EMR 6550 Experimental and Quasi Experimental Designs Dr
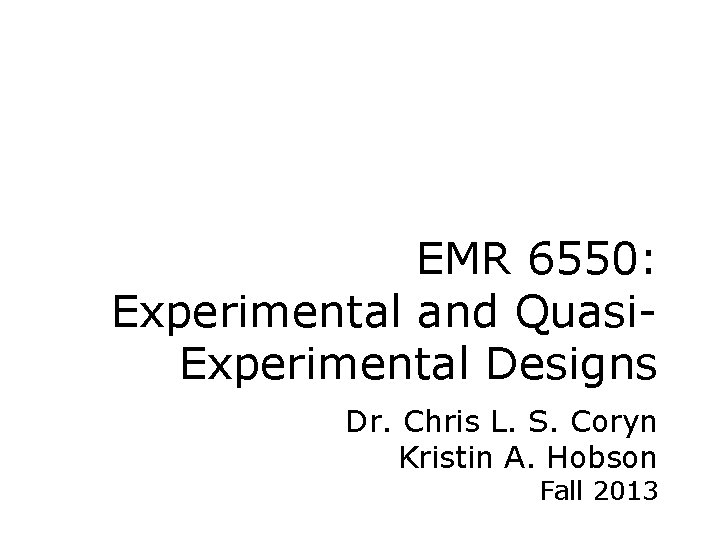
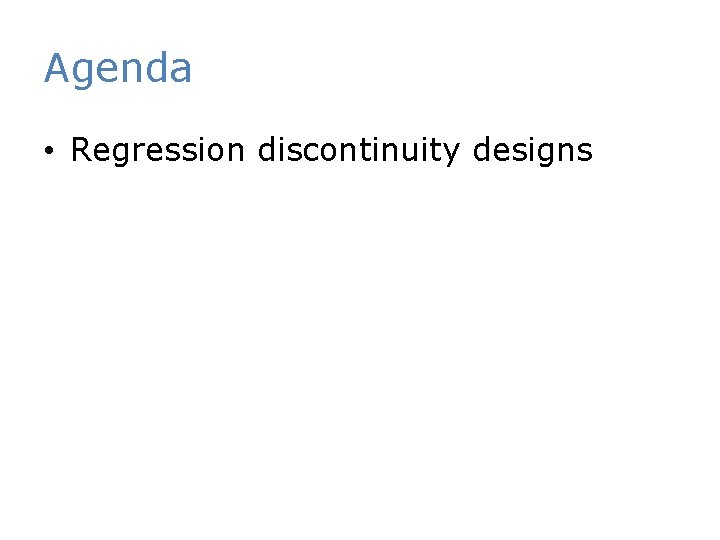
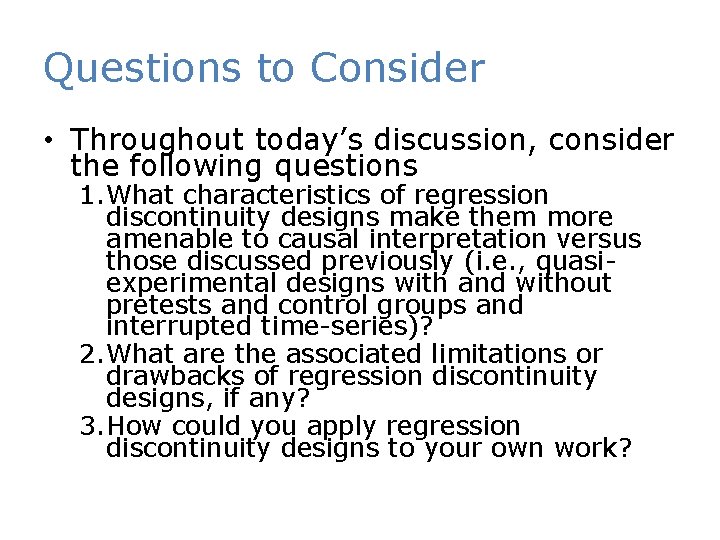
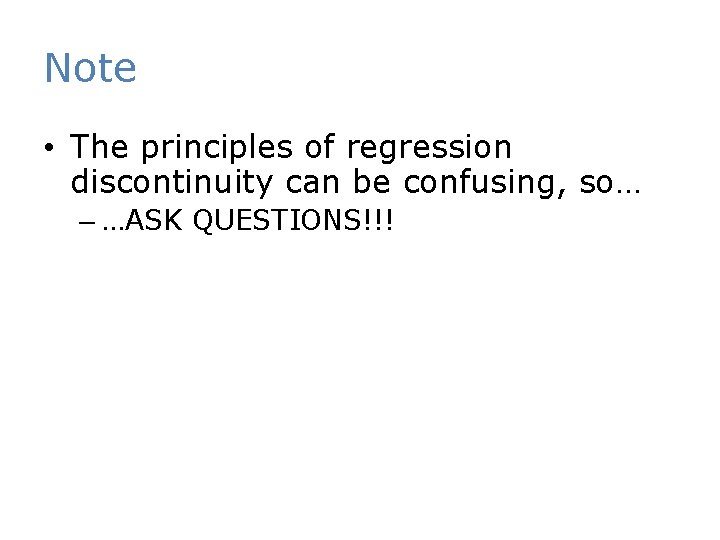
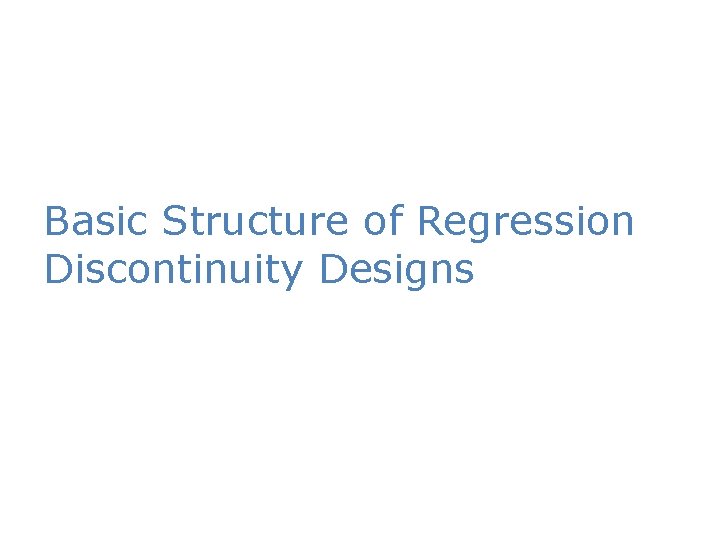
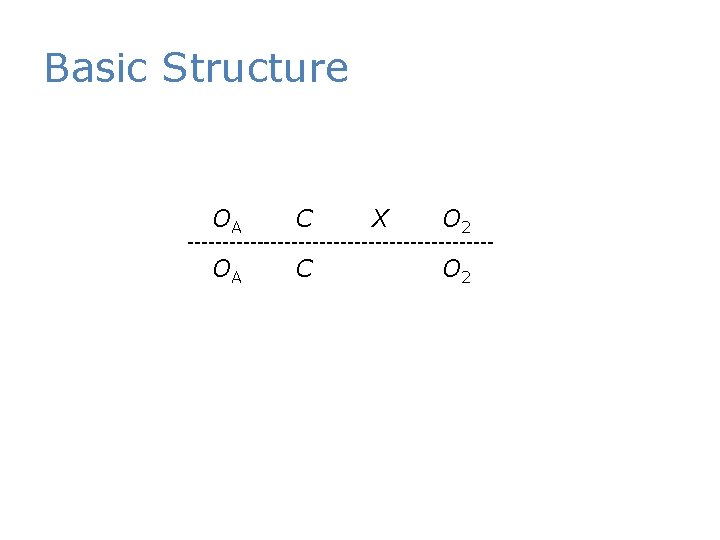
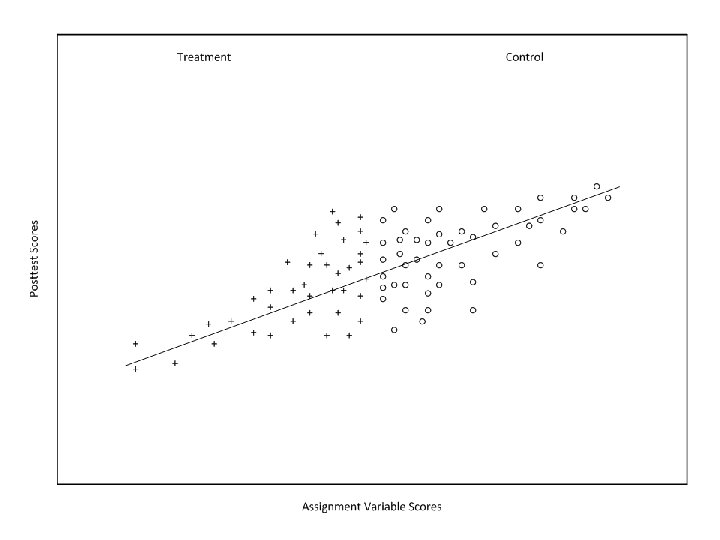
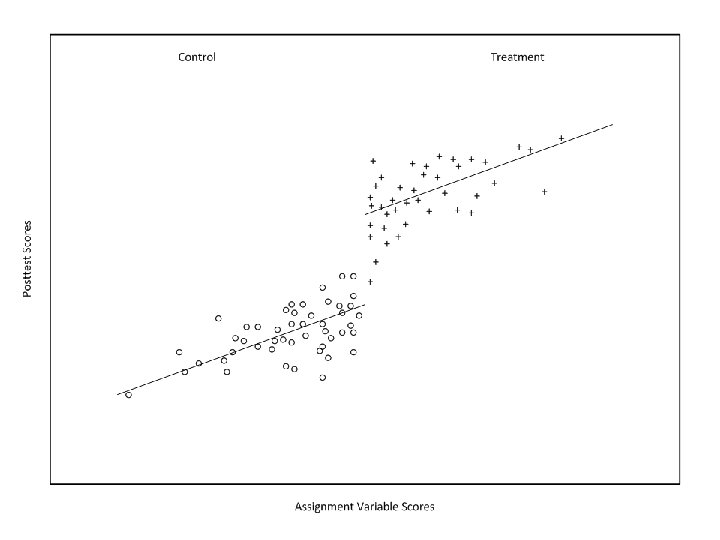
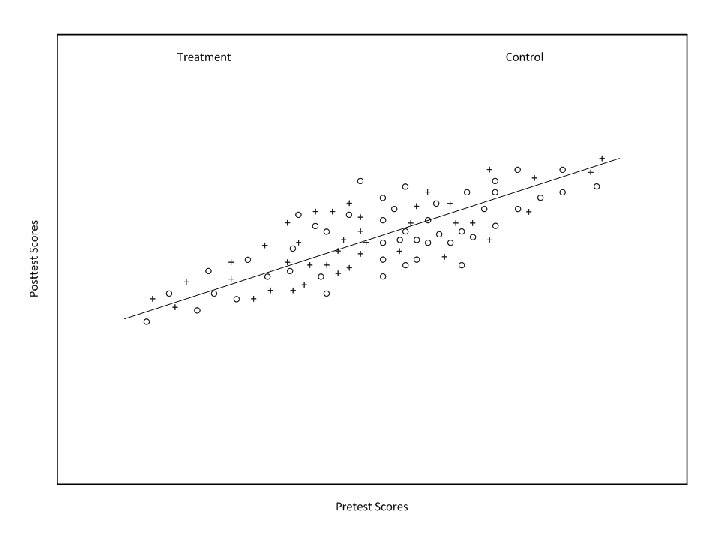
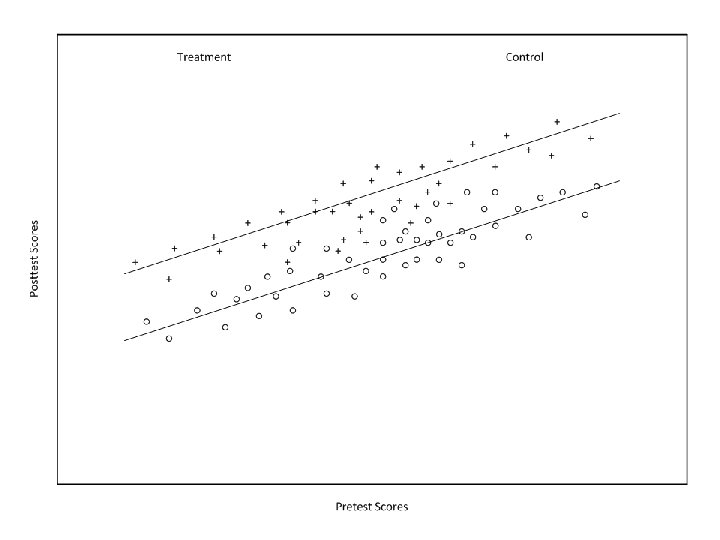
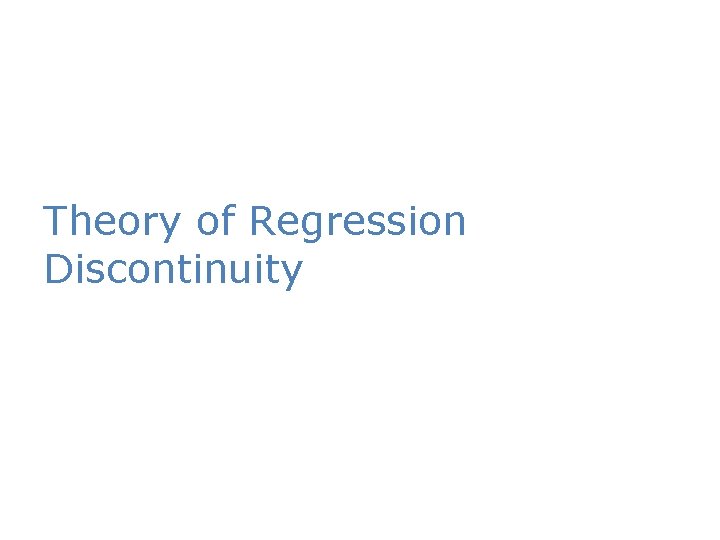
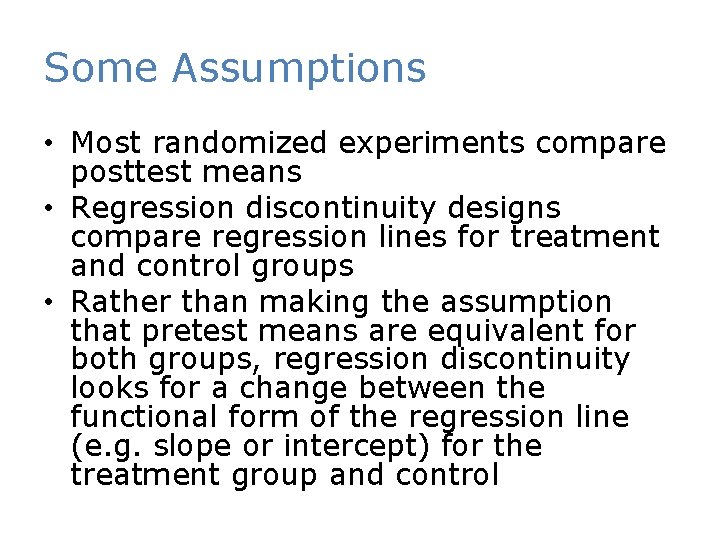
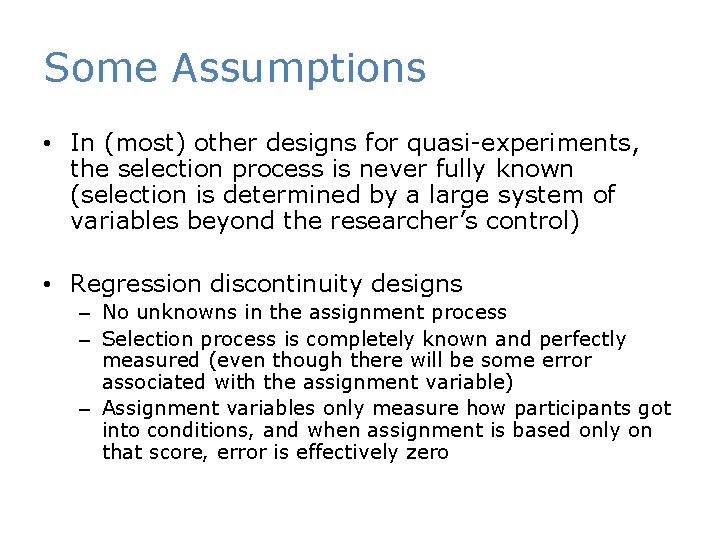
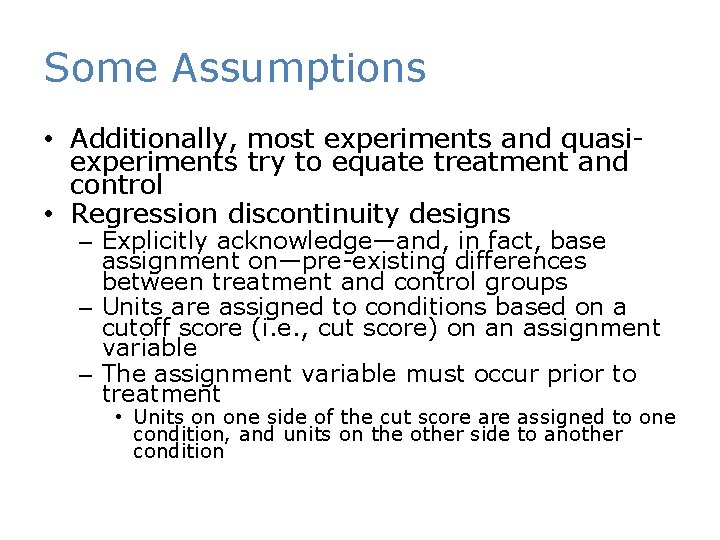
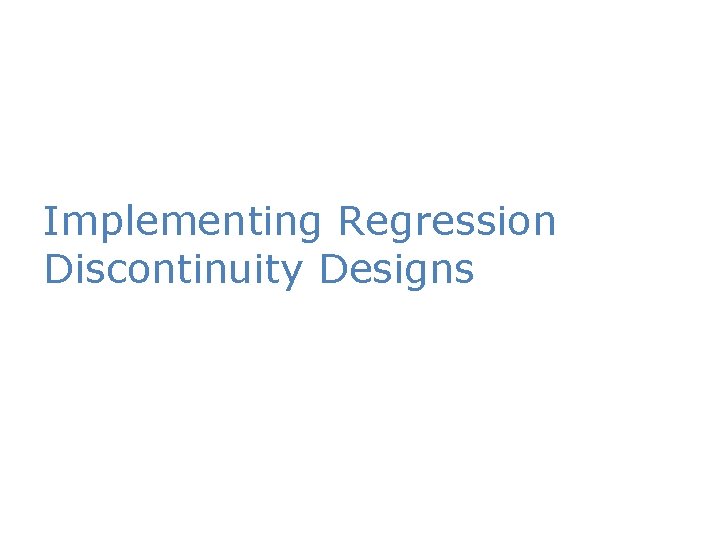
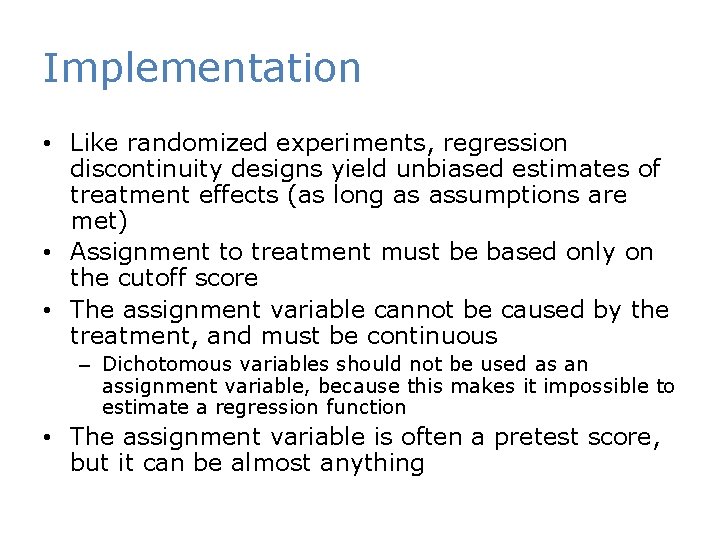
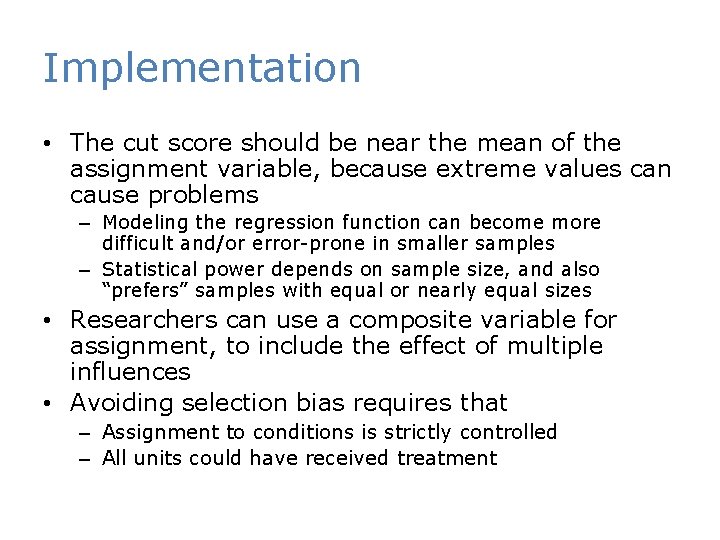
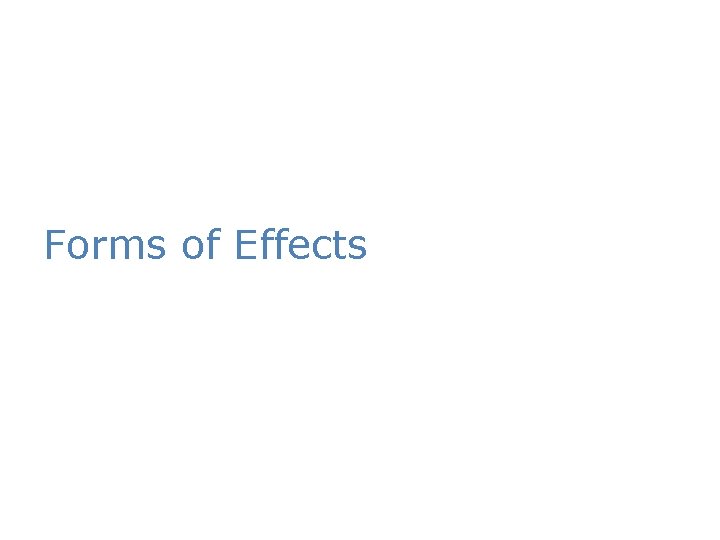
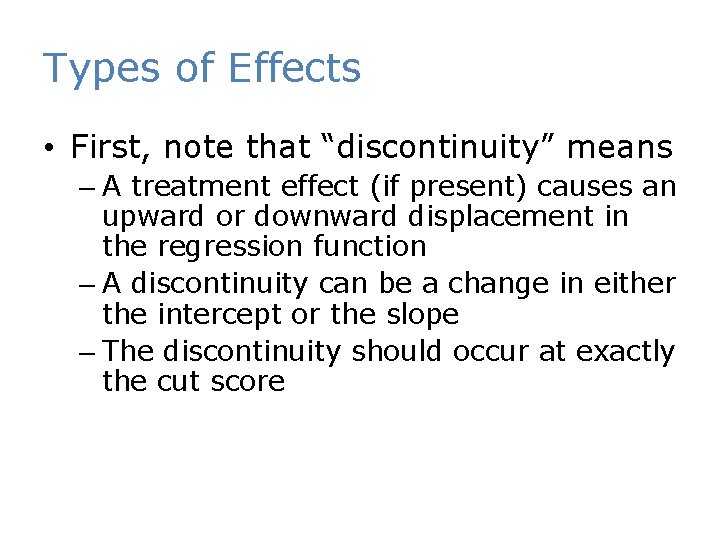
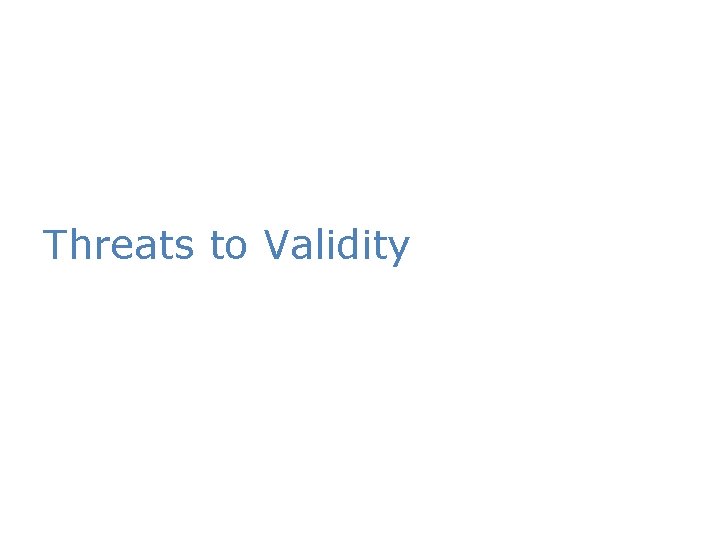
