Fairness Privacy and Social Norms Omer Reingold MSRSVC
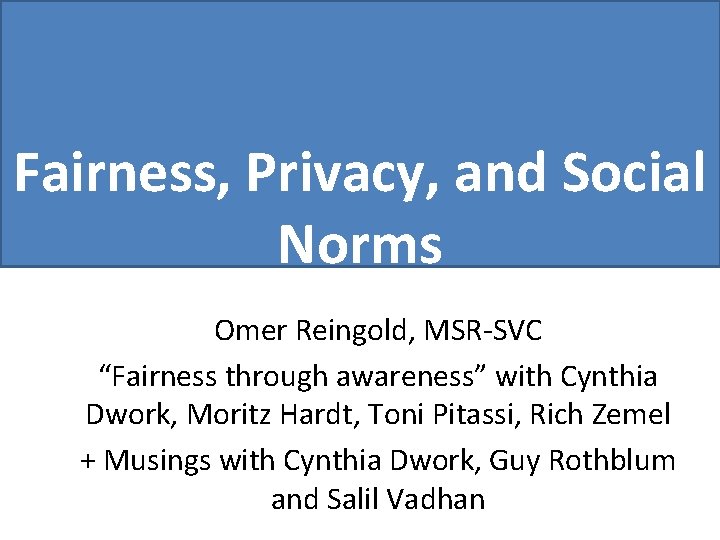
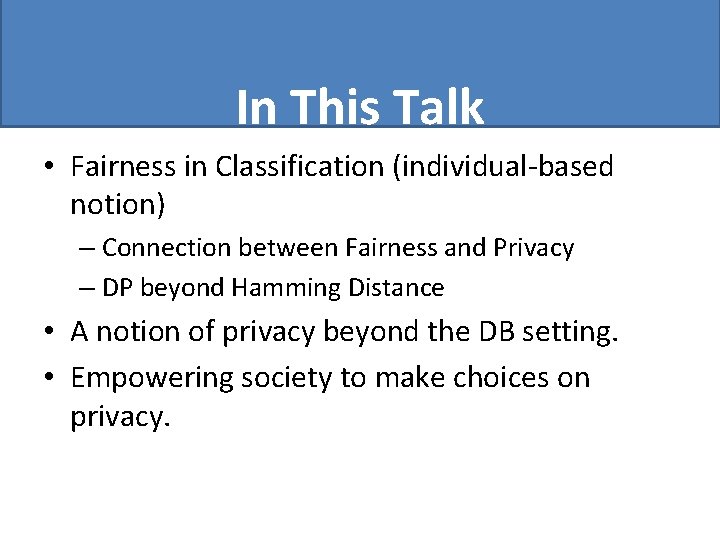
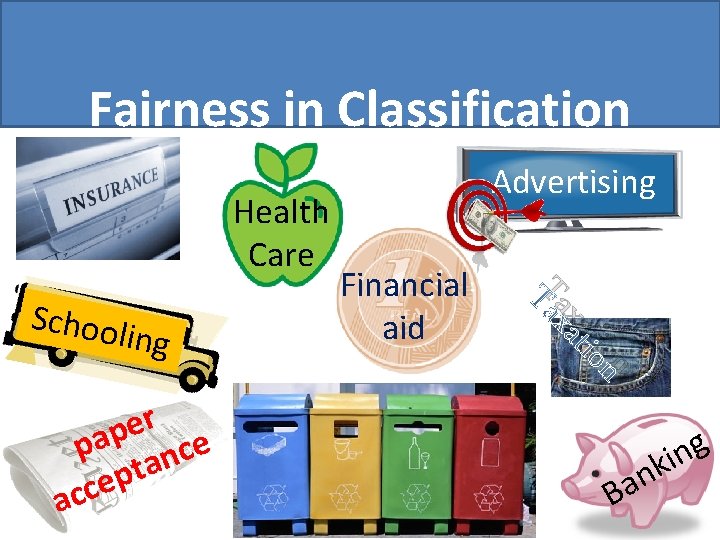
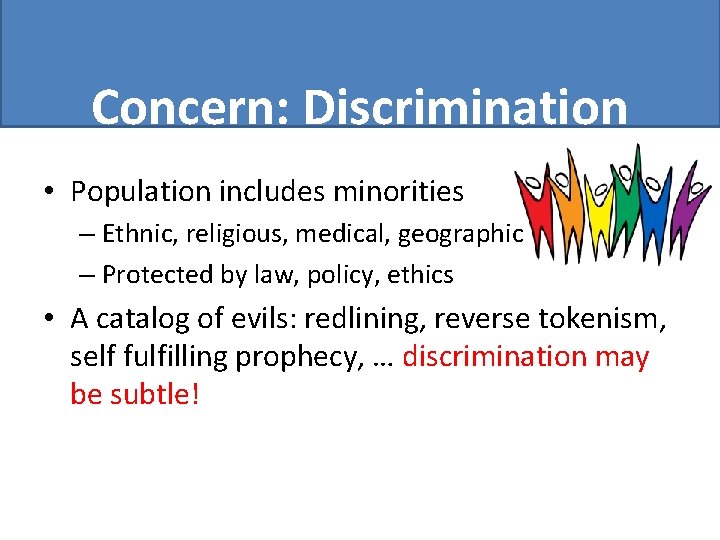
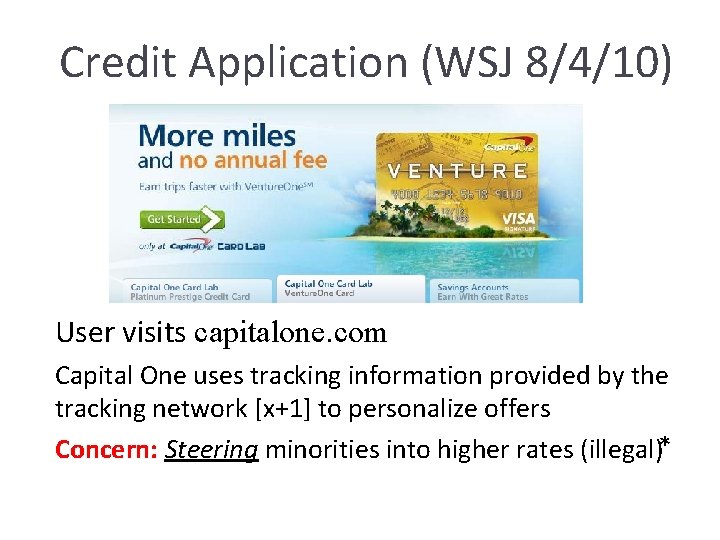
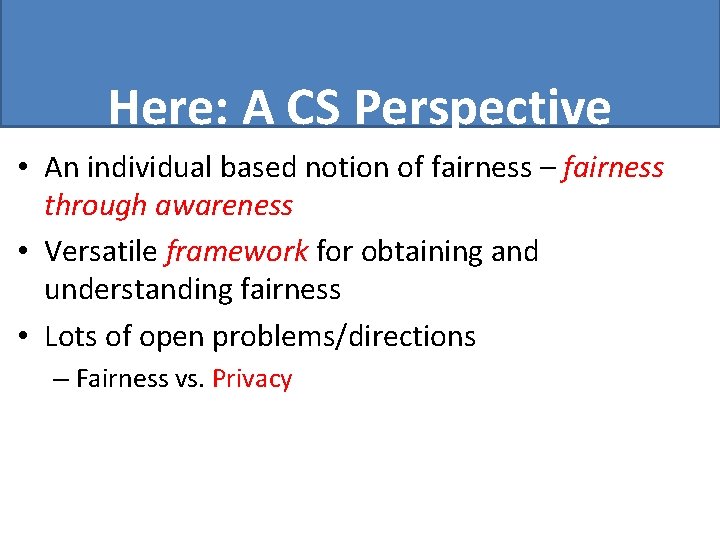
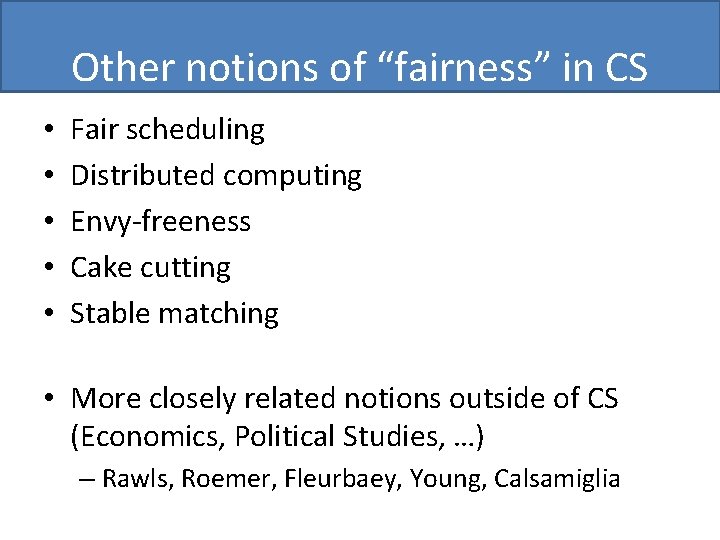
![Fairness and Privacy (1) • [Dwork & Mulligan 2012] objections to online behavioral targeting Fairness and Privacy (1) • [Dwork & Mulligan 2012] objections to online behavioral targeting](https://slidetodoc.com/presentation_image_h/fb3938be4f2b241455458125d3b53db7/image-8.jpg)
