Digital Image Processing Lecture 15 Morphological Algorithms April
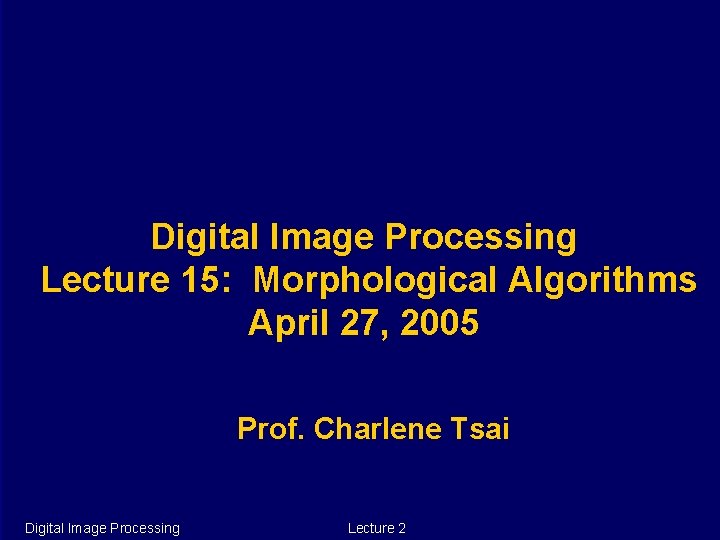
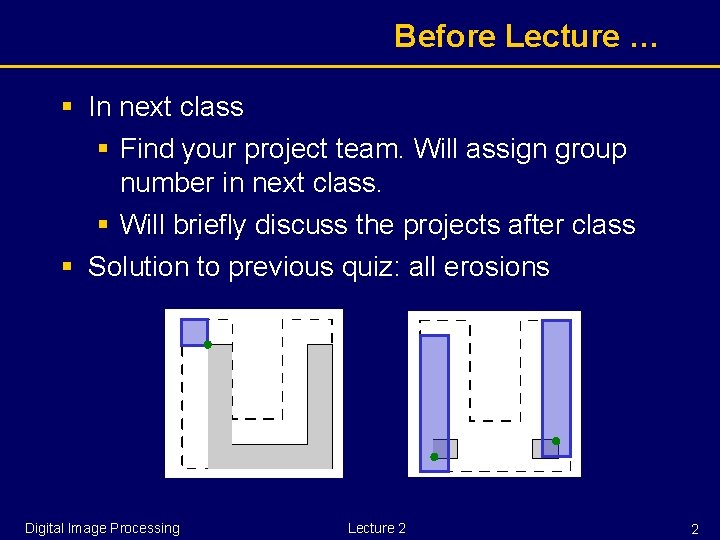
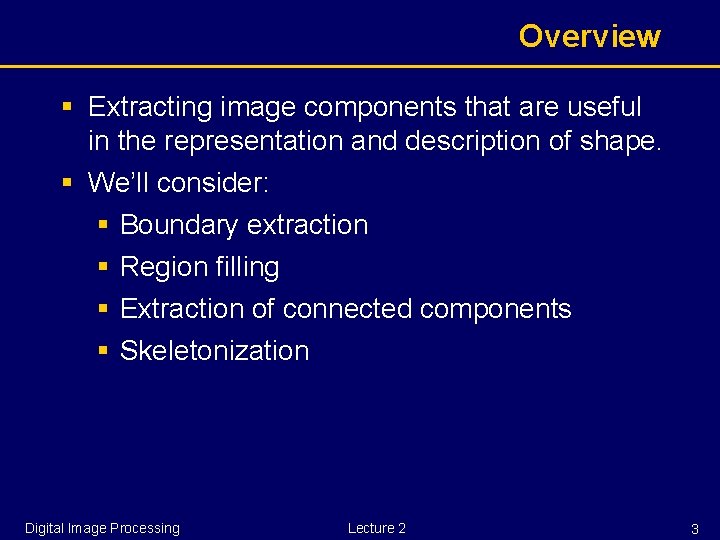
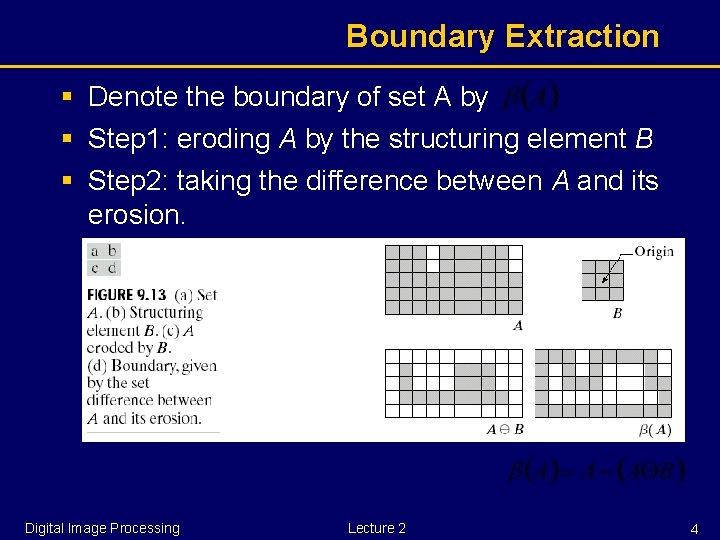
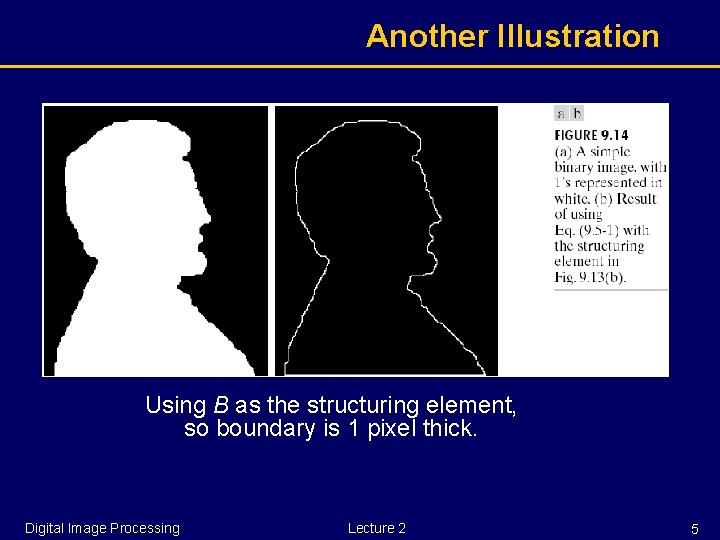
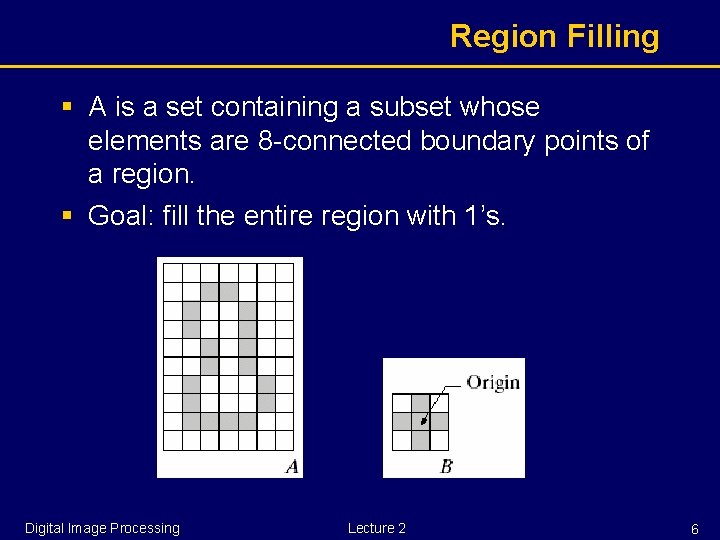
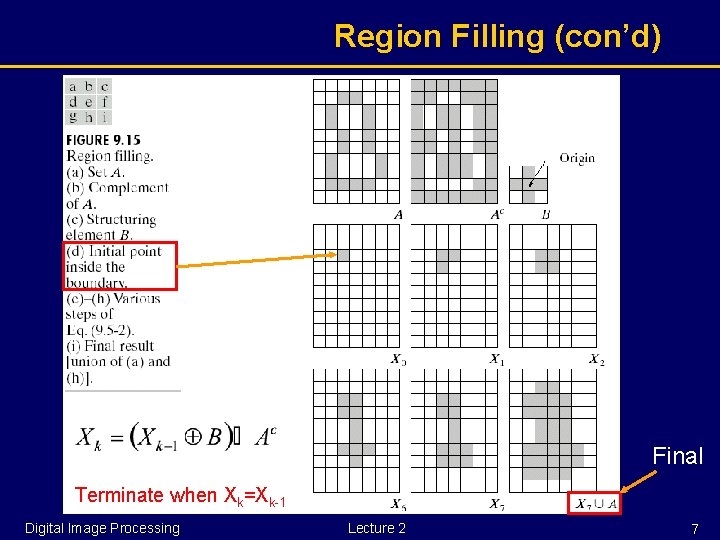
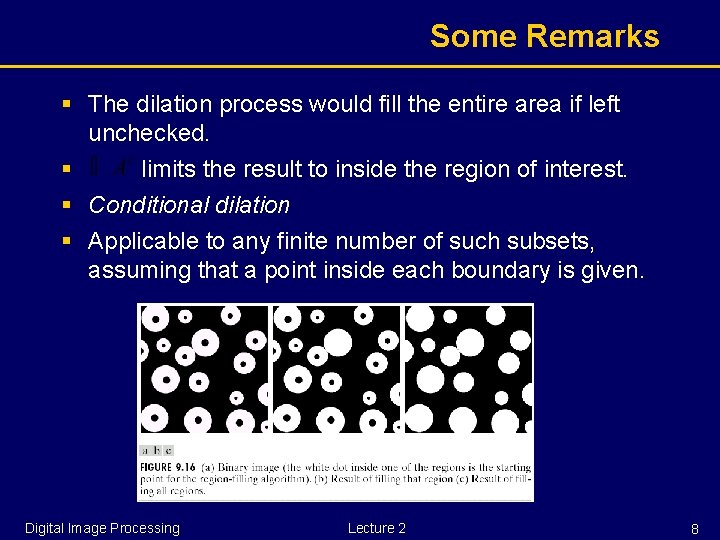
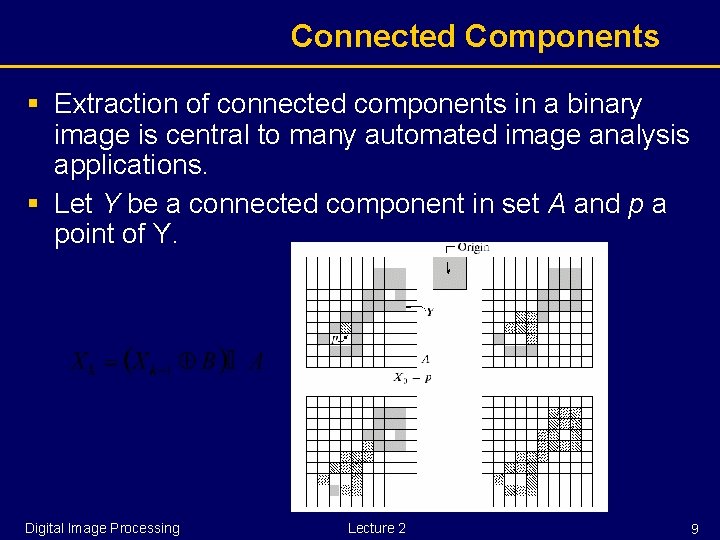
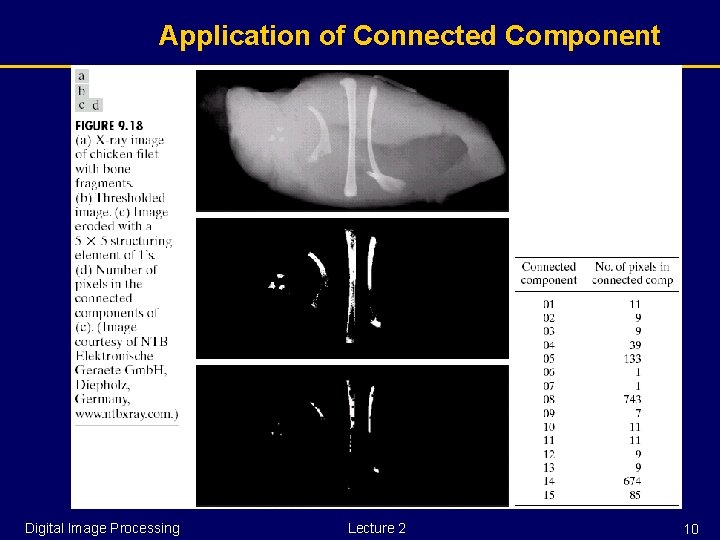
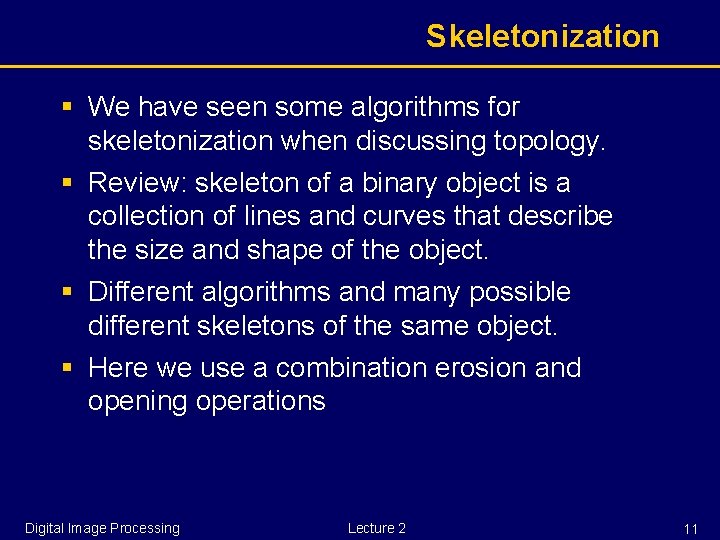
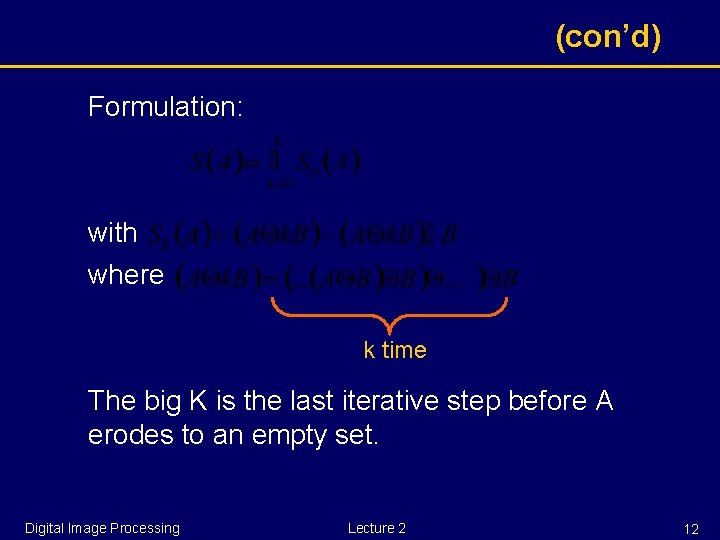
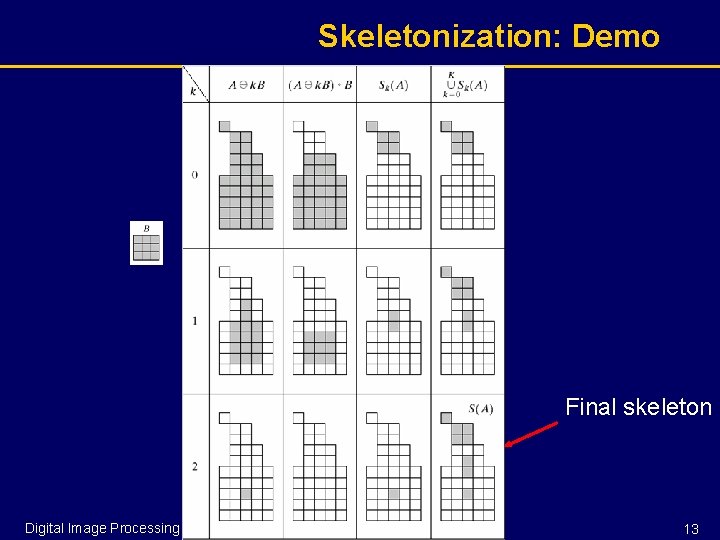
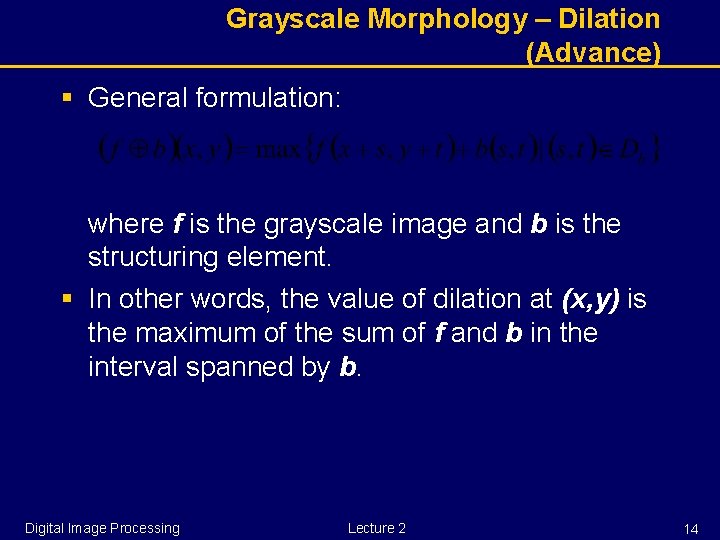
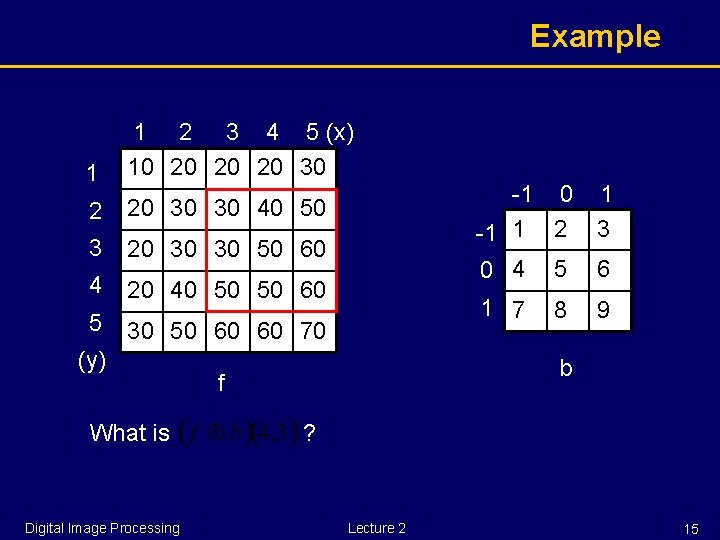
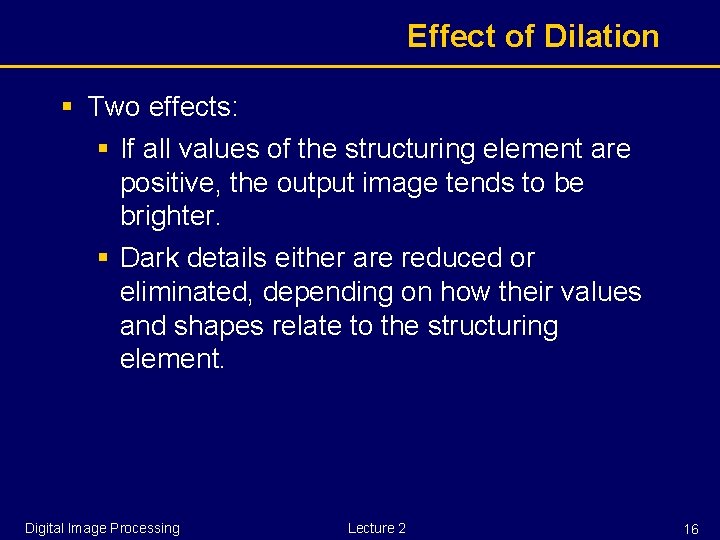
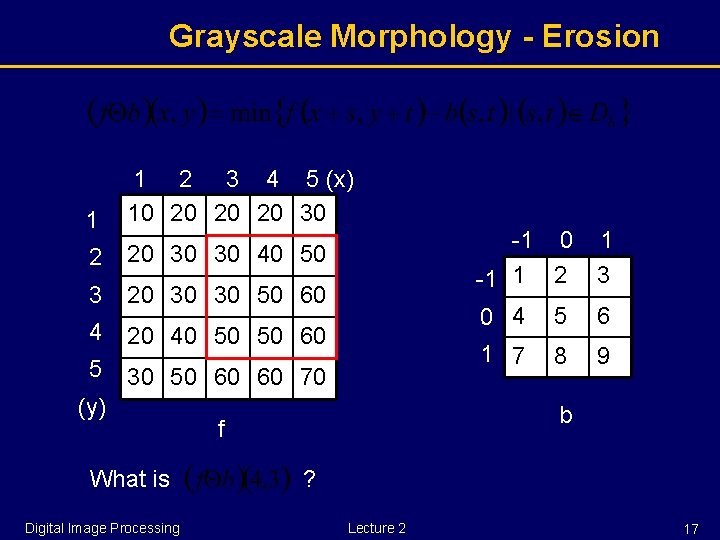
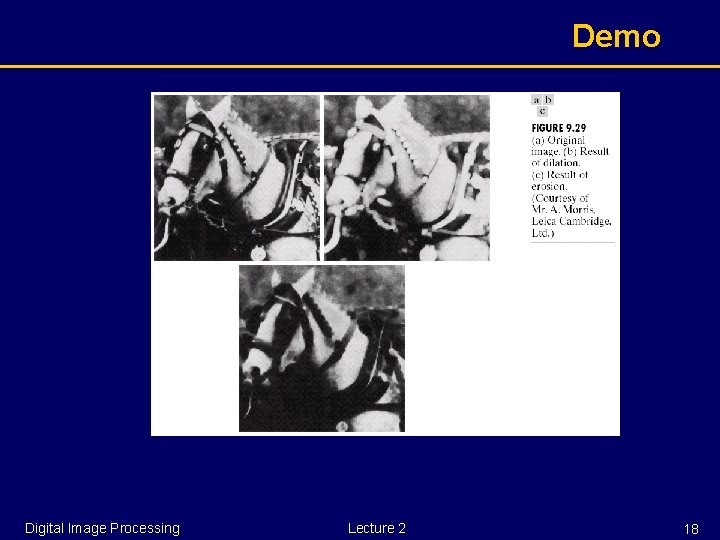
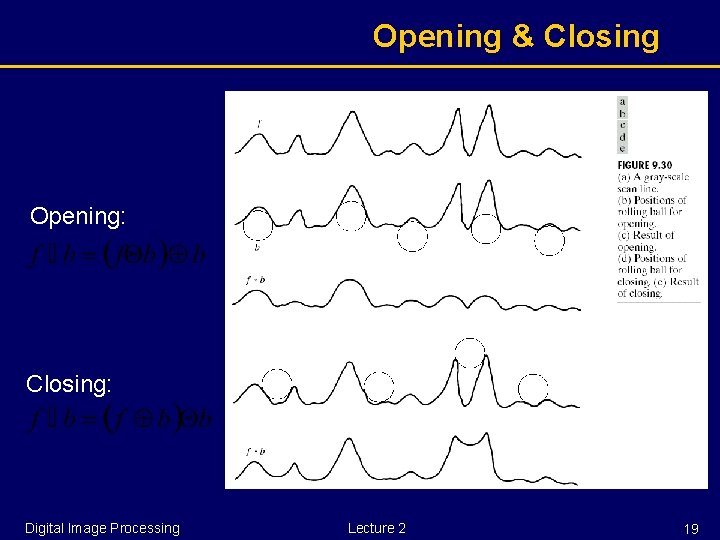
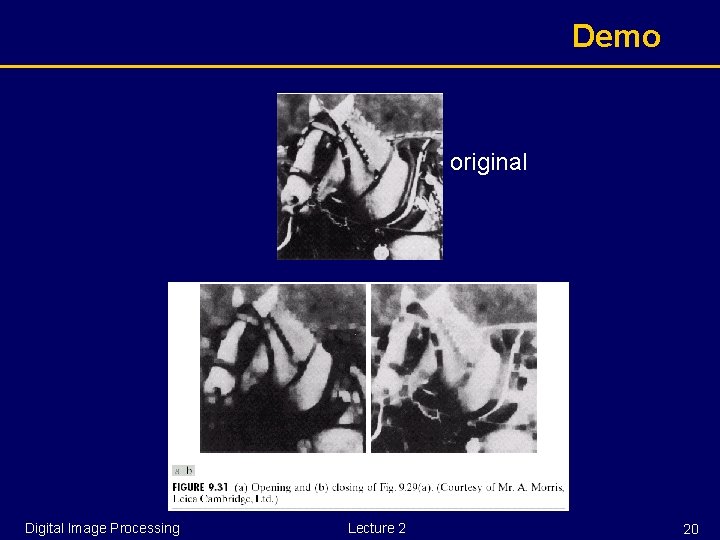
