Financial Risk Management Zvi Wiener Following P Jorion
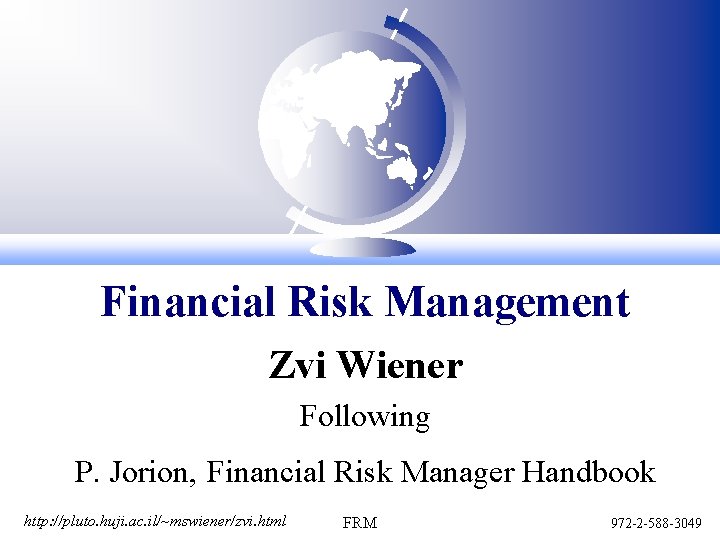
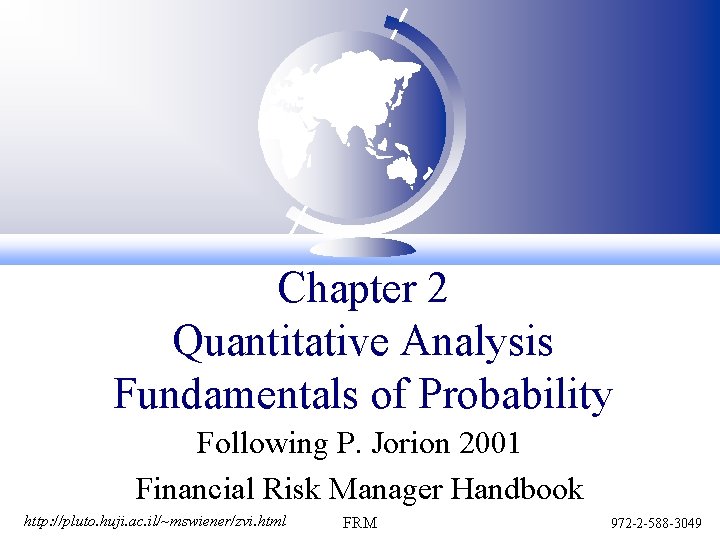
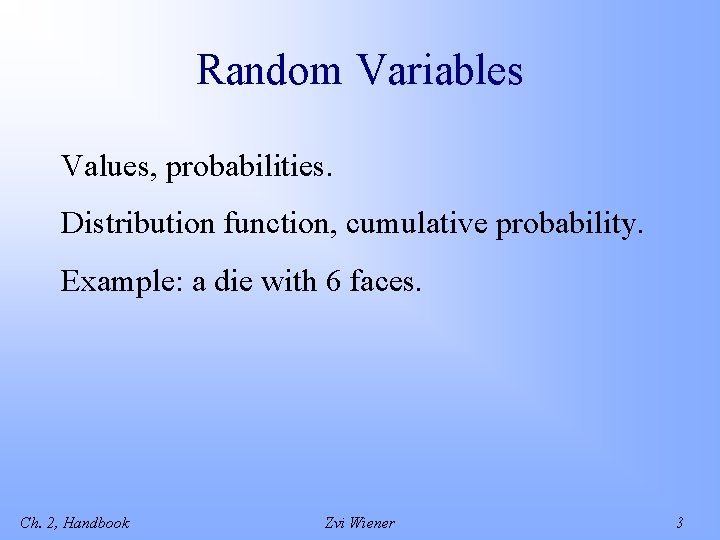
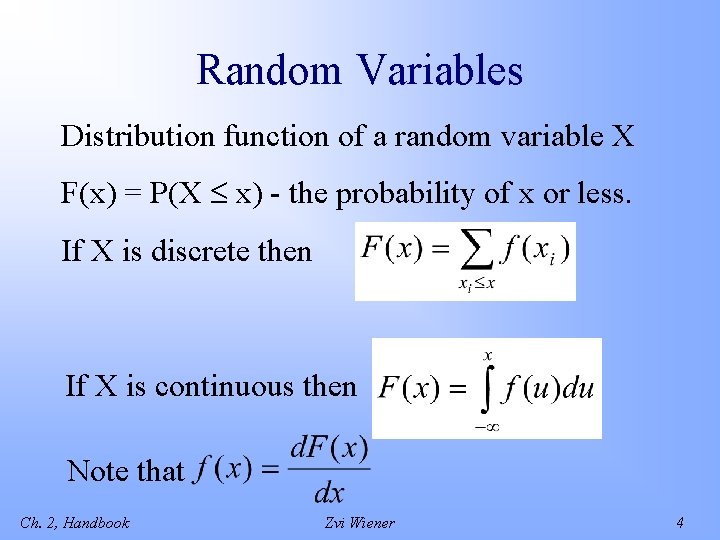
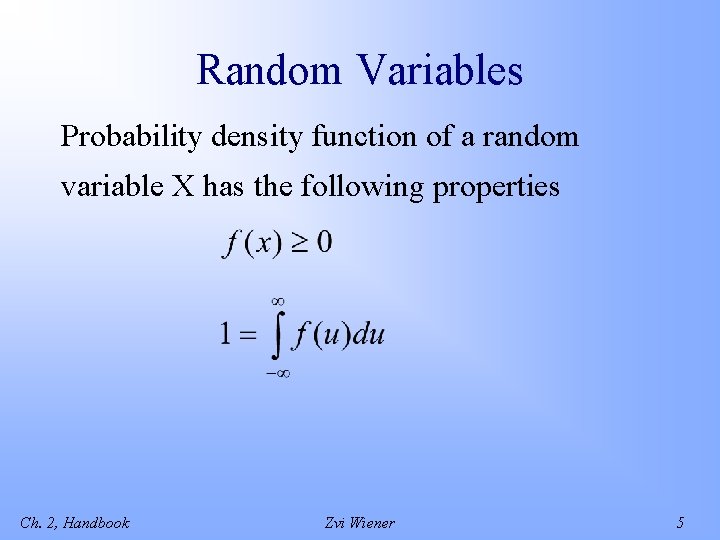
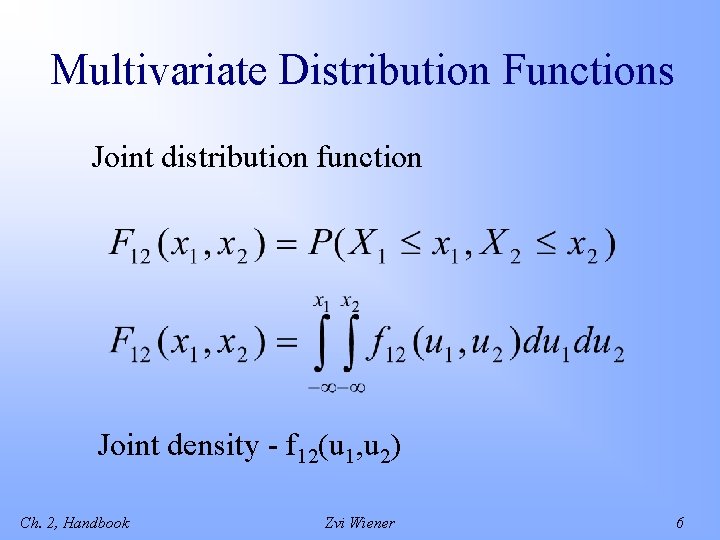
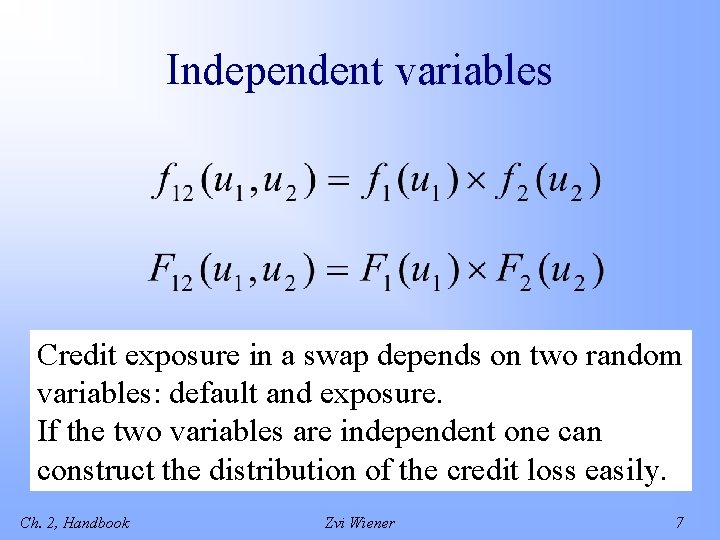
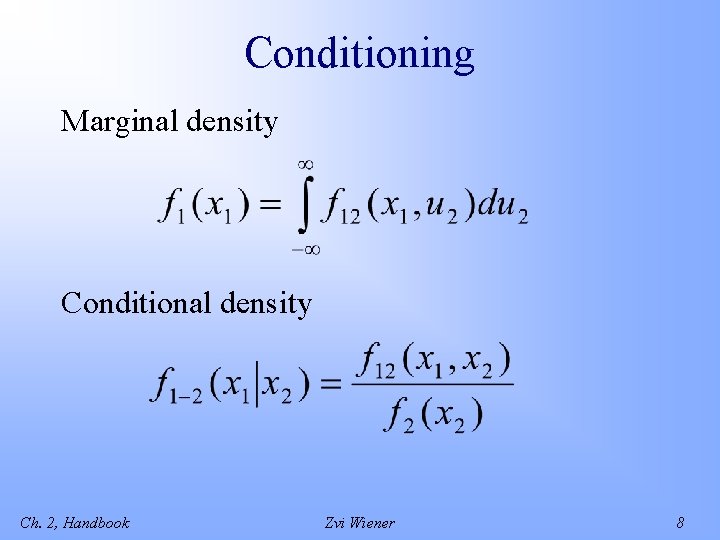
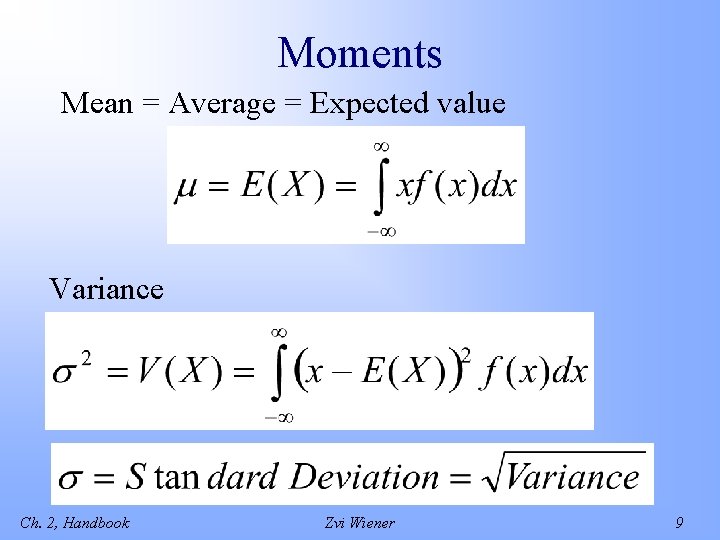
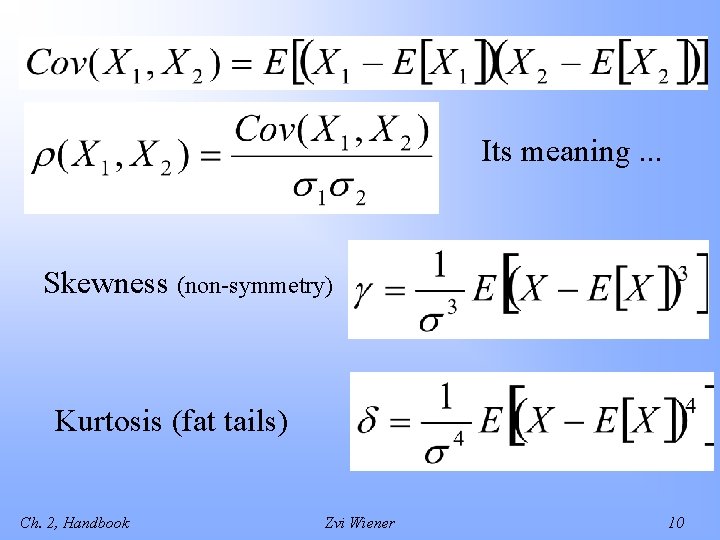
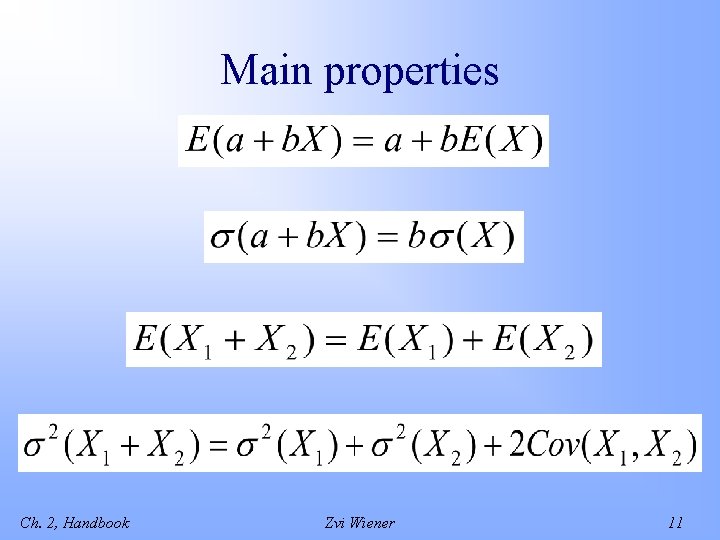
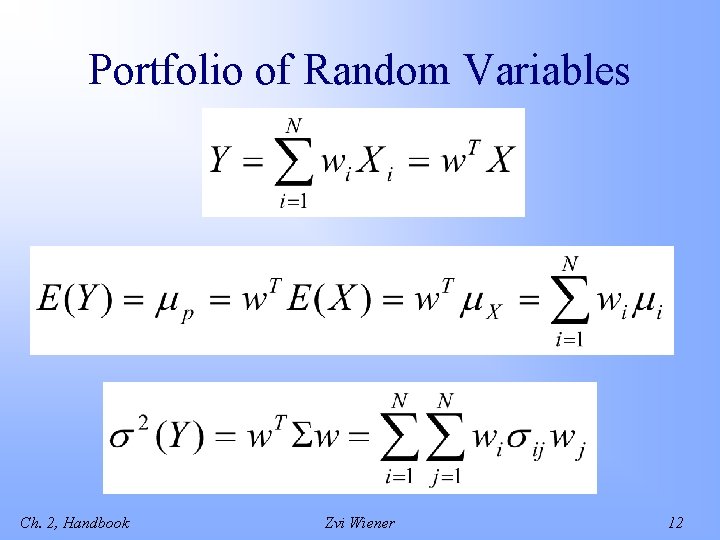
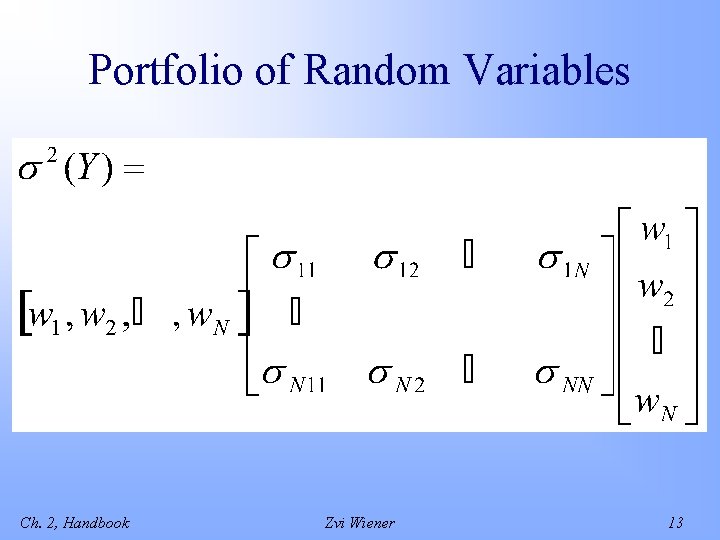
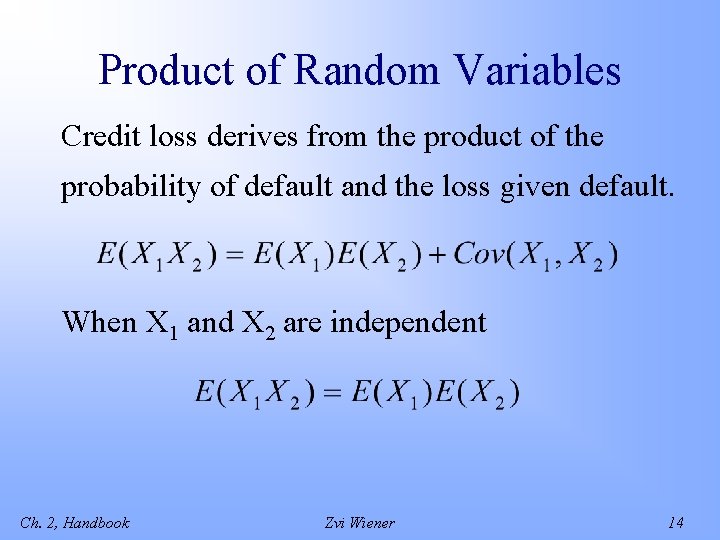
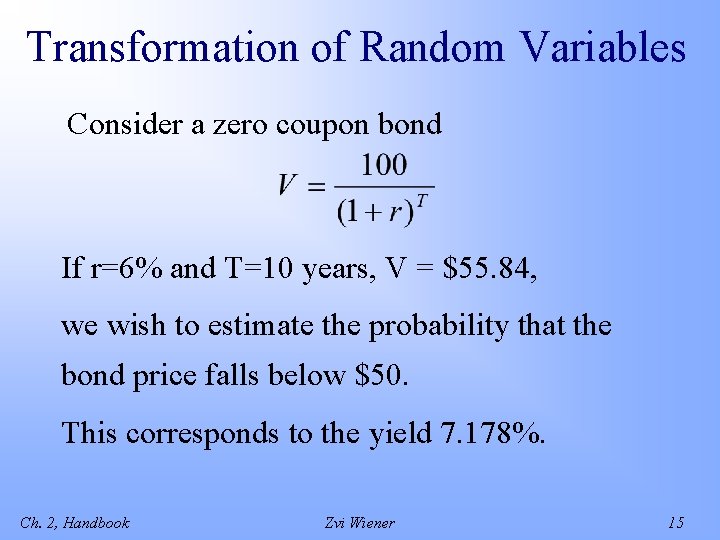
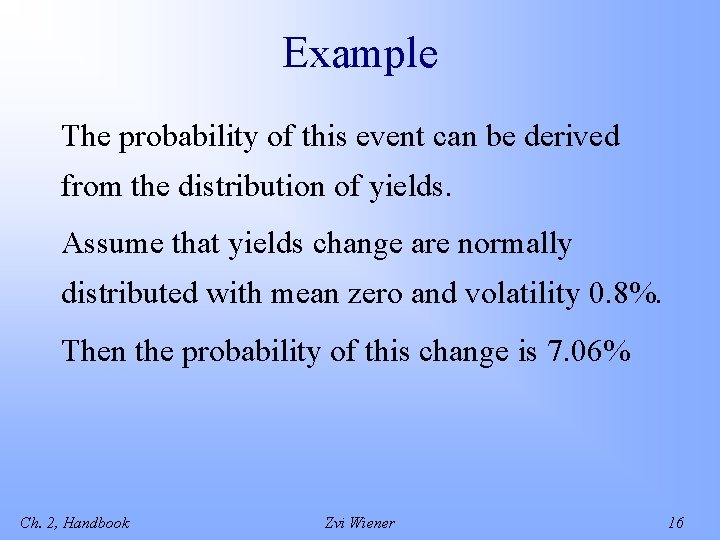
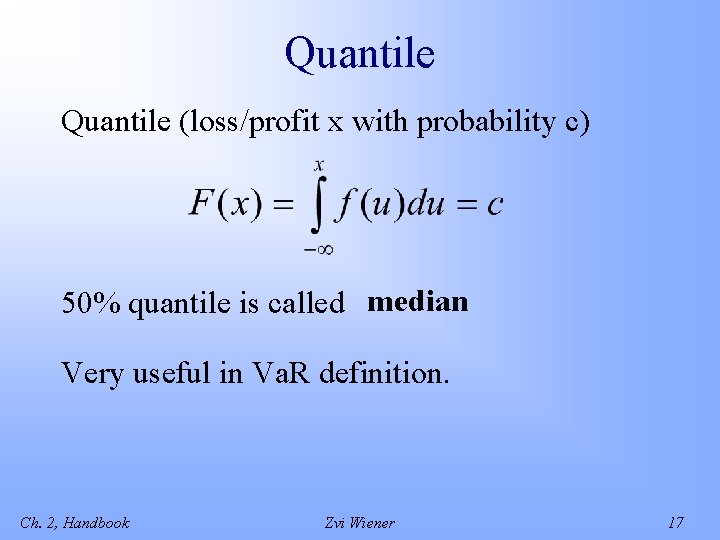
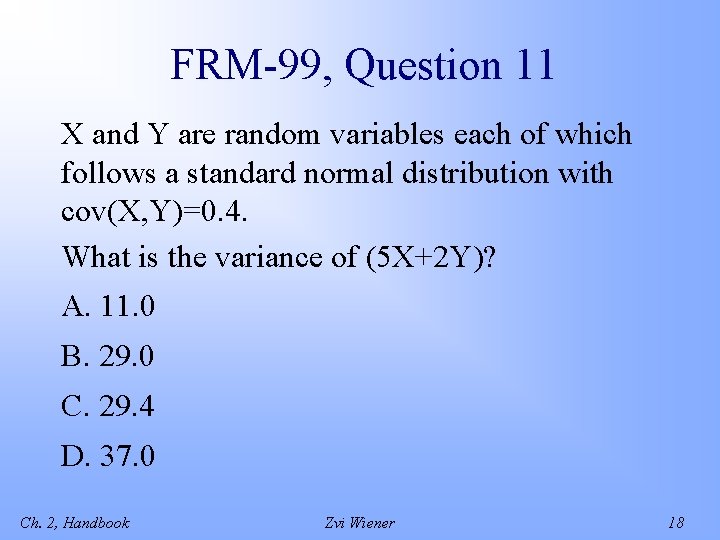
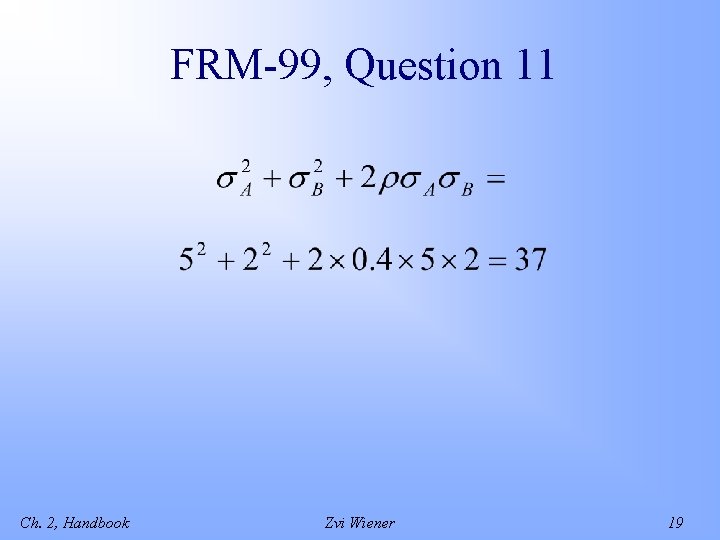
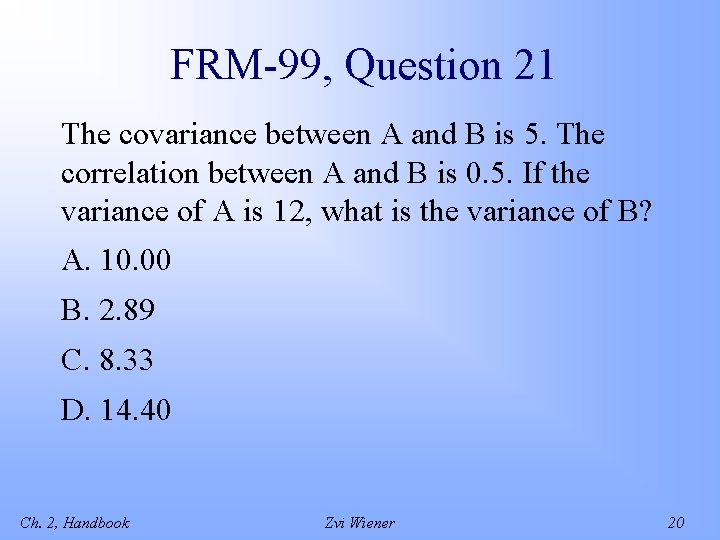
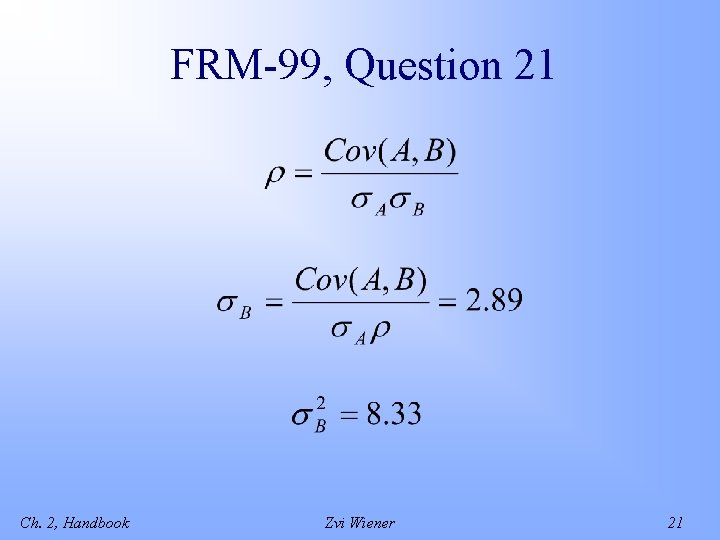
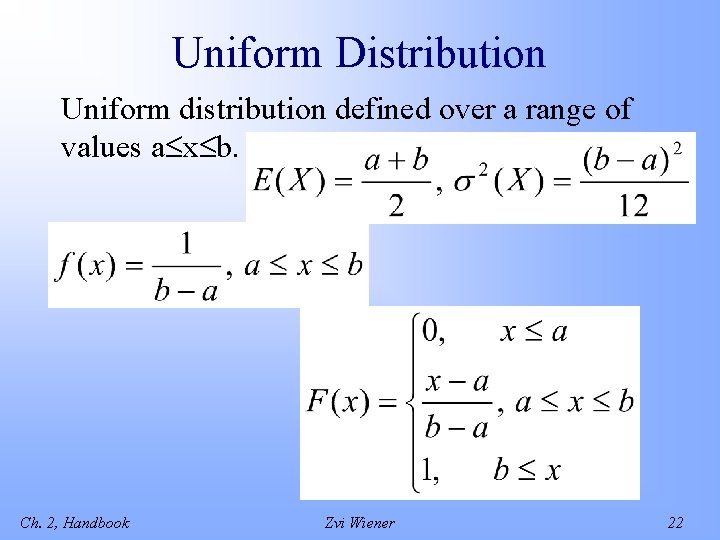
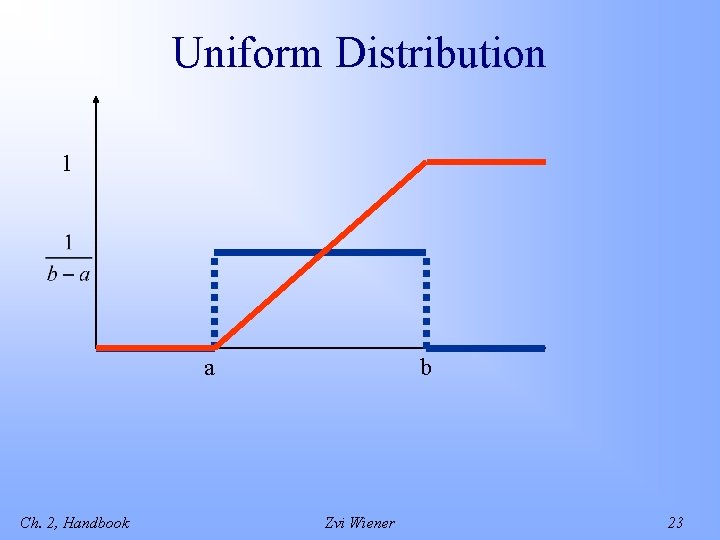
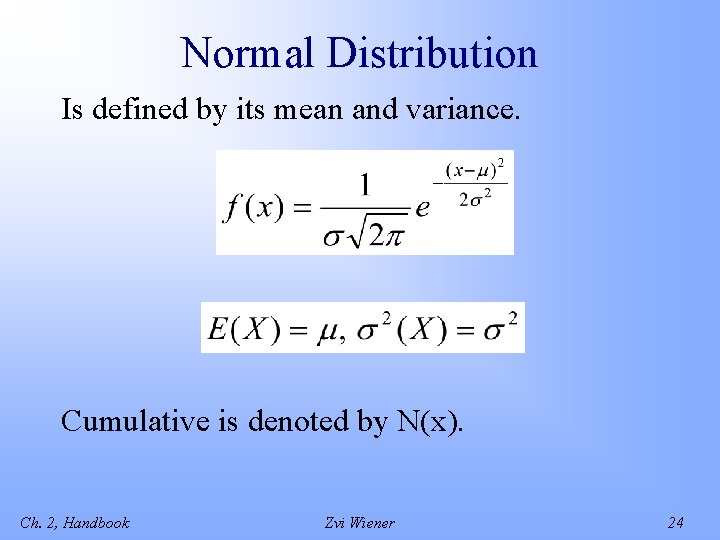
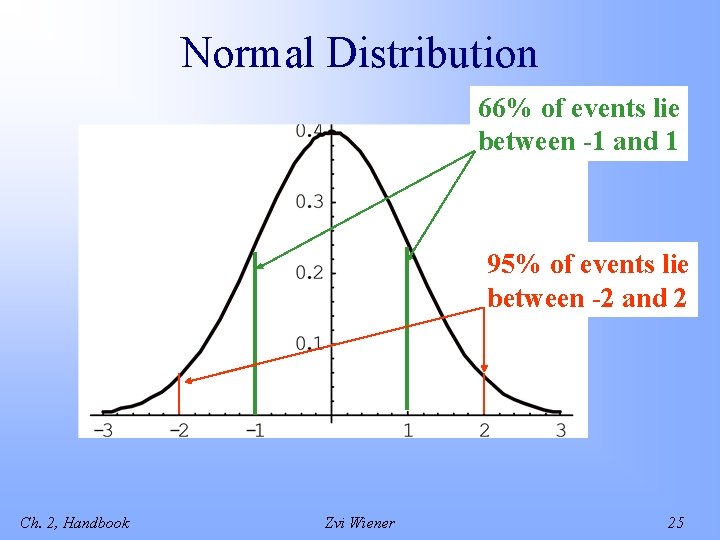
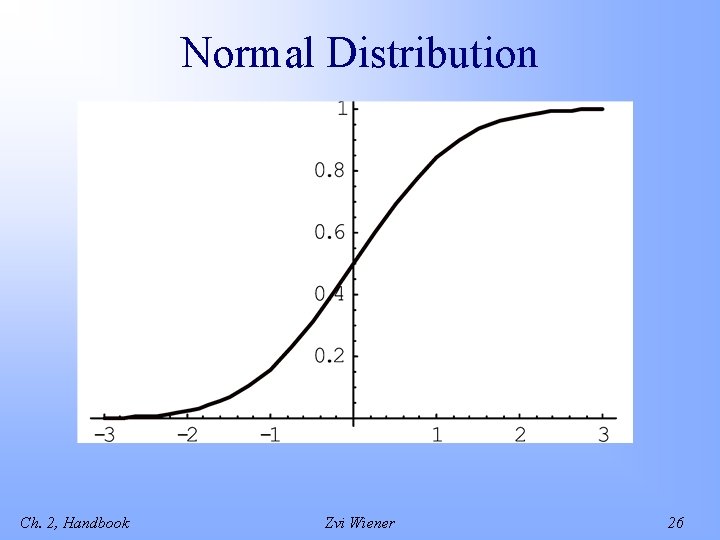
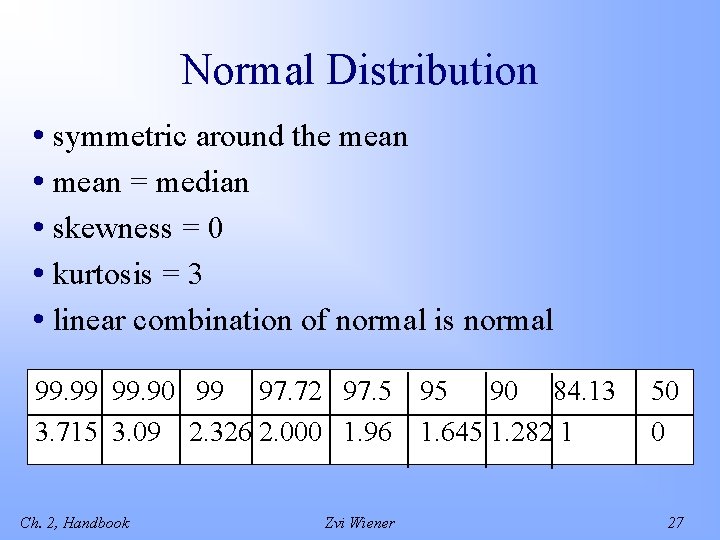
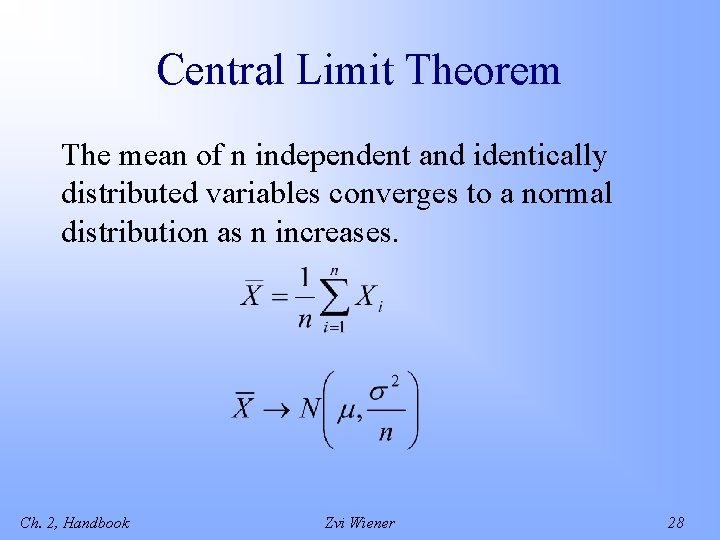
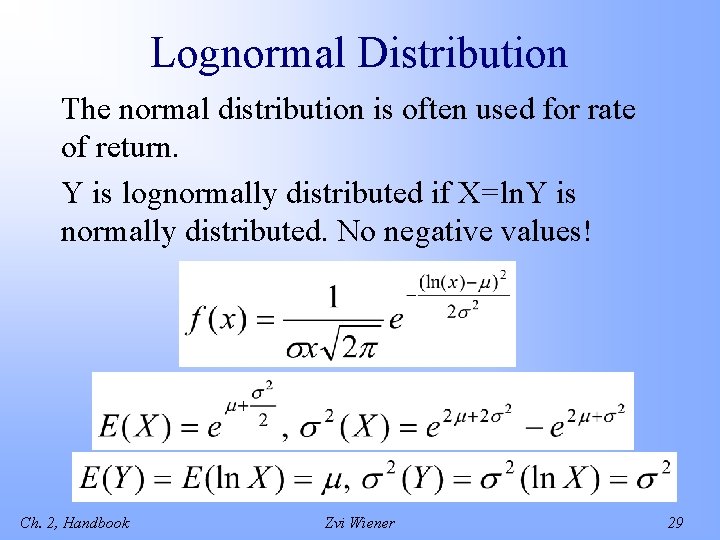
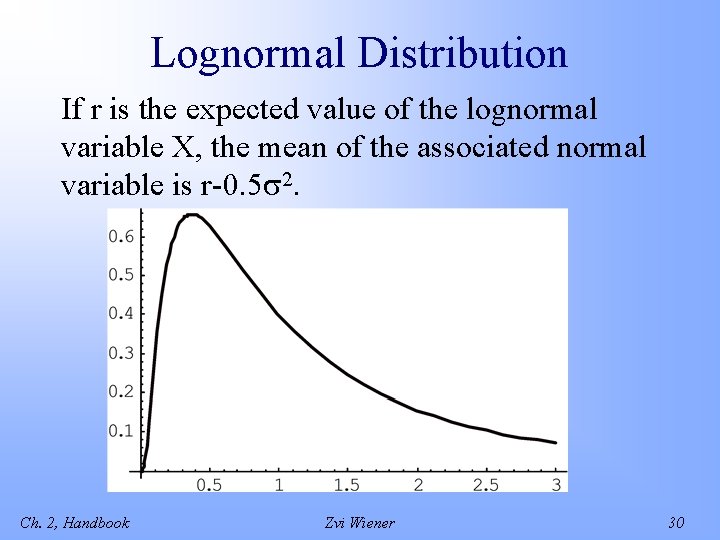
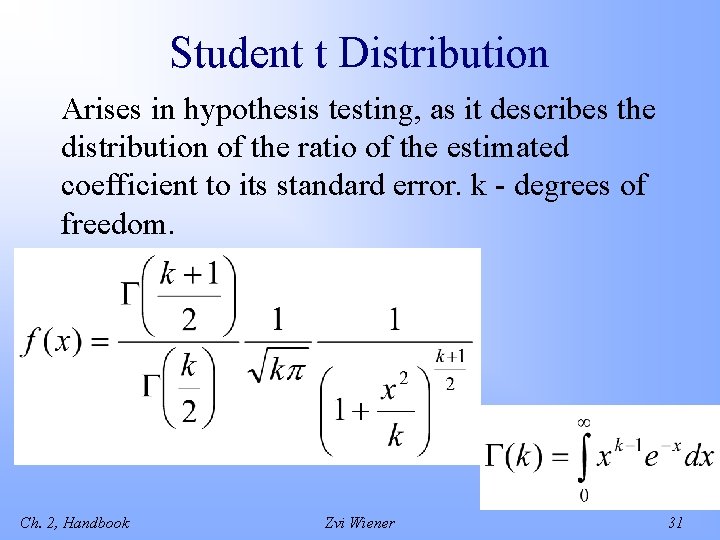
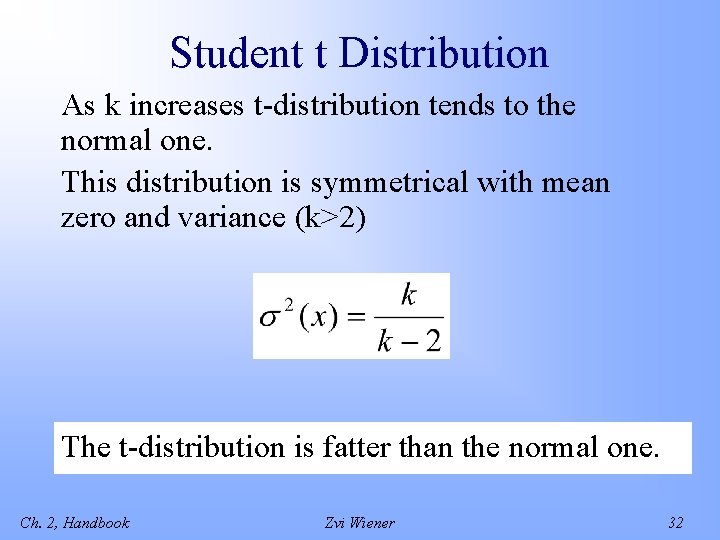
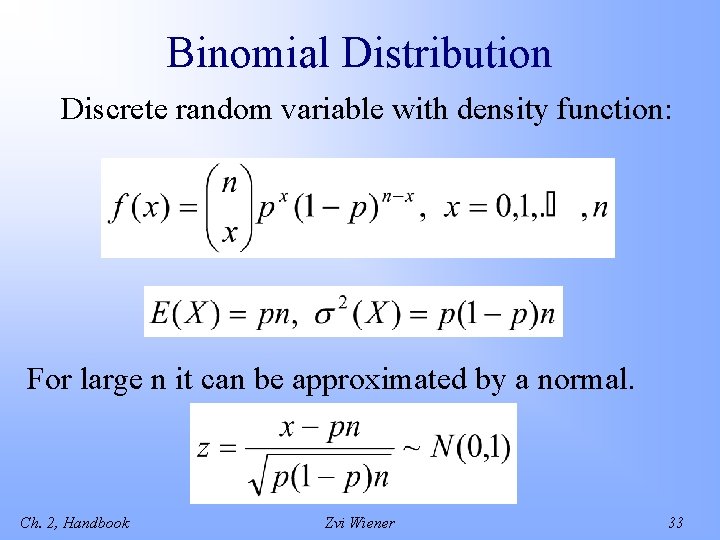
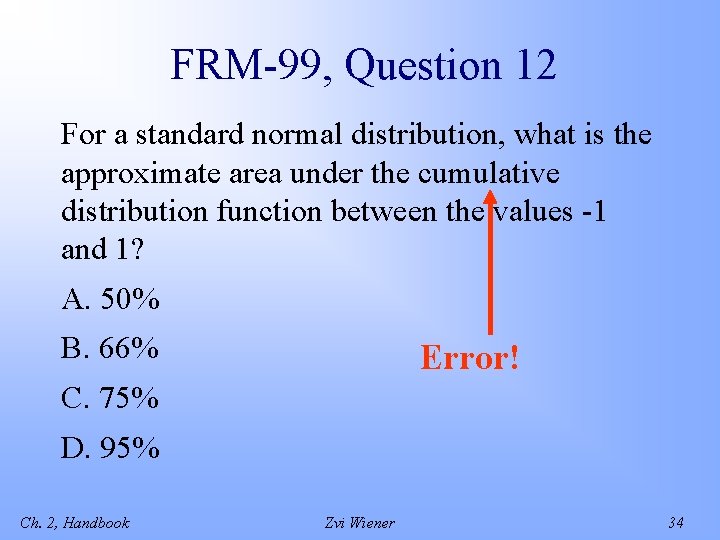
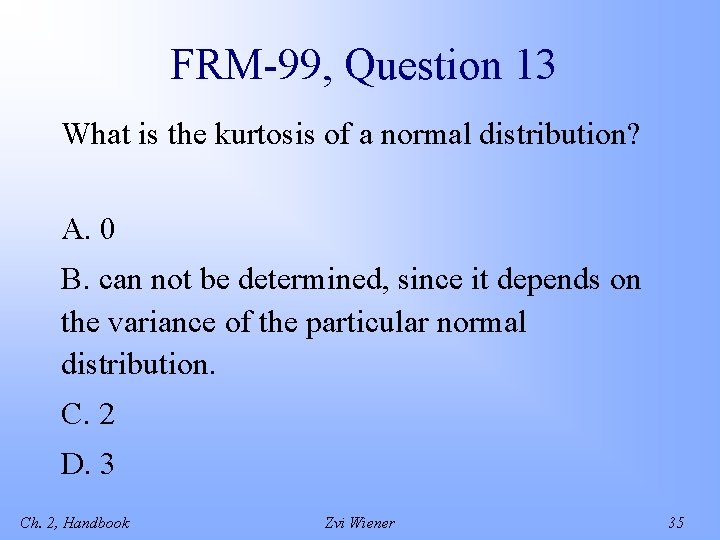
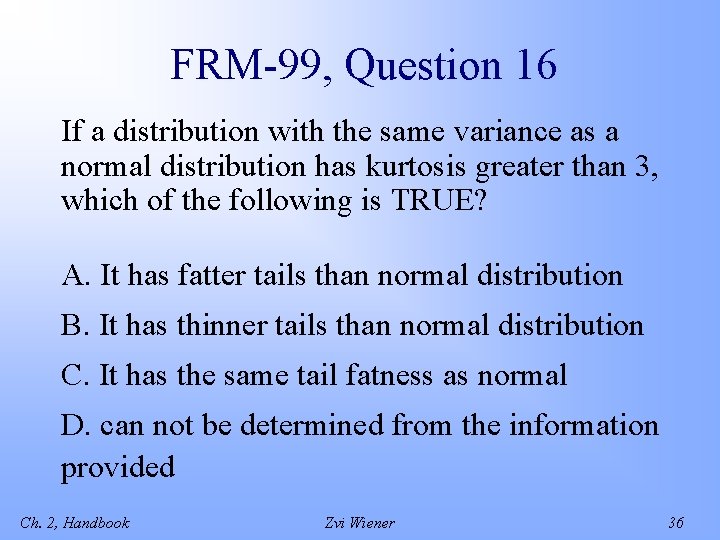
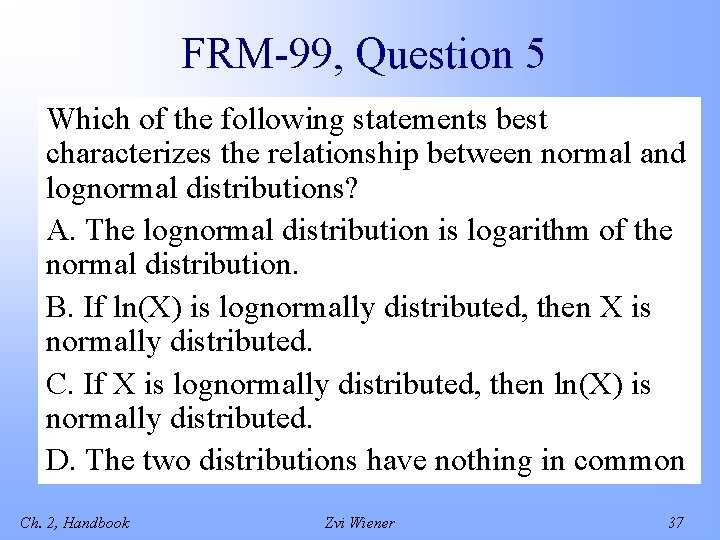