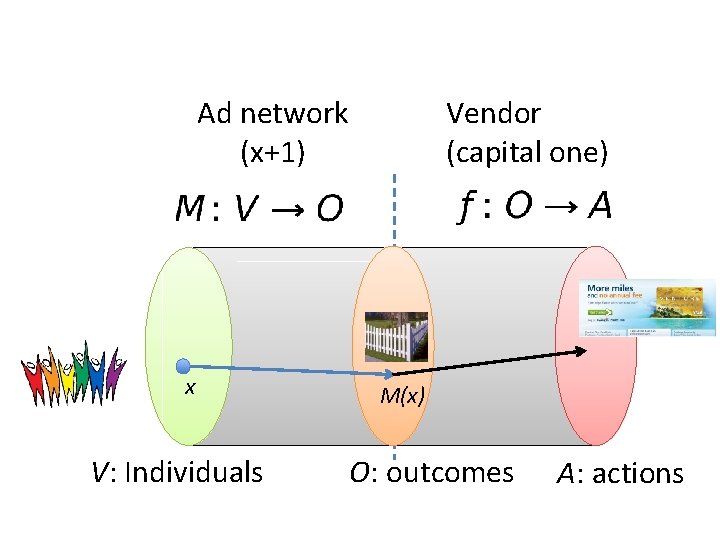
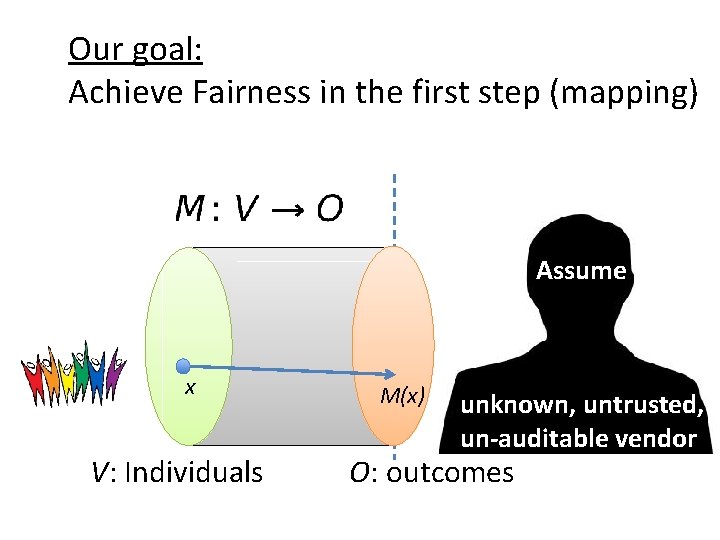
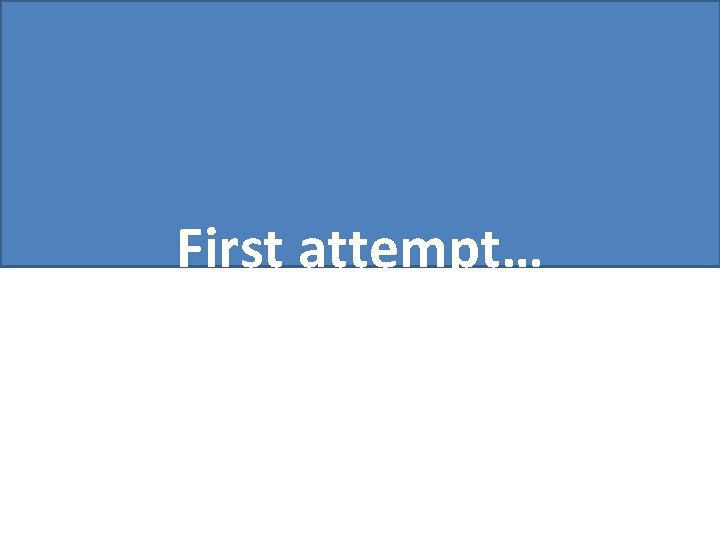
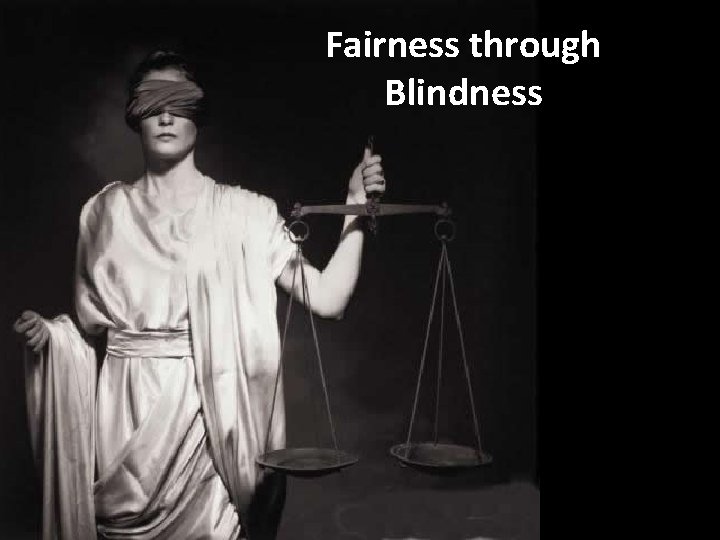
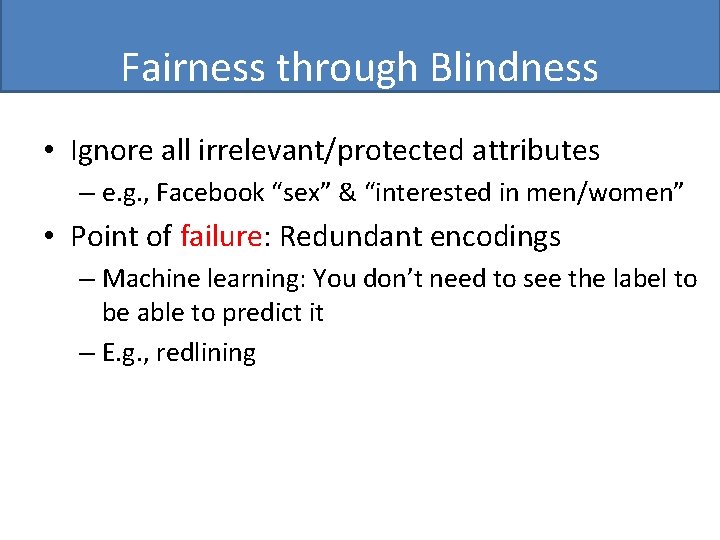
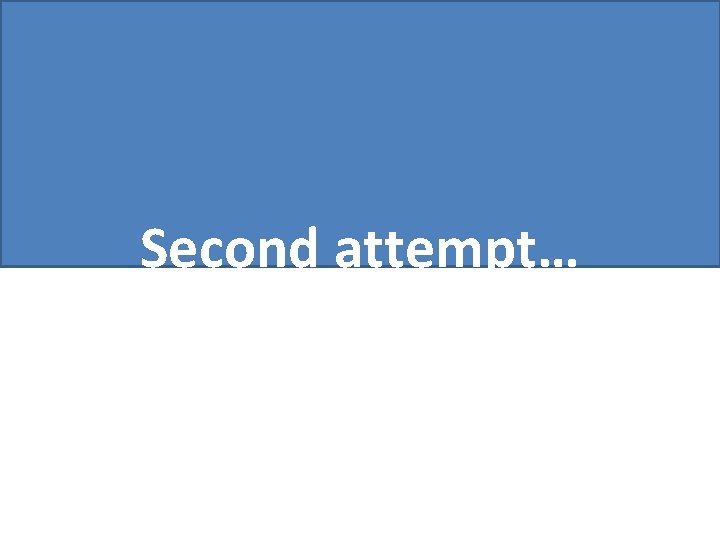
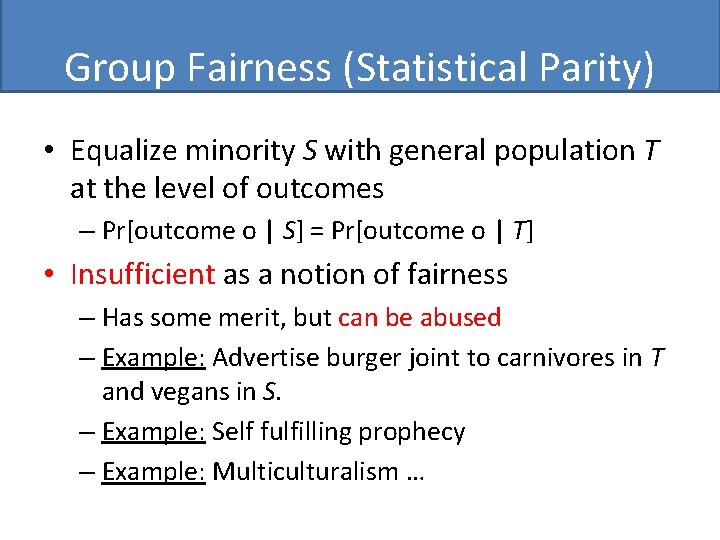
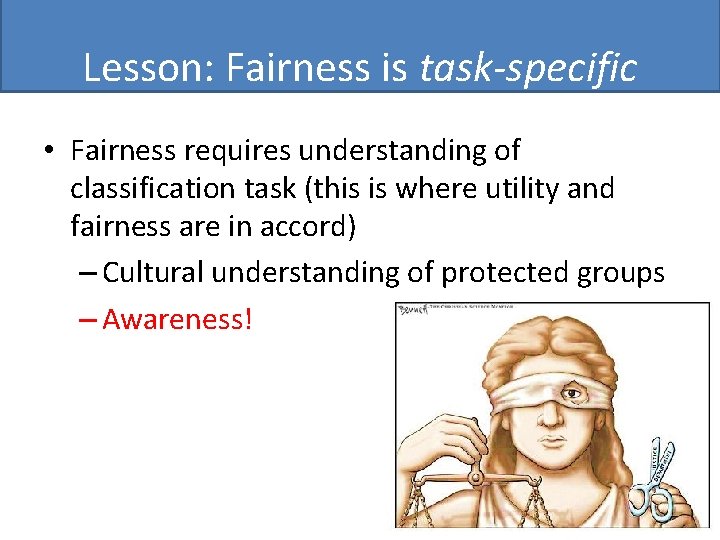
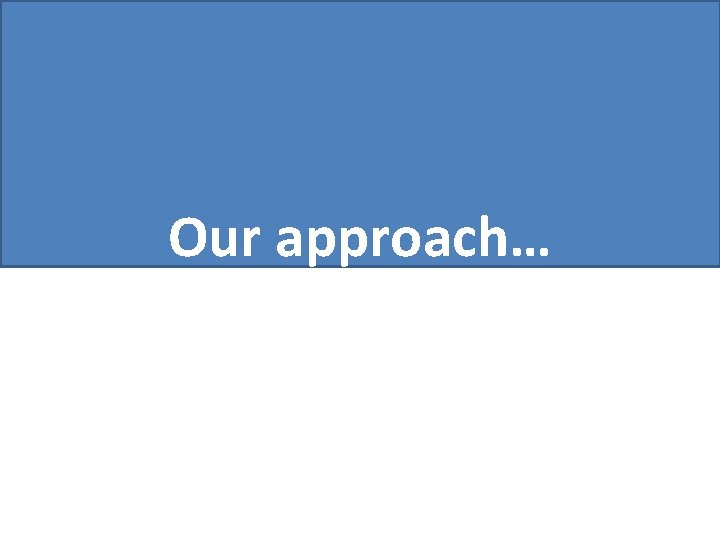
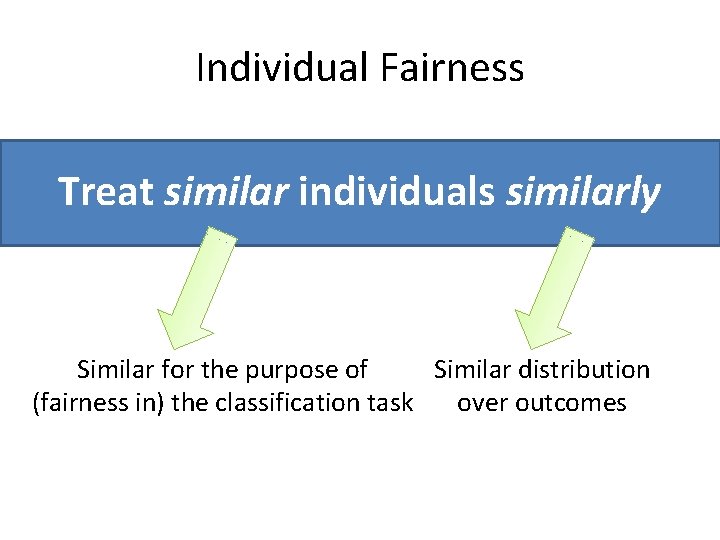
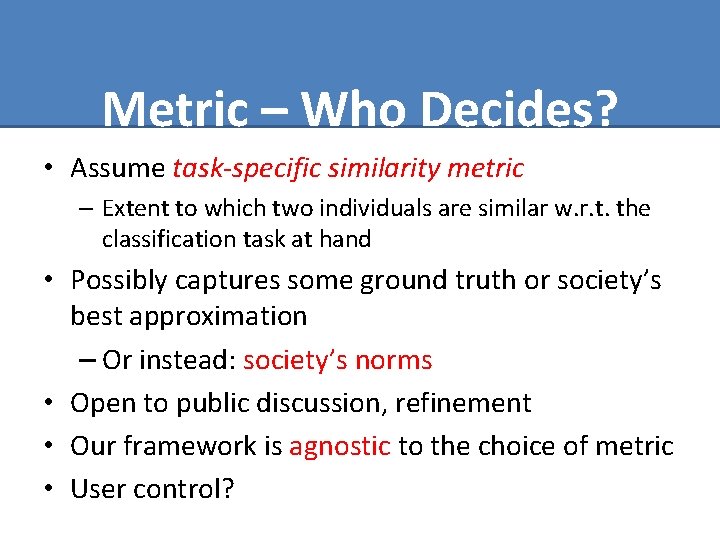
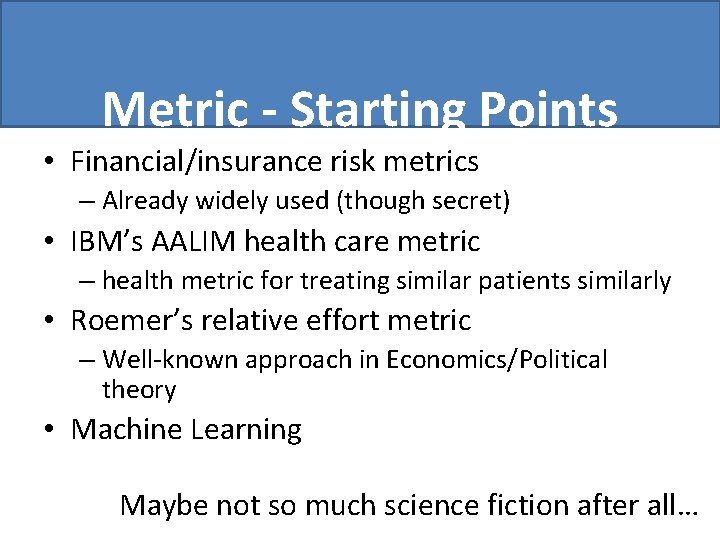
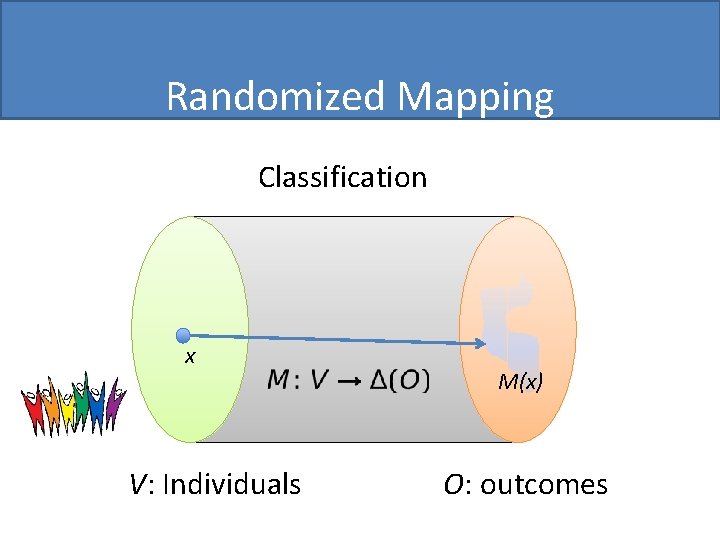
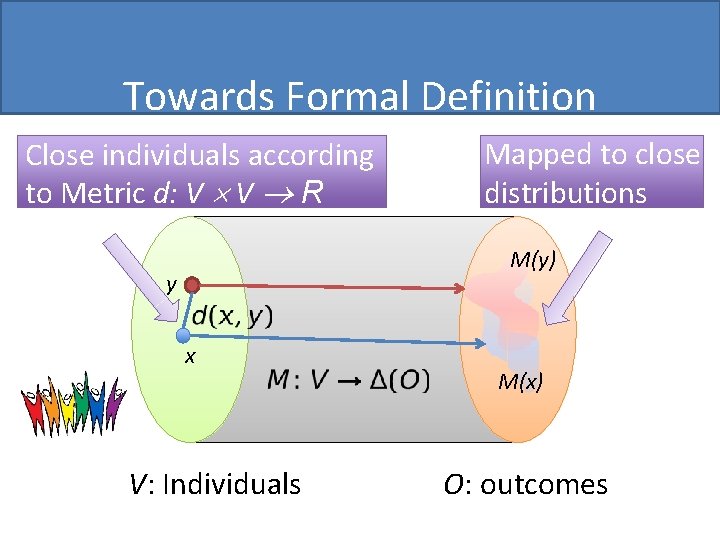
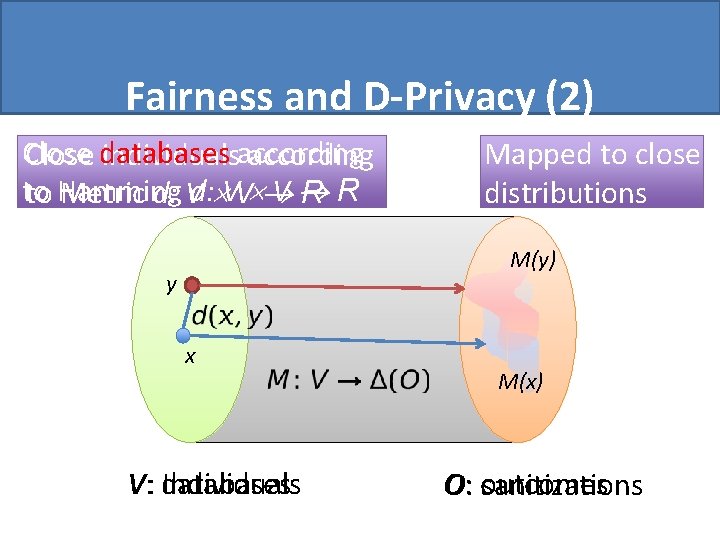
