Fraud Detection and Deterrence in Workers Compensation Richard
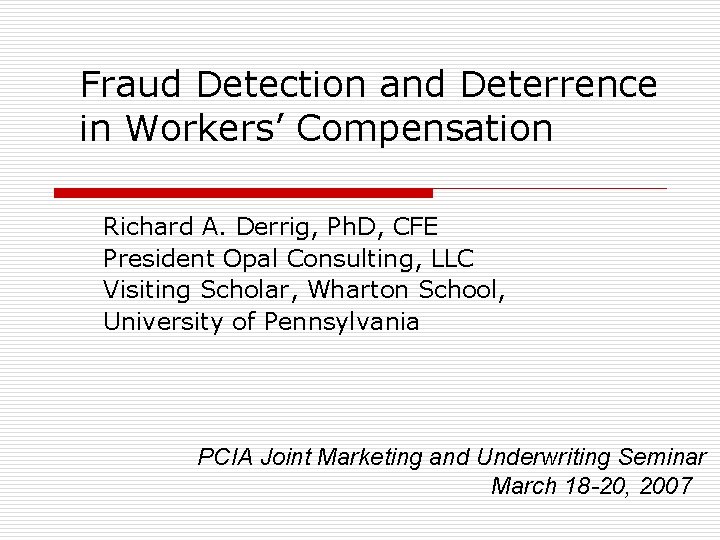
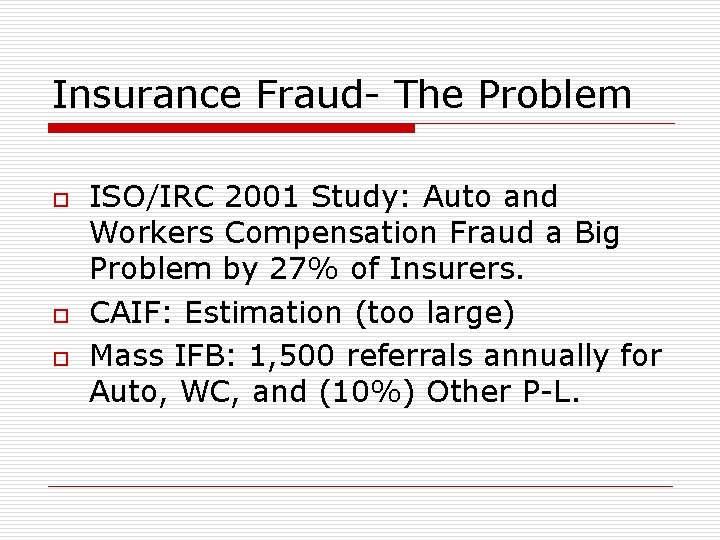
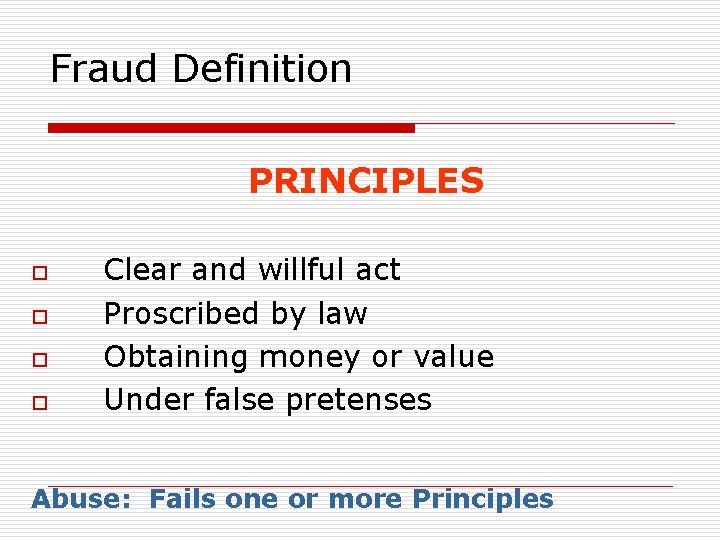
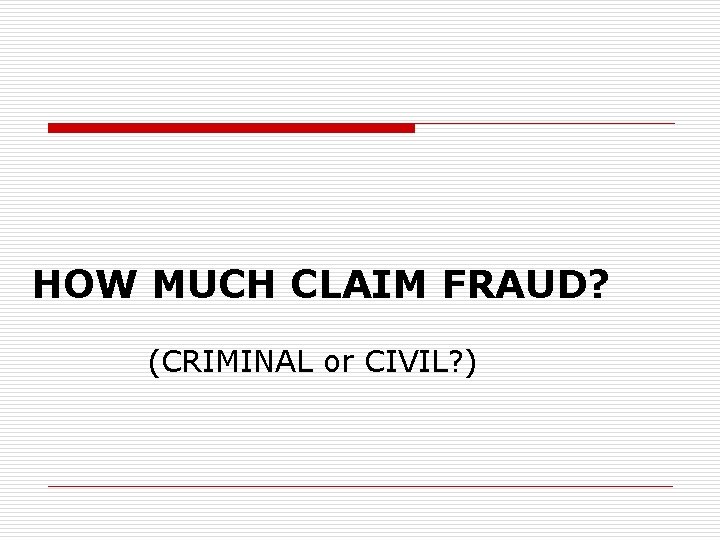
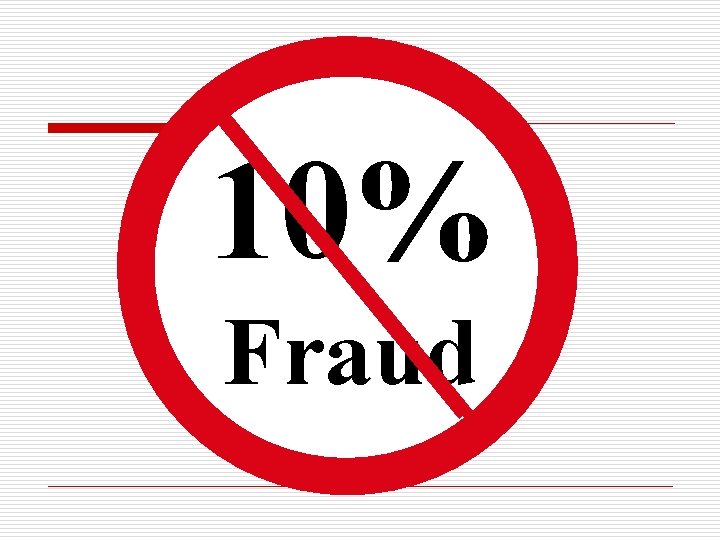
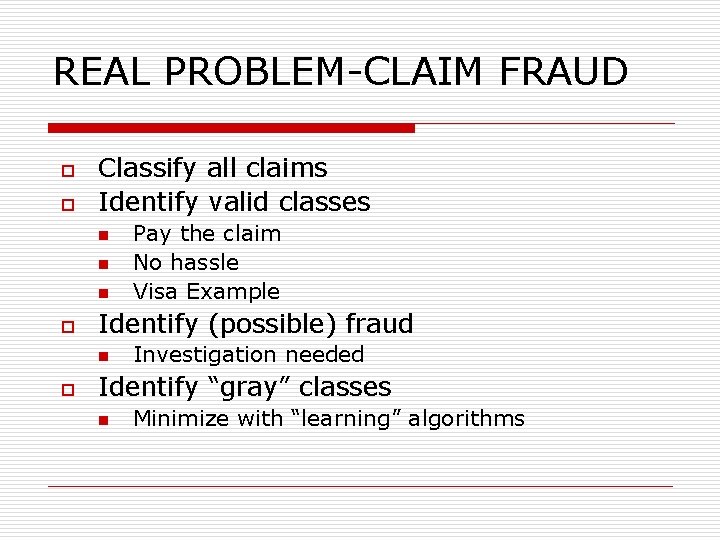
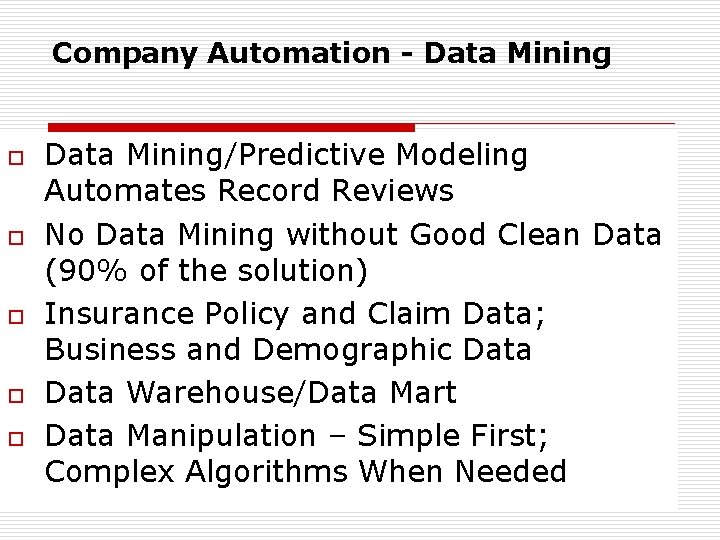
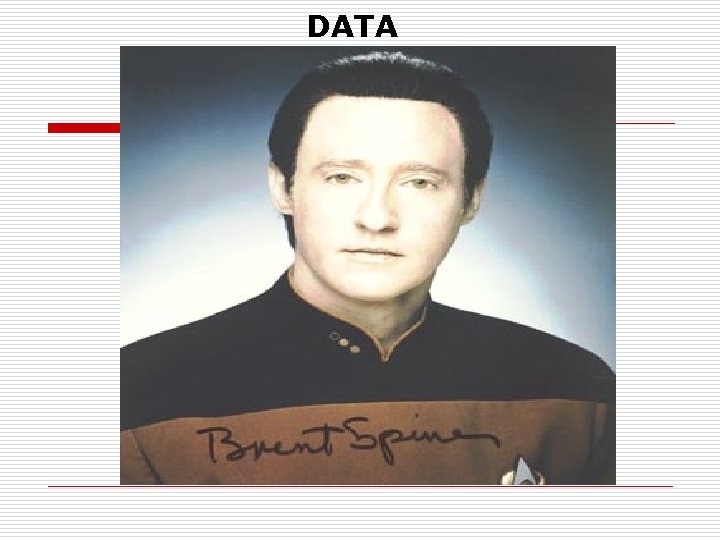
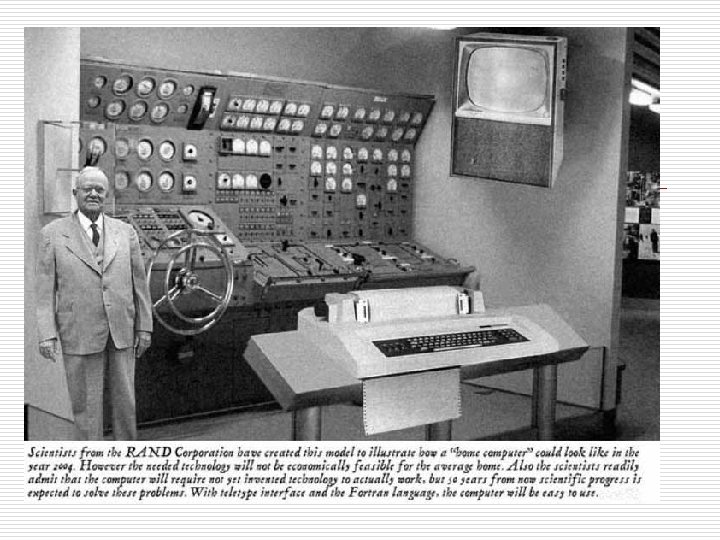
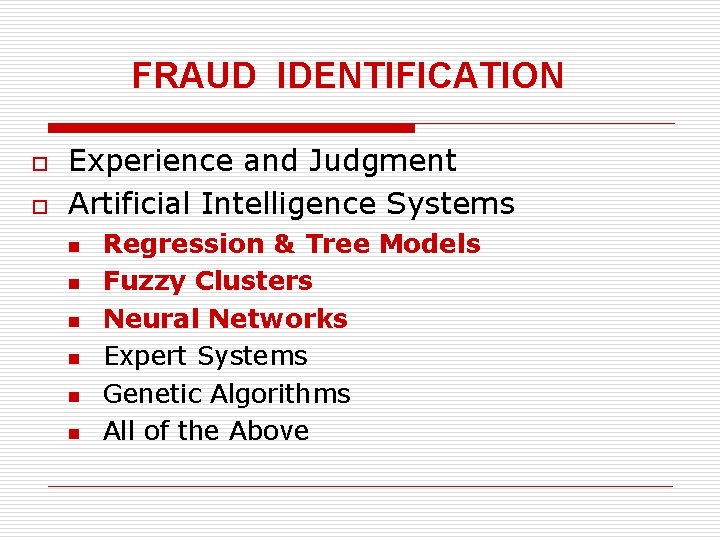
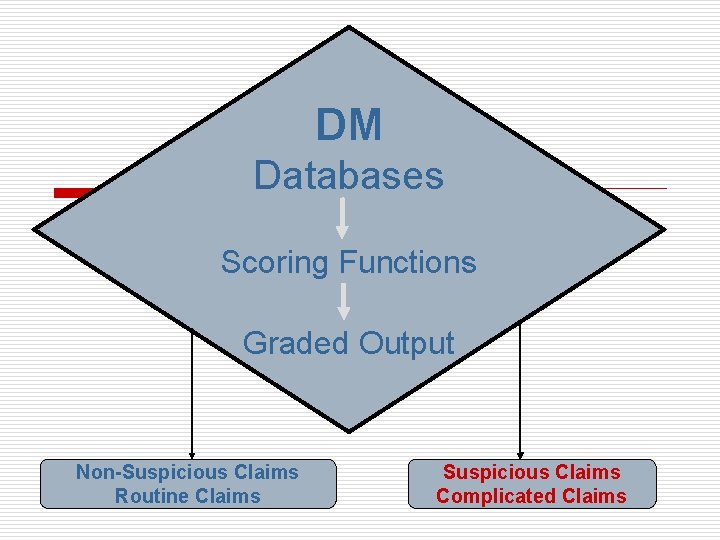
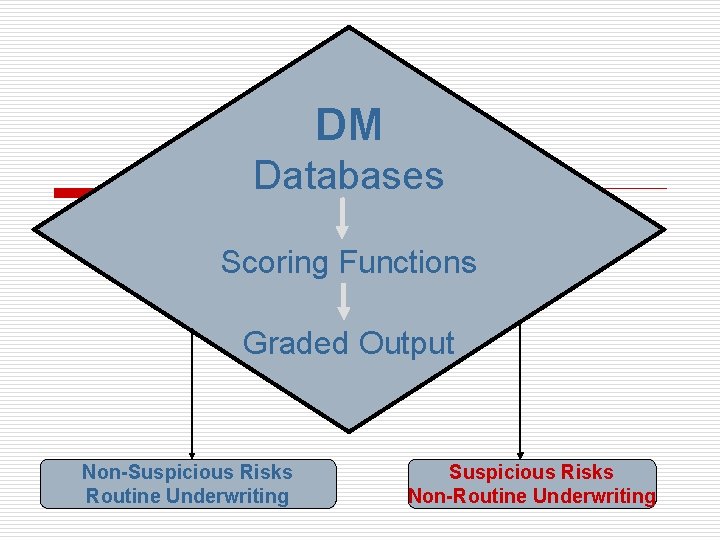
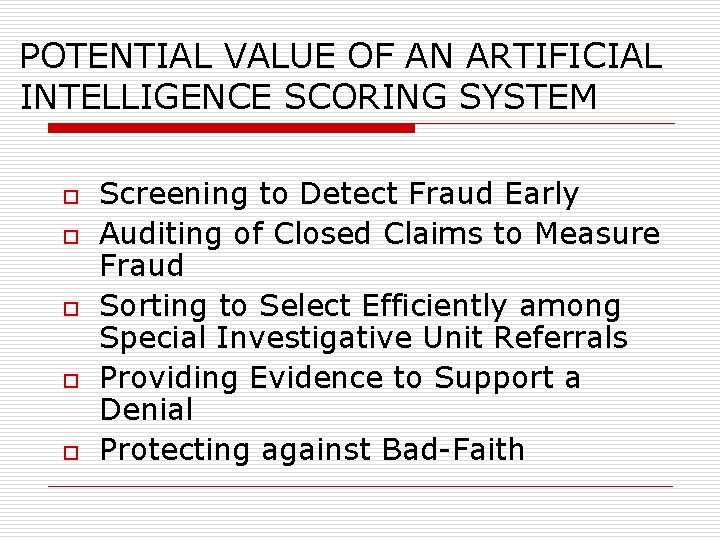
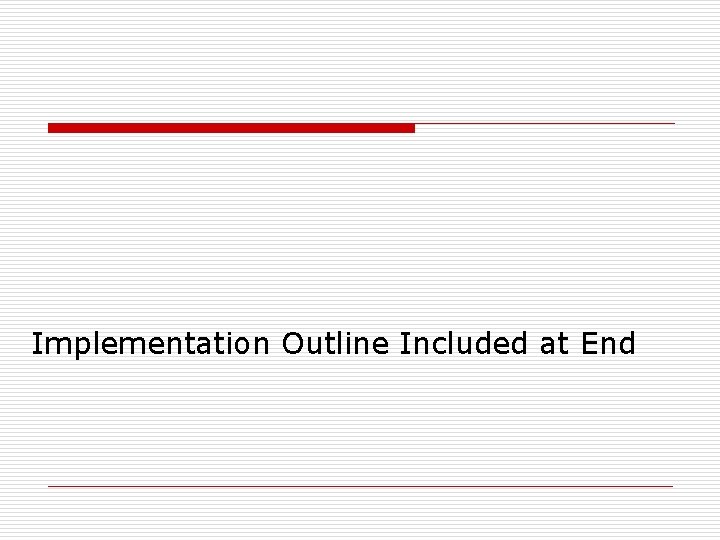
