Experimental Research Methods in Language Learning Chapter 14
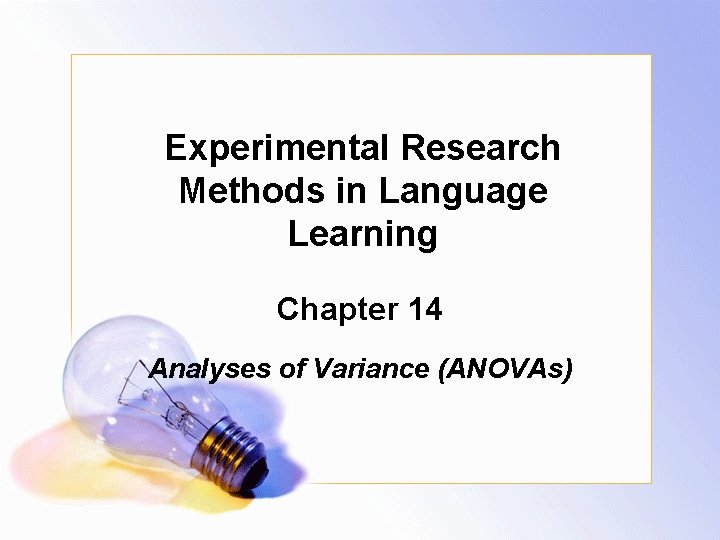
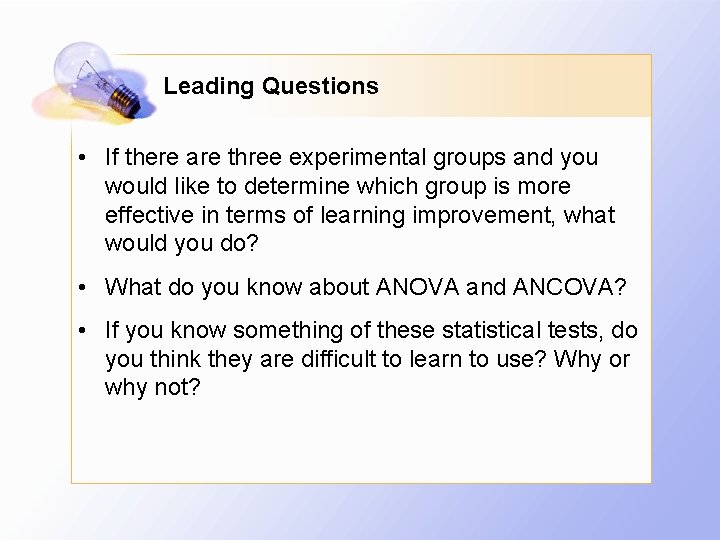
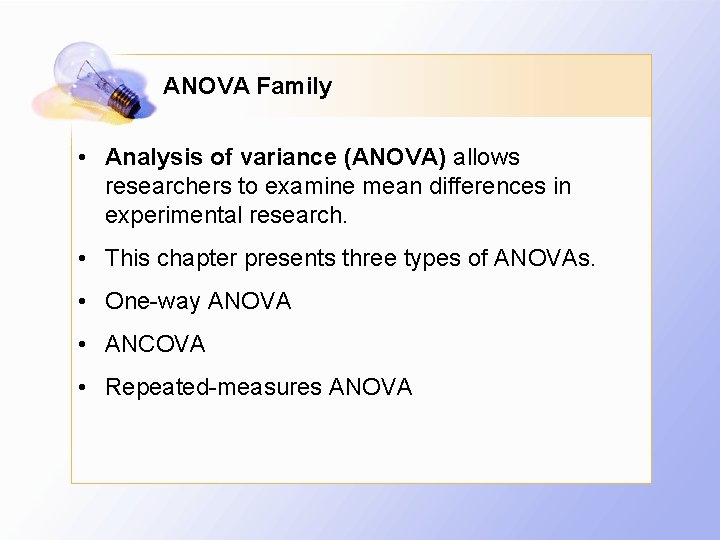
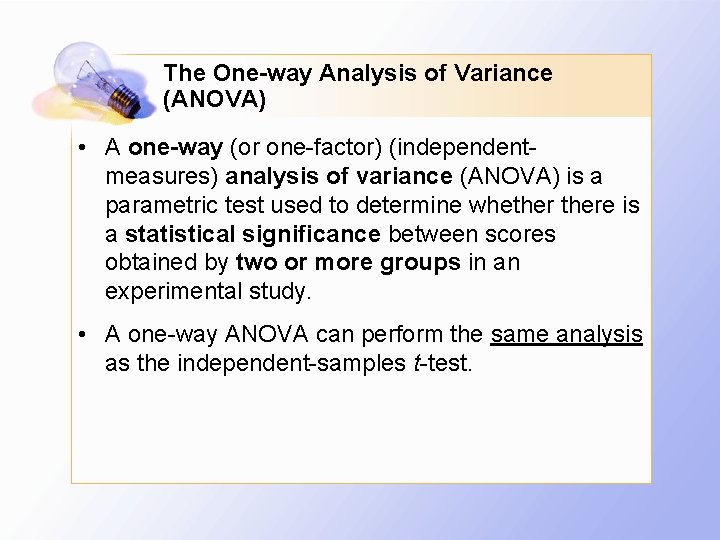
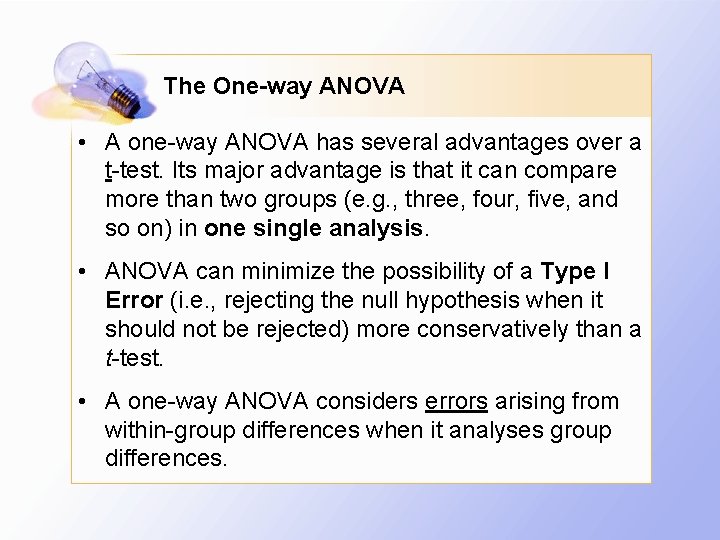
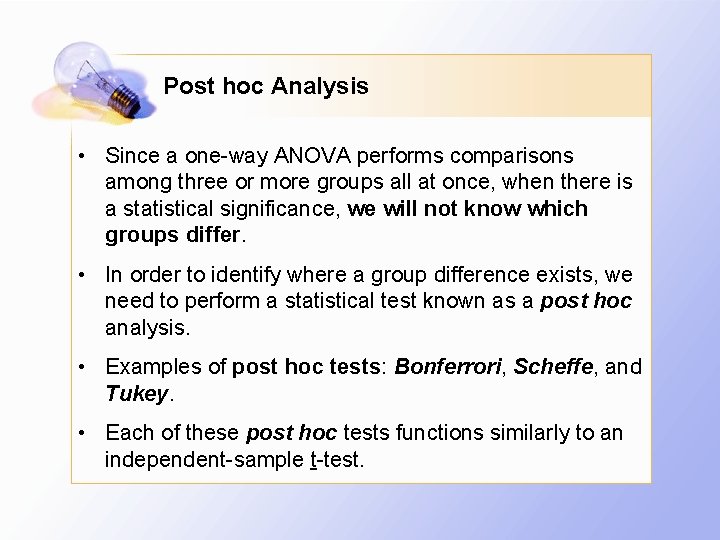
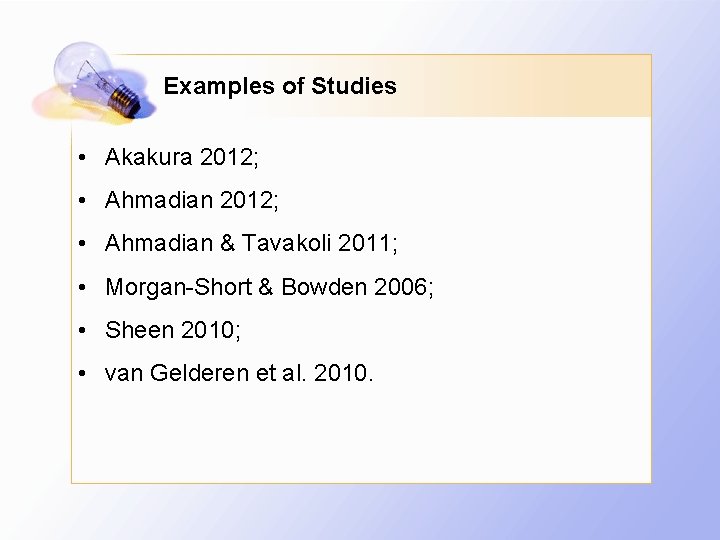
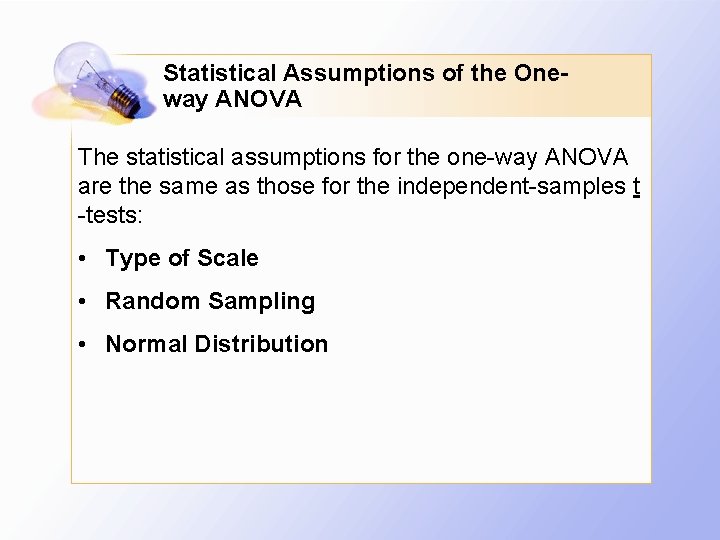
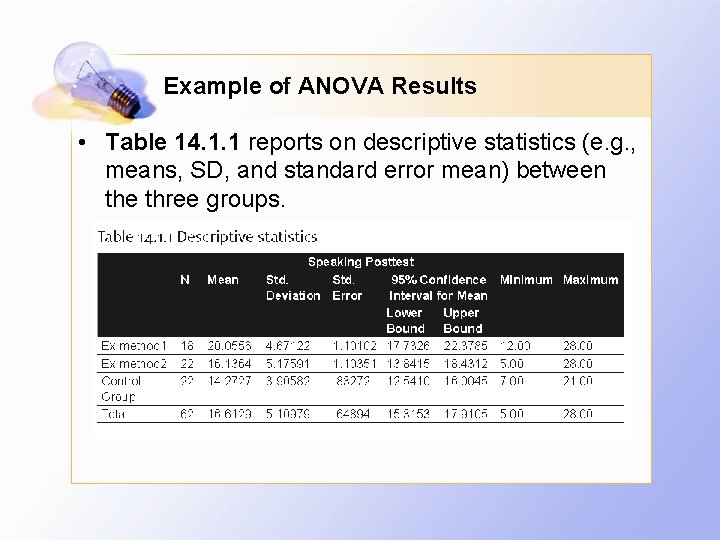
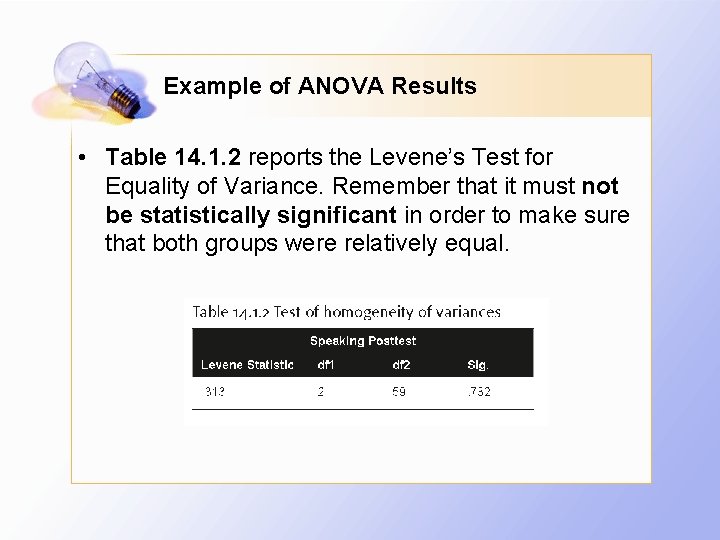
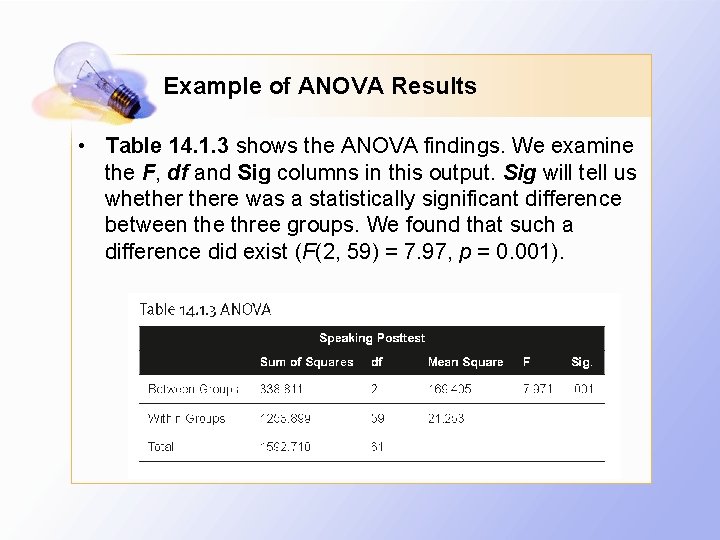
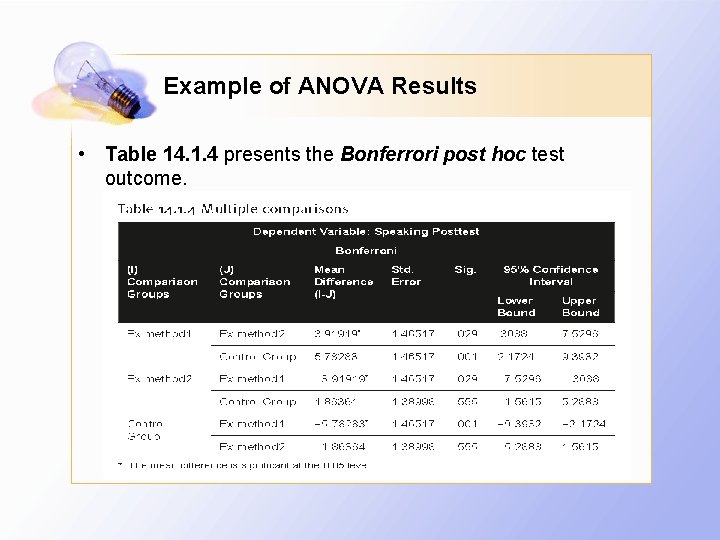
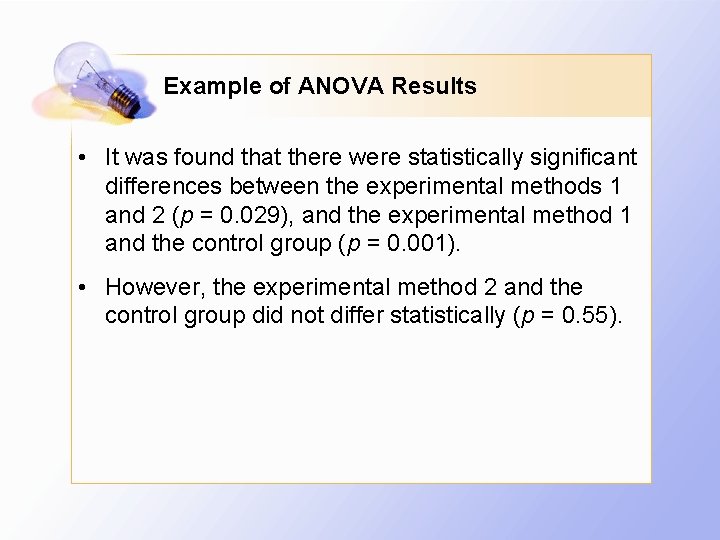
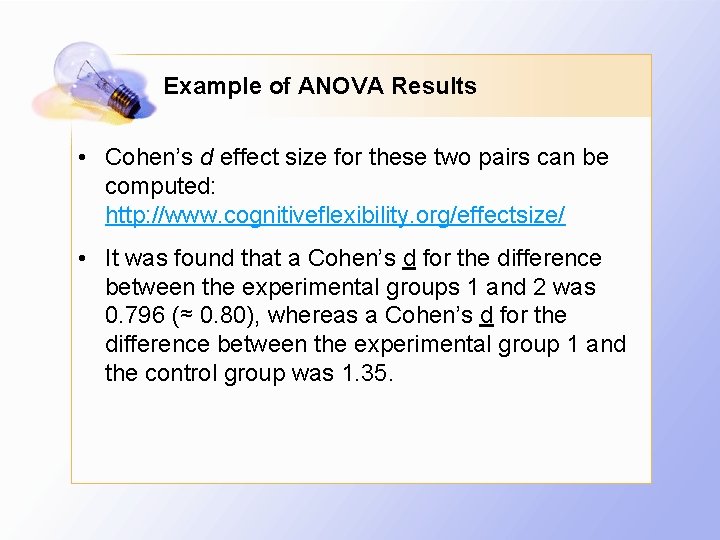
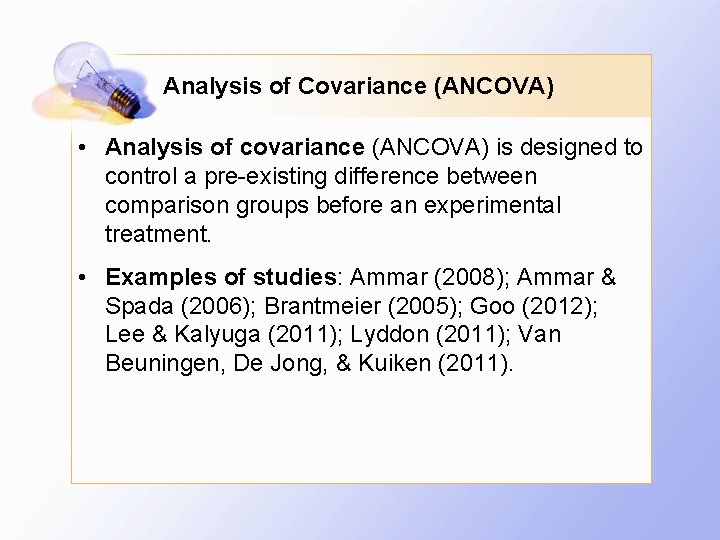