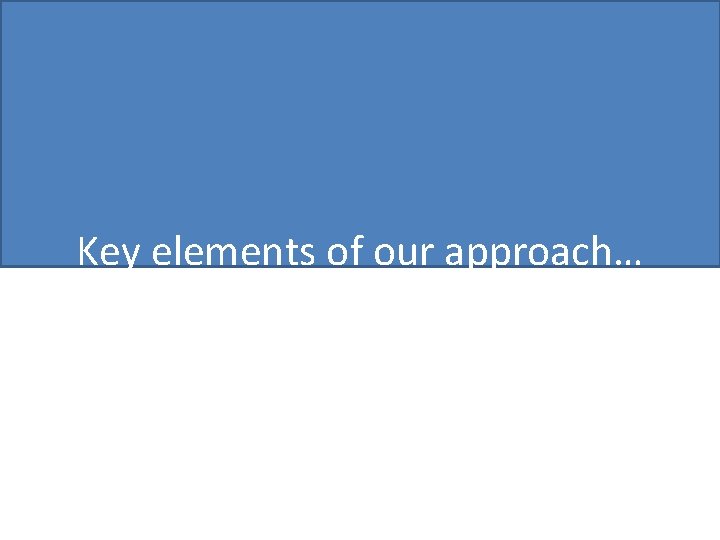
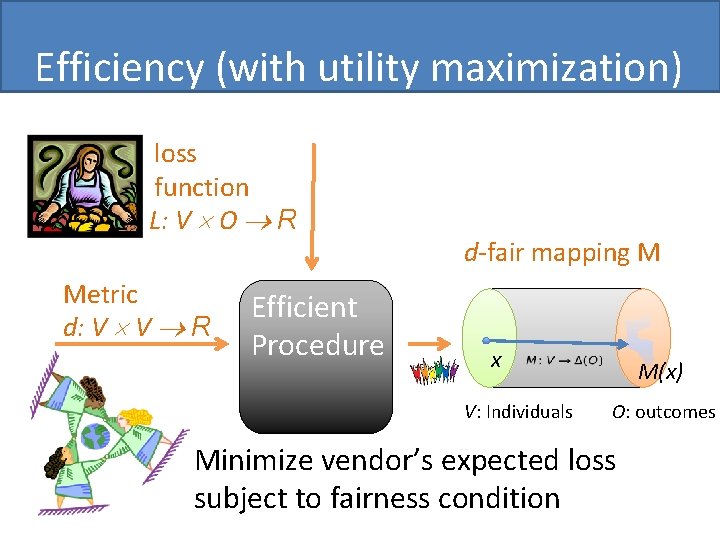
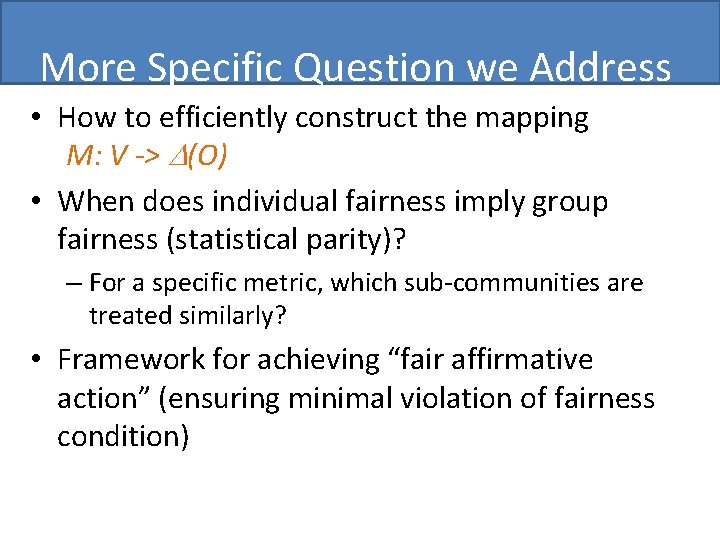
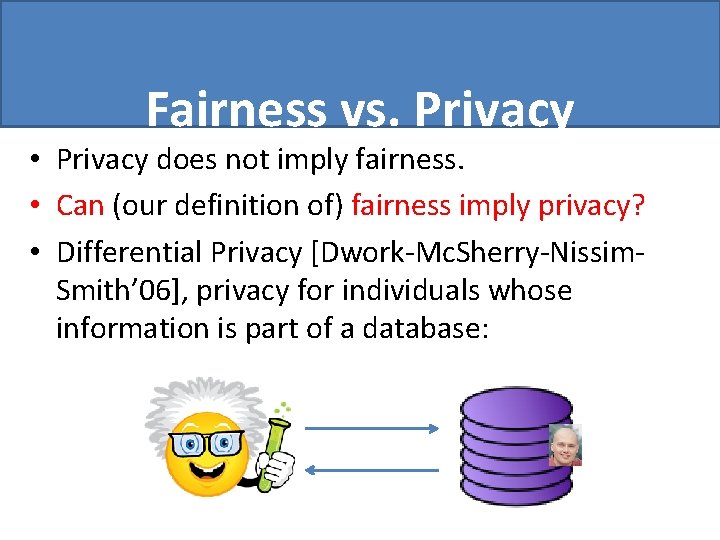
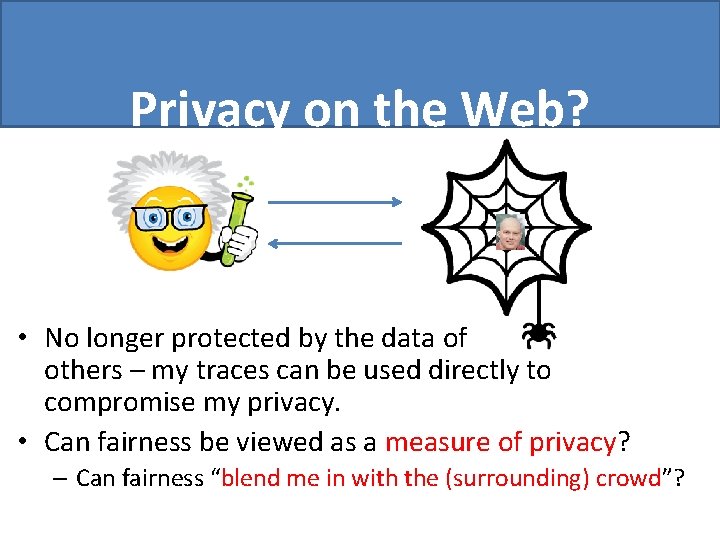
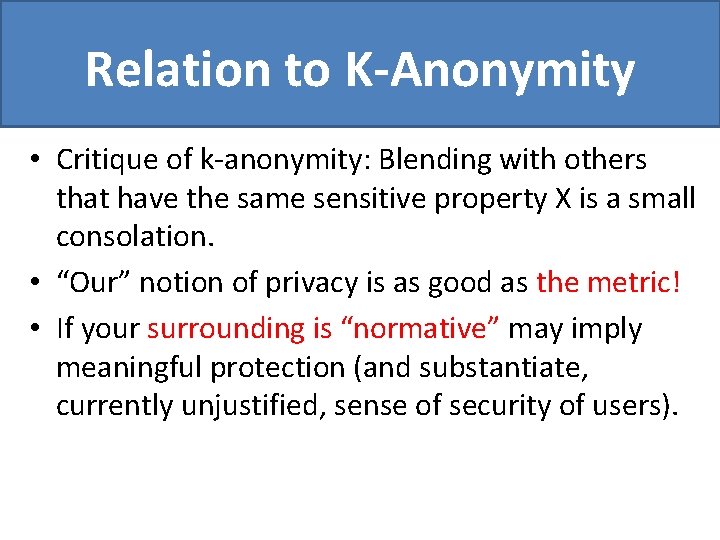
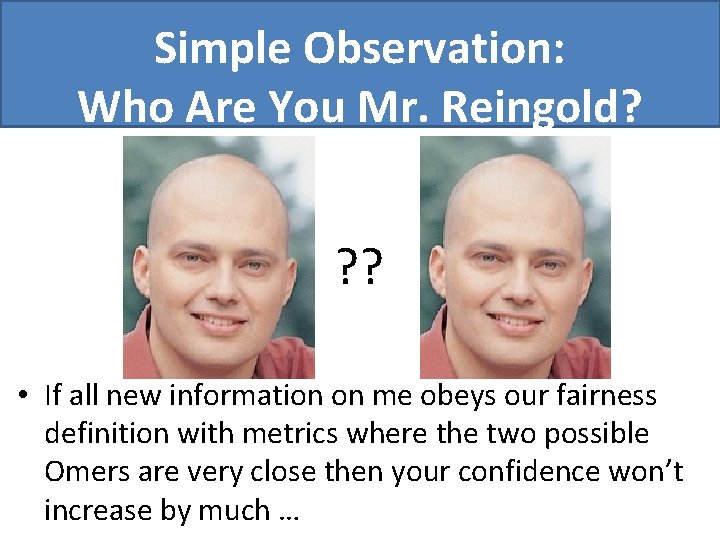
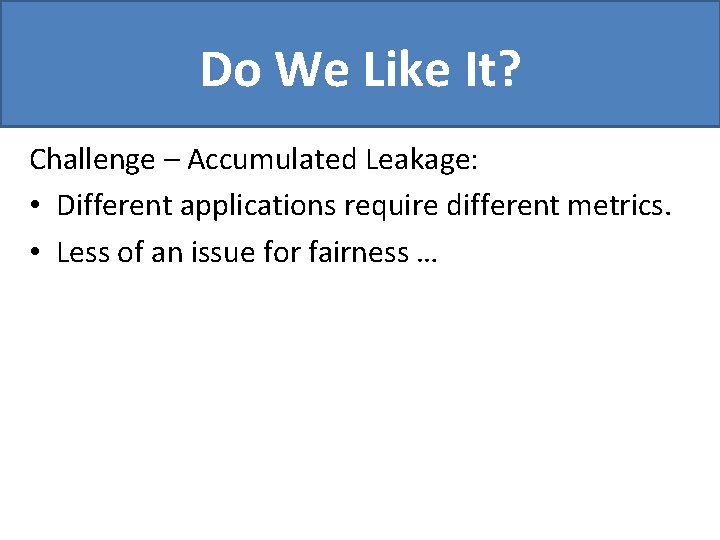
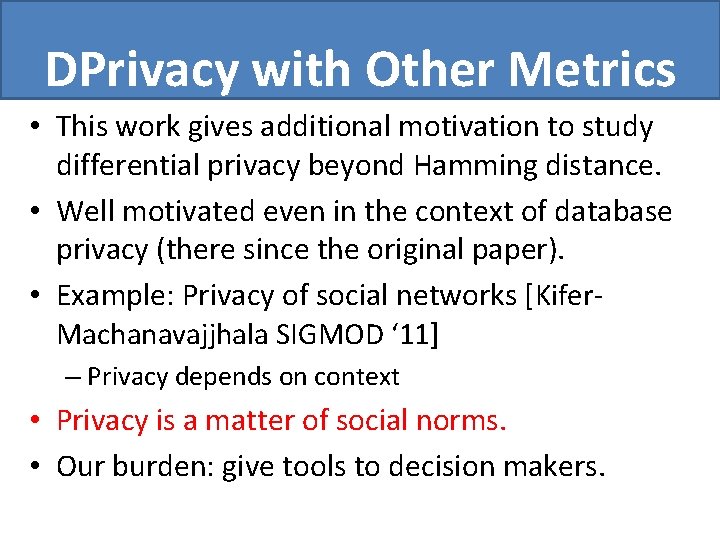
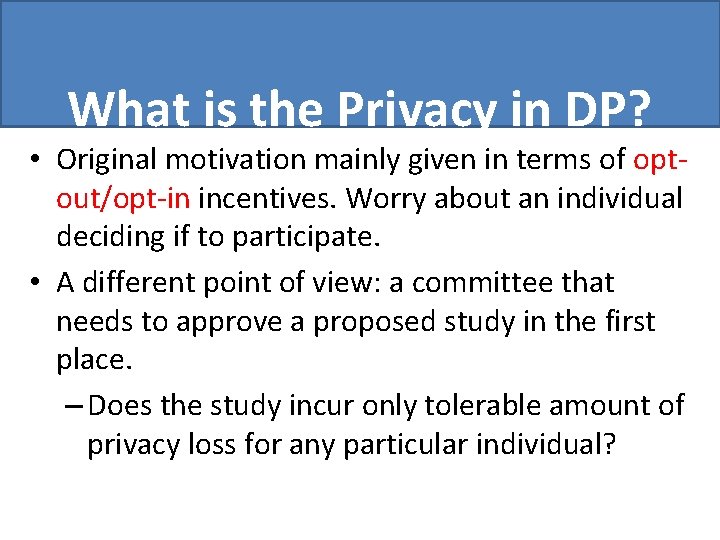
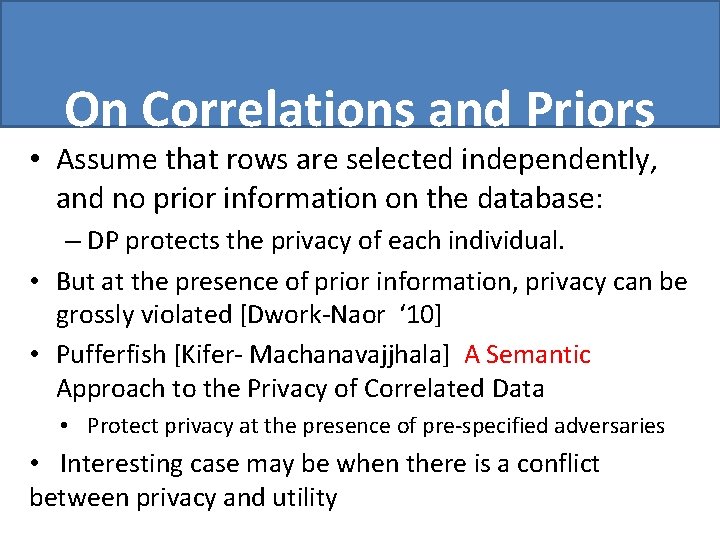
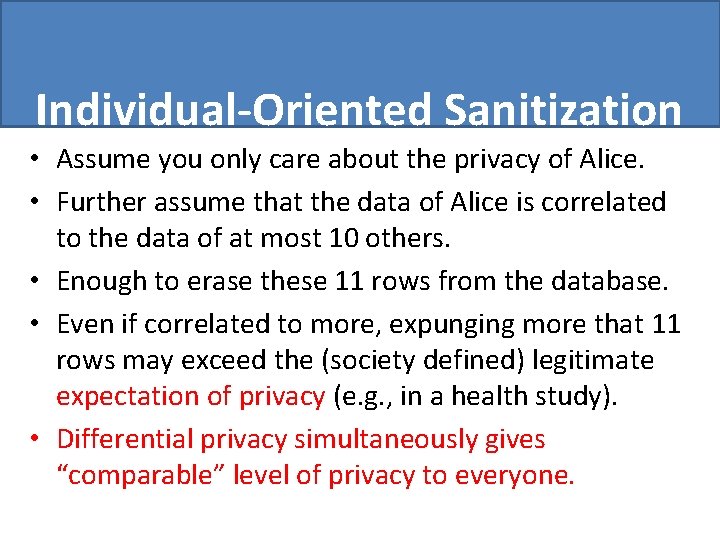
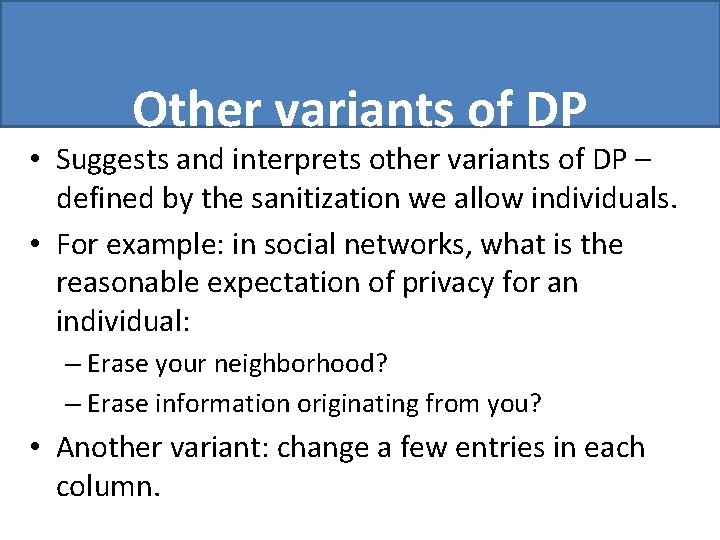
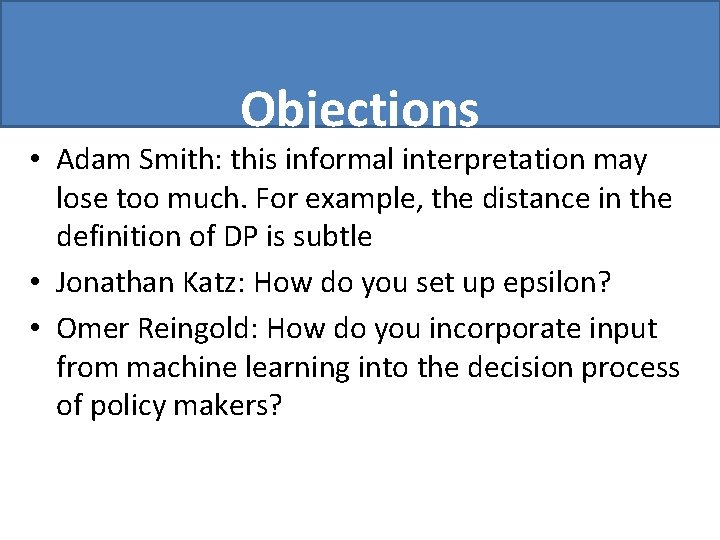
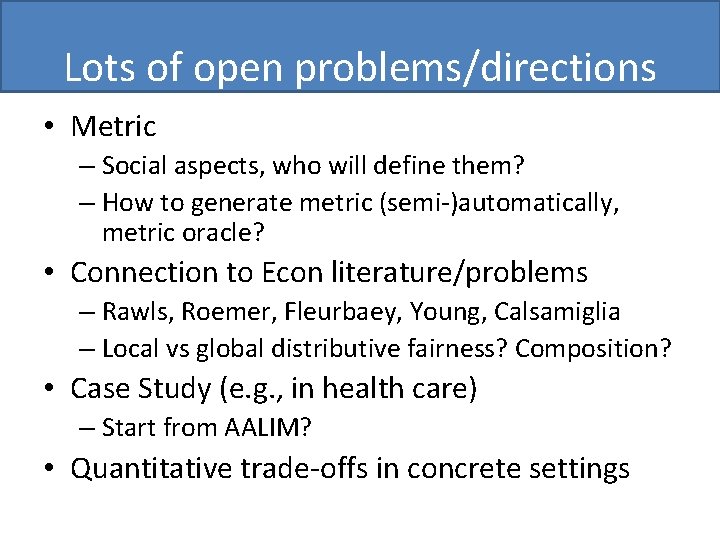
