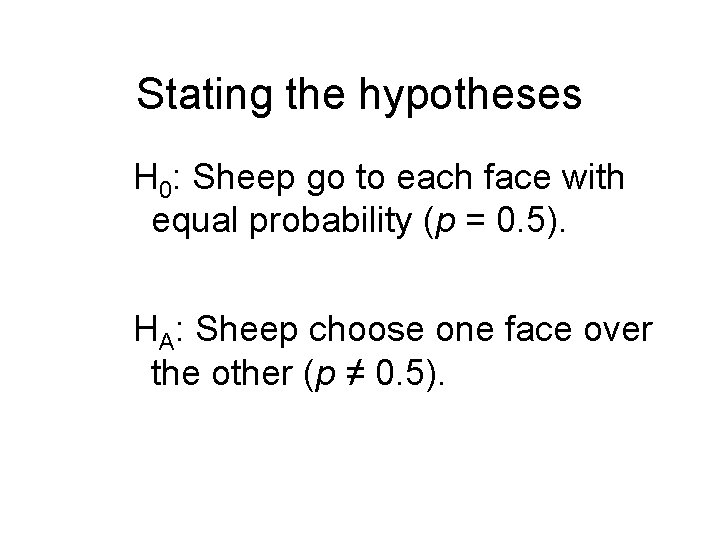
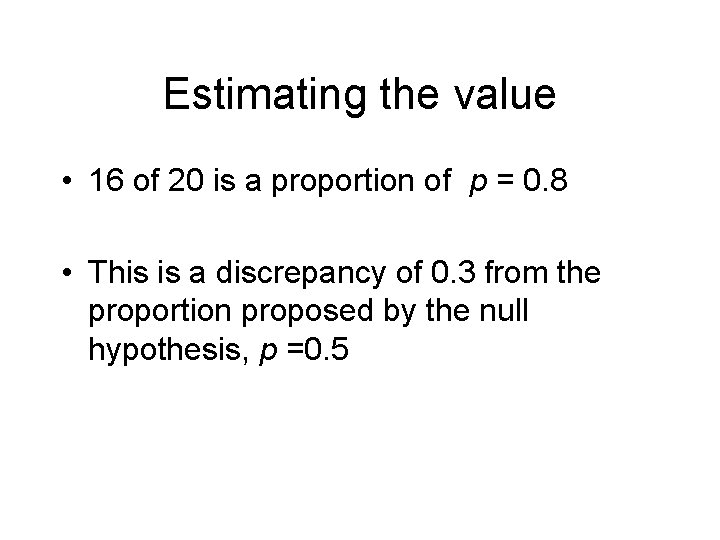
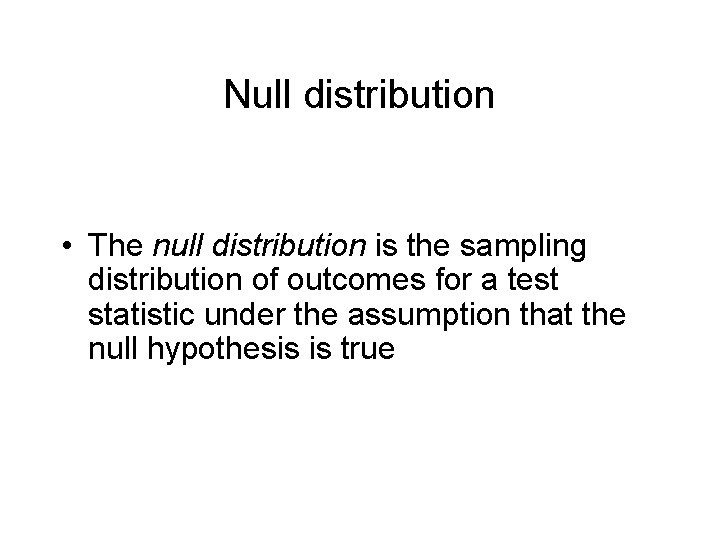
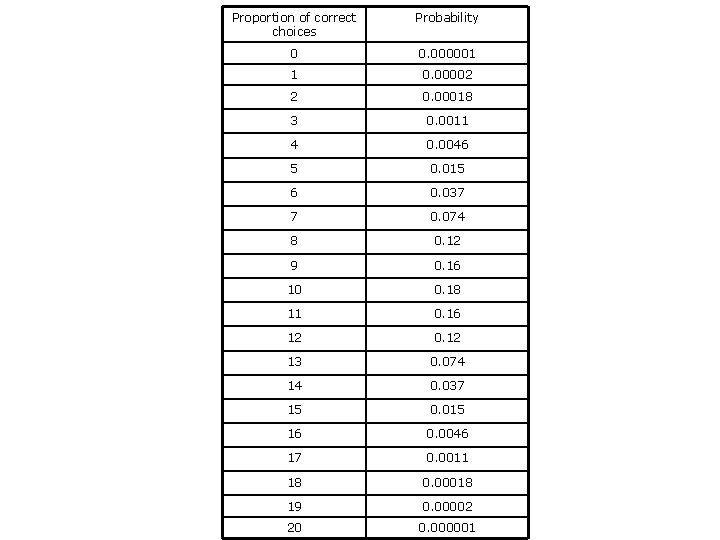
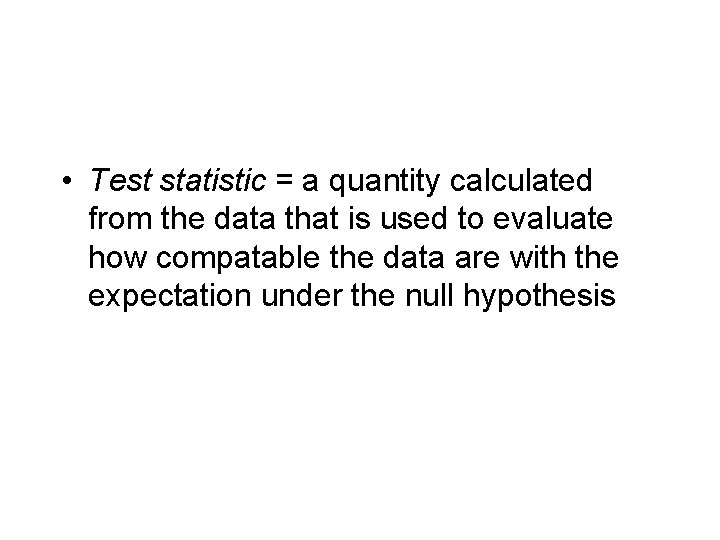
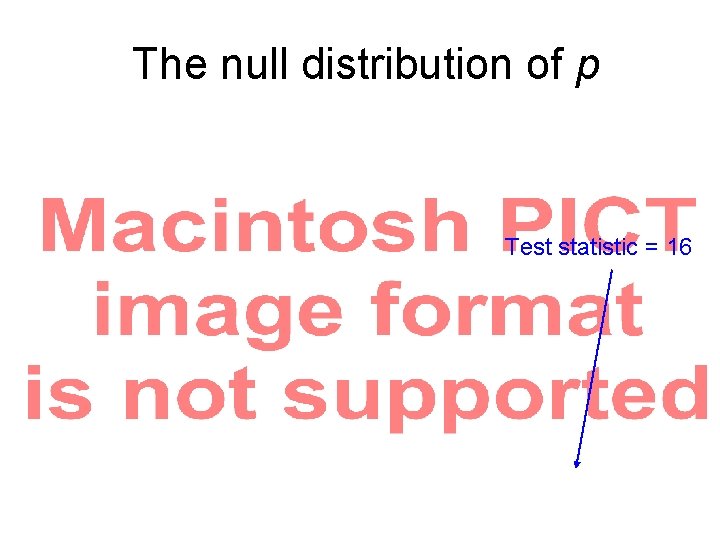
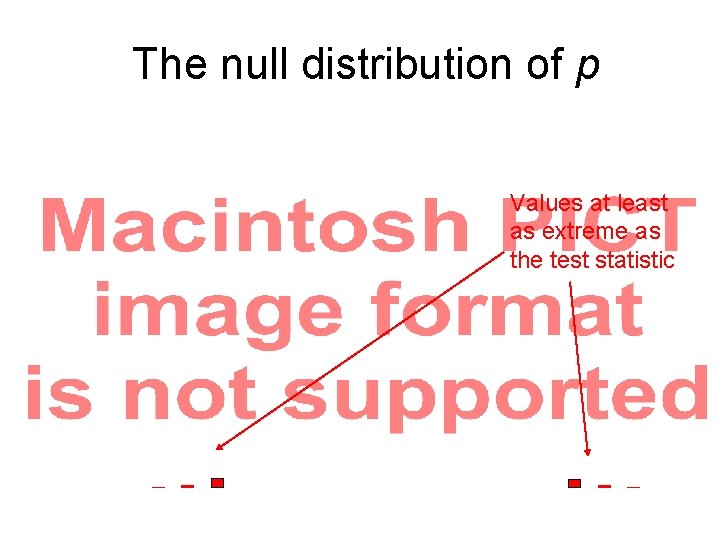
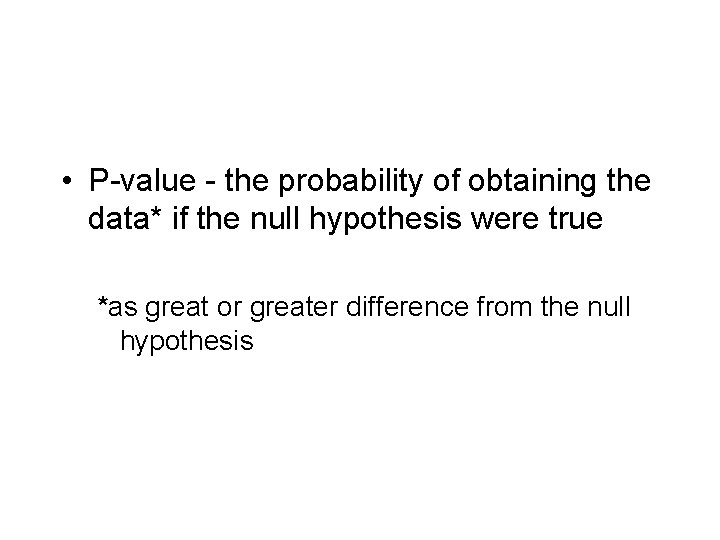
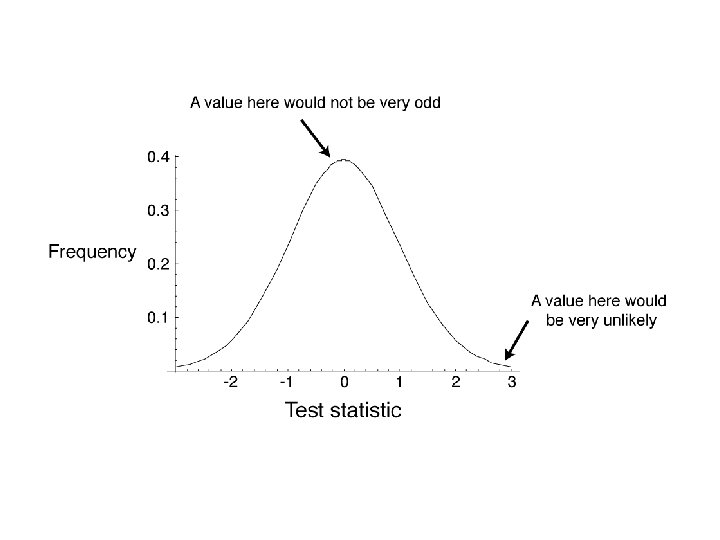
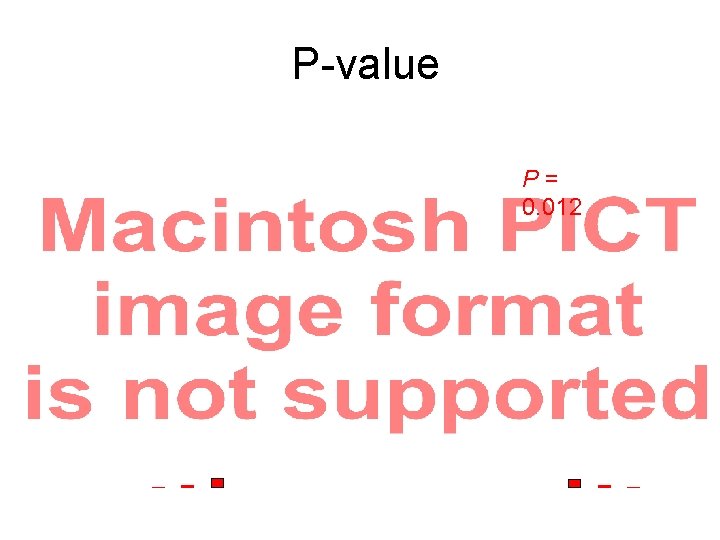
![P-value calculation P =2*(Pr[16]+Pr[17]+Pr[18]+Pr[19]+Pr[20]) =2*(0. 005+0. 001+0. 0002+0. 000001) = 0. 012 P-value calculation P =2*(Pr[16]+Pr[17]+Pr[18]+Pr[19]+Pr[20]) =2*(0. 005+0. 001+0. 0002+0. 000001) = 0. 012](https://slidetodoc.com/presentation_image_h/334d8d30b30d20e7500e7000e6eb8a74/image-21.jpg)
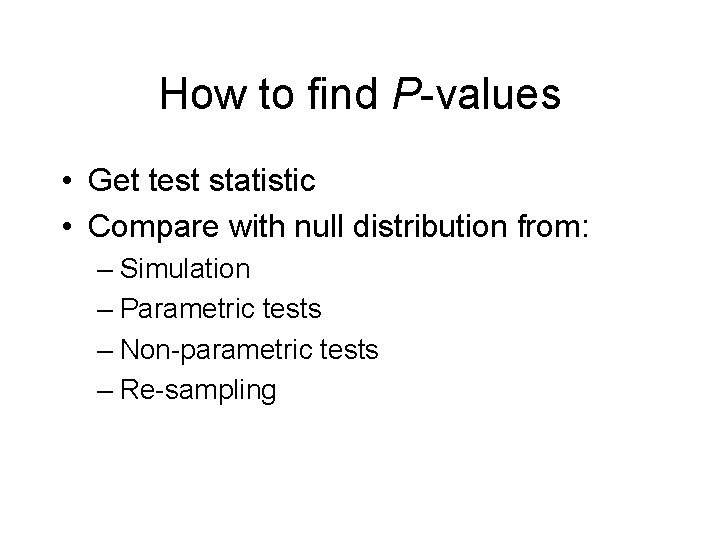