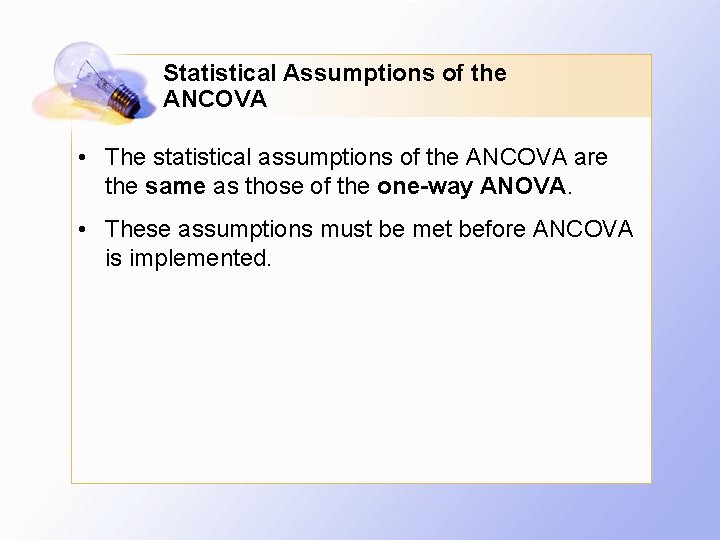
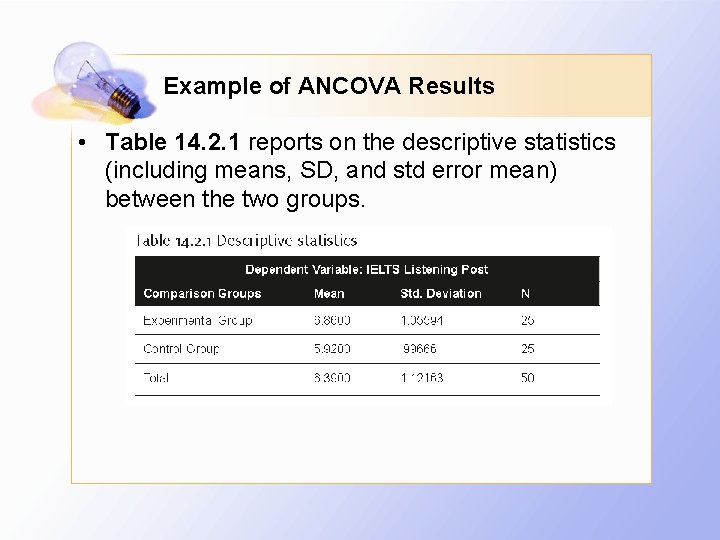
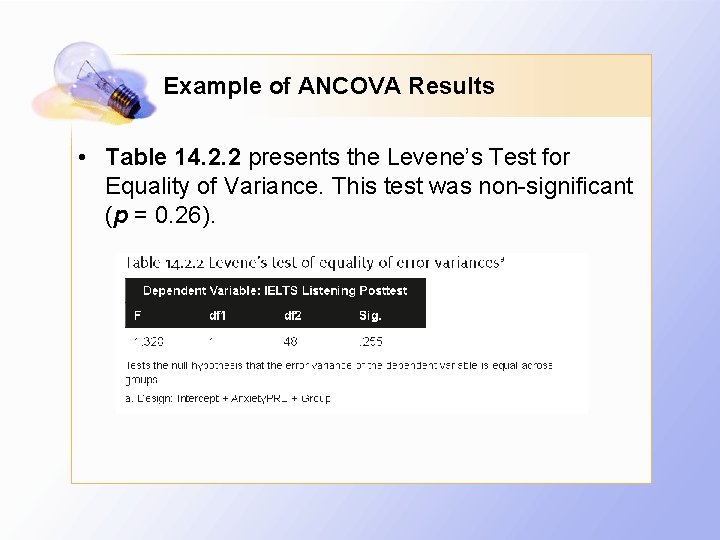
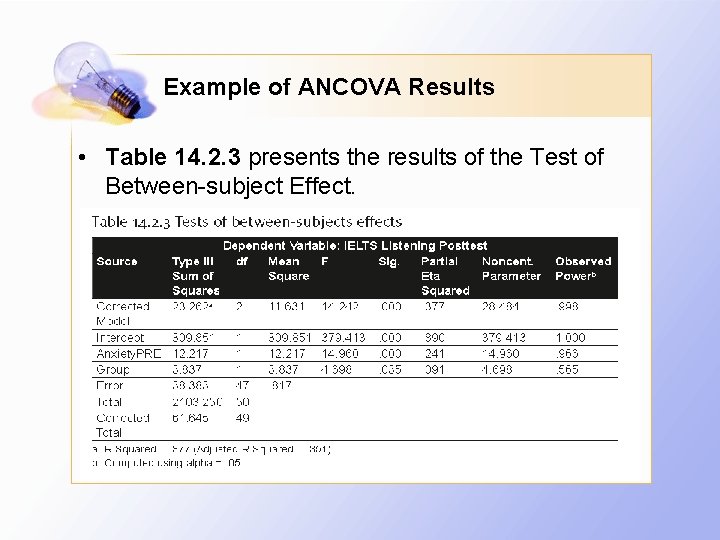
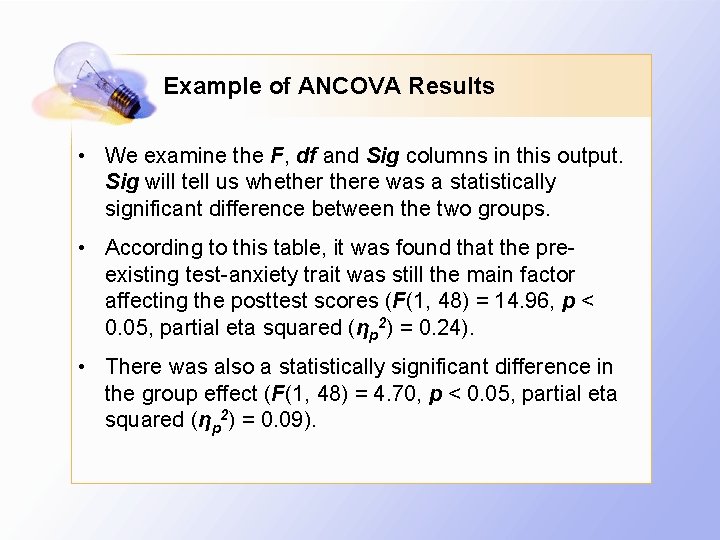
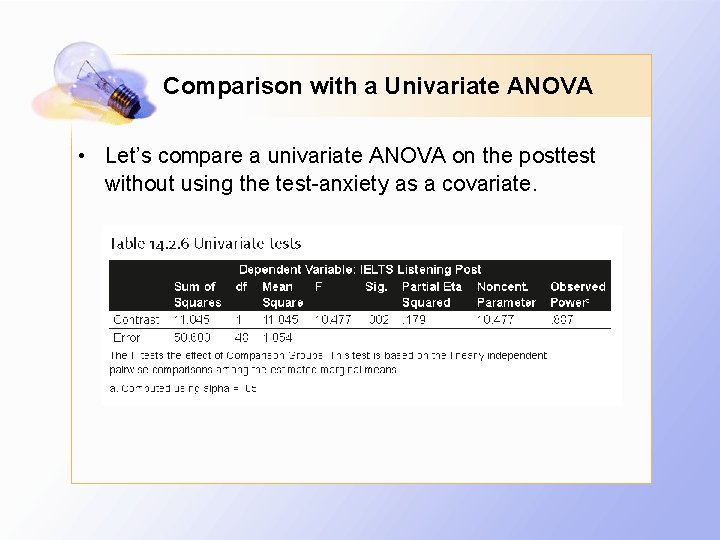
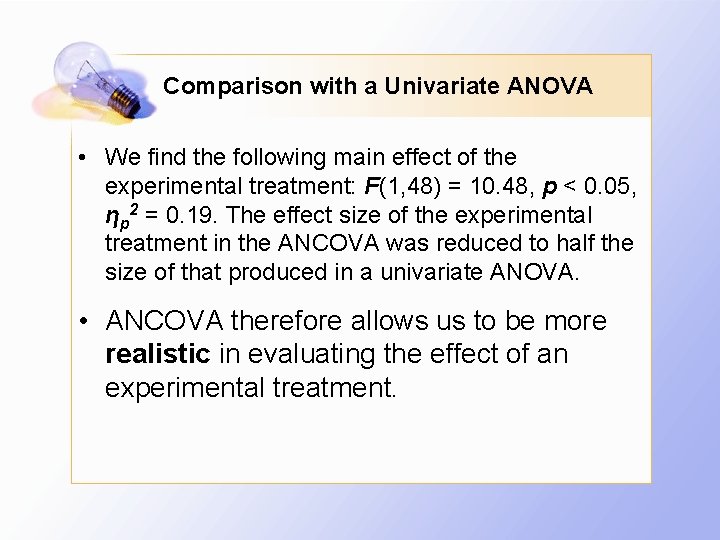
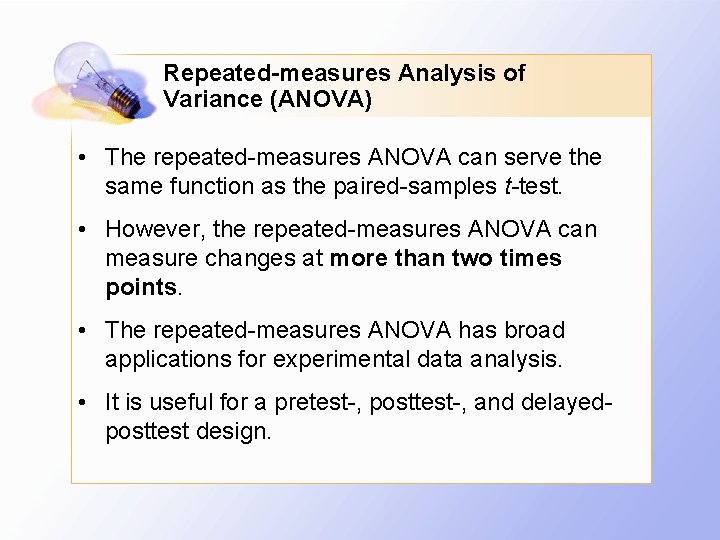
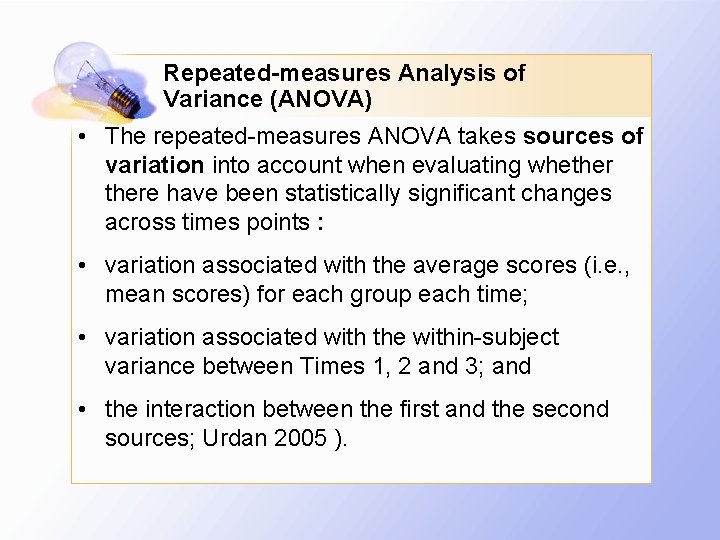
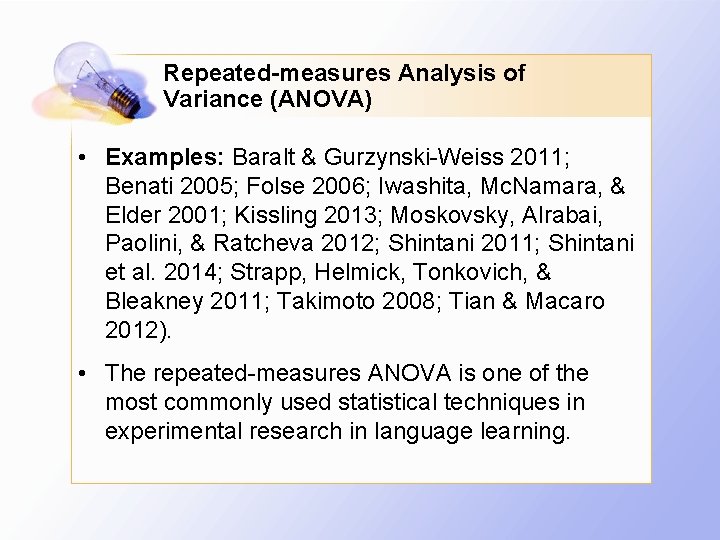
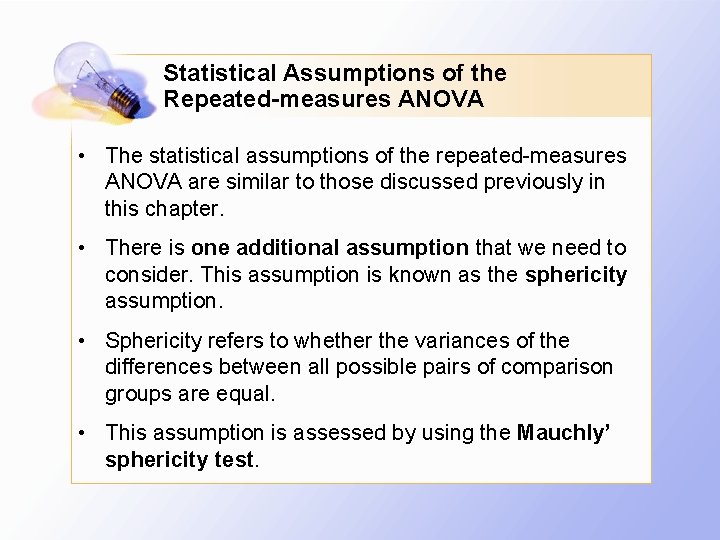
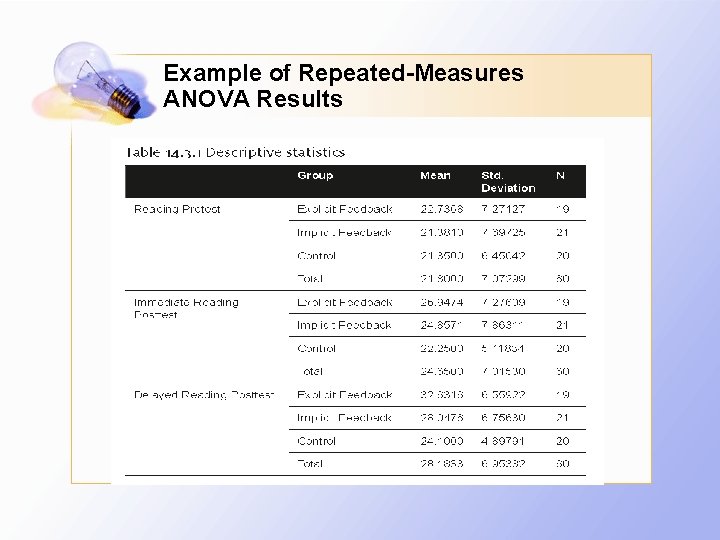
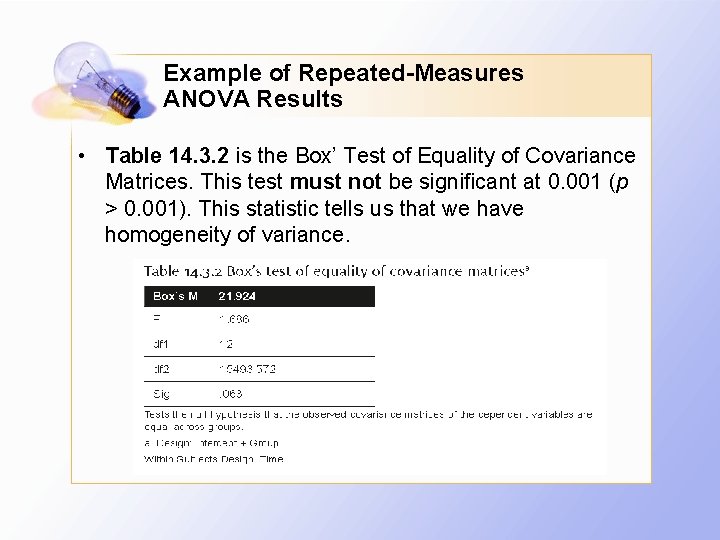
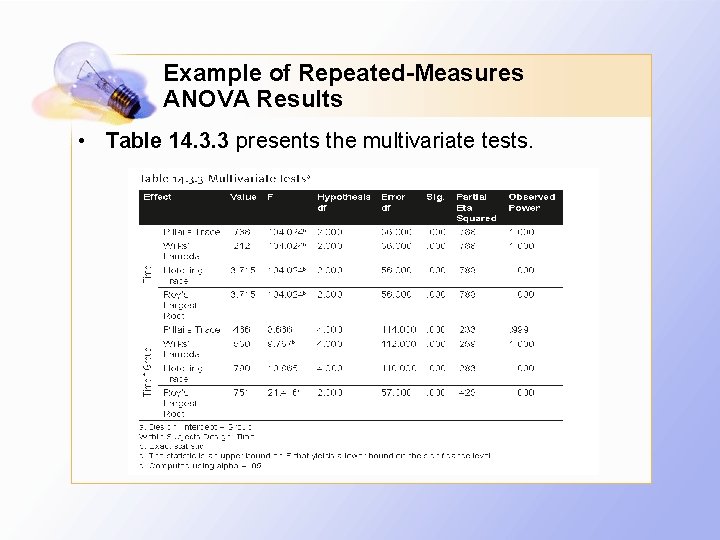
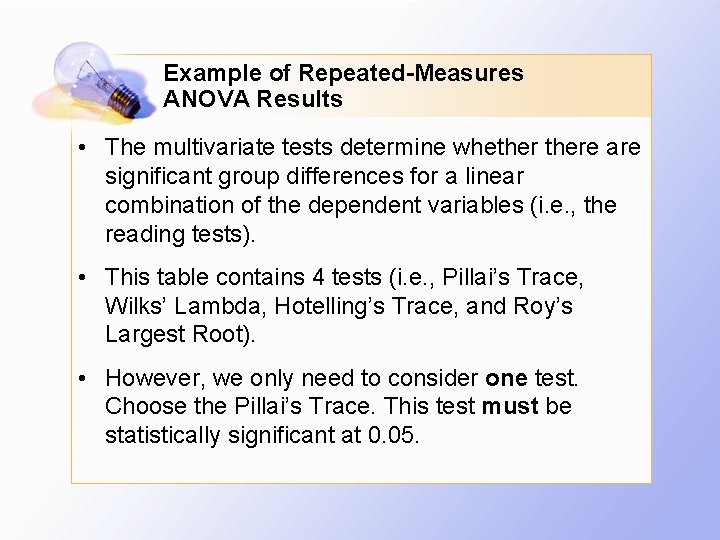
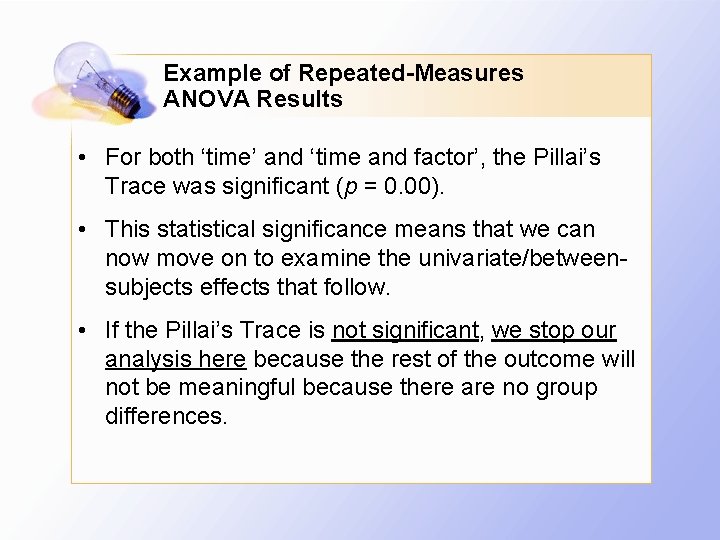
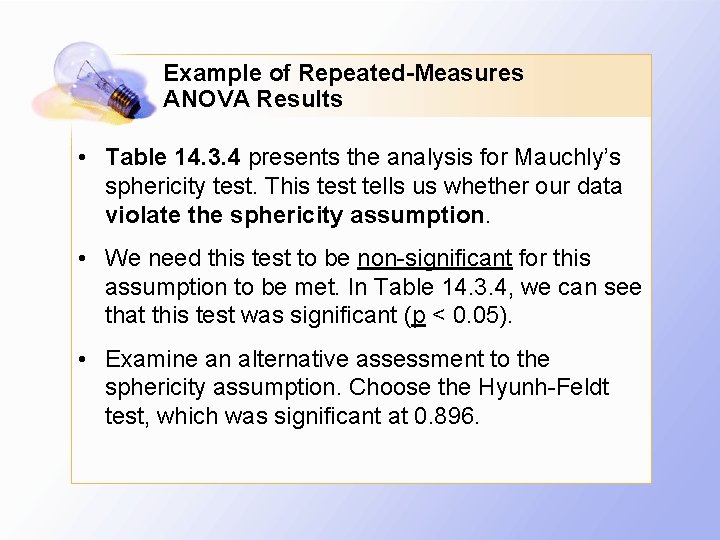