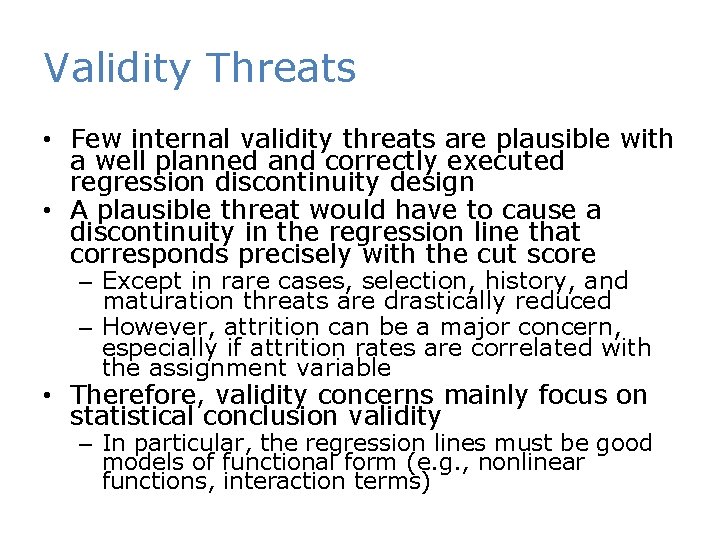
- Slides: 21
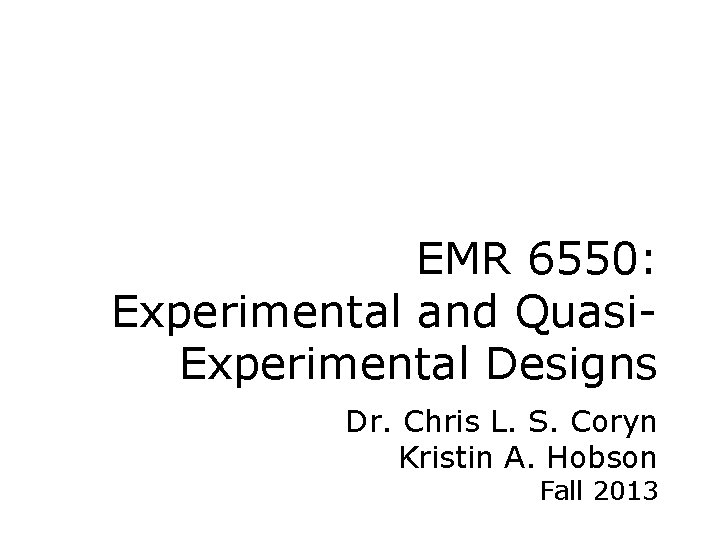
EMR 6550: Experimental and Quasi. Experimental Designs Dr. Chris L. S. Coryn Kristin A. Hobson Fall 2013
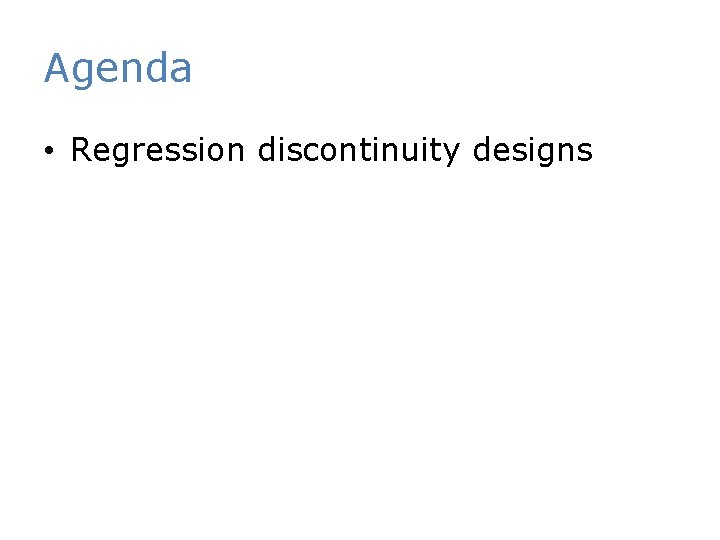
Agenda • Regression discontinuity designs
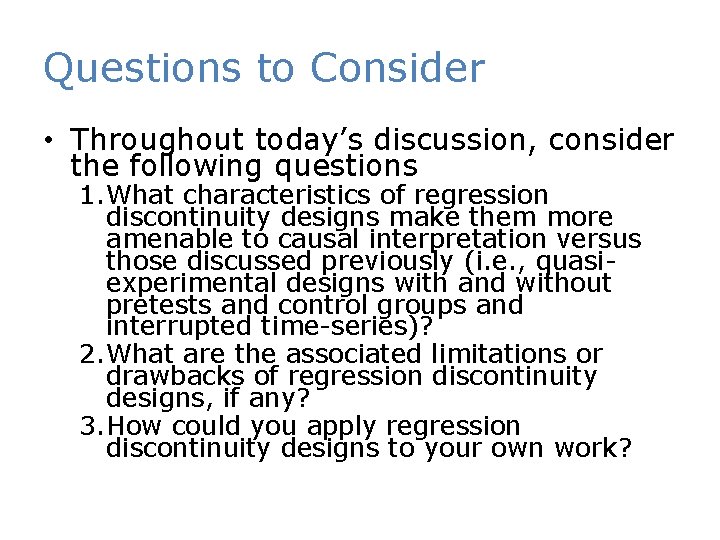
Questions to Consider • Throughout today’s discussion, consider the following questions 1. What characteristics of regression discontinuity designs make them more amenable to causal interpretation versus those discussed previously (i. e. , quasiexperimental designs with and without pretests and control groups and interrupted time-series)? 2. What are the associated limitations or drawbacks of regression discontinuity designs, if any? 3. How could you apply regression discontinuity designs to your own work?
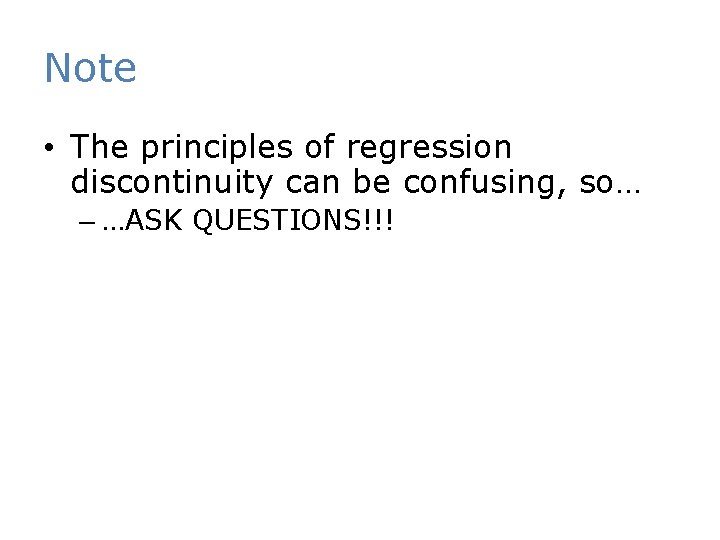