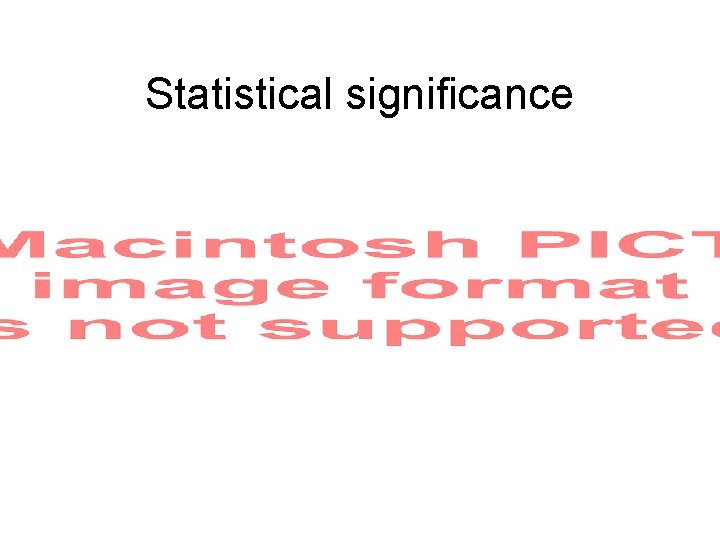
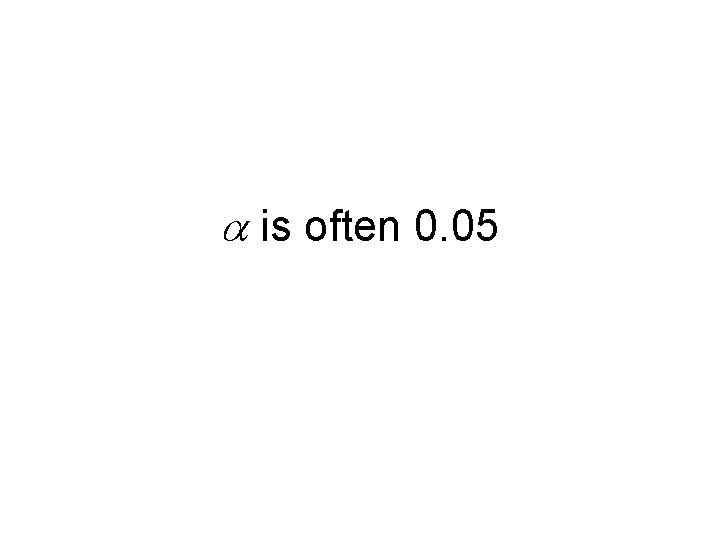
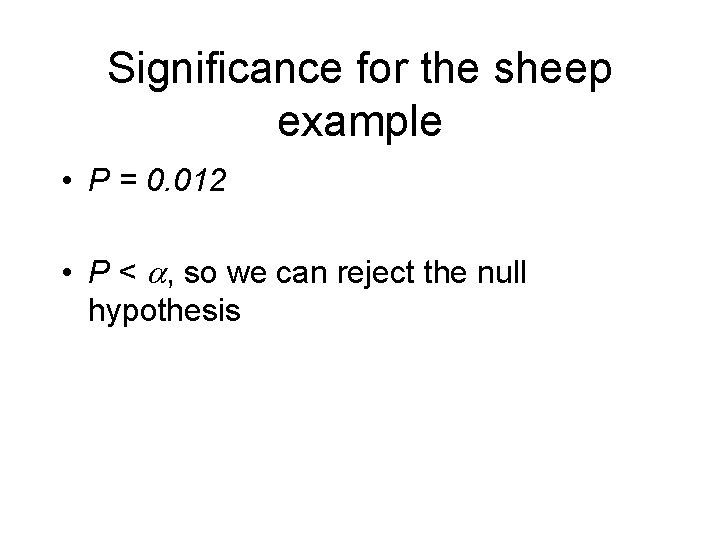
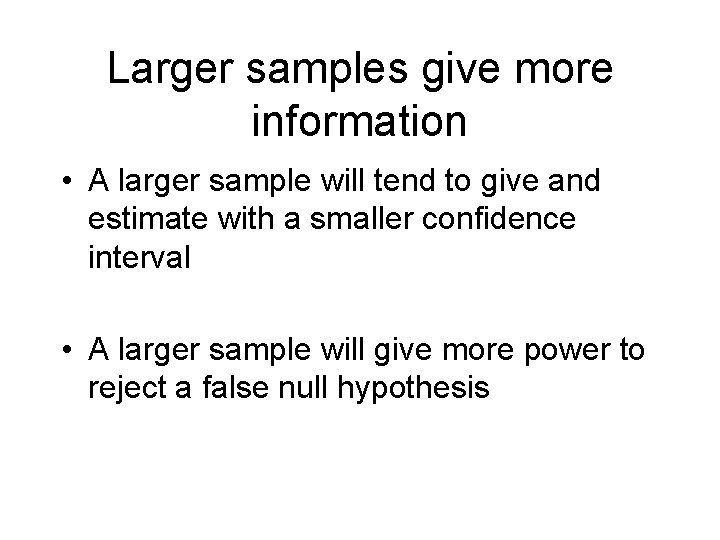
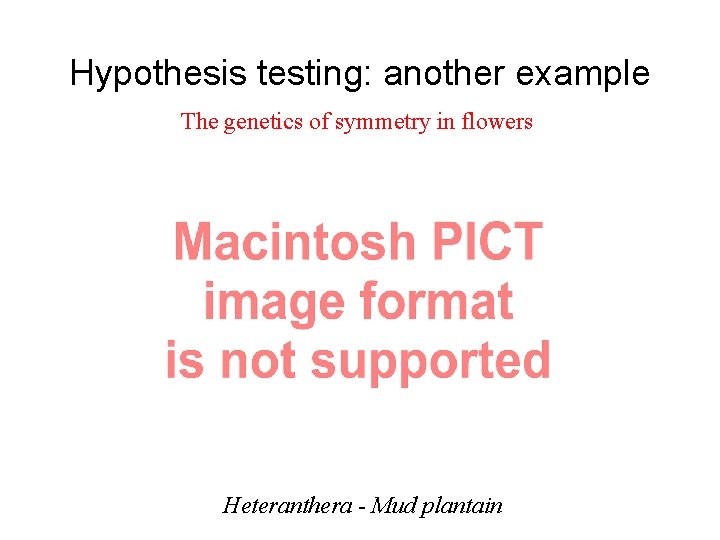
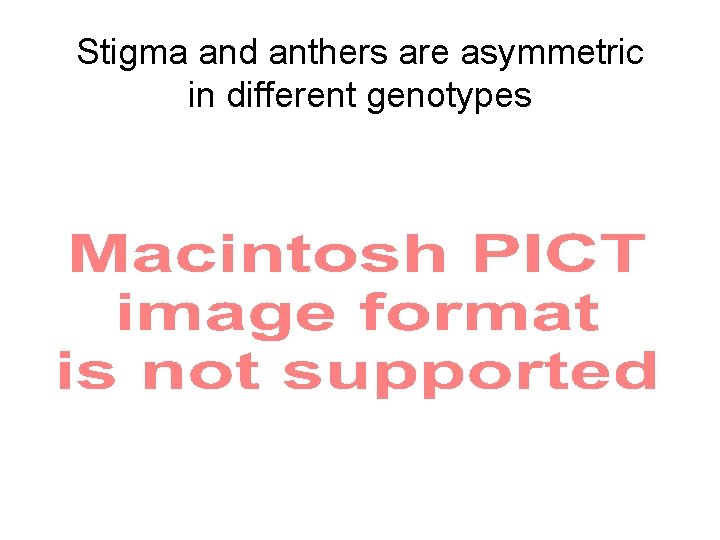
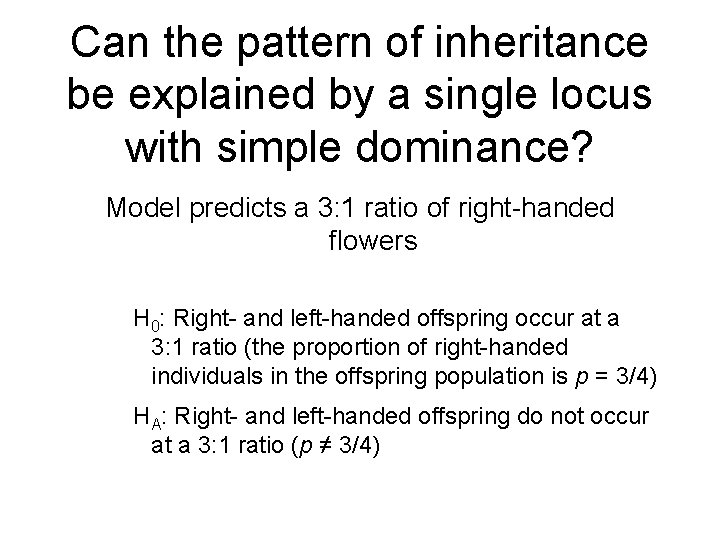
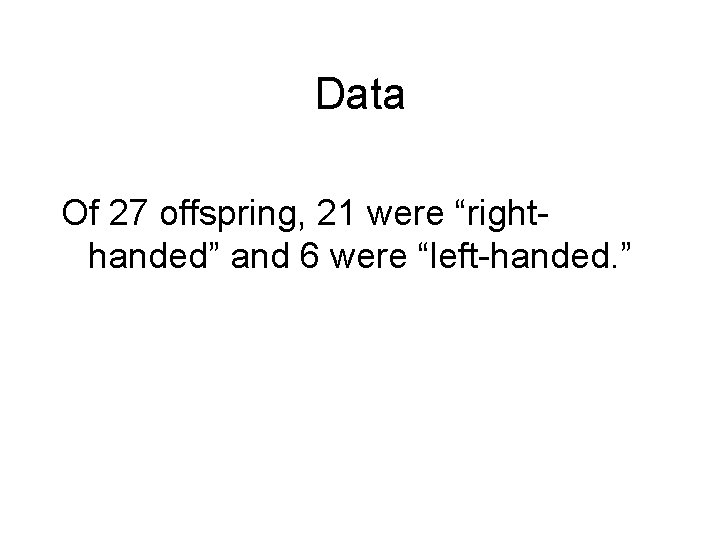
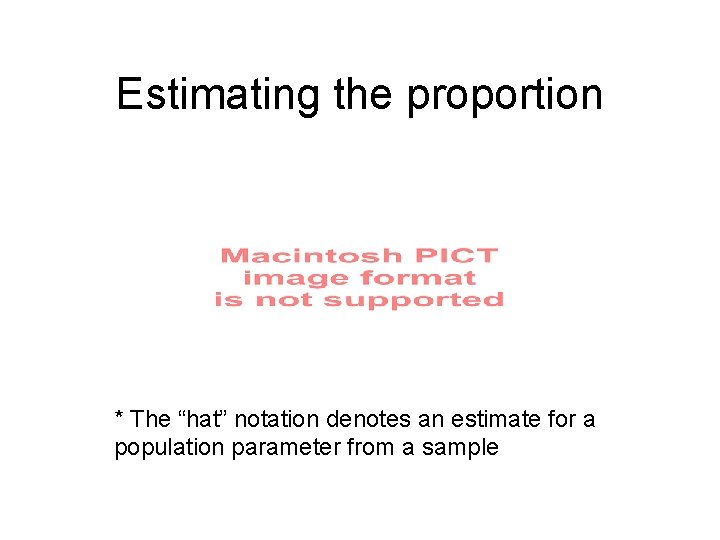
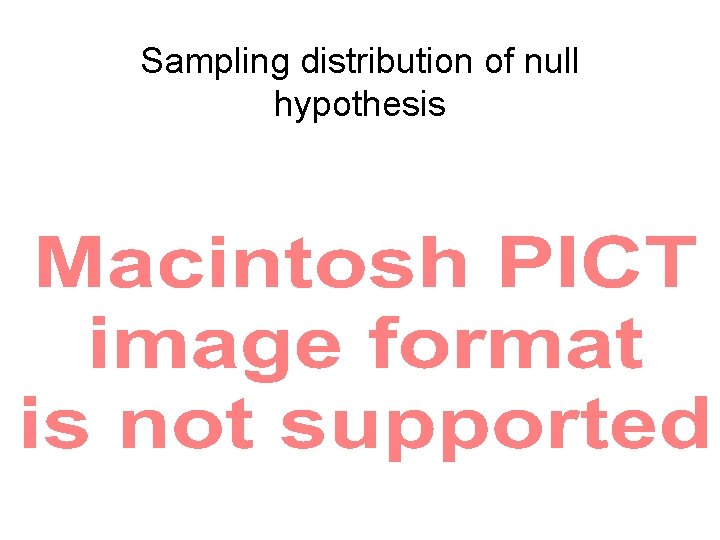
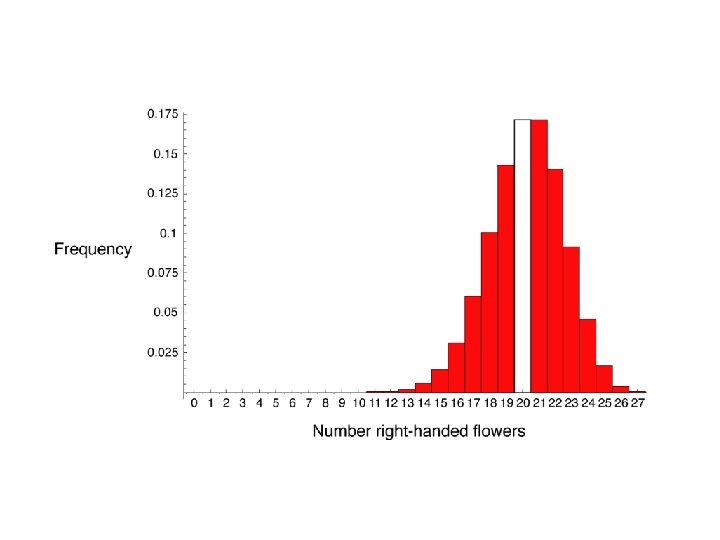
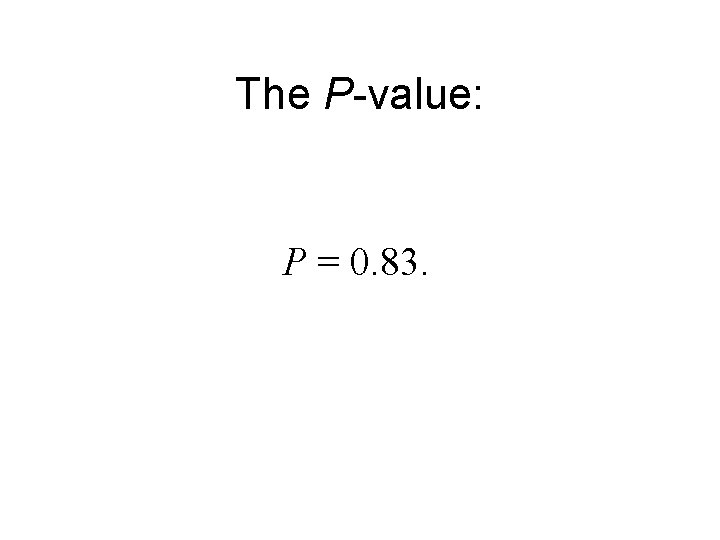
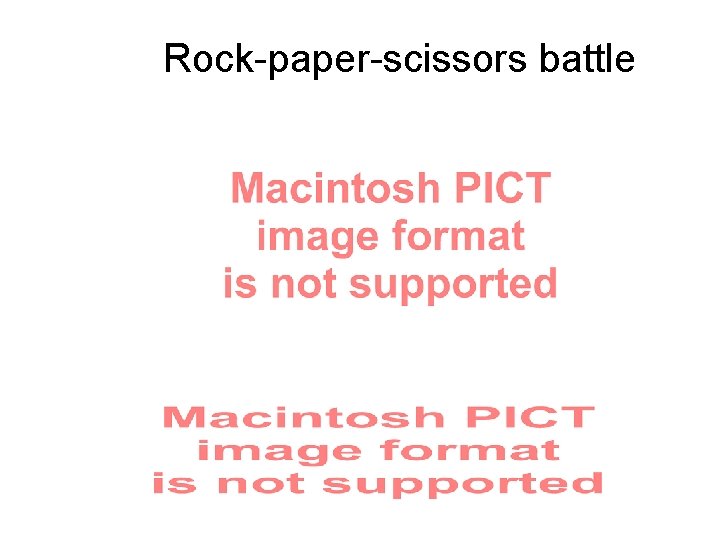
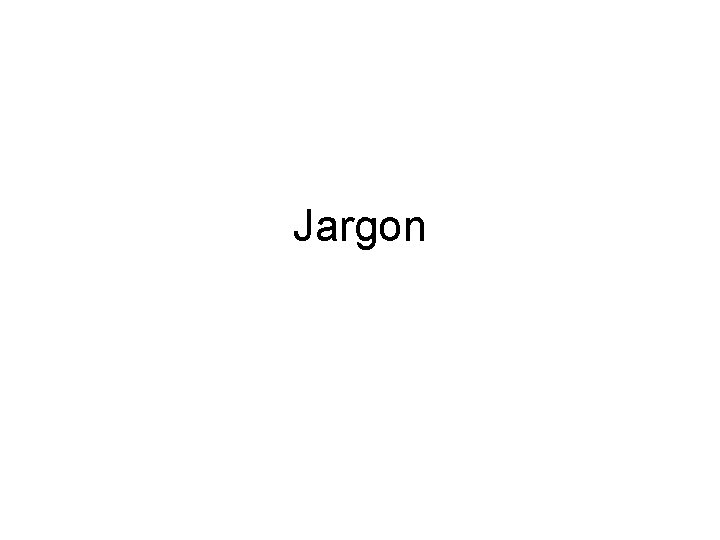
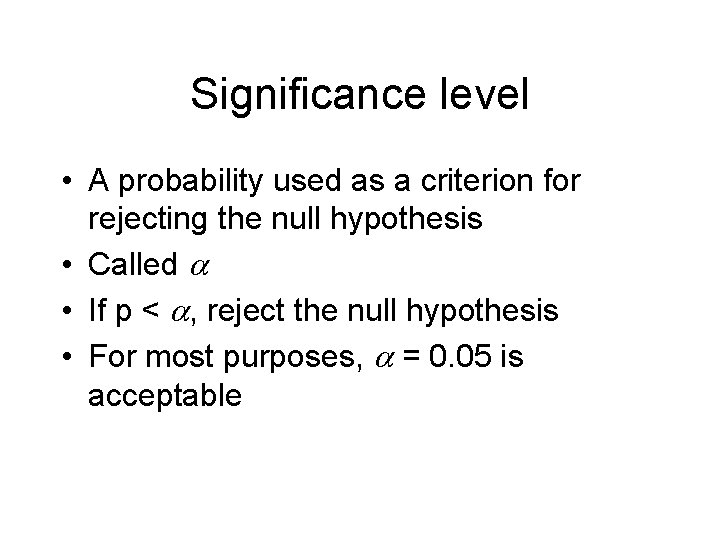
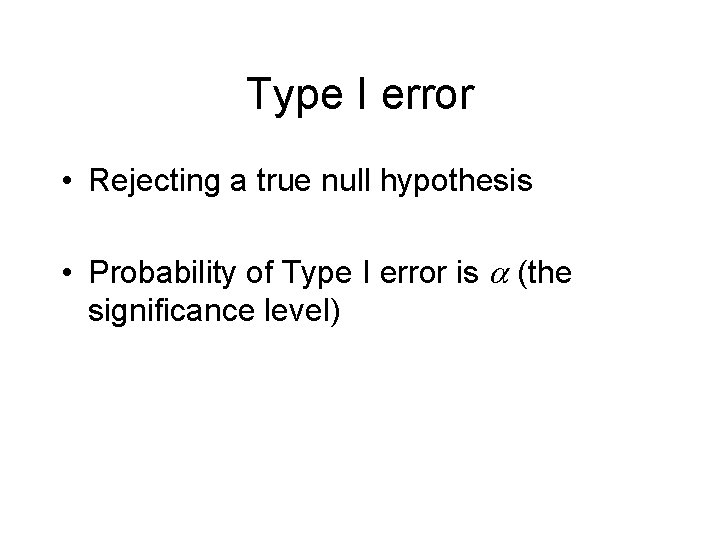
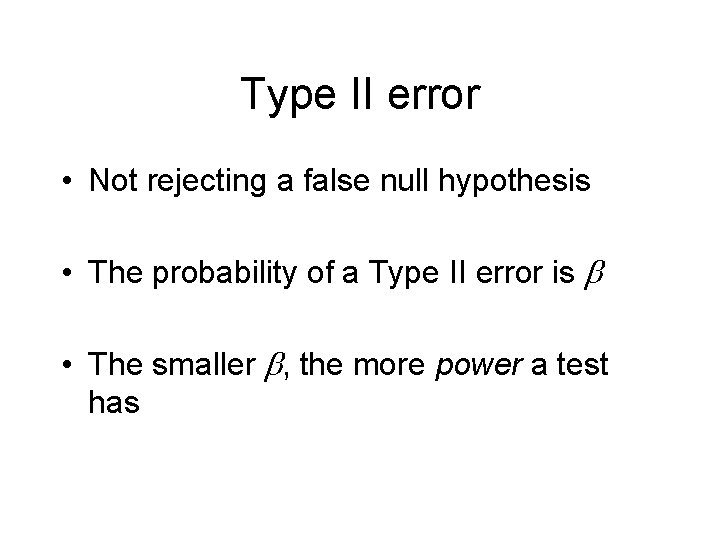
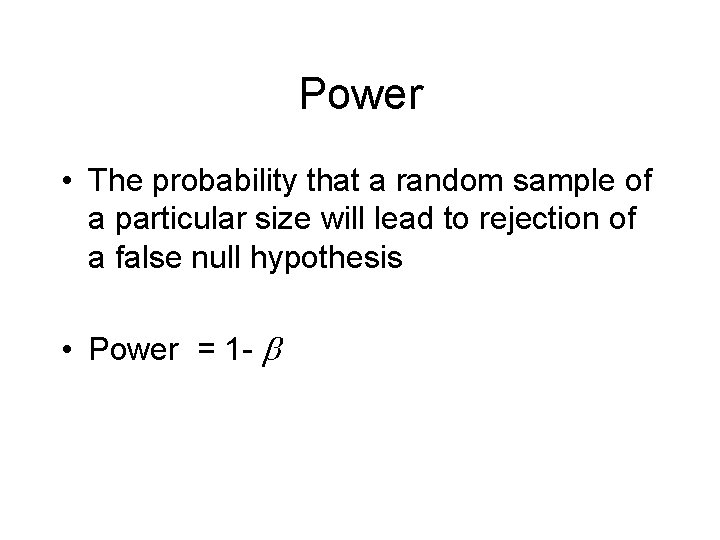
