Design and evaluation of an editing and imputation
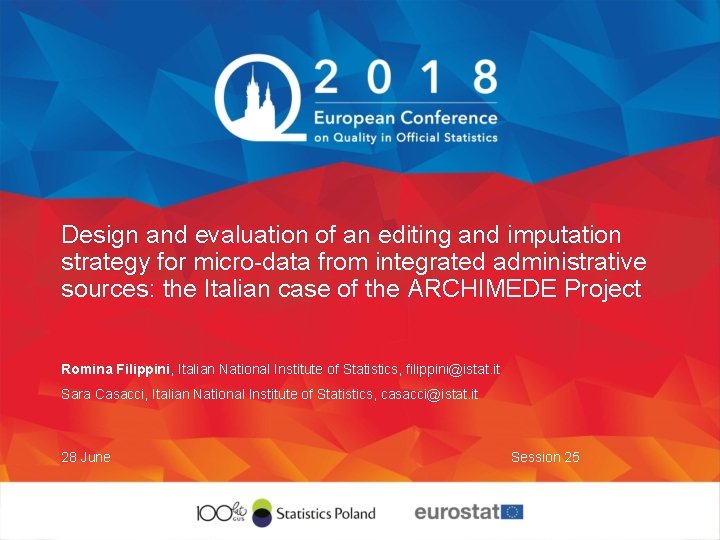
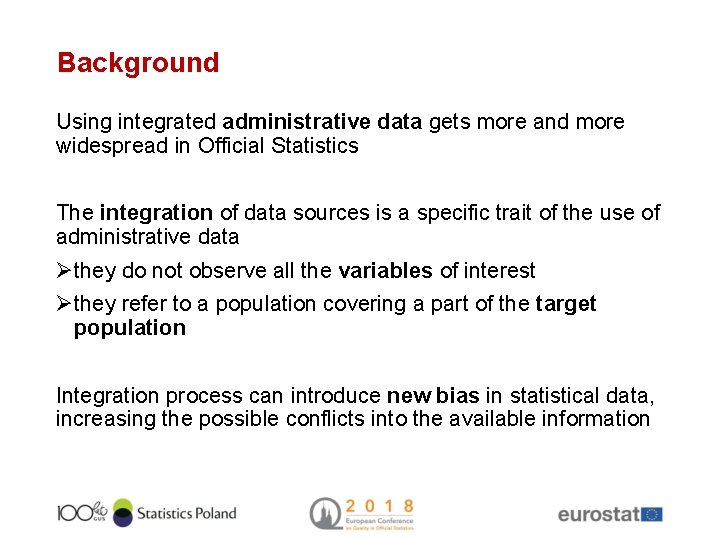
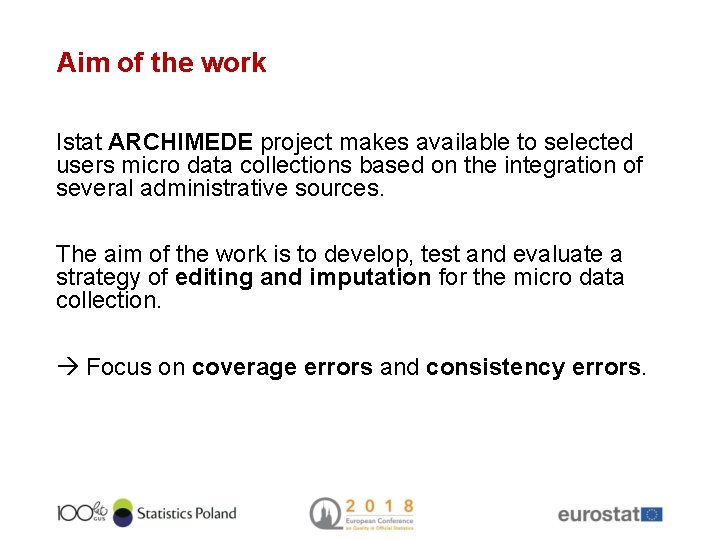
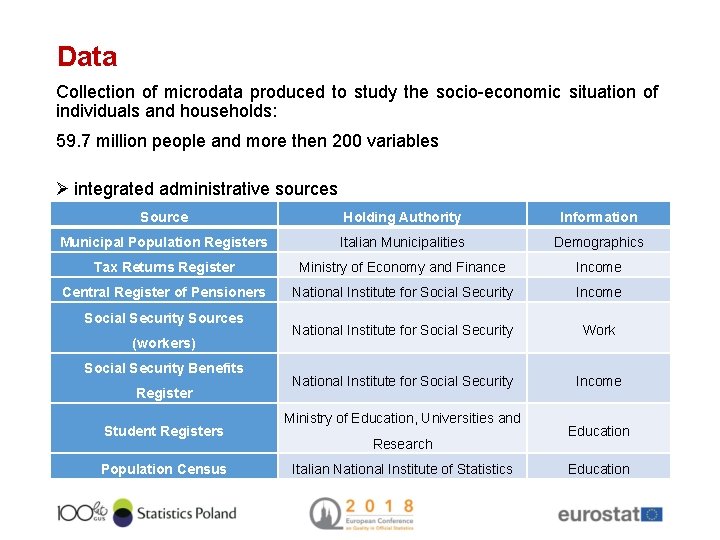
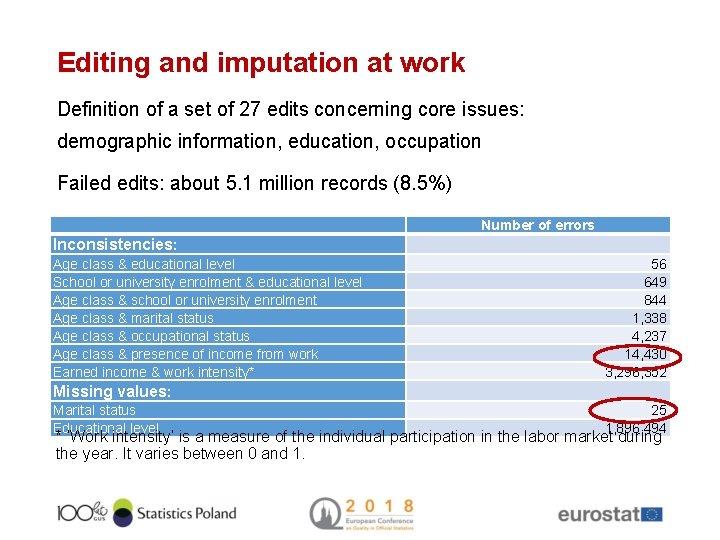
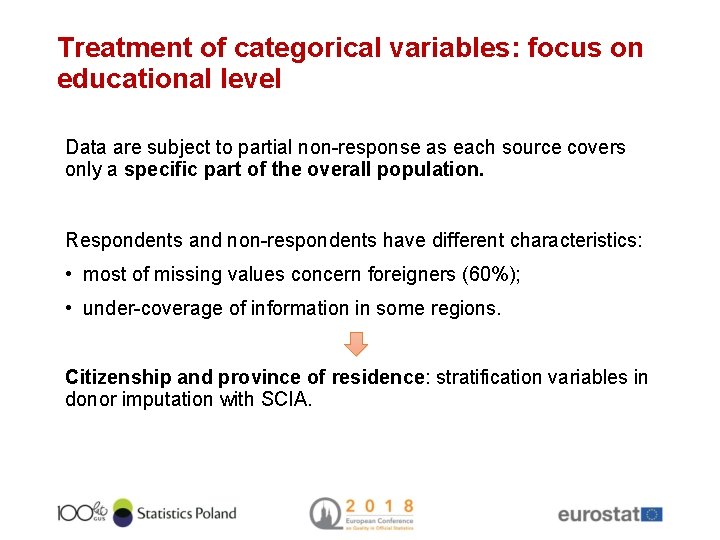
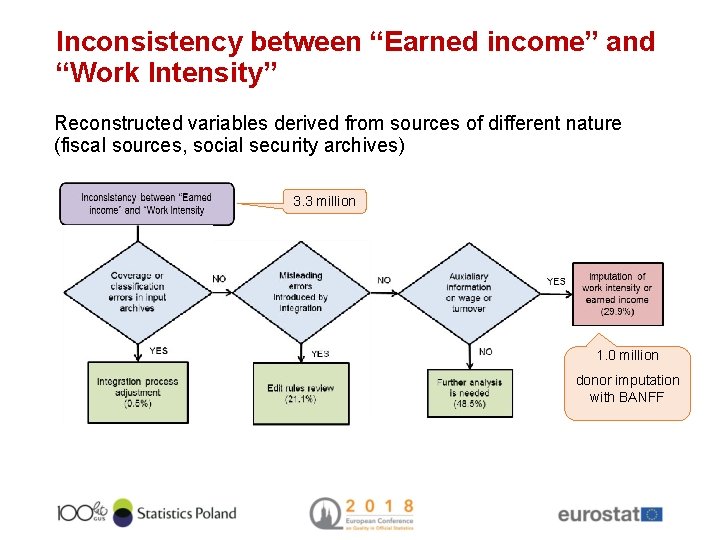
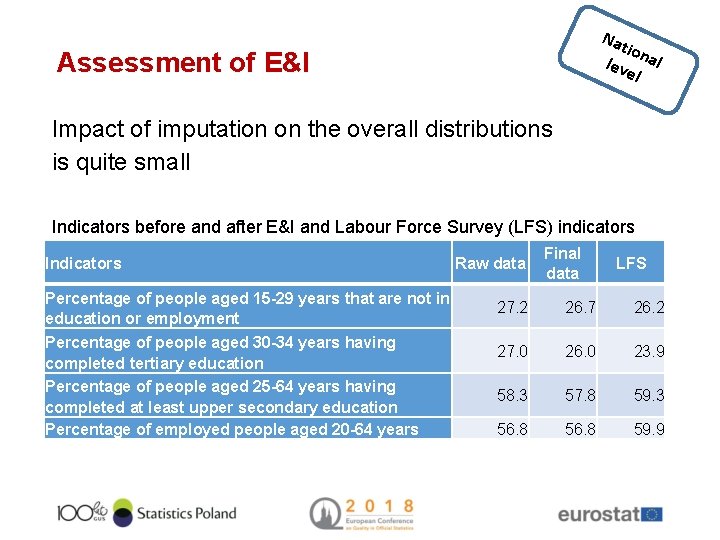