Note • The principles of regression discontinuity can be confusing, so… – …ASK QUESTIONS!!!
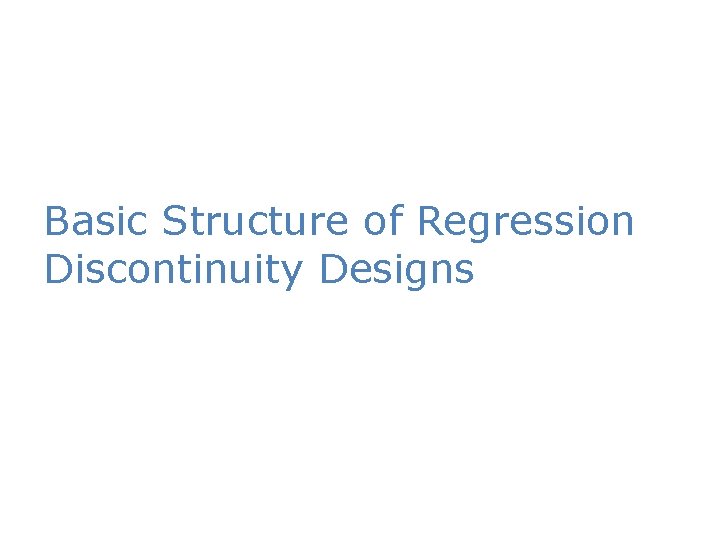
Basic Structure of Regression Discontinuity Designs
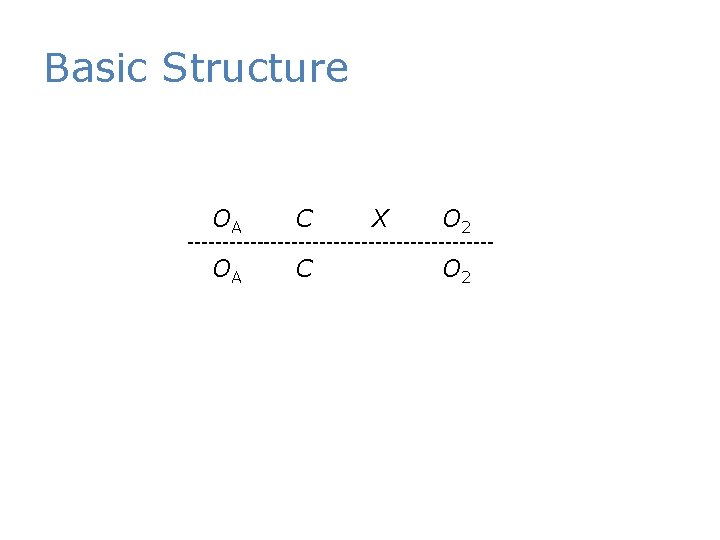
Basic Structure OA C X O 2
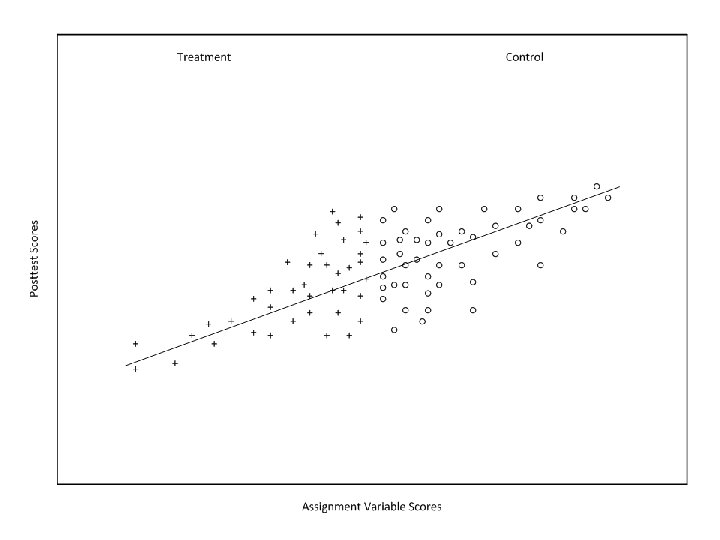
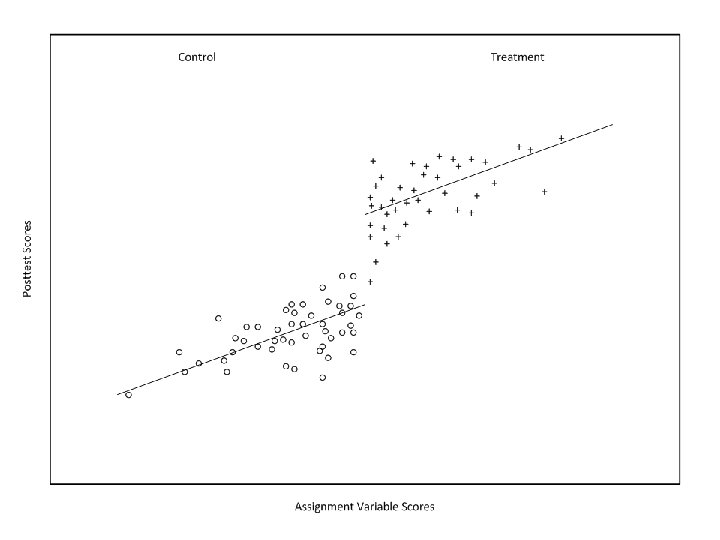
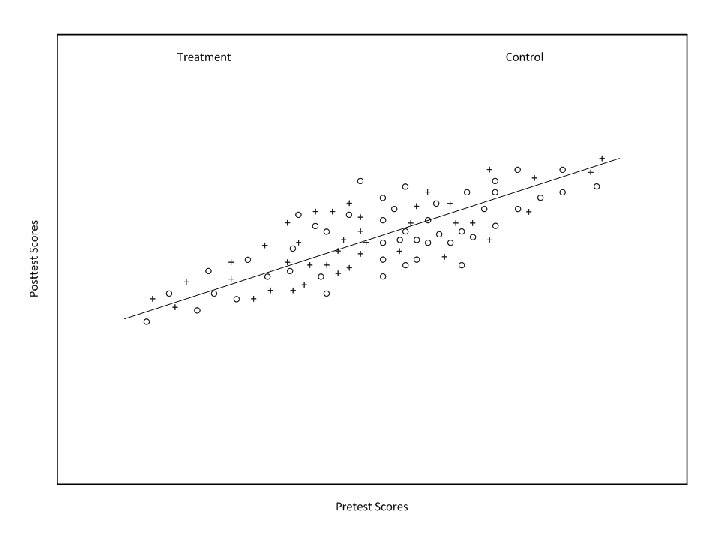
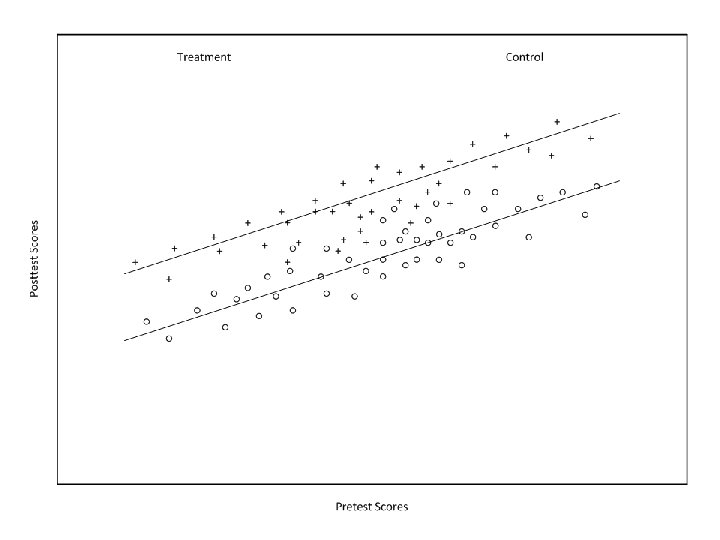
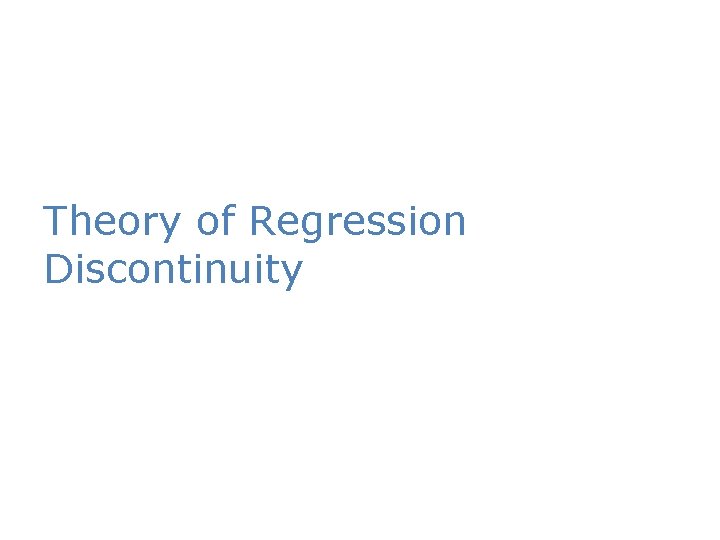
Theory of Regression Discontinuity
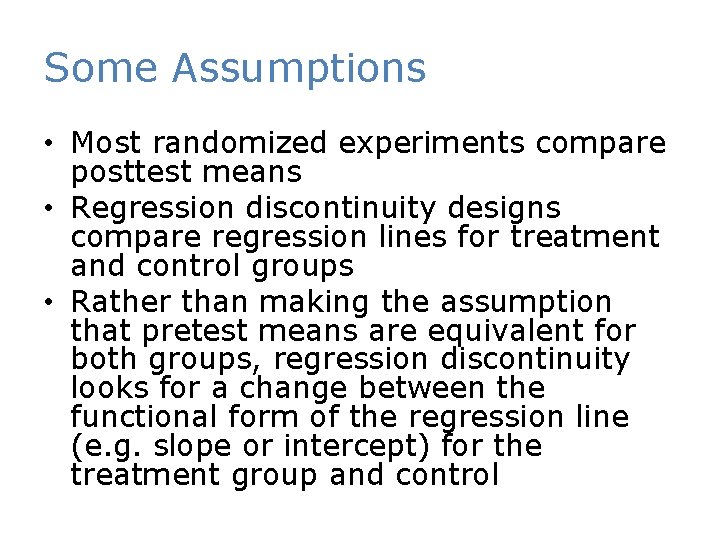
Some Assumptions • Most randomized experiments compare posttest means • Regression discontinuity designs compare regression lines for treatment and control groups • Rather than making the assumption that pretest means are equivalent for both groups, regression discontinuity looks for a change between the functional form of the regression line (e. g. slope or intercept) for the treatment group and control