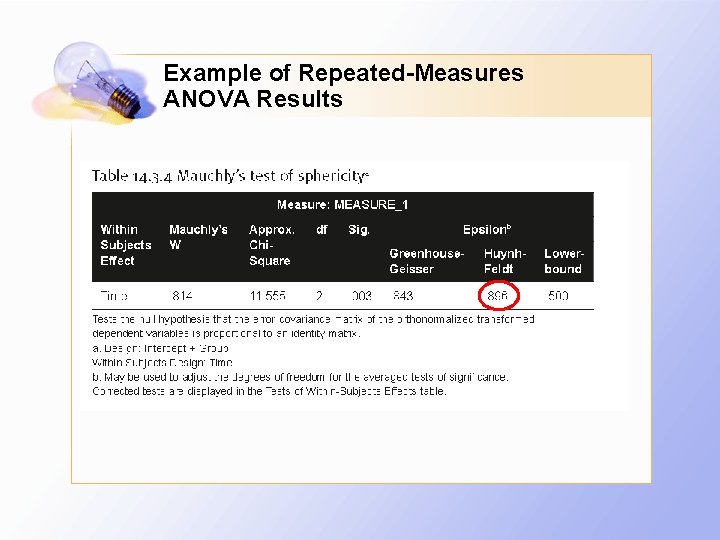
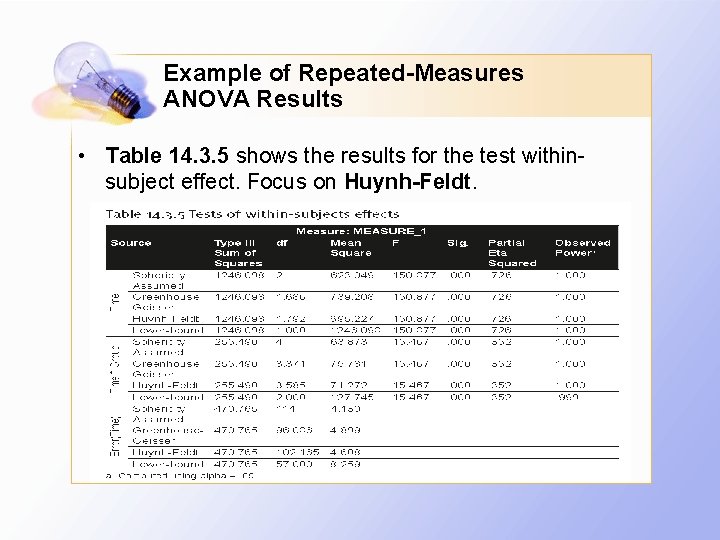
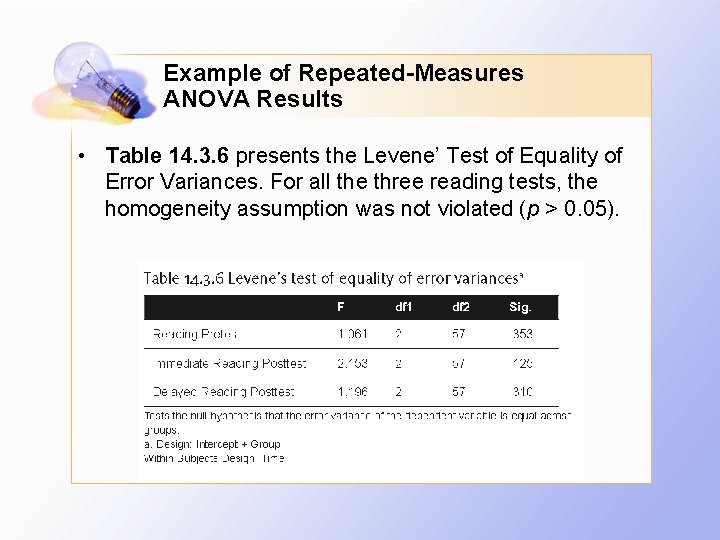
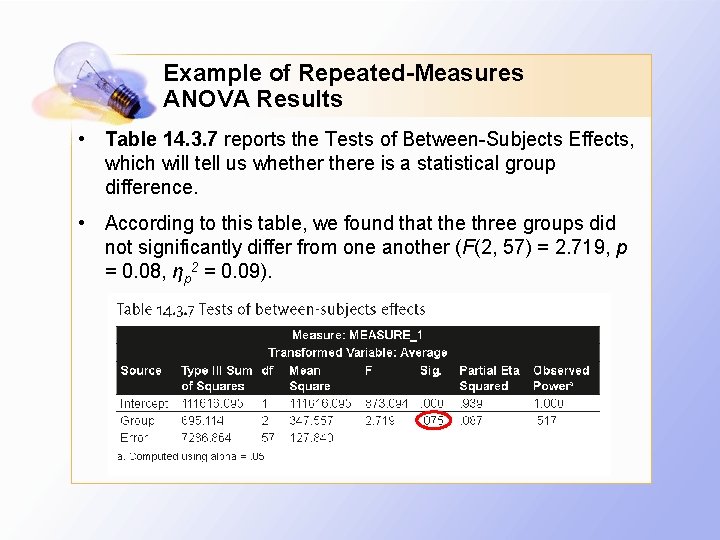
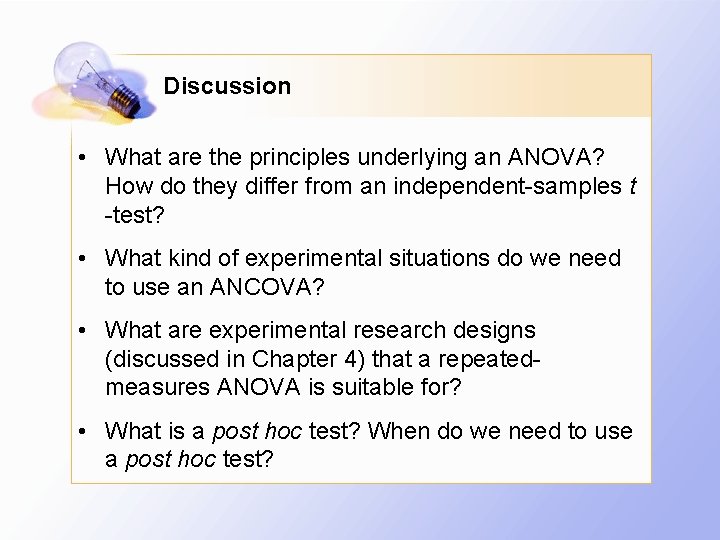
- Slides: 37
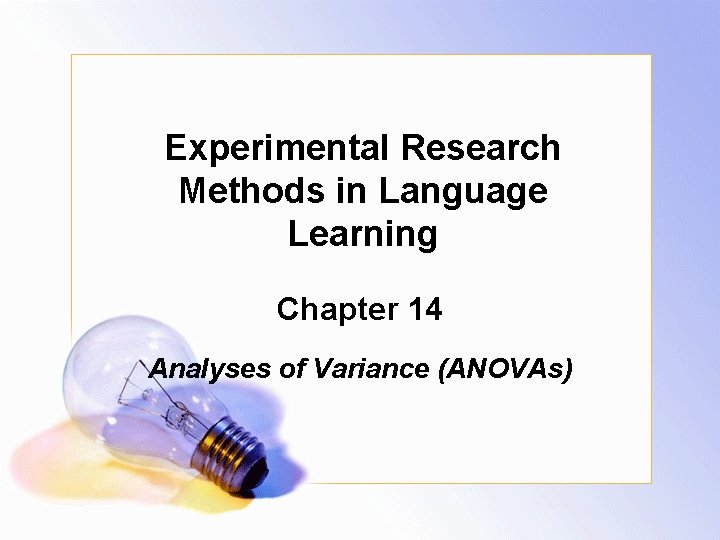
Experimental Research Methods in Language Learning Chapter 14 Analyses of Variance (ANOVAs)
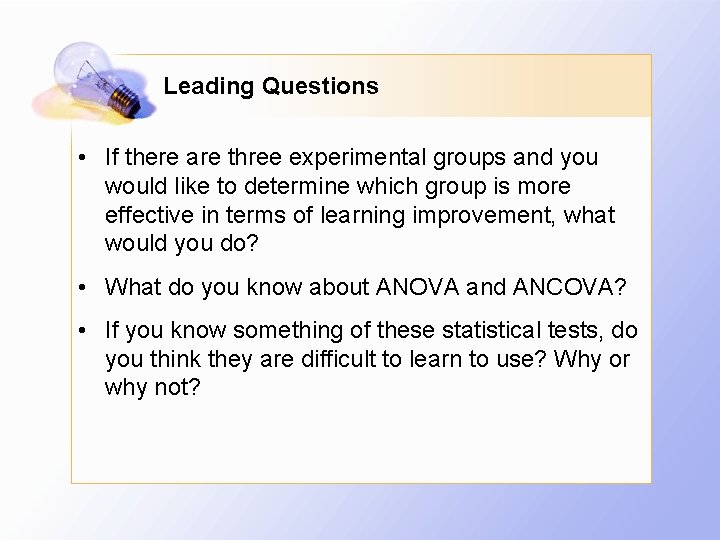
Leading Questions • If there are three experimental groups and you would like to determine which group is more effective in terms of learning improvement, what would you do? • What do you know about ANOVA and ANCOVA? • If you know something of these statistical tests, do you think they are difficult to learn to use? Why or why not?
