QUASIEXPERIMENTAL DESIGNS SMALL N DESIGNS CORRELATIONAL DESIGNS Week
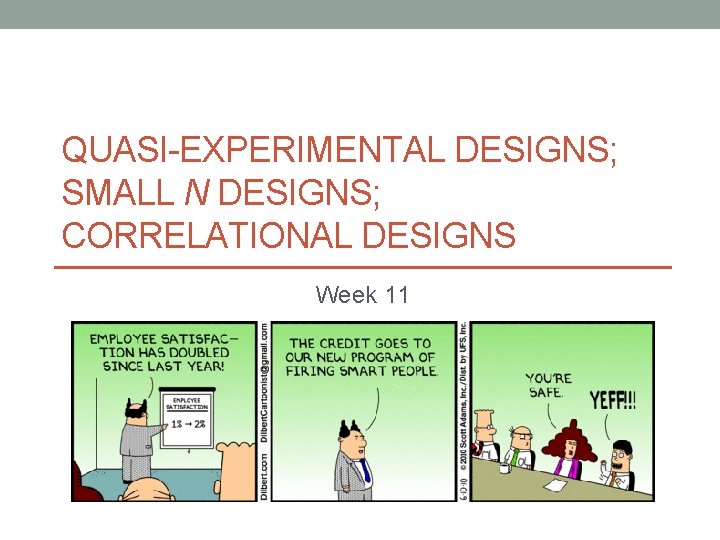
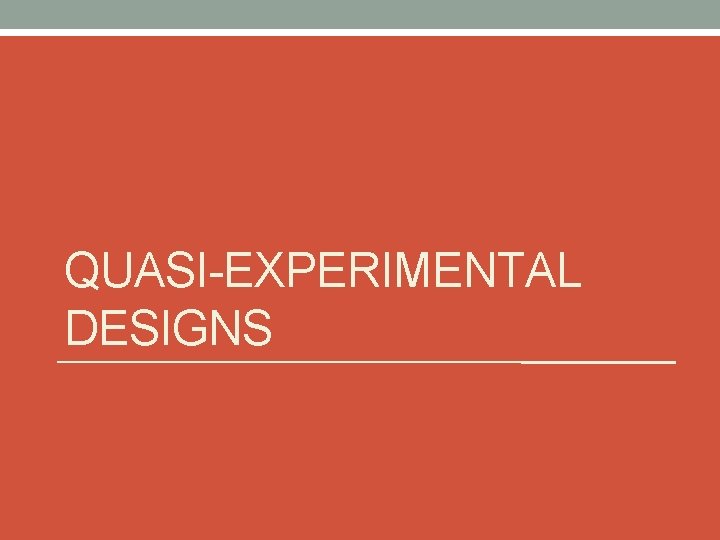
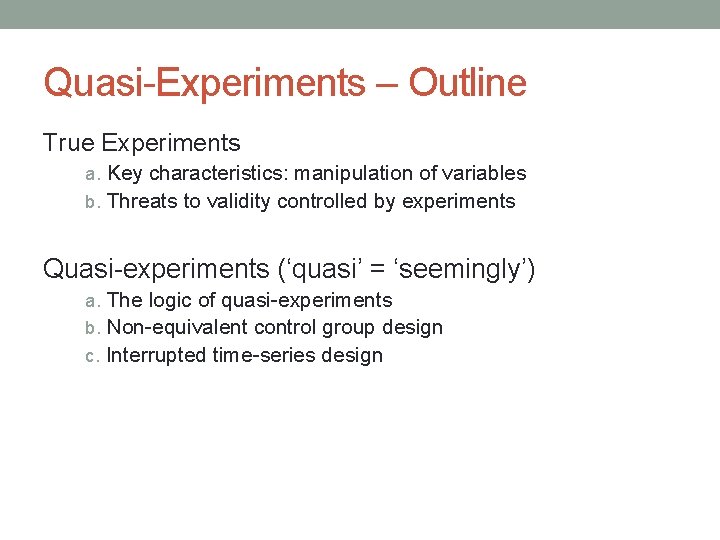
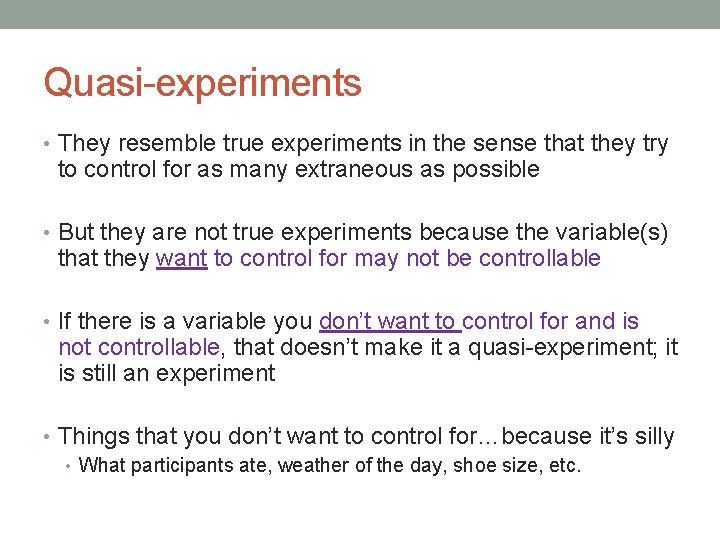
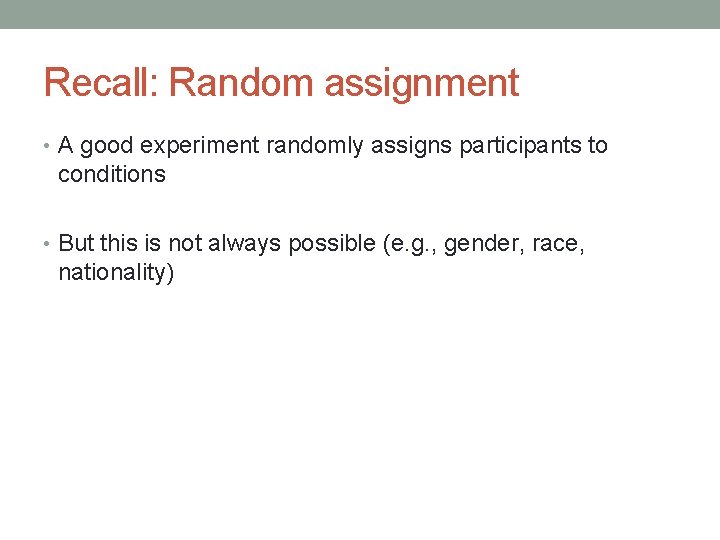
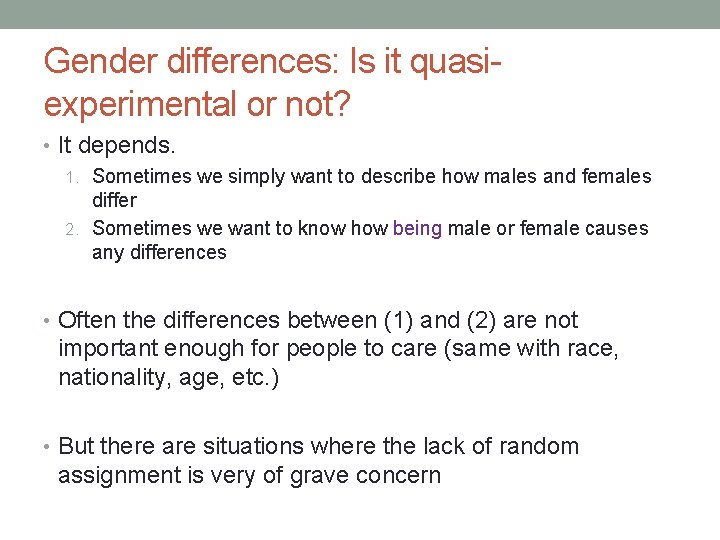
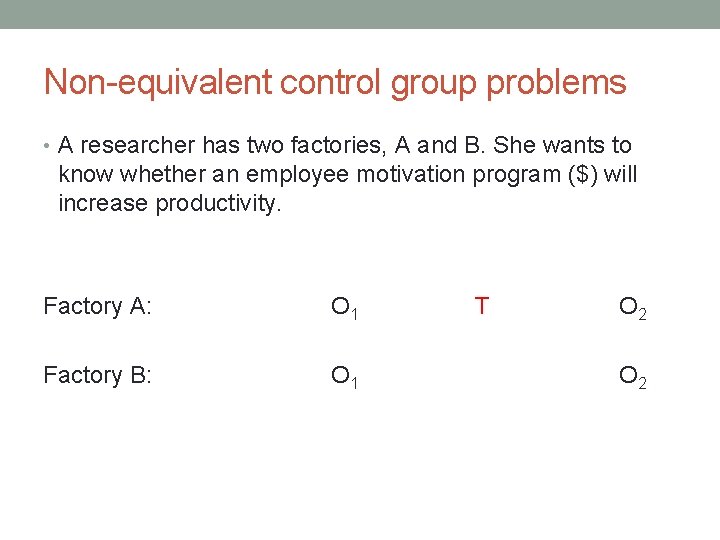
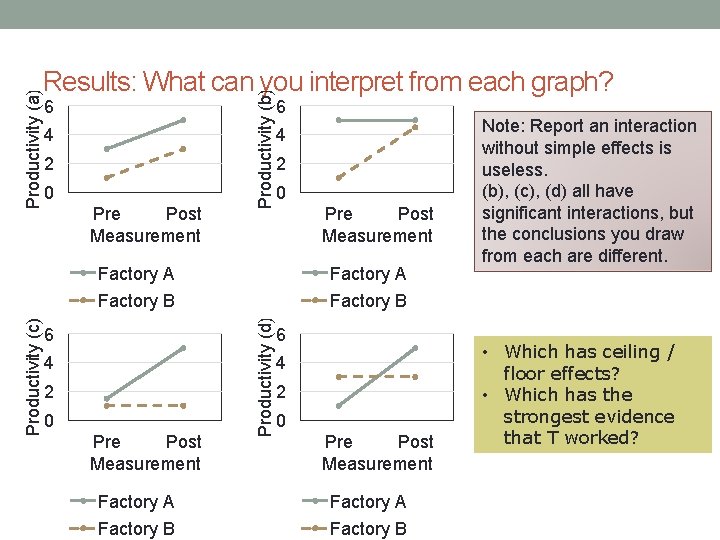
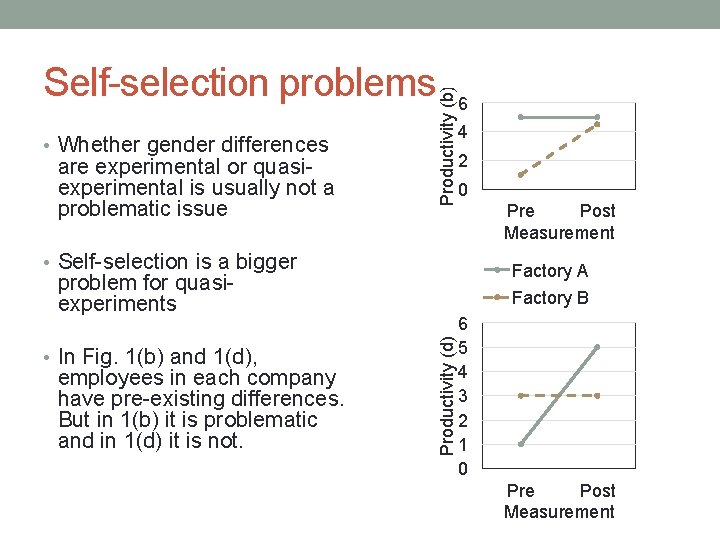
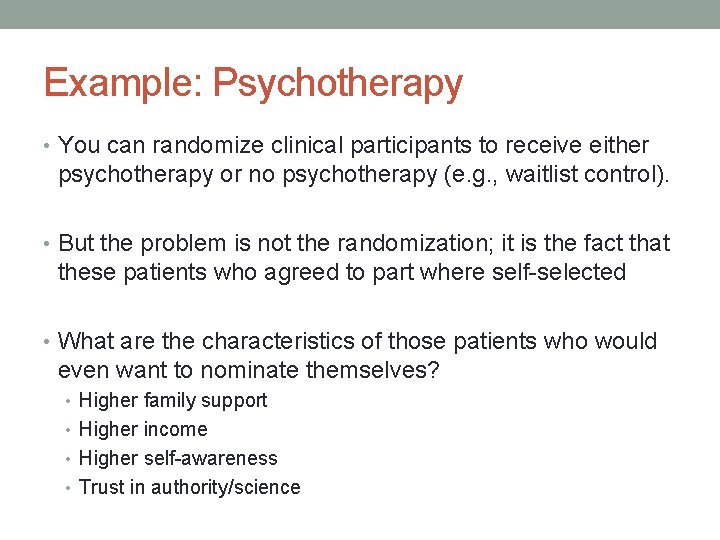
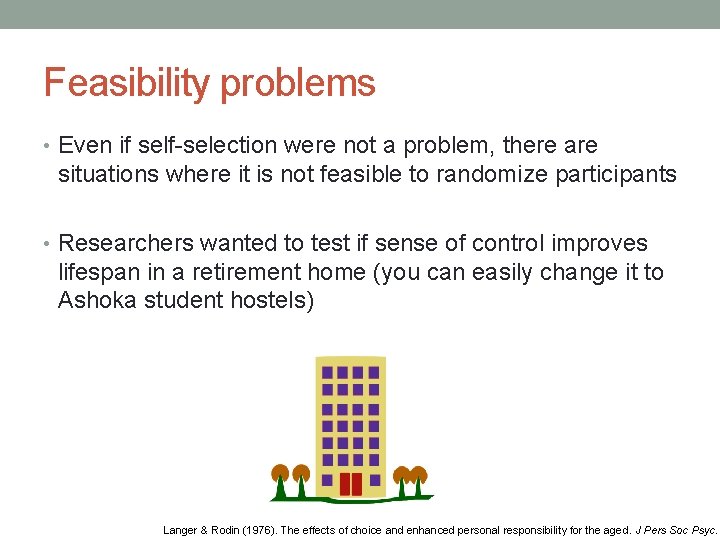
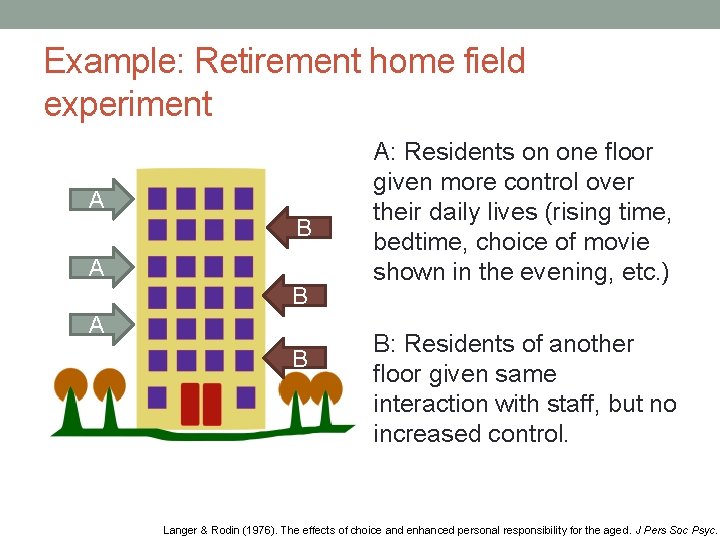
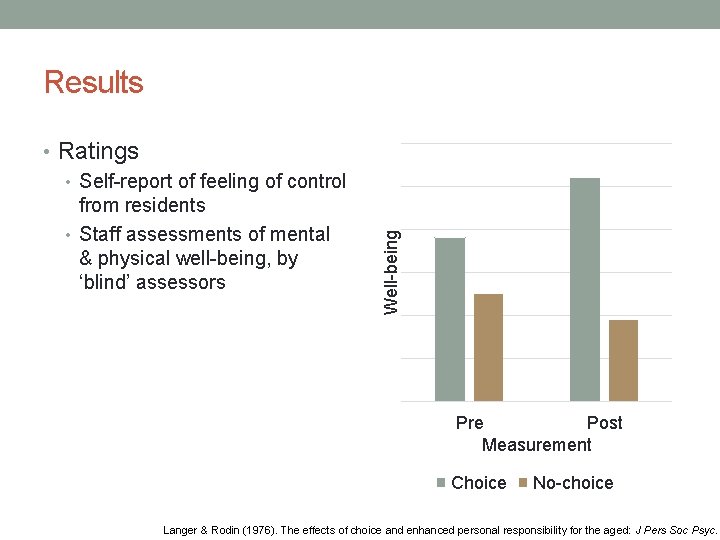
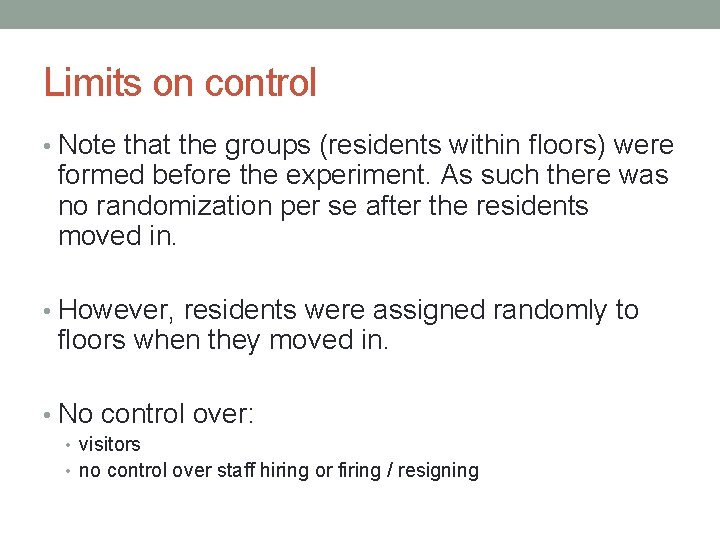
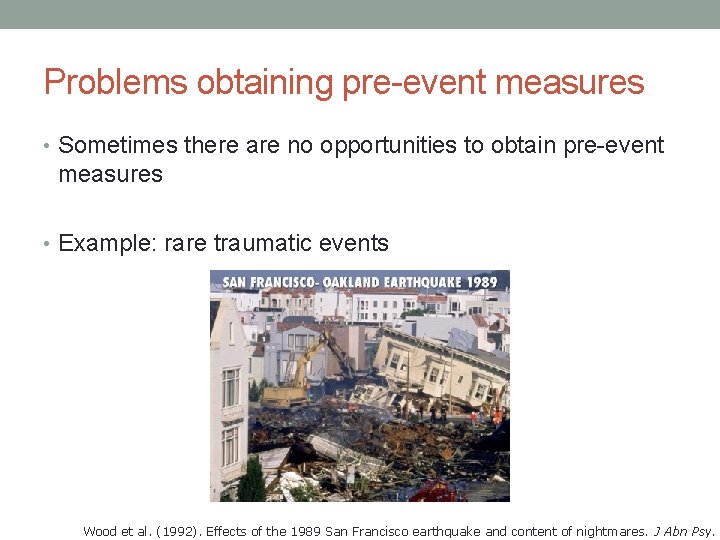