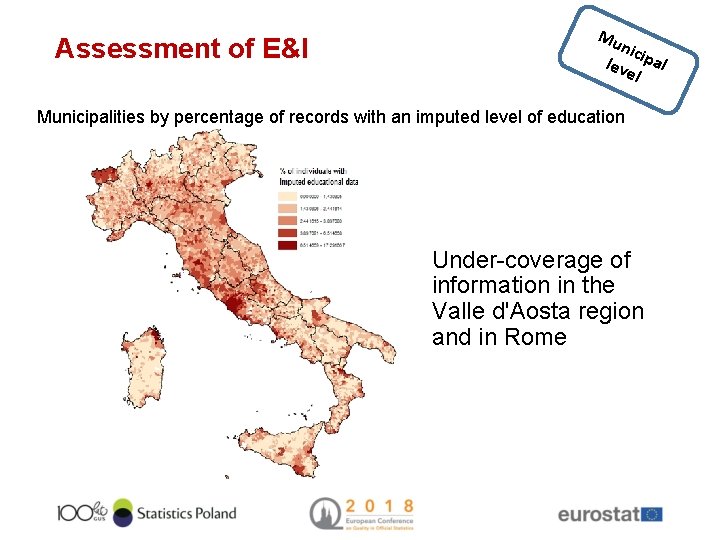
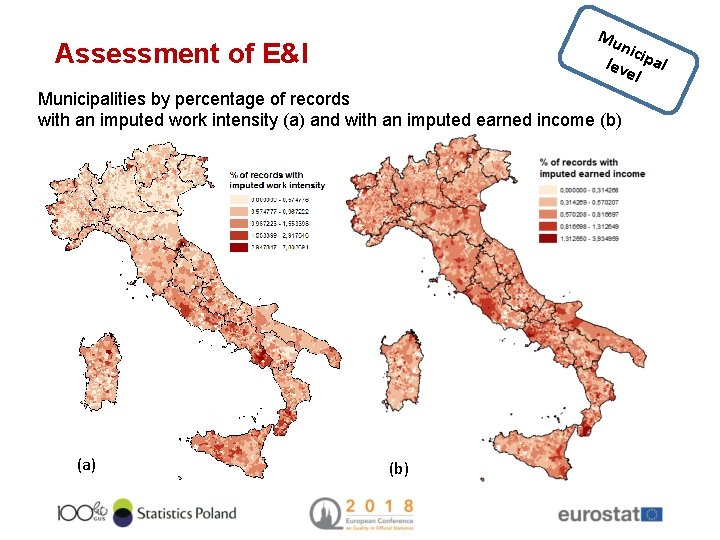
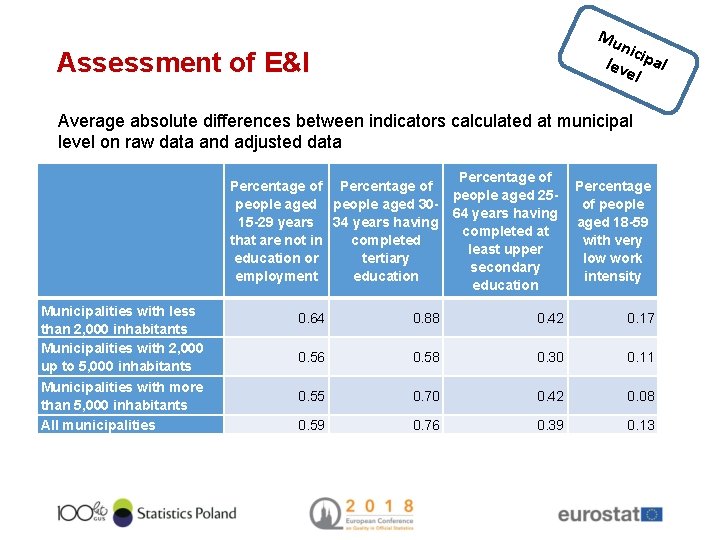
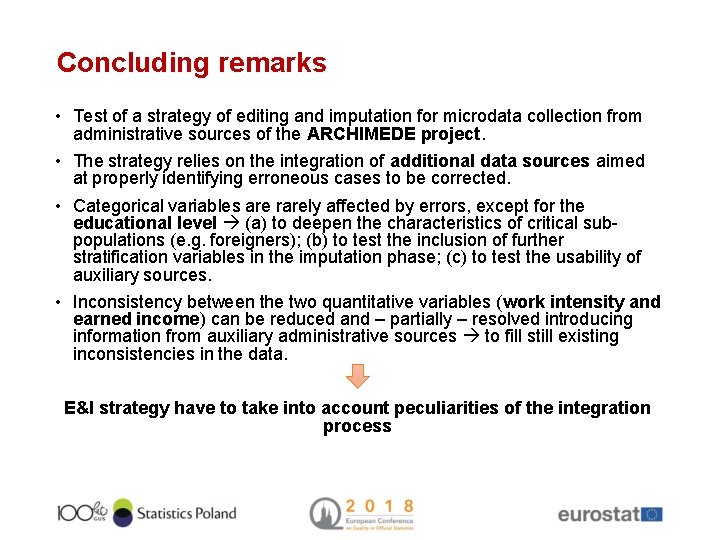
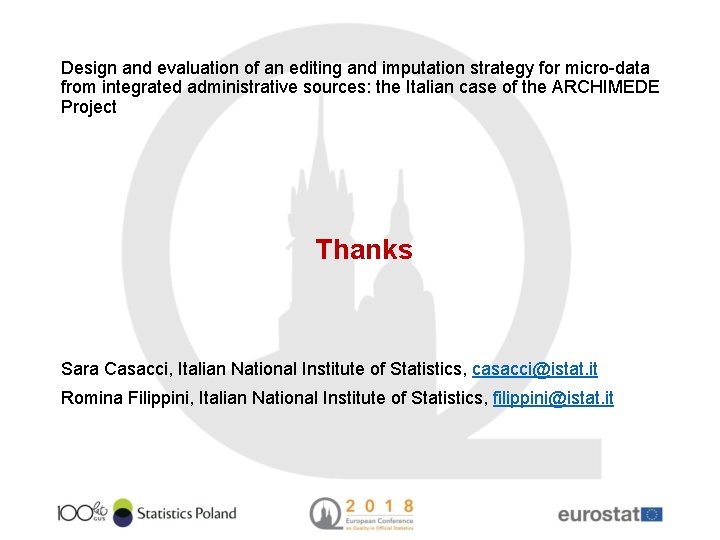
- Slides: 13
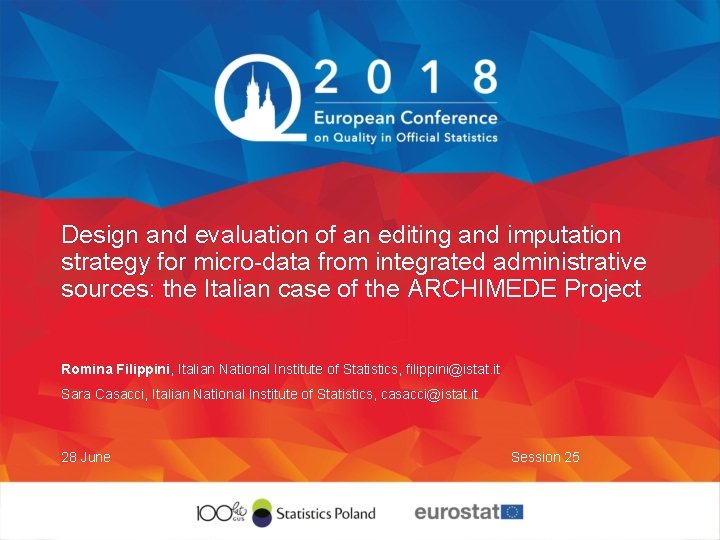
Design and evaluation of an editing and imputation strategy for micro-data from integrated