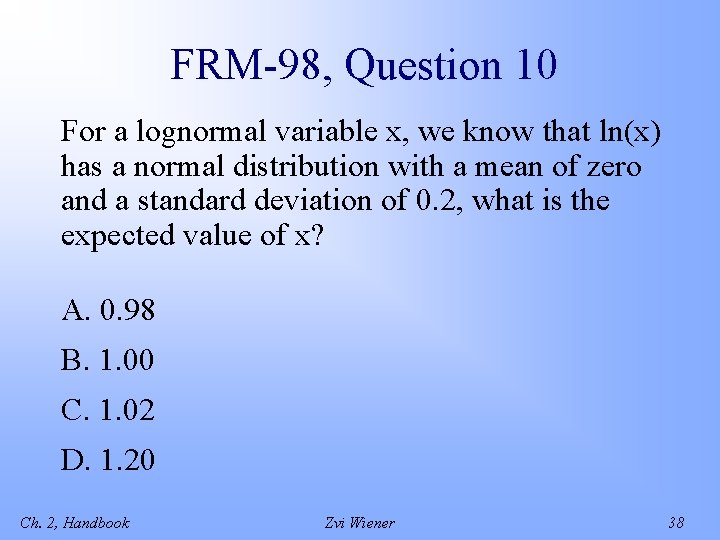
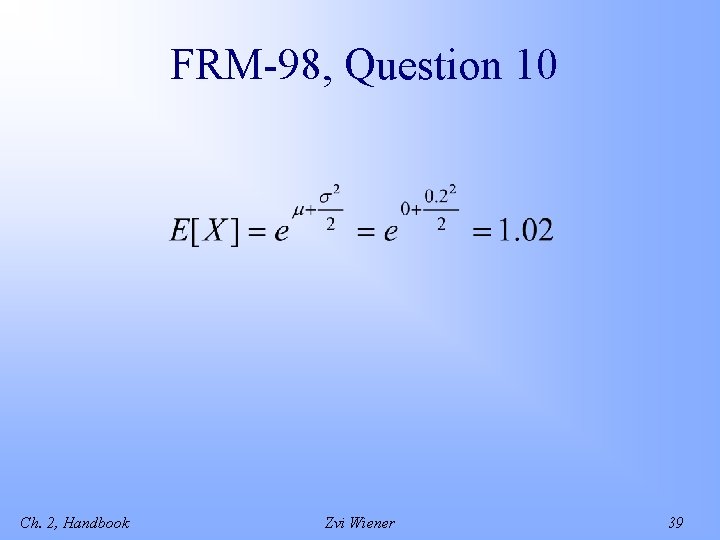
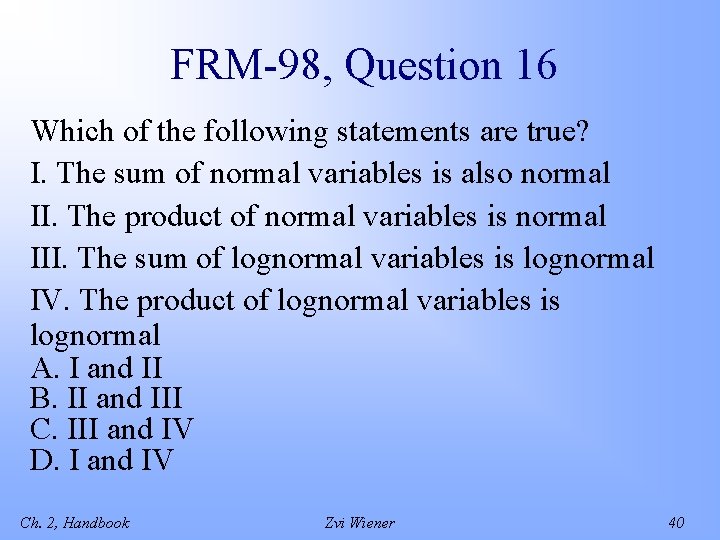
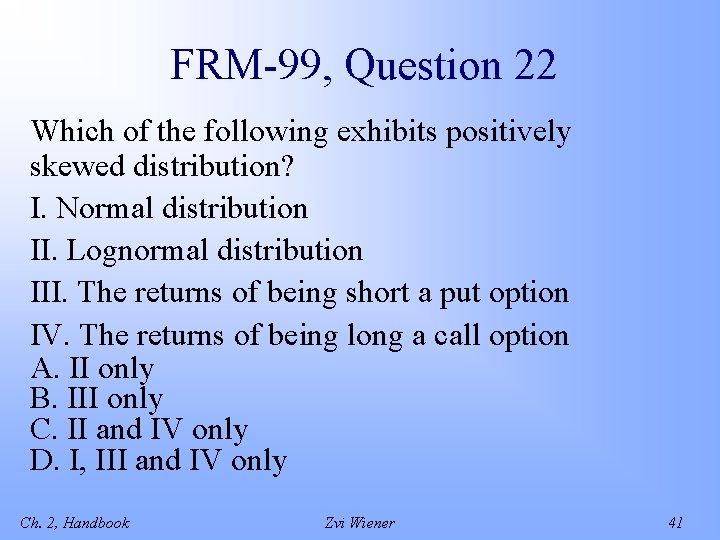
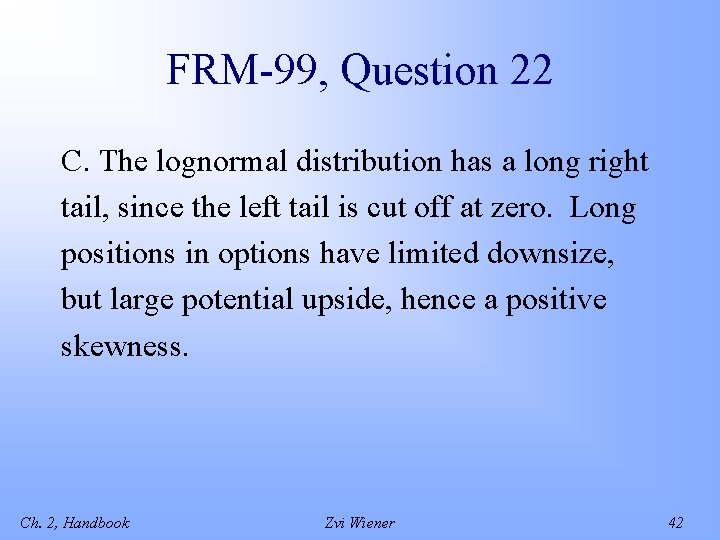
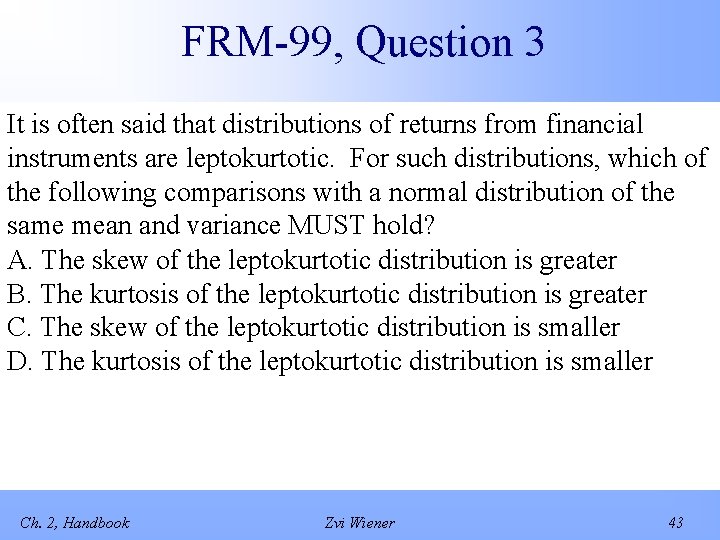
- Slides: 43
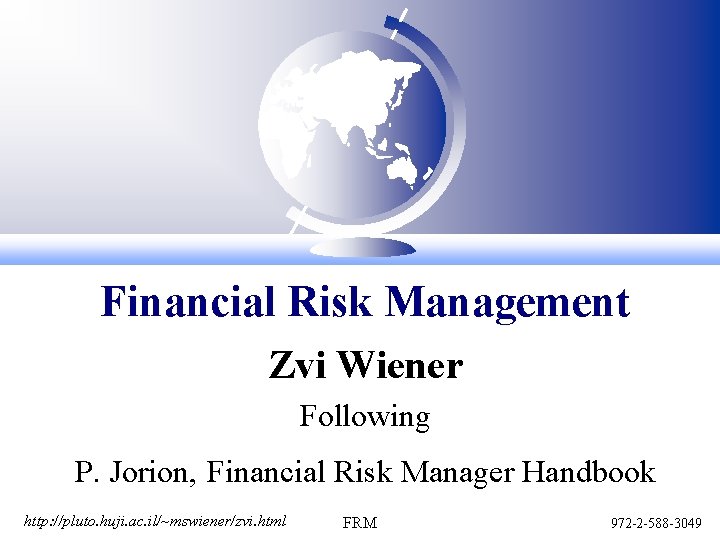
Financial Risk Management Zvi Wiener Following P. Jorion, Financial Risk Manager Handbook http: //pluto. huji. ac. il/~mswiener/zvi. html FRM 972 -2 -588 -3049
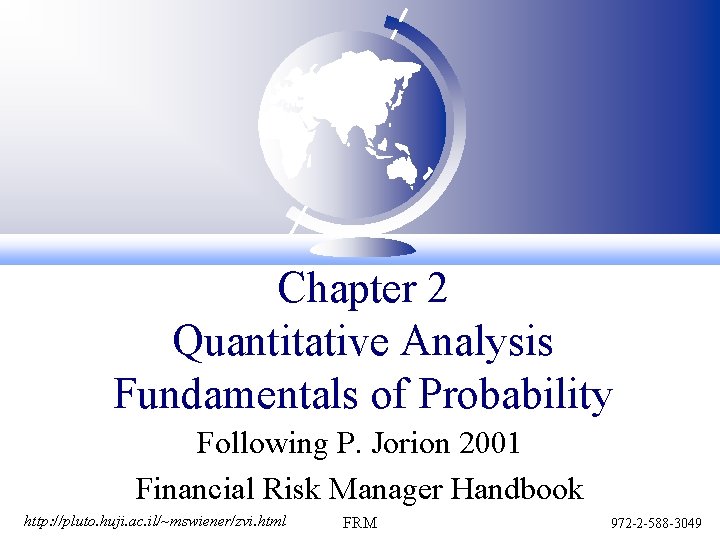
Chapter 2 Quantitative Analysis Fundamentals of Probability Following P. Jorion 2001 Financial Risk Manager Handbook http: //pluto. huji. ac. il/~mswiener/zvi. html FRM 972 -2 -588 -3049
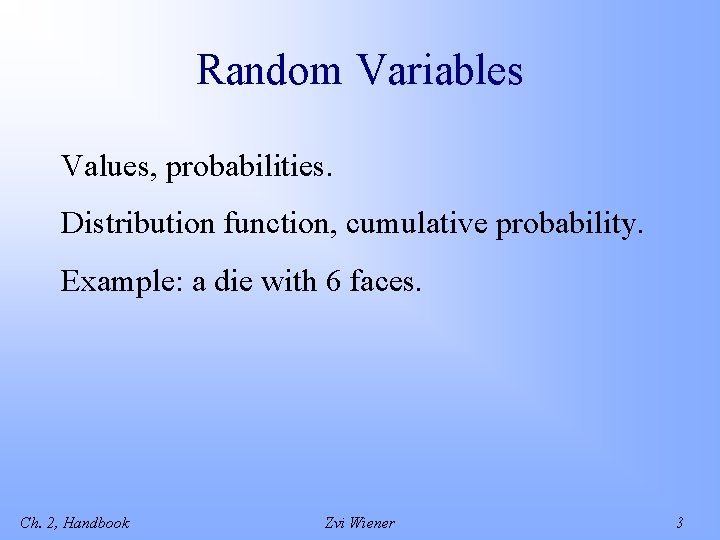
Random Variables Values, probabilities. Distribution function, cumulative probability. Example: a die with 6 faces. Ch. 2, Handbook Zvi Wiener 3
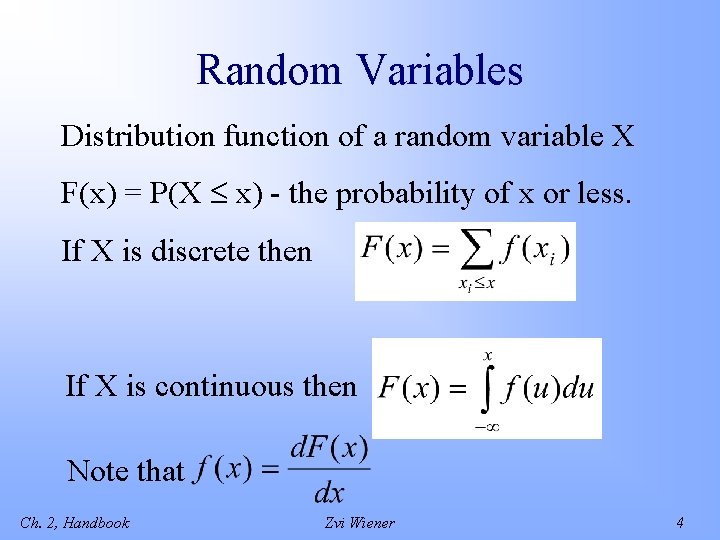
Random Variables Distribution function of a random variable X F(x) = P(X x) - the probability of x or less. If X is discrete then If X is continuous then Note that Ch. 2, Handbook Zvi Wiener 4
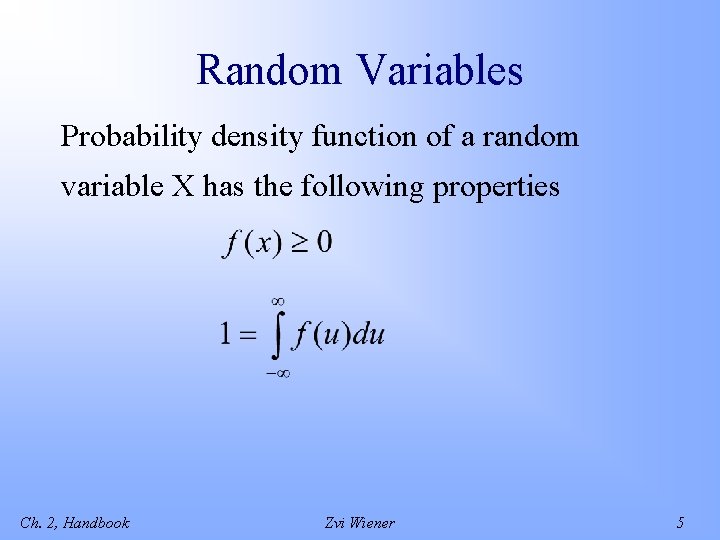
Random Variables Probability density function of a random variable X has the following properties Ch. 2, Handbook Zvi Wiener 5
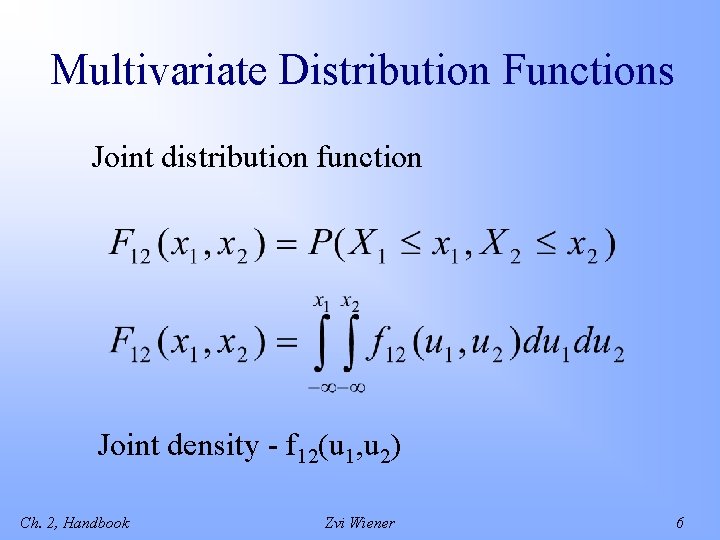
Multivariate Distribution Functions Joint distribution function Joint density - f 12(u 1, u 2) Ch. 2, Handbook Zvi Wiener 6
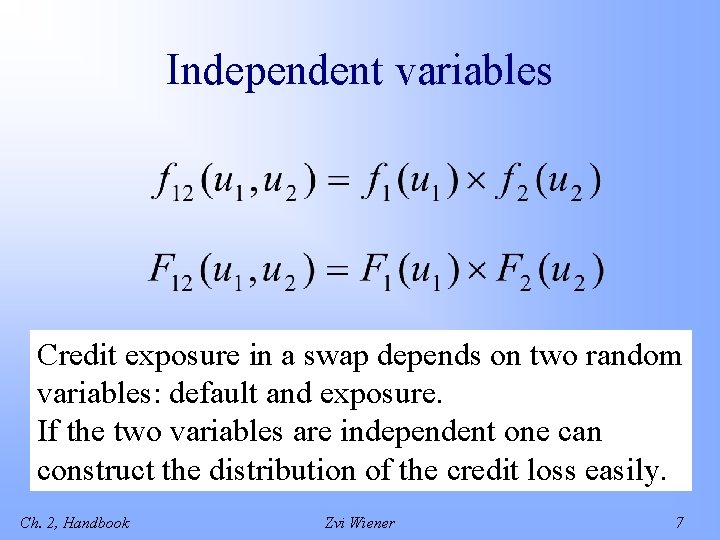