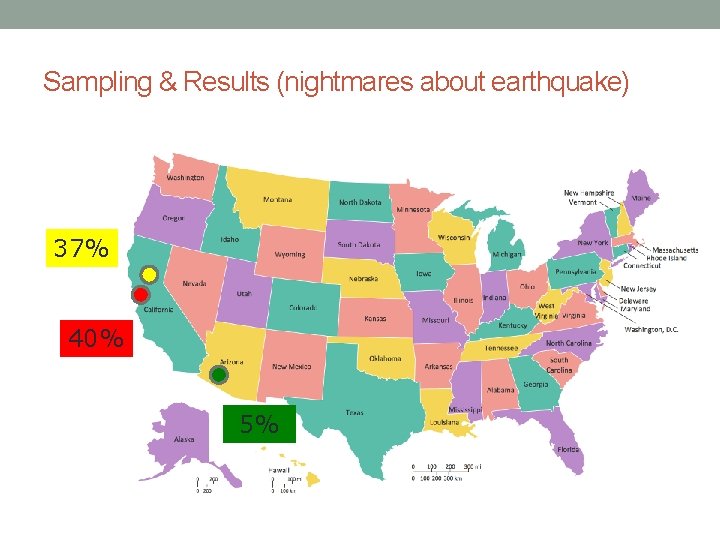
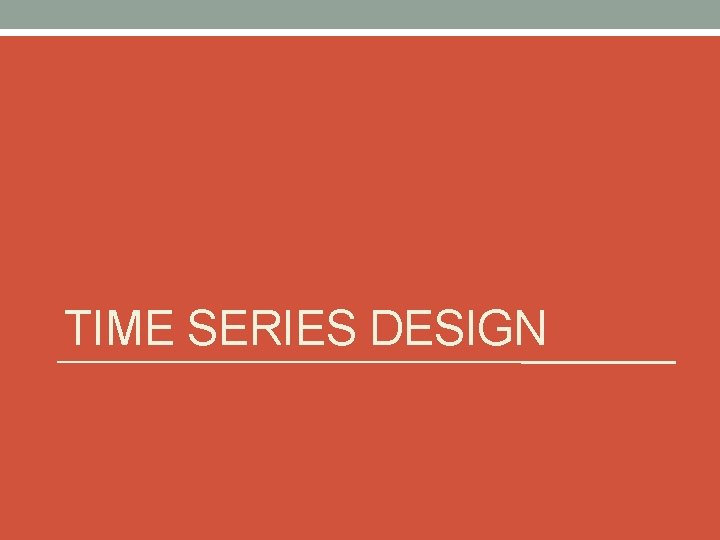
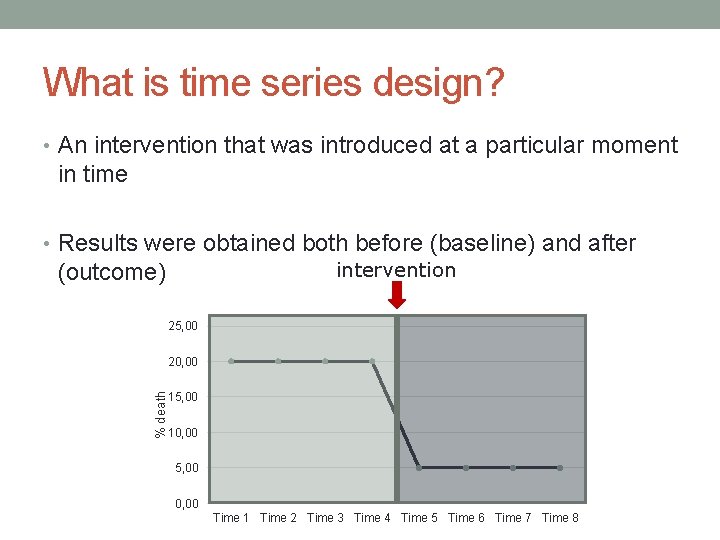
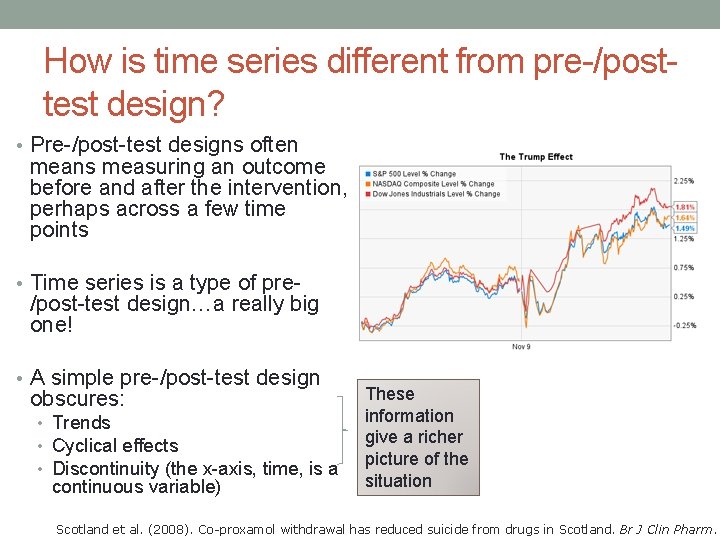
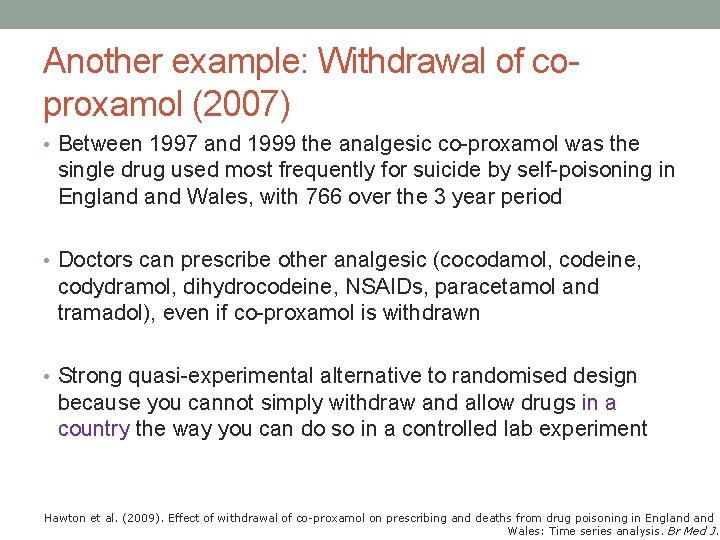
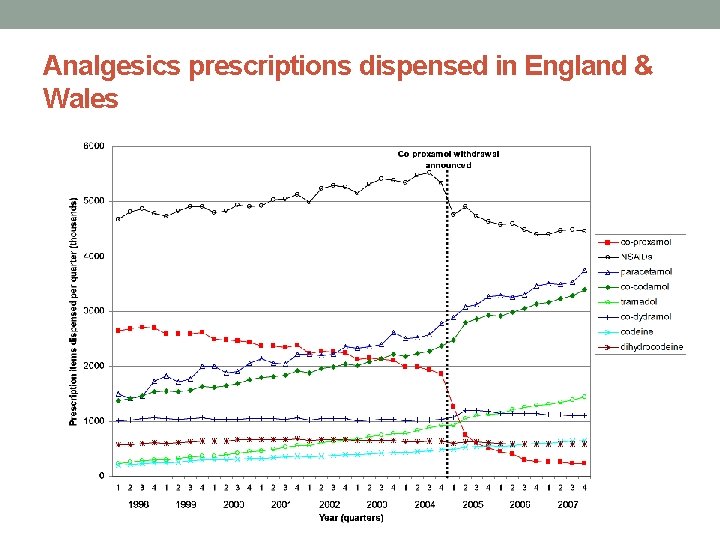
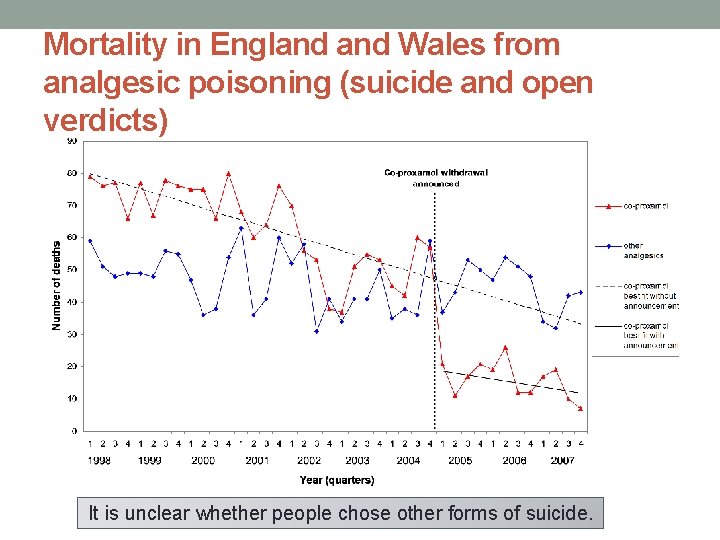
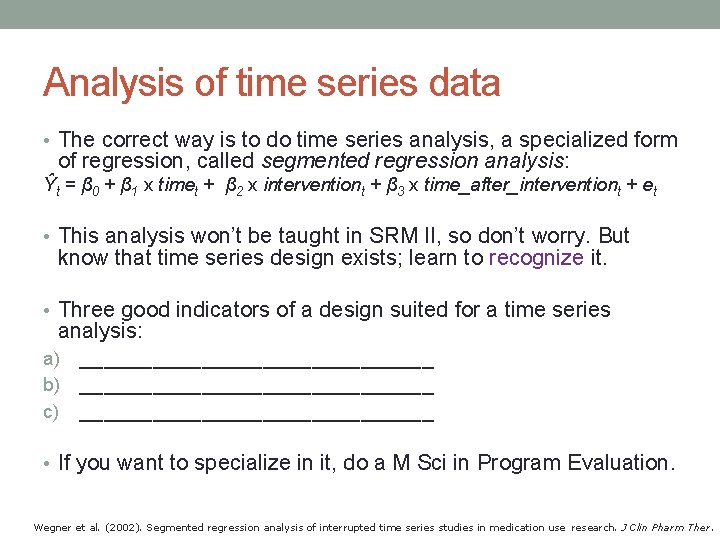
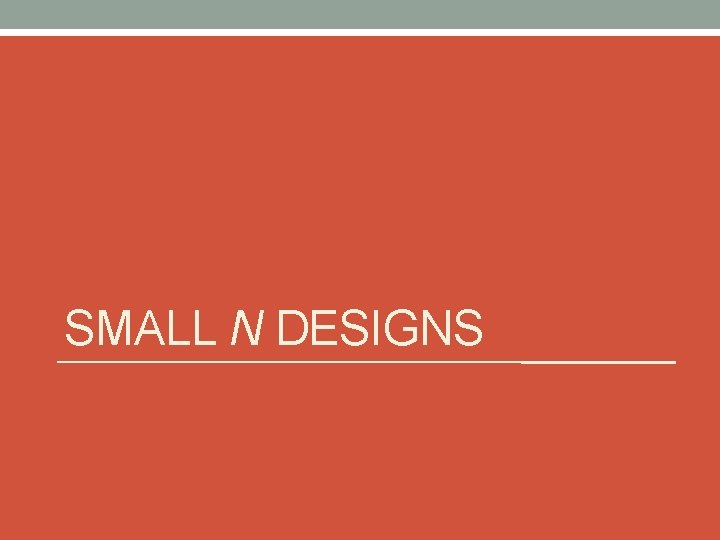
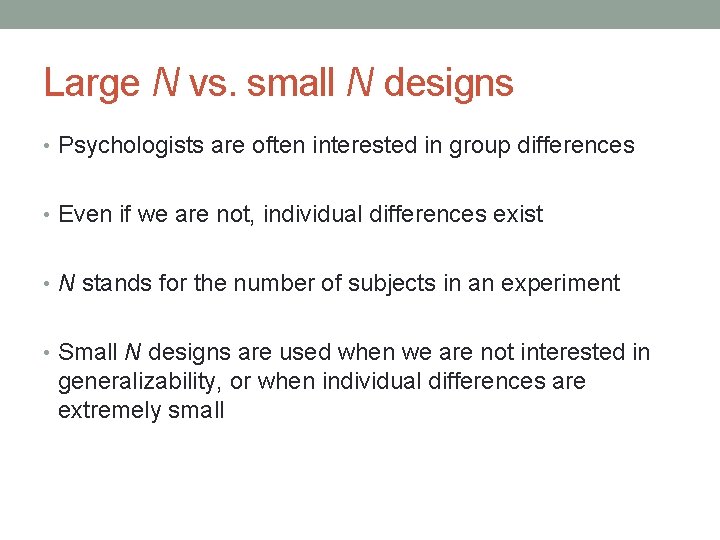
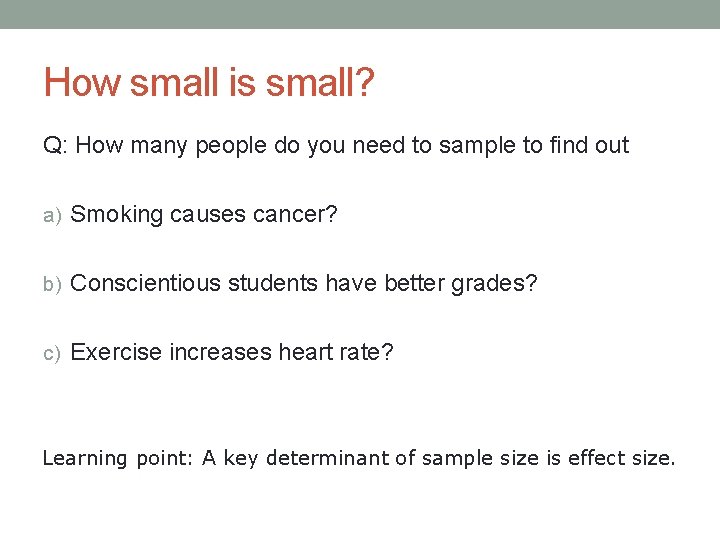
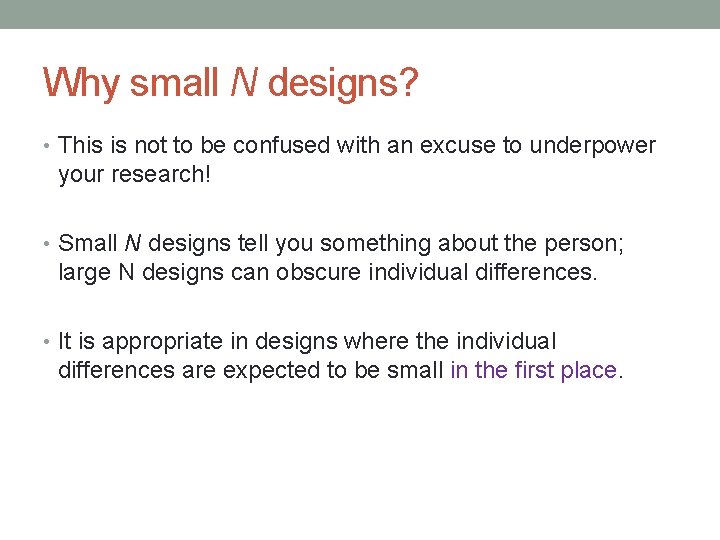
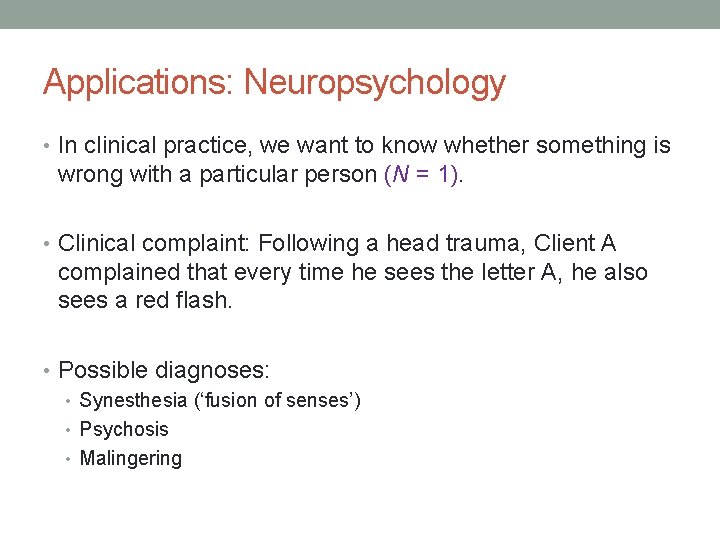
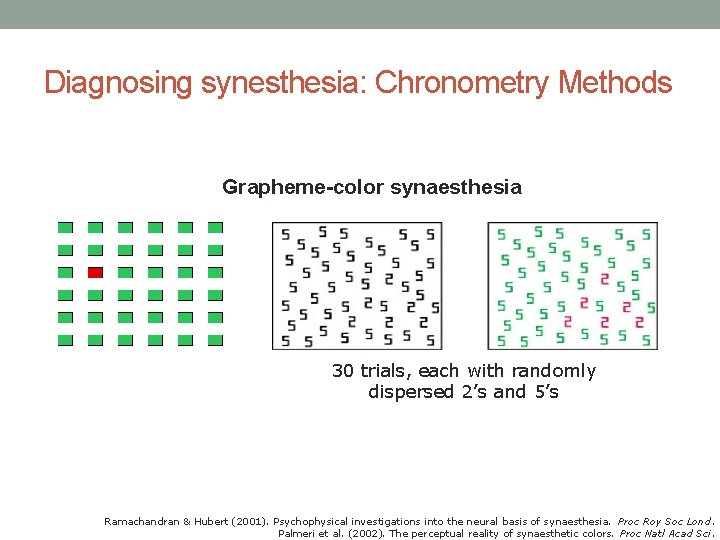
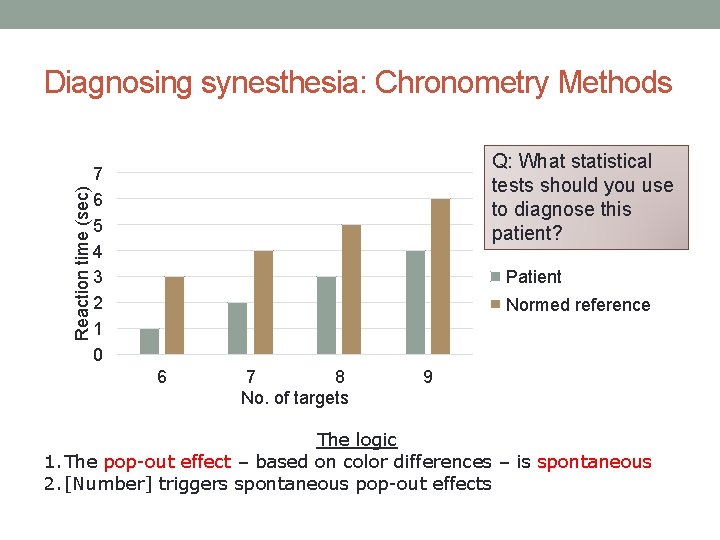
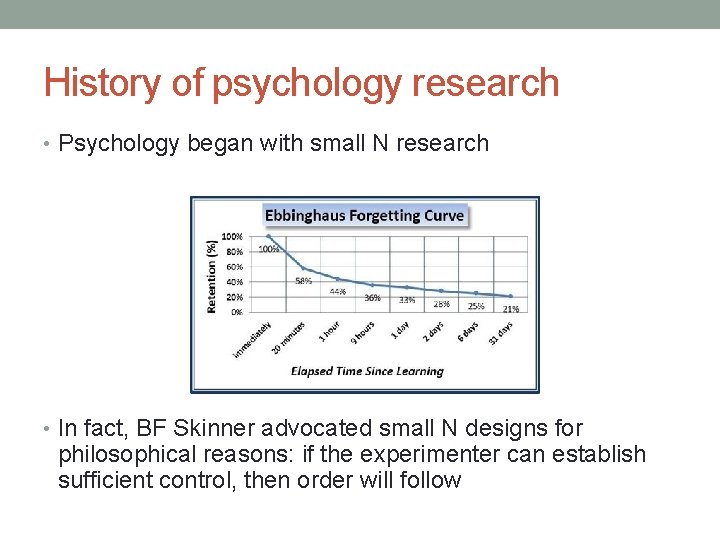