administrative sources: the Italian case of the ARCHIMEDE Project Romina Filippini, Italian National Institute of Statistics, filippini@istat. it Sara Casacci, Italian National Institute of Statistics, casacci@istat. it 28 June Session 25
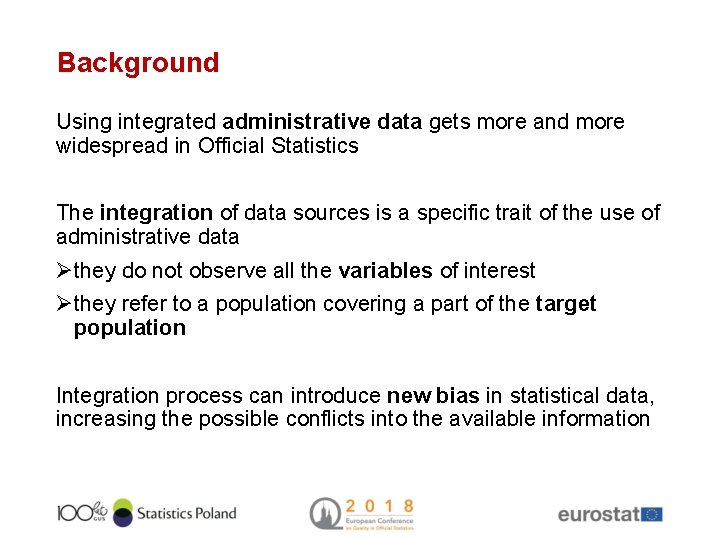
Background Using integrated administrative data gets more and more widespread