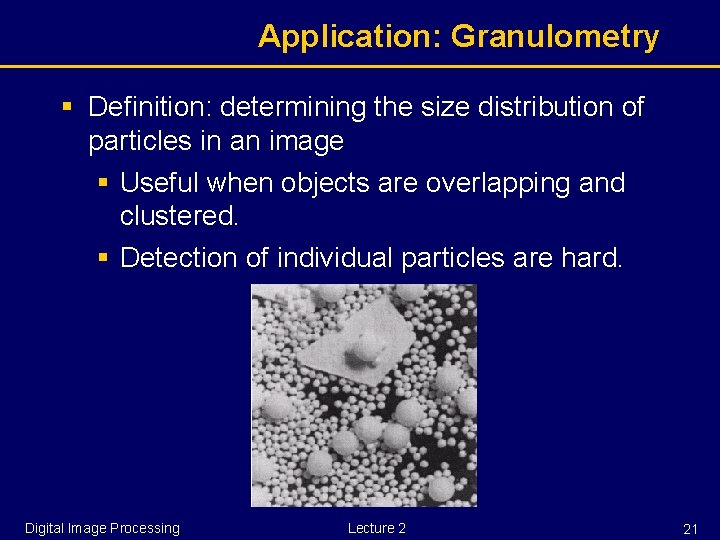
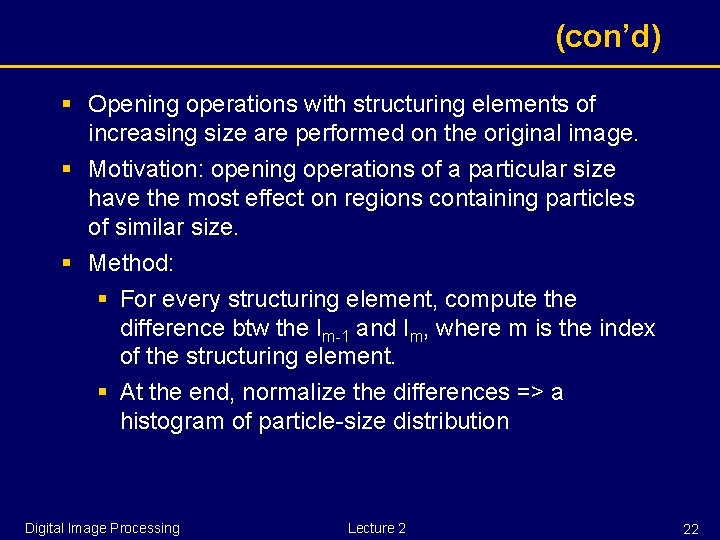
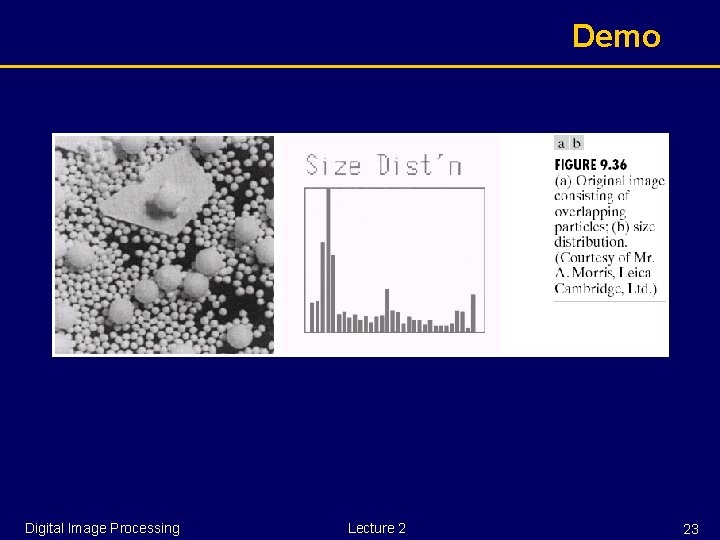
- Slides: 23
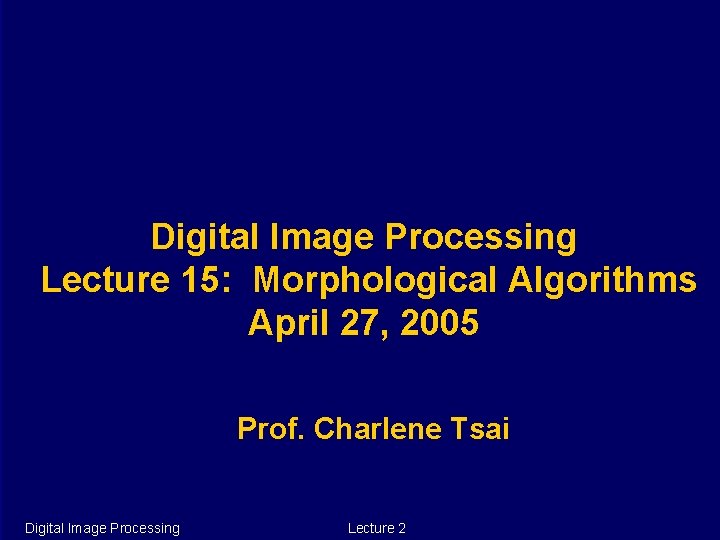
Digital Image Processing Lecture 15: Morphological Algorithms April 27, 2005 Prof. Charlene Tsai Digital Image Processing Lecture 2
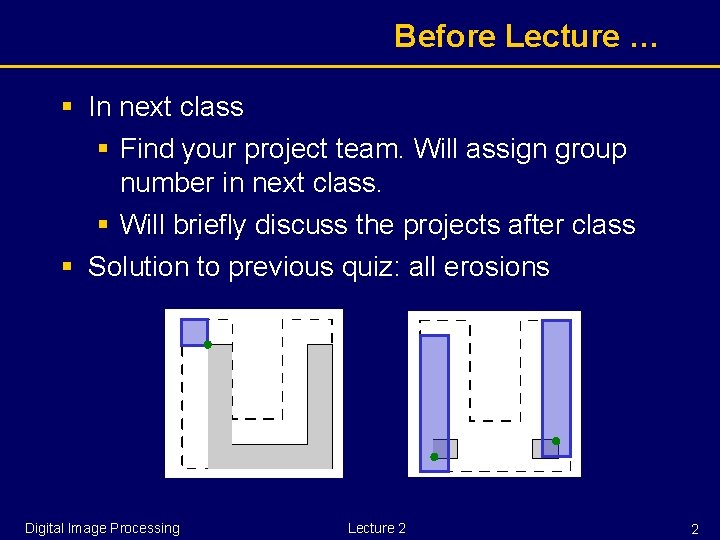
Before Lecture … § In next class § Find your project team. Will assign group number in next class. § Will briefly discuss the projects after class § Solution to previous quiz: all erosions Digital Image Processing Lecture 2 2
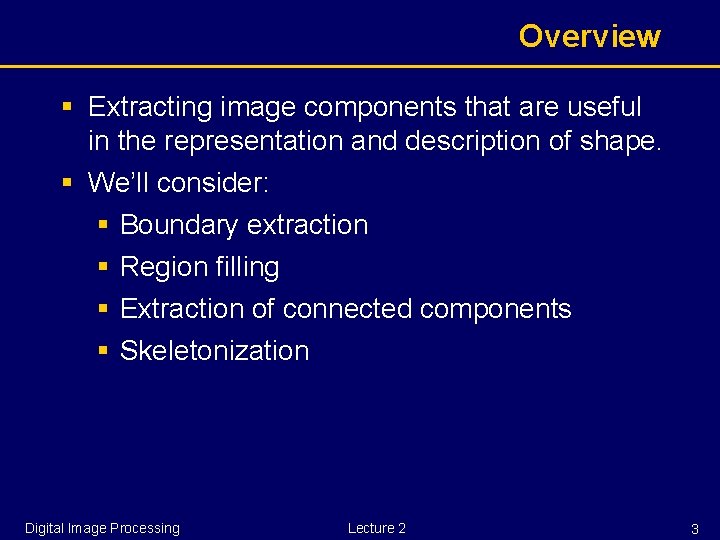
Overview § Extracting image components that are useful in the representation and description of shape. § We’ll consider: § Boundary extraction § Region filling § Extraction of connected components § Skeletonization Digital Image Processing Lecture 2 3