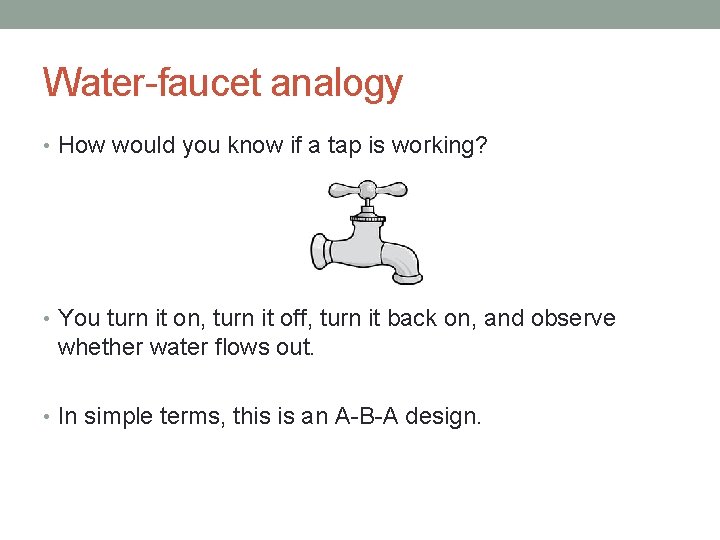
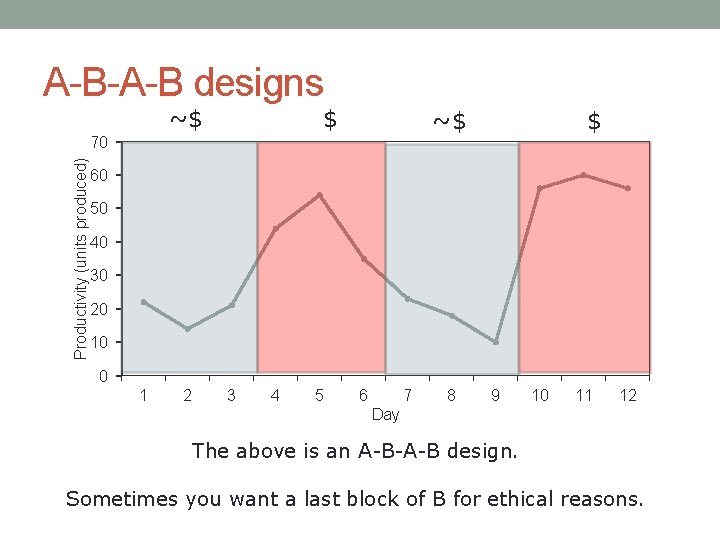
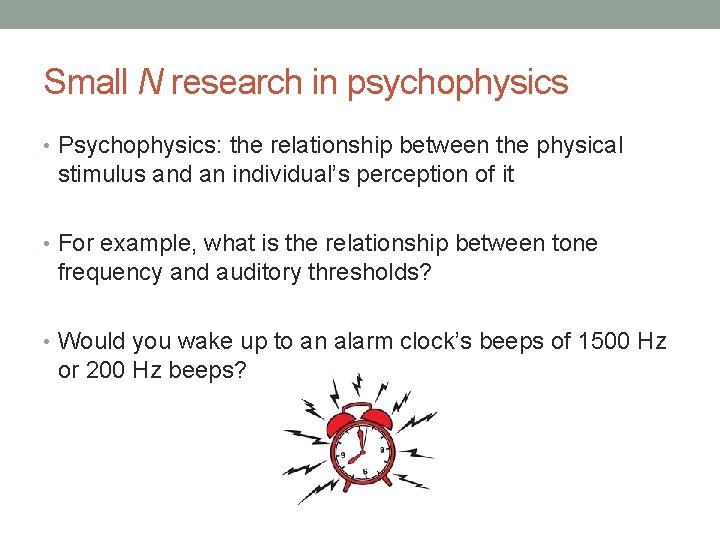
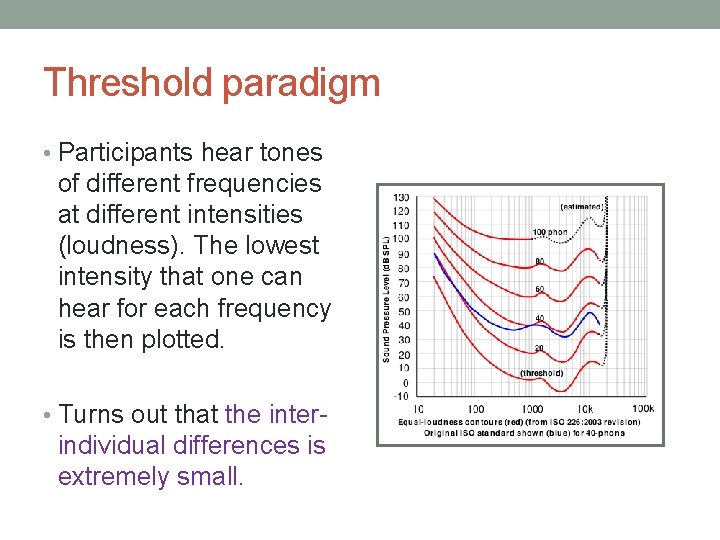
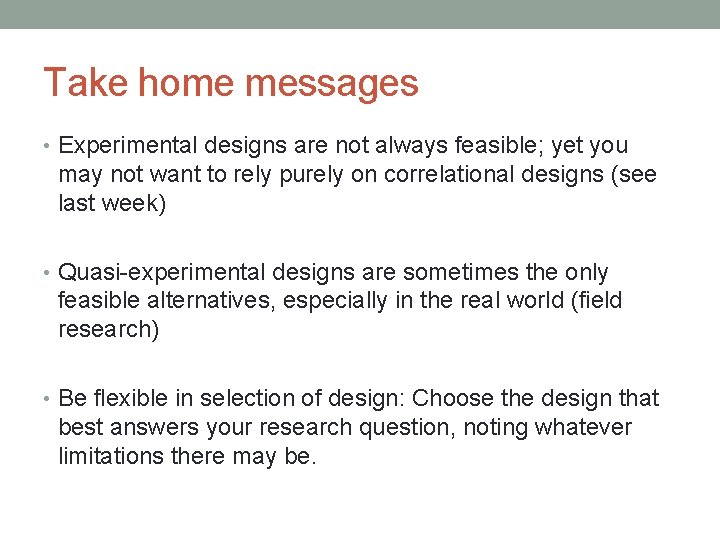
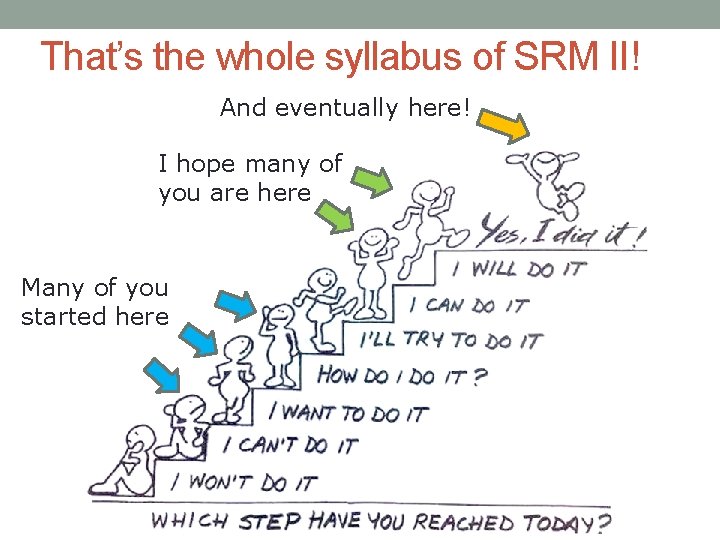
- Slides: 37
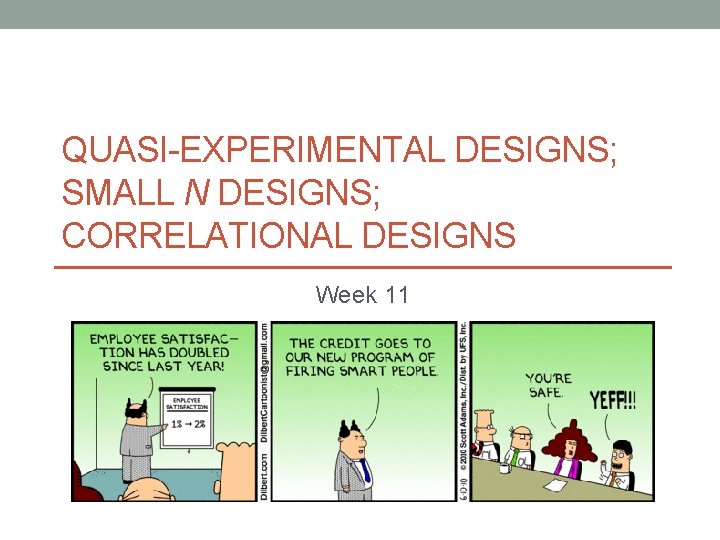
QUASI-EXPERIMENTAL DESIGNS; SMALL N DESIGNS; CORRELATIONAL DESIGNS Week 11
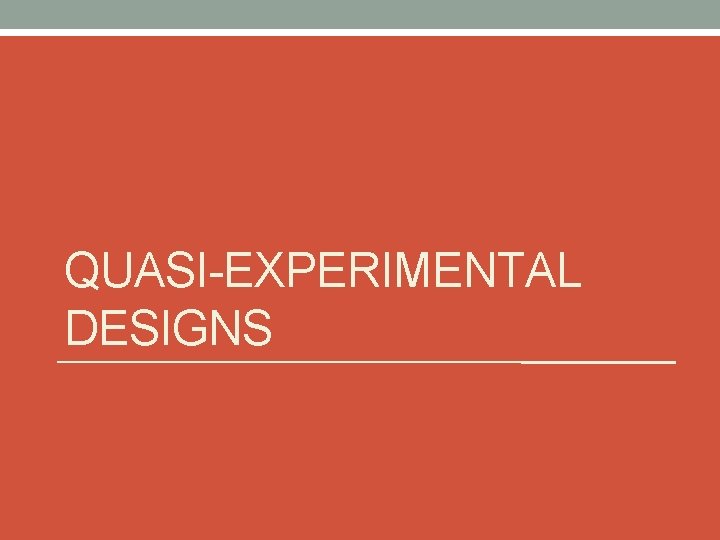
QUASI-EXPERIMENTAL DESIGNS
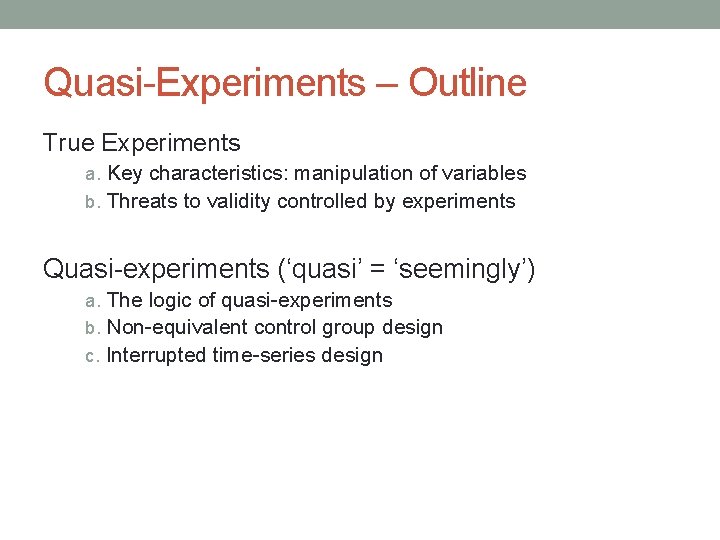
Quasi-Experiments – Outline True Experiments a. Key characteristics: manipulation of variables b. Threats to validity controlled by experiments Quasi-experiments (‘quasi’ = ‘seemingly’) a. The logic of quasi-experiments b. Non-equivalent control group design c. Interrupted time-series design
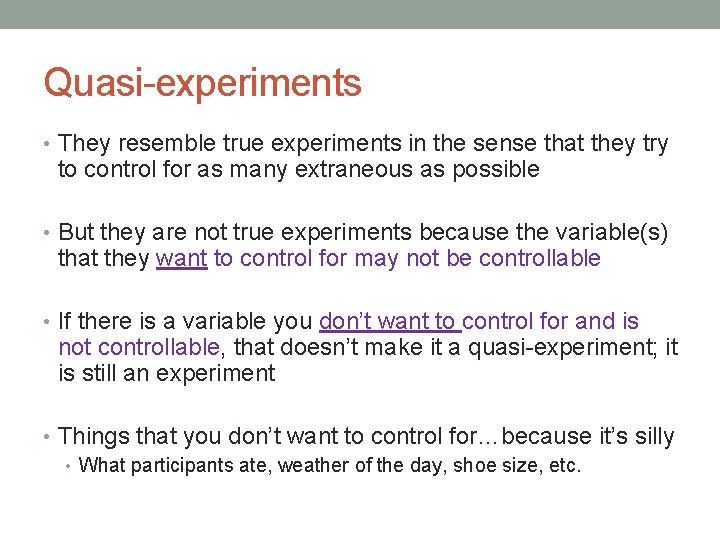
Quasi-experiments • They resemble true experiments in the sense that they try to control for as many extraneous as possible • But they are not true experiments because the variable(s) that they want to control for may not be controllable • If there is a variable you don’t want to control for and is not controllable, that doesn’t make it a quasi-experiment; it is still an experiment • Things that you don’t want to control for…because it’s silly • What participants ate, weather of the day, shoe size, etc.