Independent variables Credit exposure in a swap depends on two random variables: default and exposure. If the two variables are independent one can construct the distribution of the credit loss easily. Ch. 2, Handbook Zvi Wiener 7
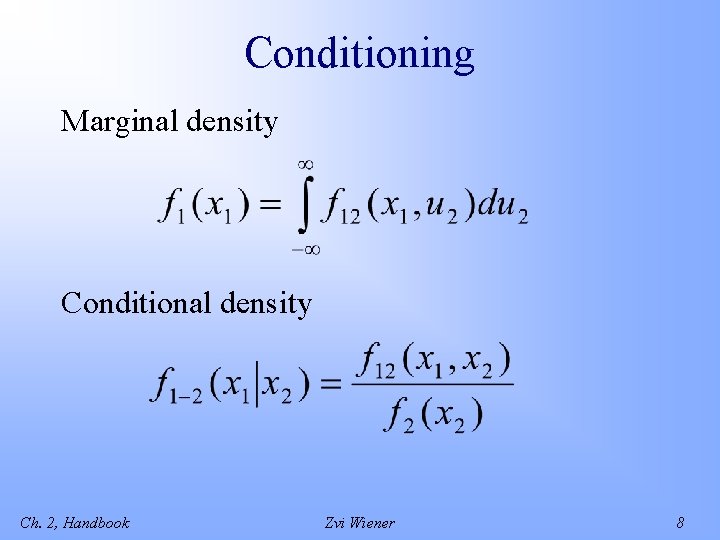
Conditioning Marginal density Conditional density Ch. 2, Handbook Zvi Wiener 8
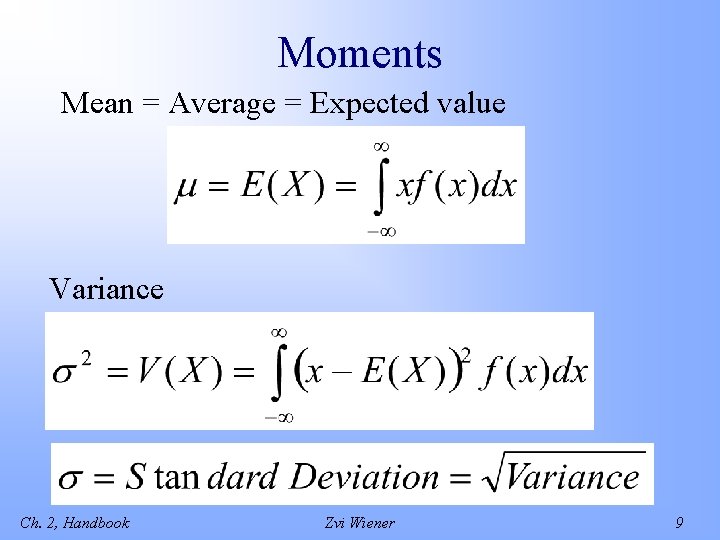
Moments Mean = Average = Expected value Variance Ch. 2, Handbook Zvi Wiener 9
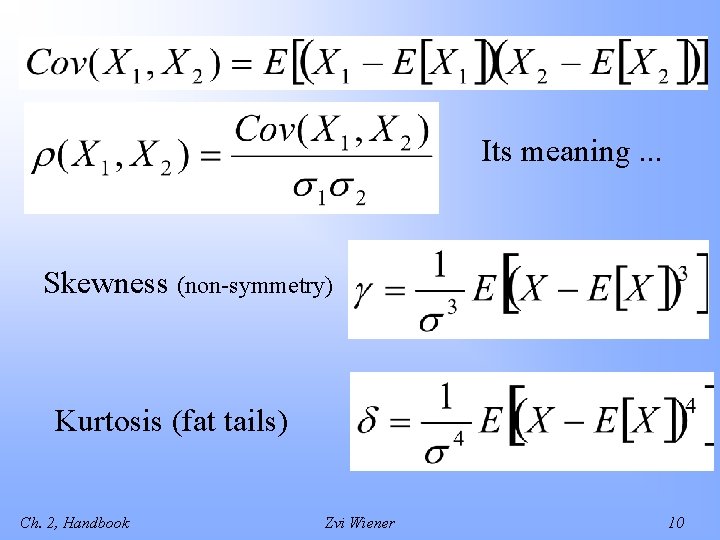
Its meaning. . . Skewness (non-symmetry) Kurtosis (fat tails) Ch. 2, Handbook Zvi Wiener 10
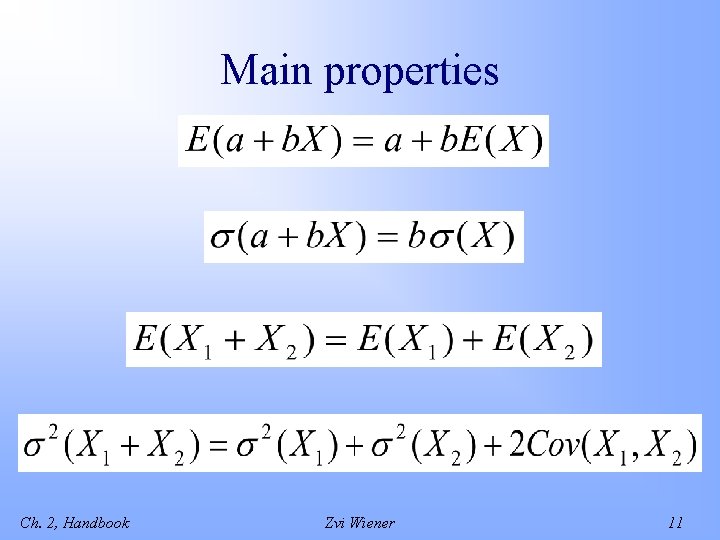
Main properties Ch. 2, Handbook Zvi Wiener 11
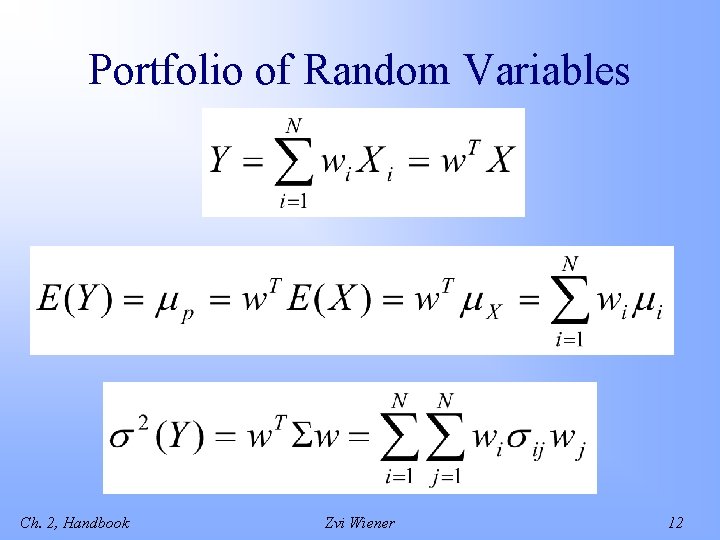
Portfolio of Random Variables Ch. 2, Handbook Zvi Wiener 12
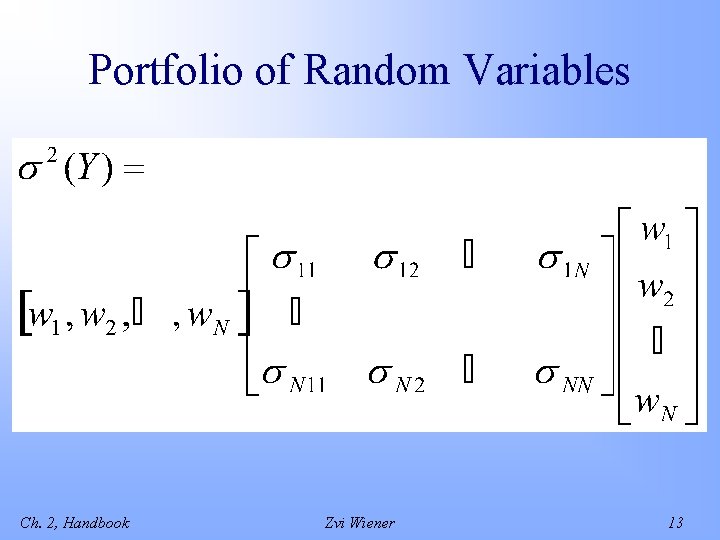
Portfolio of Random Variables Ch. 2, Handbook Zvi Wiener 13
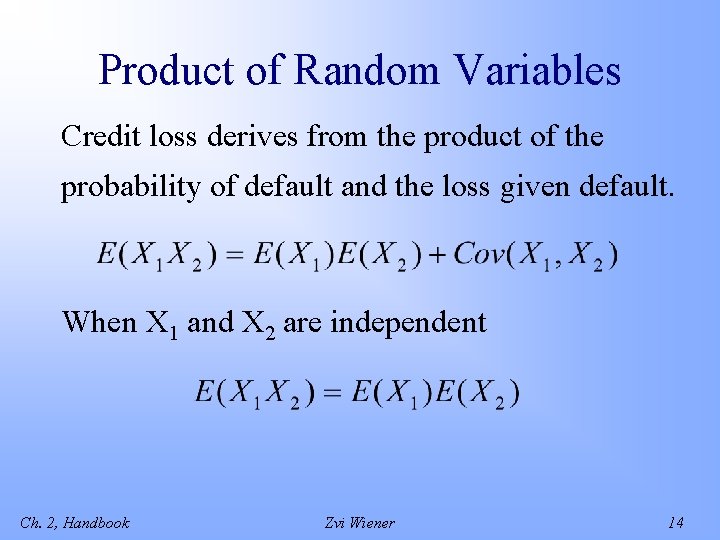
Product of Random Variables Credit loss derives from the product of the probability of default and the loss given default. When X 1 and X 2 are independent Ch. 2, Handbook Zvi Wiener 14
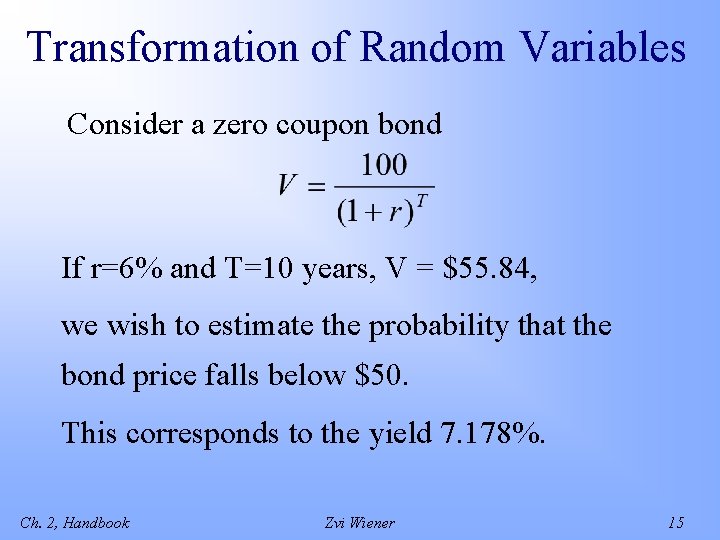
Transformation of Random Variables Consider a zero coupon bond If r=6% and T=10 years, V = $55. 84, we wish to estimate the probability that the bond price falls below $50. This corresponds to the yield 7. 178%. Ch. 2, Handbook Zvi Wiener 15
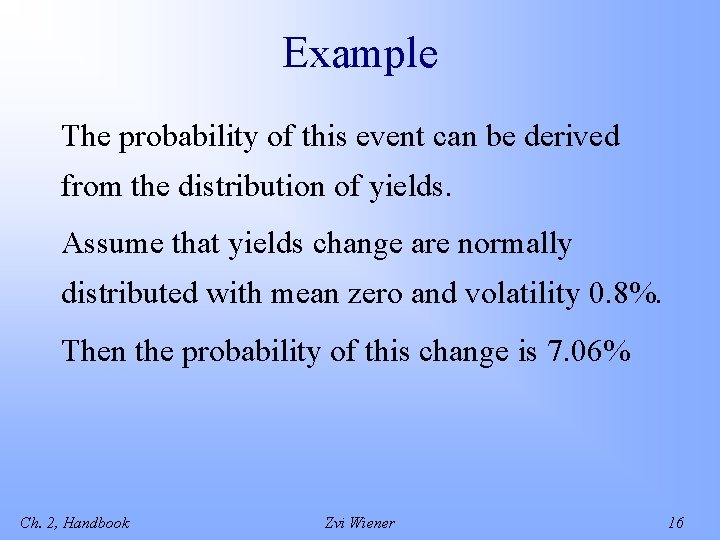
Example The probability of this event can be derived from the distribution of yields. Assume that yields change are normally distributed with mean zero and volatility 0. 8%. Then the probability of this change is 7. 06% Ch. 2, Handbook Zvi Wiener 16