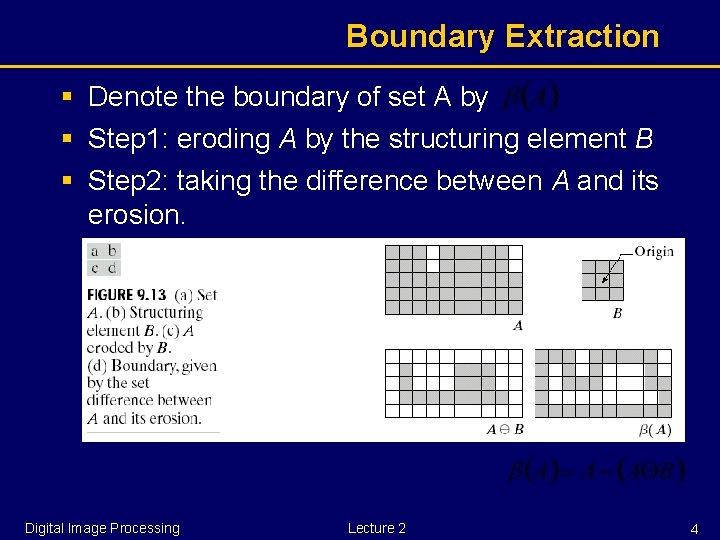
Boundary Extraction § Denote the boundary of set A by § Step 1: eroding A by the structuring element B § Step 2: taking the difference between A and its erosion. Digital Image Processing Lecture 2 4
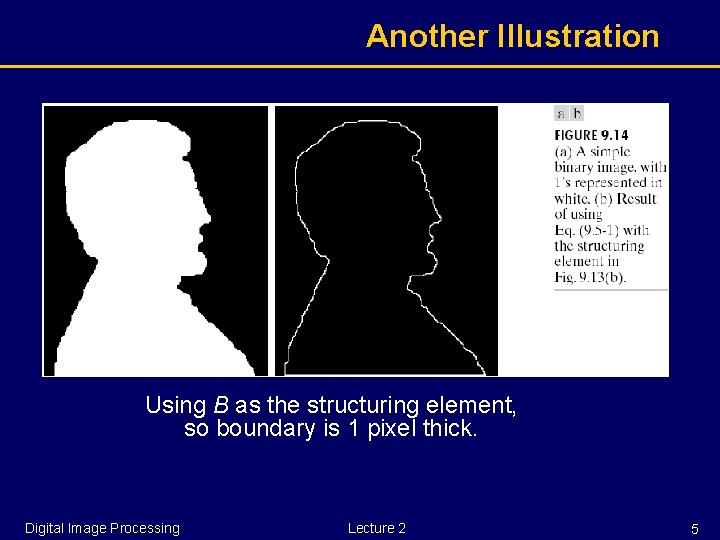
Another Illustration Using B as the structuring element, so boundary is 1 pixel thick. Digital Image Processing Lecture 2 5
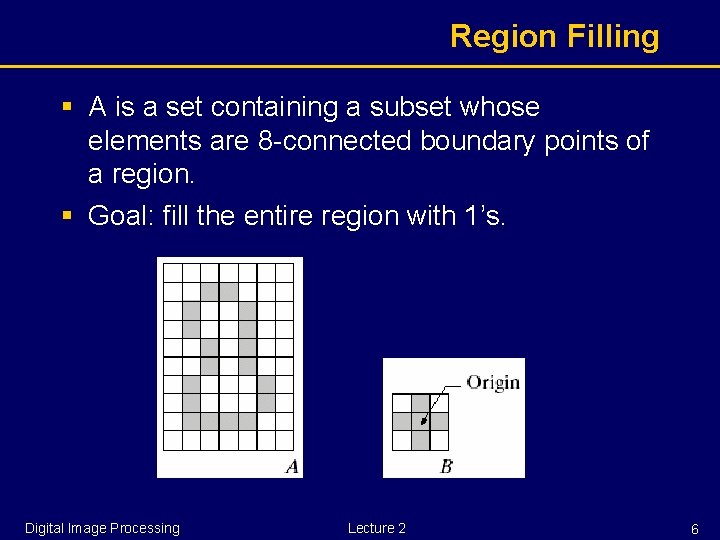
Region Filling § A is a set containing a subset whose elements are 8 -connected boundary points of a region. § Goal: fill the entire region with 1’s. Digital Image Processing Lecture 2 6
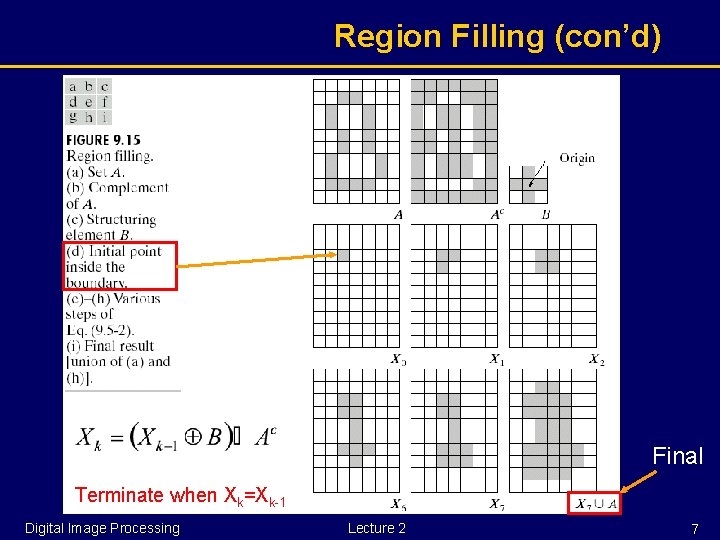
Region Filling (con’d) Final Terminate when Xk=Xk-1 Digital Image Processing Lecture 2 7
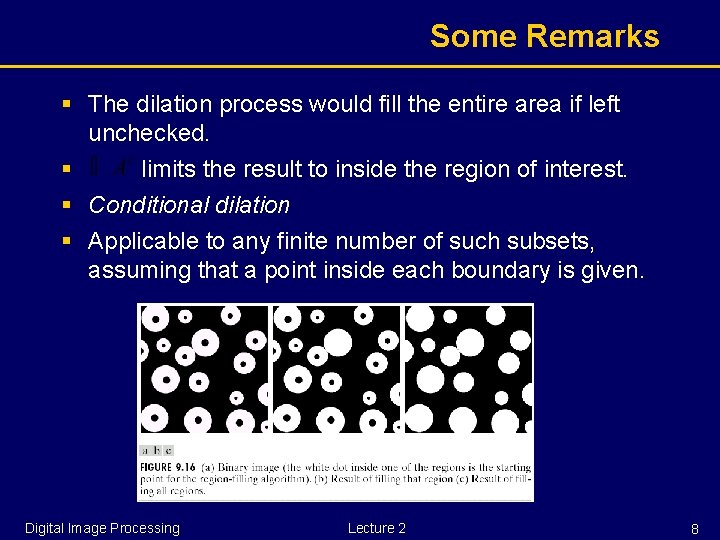