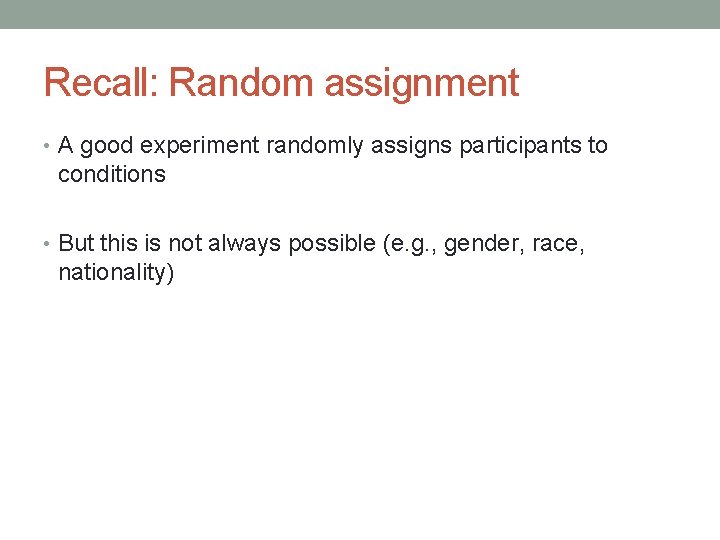
Recall: Random assignment • A good experiment randomly assigns participants to conditions • But this is not always possible (e. g. , gender, race, nationality)
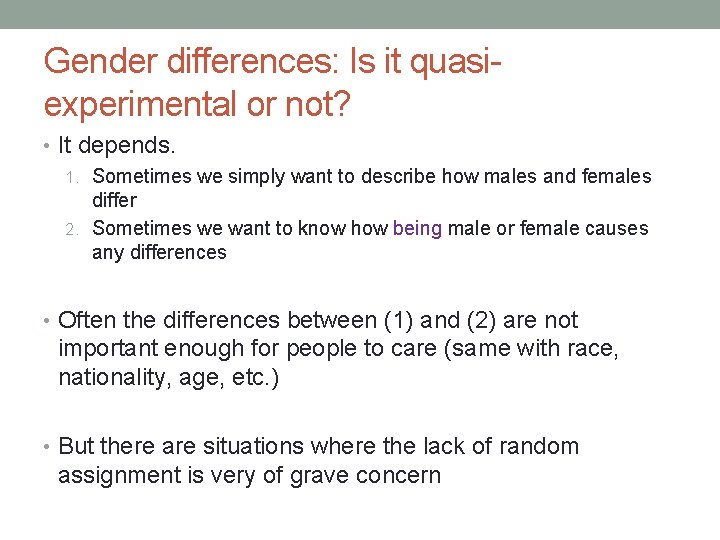
Gender differences: Is it quasiexperimental or not? • It depends. 1. Sometimes we simply want to describe how males and females differ 2. Sometimes we want to know how being male or female causes any differences • Often the differences between (1) and (2) are not important enough for people to care (same with race, nationality, age, etc. ) • But there are situations where the lack of random assignment is very of grave concern
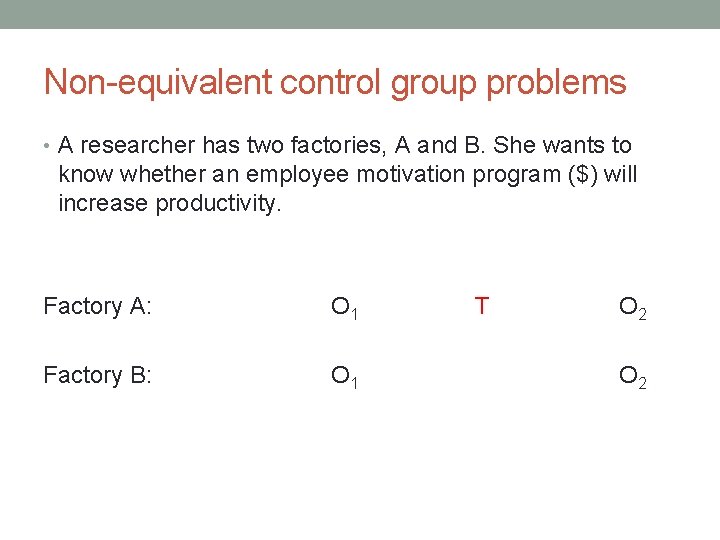
Non-equivalent control group problems • A researcher has two factories, A and B. She wants to know whether an employee motivation program ($) will increase productivity. Factory A: O 1 Factory B: O 1 T O 2
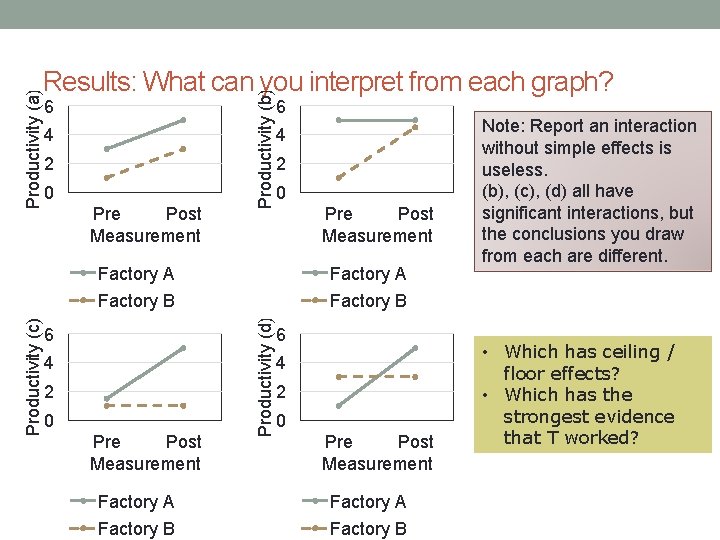
4 2 0 Pre Post Measurement Productivity (b) 6 6 4 2 0 Pre Post Measurement Factory A Factory B 6 4 2 0 Pre Post Measurement Productivity (d) Productivity (a) Productivity (c) Results: What can you interpret from each graph? 6 4 2 0 Pre Post Measurement Factory A Factory B Note: Report an interaction without simple effects is useless. (b), (c), (d) all have significant interactions, but the conclusions you draw from each are different. • Which has ceiling / floor effects? • Which has the strongest evidence that T worked?
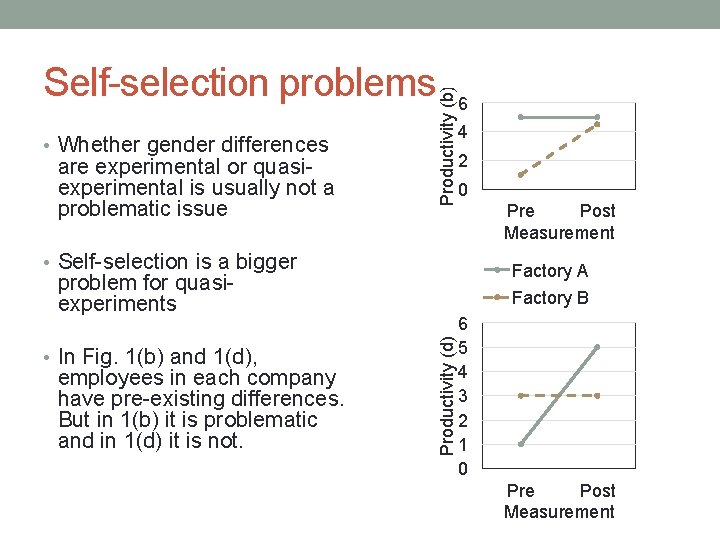
• Whether gender differences are experimental or quasiexperimental is usually not a problematic issue Productivity (b) Self-selection problems 6 4 2 0 Pre Post Measurement • Self-selection is a bigger Factory A problem for quasiexperiments employees in each company have pre-existing differences. But in 1(b) it is problematic and in 1(d) it is not. Productivity (d) • In Fig. 1(b) and 1(d), Factory B 6 5 4 3 2 1 0 Pre Post Measurement
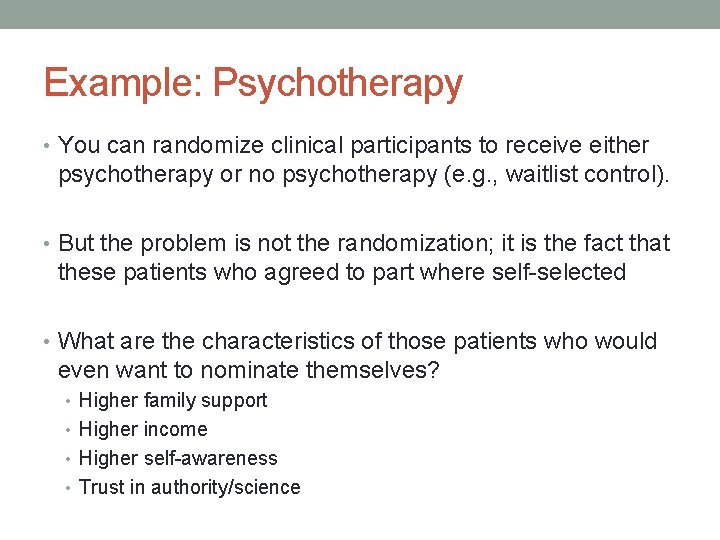
Example: Psychotherapy • You can randomize clinical participants to receive either psychotherapy or no psychotherapy (e. g. , waitlist control). • But the problem is not the randomization; it is the fact that these patients who agreed to part where self-selected • What are the characteristics of those patients who would even want to nominate themselves? • Higher family support • Higher income • Higher self-awareness • Trust in authority/science
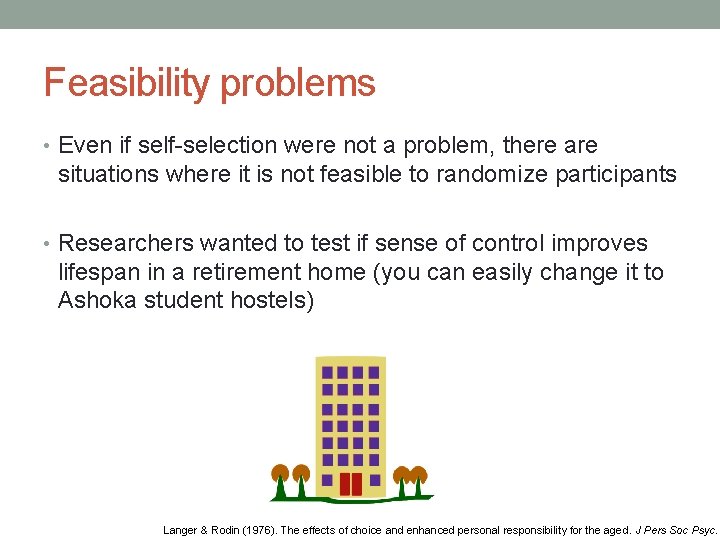
Feasibility problems • Even if self-selection were not a problem, there are situations where it is not feasible to randomize participants • Researchers wanted to test if sense of control improves lifespan in a retirement home (you can easily change it to Ashoka student hostels) Langer & Rodin (1976). The effects of choice and enhanced personal responsibility for the aged. J Pers Soc Psyc.