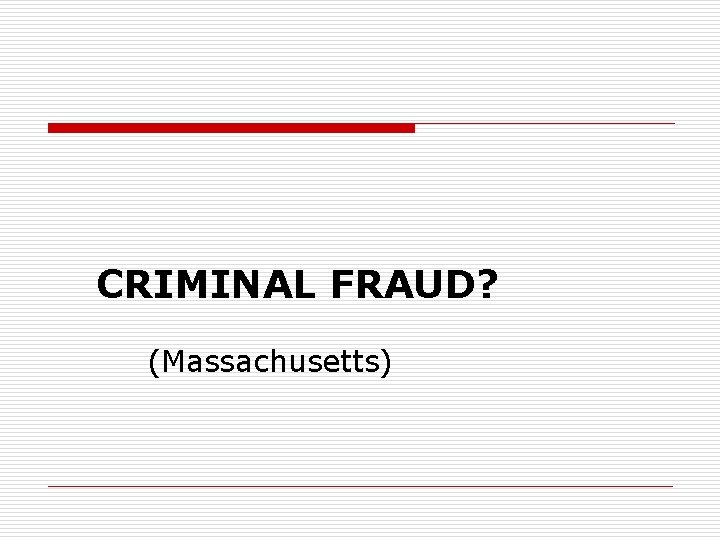
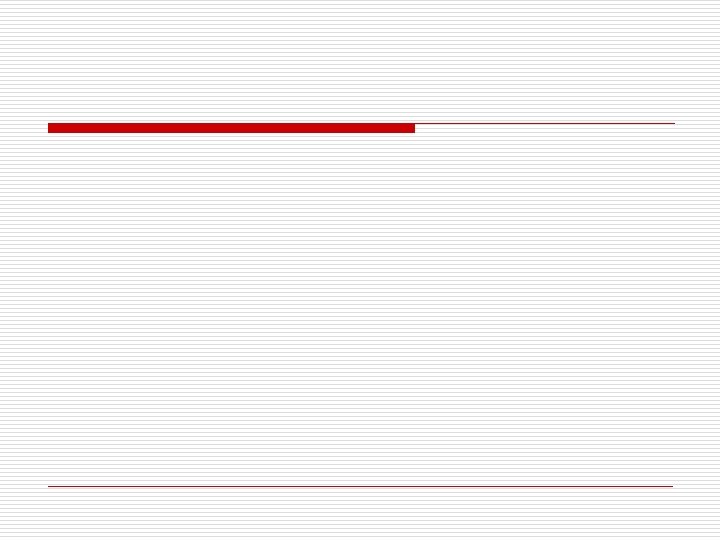
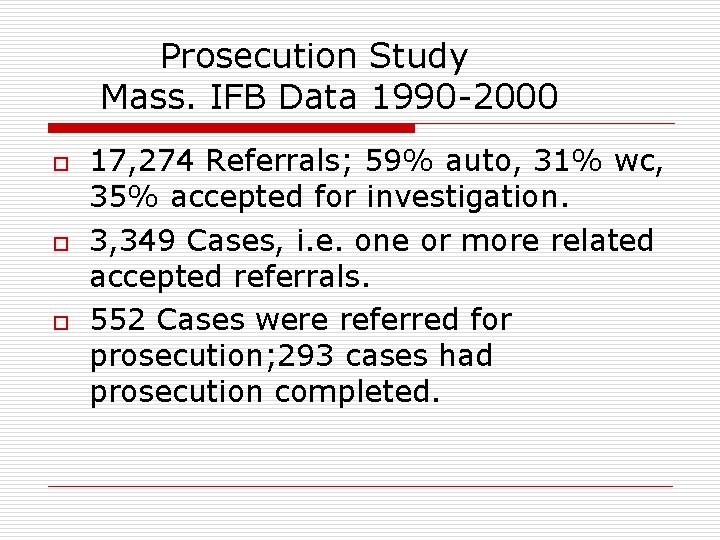
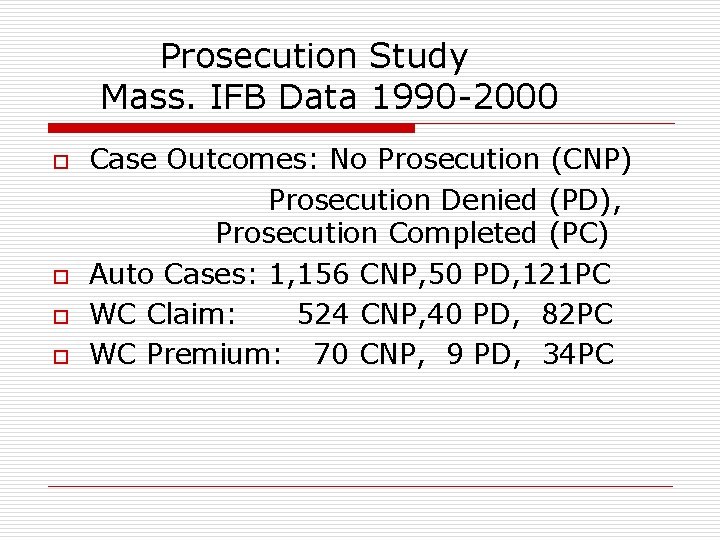
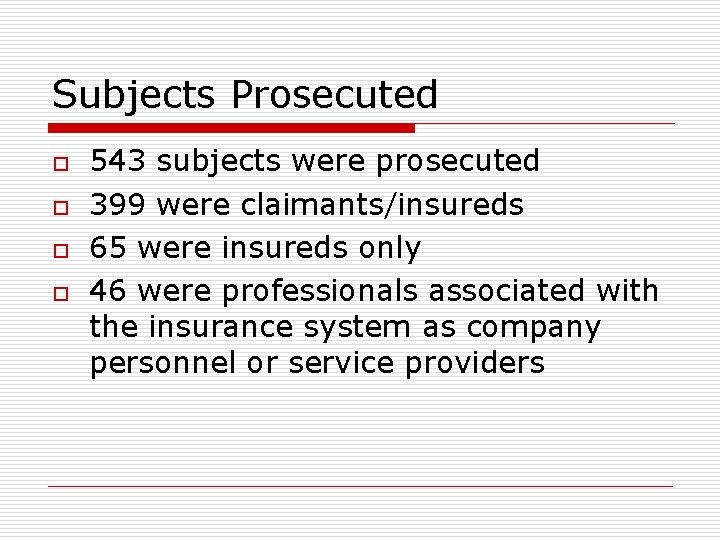
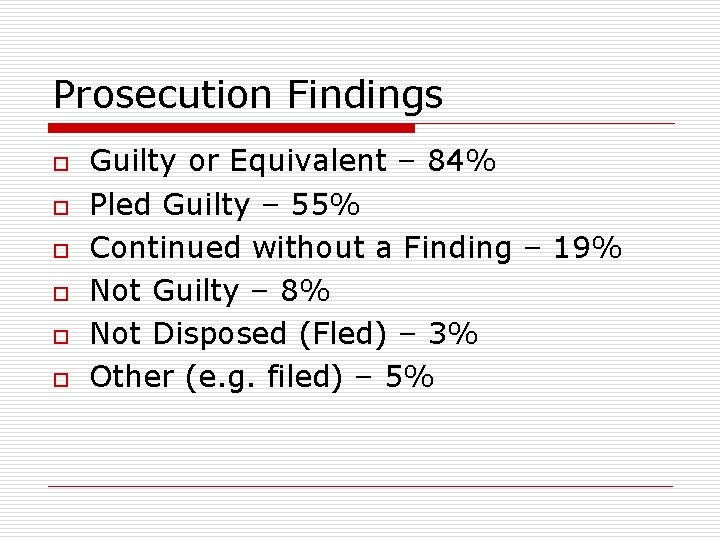
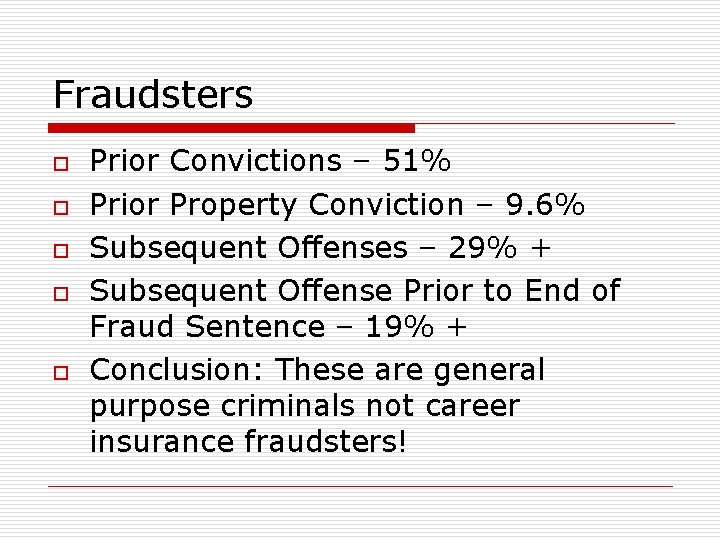
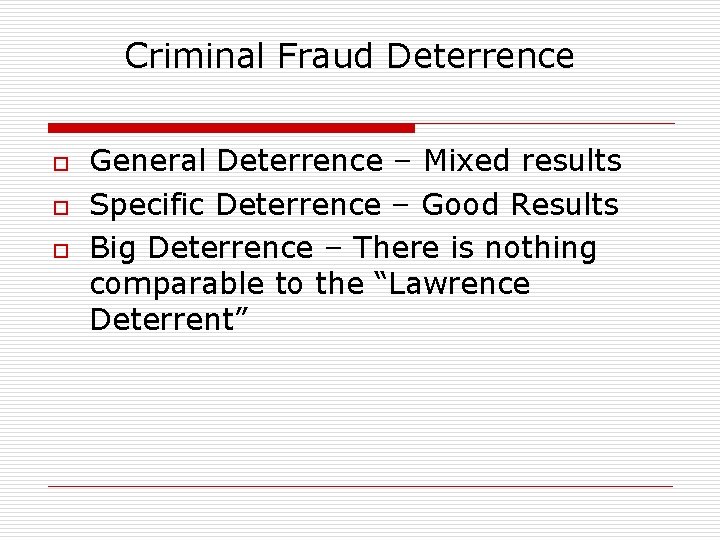
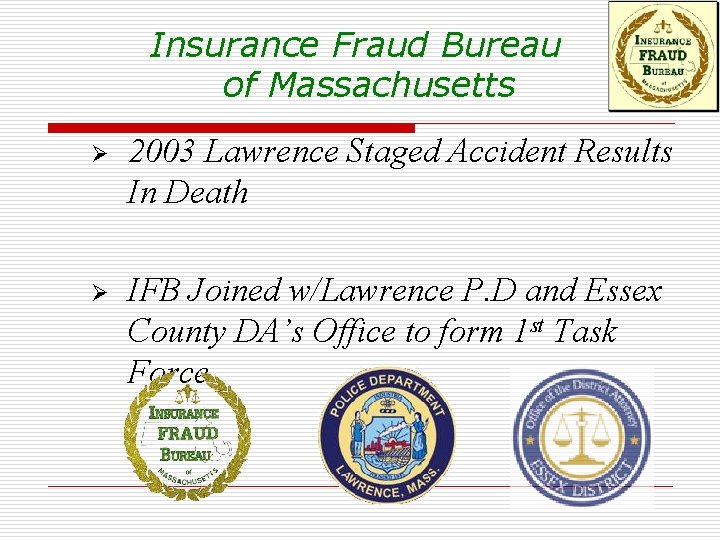
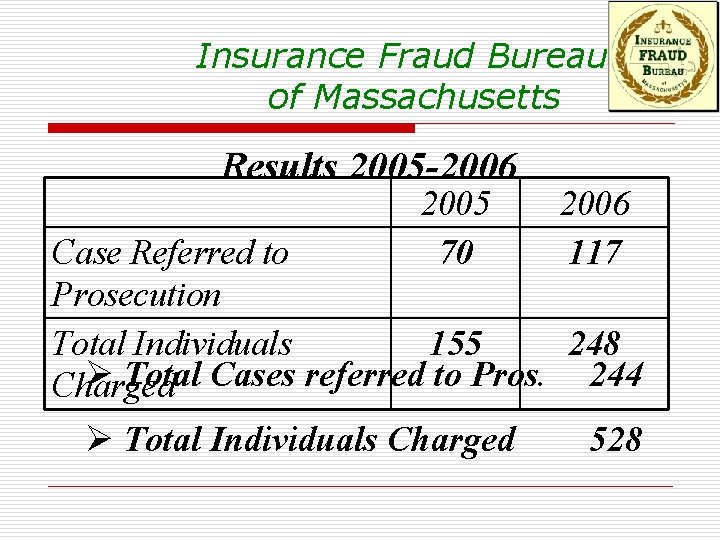
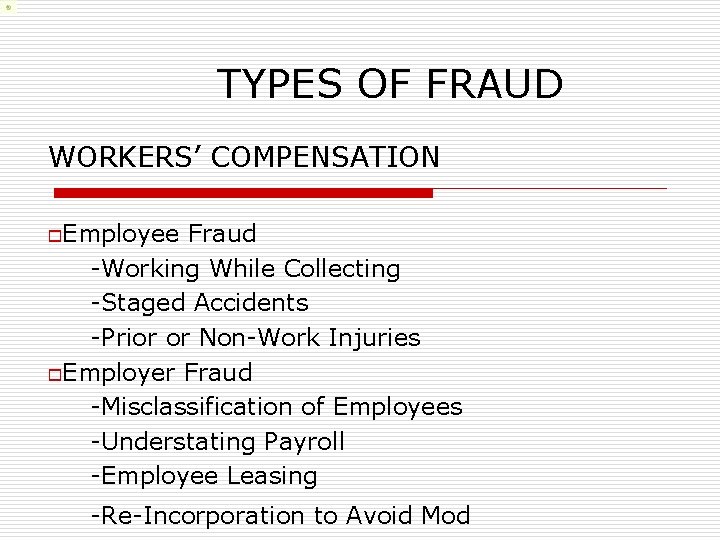
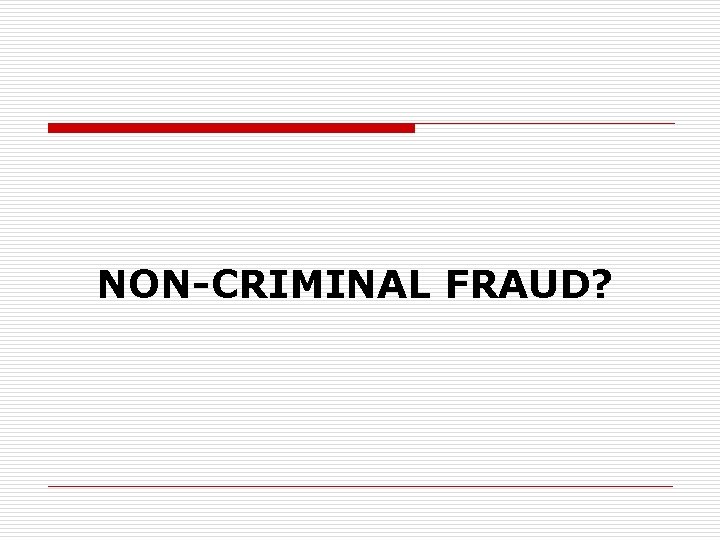
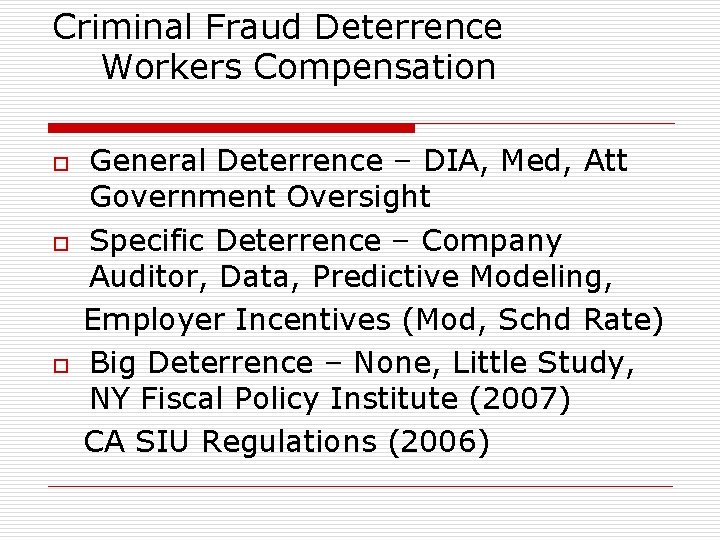
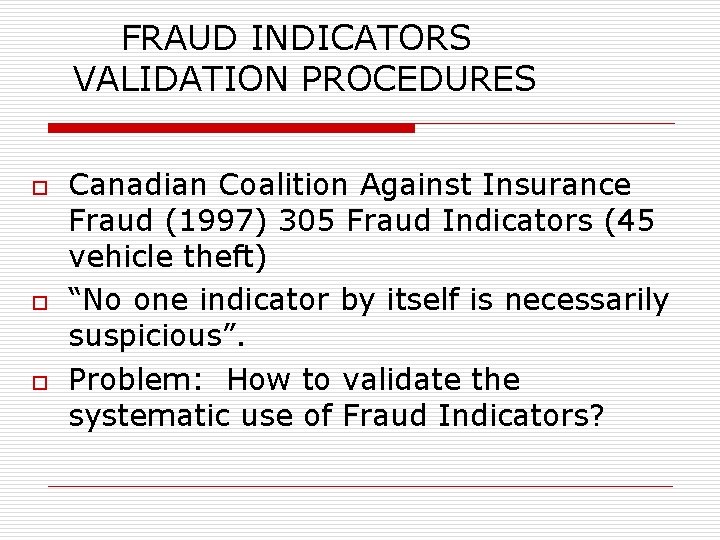
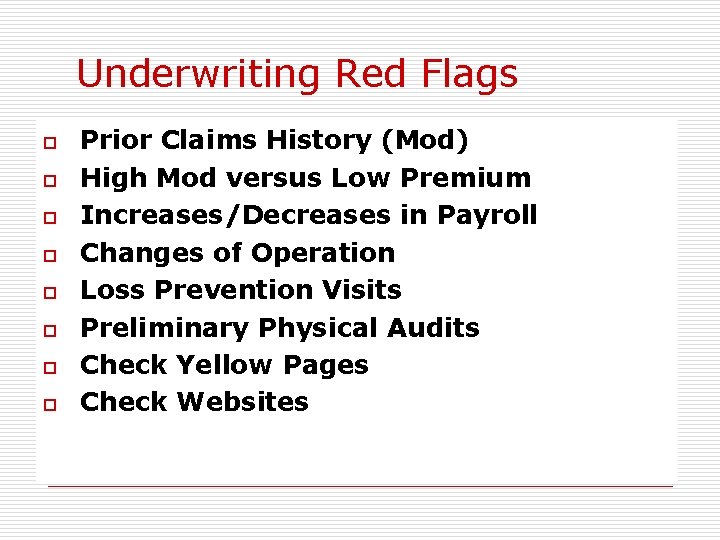