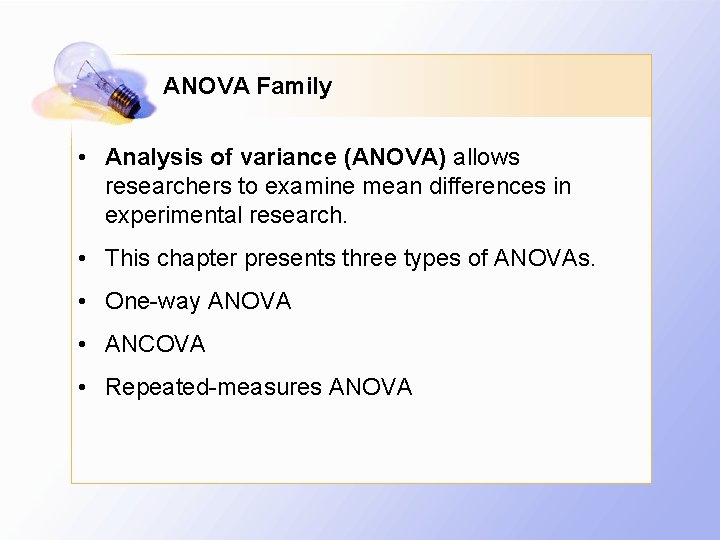
ANOVA Family • Analysis of variance (ANOVA) allows researchers to examine mean differences in experimental research. • This chapter presents three types of ANOVAs. • One-way ANOVA • ANCOVA • Repeated-measures ANOVA
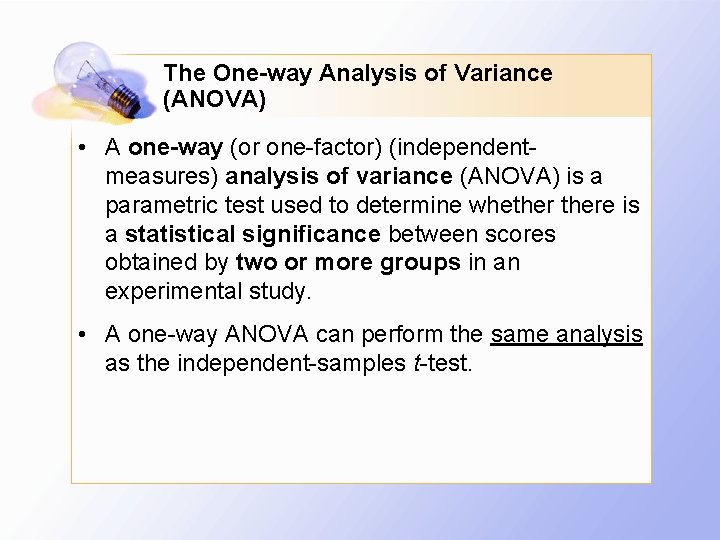
The One-way Analysis of Variance (ANOVA) • A one-way (or one-factor) (independentmeasures) analysis of variance (ANOVA) is a parametric test used to determine whethere is a statistical significance between scores obtained by two or more groups in an experimental study. • A one-way ANOVA can perform the same analysis as the independent-samples t-test.