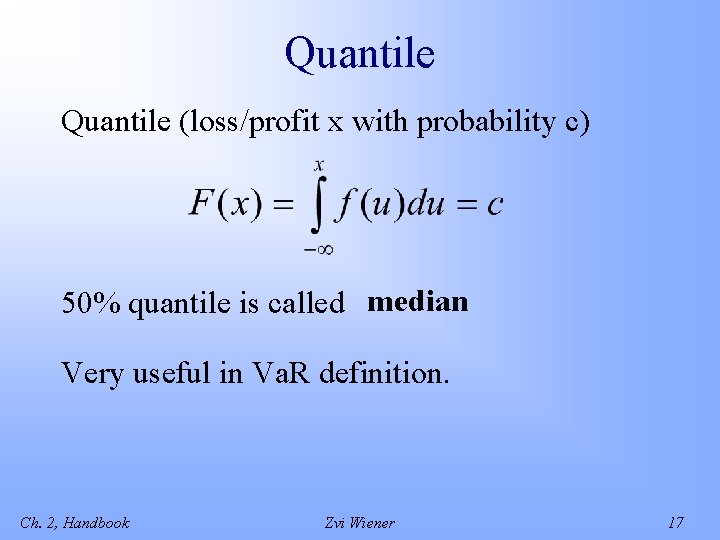
Quantile (loss/profit x with probability c) 50% quantile is called median Very useful in Va. R definition. Ch. 2, Handbook Zvi Wiener 17
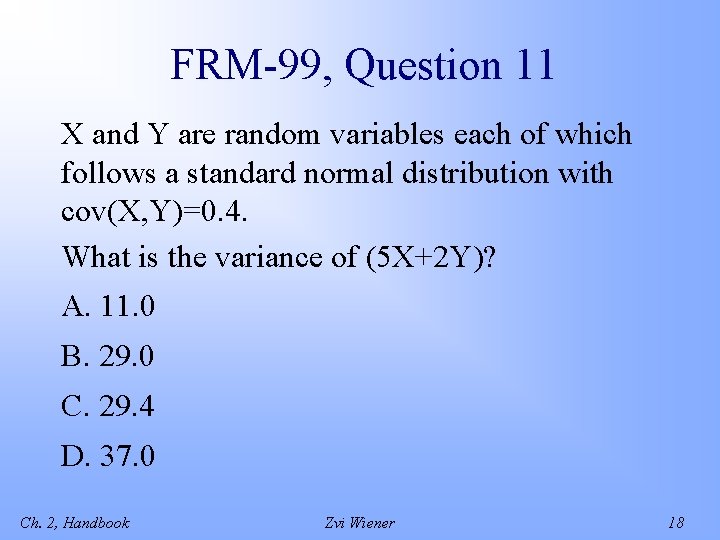
FRM-99, Question 11 X and Y are random variables each of which follows a standard normal distribution with cov(X, Y)=0. 4. What is the variance of (5 X+2 Y)? A. 11. 0 B. 29. 0 C. 29. 4 D. 37. 0 Ch. 2, Handbook Zvi Wiener 18
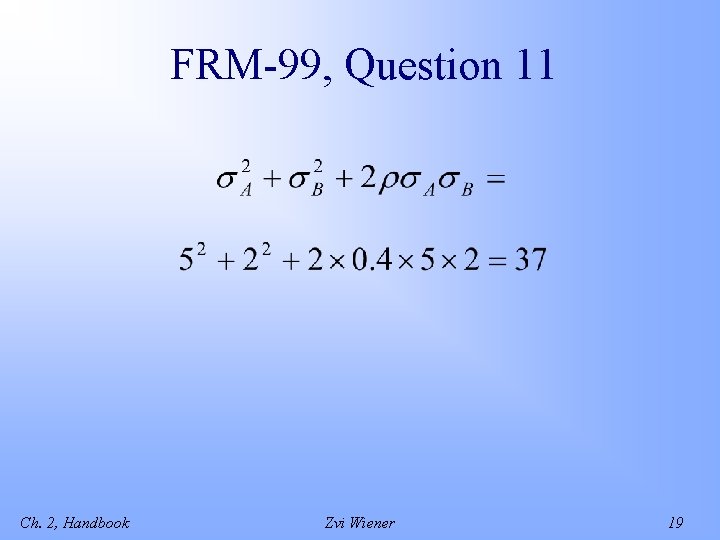
FRM-99, Question 11 Ch. 2, Handbook Zvi Wiener 19
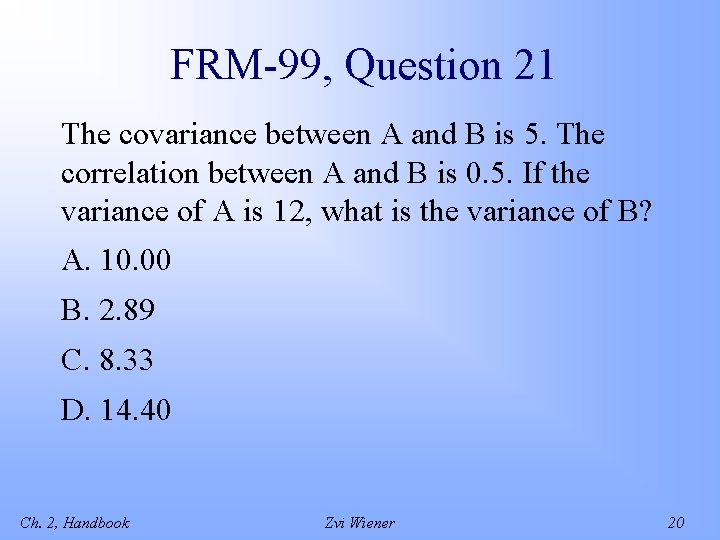
FRM-99, Question 21 The covariance between A and B is 5. The correlation between A and B is 0. 5. If the variance of A is 12, what is the variance of B? A. 10. 00 B. 2. 89 C. 8. 33 D. 14. 40 Ch. 2, Handbook Zvi Wiener 20
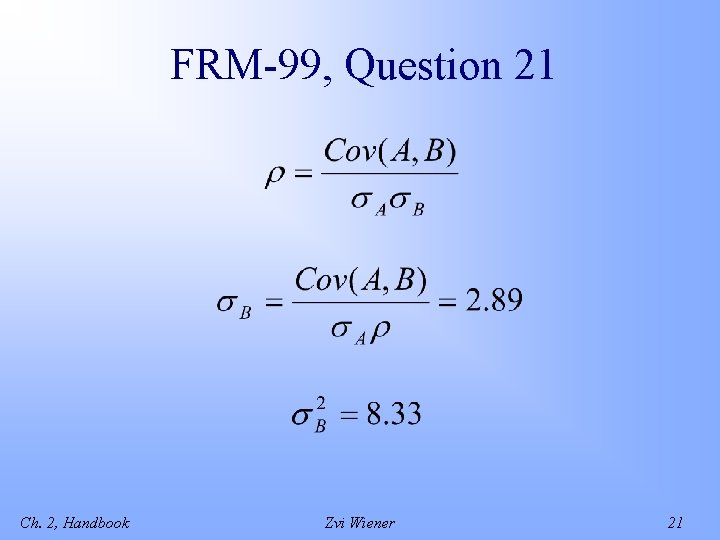
FRM-99, Question 21 Ch. 2, Handbook Zvi Wiener 21
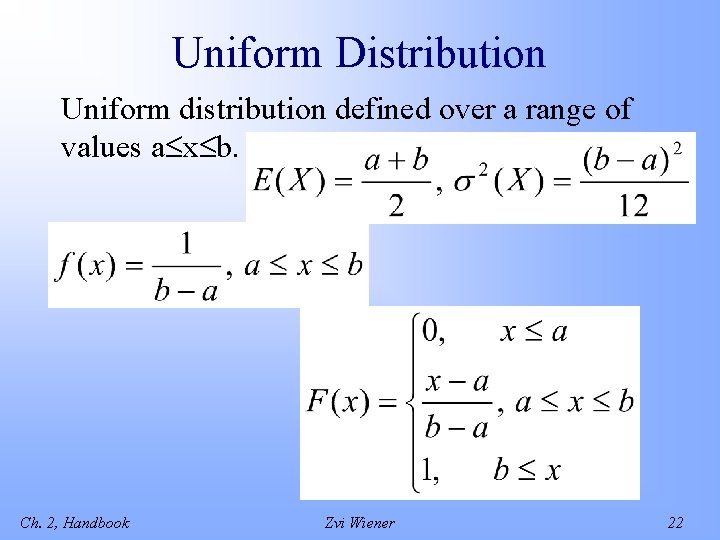
Uniform Distribution Uniform distribution defined over a range of values a x b. Ch. 2, Handbook Zvi Wiener 22
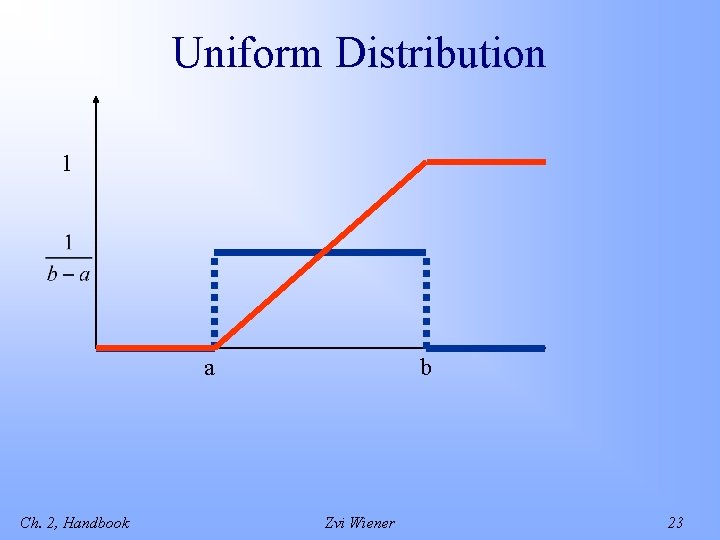
Uniform Distribution 1 a Ch. 2, Handbook b Zvi Wiener 23
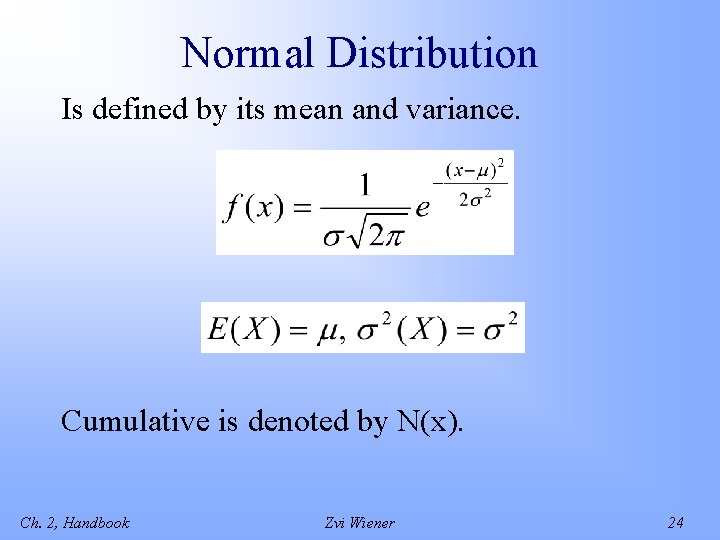
Normal Distribution Is defined by its mean and variance. Cumulative is denoted by N(x). Ch. 2, Handbook Zvi Wiener 24
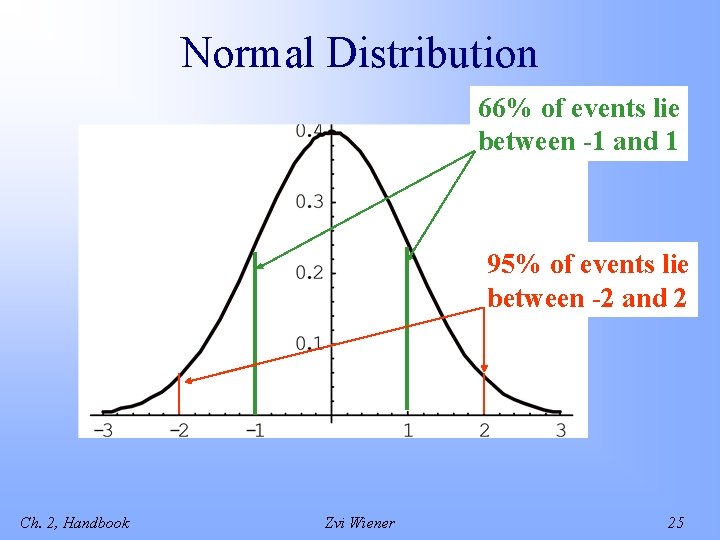
Normal Distribution 66% of events lie between -1 and 1 95% of events lie between -2 and 2 Ch. 2, Handbook Zvi Wiener 25
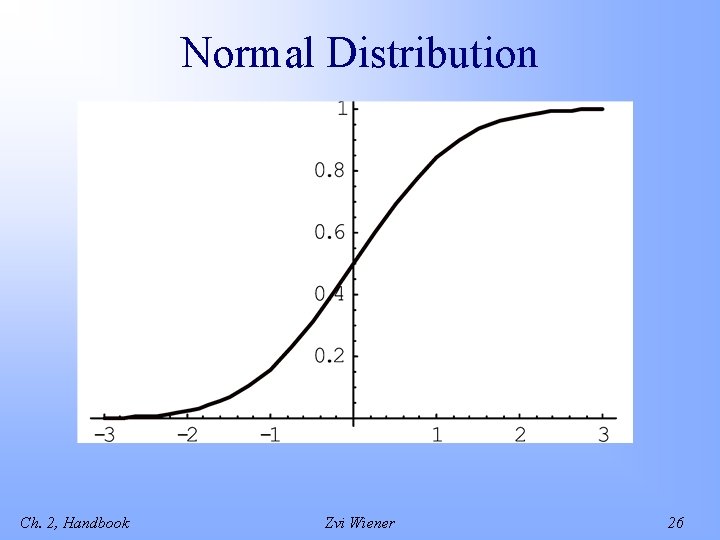