Some Remarks § The dilation process would fill the entire area if left unchecked. § limits the result to inside the region of interest. § Conditional dilation § Applicable to any finite number of such subsets, assuming that a point inside each boundary is given. Digital Image Processing Lecture 2 8
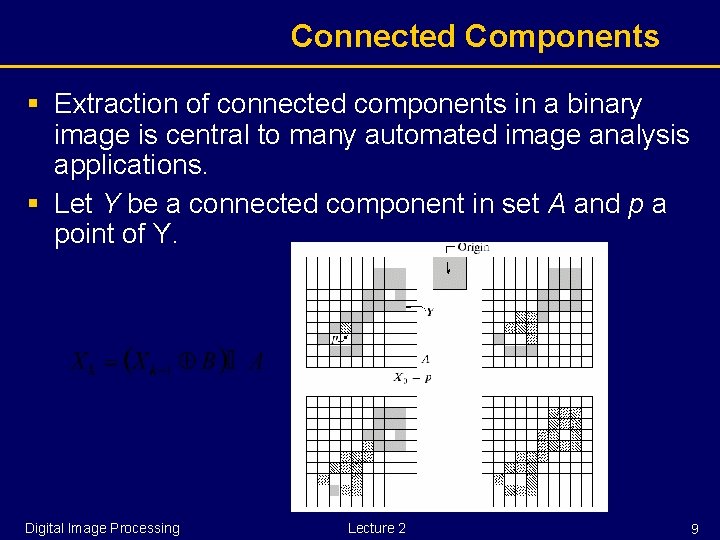
Connected Components § Extraction of connected components in a binary image is central to many automated image analysis applications. § Let Y be a connected component in set A and p a point of Y. Digital Image Processing Lecture 2 9
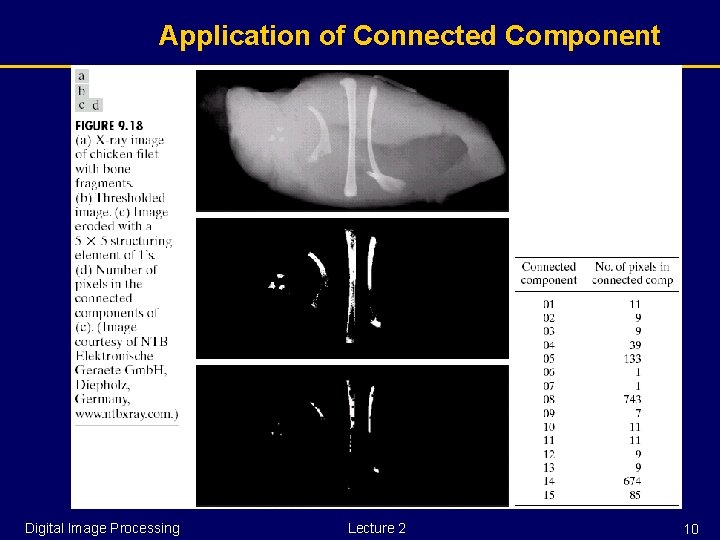
Application of Connected Component Digital Image Processing Lecture 2 10
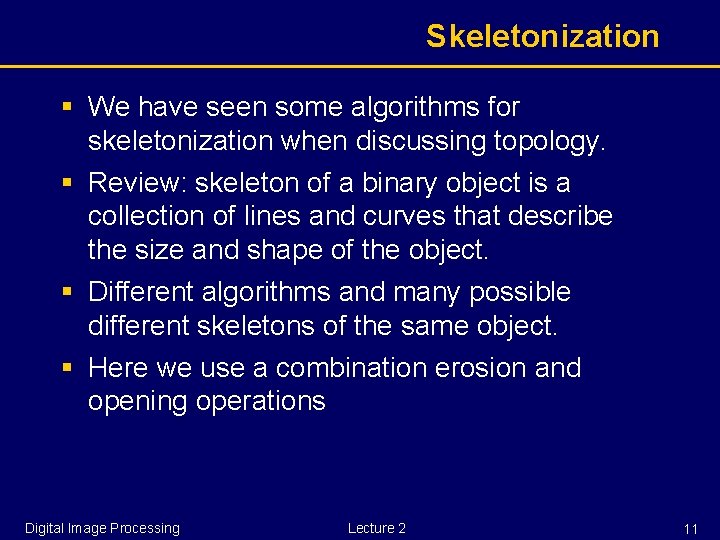
Skeletonization § We have seen some algorithms for skeletonization when discussing topology. § Review: skeleton of a binary object is a collection of lines and curves that describe the size and shape of the object. § Different algorithms and