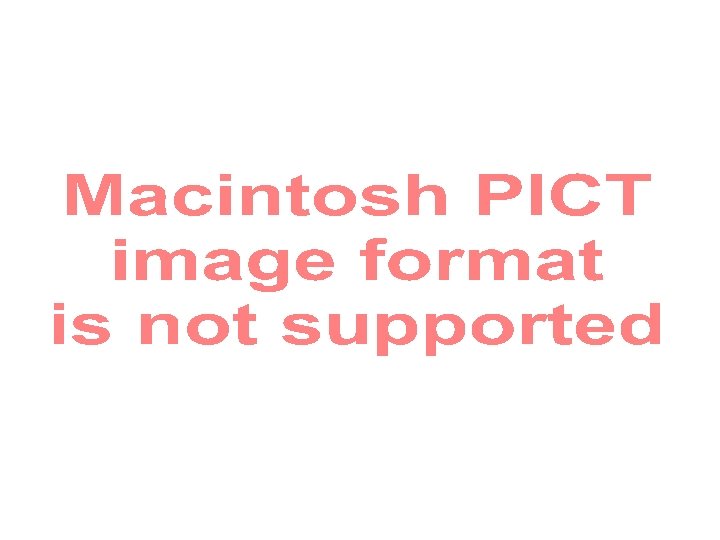
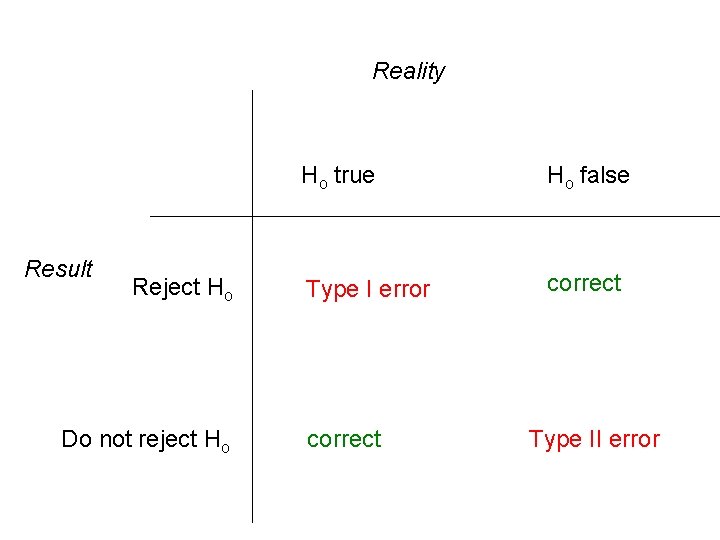
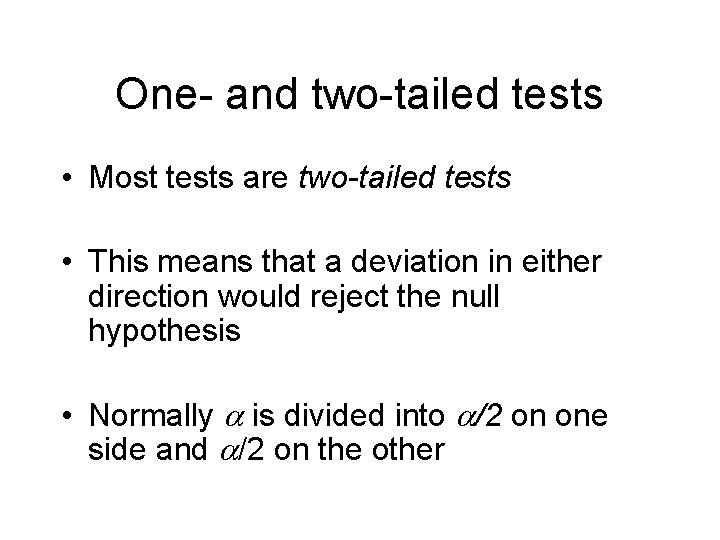
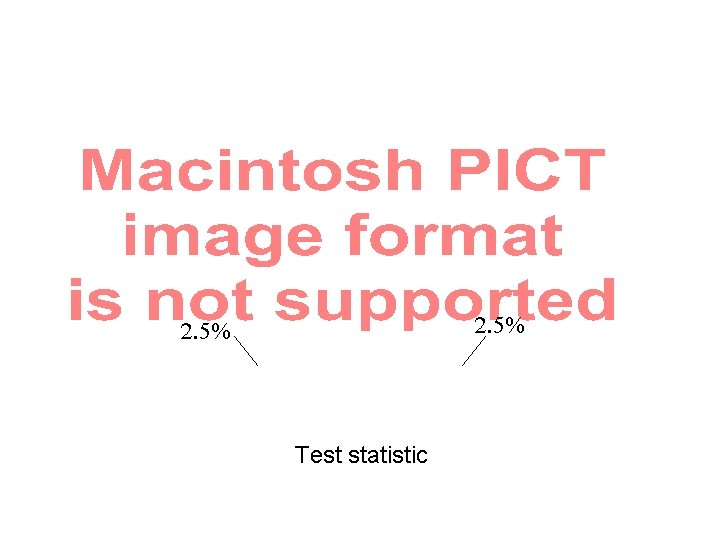
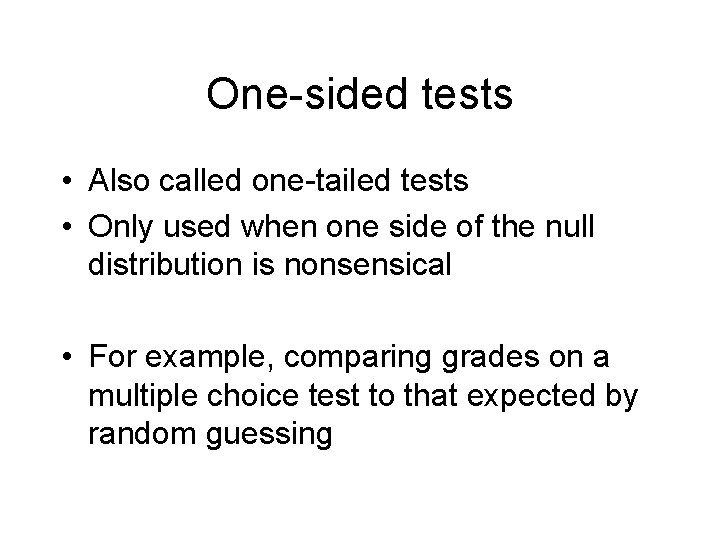
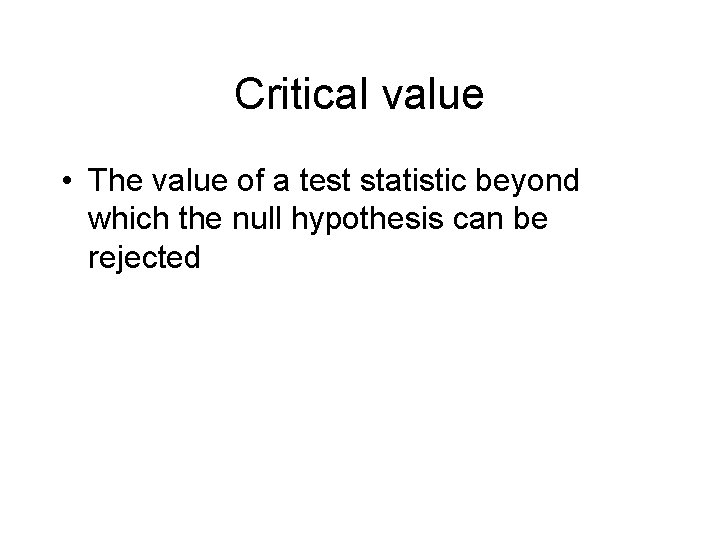
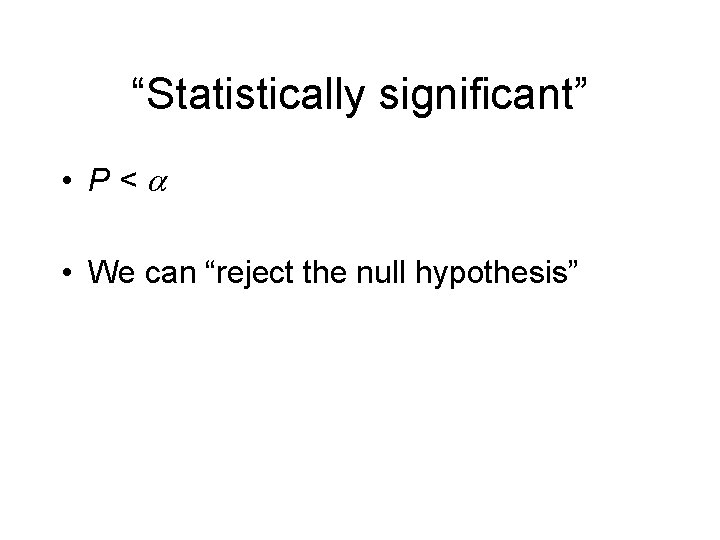
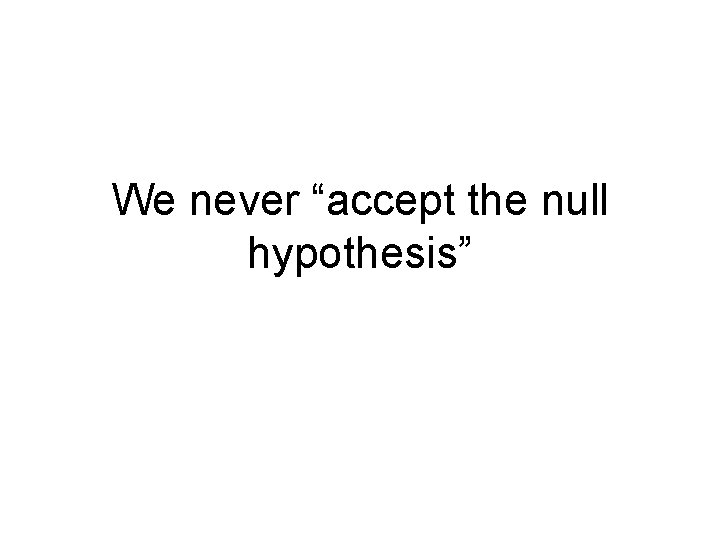
- Slides: 48
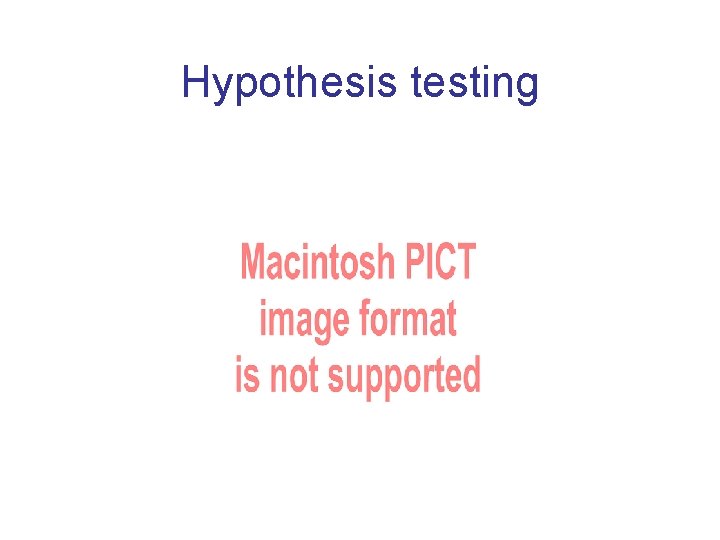
Hypothesis testing
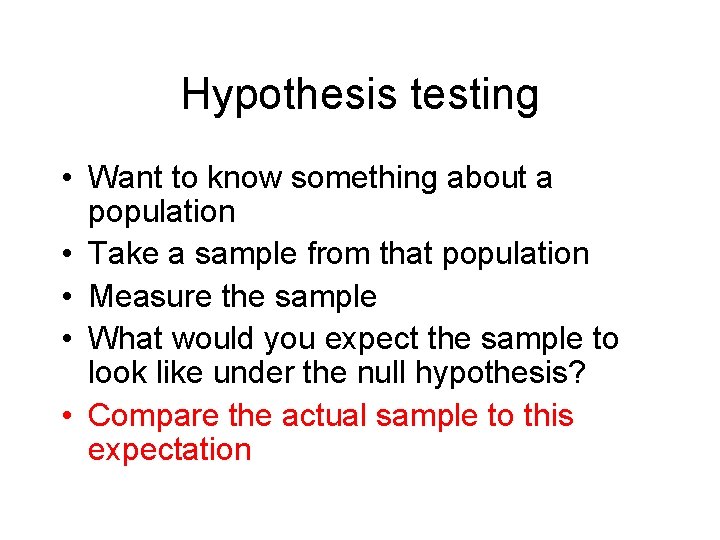
Hypothesis testing • Want to know something about a population • Take a sample from that population • Measure the sample • What would you expect the sample to look like under the null hypothesis? • Compare the actual sample to this expectation
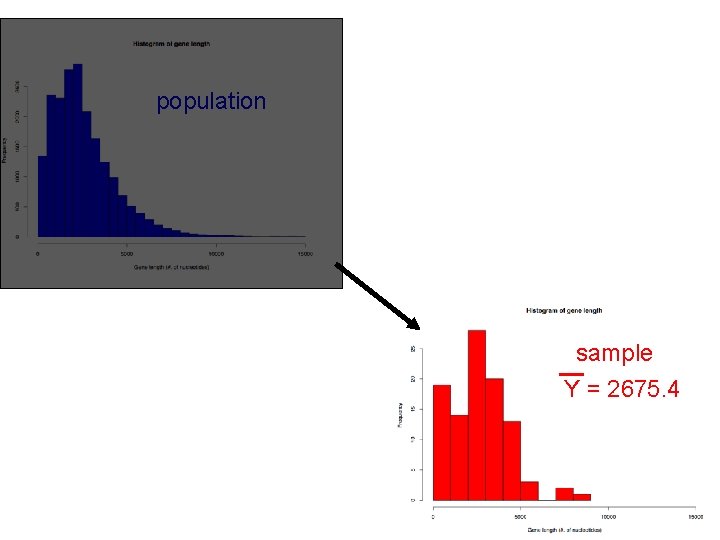
population sample Y = 2675. 4