Normal Distribution Ch. 2, Handbook Zvi Wiener 26
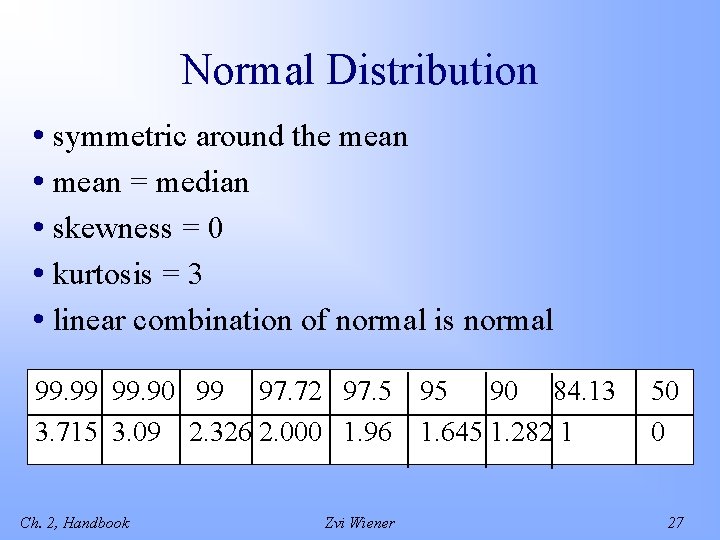
Normal Distribution • symmetric around the mean • mean = median • skewness = 0 • kurtosis = 3 • linear combination of normal is normal 99. 99 99. 90 99 97. 72 97. 5 3. 715 3. 09 2. 326 2. 000 1. 96 Ch. 2, Handbook Zvi Wiener 95 90 84. 13 1. 645 1. 282 1 50 0 27
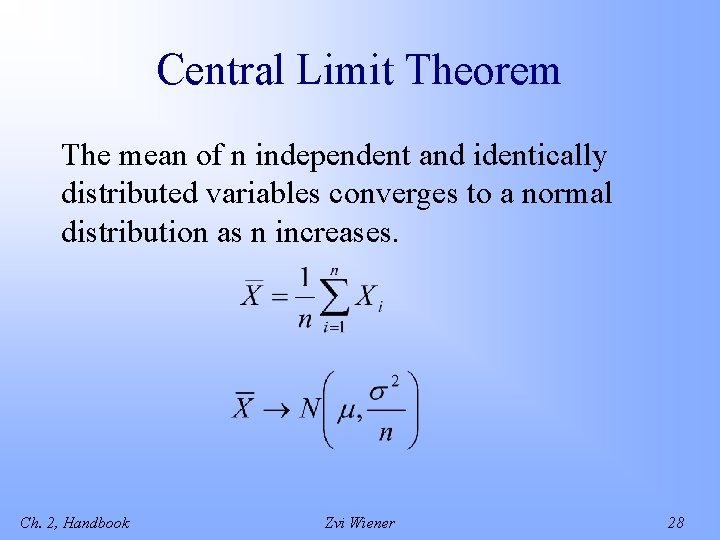
Central Limit Theorem The mean of n independent and identically distributed variables converges to a normal distribution as n increases. Ch. 2, Handbook Zvi Wiener 28
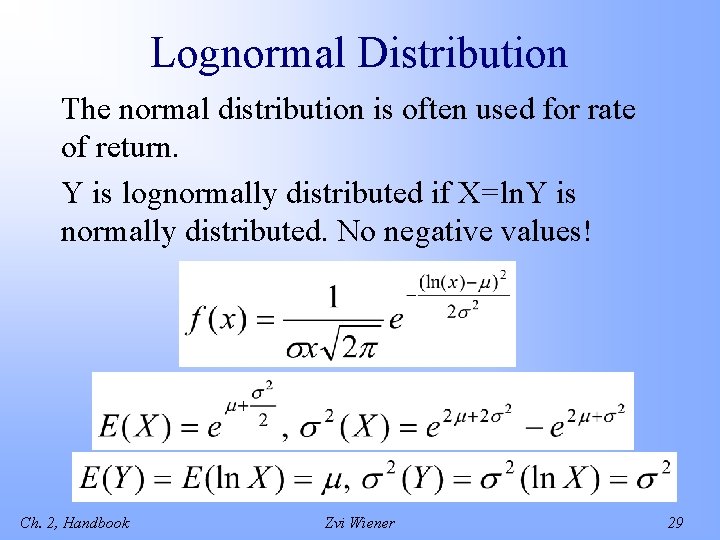
Lognormal Distribution The normal distribution is often used for rate of return. Y is lognormally distributed if X=ln. Y is normally distributed. No negative values! Ch. 2, Handbook Zvi Wiener 29
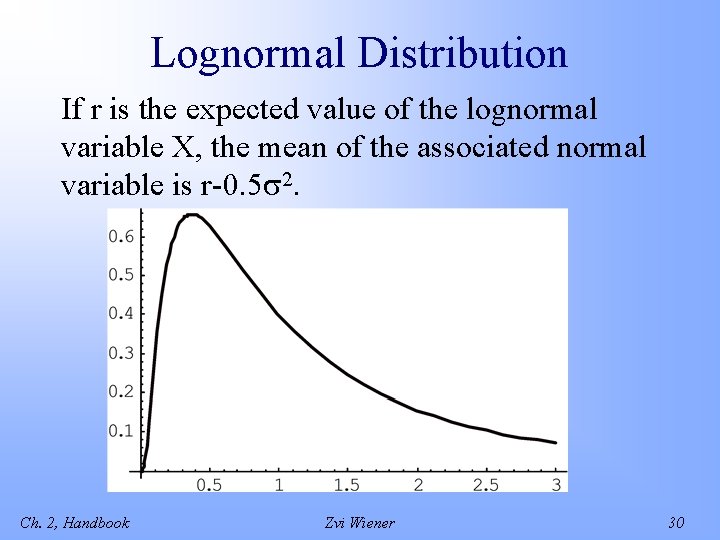
Lognormal Distribution If r is the expected value of the lognormal variable X, the mean of the associated normal variable is r-0. 5 2. Ch. 2, Handbook Zvi Wiener 30
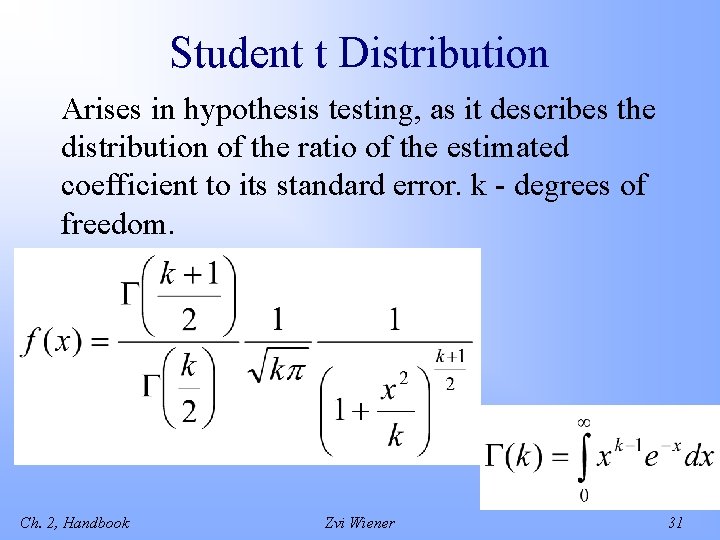
Student t Distribution Arises in hypothesis testing, as it describes the distribution of the ratio of the estimated coefficient to its standard error. k - degrees of freedom. Ch. 2, Handbook Zvi Wiener 31
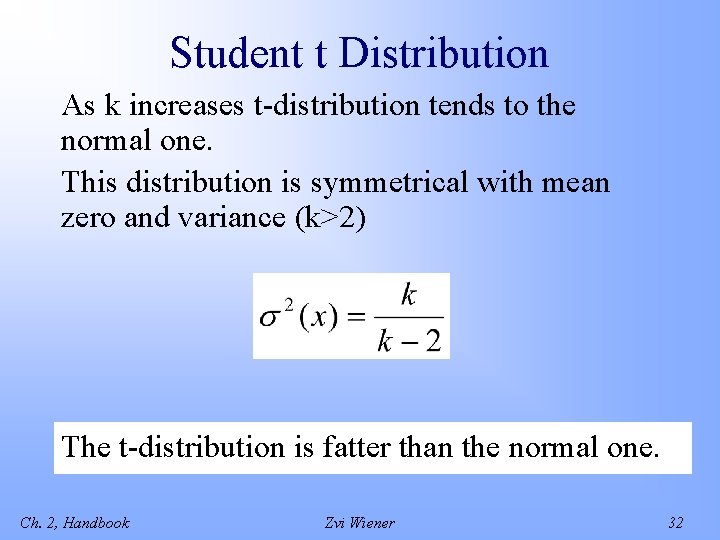
Student t Distribution As k increases t-distribution tends to the normal one. This distribution is symmetrical with mean zero and variance (k>2) The t-distribution is fatter than the normal one. Ch. 2, Handbook Zvi Wiener 32
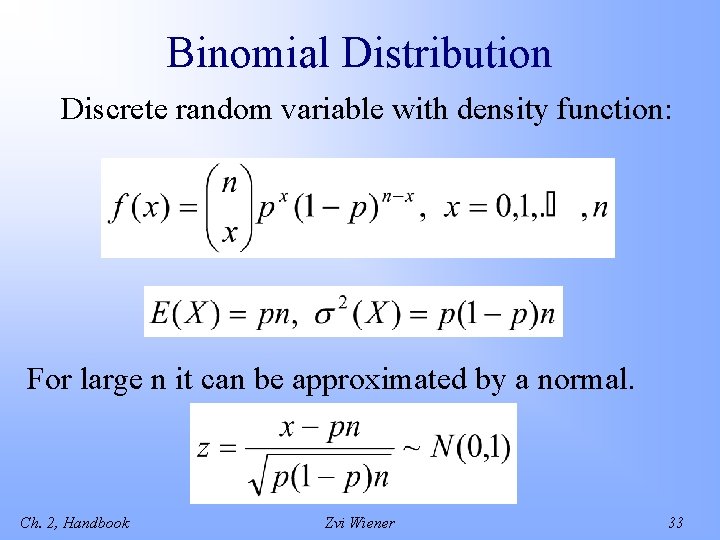