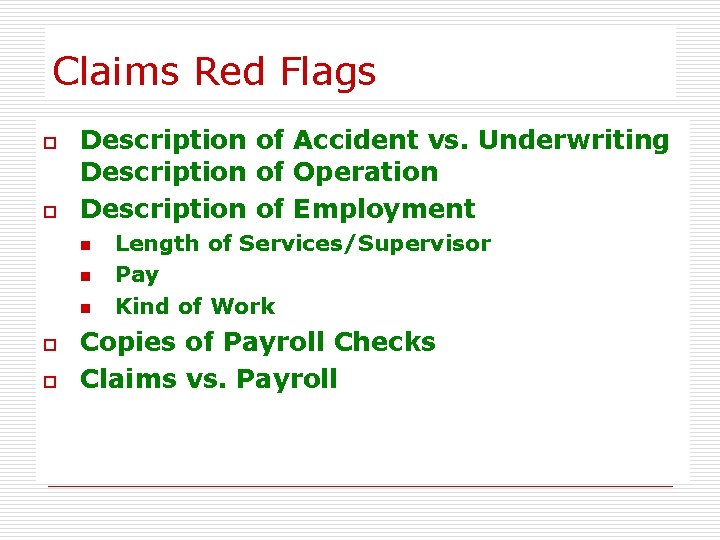
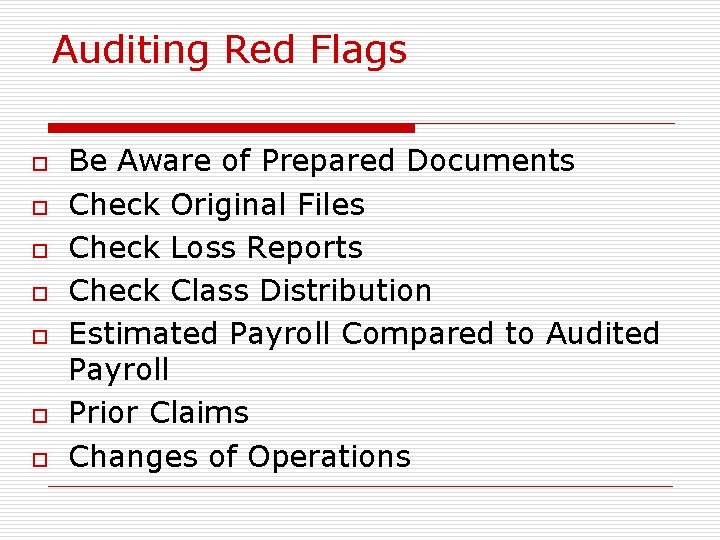
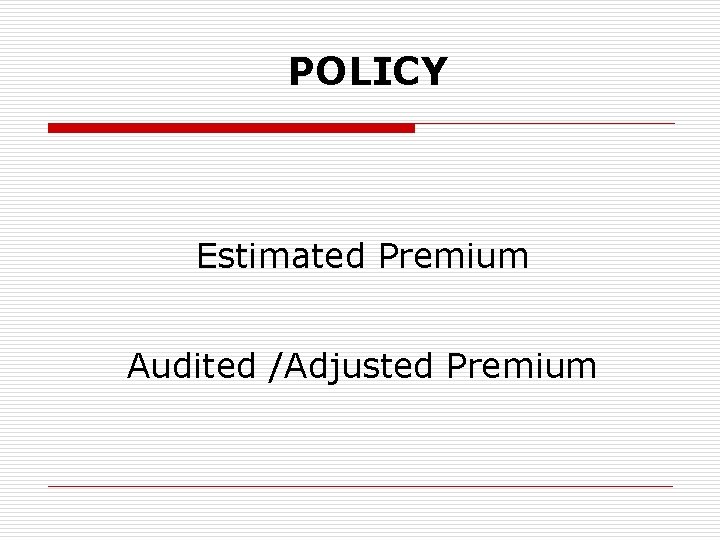
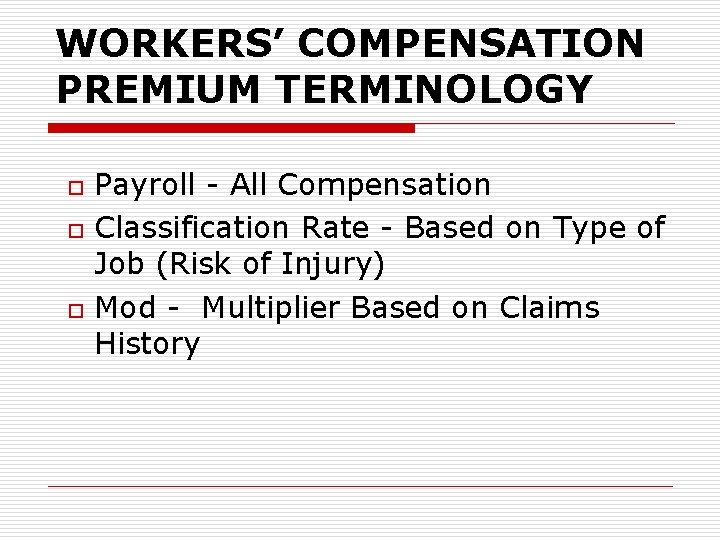
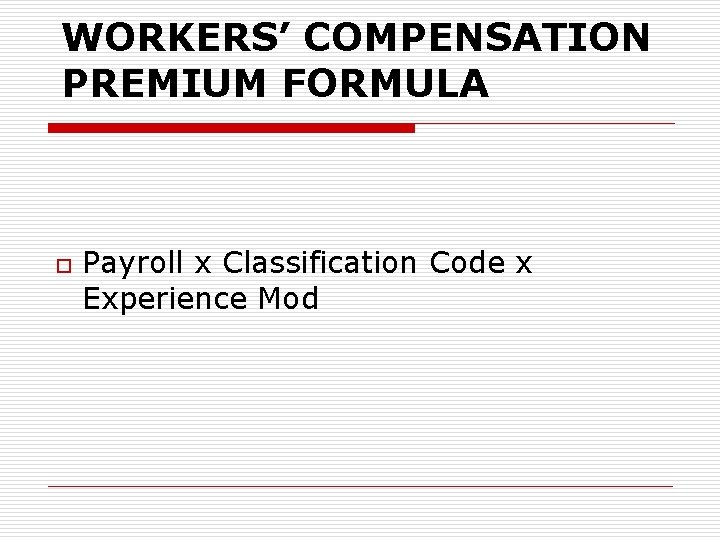
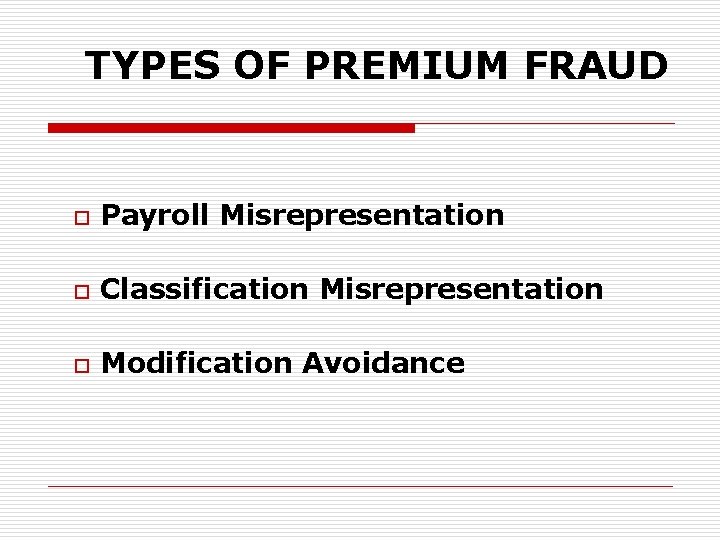
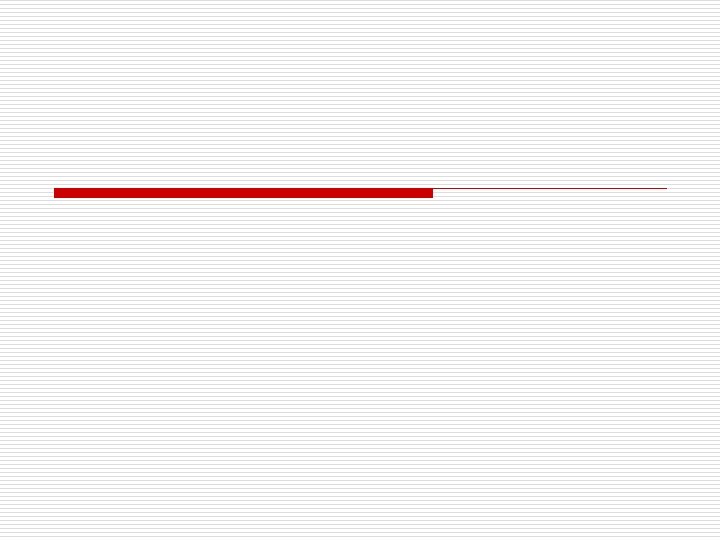
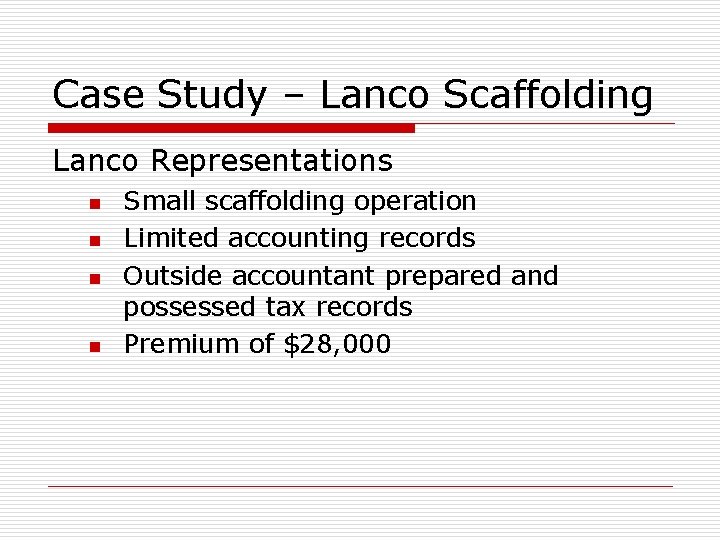
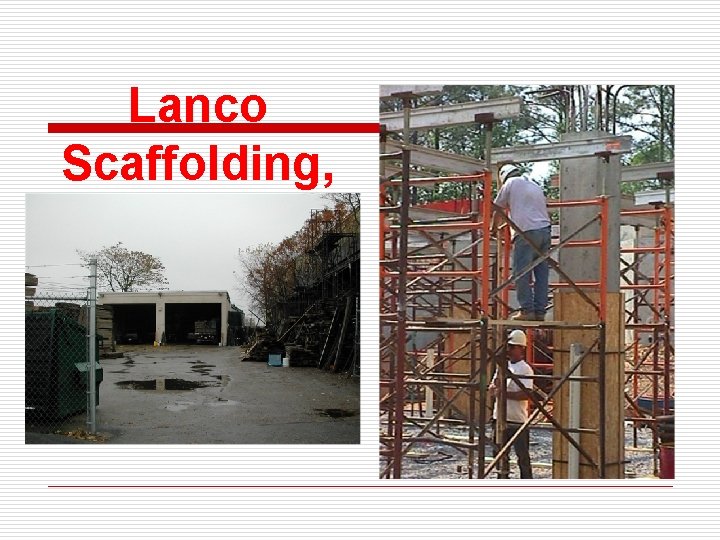
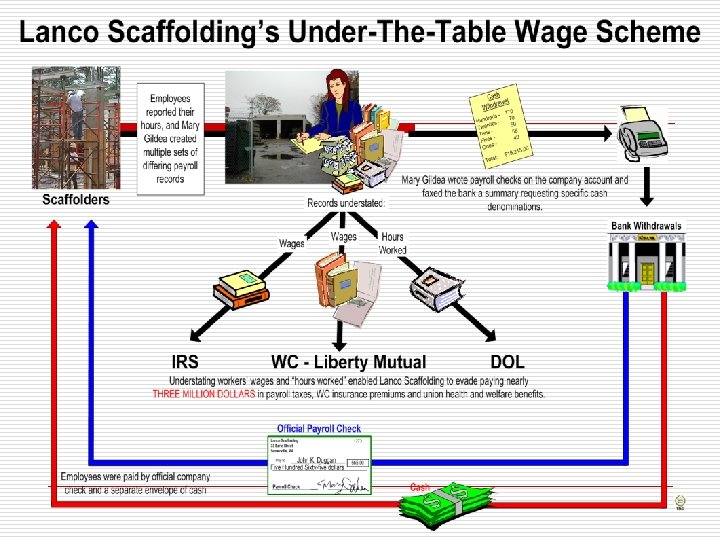
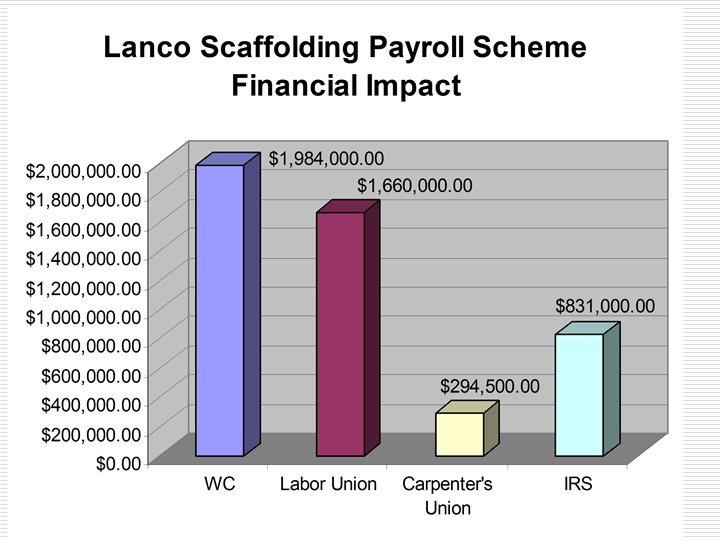
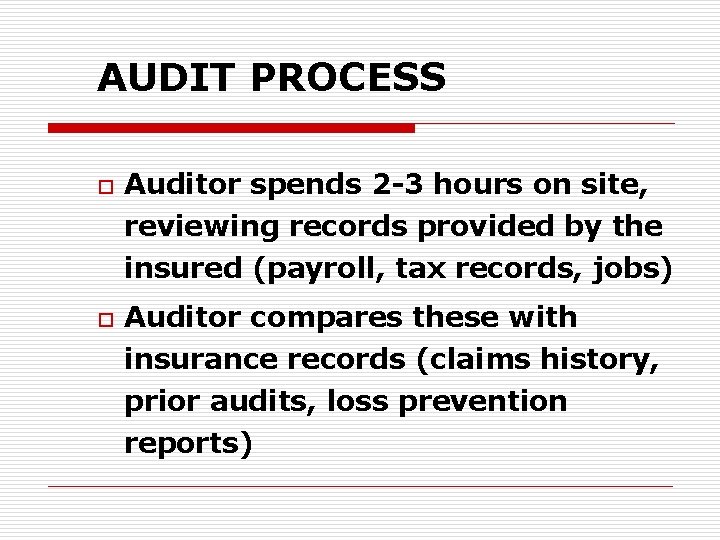
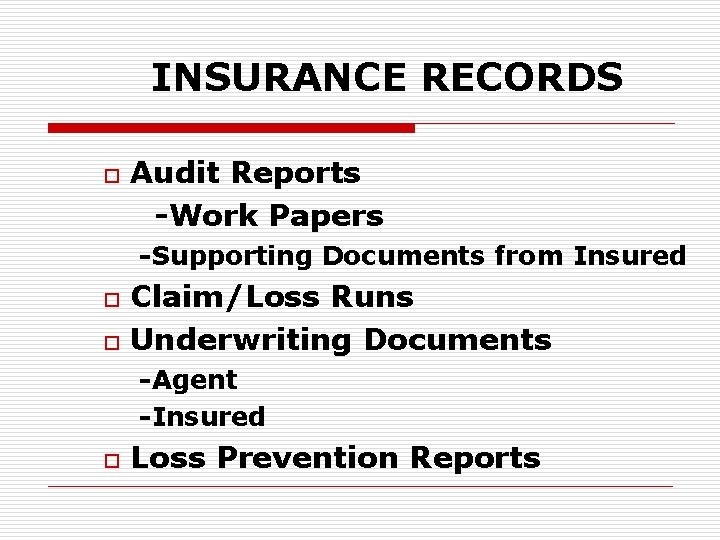
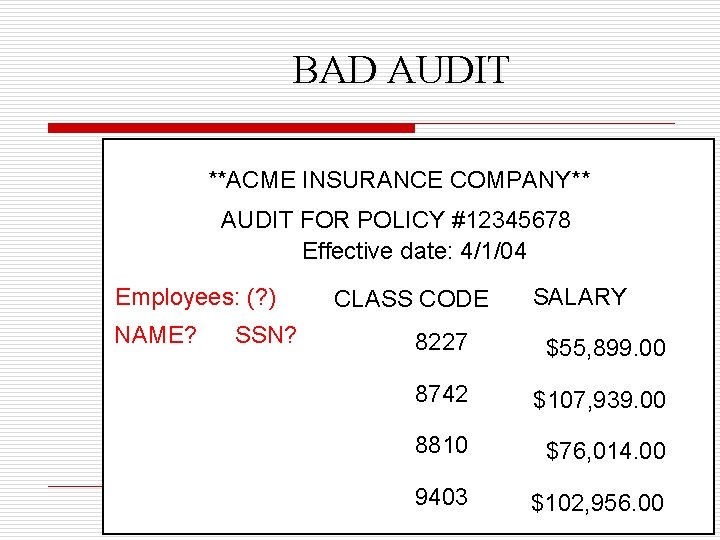
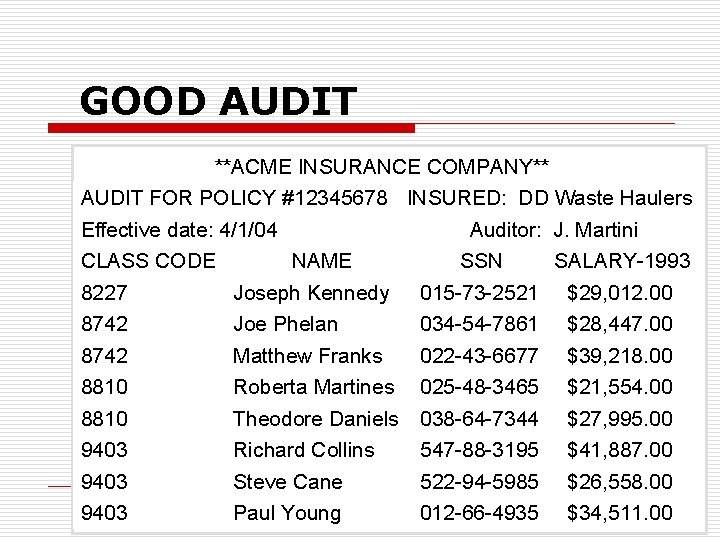