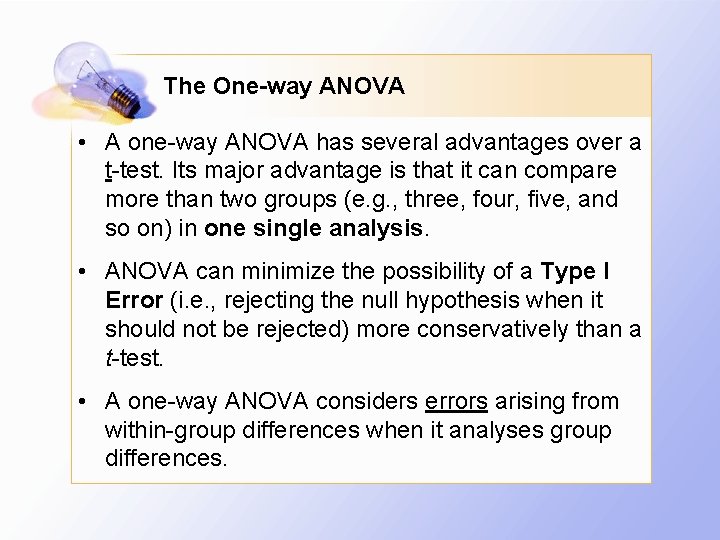
The One-way ANOVA • A one-way ANOVA has several advantages over a t-test. Its major advantage is that it can compare more than two groups (e. g. , three, four, five, and so on) in one single analysis. • ANOVA can minimize the possibility of a Type I Error (i. e. , rejecting the null hypothesis when it should not be rejected) more conservatively than a t-test. • A one-way ANOVA considers errors arising from within-group differences when it analyses group differences.
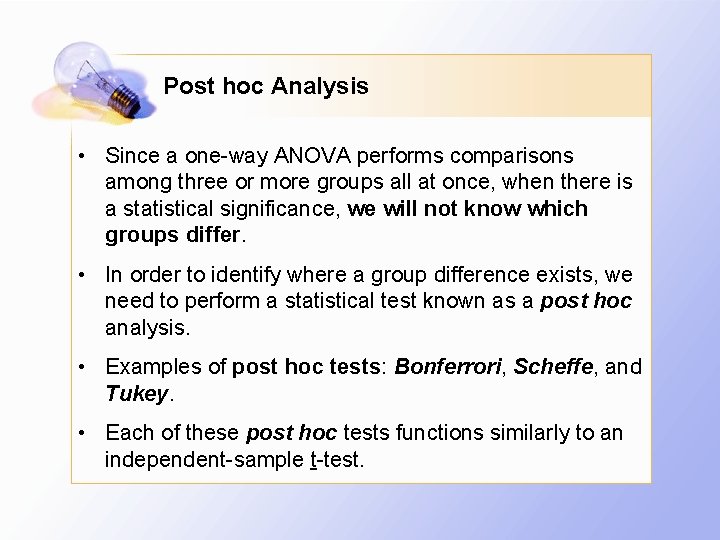
Post hoc Analysis • Since a one-way ANOVA performs comparisons among three or more groups all at once, when there is a statistical significance, we will not know which groups differ. • In order to identify where a group difference exists, we need to perform a statistical test known as a post hoc analysis. • Examples of post hoc tests: Bonferrori, Scheffe, and Tukey. • Each of these post hoc tests functions similarly to an independent-sample t-test.
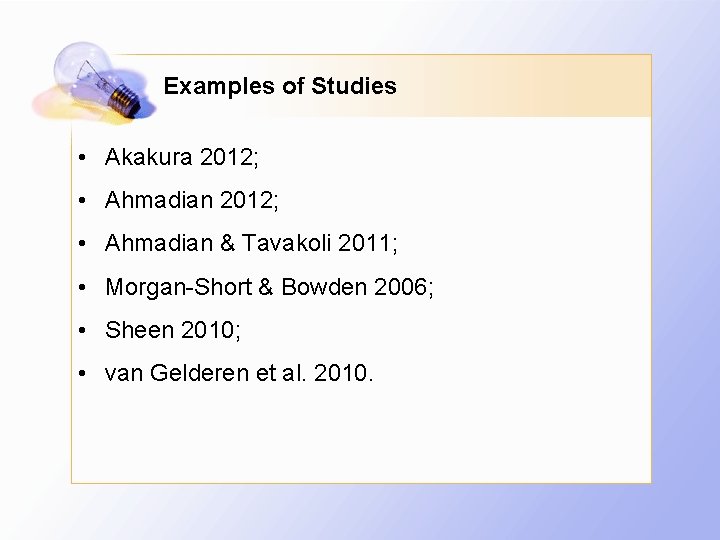
Examples of Studies • Akakura 2012; • Ahmadian & Tavakoli 2011; • Morgan-Short & Bowden 2006; • Sheen 2010; • van Gelderen et al. 2010.
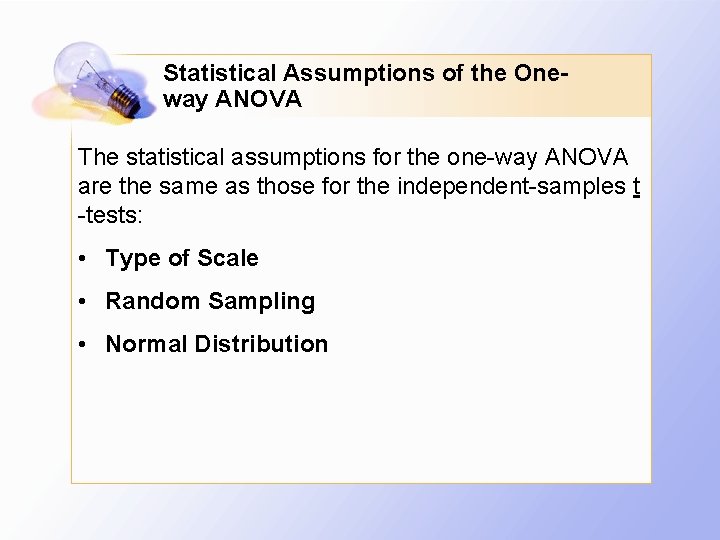
Statistical Assumptions of the Oneway ANOVA The statistical assumptions for the one-way ANOVA are the same as those for the independent-samples t -tests: • Type of Scale • Random Sampling • Normal Distribution
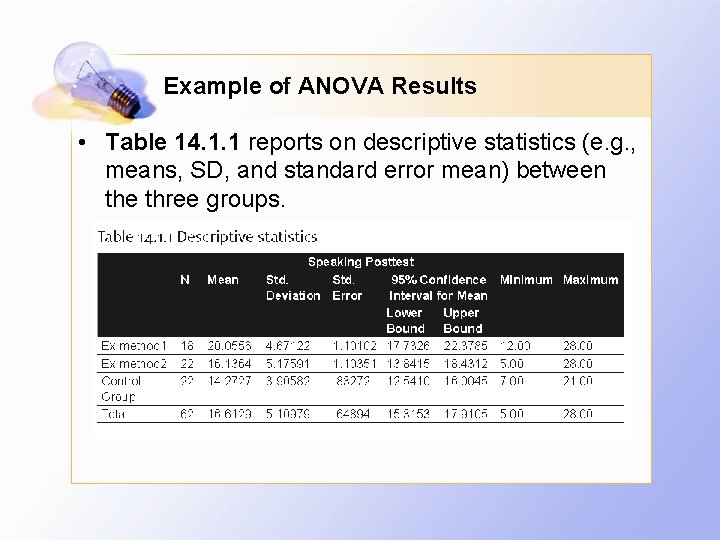
Example of ANOVA Results • Table 14. 1. 1 reports on descriptive statistics (e. g. , means, SD, and standard error mean) between the three groups.
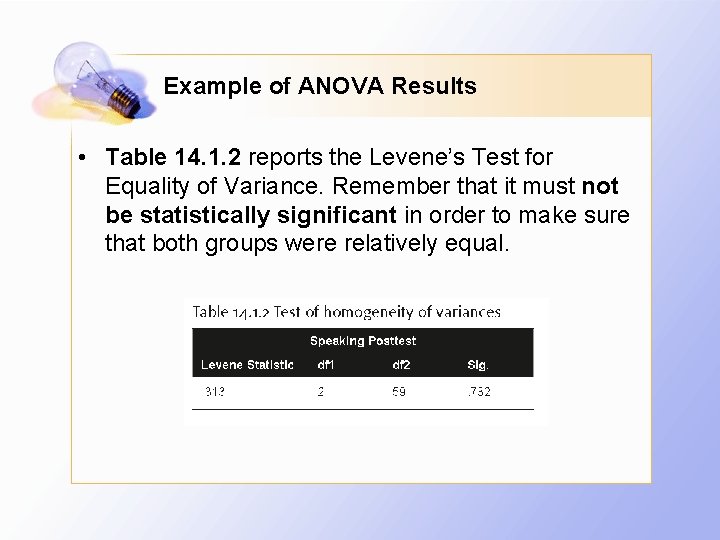
Example of ANOVA Results • Table 14. 1. 2 reports the Levene’s Test for Equality of Variance. Remember that it must not be statistically significant in order to make sure that both groups were relatively equal.
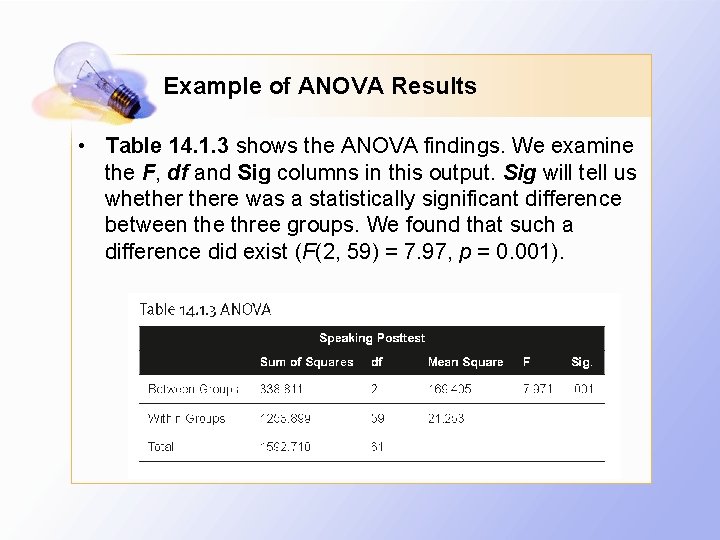
Example of ANOVA Results • Table 14. 1. 3 shows the ANOVA findings. We examine the F, df and Sig columns in this output. Sig will tell us whethere was a statistically significant difference between the three groups. We found that such a difference did exist (F(2, 59) = 7. 97, p = 0. 001).
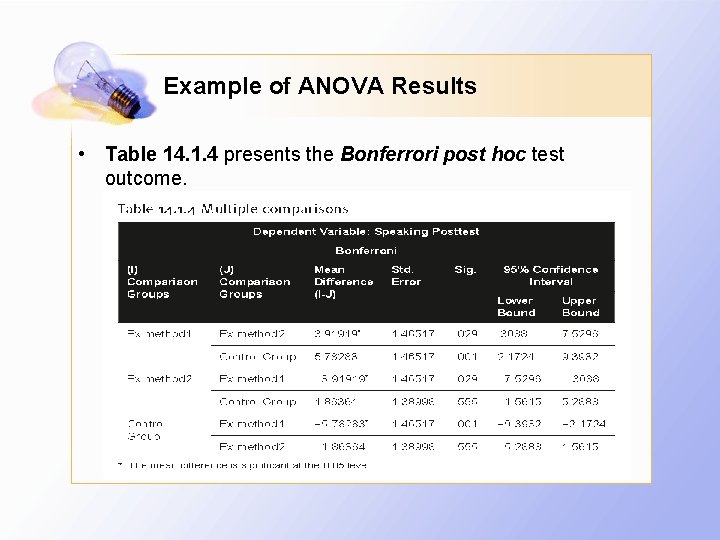
Example of ANOVA Results • Table 14. 1. 4 presents the Bonferrori post hoc test outcome.