many possible different skeletons of the same object. § Here we use a combination erosion and opening operations Digital Image Processing Lecture 2 11
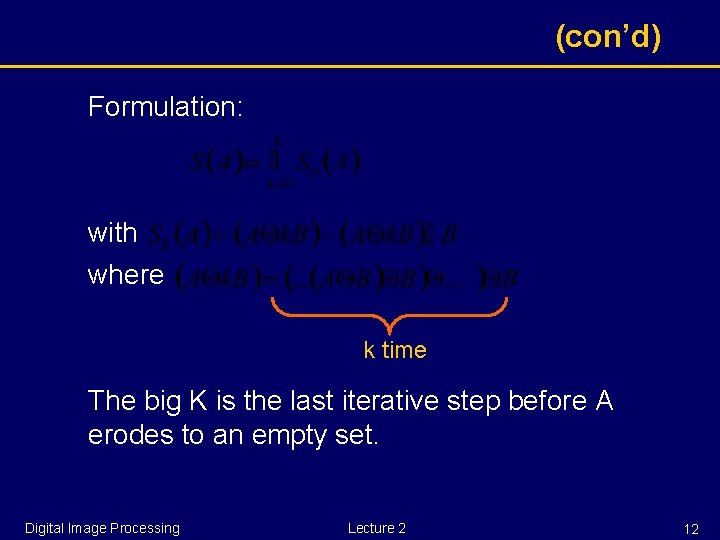
(con’d) Formulation: with where k time The big K is the last iterative step before A erodes to an empty set. Digital Image Processing Lecture 2 12
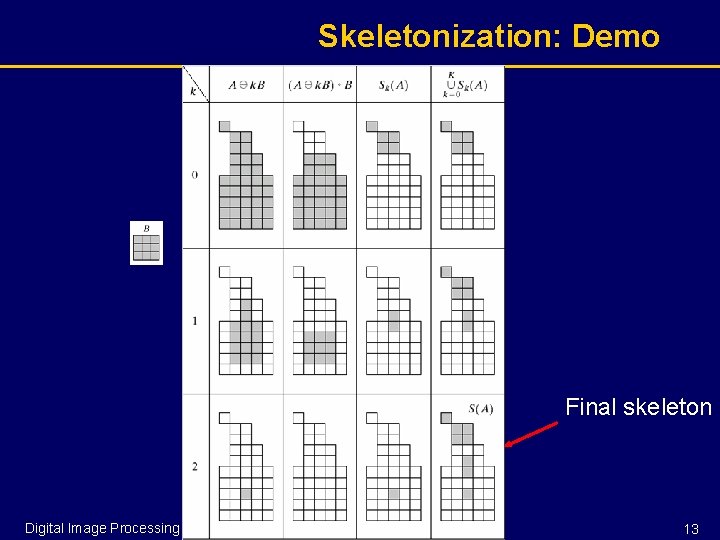
Skeletonization: Demo Final skeleton Digital Image Processing Lecture 2 13
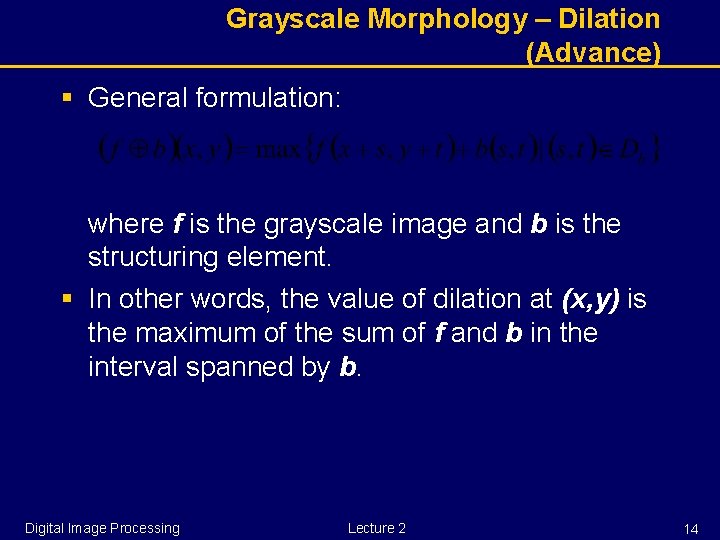
Grayscale Morphology – Dilation (Advance) § General formulation: where f is the grayscale image and b is the structuring element. § In other words, the value of dilation at (x, y) is the maximum of the sum of f and b in the interval spanned by b. Digital Image Processing Lecture 2 14
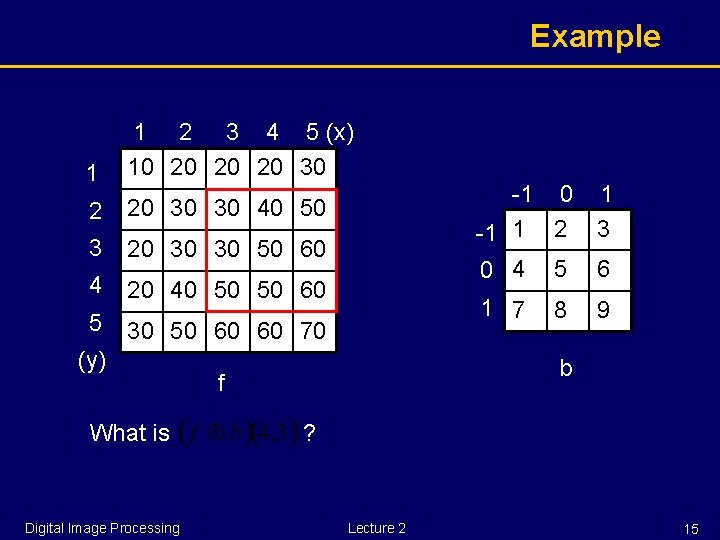