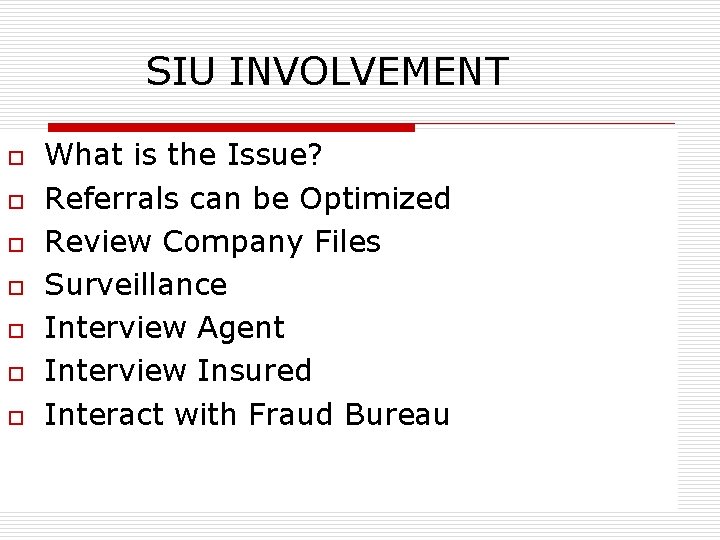
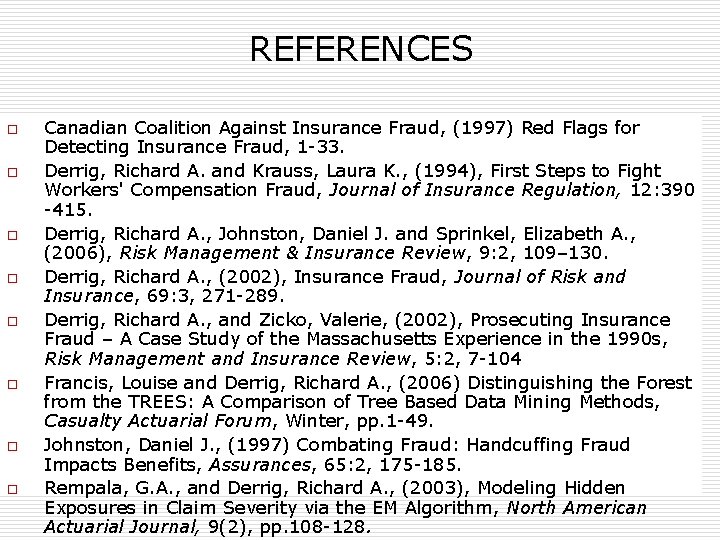
- Slides: 46
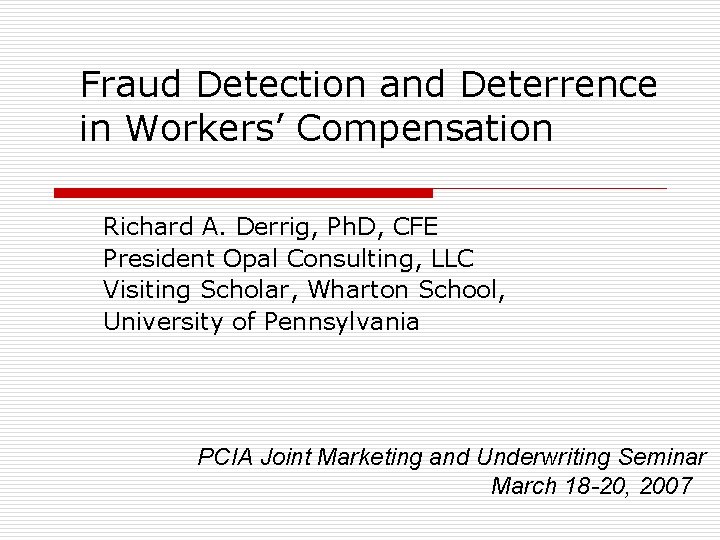
Fraud Detection and Deterrence in Workers’ Compensation Richard A. Derrig, Ph. D, CFE President Opal Consulting, LLC Visiting Scholar, Wharton School, University of Pennsylvania PCIA Joint Marketing and Underwriting Seminar March 18 -20, 2007
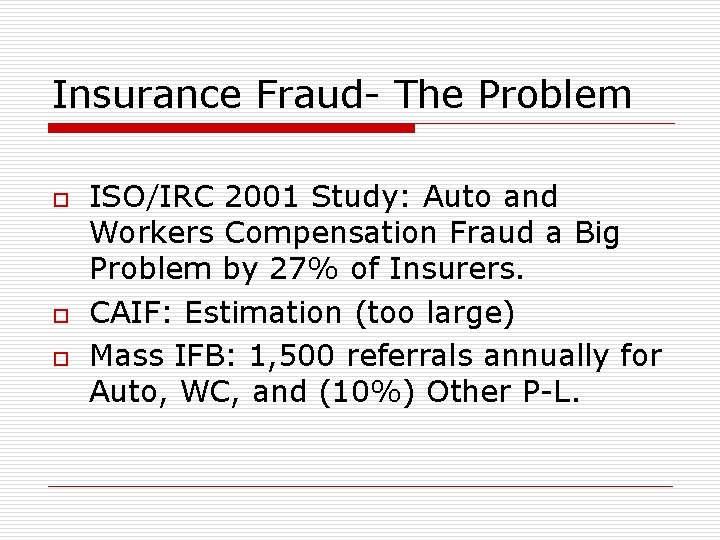
Insurance Fraud- The Problem o o o ISO/IRC 2001 Study: Auto and Workers Compensation Fraud a Big Problem by 27% of Insurers. CAIF: Estimation (too large) Mass IFB: 1, 500 referrals annually for Auto, WC, and (10%) Other P-L.