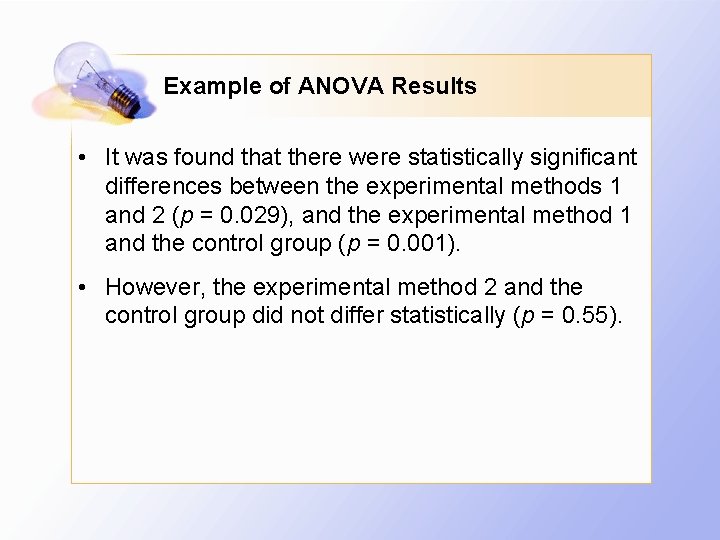
Example of ANOVA Results • It was found that there were statistically significant differences between the experimental methods 1 and 2 (p = 0. 029), and the experimental method 1 and the control group (p = 0. 001). • However, the experimental method 2 and the control group did not differ statistically (p = 0. 55).
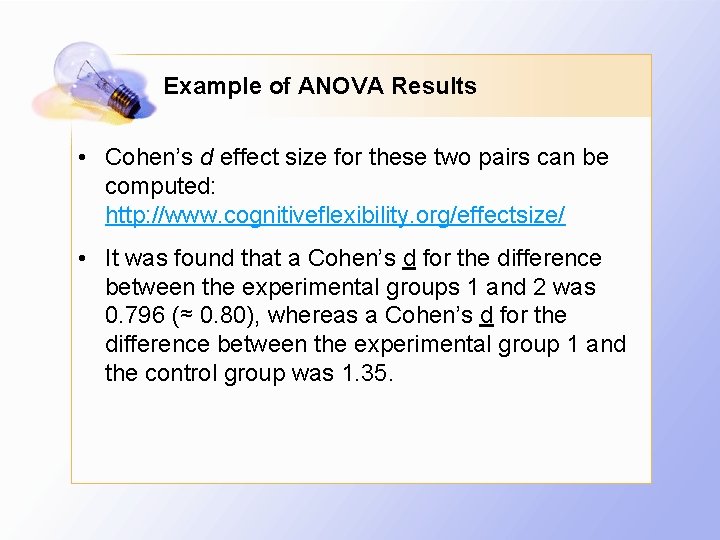
Example of ANOVA Results • Cohen’s d effect size for these two pairs can be computed: http: //www. cognitiveflexibility. org/effectsize/ • It was found that a Cohen’s d for the difference between the experimental groups 1 and 2 was 0. 796 (≈ 0. 80), whereas a Cohen’s d for the difference between the experimental group 1 and the control group was 1. 35.
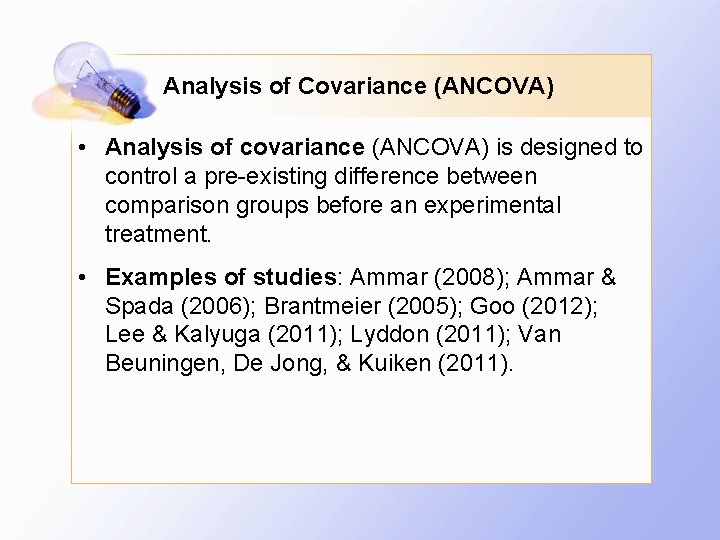
Analysis of Covariance (ANCOVA) • Analysis of covariance (ANCOVA) is designed to control a pre-existing difference between comparison groups before an experimental treatment. • Examples of studies: Ammar (2008); Ammar & Spada (2006); Brantmeier (2005); Goo (2012); Lee & Kalyuga (2011); Lyddon (2011); Van Beuningen, De Jong, & Kuiken (2011).
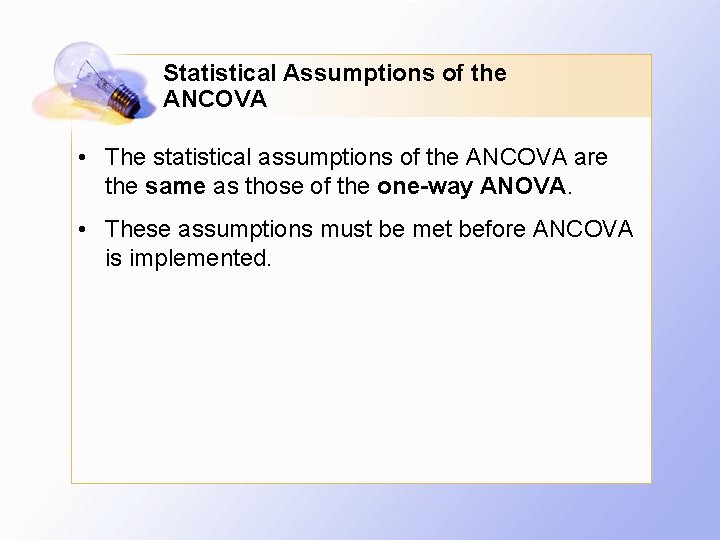
Statistical Assumptions of the ANCOVA • The statistical assumptions of the ANCOVA are the same as those of the one-way ANOVA. • These assumptions must be met before ANCOVA is implemented.
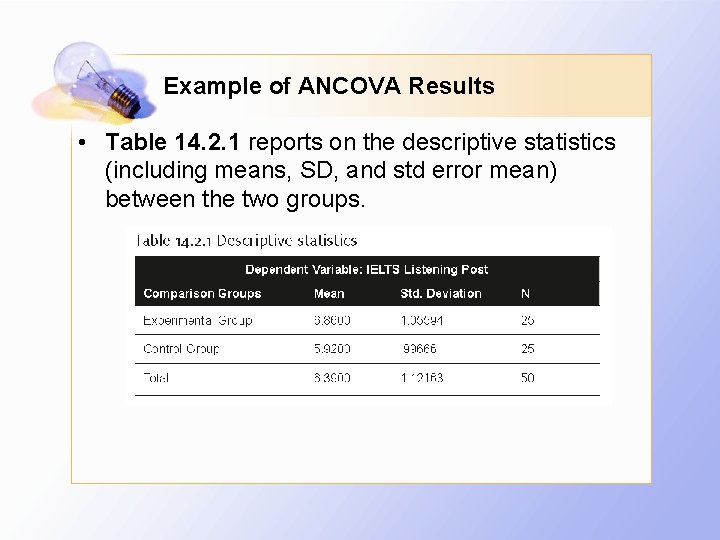
Example of ANCOVA Results • Table 14. 2. 1 reports on the descriptive statistics (including means, SD, and std error mean) between the two groups.
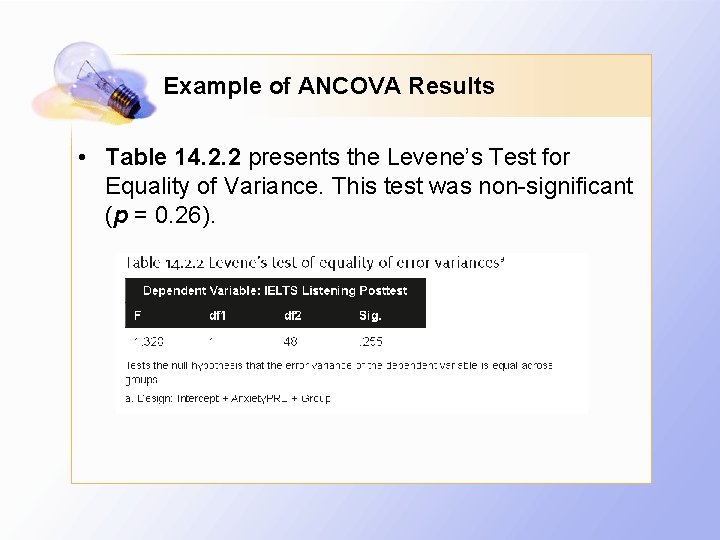
Example of ANCOVA Results • Table 14. 2. 2 presents the Levene’s Test for Equality of Variance. This test was non-significant (p = 0. 26).
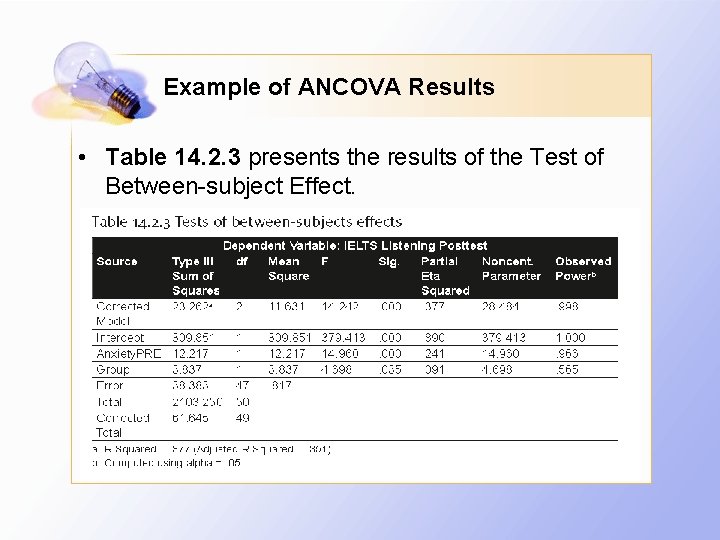
Example of ANCOVA Results • Table 14. 2. 3 presents the results of the Test of Between-subject Effect.
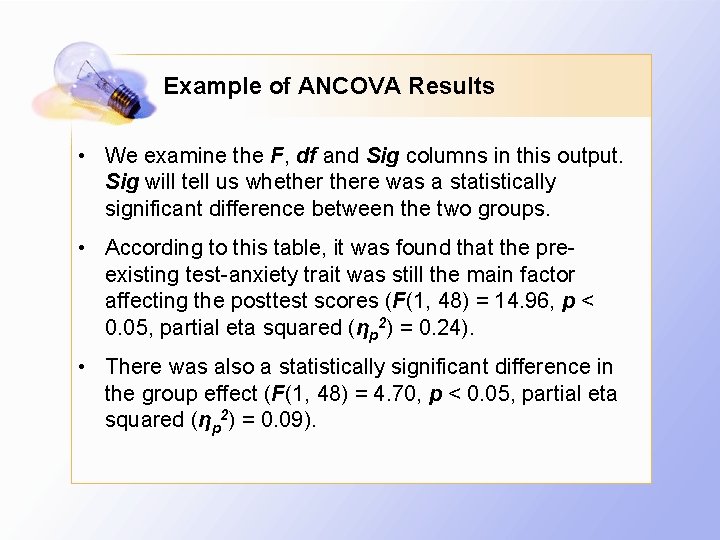
Example of ANCOVA Results • We examine the F, df and Sig columns in this output. Sig will tell us whethere was a statistically significant difference between the two groups. • According to this table, it was found that the preexisting test-anxiety trait was still the main factor affecting the posttest scores (F(1, 48) = 14. 96, p < 0. 05, partial eta squared (ηp 2) = 0. 24). • There was also a statistically significant difference in the group effect (F(1, 48) = 4. 70, p < 0. 05, partial eta squared (ηp 2) = 0. 09).
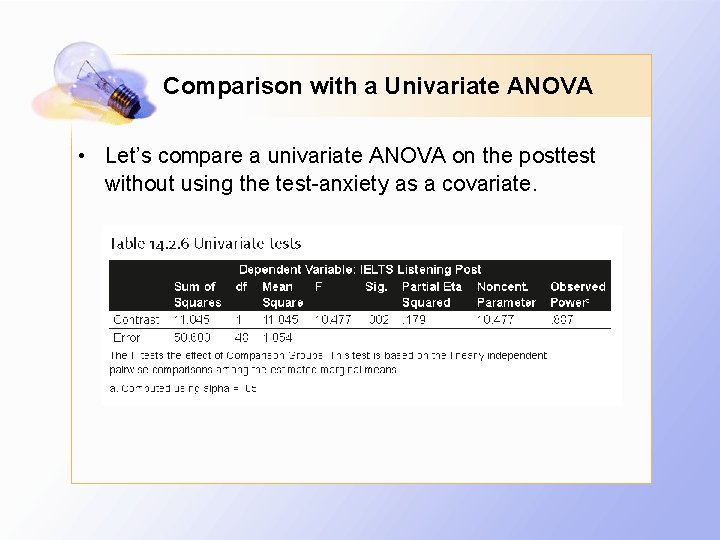
Comparison with a Univariate ANOVA • Let’s compare a univariate ANOVA on the posttest without using the test-anxiety as a covariate.