Example 1 1 2 3 4 5 (x) 10 20 20 20 30 2 20 30 30 40 50 3 20 30 30 50 60 4 20 40 50 50 60 5 30 50 60 60 70 (y) What is Digital Image Processing -1 0 1 -1 1 2 3 0 4 5 6 1 7 8 9 b f ? Lecture 2 15
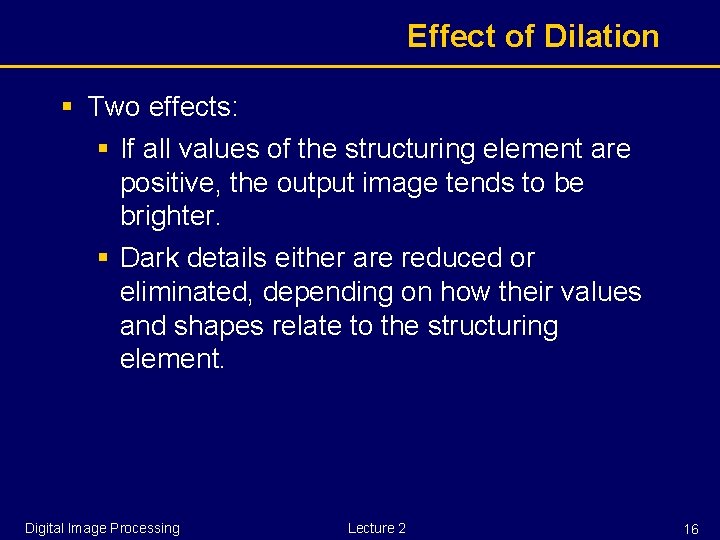
Effect of Dilation § Two effects: § If all values of the structuring element are positive, the output image tends to be brighter. § Dark details either are reduced or eliminated, depending on how their values and shapes relate to the structuring element. Digital Image Processing Lecture 2 16
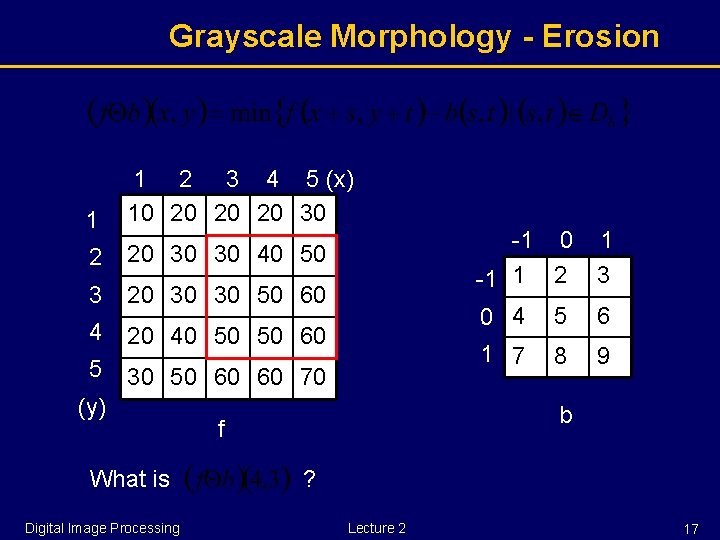
Grayscale Morphology - Erosion 1 1 2 3 4 5 (x) 10 20 20 20 30 2 20 30 30 40 50 3 20 30 30 50 60 4 20 40 50 50 60 5 30 50 60 60 70 (y) What is Digital Image Processing -1 0 1 -1 1 2 3 0 4 5 6 1 7 8 9 b f ? Lecture 2 17
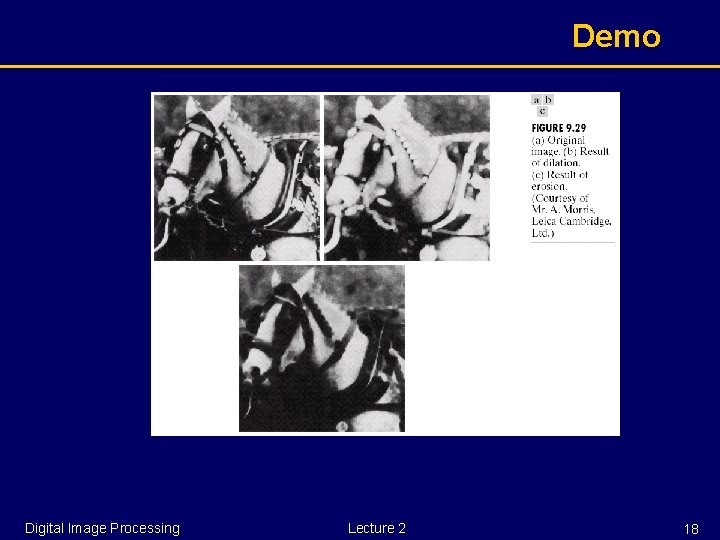
Demo Digital Image Processing Lecture 2 18
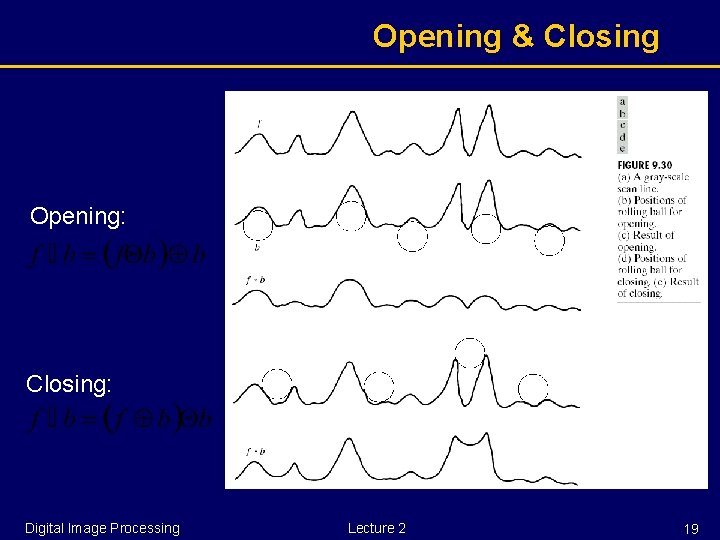