Binomial Distribution Discrete random variable with density function: For large n it can be approximated by a normal. Ch. 2, Handbook Zvi Wiener 33
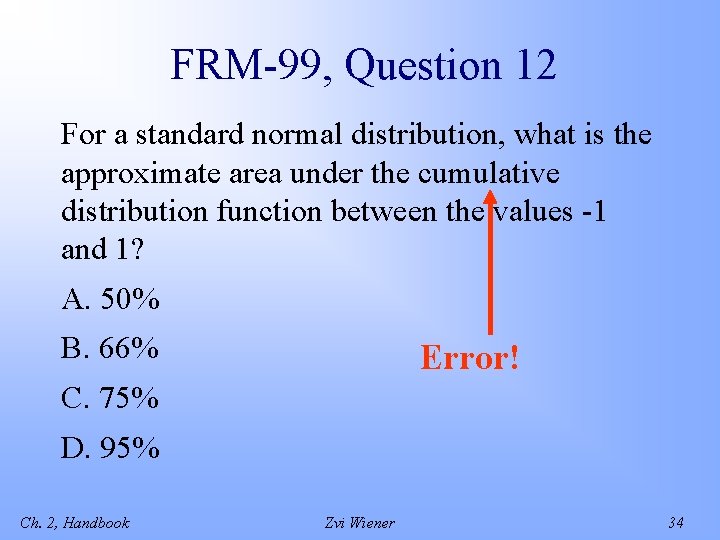
FRM-99, Question 12 For a standard normal distribution, what is the approximate area under the cumulative distribution function between the values -1 and 1? A. 50% B. 66% Error! C. 75% D. 95% Ch. 2, Handbook Zvi Wiener 34
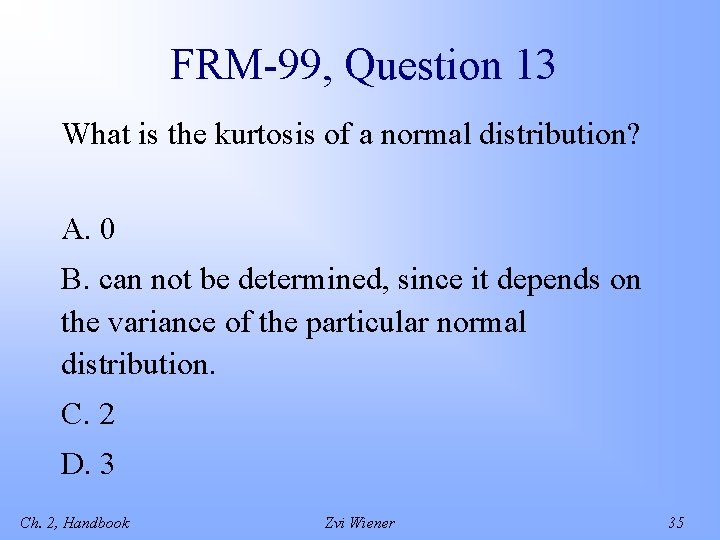
FRM-99, Question 13 What is the kurtosis of a normal distribution? A. 0 B. can not be determined, since it depends on the variance of the particular normal distribution. C. 2 D. 3 Ch. 2, Handbook Zvi Wiener 35
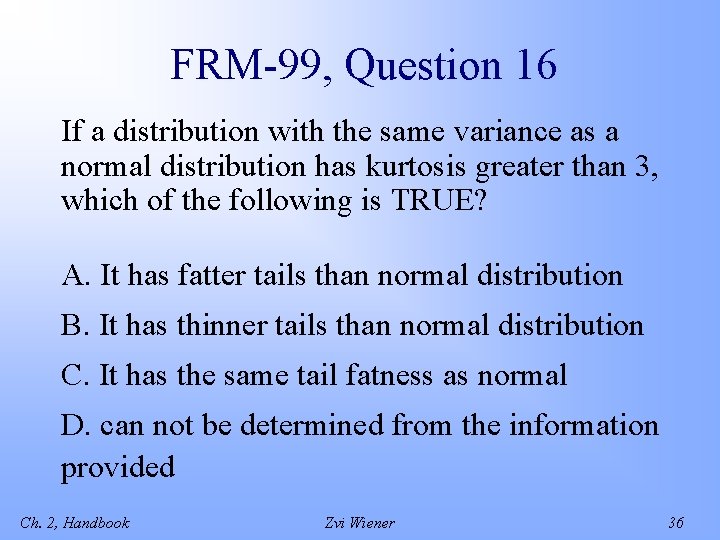
FRM-99, Question 16 If a distribution with the same variance as a normal distribution has kurtosis greater than 3, which of the following is TRUE? A. It has fatter tails than normal distribution B. It has thinner tails than normal distribution C. It has the same tail fatness as normal D. can not be determined from the information provided Ch. 2, Handbook Zvi Wiener 36
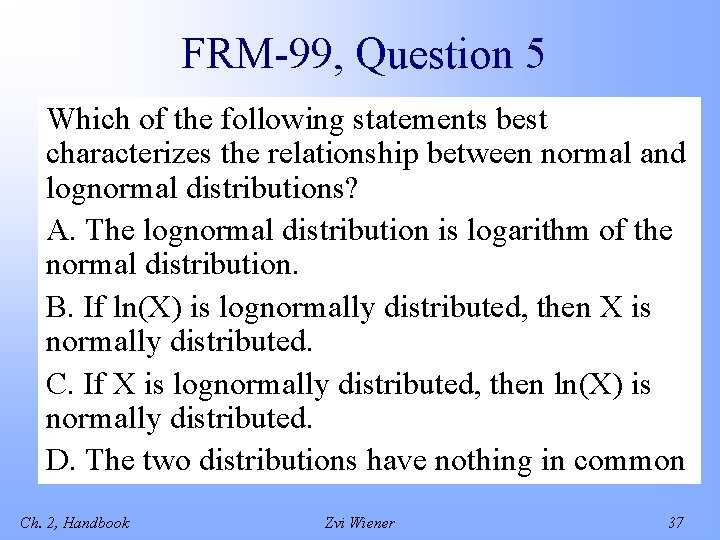
FRM-99, Question 5 Which of the following statements best characterizes the relationship between normal and lognormal distributions? A. The lognormal distribution is logarithm of the normal distribution. B. If ln(X) is lognormally distributed, then X is normally distributed. C. If X is lognormally distributed, then ln(X) is normally distributed. D. The two distributions have nothing in common Ch. 2, Handbook Zvi Wiener 37