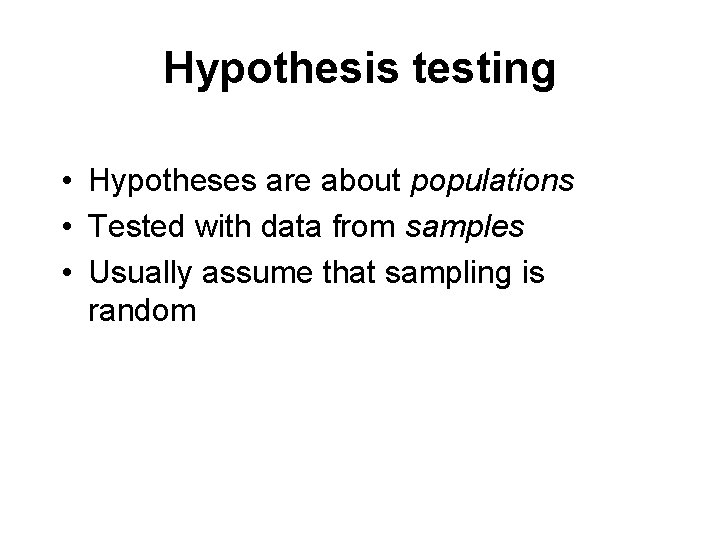
Hypothesis testing • Hypotheses are about populations • Tested with data from samples • Usually assume that sampling is random
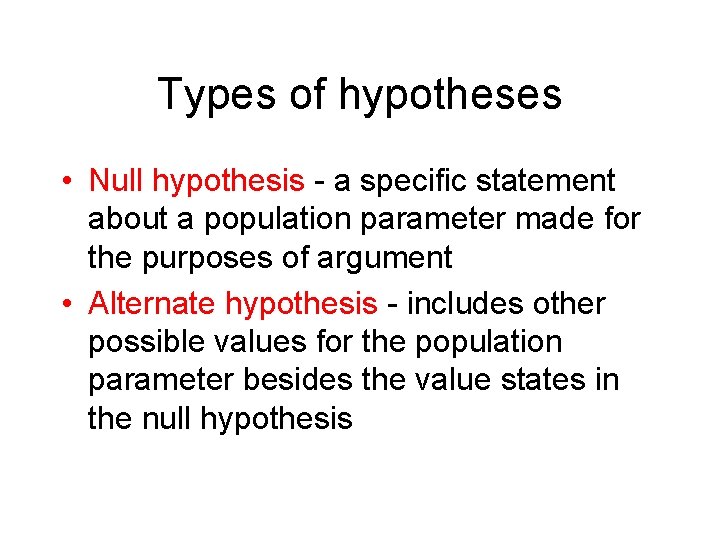
Types of hypotheses • Null hypothesis - a specific statement about a population parameter made for the purposes of argument • Alternate hypothesis - includes other possible values for the population parameter besides the value states in the null hypothesis
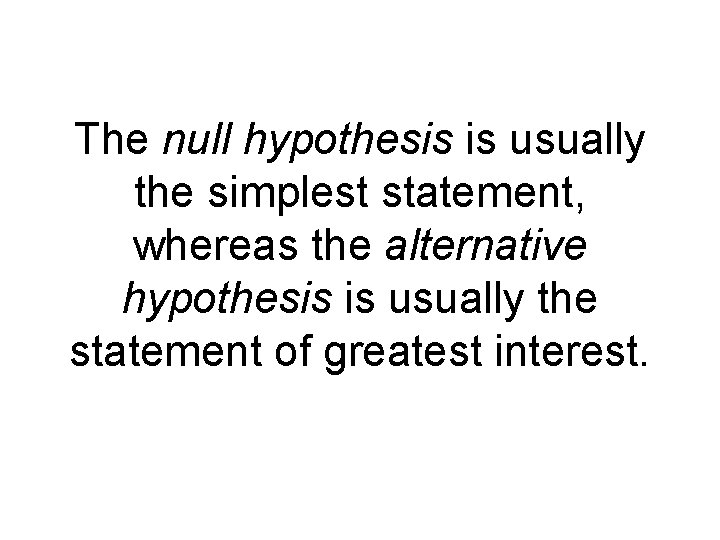
The null hypothesis is usually the simplest statement, whereas the alternative hypothesis is usually the statement of greatest interest.
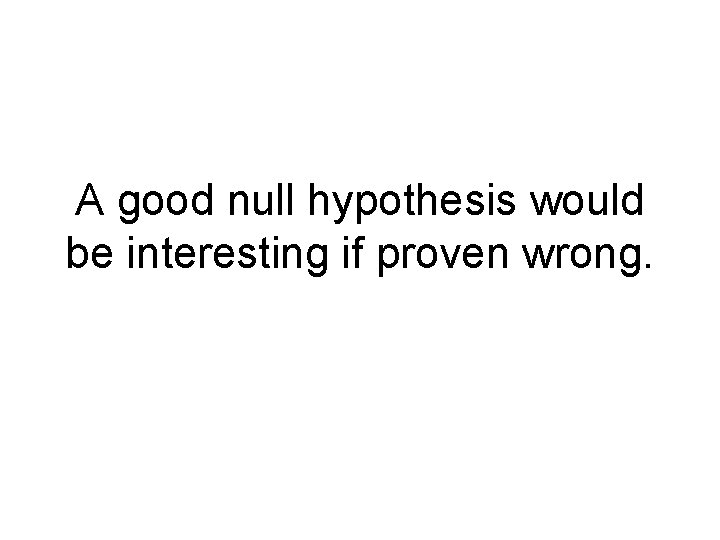
A good null hypothesis would be interesting if proven wrong.