in Official Statistics The integration of data sources is a specific trait of the use of administrative data Øthey do not observe all the variables of interest Øthey refer to a population covering a part of the target population Integration process can introduce new bias in statistical data, increasing the possible conflicts into the available information
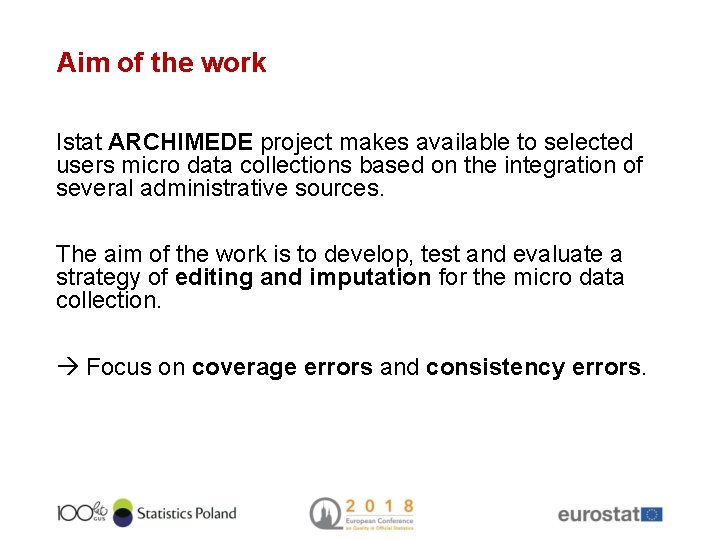
Aim of the work Istat ARCHIMEDE project makes available to selected users micro data collections based on the integration of several administrative sources. The aim of the work is to develop, test and evaluate a strategy of editing and imputation for the micro data collection. Focus on coverage errors and consistency errors.