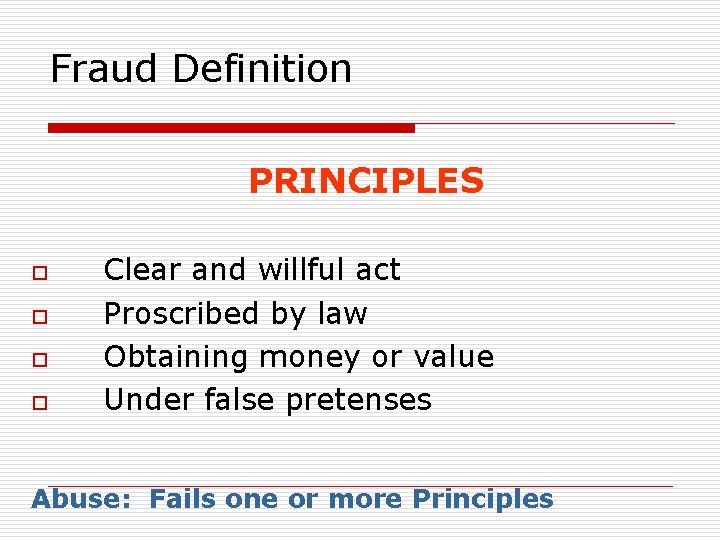
Fraud Definition PRINCIPLES o o Clear and willful act Proscribed by law Obtaining money or value Under false pretenses Abuse: Fails one or more Principles
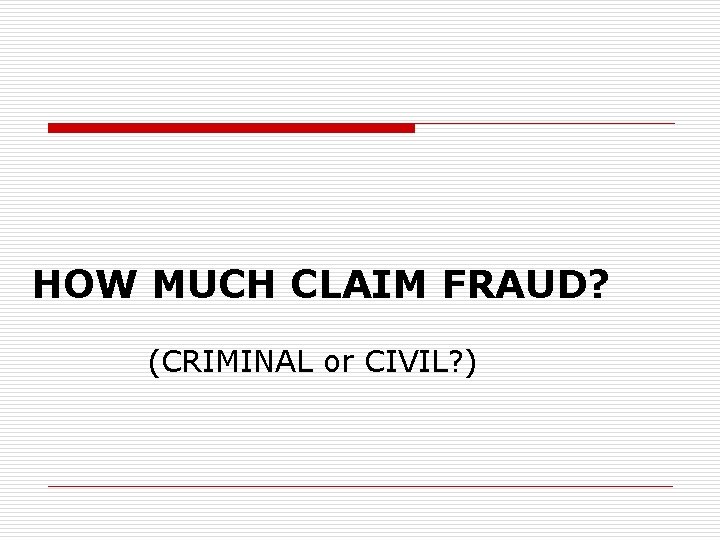
HOW MUCH CLAIM FRAUD? (CRIMINAL or CIVIL? )
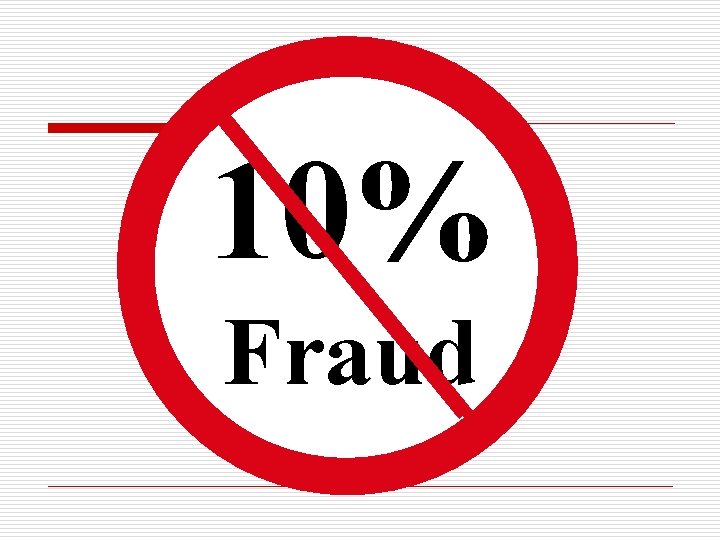
10% Fraud
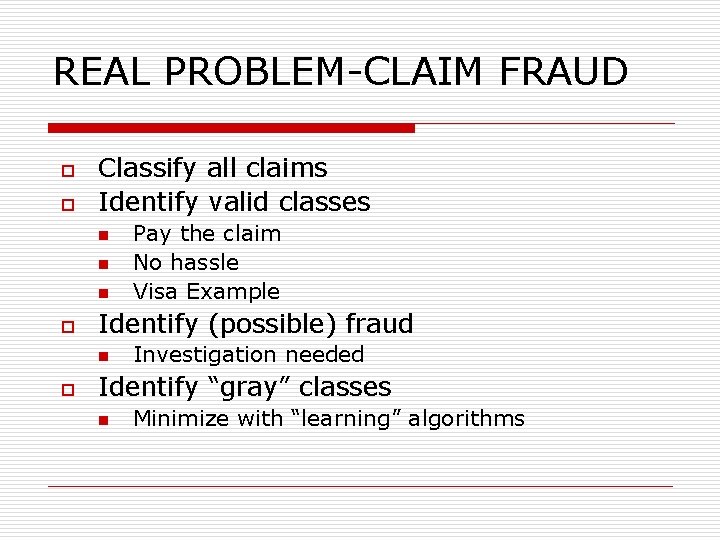
REAL PROBLEM-CLAIM FRAUD o o Classify all claims Identify valid classes n n n o Identify (possible) fraud n o Pay the claim No hassle Visa Example Investigation needed Identify “gray” classes n Minimize with “learning” algorithms
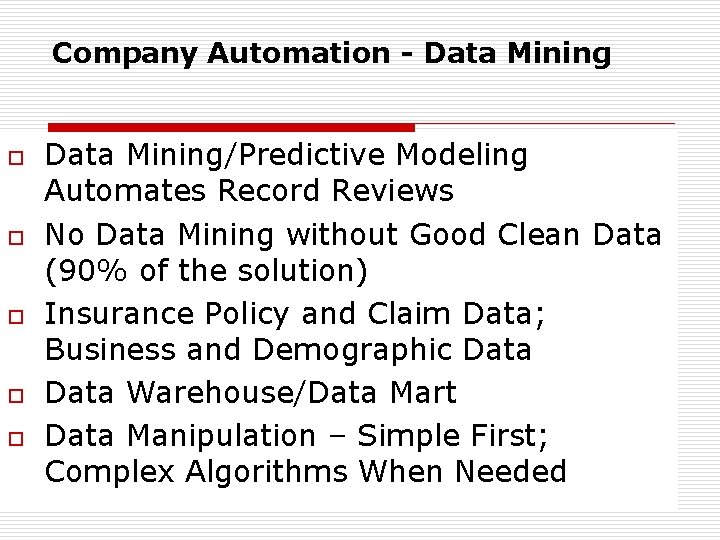
Company Automation - Data Mining o o o Data Mining/Predictive Modeling Automates Record Reviews No Data Mining without Good Clean Data (90% of the solution) Insurance Policy and Claim Data; Business and Demographic Data Warehouse/Data Mart Data Manipulation – Simple First; Complex Algorithms When Needed
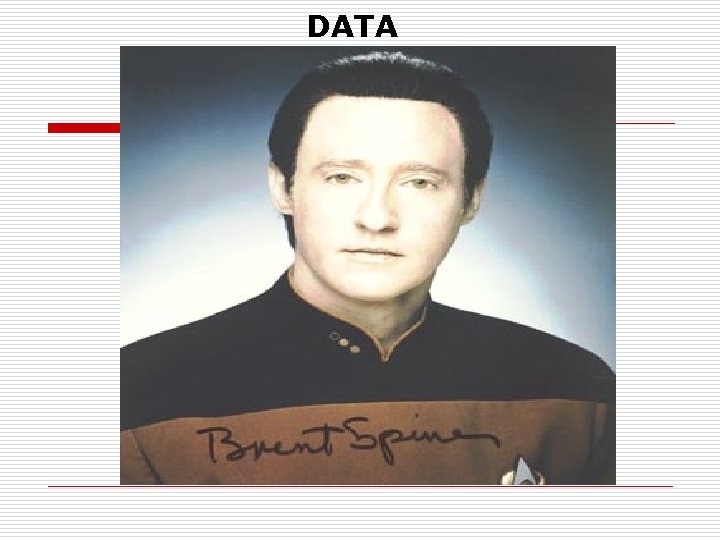
DATA
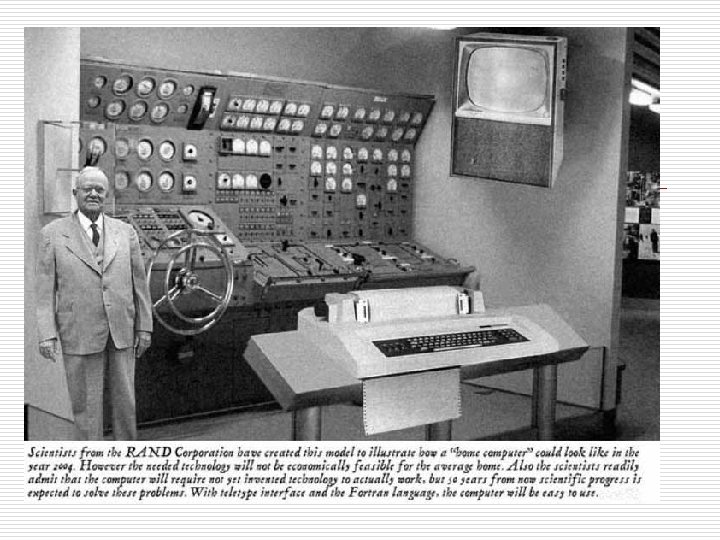
Computers advance
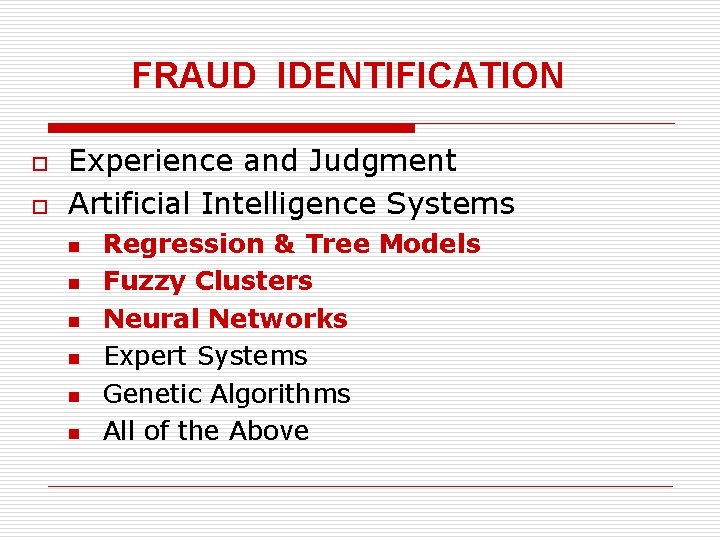
FRAUD IDENTIFICATION o o Experience and Judgment Artificial Intelligence Systems n n n Regression & Tree Models Fuzzy Clusters Neural Networks Expert Systems Genetic Algorithms All of the Above
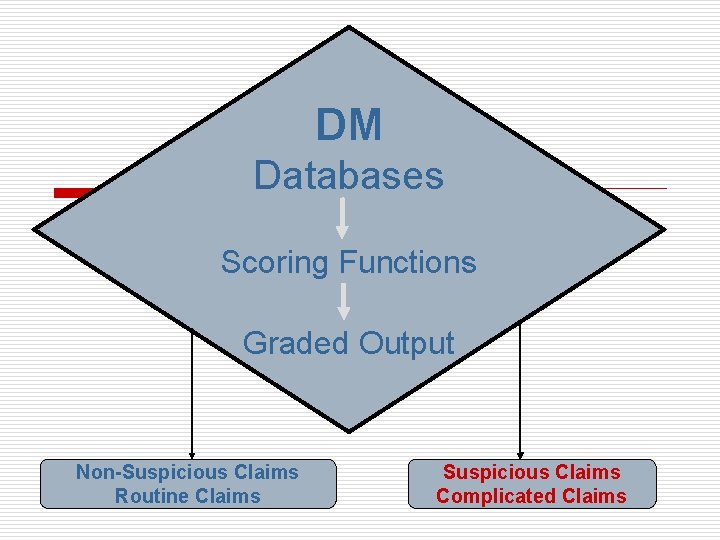
DM Databases Scoring Functions Graded Output Non-Suspicious Claims Routine Claims Suspicious Claims Complicated Claims
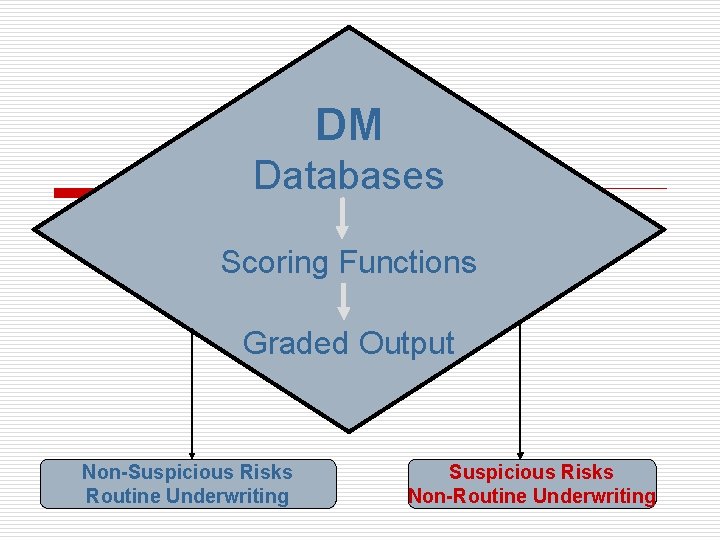
DM Databases Scoring Functions Graded Output Non-Suspicious Risks Routine Underwriting Suspicious Risks Non-Routine Underwriting
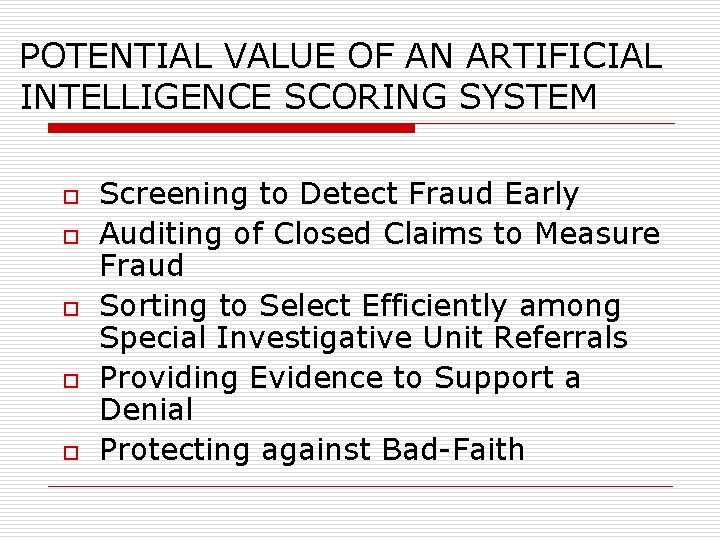
POTENTIAL VALUE OF AN ARTIFICIAL INTELLIGENCE SCORING SYSTEM o o o Screening to Detect Fraud Early Auditing of Closed Claims to Measure Fraud Sorting to Select Efficiently among Special Investigative Unit Referrals Providing Evidence to Support a Denial Protecting against Bad-Faith
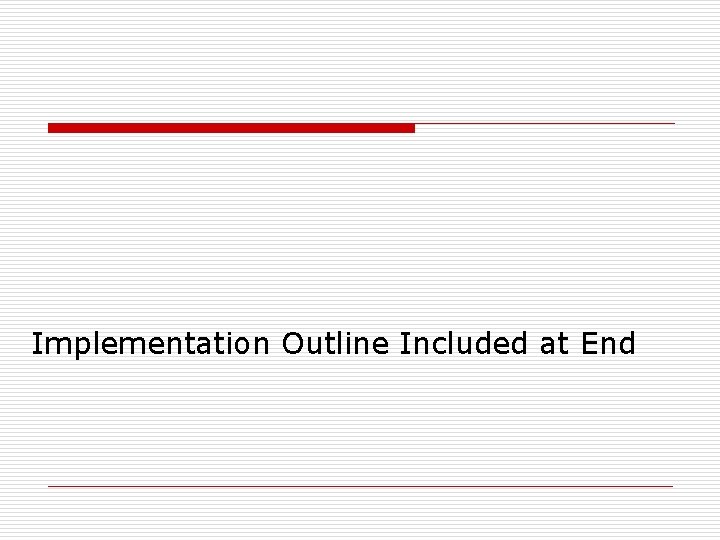
Implementation Outline Included at End
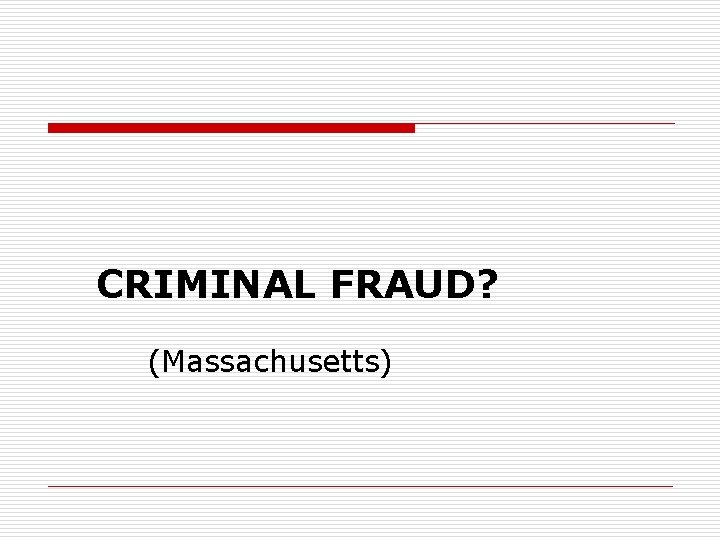
CRIMINAL FRAUD? (Massachusetts)
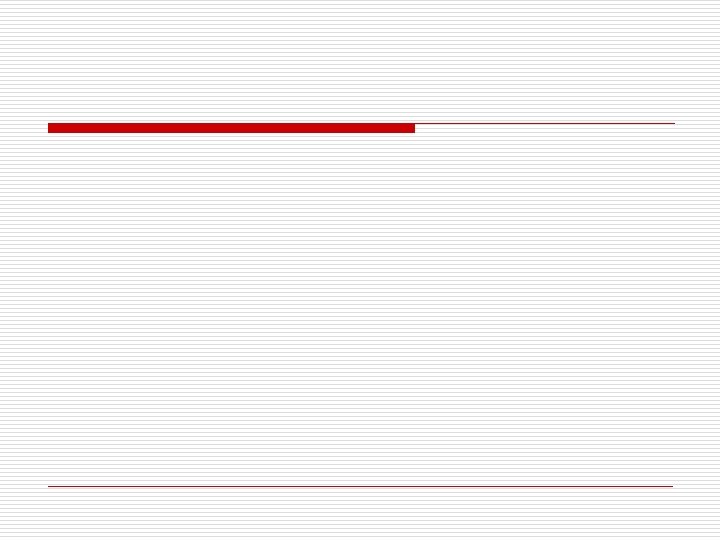
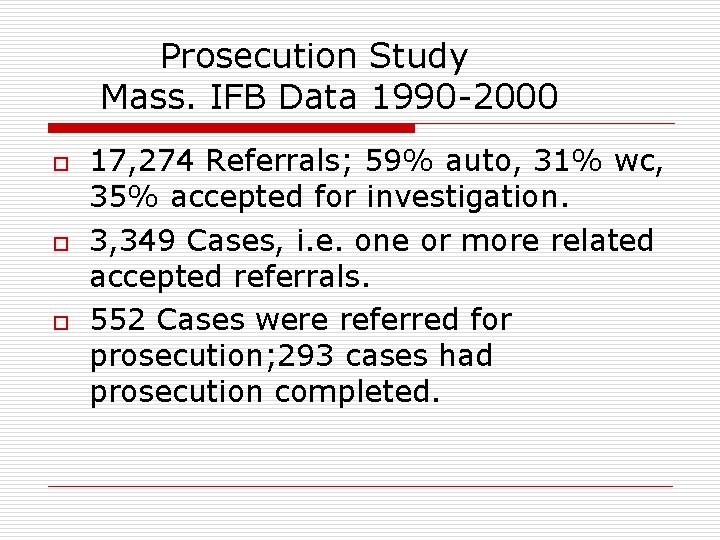
Prosecution Study Mass. IFB Data 1990 -2000 o o o 17, 274 Referrals; 59% auto, 31% wc, 35% accepted for investigation. 3, 349 Cases, i. e. one or more related accepted referrals. 552 Cases were referred for prosecution; 293 cases had prosecution completed.