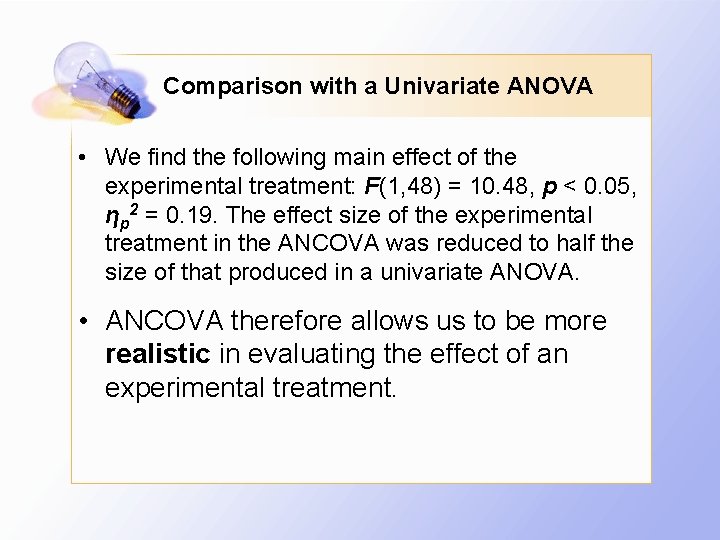
Comparison with a Univariate ANOVA • We find the following main effect of the experimental treatment: F(1, 48) = 10. 48, p < 0. 05, ηp 2 = 0. 19. The effect size of the experimental treatment in the ANCOVA was reduced to half the size of that produced in a univariate ANOVA. • ANCOVA therefore allows us to be more realistic in evaluating the effect of an experimental treatment.
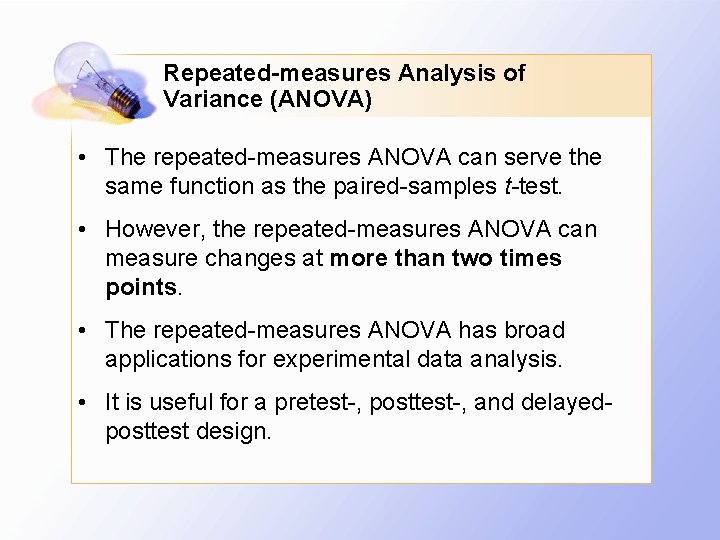
Repeated-measures Analysis of Variance (ANOVA) • The repeated-measures ANOVA can serve the same function as the paired-samples t-test. • However, the repeated-measures ANOVA can measure changes at more than two times points. • The repeated-measures ANOVA has broad applications for experimental data analysis. • It is useful for a pretest-, posttest-, and delayedposttest design.
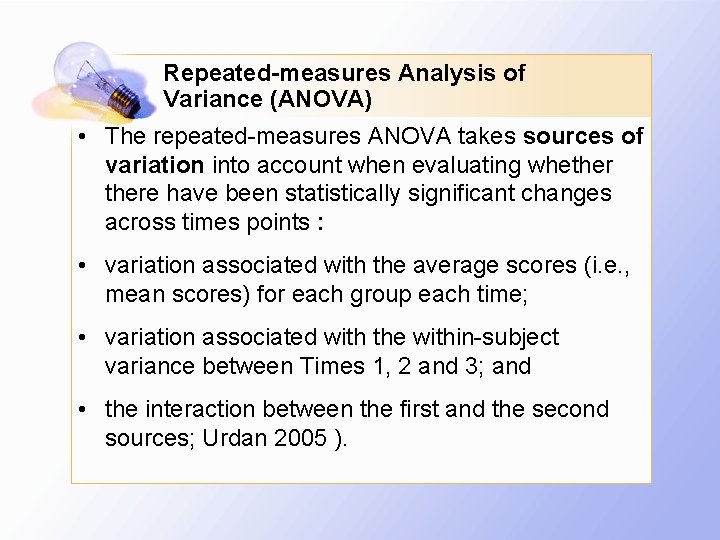
Repeated-measures Analysis of Variance (ANOVA) • The repeated-measures ANOVA takes sources of variation into account when evaluating whethere have been statistically significant changes across times points : • variation associated with the average scores (i. e. , mean scores) for each group each time; • variation associated with the within-subject variance between Times 1, 2 and 3; and • the interaction between the first and the second sources; Urdan 2005 ).
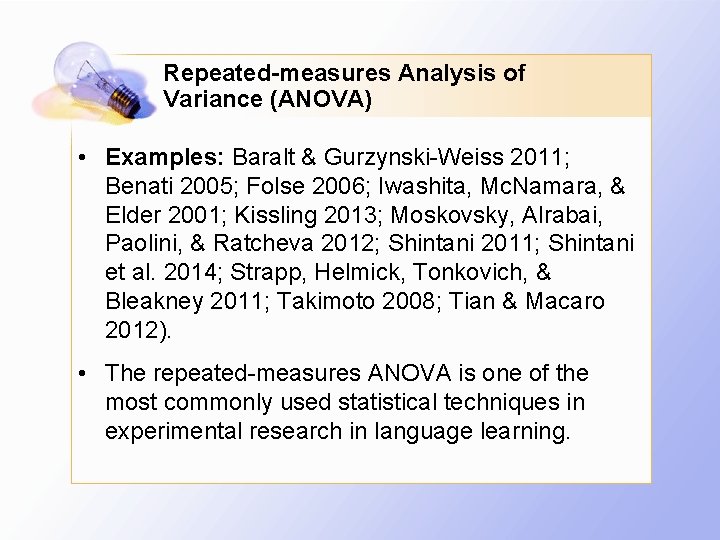
Repeated-measures Analysis of Variance (ANOVA) • Examples: Baralt & Gurzynski-Weiss 2011; Benati 2005; Folse 2006; Iwashita, Mc. Namara, & Elder 2001; Kissling 2013; Moskovsky, Alrabai, Paolini, & Ratcheva 2012; Shintani 2011; Shintani et al. 2014; Strapp, Helmick, Tonkovich, & Bleakney 2011; Takimoto 2008; Tian & Macaro 2012). • The repeated-measures ANOVA is one of the most commonly used statistical techniques in experimental research in language learning.
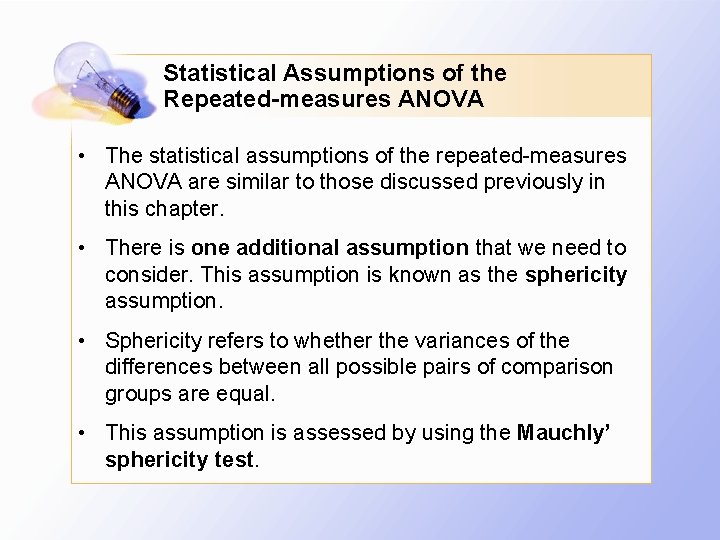
Statistical Assumptions of the Repeated-measures ANOVA • The statistical assumptions of the repeated-measures ANOVA are similar to those discussed previously in this chapter. • There is one additional assumption that we need to consider. This assumption is known as the sphericity assumption. • Sphericity refers to whether the variances of the differences between all possible pairs of comparison groups are equal. • This assumption is assessed by using the Mauchly’ sphericity test.
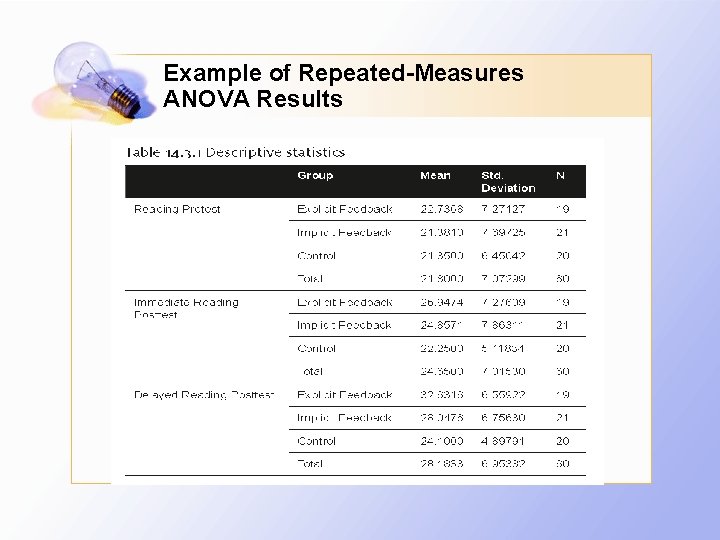
Example of Repeated-Measures ANOVA Results
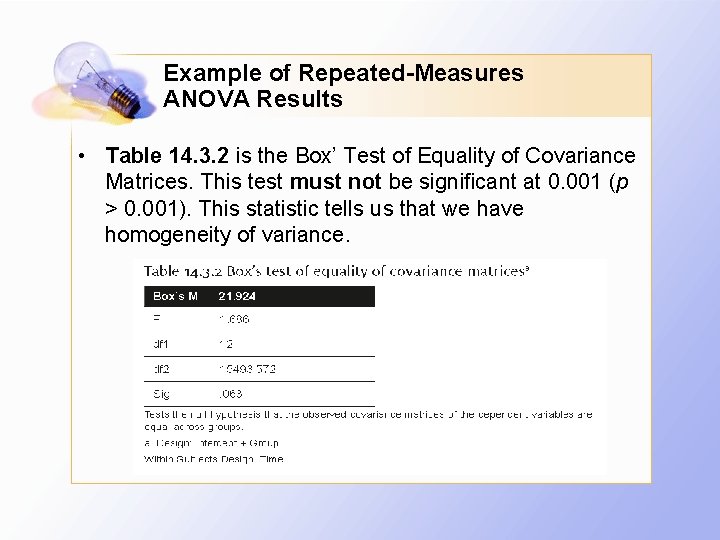
Example of Repeated-Measures ANOVA Results • Table 14. 3. 2 is the Box’ Test of Equality of Covariance Matrices. This test must not be significant at 0. 001 (p > 0. 001). This statistic tells us that we have homogeneity of variance.
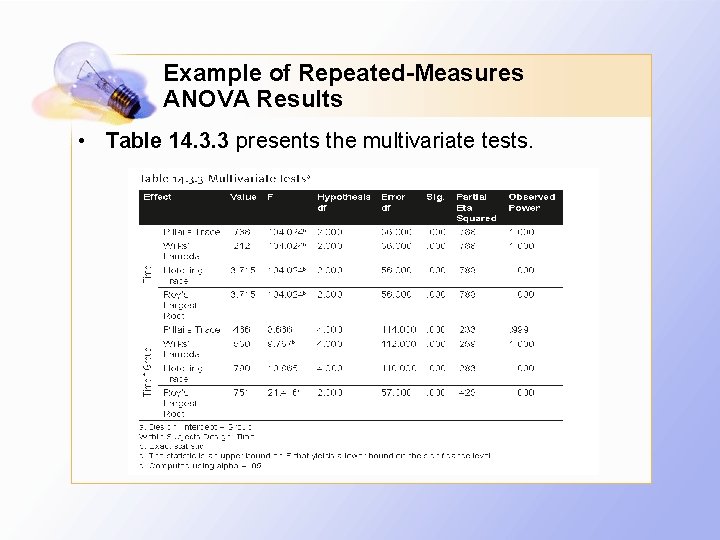
Example of Repeated-Measures ANOVA Results • Table 14. 3. 3 presents the multivariate tests.