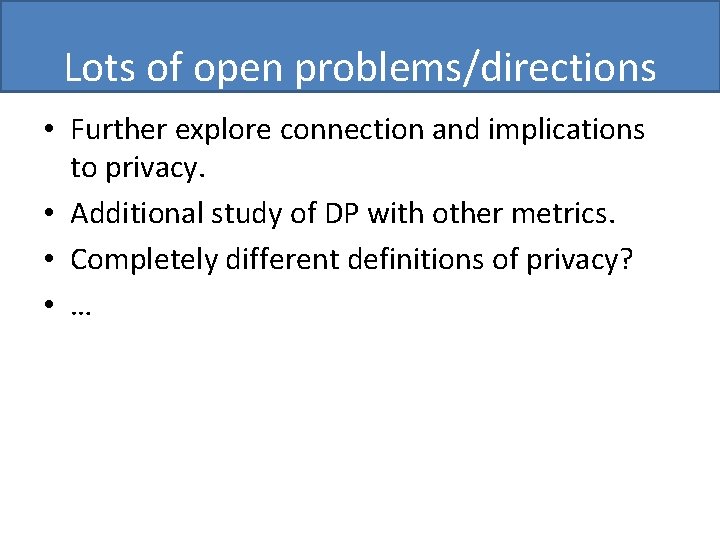
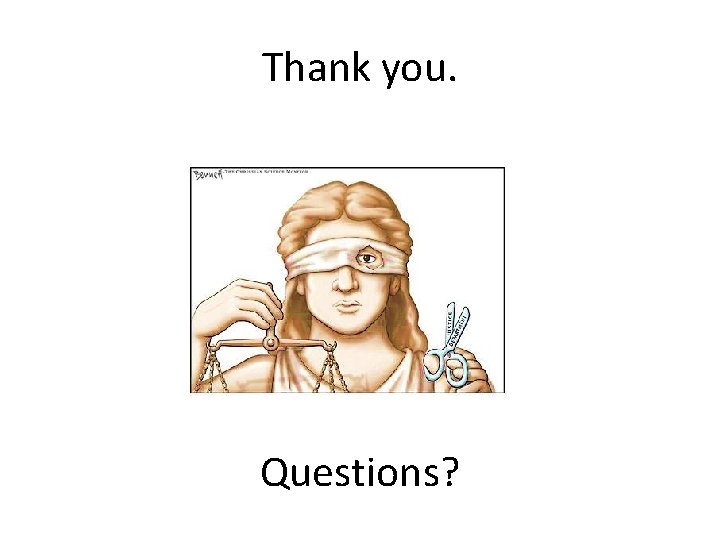
- Slides: 40
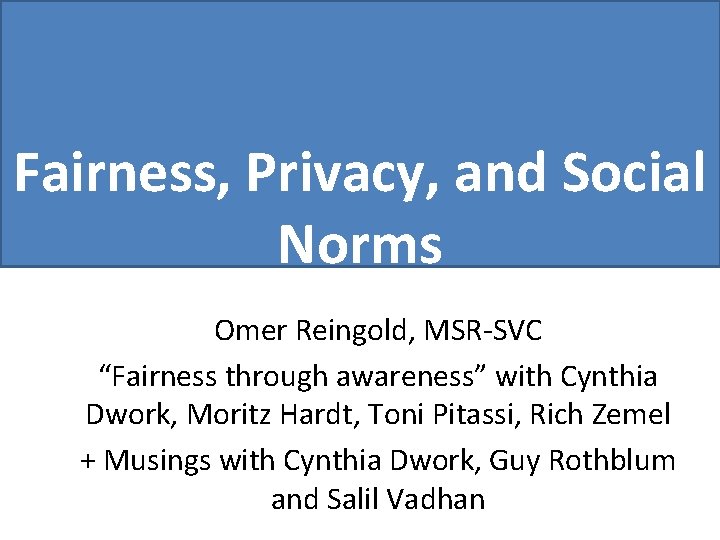
Fairness, Privacy, and Social Norms Omer Reingold, MSR-SVC “Fairness through awareness” with Cynthia Dwork, Moritz Hardt, Toni Pitassi, Rich Zemel + Musings with Cynthia Dwork, Guy Rothblum and Salil Vadhan
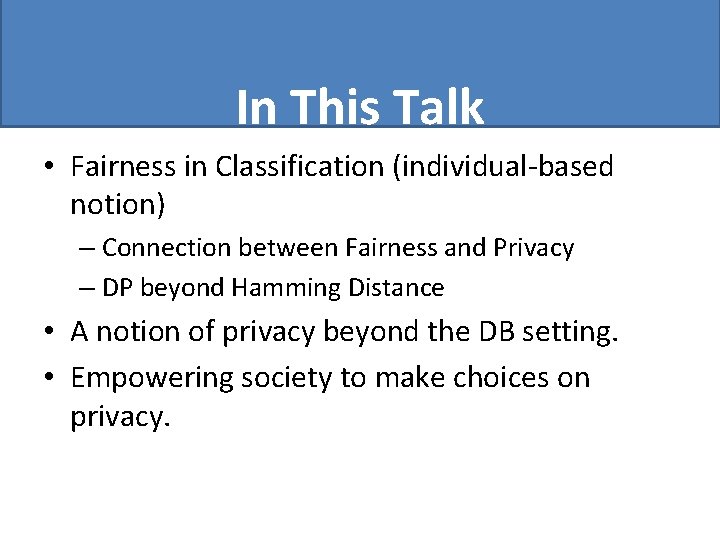
In This Talk • Fairness in Classification (individual-based notion) – Connection between Fairness and Privacy – DP beyond Hamming Distance • A notion of privacy beyond the DB setting. • Empowering society to make choices on privacy.