Opening & Closing Opening: Closing: Digital Image Processing Lecture 2 19
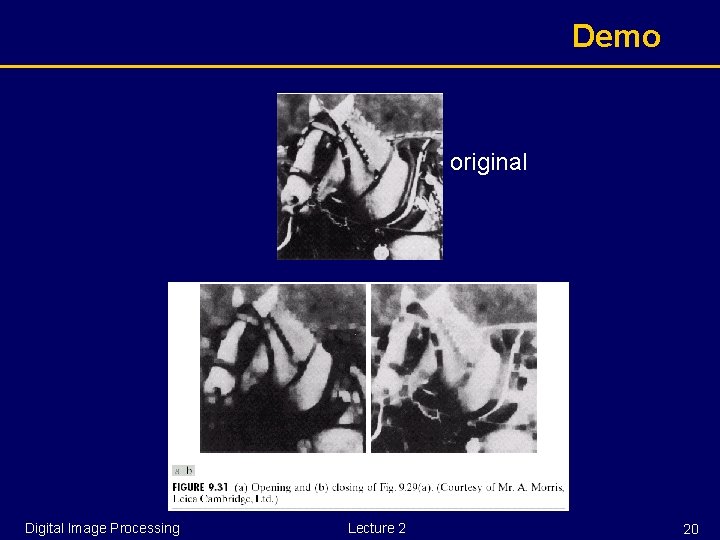
Demo original Digital Image Processing Lecture 2 20
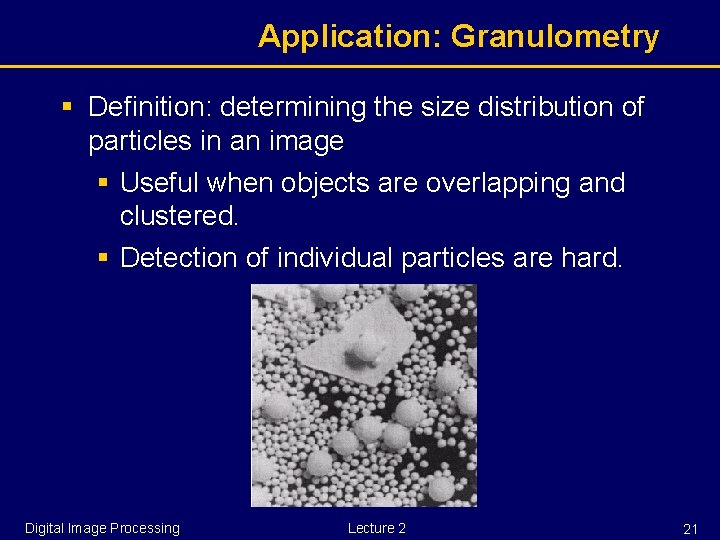
Application: Granulometry § Definition: determining the size distribution of particles in an image § Useful when objects are overlapping and clustered. § Detection of individual particles are hard. Digital Image Processing Lecture 2 21
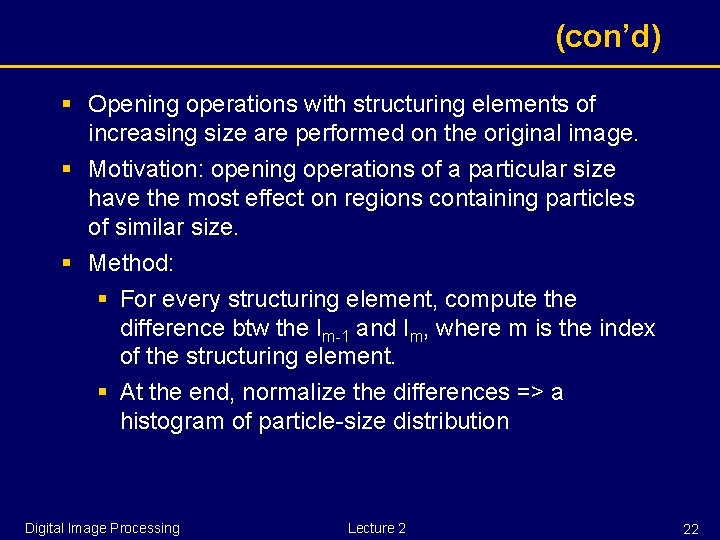
(con’d) § Opening operations with structuring elements of increasing size are performed on the original image. § Motivation: opening operations of a particular size have the most effect on regions containing particles of similar size. § Method: § For every structuring element, compute the difference btw the Im-1 and Im, where m is the index of the structuring element. § At the end, normalize the differences => a histogram of particle-size distribution Digital Image Processing Lecture 2 22