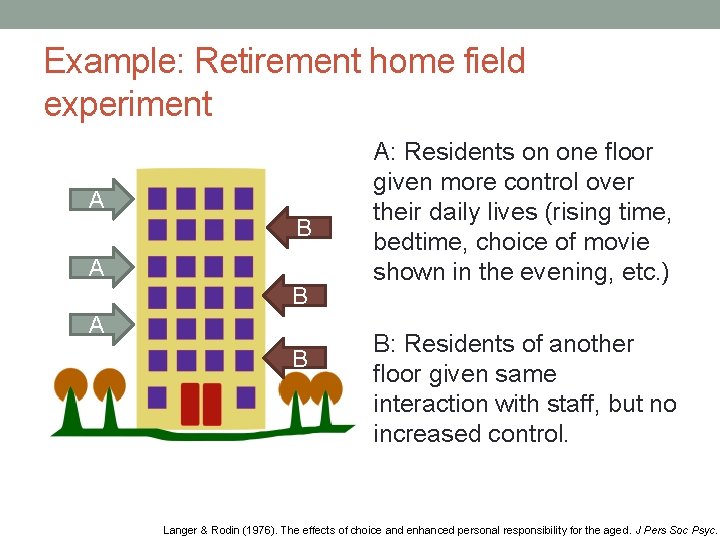
Example: Retirement home field experiment A B A B A: Residents on one floor given more control over their daily lives (rising time, bedtime, choice of movie shown in the evening, etc. ) B: Residents of another floor given same interaction with staff, but no increased control. Langer & Rodin (1976). The effects of choice and enhanced personal responsibility for the aged. J Pers Soc Psyc.
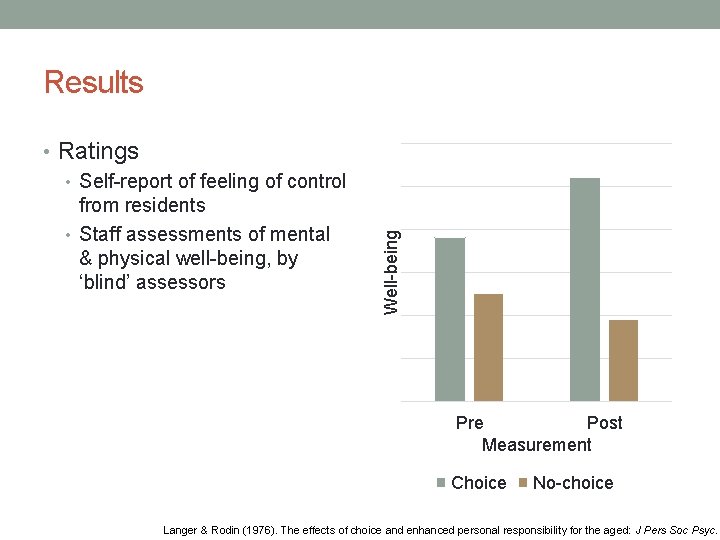
• Ratings • Self-report of feeling of control from residents • Staff assessments of mental & physical well-being, by ‘blind’ assessors Well-being Results Pre Post Measurement Choice No-choice Langer & Rodin (1976). The effects of choice and enhanced personal responsibility for the aged: J Pers Soc Psyc.
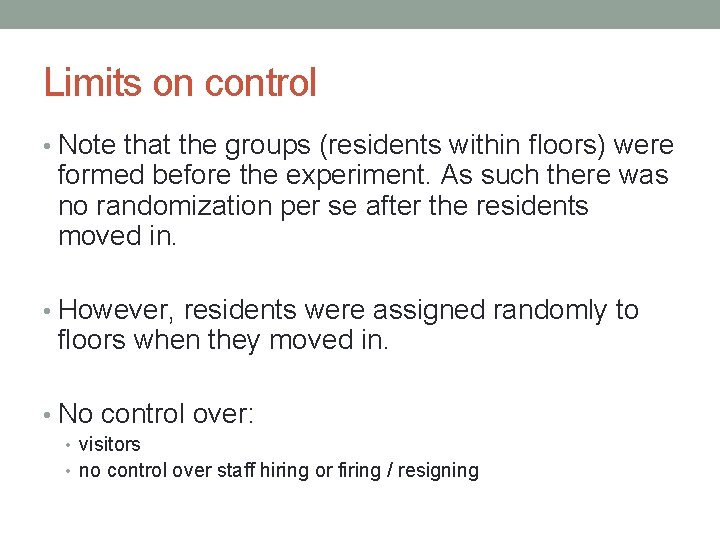
Limits on control • Note that the groups (residents within floors) were formed before the experiment. As such there was no randomization per se after the residents moved in. • However, residents were assigned randomly to floors when they moved in. • No control over: • visitors • no control over staff hiring or firing / resigning
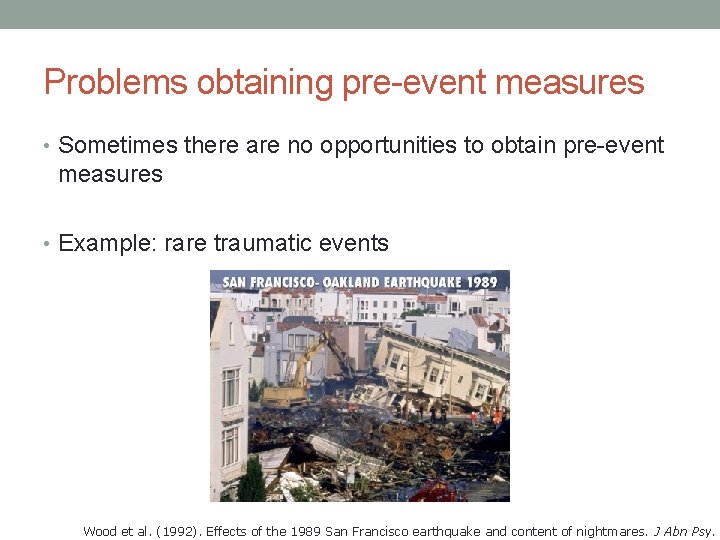
Problems obtaining pre-event measures • Sometimes there are no opportunities to obtain pre-event measures • Example: rare traumatic events Wood et al. (1992). Effects of the 1989 San Francisco earthquake and content of nightmares. J Abn Psy.
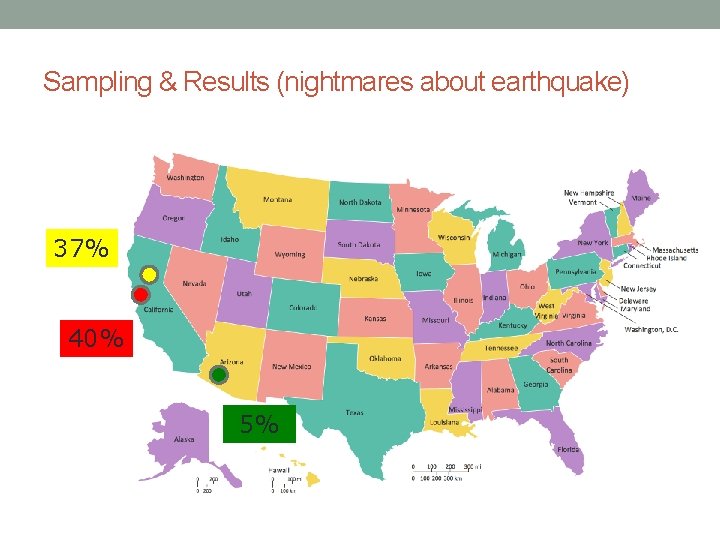
Sampling & Results (nightmares about earthquake) 37% 40% 5%
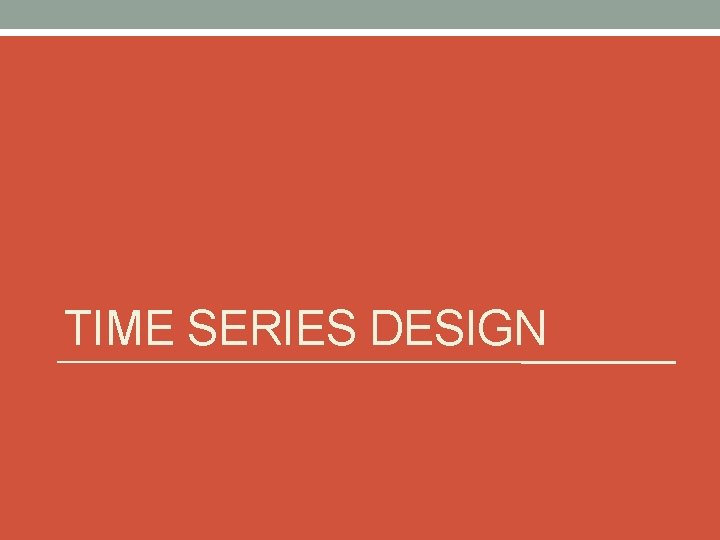
TIME SERIES DESIGN
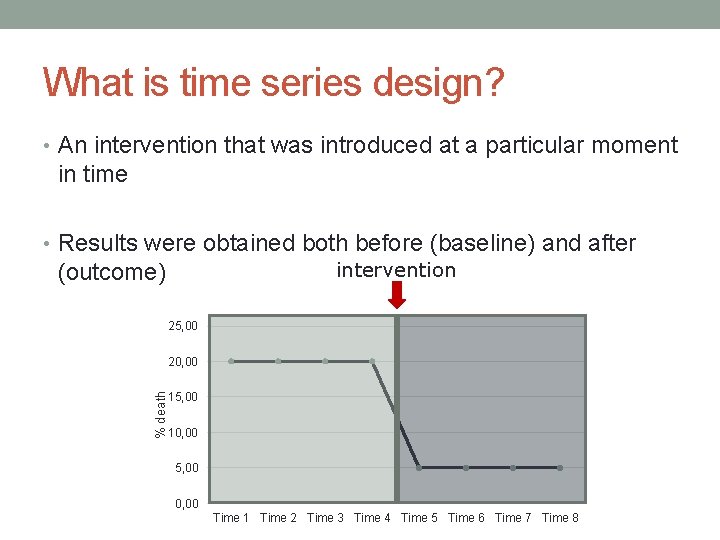
What is time series design? • An intervention that was introduced at a particular moment in time • Results were obtained both before (baseline) and after intervention (outcome) 25, 00 % death 20, 00 15, 00 10, 00 5, 00 0, 00 Time 1 Time 2 Time 3 Time 4 Time 5 Time 6 Time 7 Time 8
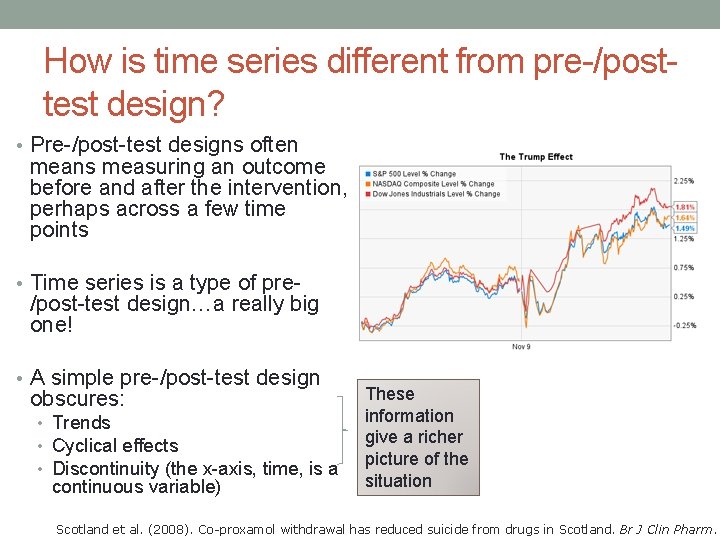
How is time series different from pre-/posttest design? • Pre-/post-test designs often means measuring an outcome before and after the intervention, perhaps across a few time points • Time series is a type of pre- /post-test design…a really big one! • A simple pre-/post-test design obscures: • Trends • Cyclical effects • Discontinuity (the x-axis, time, is a continuous variable) These information give a richer picture of the situation Scotland et al. (2008). Co-proxamol withdrawal has reduced suicide from drugs in Scotland. Br J Clin Pharm.
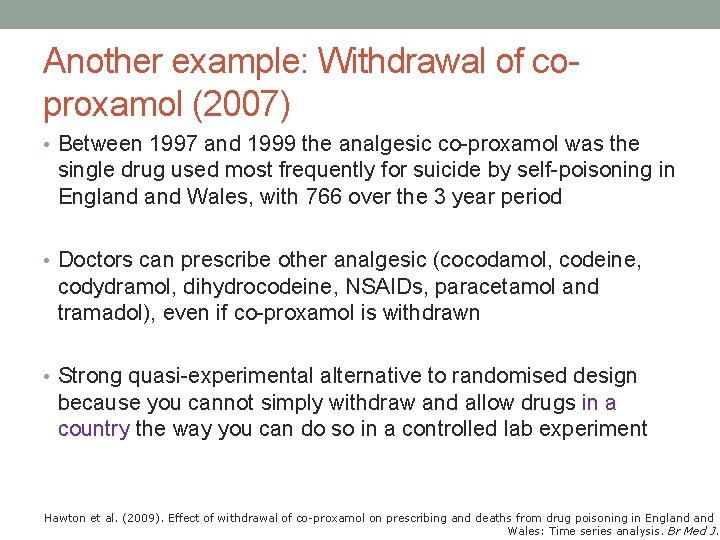
Another example: Withdrawal of coproxamol (2007) • Between 1997 and 1999 the analgesic co-proxamol was the single drug used most frequently for suicide by self-poisoning in England Wales, with 766 over the 3 year period • Doctors can prescribe other analgesic (cocodamol, codeine, codydramol, dihydrocodeine, NSAIDs, paracetamol and tramadol), even if co-proxamol is withdrawn • Strong quasi-experimental alternative to randomised design because you cannot simply withdraw and allow drugs in a country the way you can do so in a controlled lab experiment Hawton et al. (2009). Effect of withdrawal of co-proxamol on prescribing and deaths from drug poisoning in England Wales: Time series analysis. Br Med J.