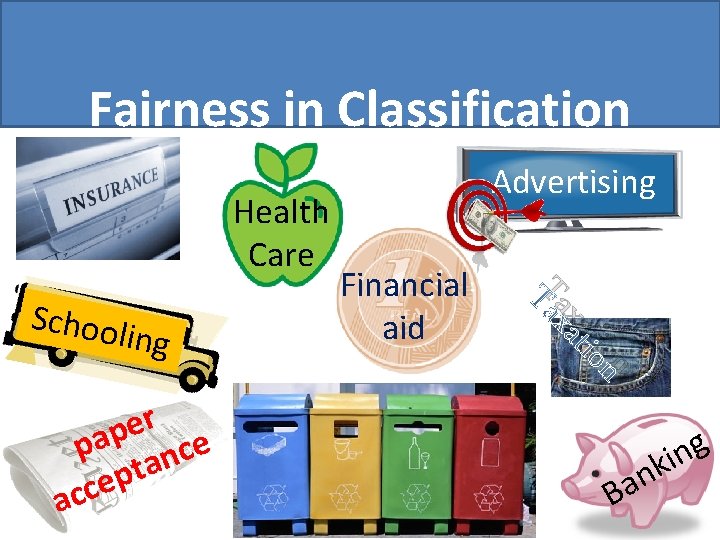
Fairness in Classification Health Care ion r e pap nce a t p e c c a xat ing Financial aid Ta School Advertising g n i k n Ba
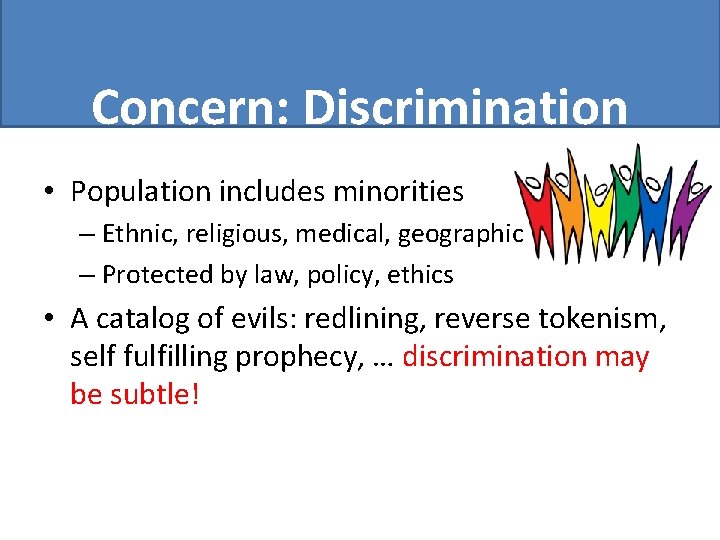
Concern: Discrimination • Population includes minorities – Ethnic, religious, medical, geographic – Protected by law, policy, ethics • A catalog of evils: redlining, reverse tokenism, self fulfilling prophecy, … discrimination may be subtle!
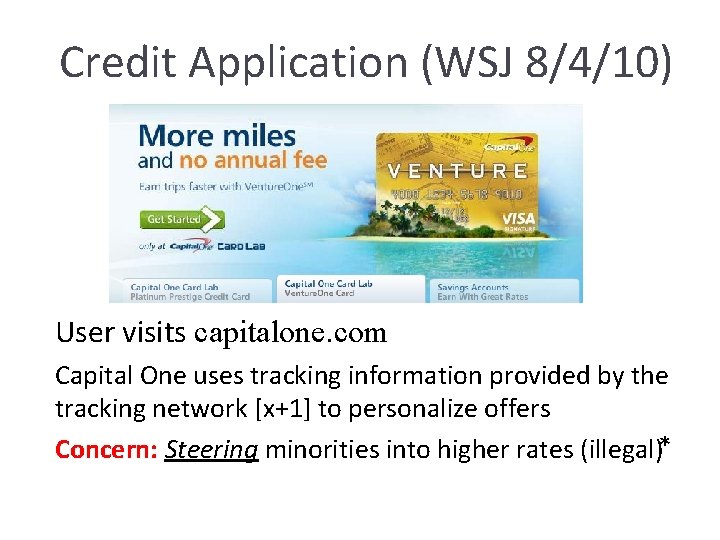
Credit Application (WSJ 8/4/10) User visits capitalone. com Capital One uses tracking information provided by the tracking network [x+1] to personalize offers Concern: Steering minorities into higher rates (illegal)*
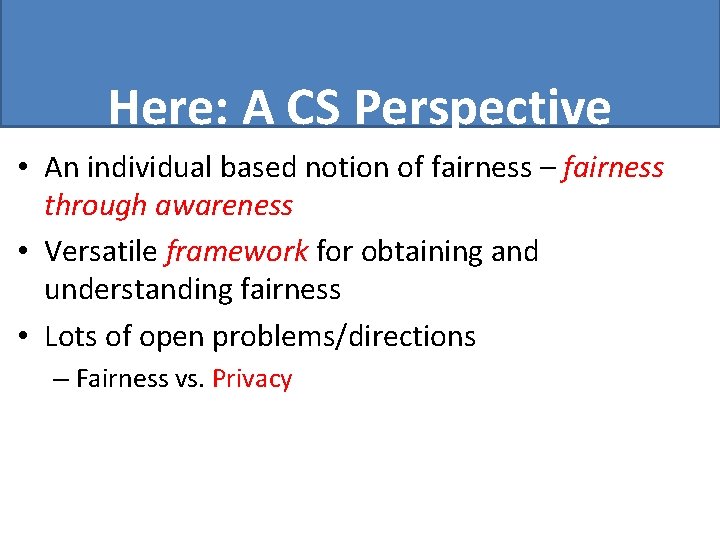
Here: A CS Perspective • An individual based notion of fairness – fairness through awareness • Versatile framework for obtaining and understanding fairness • Lots of open problems/directions – Fairness vs. Privacy
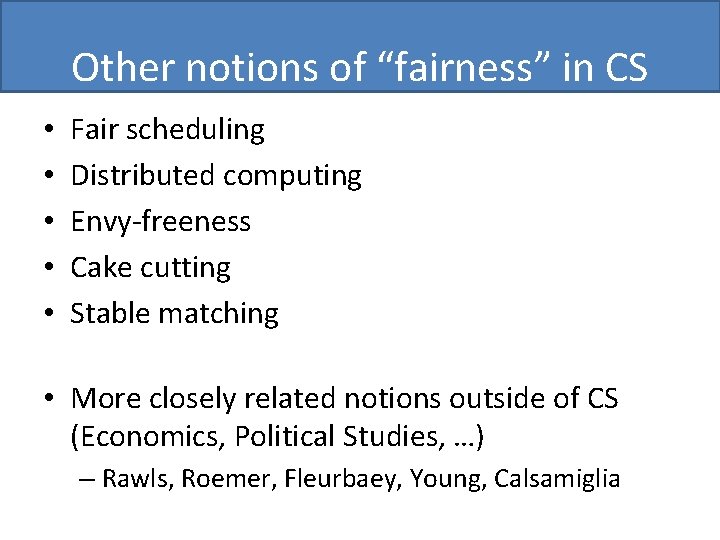
Other notions of “fairness” in CS • • • Fair scheduling Distributed computing Envy-freeness Cake cutting Stable matching • More closely related notions outside of CS (Economics, Political Studies, …) – Rawls, Roemer, Fleurbaey, Young, Calsamiglia
![Fairness and Privacy 1 Dwork Mulligan 2012 objections to online behavioral targeting Fairness and Privacy (1) • [Dwork & Mulligan 2012] objections to online behavioral targeting](https://slidetodoc.com/presentation_image_h/fb3938be4f2b241455458125d3b53db7/image-8.jpg)
Fairness and Privacy (1) • [Dwork & Mulligan 2012] objections to online behavioral targeting often expressed in terms of privacy. In many cases the underlying concern is better described in terms of fairness (e. g. , price discrimination, being mistreated). – Other major concern: feeling of “ickiness” [Tene] • Privacy does not imply fairness – Definitions and techniques useful. – Can Fairness Imply Privacy (beyond DB setting)?
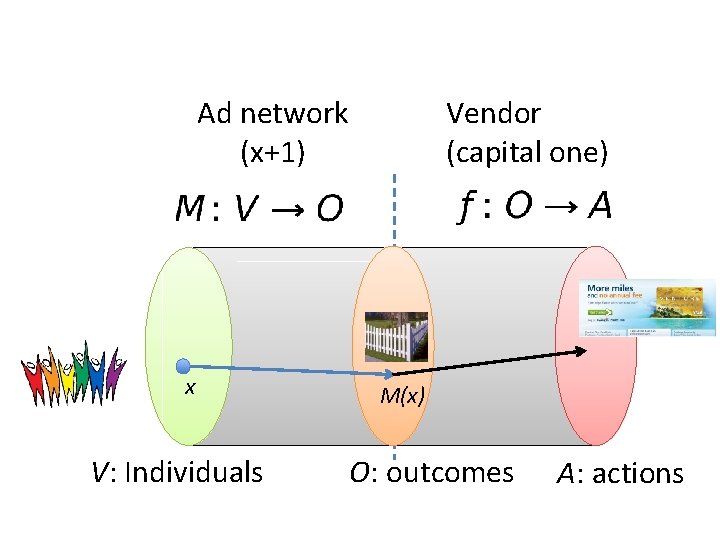
Ad network (x+1) x V: Individuals Vendor (capital one) M(x) O: outcomes A: actions
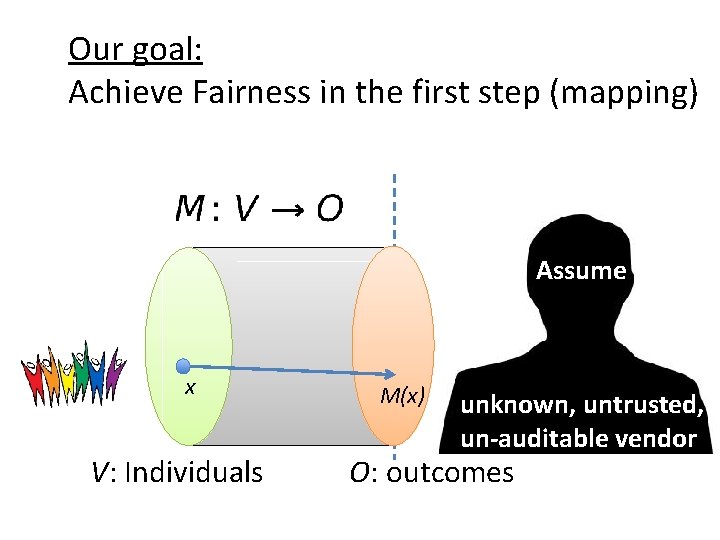
Our goal: Achieve Fairness in the first step (mapping) Assume x V: Individuals M(x) unknown, untrusted, un-auditable vendor O: outcomes
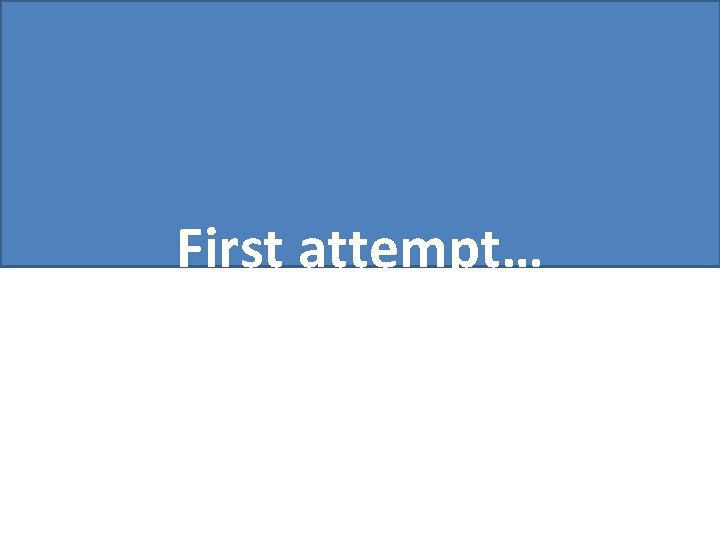
First attempt…
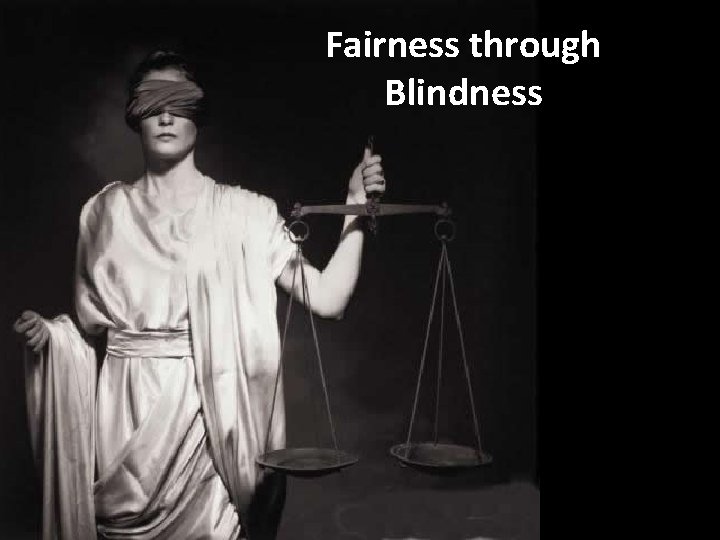
Fairness through Blindness
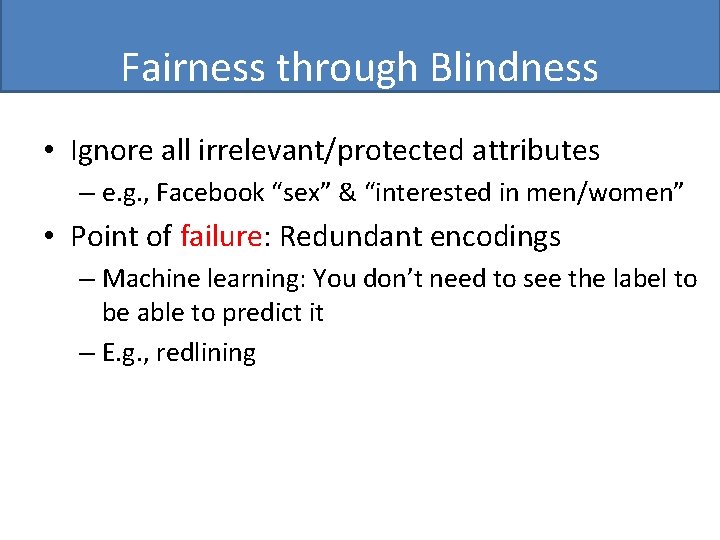