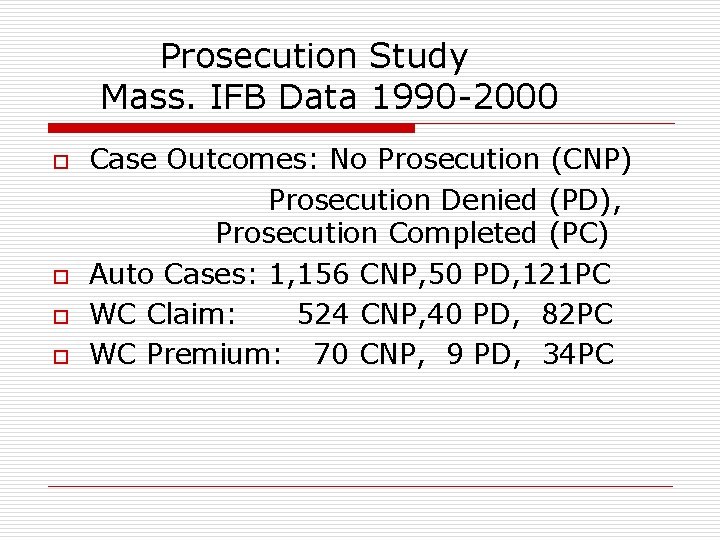
Prosecution Study Mass. IFB Data 1990 -2000 Case Outcomes: No Prosecution (CNP) Prosecution Denied (PD), Prosecution Completed (PC) o Auto Cases: 1, 156 CNP, 50 PD, 121 PC o WC Claim: 524 CNP, 40 PD, 82 PC o WC Premium: 70 CNP, 9 PD, 34 PC o
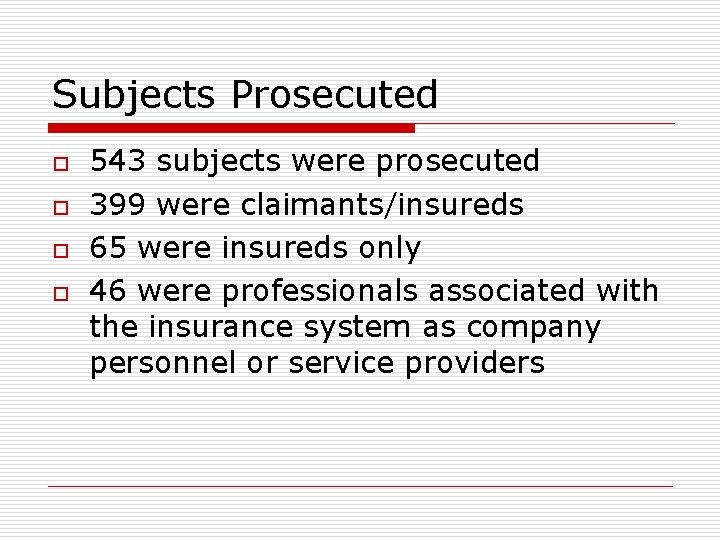
Subjects Prosecuted o o 543 subjects were prosecuted 399 were claimants/insureds 65 were insureds only 46 were professionals associated with the insurance system as company personnel or service providers
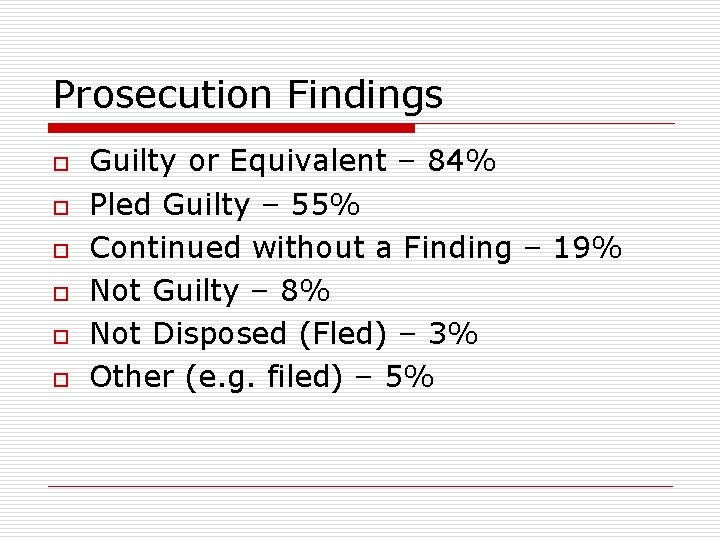
Prosecution Findings o o o Guilty or Equivalent – 84% Pled Guilty – 55% Continued without a Finding – 19% Not Guilty – 8% Not Disposed (Fled) – 3% Other (e. g. filed) – 5%
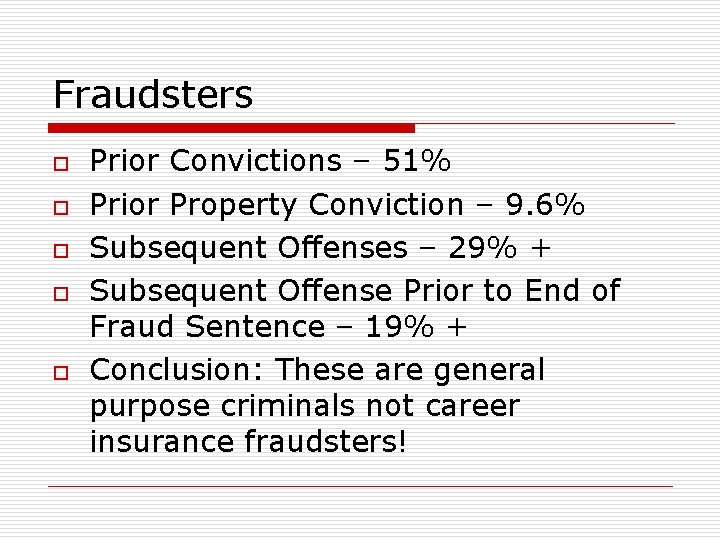
Fraudsters o o o Prior Convictions – 51% Prior Property Conviction – 9. 6% Subsequent Offenses – 29% + Subsequent Offense Prior to End of Fraud Sentence – 19% + Conclusion: These are general purpose criminals not career insurance fraudsters!
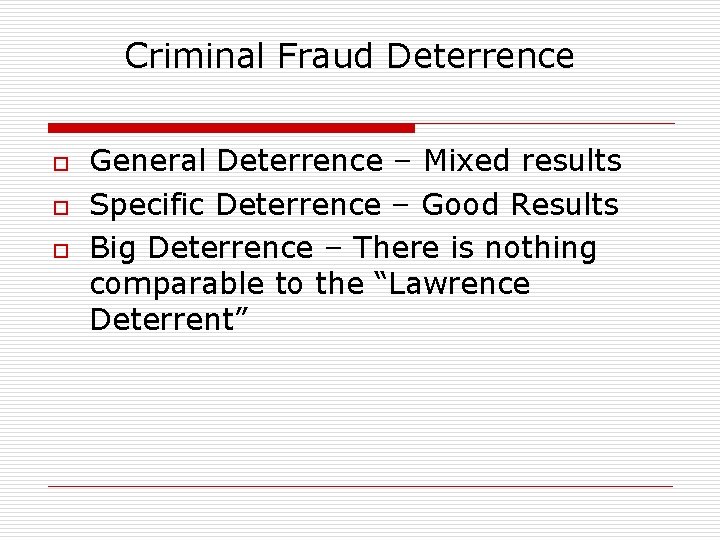
Criminal Fraud Deterrence o o o General Deterrence – Mixed results Specific Deterrence – Good Results Big Deterrence – There is nothing comparable to the “Lawrence Deterrent”
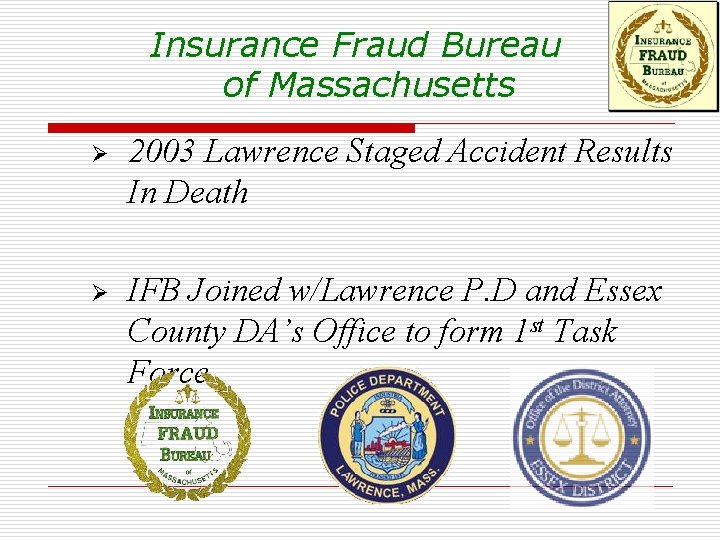
Insurance Fraud Bureau of Massachusetts Ø 2003 Lawrence Staged Accident Results In Death Ø IFB Joined w/Lawrence P. D and Essex County DA’s Office to form 1 st Task Force
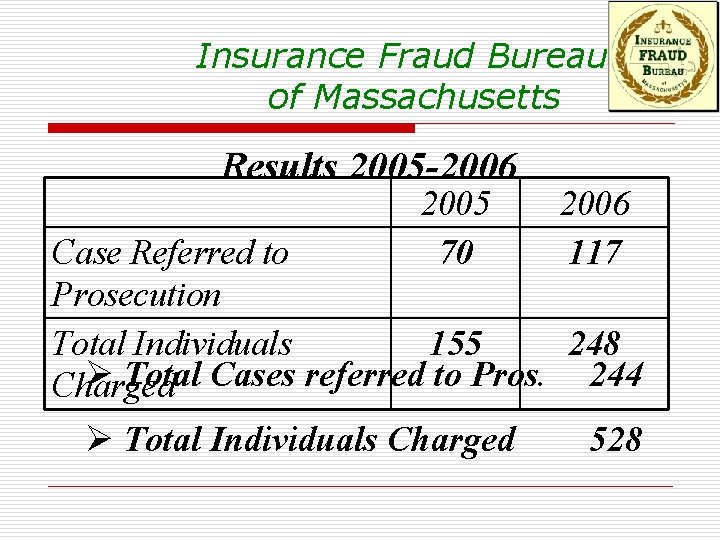
Insurance Fraud Bureau of Massachusetts Results 2005 -2006 2005 70 2006 117 Case Referred to Prosecution Total Individuals 155 248 Ø Total Cases referred to Pros. 244 Charged Ø Total Individuals Charged 528
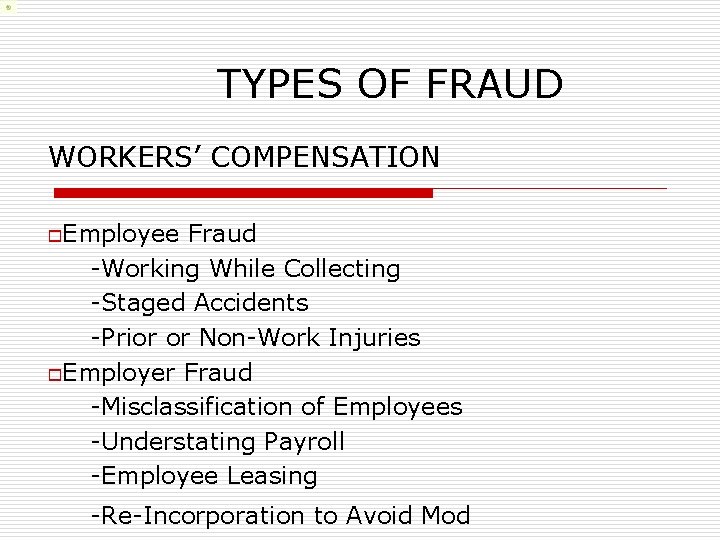
TYPES OF FRAUD WORKERS’ COMPENSATION o. Employee Fraud -Working While Collecting -Staged Accidents -Prior or Non-Work Injuries o. Employer Fraud -Misclassification of Employees -Understating Payroll -Employee Leasing -Re-Incorporation to Avoid Mod
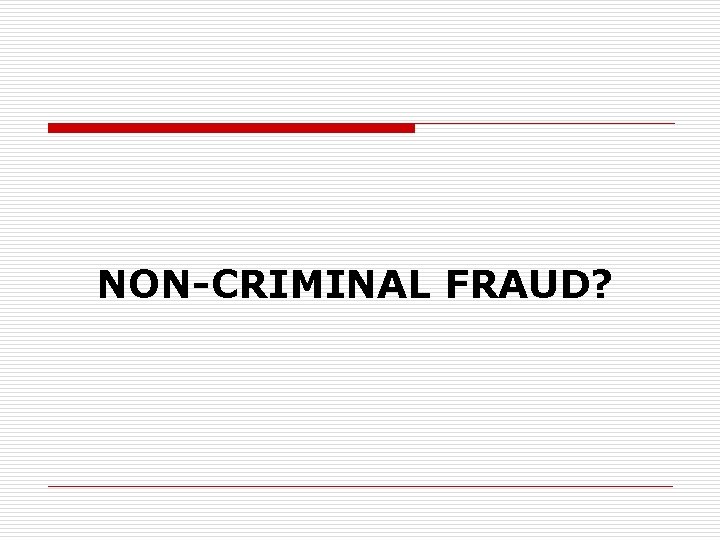
NON-CRIMINAL FRAUD?