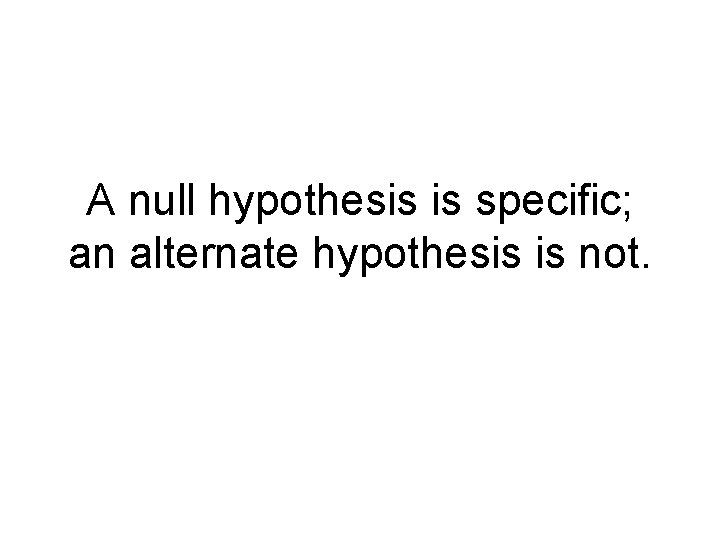
A null hypothesis is specific; an alternate hypothesis is not.
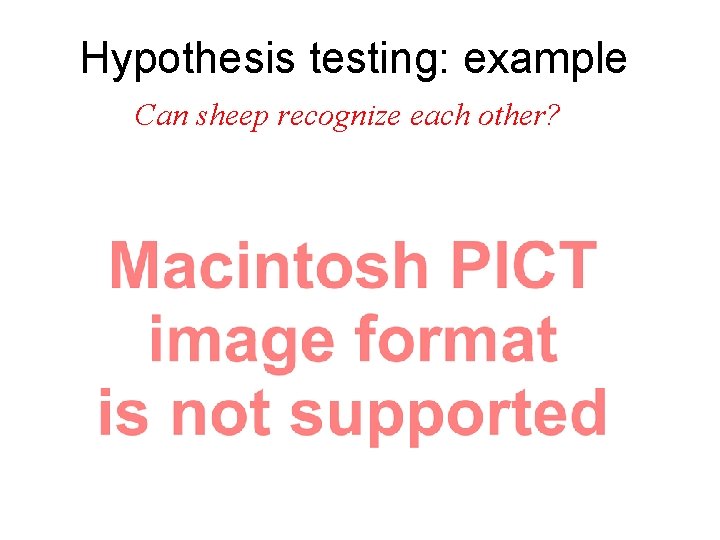
Hypothesis testing: example Can sheep recognize each other?
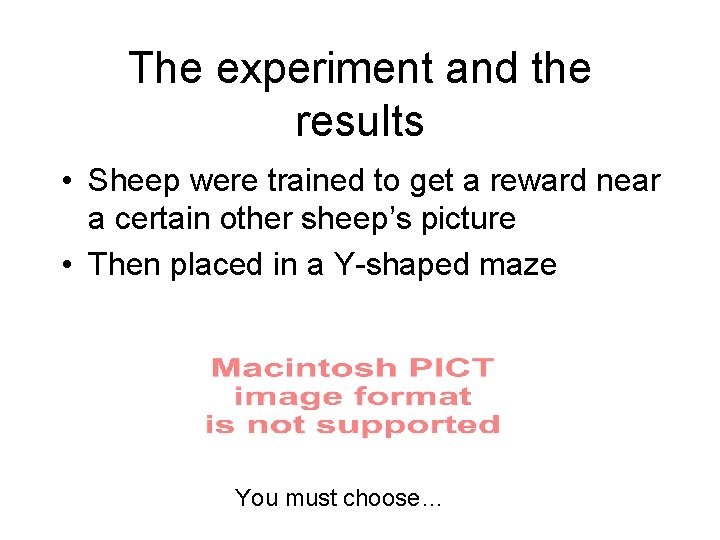
The experiment and the results • Sheep were trained to get a reward near a certain other sheep’s picture • Then placed in a Y-shaped maze You must choose…
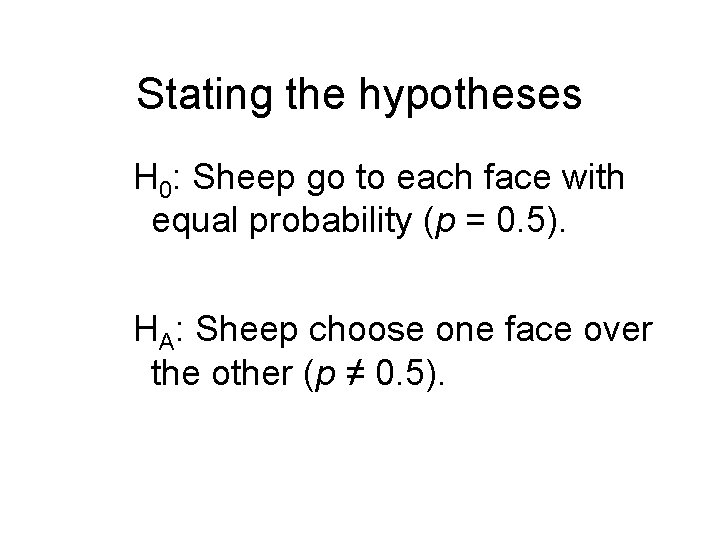
Stating the hypotheses H 0: Sheep go to each face with equal probability (p = 0. 5). HA: Sheep choose one face over the other (p ≠ 0. 5).
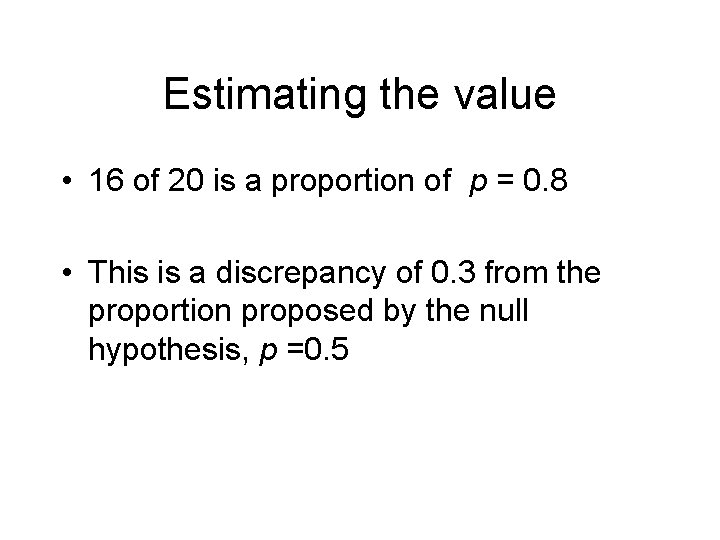
Estimating the value • 16 of 20 is a proportion of p = 0. 8 • This is a discrepancy of 0. 3 from the proportion proposed by the null hypothesis, p =0. 5
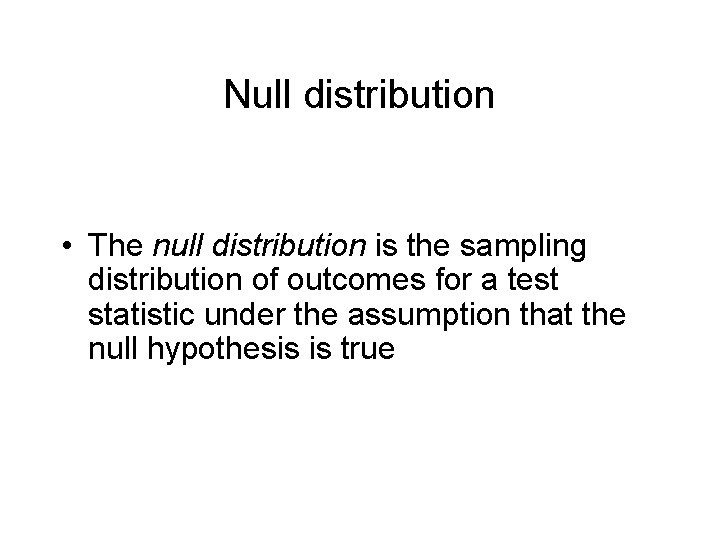
Null distribution • The null distribution is the sampling distribution of outcomes for a test statistic under the assumption that the null hypothesis is true
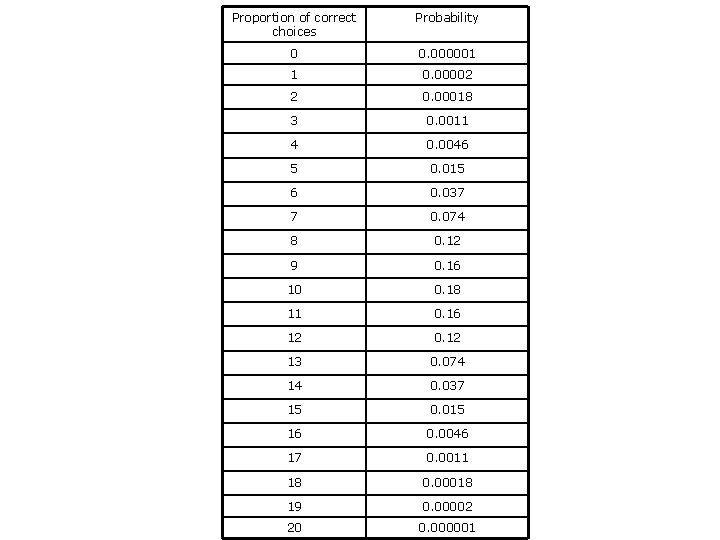
Proportion of correct choices Probability 0 0. 000001 1 0. 00002 2 0. 00018 3 0. 0011 4 0. 0046 5 0. 015 6 0. 037 7 0. 074 8 0. 12 9 0. 16 10 0. 18 11 0. 16 12 0. 12 13 0. 074 14 0. 037 15 0. 015 16 0. 0046 17 0. 0011 18 0. 00018 19 0. 00002 20 0. 000001
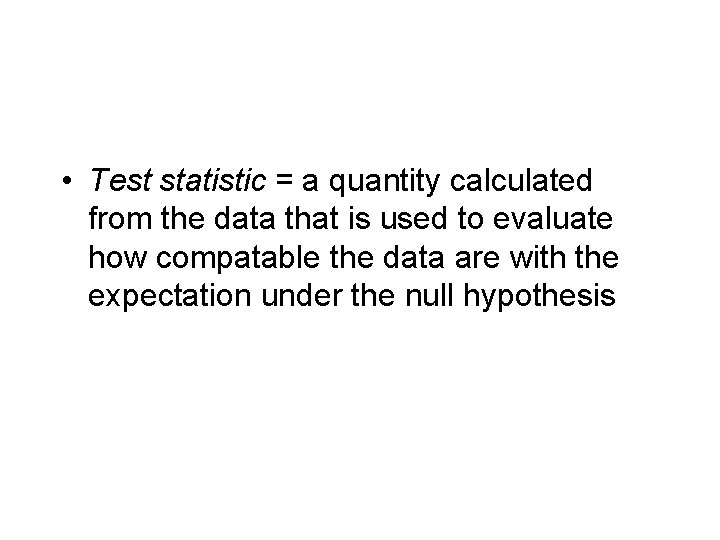
• Test statistic = a quantity calculated from the data that is used to evaluate how compatable the data are with the expectation under the null hypothesis
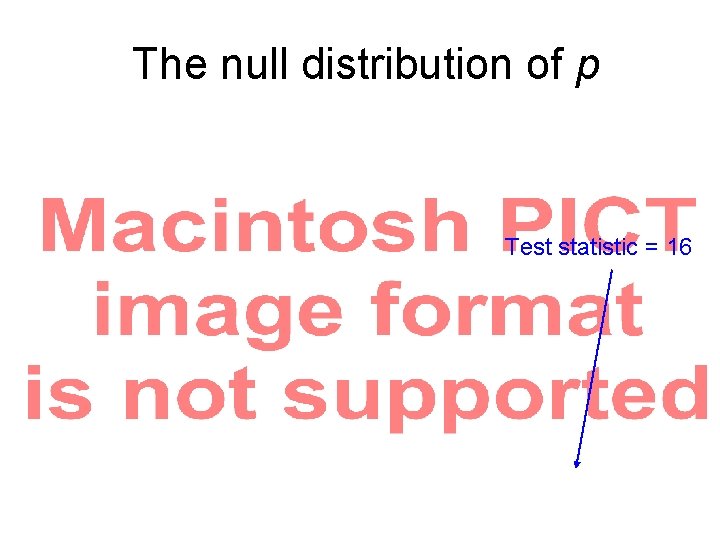
The null distribution of p Test statistic = 16
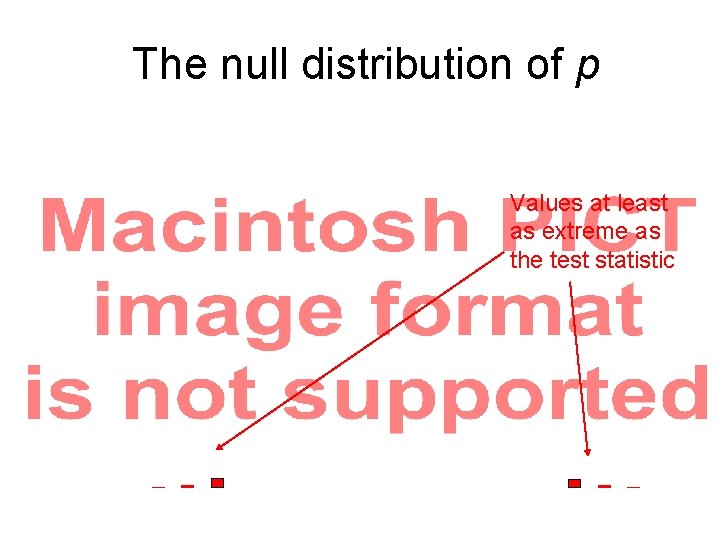
The null distribution of p Values at least as extreme as the test statistic
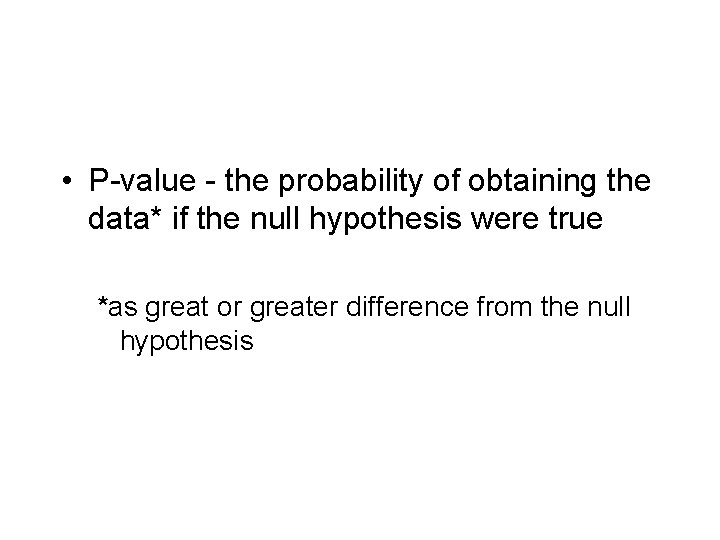
• P-value - the probability of obtaining the data* if the null hypothesis were true *as great or greater difference from the null hypothesis
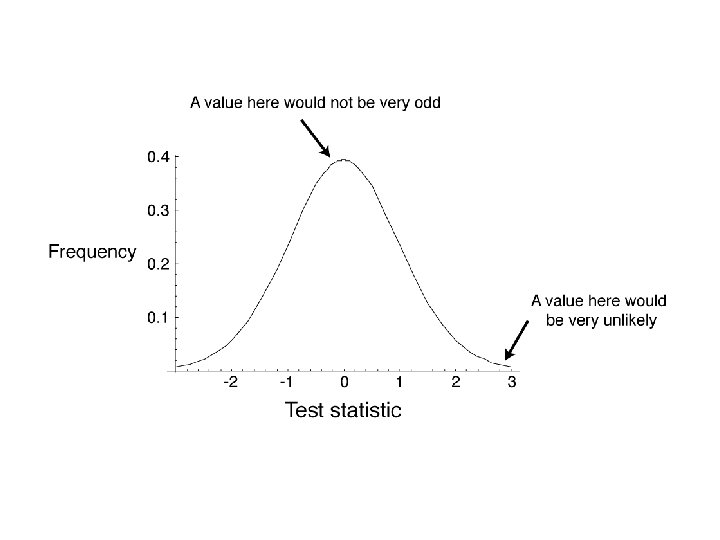