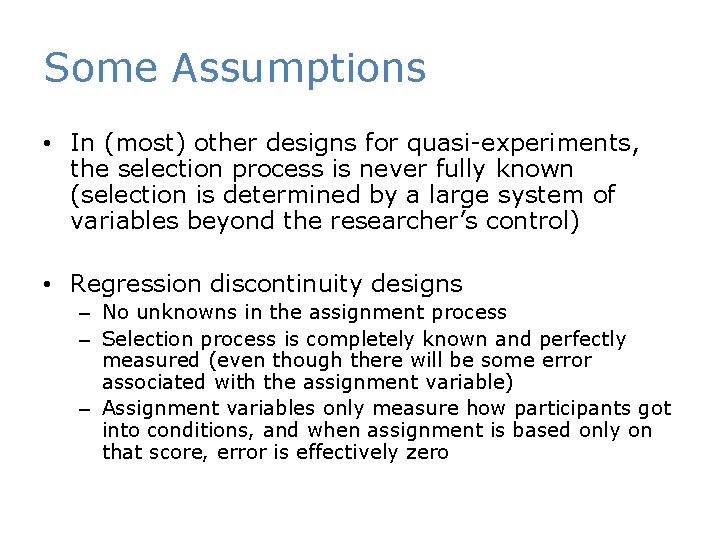
Some Assumptions • In (most) other designs for quasi-experiments, the selection process is never fully known (selection is determined by a large system of variables beyond the researcher’s control) • Regression discontinuity designs – No unknowns in the assignment process – Selection process is completely known and perfectly measured (even though there will be some error associated with the assignment variable) – Assignment variables only measure how participants got into conditions, and when assignment is based only on that score, error is effectively zero
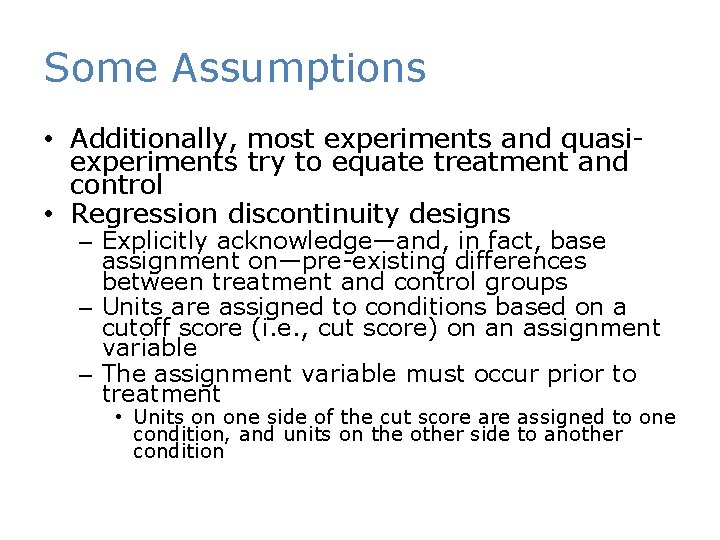
Some Assumptions • Additionally, most experiments and quasiexperiments try to equate treatment and control • Regression discontinuity