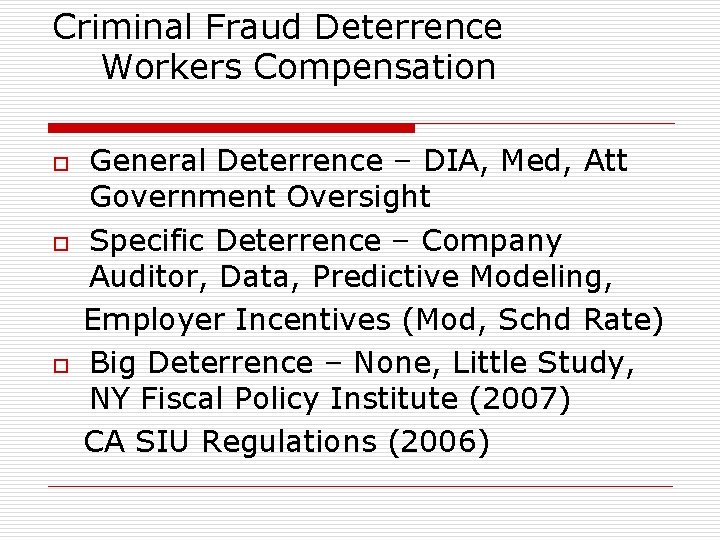
Criminal Fraud Deterrence Workers Compensation General Deterrence – DIA, Med, Att Government Oversight o Specific Deterrence – Company Auditor, Data, Predictive Modeling, Employer Incentives (Mod, Schd Rate) o Big Deterrence – None, Little Study, NY Fiscal Policy Institute (2007) CA SIU Regulations (2006) o
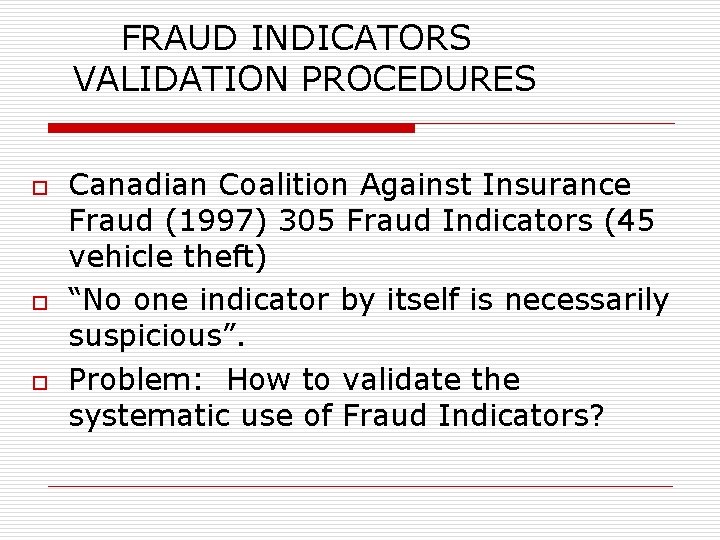
FRAUD INDICATORS VALIDATION PROCEDURES o o o Canadian Coalition Against Insurance Fraud (1997) 305 Fraud Indicators (45 vehicle theft) “No one indicator by itself is necessarily suspicious”. Problem: How to validate the systematic use of Fraud Indicators?
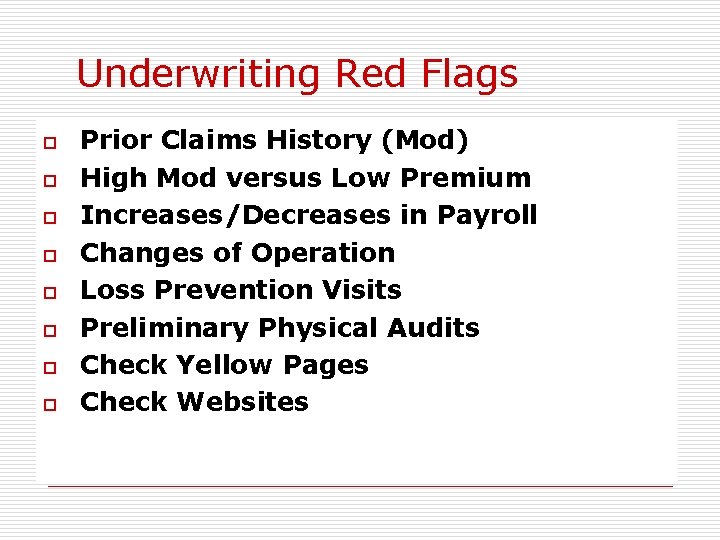
Underwriting Red Flags o o o o Prior Claims History (Mod) High Mod versus Low Premium Increases/Decreases in Payroll Changes of Operation Loss Prevention Visits Preliminary Physical Audits Check Yellow Pages Check Websites
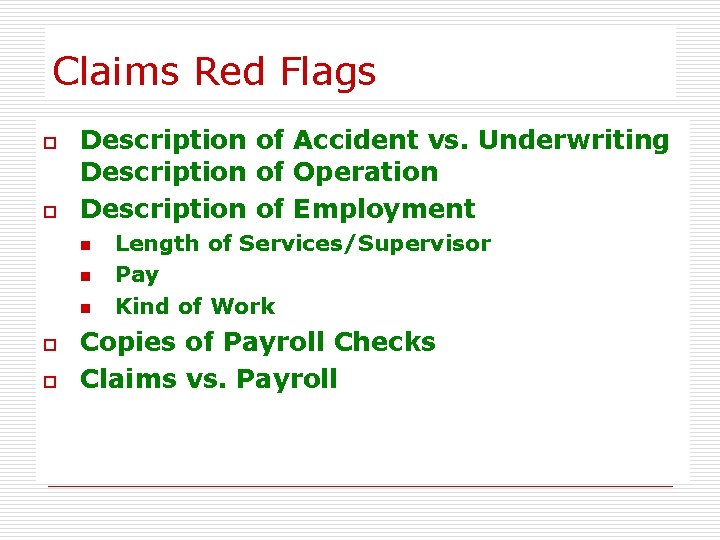
Claims Red Flags o o Description of Accident vs. Underwriting Description of Operation Description of Employment n n n o o Length of Services/Supervisor Pay Kind of Work Copies of Payroll Checks Claims vs. Payroll
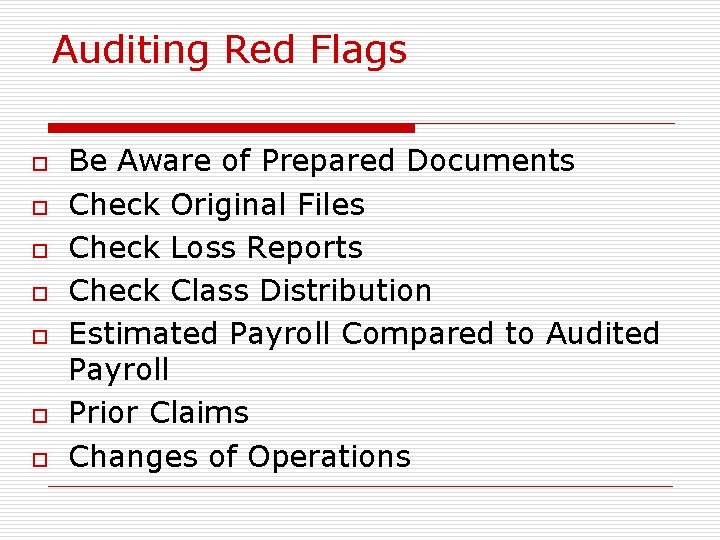
Auditing Red Flags o o o o Be Aware of Prepared Documents Check Original Files Check Loss Reports Check Class Distribution Estimated Payroll Compared to Audited Payroll Prior Claims Changes of Operations
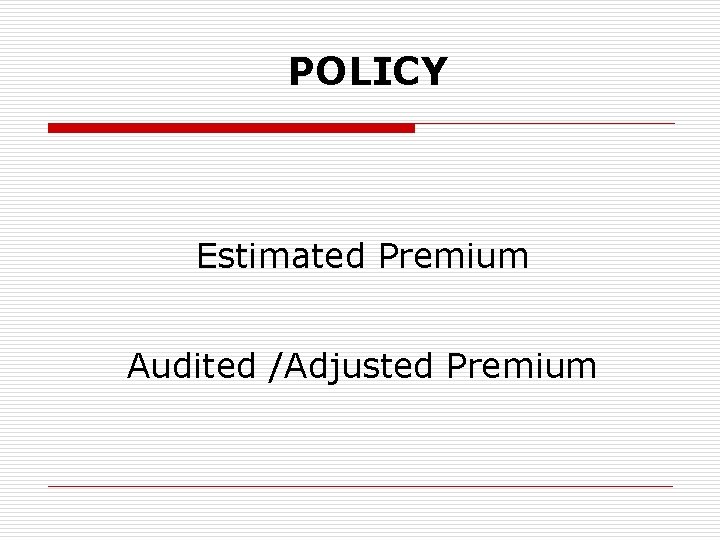
POLICY Estimated Premium Audited /Adjusted Premium
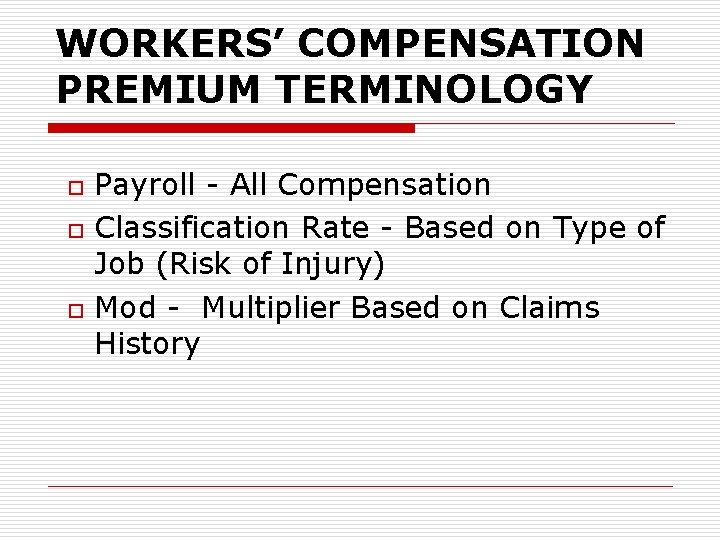
WORKERS’ COMPENSATION PREMIUM TERMINOLOGY o o o Payroll - All Compensation Classification Rate - Based on Type of Job (Risk of Injury) Mod - Multiplier Based on Claims History
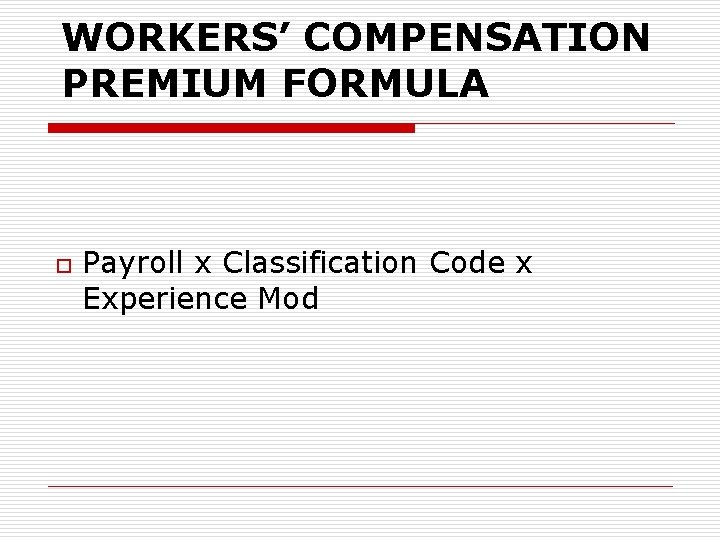
WORKERS’ COMPENSATION PREMIUM FORMULA o Payroll x Classification Code x Experience Mod
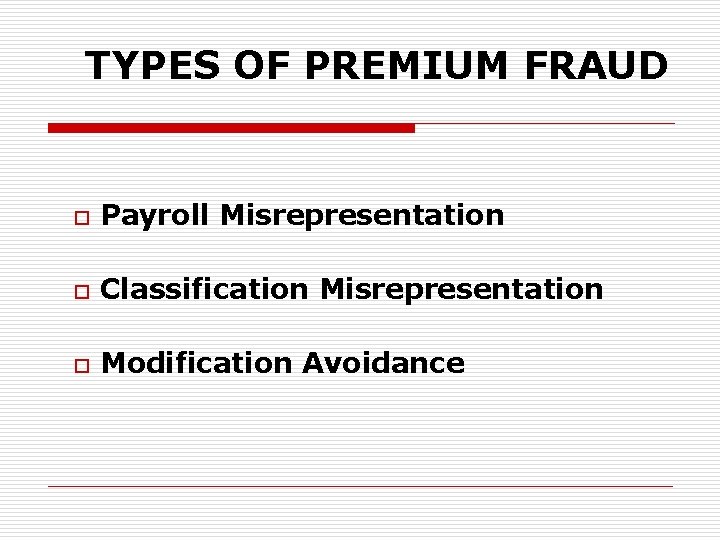
TYPES OF PREMIUM FRAUD o Payroll Misrepresentation o Classification Misrepresentation o Modification Avoidance
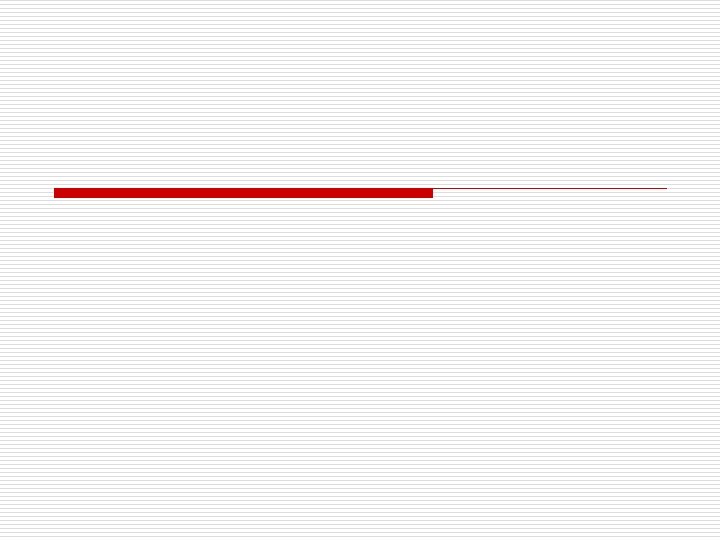
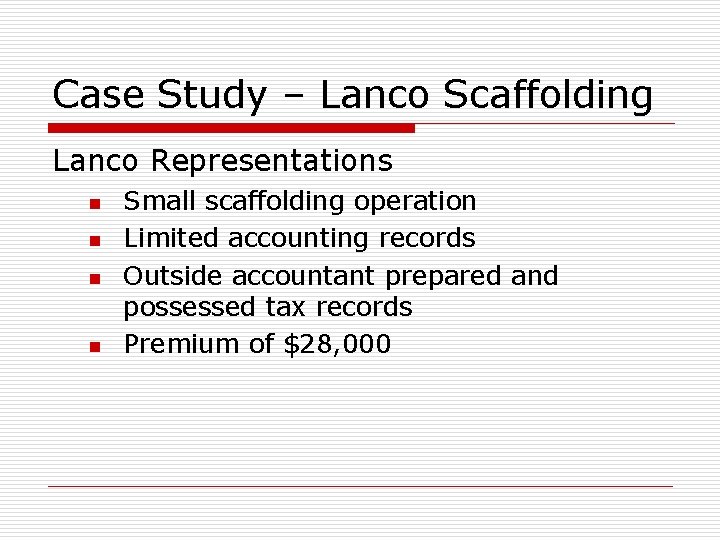
Case Study – Lanco Scaffolding Lanco Representations n n Small scaffolding operation Limited accounting records Outside accountant prepared and possessed tax records Premium of $28, 000
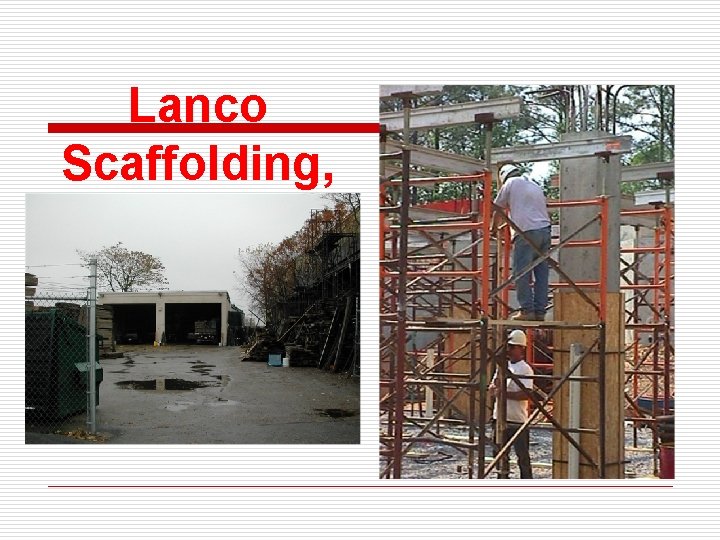
Lanco Scaffolding, Inc.