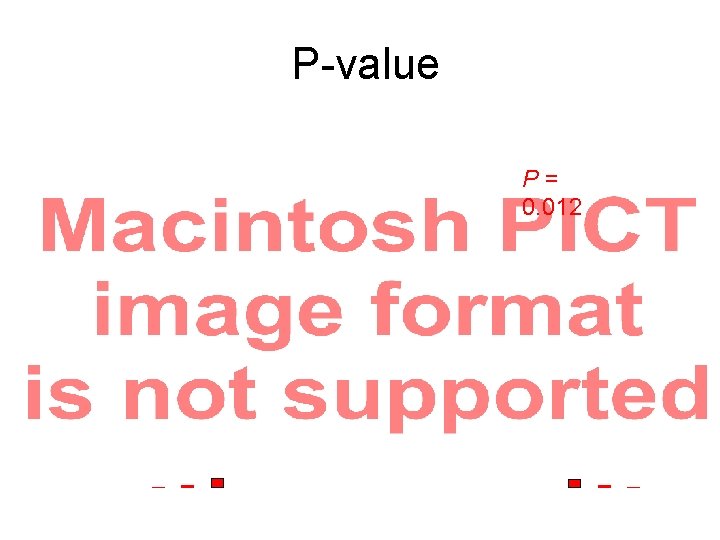
P-value P= 0. 012
![Pvalue calculation P 2Pr16Pr17Pr18Pr19Pr20 20 0050 0010 00020 000001 0 012 P-value calculation P =2*(Pr[16]+Pr[17]+Pr[18]+Pr[19]+Pr[20]) =2*(0. 005+0. 001+0. 0002+0. 000001) = 0. 012](https://slidetodoc.com/presentation_image_h/334d8d30b30d20e7500e7000e6eb8a74/image-21.jpg)
P-value calculation P =2*(Pr[16]+Pr[17]+Pr[18]+Pr[19]+Pr[20]) =2*(0. 005+0. 001+0. 0002+0. 000001) = 0. 012
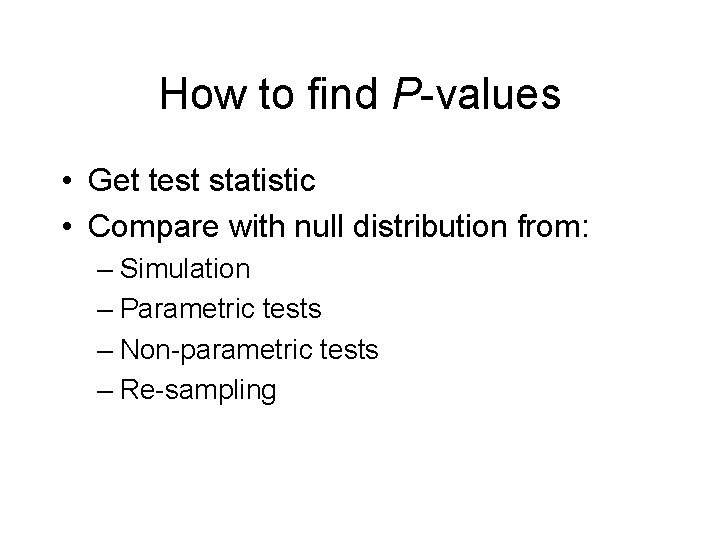
How to find P-values • Get test statistic • Compare with null distribution from: – Simulation – Parametric tests – Non-parametric tests – Re-sampling
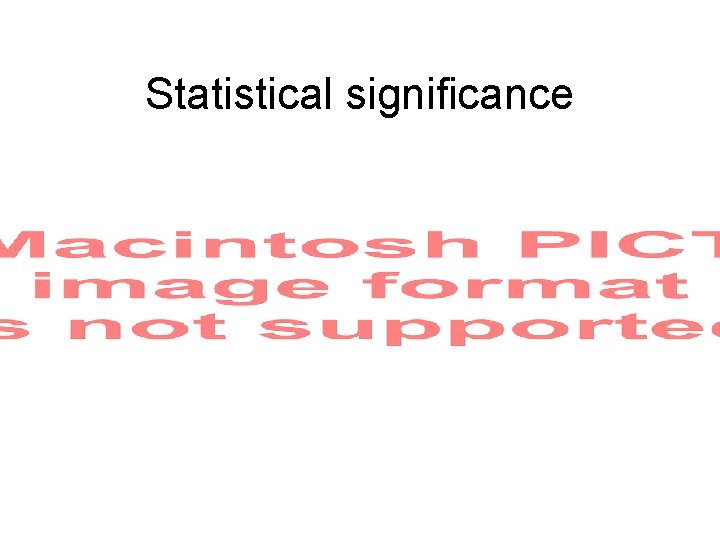
Statistical significance
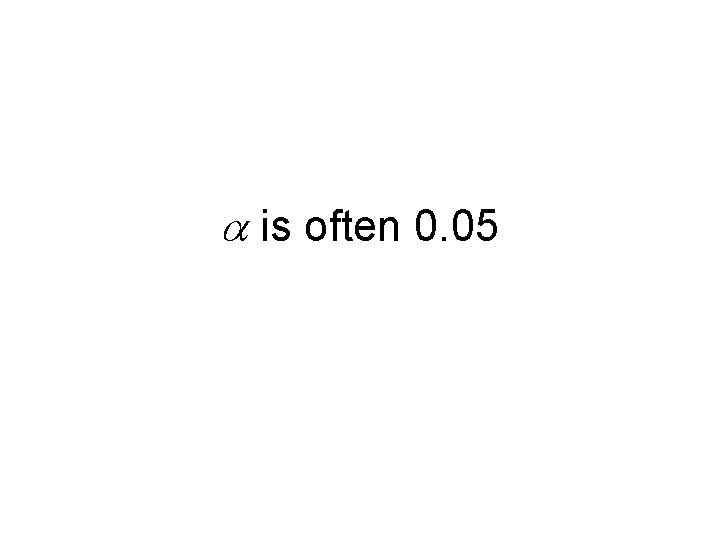
a is often 0. 05
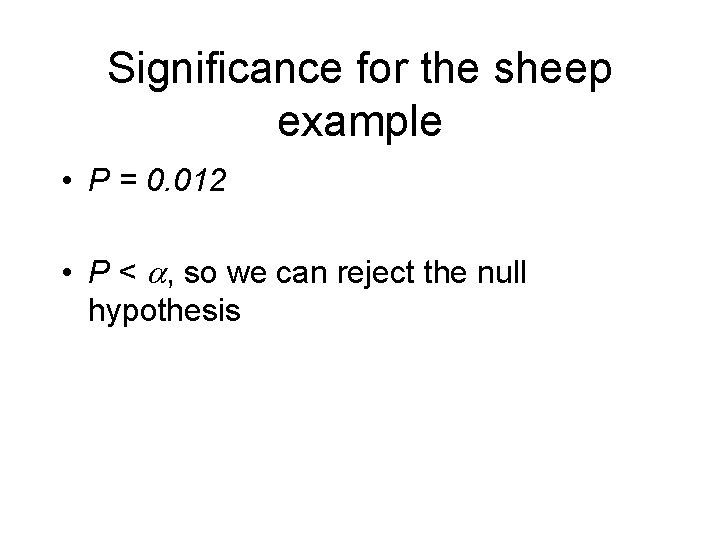
Significance for the sheep example • P = 0. 012 • P < a, so we can reject the null hypothesis
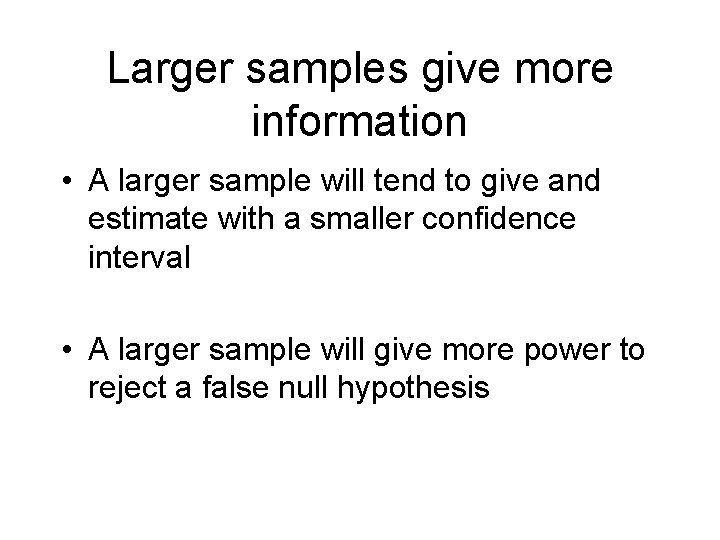
Larger samples give more information • A larger sample will tend to give and estimate with a smaller confidence interval • A larger sample will give more power to reject a false null hypothesis
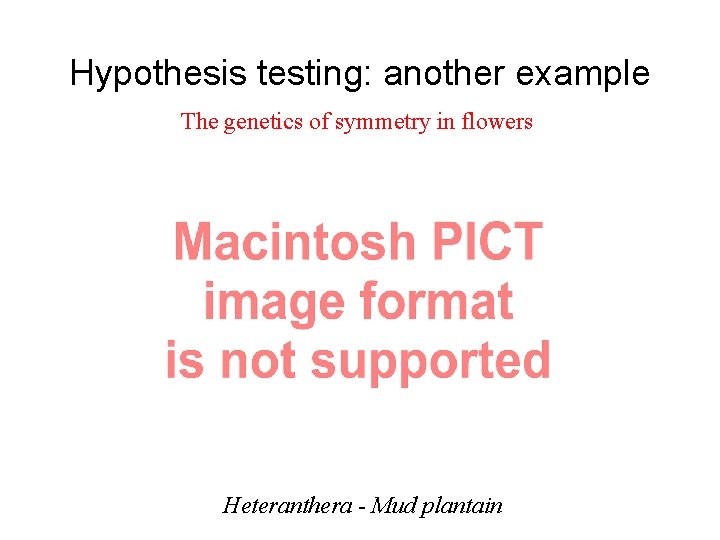
Hypothesis testing: another example The genetics of symmetry in flowers Heteranthera - Mud plantain
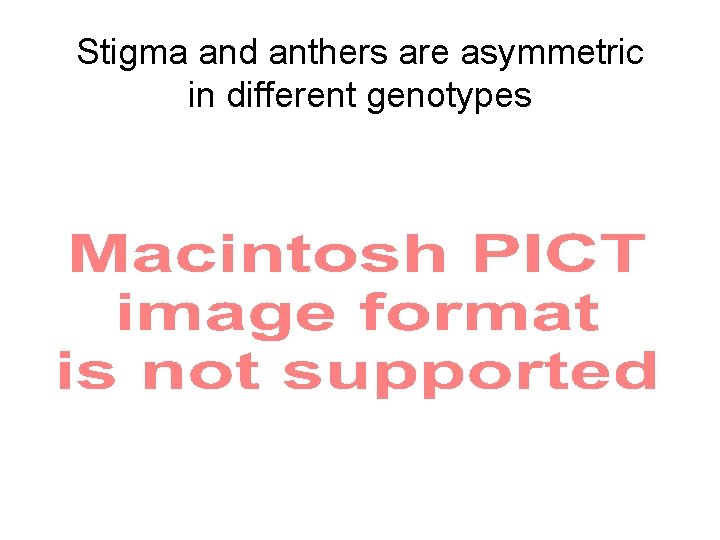
Stigma and anthers are asymmetric in different genotypes
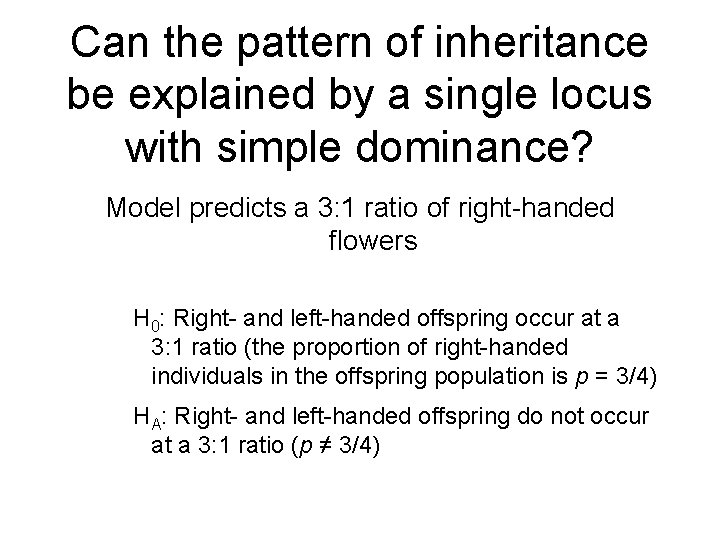
Can the pattern of inheritance be explained by a single locus with simple dominance? Model predicts a 3: 1 ratio of right-handed flowers H 0: Right- and left-handed offspring occur at a 3: 1 ratio (the proportion of right-handed individuals in the offspring population is p = 3/4) HA: Right- and left-handed offspring do not occur at a 3: 1 ratio (p ≠ 3/4)
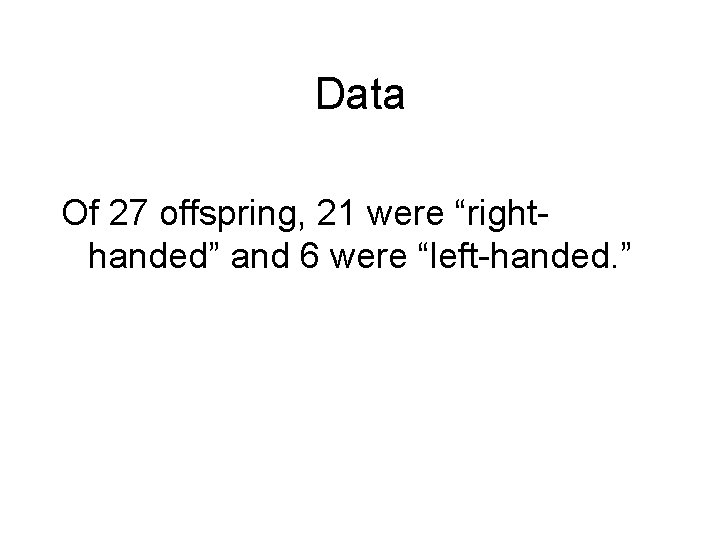
Data Of 27 offspring, 21 were “righthanded” and 6 were “left-handed. ”
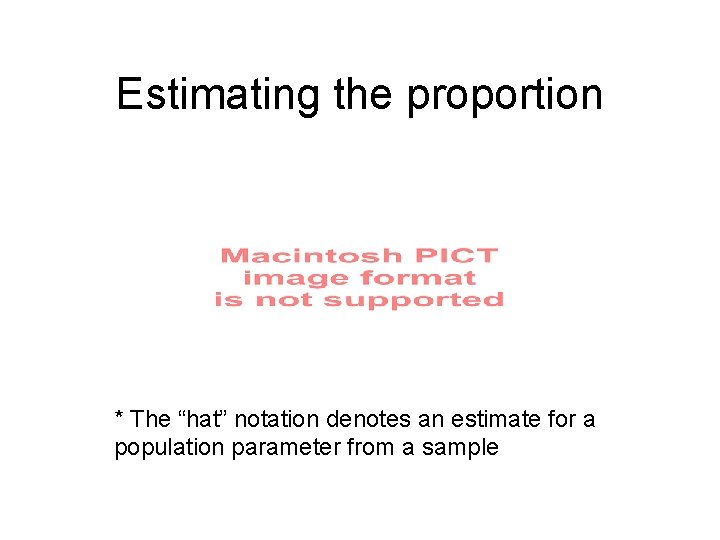