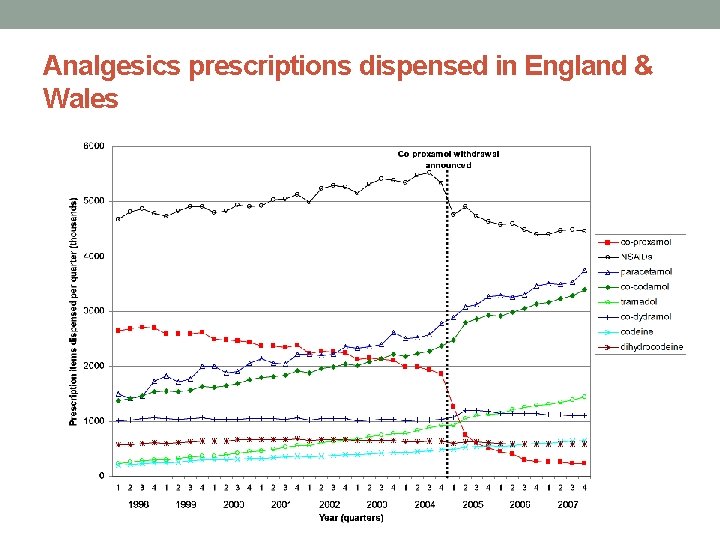
Analgesics prescriptions dispensed in England & Wales
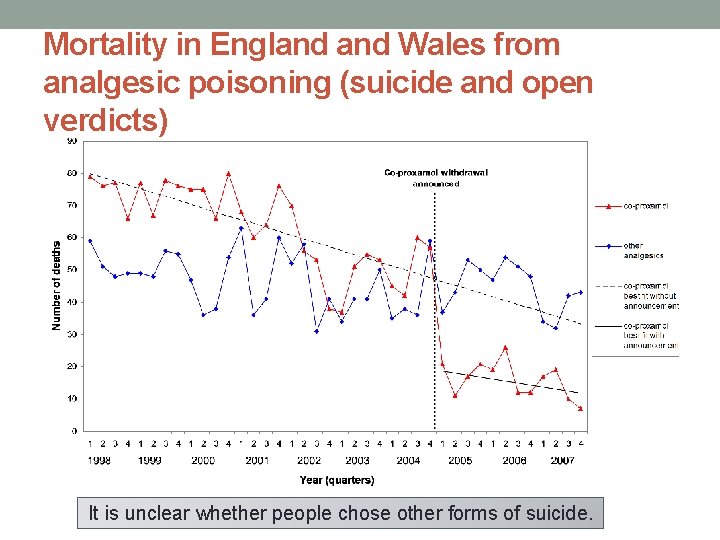
Mortality in England Wales from analgesic poisoning (suicide and open verdicts) It is unclear whether people chose other forms of suicide.
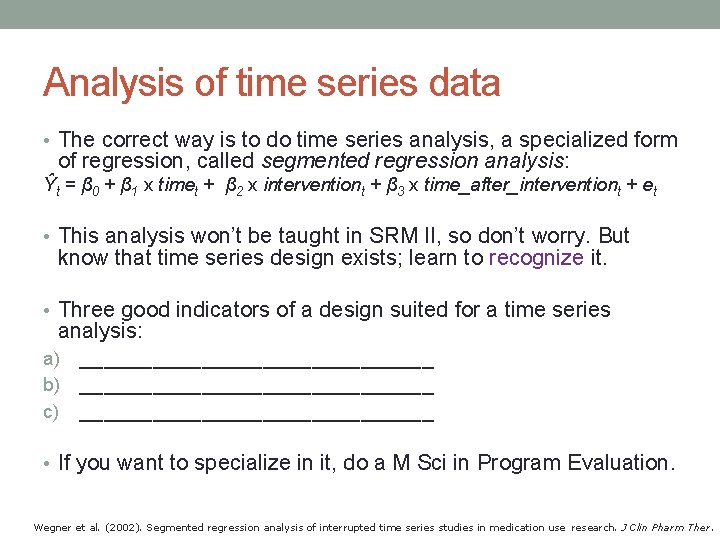
Analysis of time series data • The correct way is to do time series analysis, a specialized form of regression, called segmented regression analysis: Ŷt = β 0 + β 1 x timet + β 2 x interventiont + β 3 x time_after_interventiont + et • This analysis won’t be taught in SRM II, so don’t worry. But know that time series design exists; learn to recognize it. • Three good indicators of a design suited for a time series analysis: a) _______________ b) _______________ c) _______________ • If you want to specialize in it, do a M Sci in Program Evaluation. Wegner et al. (2002). Segmented regression analysis of interrupted time series studies in medication use research. J Clin Pharm Ther.
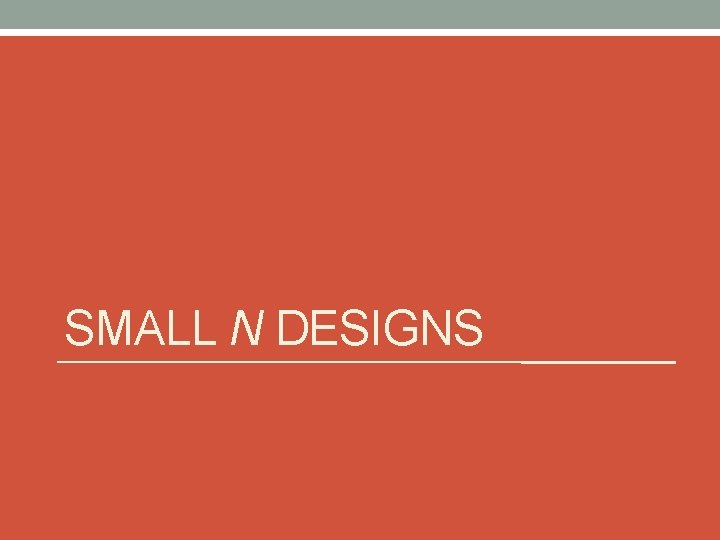
SMALL N DESIGNS
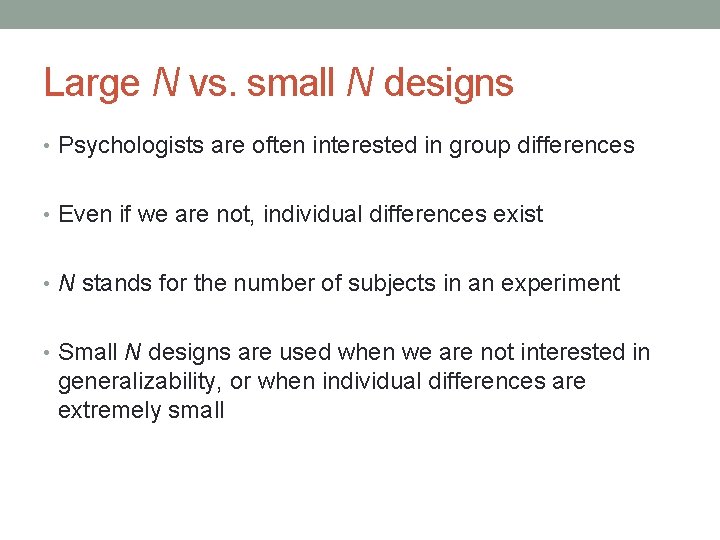
Large N vs. small N designs • Psychologists are often interested in group differences • Even if we are not, individual differences exist • N stands for the number of subjects in an experiment • Small N designs are used when we are not interested in generalizability, or when individual differences are extremely small
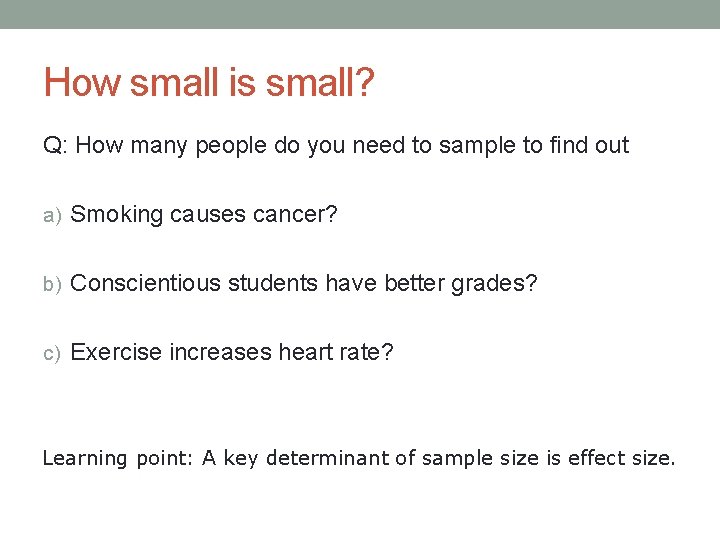
How small is small? Q: How many people do you need to sample to find out a) Smoking causes cancer? b) Conscientious students have better grades? c) Exercise increases heart rate? Learning point: A key determinant of sample size is effect size.
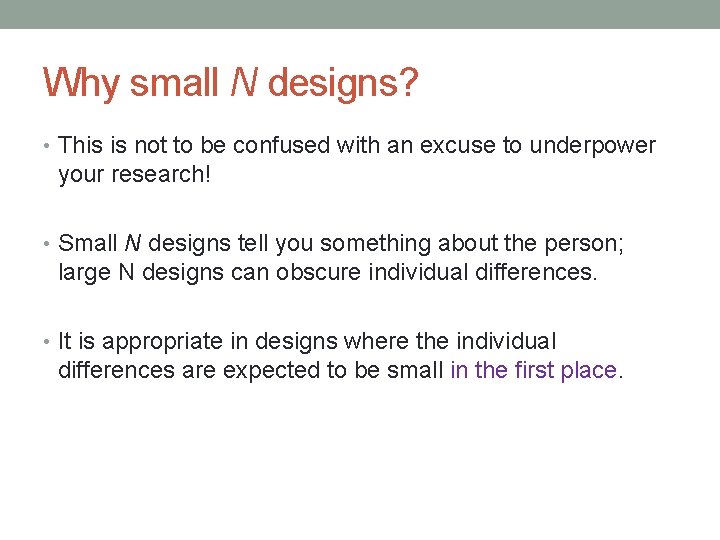
Why small N designs? • This is not to be confused with an excuse to underpower your research! • Small N designs tell you something about the person; large N designs can obscure individual differences. • It is appropriate in designs where the individual differences are expected to be small in the first place.
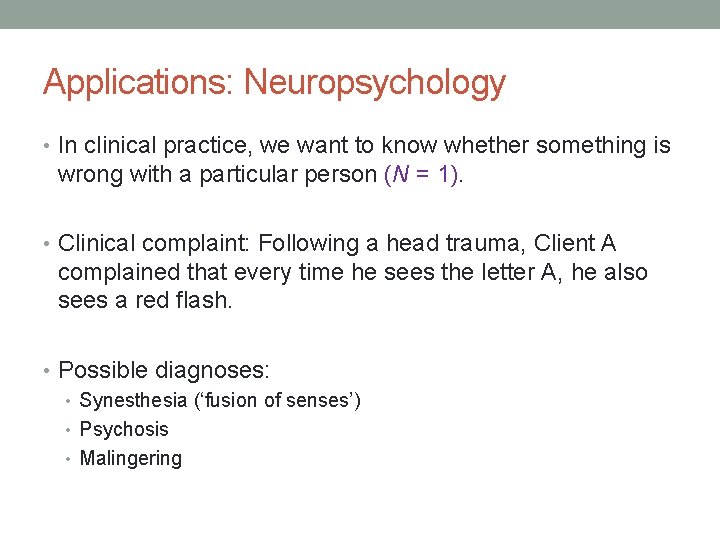
Applications: Neuropsychology • In clinical practice, we want to know whether something is wrong with a particular person (N = 1). • Clinical complaint: Following a head trauma, Client A complained that every time he sees the letter A, he also sees a red flash. • Possible diagnoses: • Synesthesia (‘fusion of senses’) • Psychosis • Malingering
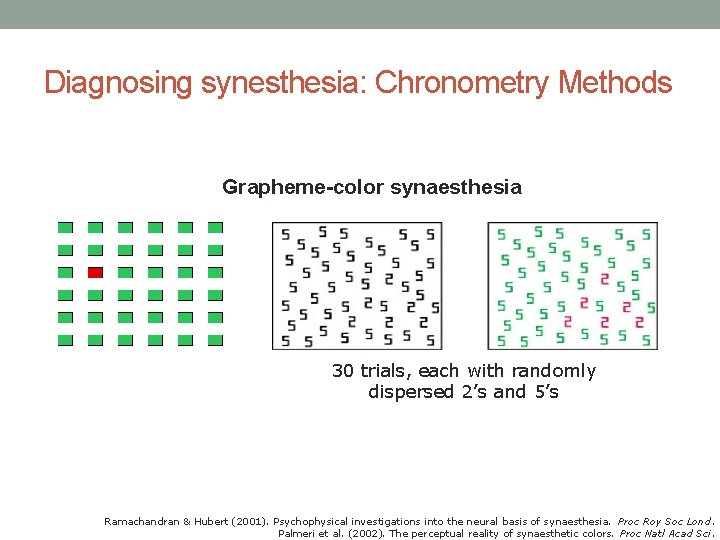
Diagnosing synesthesia: Chronometry Methods Grapheme-color synaesthesia 30 trials, each with randomly dispersed 2’s and 5’s Ramachandran & Hubert (2001). Psychophysical investigations into the neural basis of synaesthesia. Proc Roy Soc Lond. Palmeri et al. (2002). The perceptual reality of synaesthetic colors. Proc Natl Acad Sci.