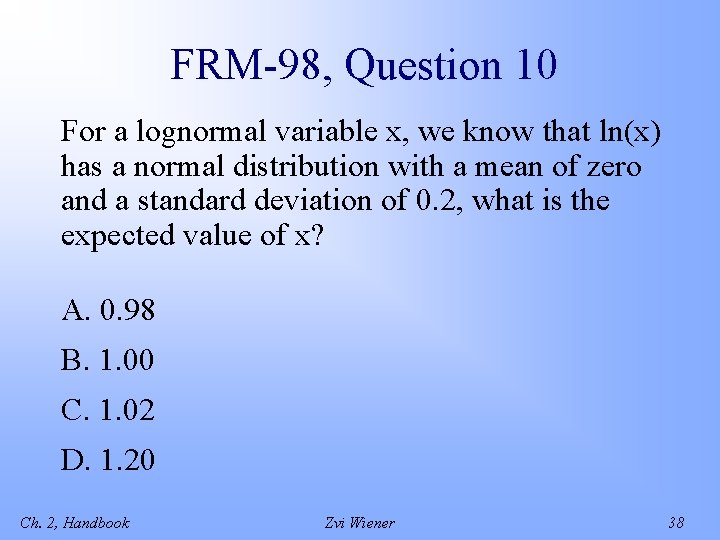
FRM-98, Question 10 For a lognormal variable x, we know that ln(x) has a normal distribution with a mean of zero and a standard deviation of 0. 2, what is the expected value of x? A. 0. 98 B. 1. 00 C. 1. 02 D. 1. 20 Ch. 2, Handbook Zvi Wiener 38
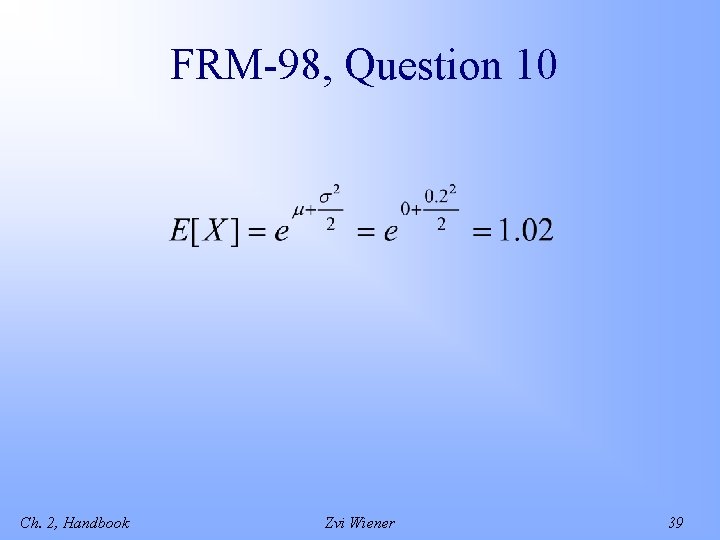
FRM-98, Question 10 Ch. 2, Handbook Zvi Wiener 39
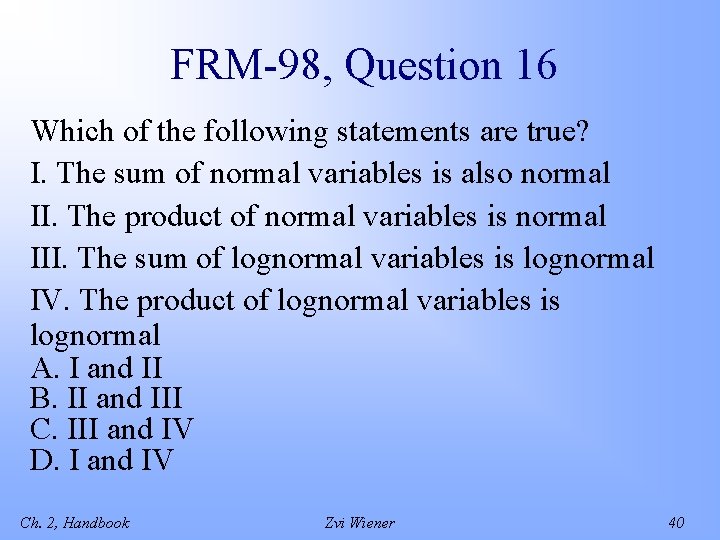
FRM-98, Question 16 Which of the following statements are true? I. The sum of normal variables is also normal II. The product of normal variables is normal III. The sum of lognormal variables is lognormal IV. The product of lognormal variables is lognormal A. I and II B. II and III C. III and IV D. I and IV Ch. 2, Handbook Zvi Wiener 40
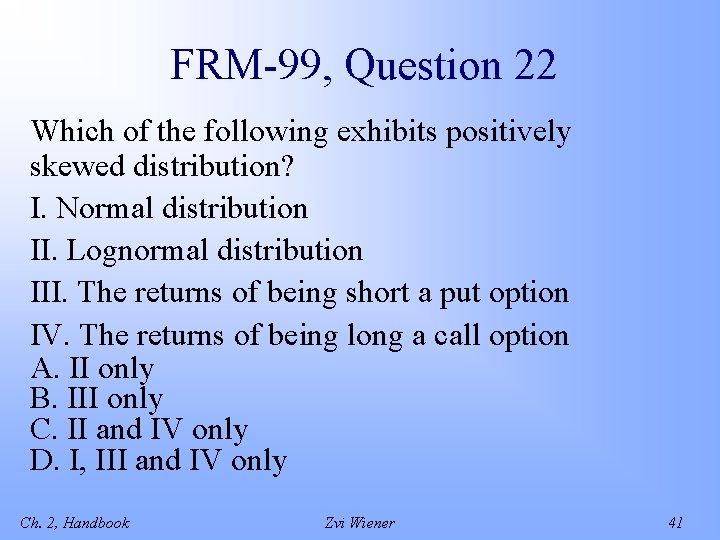
FRM-99, Question 22 Which of the following exhibits positively skewed distribution? I. Normal distribution II. Lognormal distribution III. The returns of being short a put option IV. The returns of being long a call option A. II only B. III only C. II and IV only D. I, III and IV only Ch. 2, Handbook Zvi Wiener 41
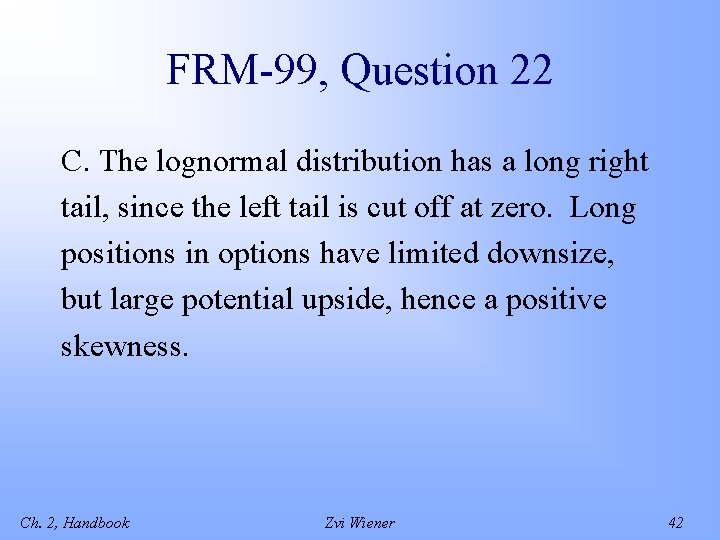
FRM-99, Question 22 C. The lognormal distribution has a long right tail, since the left tail is cut off at zero. Long positions in options have limited downsize, but large potential upside, hence a positive skewness. Ch. 2, Handbook Zvi Wiener 42
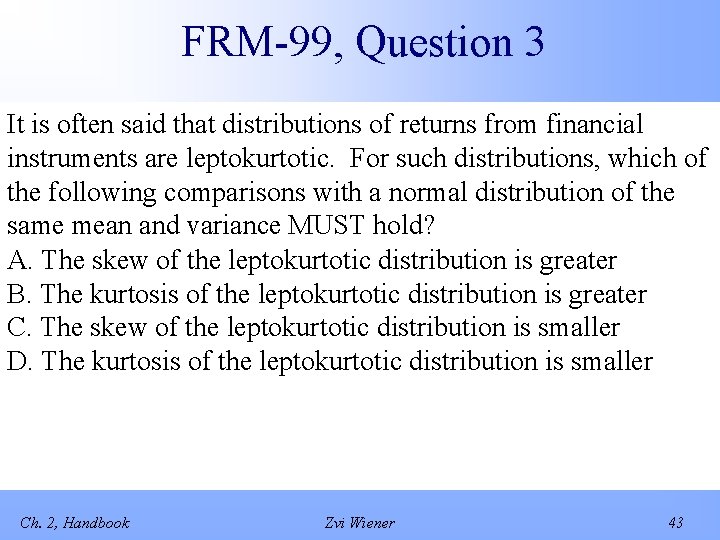