Fairness through Blindness • Ignore all irrelevant/protected attributes – e. g. , Facebook “sex” & “interested in men/women” • Point of failure: Redundant encodings – Machine learning: You don’t need to see the label to be able to predict it – E. g. , redlining
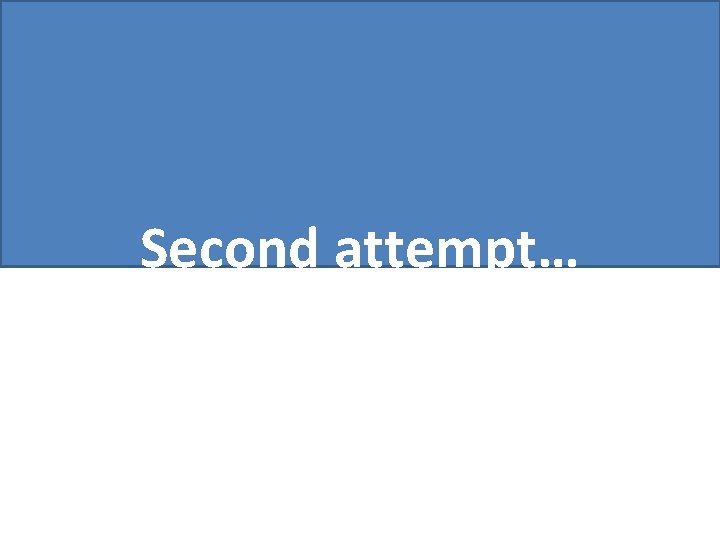
Second attempt…
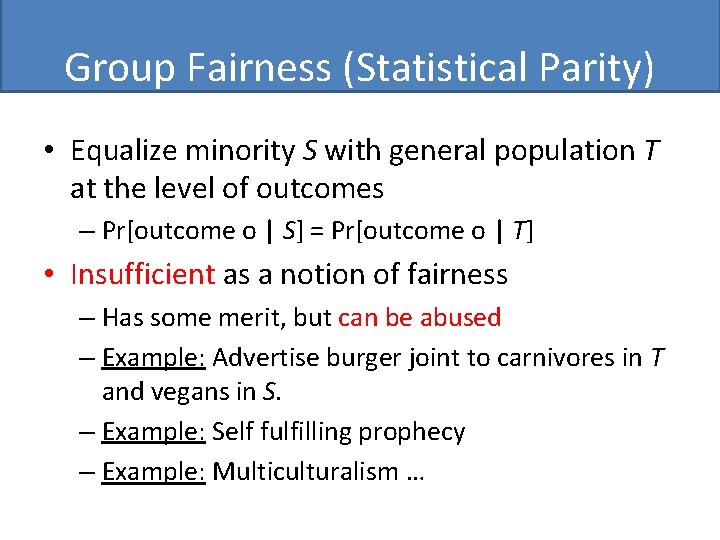
Group Fairness (Statistical Parity) • Equalize minority S with general population T at the level of outcomes – Pr[outcome o | S] = Pr[outcome o | T] • Insufficient as a notion of fairness – Has some merit, but can be abused – Example: Advertise burger joint to carnivores in T and vegans in S. – Example: Self fulfilling prophecy – Example: Multiculturalism …
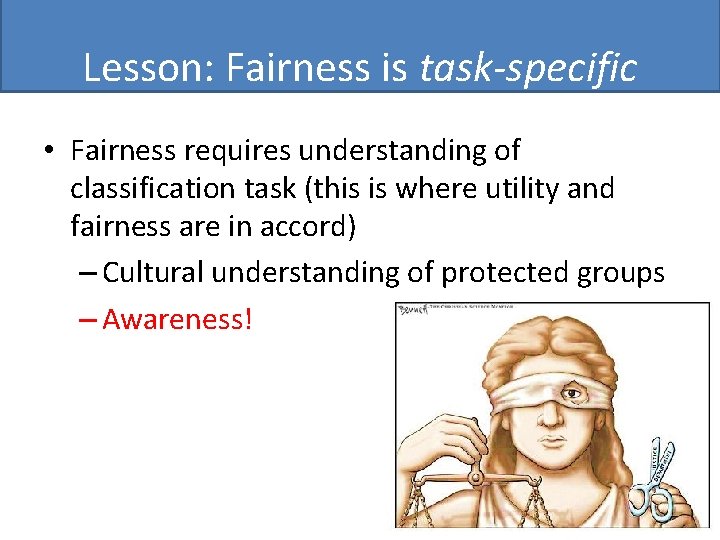
Lesson: Fairness is task-specific • Fairness requires understanding of classification task (this is where utility and fairness are in accord) – Cultural understanding of protected groups – Awareness!
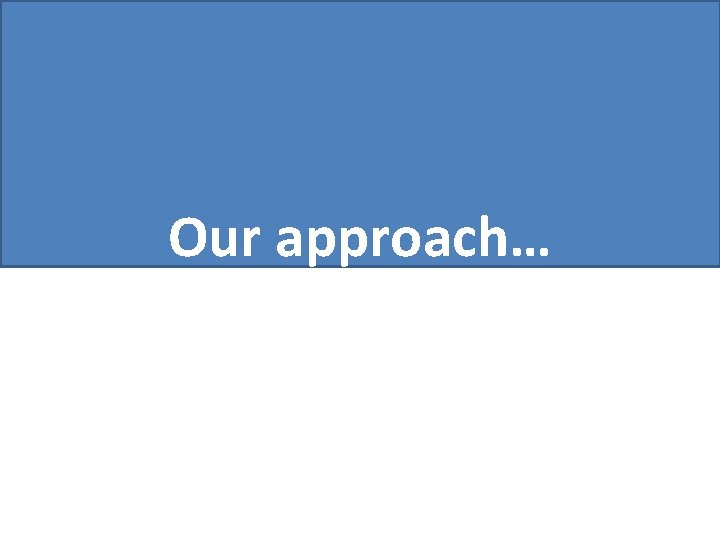
Our approach…
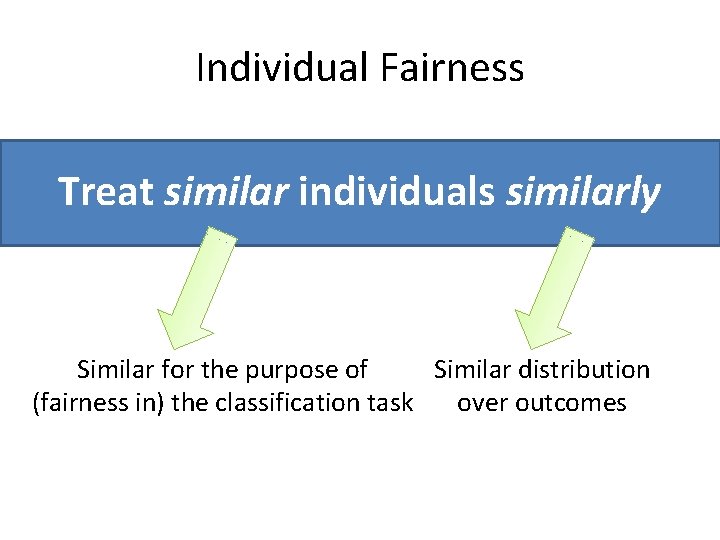
Individual Fairness Treat similar individuals similarly Similar for the purpose of Similar distribution (fairness in) the classification task over outcomes
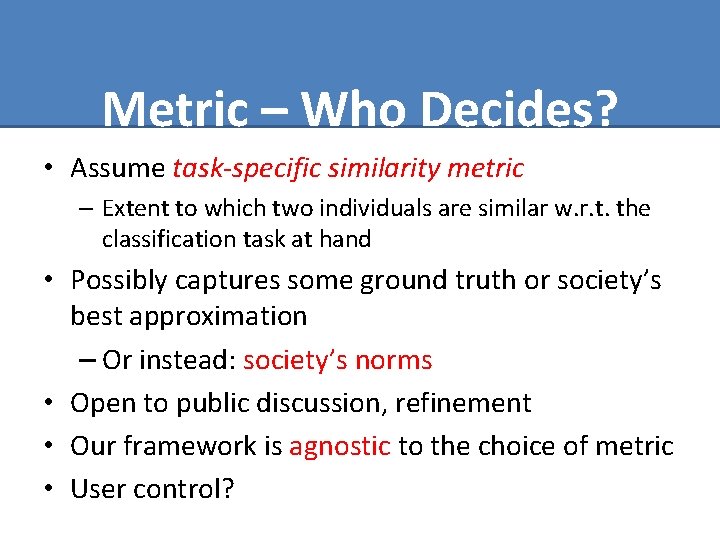
Metric – Who Decides? • Assume task-specific similarity metric – Extent to which two individuals are similar w. r. t. the classification task at hand • Possibly captures some ground truth or society’s best approximation – Or instead: society’s norms • Open to public discussion, refinement • Our framework is agnostic to the choice of metric • User control?
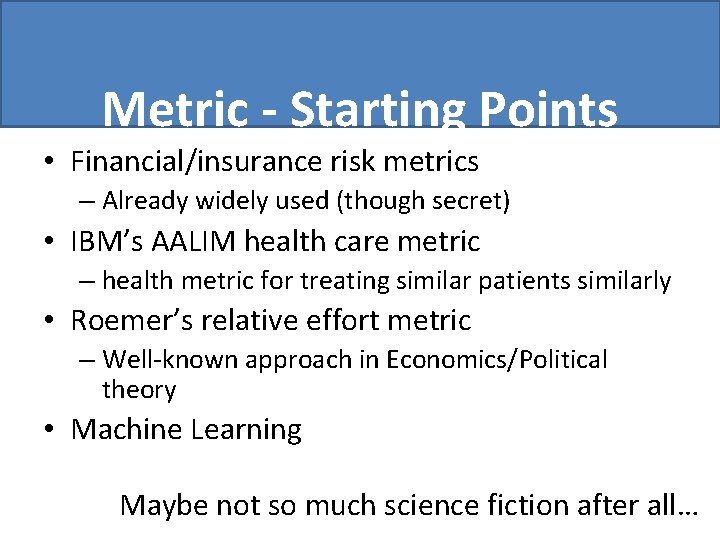
Metric - Starting Points • Financial/insurance risk metrics – Already widely used (though secret) • IBM’s AALIM health care metric – health metric for treating similar patients similarly • Roemer’s relative effort metric – Well-known approach in Economics/Political theory • Machine Learning Maybe not so much science fiction after all…
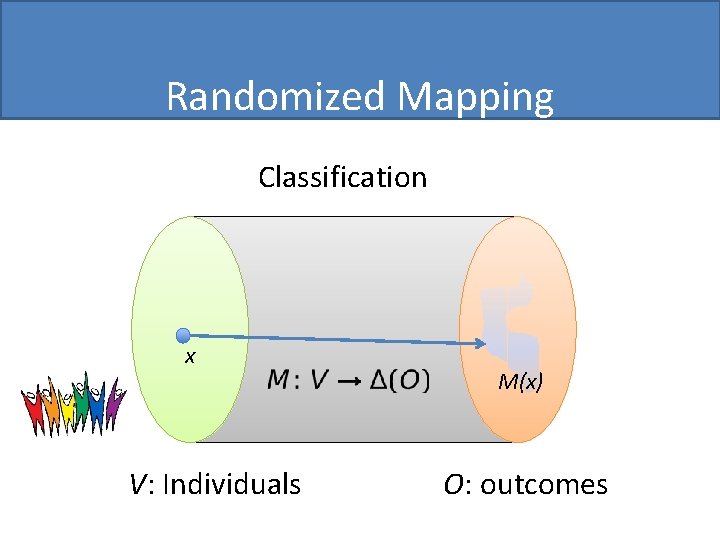
Randomized Mapping Classification x V: Individuals M(x) O: outcomes
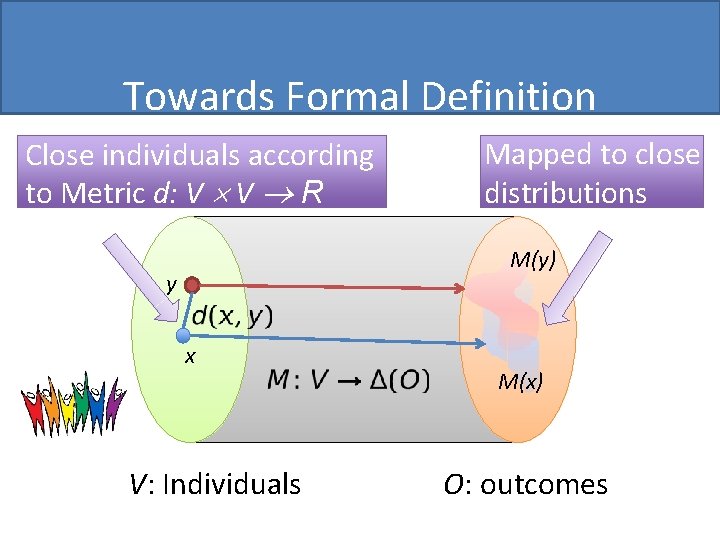
Towards Formal Definition Close individuals according to Metric d: V V R Mapped to close distributions M(y) y x V: Individuals M(x) O: outcomes
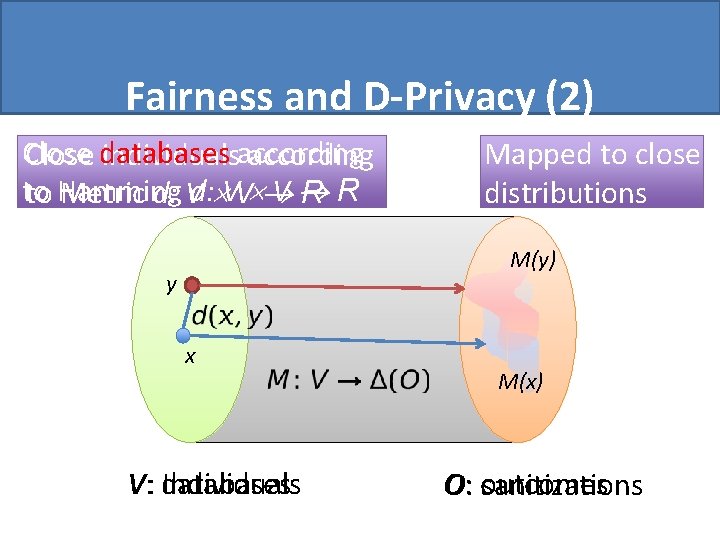
Fairness and D-Privacy (2) Close databases individualsaccording to Hamming V Metric d: VV R R Mapped to close distributions M(y) y x V: databases Individuals M(x) O: outcomes sanitizations