designs – Explicitly acknowledge—and, in fact, base assignment on—pre-existing differences between treatment and control groups – Units are assigned to conditions based on a cutoff score (i. e. , cut score) on an assignment variable – The assignment variable must occur prior to treatment • Units on one side of the cut score assigned to one condition, and units on the other side to another condition
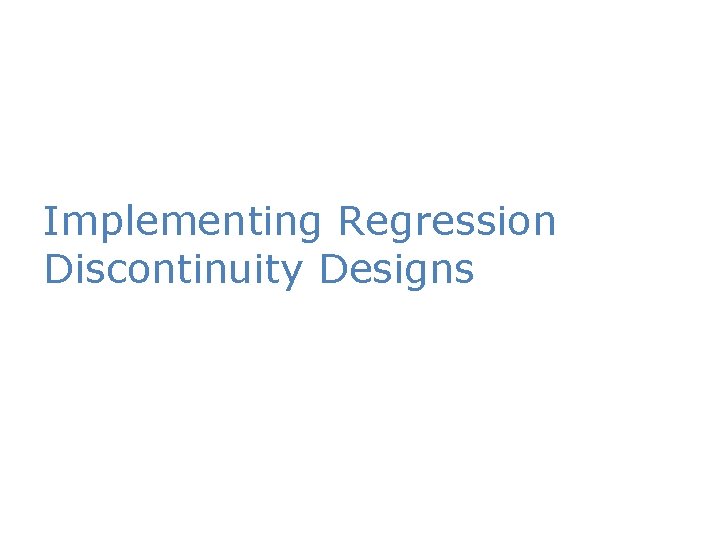
Implementing Regression Discontinuity Designs
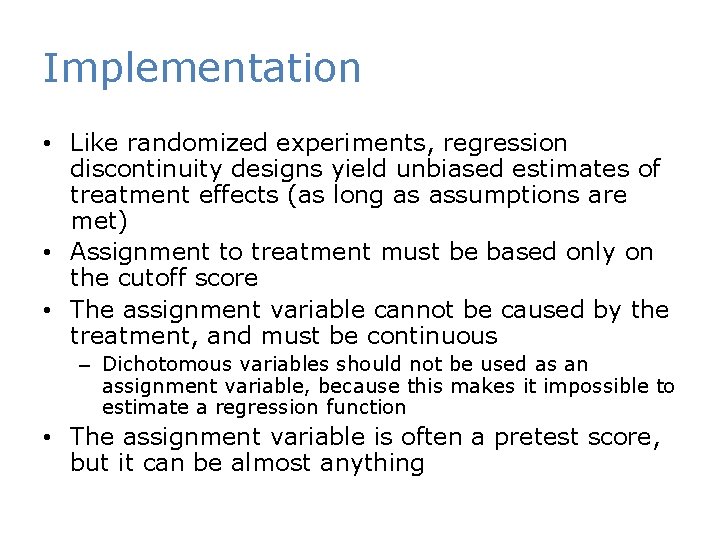
Implementation • Like randomized experiments, regression discontinuity designs yield unbiased estimates of treatment effects (as long as assumptions are met) • Assignment to treatment must be based only on the cutoff score • The assignment variable cannot be caused by the treatment, and must be continuous – Dichotomous variables should