Estimating the proportion * The “hat” notation denotes an estimate for a population parameter from a sample
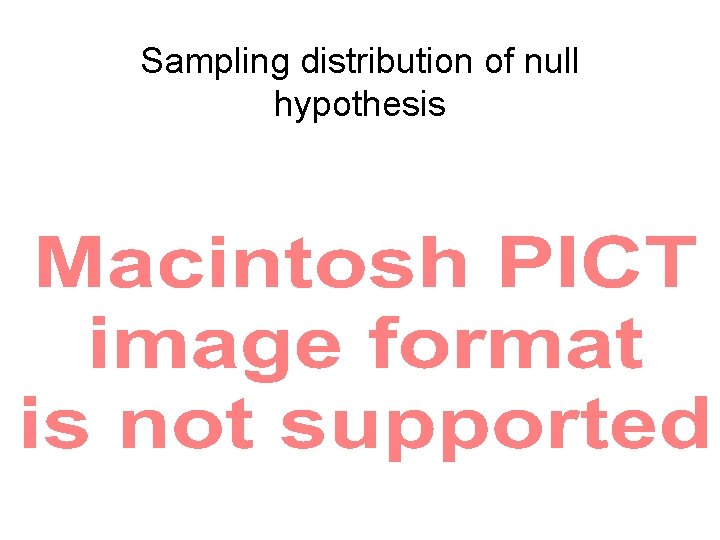
Sampling distribution of null hypothesis
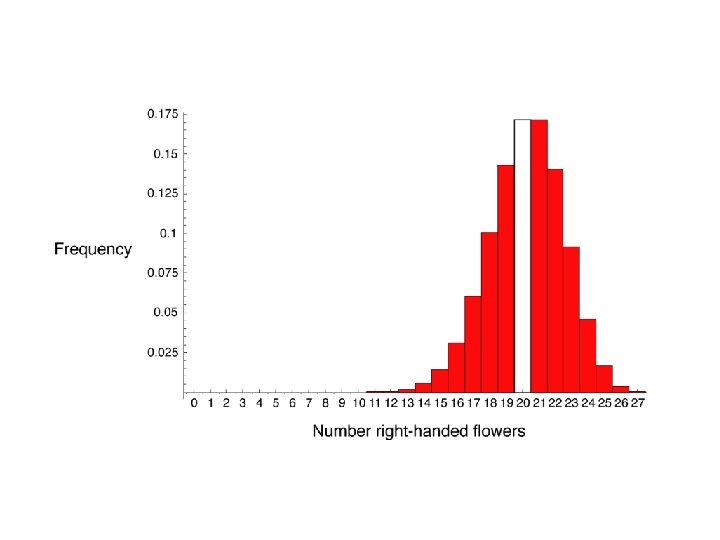
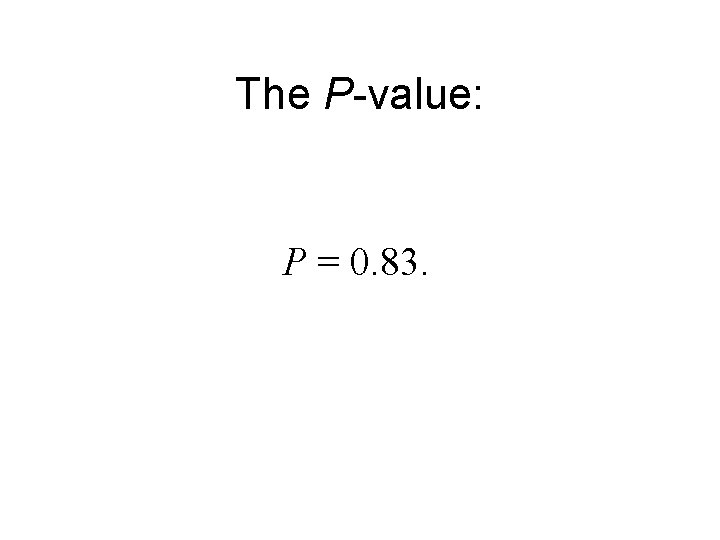
The P-value: P = 0. 83.
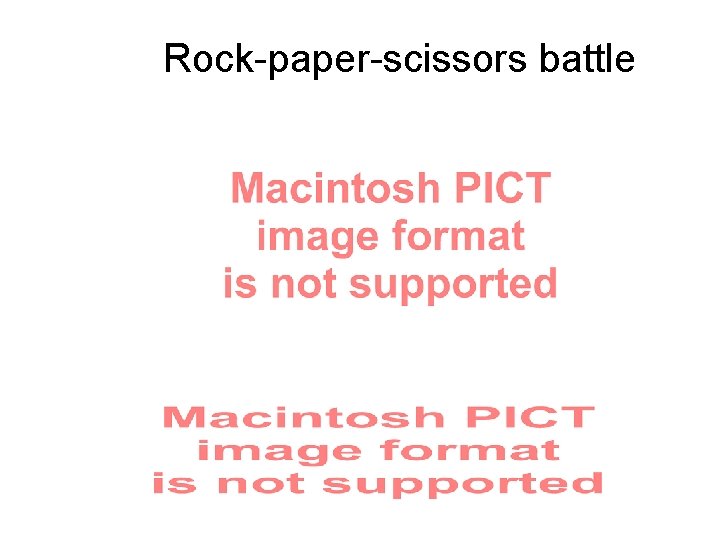
Rock-paper-scissors battle
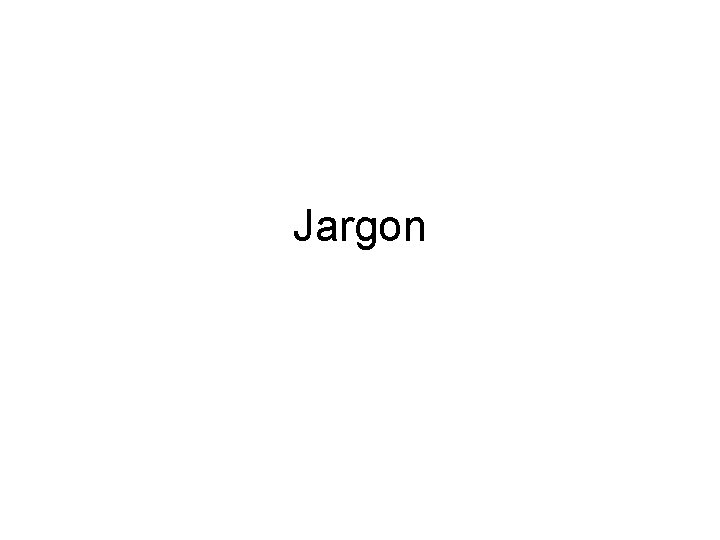
Jargon
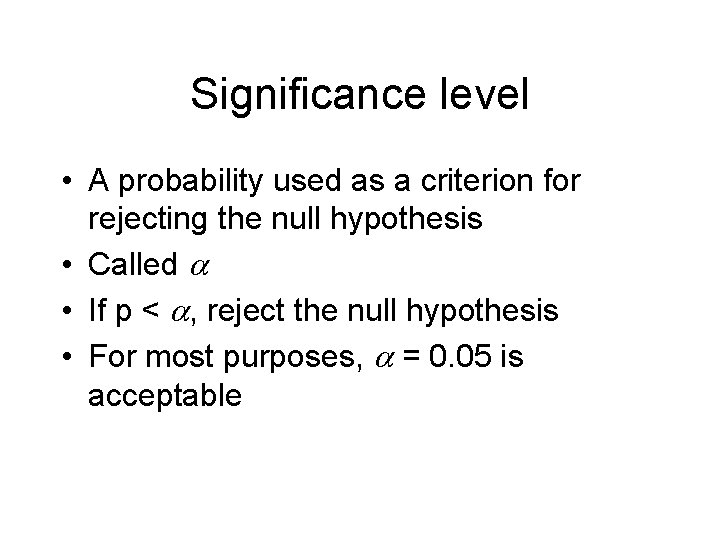
Significance level • A probability used as a criterion for rejecting the null hypothesis • Called a • If p < a, reject the null hypothesis • For most purposes, a = 0. 05 is acceptable
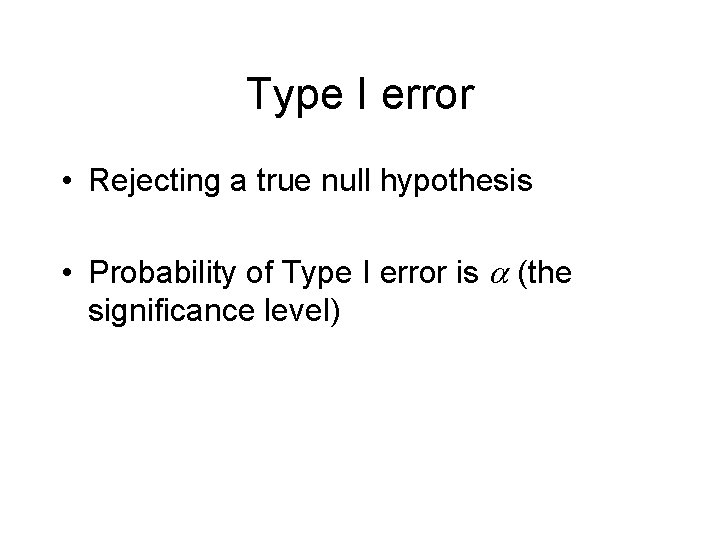
Type I error • Rejecting a true null hypothesis • Probability of Type I error is a (the significance level)
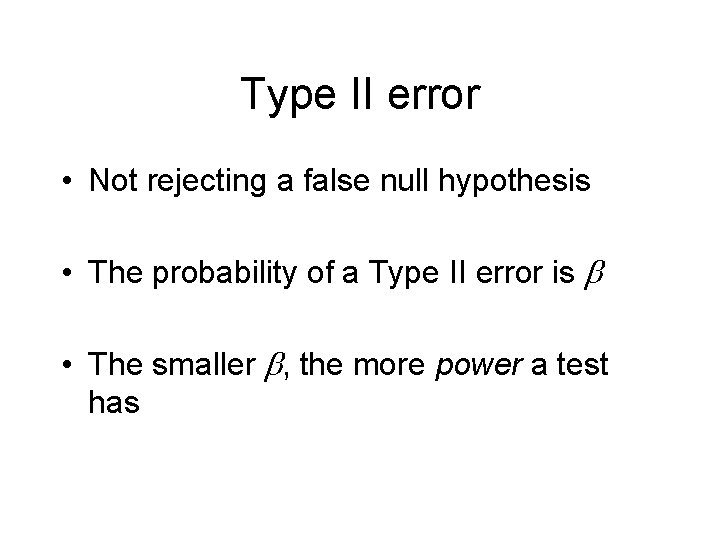
Type II error • Not rejecting a false null hypothesis • The probability of a Type II error is b • The smaller b, the more power a test has
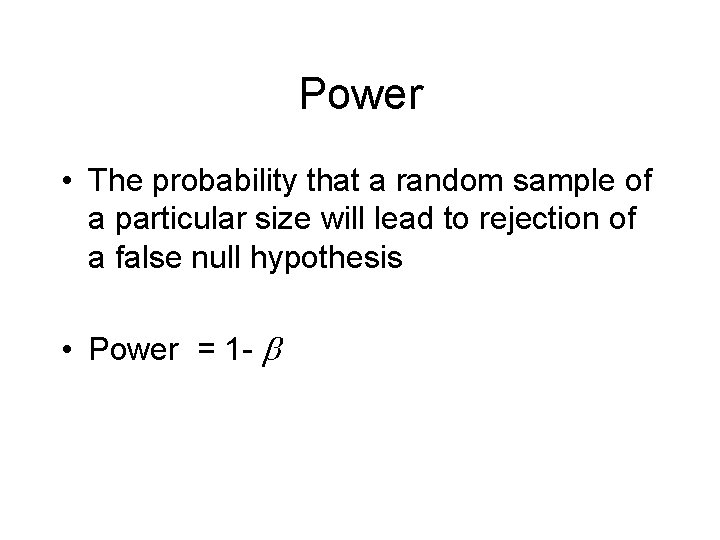
Power • The probability that a random sample of a particular size will lead to rejection of a false null hypothesis • Power = 1 - b
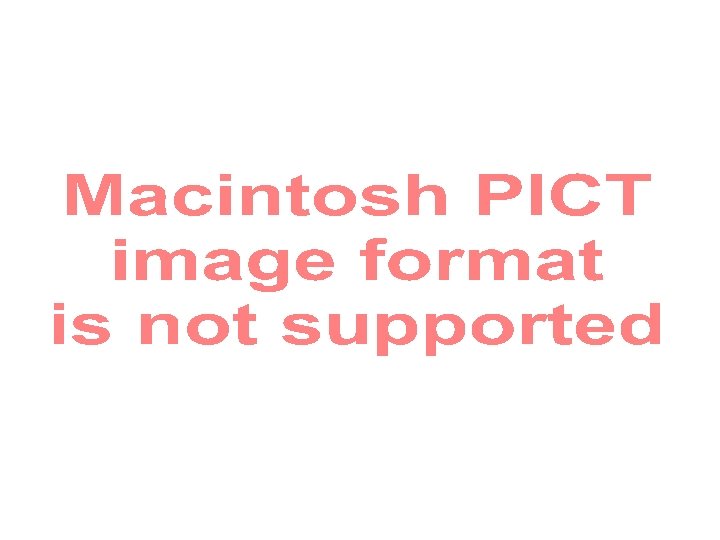
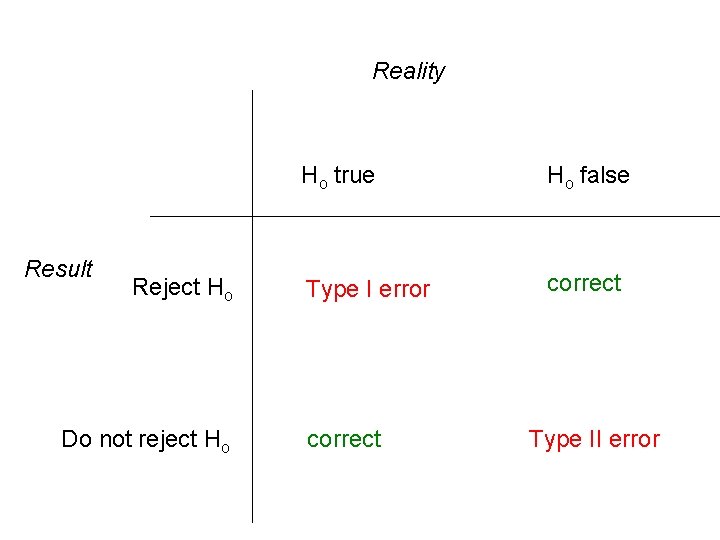
Reality Result Reject Ho Do not reject Ho Ho true Ho false Type I error correct Type II error
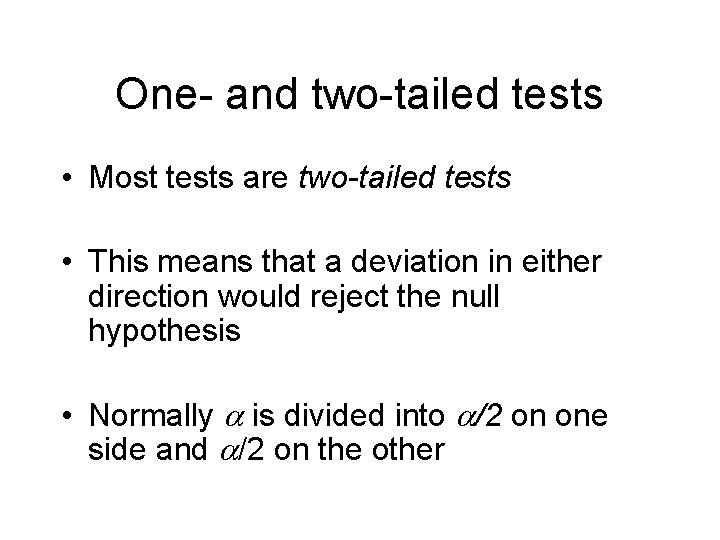
One- and two-tailed tests • Most tests are two-tailed tests • This means that a deviation in either direction would reject the null hypothesis • Normally a is divided into a/2 on one side and a/2 on the other
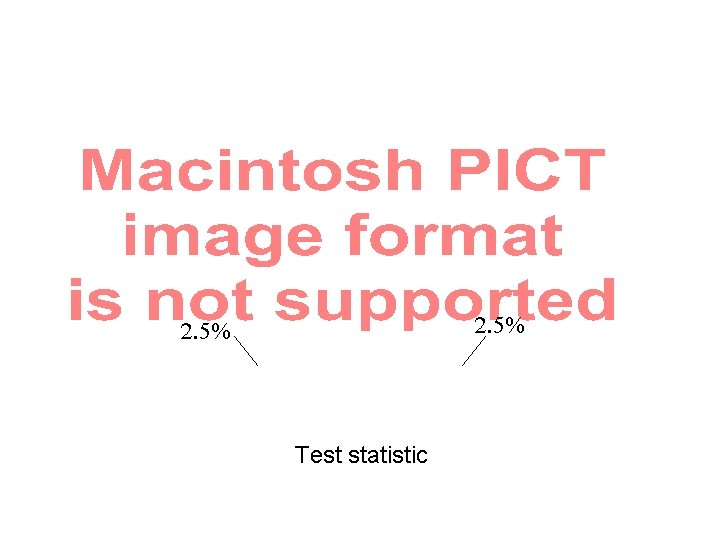
2. 5% Test statistic
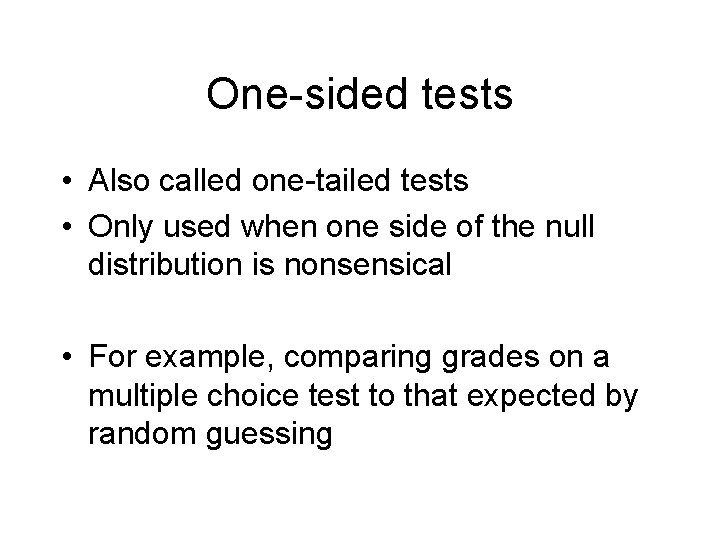
One-sided tests • Also called one-tailed tests • Only used when one side of the null distribution is nonsensical • For example, comparing grades on a multiple choice test to that expected by random guessing