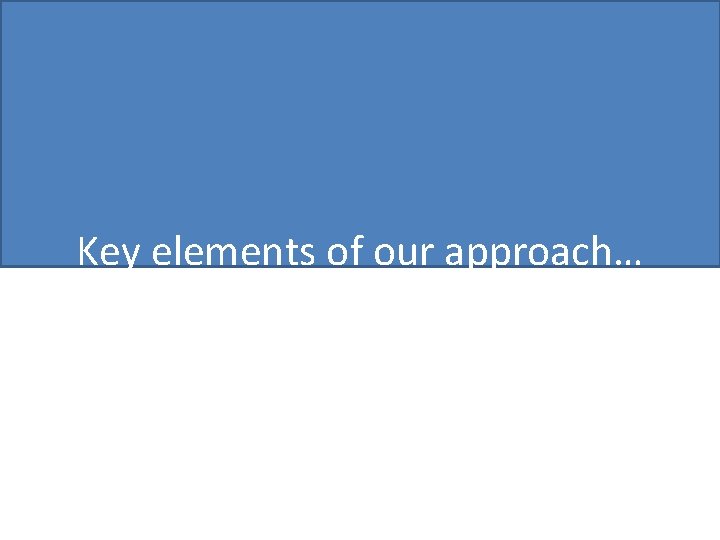
Key elements of our approach…
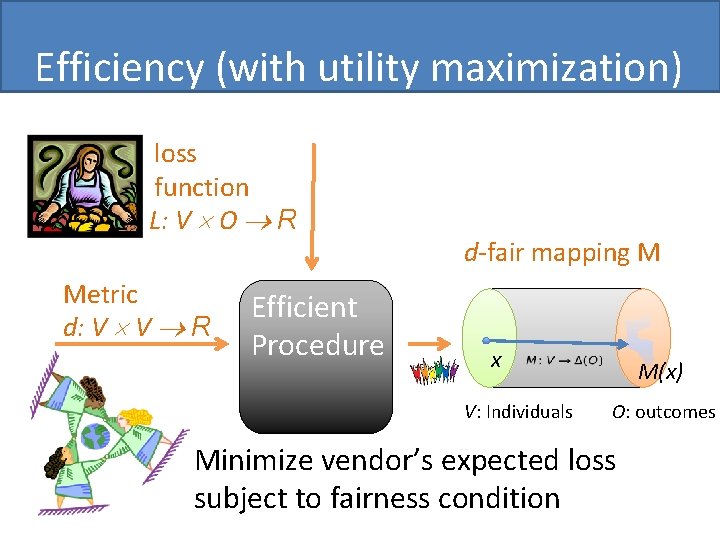
Efficiency (with utility maximization) loss function L: V O R Metric d: V V R Efficient Procedure d-fair mapping M x V: Individuals M(x) O: outcomes Minimize vendor’s expected loss subject to fairness condition
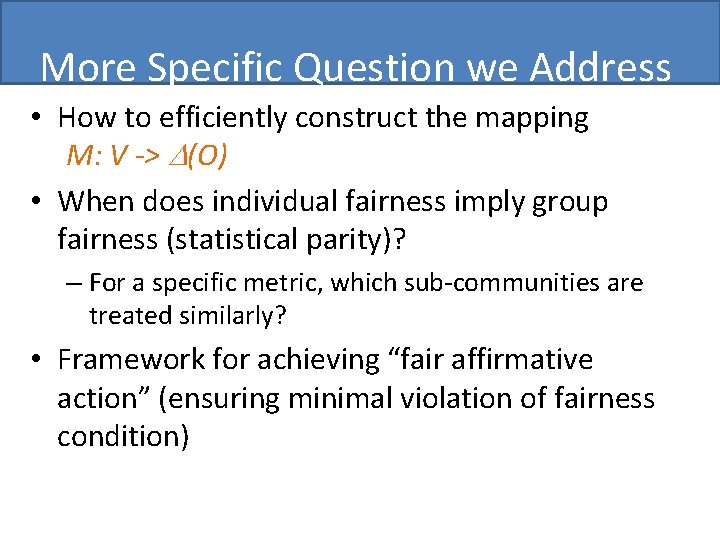
More Specific Question we Address • How to efficiently construct the mapping M: V -> (O) • When does individual fairness imply group fairness (statistical parity)? – For a specific metric, which sub-communities are treated similarly? • Framework for achieving “fair affirmative action” (ensuring minimal violation of fairness condition)
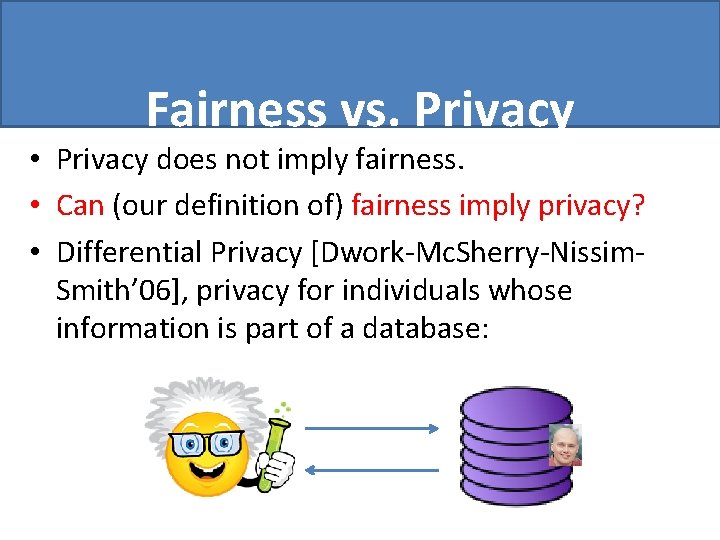
Fairness vs. Privacy • Privacy does not imply fairness. • Can (our definition of) fairness imply privacy? • Differential Privacy [Dwork-Mc. Sherry-Nissim. Smith’ 06], privacy for individuals whose information is part of a database:
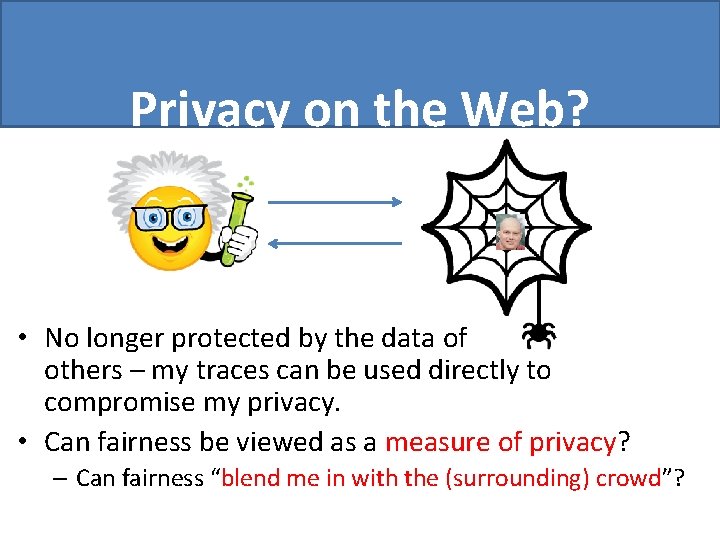
Privacy on the Web? • No longer protected by the data of others – my traces can be used directly to compromise my privacy. • Can fairness be viewed as a measure of privacy? – Can fairness “blend me in with the (surrounding) crowd”?
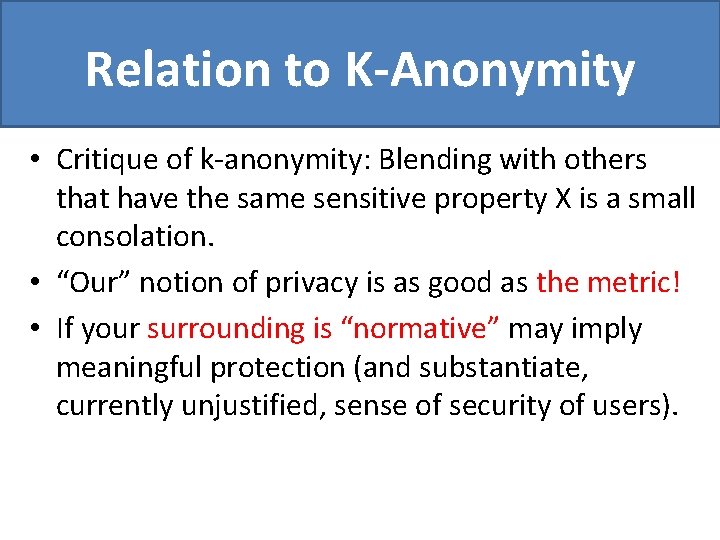
Relation to K-Anonymity • Critique of k-anonymity: Blending with others that have the same sensitive property X is a small consolation. • “Our” notion of privacy is as good as the metric! • If your surrounding is “normative” may imply meaningful protection (and substantiate, currently unjustified, sense of security of users).
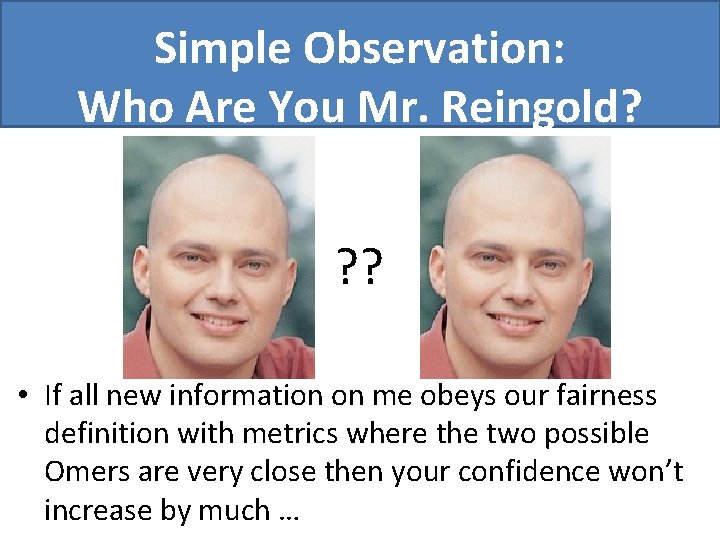
Simple Observation: Who Are You Mr. Reingold? ? ? • If all new information on me obeys our fairness definition with metrics where the two possible Omers are very close then your confidence won’t increase by much …
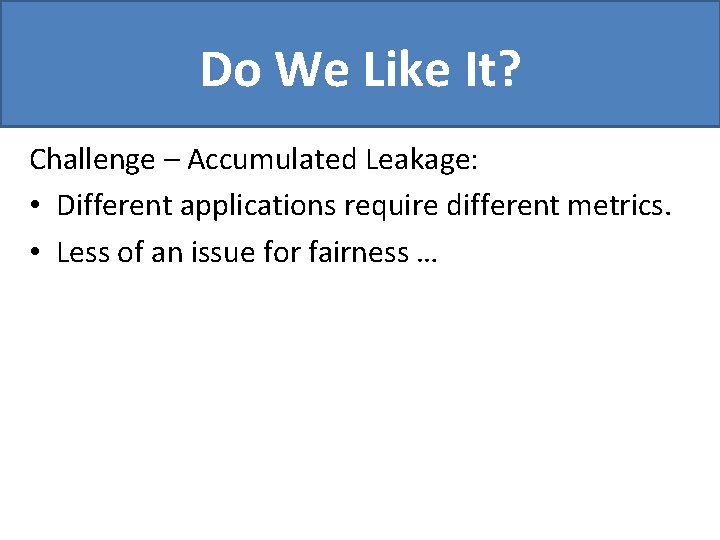
Do We Like It? Challenge – Accumulated Leakage: • Different applications require different metrics. • Less of an issue for fairness …
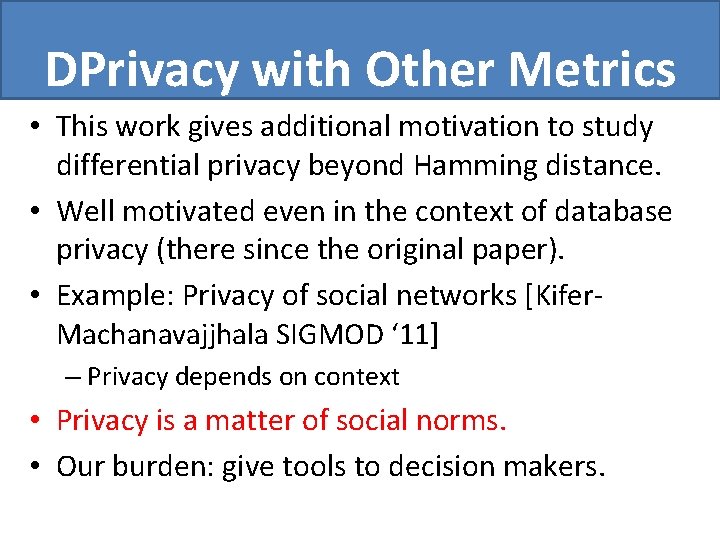
DPrivacy with Other Metrics • This work gives additional motivation to study differential privacy beyond Hamming distance. • Well motivated even in the context of database privacy (there since the original paper). • Example: Privacy of social networks [Kifer. Machanavajjhala SIGMOD ‘ 11] – Privacy depends on context • Privacy is a matter of social norms. • Our burden: give tools to decision makers.