not be used as an assignment variable, because this makes it impossible to estimate a regression function • The assignment variable is often a pretest score, but it can be almost anything
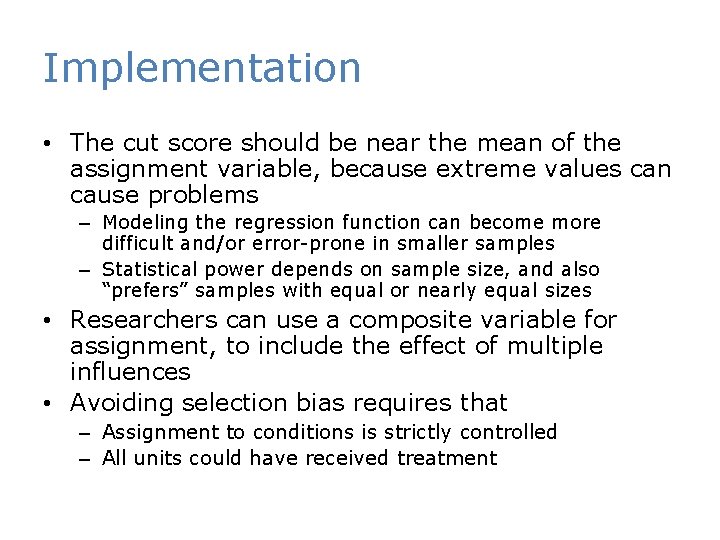
Implementation • The cut score should be near the mean of the assignment variable, because extreme values can cause problems – Modeling the regression function can become more difficult and/or error-prone in smaller samples – Statistical power depends on sample size, and also “prefers” samples with equal or nearly equal sizes • Researchers can use a composite variable for assignment, to include the effect of multiple influences • Avoiding selection bias requires that – Assignment to conditions is strictly controlled – All units could have received treatment
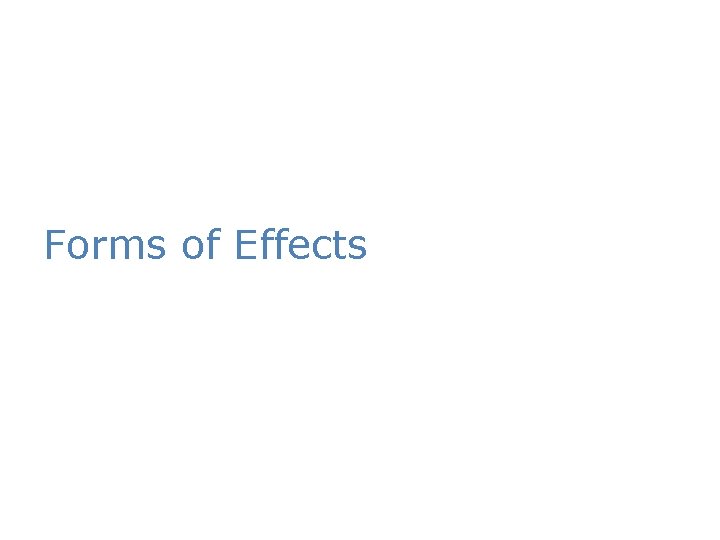
Forms of Effects