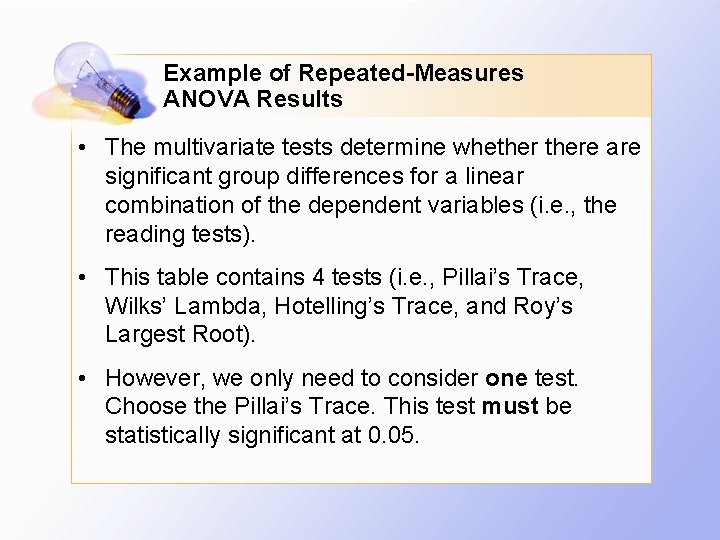
Example of Repeated-Measures ANOVA Results • The multivariate tests determine whethere are significant group differences for a linear combination of the dependent variables (i. e. , the reading tests). • This table contains 4 tests (i. e. , Pillai’s Trace, Wilks’ Lambda, Hotelling’s Trace, and Roy’s Largest Root). • However, we only need to consider one test. Choose the Pillai’s Trace. This test must be statistically significant at 0. 05.
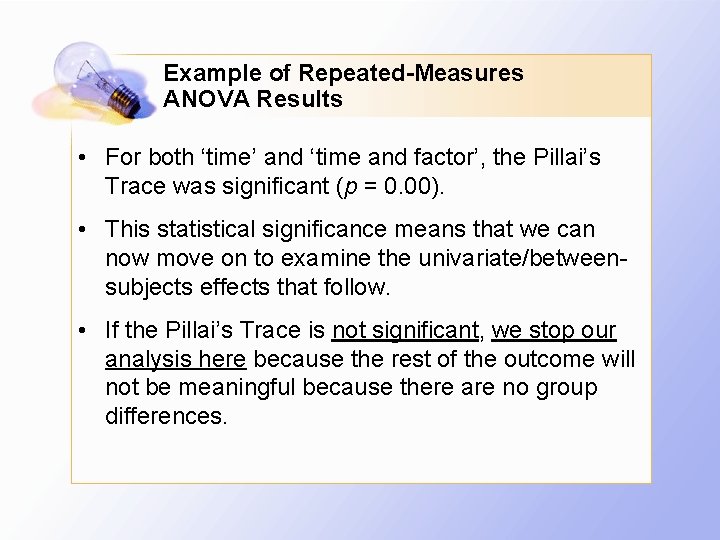
Example of Repeated-Measures ANOVA Results • For both ‘time’ and ‘time and factor’, the Pillai’s Trace was significant (p = 0. 00). • This statistical significance means that we can now move on to examine the univariate/betweensubjects effects that follow. • If the Pillai’s Trace is not significant, we stop our analysis here because the rest of the outcome will not be meaningful because there are no group differences.
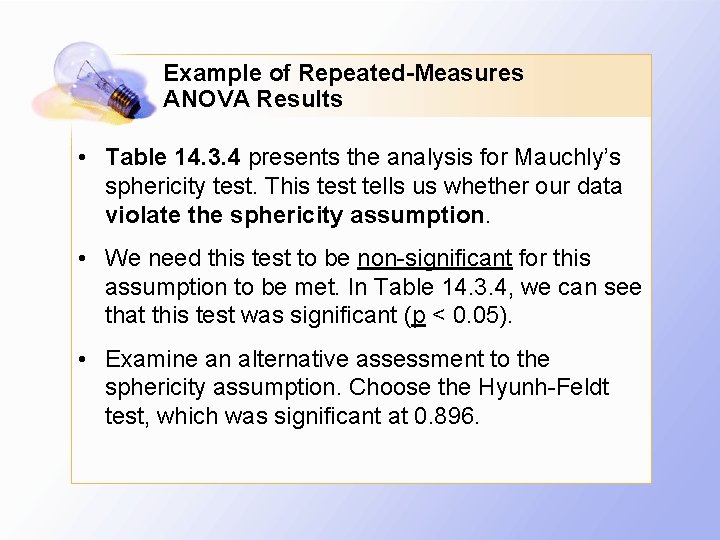
Example of Repeated-Measures ANOVA Results • Table 14. 3. 4 presents the analysis for Mauchly’s sphericity test. This test tells us whether our data violate the sphericity assumption. • We need this test to be non-significant for this assumption to be met. In Table 14. 3. 4, we can see that this test was significant (p < 0. 05). • Examine an alternative assessment to the sphericity assumption. Choose the Hyunh-Feldt test, which was significant at 0. 896.
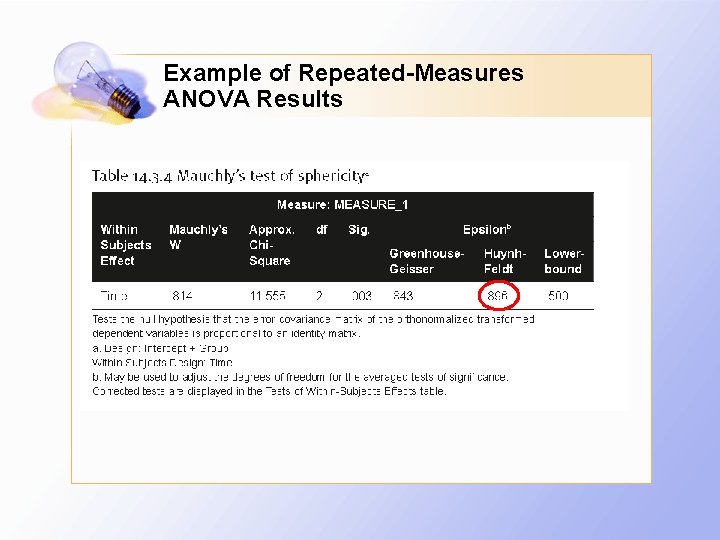
Example of Repeated-Measures ANOVA Results
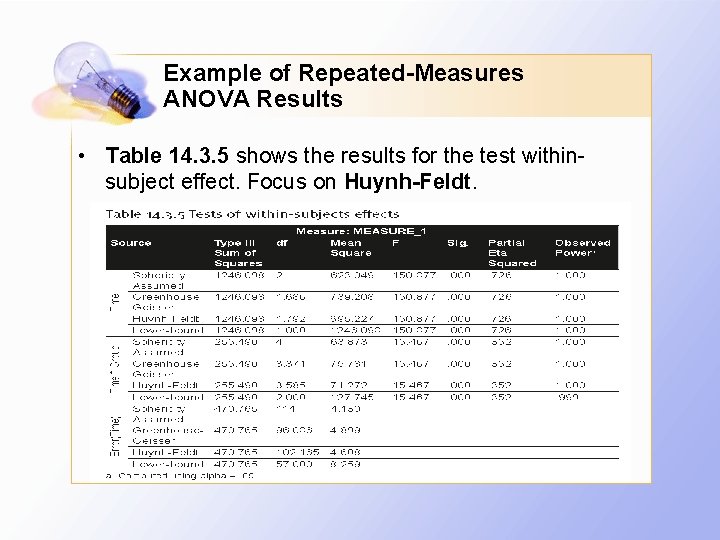
Example of Repeated-Measures ANOVA Results • Table 14. 3. 5 shows the results for the test withinsubject effect. Focus on Huynh-Feldt.
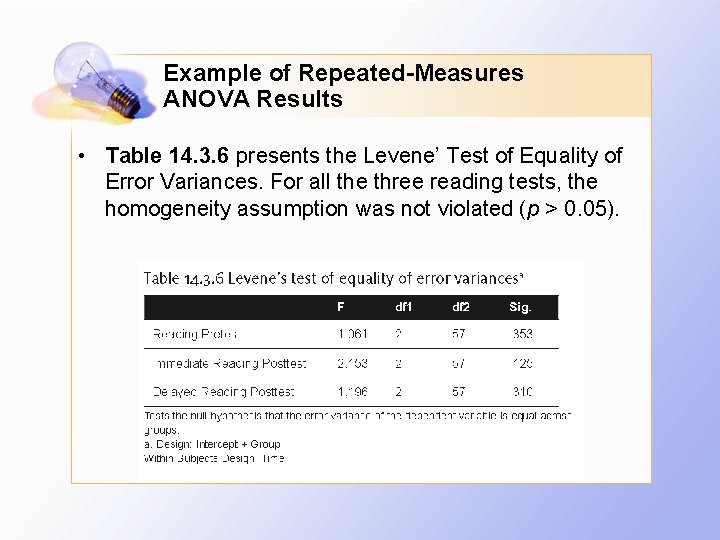
Example of Repeated-Measures ANOVA Results • Table 14. 3. 6 presents the Levene’ Test of Equality of Error Variances. For all the three reading tests, the homogeneity assumption was not violated (p > 0. 05).
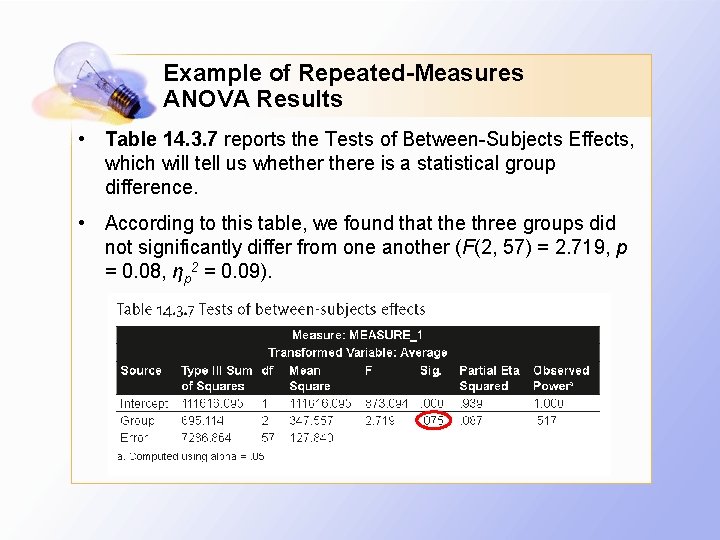
Example of Repeated-Measures ANOVA Results • Table 14. 3. 7 reports the Tests of Between-Subjects Effects, which will tell us whethere is a statistical group difference. • According to this table, we found that the three groups did not significantly differ from one another (F(2, 57) = 2. 719, p = 0. 08, ηp 2 = 0. 09).
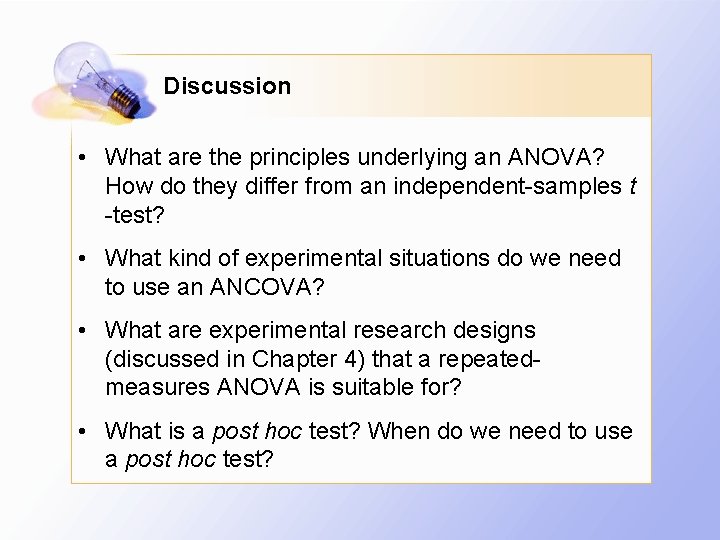
Discussion • What are the principles underlying an ANOVA? How do they differ from an independent-samples t -test? • What kind of experimental situations do we need to use an ANCOVA? • What are experimental research designs (discussed in Chapter 4) that a repeatedmeasures ANOVA is suitable for? • What is a post hoc test? When do we need to use a post hoc test?
Disadvantages of experimental research
Research instrument in experimental research
Research instrument in experimental research
Experimental vs non experimental
Univariate descriptive design
Non experimental design vs experimental
Experimental vs non experimental
What is basic research
Chapter 4 experimental research
Research methods for business students chapter 5
Cuadro comparativo e-learning y b-learning
Wax pattern in dentistry
Crossover design slideshare
Example of experiment research
Characteristics of experimental research design
Experimental research report
Contoh variabel sekunder
Non experimental research correlational design
Experimental research design
Descriptive vs correlational vs experimental research
Cohort meaning in research
Characteristics of experimental research design
Steps in experimental research
Matching by holding variables constant
2x3 within subjects design
Experimental psychology research topics
Non experimental quantitative research
Extraneous variable example
Ethical considerations in experimental research
Correlation vs comparative
Experimental research outline
Types of quantitative research designs
Quasi experimental
Cross-sectional correlational design
Experimental research outline
Field experiment example
Quasi-experimental research designs
Quasi-experimental research designs