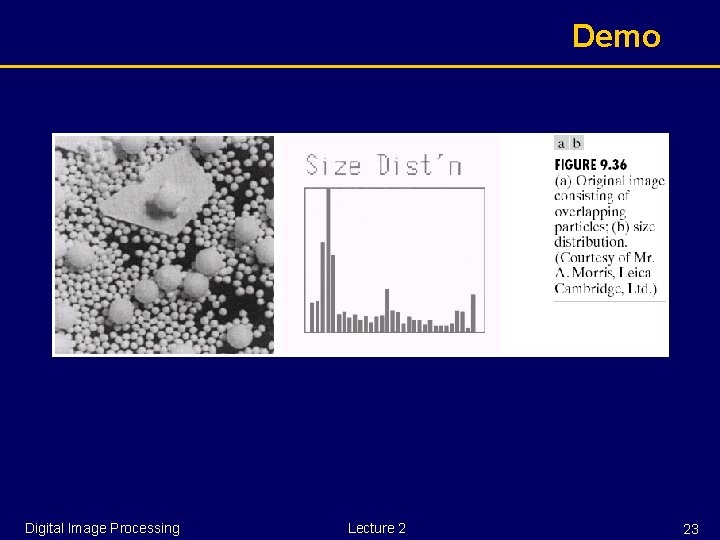
Demo Digital Image Processing Lecture 2 23
Gonzalez
Histogram processing in digital image processing
Unsharp masking matlab
Neighborhood processing in digital image processing
Point processing in image processing
Point processing
Image processing
Translate
What is image restoration in digital image processing
Fundamentals of image compression
Key stages in digital image processing
Huffman coding example
Image sharpening in digital image processing
Image geometry in digital image processing
Zooming and shrinking of digital images
Digital image processing
Imtransform matlab
Noise
Parallel image processing algorithms
Image processing lecture notes
Fluorocein
Analysis of algorithms lecture notes
Introduction to algorithms lecture notes
10 types of morphological processes