FRM-99, Question 3 It is often said that distributions of returns from financial instruments are leptokurtotic. For such distributions, which of the following comparisons with a normal distribution of the same mean and variance MUST hold? A. The skew of the leptokurtotic distribution is greater B. The kurtosis of the leptokurtotic distribution is greater C. The skew of the leptokurtotic distribution is smaller D. The kurtosis of the leptokurtotic distribution is smaller Ch. 2, Handbook Zvi Wiener 43
Zvi wiener
02 588
Zvi wiener
Key risk indicators financial risk management
Market risk credit risk operational risk
Business vs financial risk
Corporate strategy examples
Leadership theories
Ms 640
Zvi margaliot
Zvi aronson
Zvi kedem
Dr zvi margaliot
Zvi roth
Risk map
Introduction to financial risk
Financial management chapter 8 risk and return
Elements of financial risk management peter christoffersen
Financial engineering derivatives and risk management
Financial risk management syllabus
Enterprise risk management for financial institutions
Financial risk management conference 2018
Financial management framework
Financial engineering derivatives and risk management
Financial engineering derivatives and risk management
Wiener schlosserbuben
Cornelia brezik
Indeks kesamaan komunitas
Dr craig wiener
Ito lemma example
Wiener liste antibiotika
Modèle de lasswell
Wiener tafelspitz beilagen
Thomas kutschera
Wiener rh-hr terminology
Wiener klassik geschichtlicher hintergrund
Diese landeshauptstadt liegt im vorland im südosten
Fundación wiener cursos a distancia 2020
Wiener
Wiener
Wiener salon gulasch
Thomas wiener
Normtabelle
Wiener anerkennungssystem