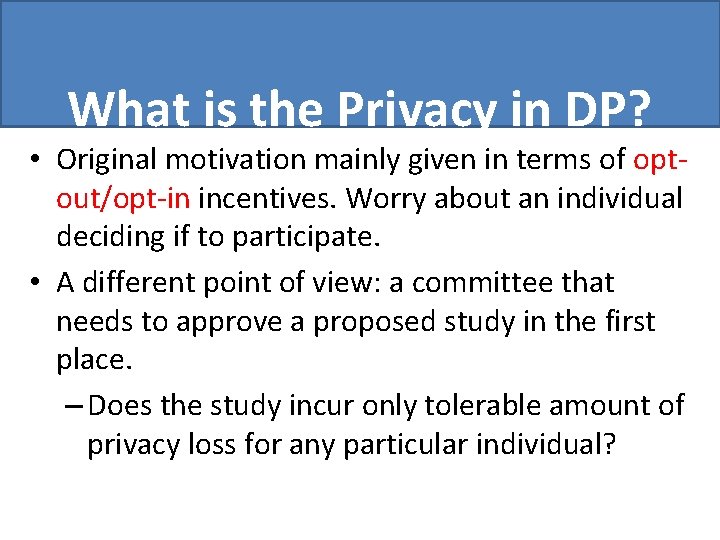
What is the Privacy in DP? • Original motivation mainly given in terms of optout/opt-in incentives. Worry about an individual deciding if to participate. • A different point of view: a committee that needs to approve a proposed study in the first place. – Does the study incur only tolerable amount of privacy loss for any particular individual?
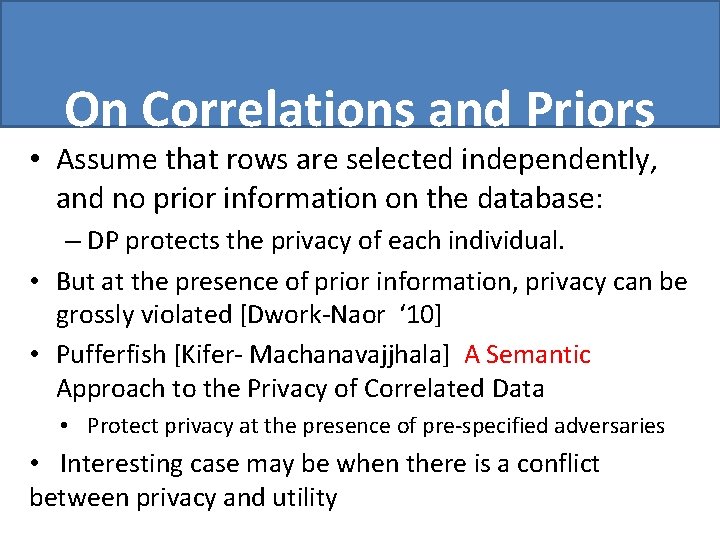
On Correlations and Priors • Assume that rows are selected independently, and no prior information on the database: – DP protects the privacy of each individual. • But at the presence of prior information, privacy can be grossly violated [Dwork-Naor ‘ 10] • Pufferfish [Kifer- Machanavajjhala] A Semantic Approach to the Privacy of Correlated Data • Protect privacy at the presence of pre-specified adversaries • Interesting case may be when there is a conflict between privacy and utility
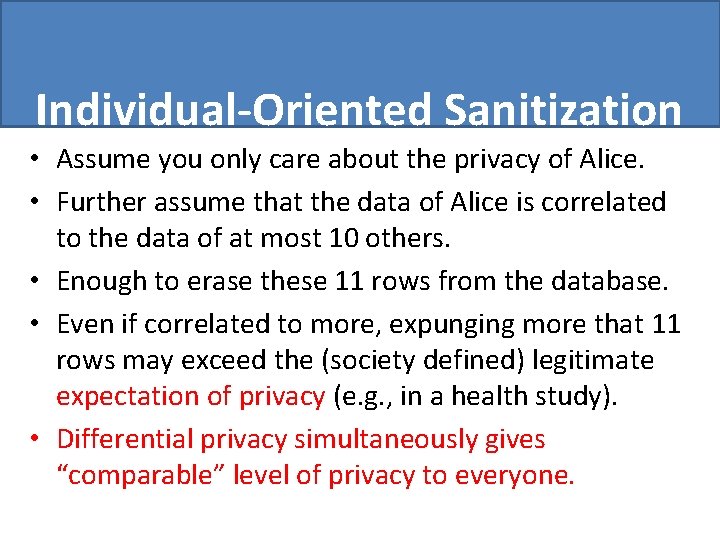
Individual-Oriented Sanitization • Assume you only care about the privacy of Alice. • Further assume that the data of Alice is correlated to the data of at most 10 others. • Enough to erase these 11 rows from the database. • Even if correlated to more, expunging more that 11 rows may exceed the (society defined) legitimate expectation of privacy (e. g. , in a health study). • Differential privacy simultaneously gives “comparable” level of privacy to everyone.
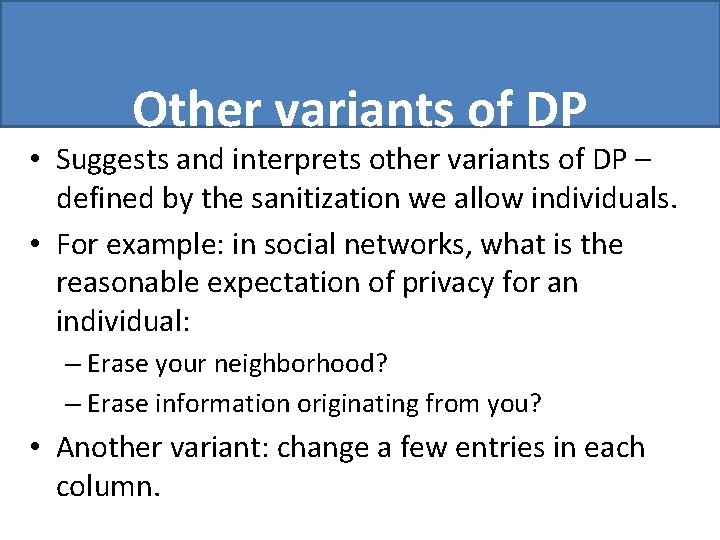
Other variants of DP • Suggests and interprets other variants of DP – defined by the sanitization we allow individuals. • For example: in social networks, what is the reasonable expectation of privacy for an individual: – Erase your neighborhood? – Erase information originating from you? • Another variant: change a few entries in each column.
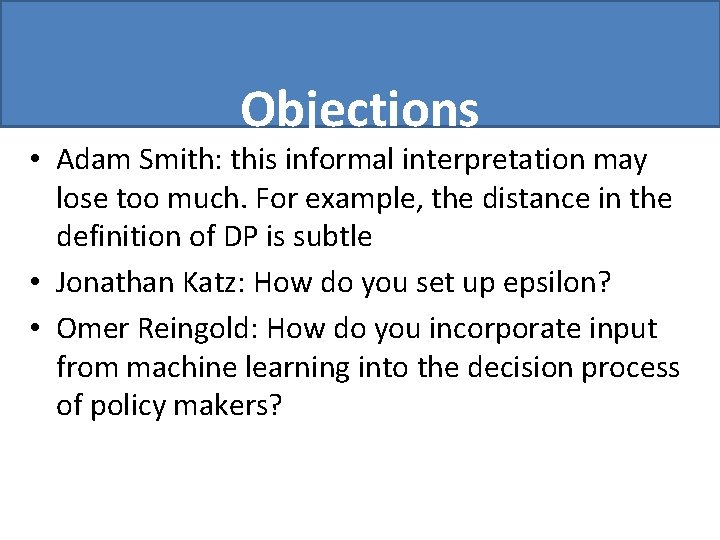
Objections • Adam Smith: this informal interpretation may lose too much. For example, the distance in the definition of DP is subtle • Jonathan Katz: How do you set up epsilon? • Omer Reingold: How do you incorporate input from machine learning into the decision process of policy makers?
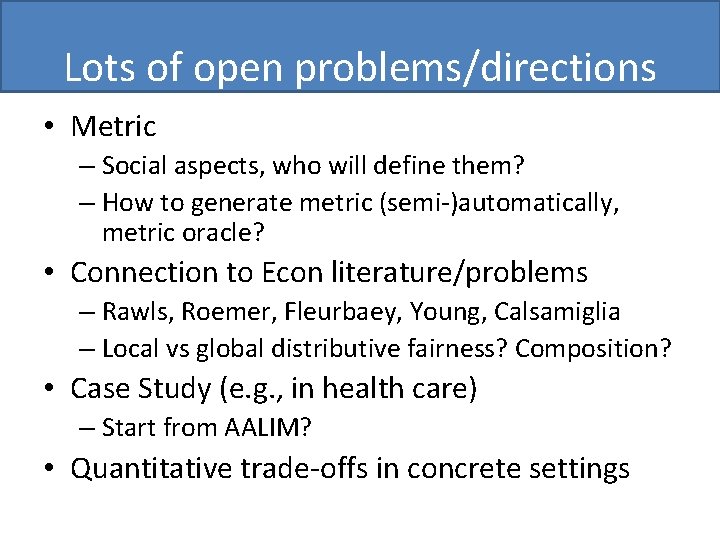
Lots of open problems/directions • Metric – Social aspects, who will define them? – How to generate metric (semi-)automatically, metric oracle? • Connection to Econ literature/problems – Rawls, Roemer, Fleurbaey, Young, Calsamiglia – Local vs global distributive fairness? Composition? • Case Study (e. g. , in health care) – Start from AALIM? • Quantitative trade-offs in concrete settings
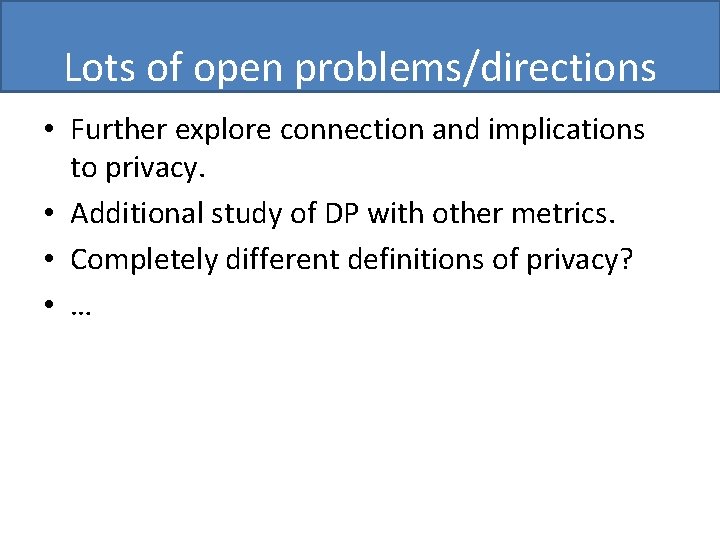
Lots of open problems/directions • Further explore connection and implications to privacy. • Additional study of DP with other metrics. • Completely different definitions of privacy? • …
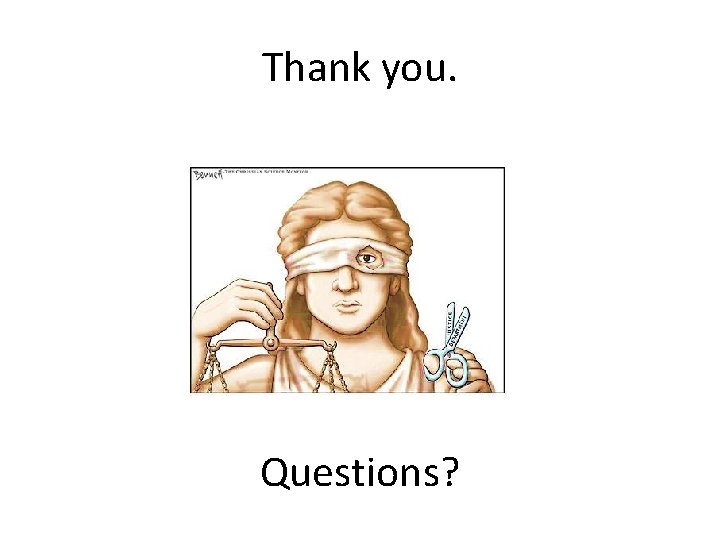
Thank you. Questions?
Omer reingold
Omer reingold
Cvs privacy awareness training answers
Global context
Fairness and diversity in the workplace
Justice as fairness
Fairness and flawless ceo
Values and norms examples
Big data privacy issues in public social media
Social media privacy a contradiction in terms
Veil of ignorance example
Demographic parity
Dominant resource fairness
Fairness slippery slope
Max-min fairness example
Fairness scenarios
Nimpact
The fairness doctrine
Procedural justice
Fairness
Fairness
Keva cream
Max-min fairness
Substantive fairness
Fairness adjective
Deviation from social norms
Norms examples
Primary and secondary deviance
Behavior that violates significant social norms.
Omer morshed
Salvatore guarnieri
Aisha omer
Victoria sece
Güneş ışını
Jean omer marie gabriel monnet
Adalet evrenin ruhudur ömer hayyam
ömer hayyam ın yaptığı çalışmalar
Omer yezdani
ömer ergenç ymm
Victoria sece
Omer berkman