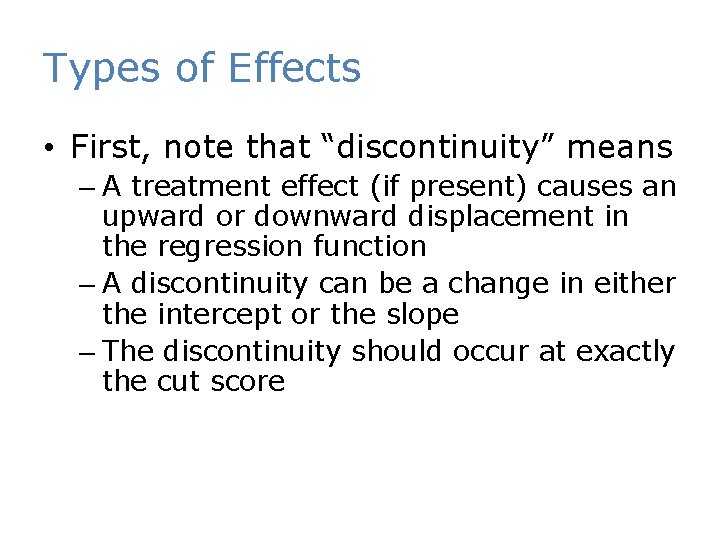
Types of Effects • First, note that “discontinuity” means – A treatment effect (if present) causes an upward or downward displacement in the regression function – A discontinuity can be a change in either the intercept or the slope – The discontinuity should occur at exactly the cut score
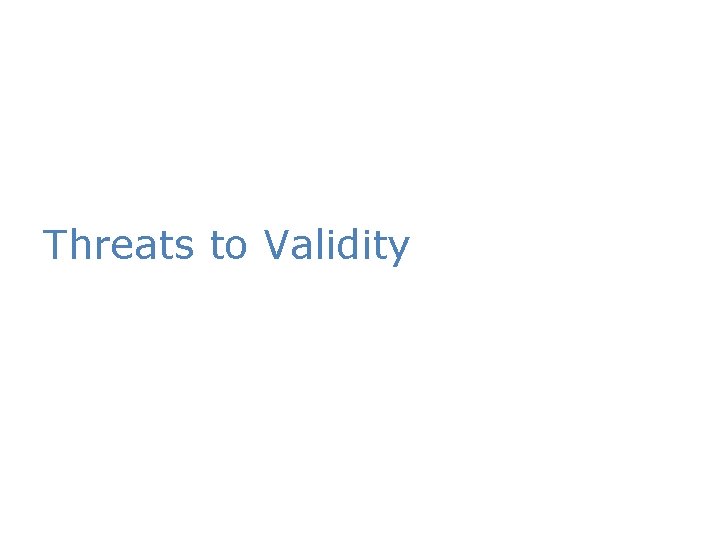
Threats to Validity
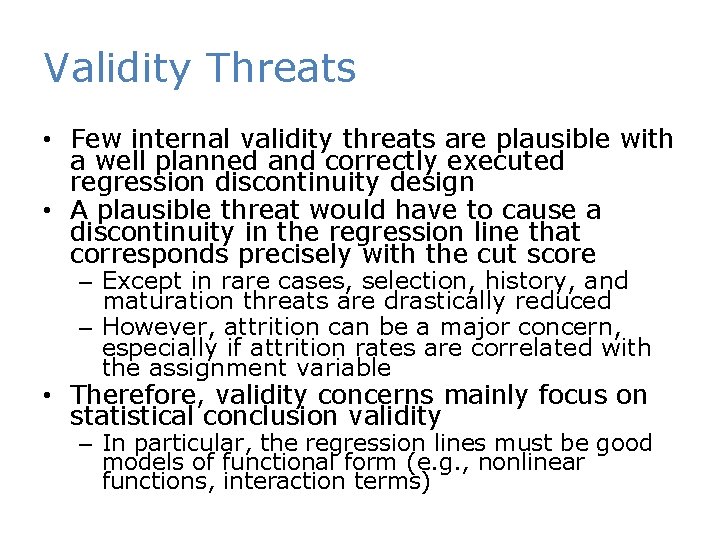
Validity Threats • Few internal validity threats are plausible with a well planned and correctly executed regression discontinuity design • A plausible threat would have to cause a discontinuity in the regression line that corresponds precisely with the cut score – Except in rare cases, selection, history, and maturation threats are drastically reduced – However, attrition can be a major concern, especially if attrition rates are correlated with the assignment variable • Therefore, validity concerns mainly focus on statistical conclusion validity – In particular, the regression lines must be good models of functional form (e. g. , nonlinear functions, interaction terms)
Quasi-experimental research designs
Quasi-experimental research designs
Mtp 6550
Quasi experimental design example
Quasi experimental design example
Quasi experimental
Ethical consideration in experimental research sample
Postnodd
Causal comparative research example
Quasi experimental study
Random assignment vs selection
Characteristics of experimental research design
Quasi experiment
Quasi experimental
Cross-sectional correlational design
Desain eksperimen
Quasi experimental design slideshare
11 principles of design
Experimental design
Types of research design ppt
Basic principles of experimental design
Experimental vs non experimental