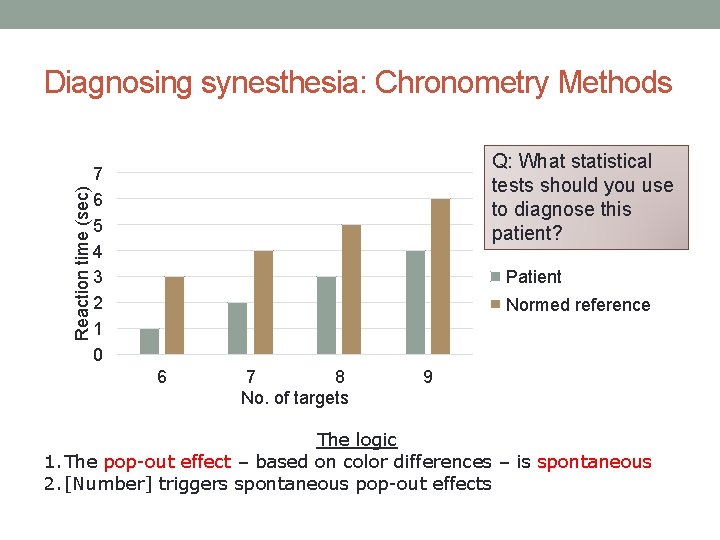
Reaction time (sec) Diagnosing synesthesia: Chronometry Methods Q: What statistical tests should you use to diagnose this patient? 7 6 5 4 3 2 1 0 Patient Normed reference 6 7 8 No. of targets 9 The logic 1. The pop-out effect – based on color differences – is spontaneous 2. [Number] triggers spontaneous pop-out effects
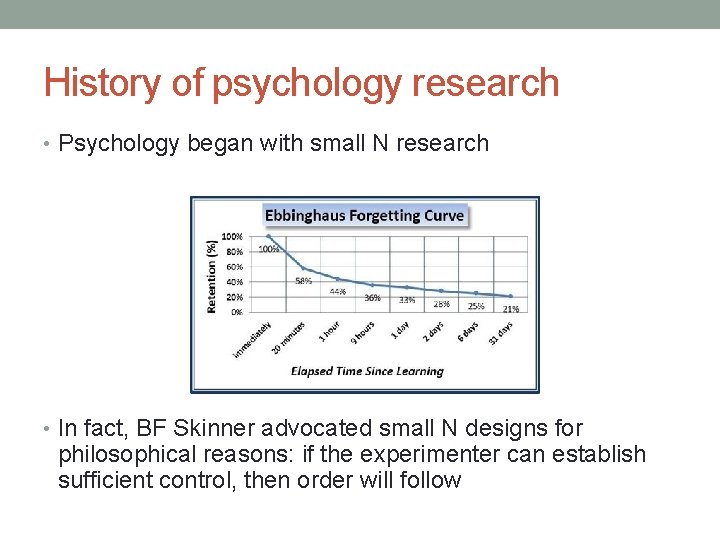
History of psychology research • Psychology began with small N research • In fact, BF Skinner advocated small N designs for philosophical reasons: if the experimenter can establish sufficient control, then order will follow
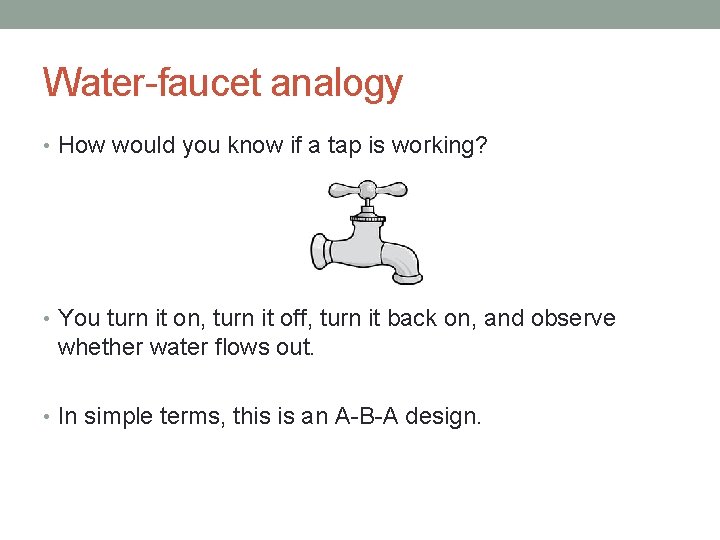
Water-faucet analogy • How would you know if a tap is working? • You turn it on, turn it off, turn it back on, and observe whether water flows out. • In simple terms, this is an A-B-A design.
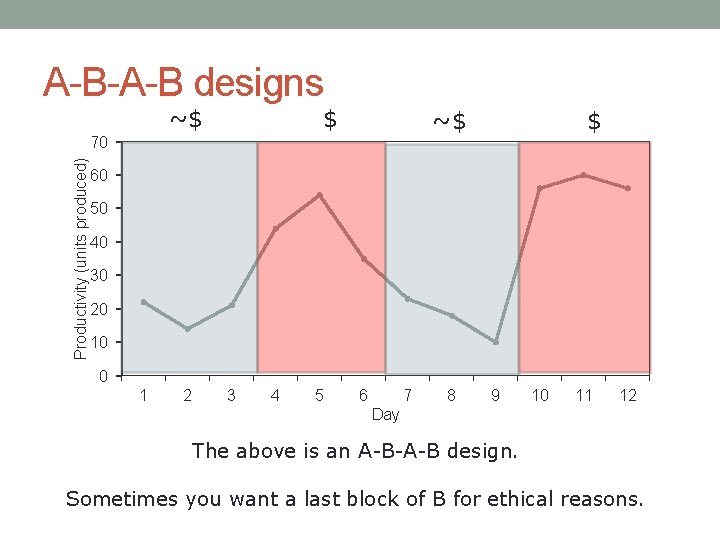
A-B-A-B designs $ ~$ 70 Productivity (units produced) $ ~$ 60 50 40 30 20 10 0 1 2 3 4 5 6 7 8 9 10 11 12 Day The above is an A-B-A-B design. Sometimes you want a last block of B for ethical reasons.
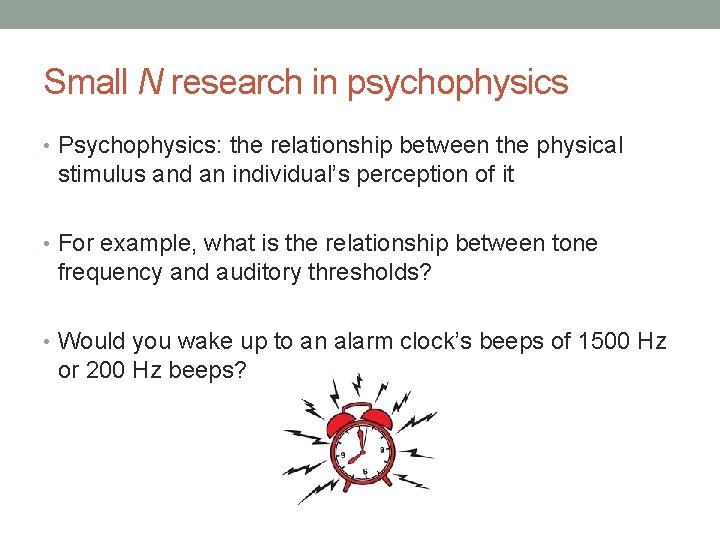
Small N research in psychophysics • Psychophysics: the relationship between the physical stimulus and an individual’s perception of it • For example, what is the relationship between tone frequency and auditory thresholds? • Would you wake up to an alarm clock’s beeps of 1500 Hz or 200 Hz beeps?
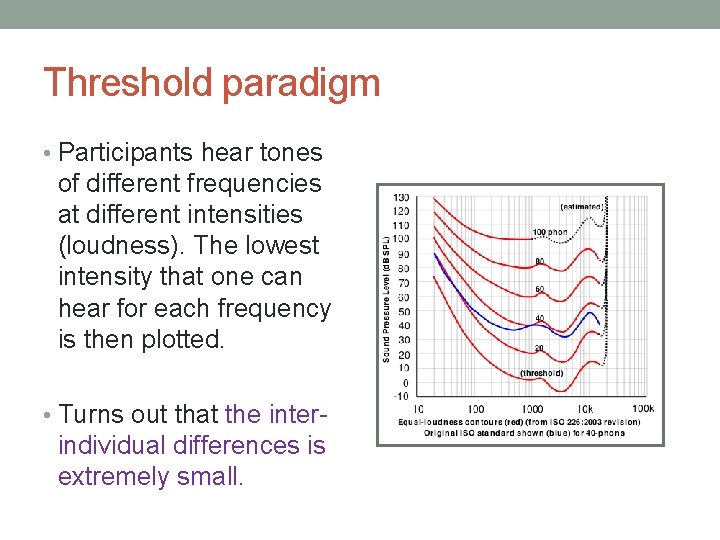
Threshold paradigm • Participants hear tones of different frequencies at different intensities (loudness). The lowest intensity that one can hear for each frequency is then plotted. • Turns out that the inter- individual differences is extremely small.
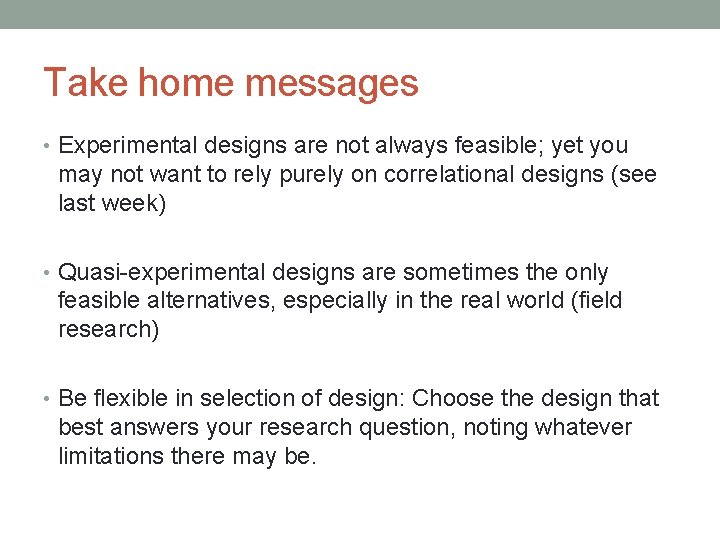
Take home messages • Experimental designs are not always feasible; yet you may not want to rely purely on correlational designs (see last week) • Quasi-experimental designs are sometimes the only feasible alternatives, especially in the real world (field research) • Be flexible in selection of design: Choose the design that best answers your research question, noting whatever limitations there may be.
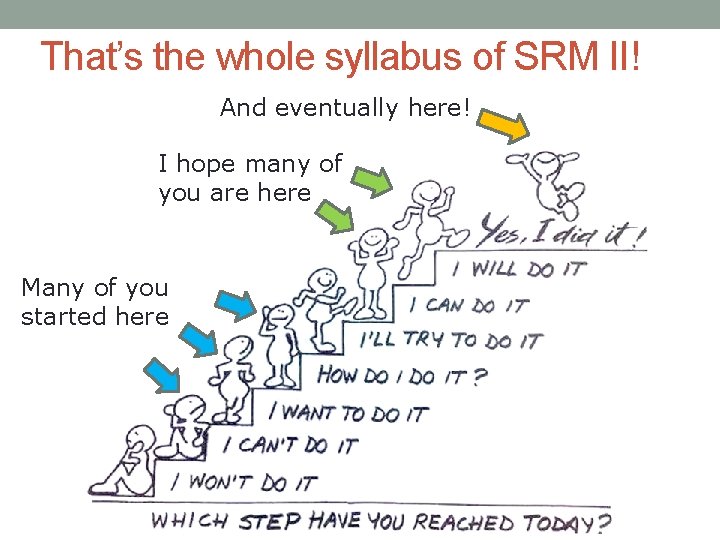
That’s the whole syllabus of SRM II! And eventually here! I hope many of you are here Many of you started here