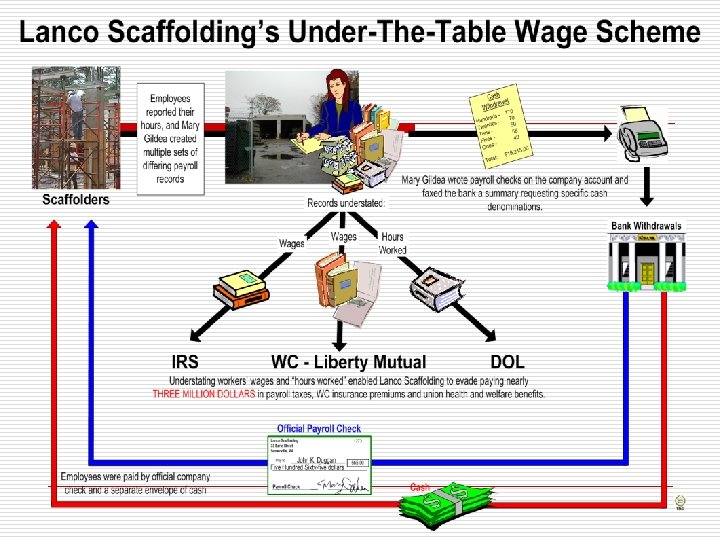
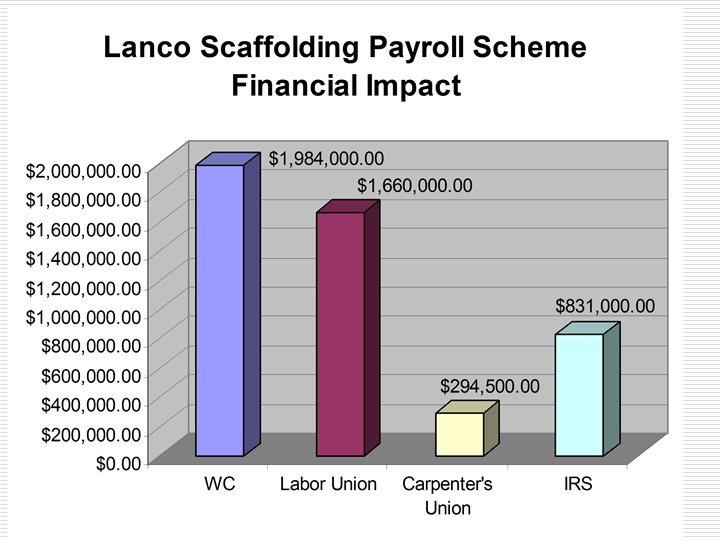
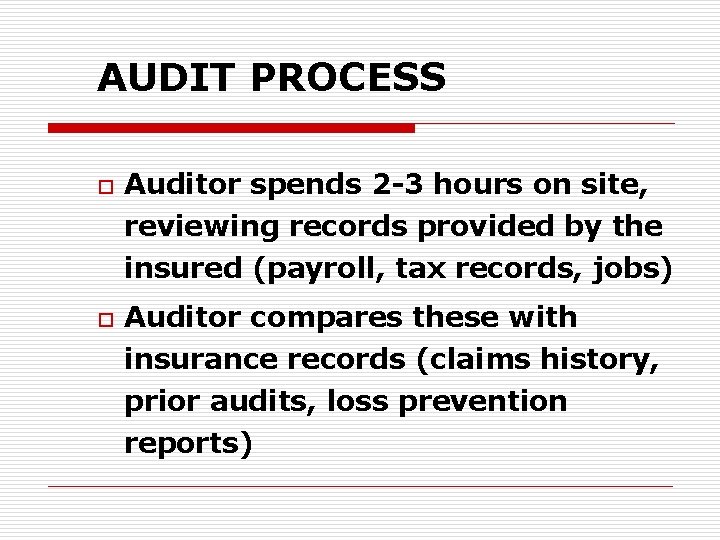
AUDIT PROCESS o Auditor spends 2 -3 hours on site, reviewing records provided by the insured (payroll, tax records, jobs) o Auditor compares these with insurance records (claims history, prior audits, loss prevention reports)
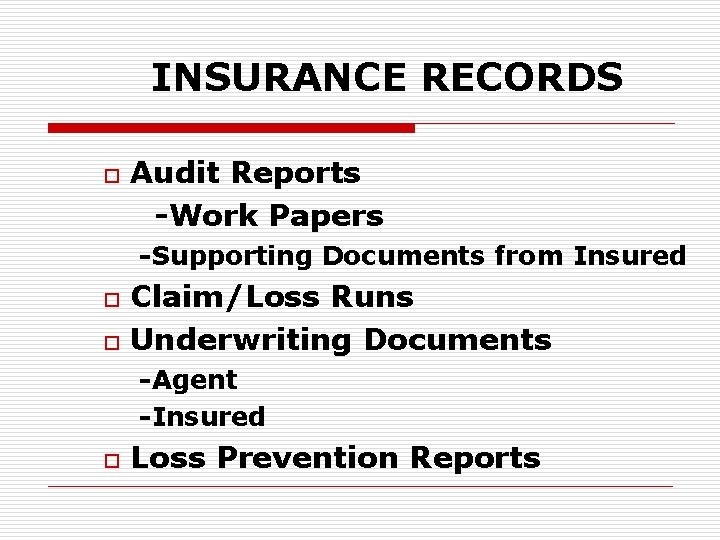
INSURANCE RECORDS o Audit Reports -Work Papers -Supporting Documents from Insured o o Claim/Loss Runs Underwriting Documents -Agent -Insured o Loss Prevention Reports
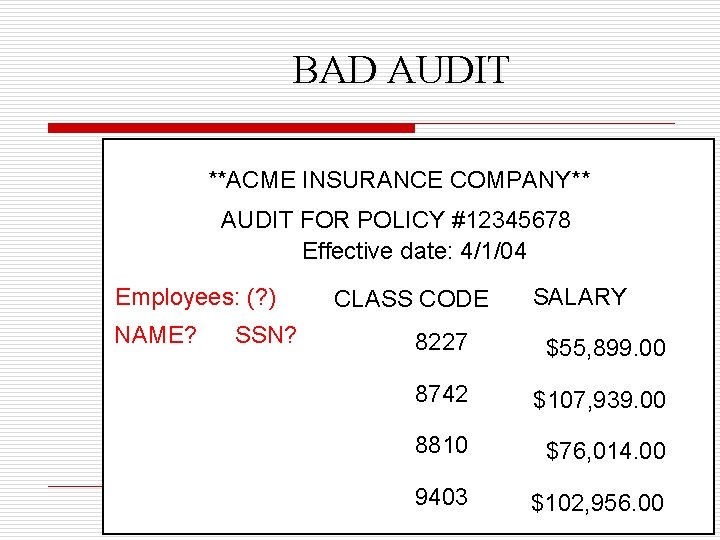
BAD AUDIT **ACME INSURANCE COMPANY** AUDIT FOR POLICY #12345678 Effective date: 4/1/04 Employees: (? ) NAME? SSN? CLASS CODE SALARY 8227 $55, 899. 00 8742 $107, 939. 00 8810 $76, 014. 00 9403 $102, 956. 00
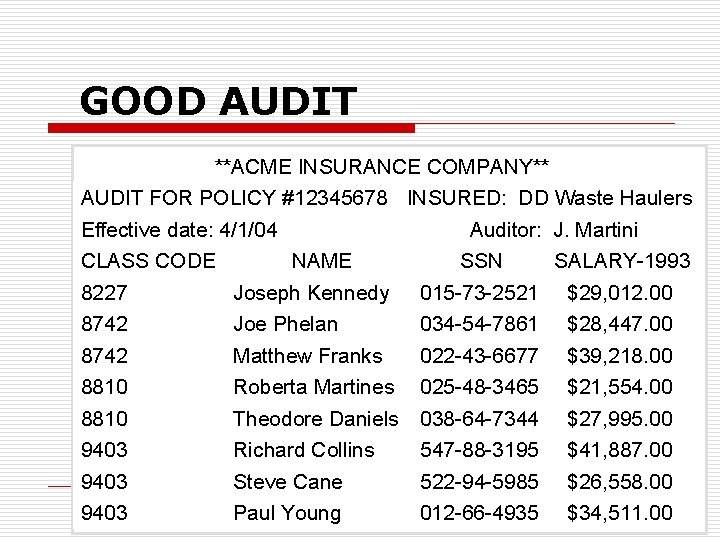
GOOD AUDIT **ACME INSURANCE COMPANY** AUDIT FOR POLICY #12345678 INSURED: DD Waste Haulers Effective date: 4/1/04 Auditor: J. Martini CLASS CODE 8227 8742 8810 NAME Joseph Kennedy Joe Phelan Matthew Franks Roberta Martines Theodore Daniels SSN SALARY-1993 015 -73 -2521 $29, 012. 00 034 -54 -7861 $28, 447. 00 022 -43 -6677 $39, 218. 00 025 -48 -3465 $21, 554. 00 038 -64 -7344 $27, 995. 00 9403 Richard Collins Steve Cane Paul Young 547 -88 -3195 522 -94 -5985 012 -66 -4935 $41, 887. 00 $26, 558. 00 $34, 511. 00
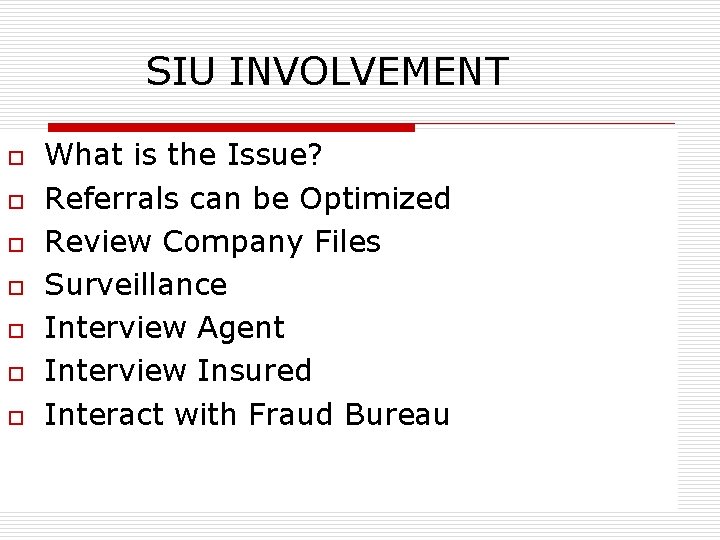
SIU INVOLVEMENT o o o o What is the Issue? Referrals can be Optimized Review Company Files Surveillance Interview Agent Interview Insured Interact with Fraud Bureau
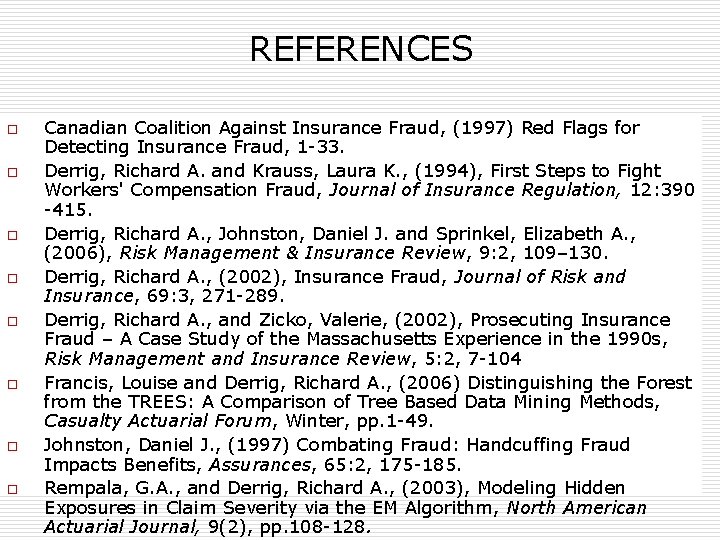
REFERENCES o o o o Canadian Coalition Against Insurance Fraud, (1997) Red Flags for Detecting Insurance Fraud, 1 -33. Derrig, Richard A. and Krauss, Laura K. , (1994), First Steps to Fight Workers' Compensation Fraud, Journal of Insurance Regulation, 12: 390 -415. Derrig, Richard A. , Johnston, Daniel J. and Sprinkel, Elizabeth A. , (2006), Risk Management & Insurance Review, 9: 2, 109– 130. Derrig, Richard A. , (2002), Insurance Fraud, Journal of Risk and Insurance, 69: 3, 271 -289. Derrig, Richard A. , and Zicko, Valerie, (2002), Prosecuting Insurance Fraud – A Case Study of the Massachusetts Experience in the 1990 s, Risk Management and Insurance Review, 5: 2, 7 -104 Francis, Louise and Derrig, Richard A. , (2006) Distinguishing the Forest from the TREES: A Comparison of Tree Based Data Mining Methods, Casualty Actuarial Forum, Winter, pp. 1 -49. Johnston, Daniel J. , (1997) Combating Fraud: Handcuffing Fraud Impacts Benefits, Assurances, 65: 2, 175 -185. Rempala, G. A. , and Derrig, Richard A. , (2003), Modeling Hidden Exposures in Claim Severity via the EM Algorithm, North American Actuarial Journal, 9(2), pp. 108 -128.
Workers compensation fraud detection
How do fraud symptoms help in detecting fraud
Noncompensation
Deterrence and rational choice theory of crime
Identity theft and assumption deterrence act
Data driven fraud detection
Fraud detection
Fraud detection conference
Sequence diagram for credit card fraud detection
Controlling workers compensation costs
Texas hcn workers compensation
Oregon workers compensation division
Maine workers compensation forms
Montana workers compensation court
Preventing cuts
Maine workers compensation forms
Specific deterrence
Specific deterrence
Looking for richard analysis
Ecommerce security issues
Ibm fraud and abuse management system
Fraud and abuse module
E commerce security and fraud protection
E-commerce security and fraud protection
Computer fraud and abuse techniques
Ethics fraud and internal control
Anti bribery and corruption analytics
Cash fraud and internal controls
Ethics and internal controls in accounting
Fraud waste and abuse training answers
Fraud waste and abuse training answers
Computer fraud and security
Distinguish between fraud and misrepresentation
Controlsbond
Chapter 7 fraud internal control and cash
The entry to establish a petty cash fund includes
Ethics fraud and internal control
Define headright system
Sfcu medford
Risk assessment fraud
Synthetic statement example
Lau hee teah v hargill engineering sdn bhd
Donald cressey
Evidence square fraud
Fraud exposure rectangle
Fraud triangle accounting
Fraud exposure rectangle