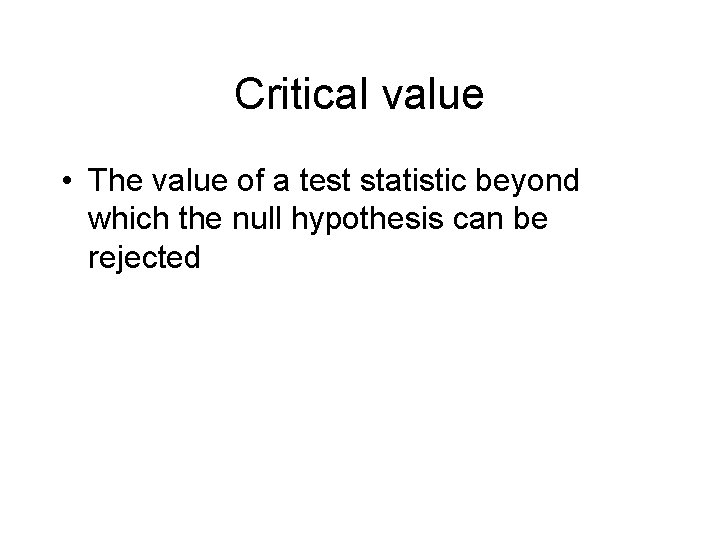
Critical value • The value of a test statistic beyond which the null hypothesis can be rejected
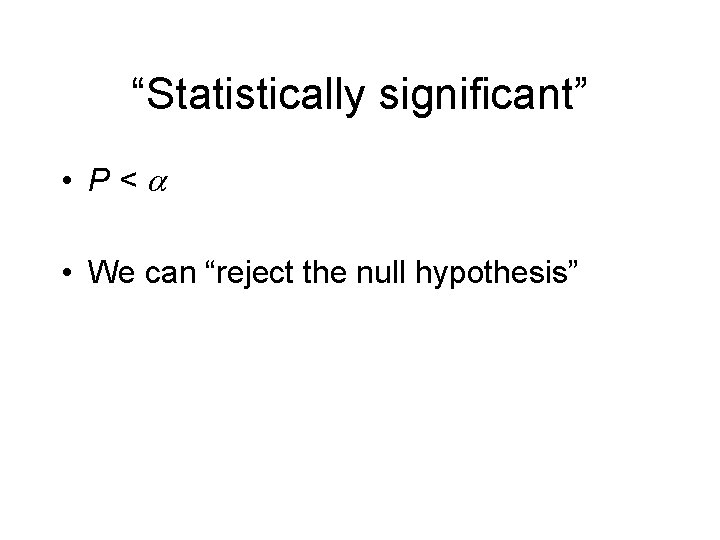
“Statistically significant” • P<a • We can “reject the null hypothesis”
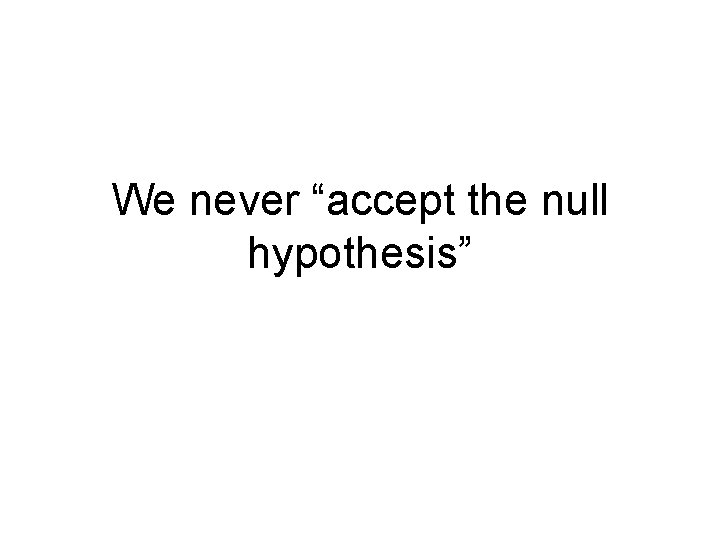
We never “accept the null hypothesis”
I want to know something about
Smart is something you become not something you are
Something old something new poem
The wind yells while blowing
Something that describes something
Concrete definition literature
Something concrete that stands for something abstract
For explanation purpose
When something represents something else
I surrender i want to know you more
Curious minds want to know
Curious minds want to know
Inquiry minds want to know
If you want to know me by noemia de sousa
How do you know something is alive
Https:/ / www.youtube.com/ watch?v=jnj8mc04r9e
How do you know if something is living
Are protozoa alive
Dilan gorur
Nothing formed against me shall stand song
"know history know self"
Null and alternative hypothesis statistics
Null and alternative hypothesis examples
Protoplanet hypothesis weakness
Null hypothesis and research hypothesis
Hypothesis testing formula
Hypothesis testing adalah
Hypothesis testing exercises and solutions
Kari is testing the hypothesis
Hypothesis testing examples and solutions doc
Hypothesis example
Hypothesis testing in r
Business statistics hypothesis testing
Hypothesis testing
Six steps of hypothesis testing
Hypothesis testing study
P-value interpretation
Fundamentals of hypothesis testing
Null hypothesis notation
Kari is testing the hypothesis
Introduction to hypothesis testing
How to test hypothesis in excel
Chapter 7 hypothesis testing with one sample answers
Goal of hypothesis testing
Null hypothesis example
Kari is testing the hypothesis
Categorical hypothesis testing
Testing hypothesis
Phantoms in statistics