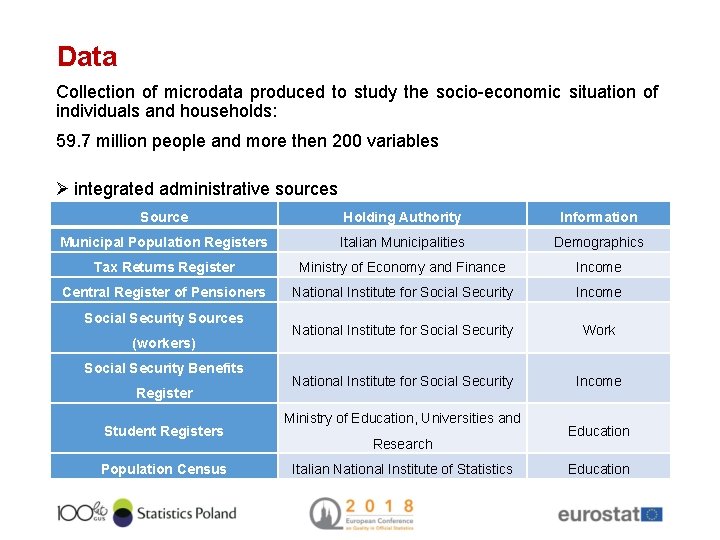
Data Collection of microdata produced to study the socio-economic situation of individuals and households: 59. 7 million people and more then 200 variables Ø integrated administrative sources Source Holding Authority Information Municipal Population Registers Italian Municipalities Demographics Tax Returns Register Ministry of Economy and Finance Income Central Register of Pensioners National Institute for Social Security Income National Institute for Social Security Work National Institute for Social Security Income Social Security Sources (workers) Social Security Benefits Register Student Registers Population Census Ministry of Education, Universities and Research Italian National Institute of Statistics Education
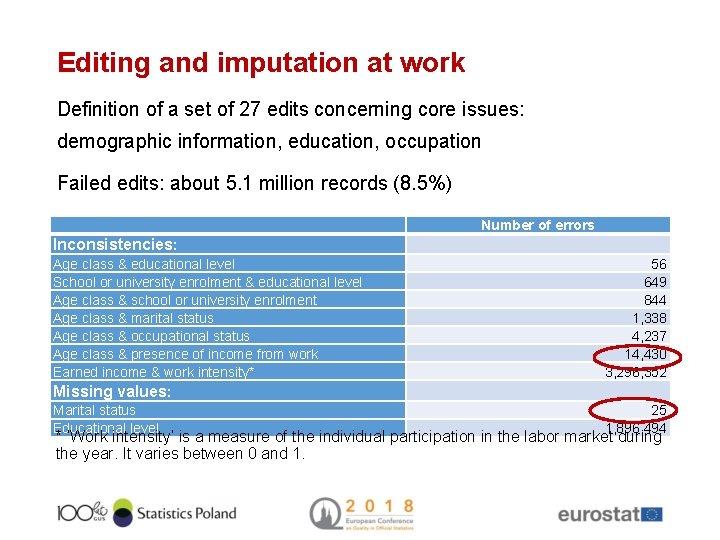
Editing and imputation at work Definition of a set of 27 edits concerning core issues: demographic information, education, occupation Failed edits: about 5. 1 million records (8. 5%) Inconsistencies: Age class & educational level School or university enrolment & educational level Age class & school or university enrolment Age class & marital status Age class & occupational status Age class & presence of income from work Earned income & work intensity* Missing values: Marital status Educational level Number of errors 56 649 844 1, 338 4, 237 14, 430 3, 296, 352 25 1, 896, 494 * ‘Work intensity’ is a measure of the individual participation in the labor market during the year. It varies between 0 and 1.
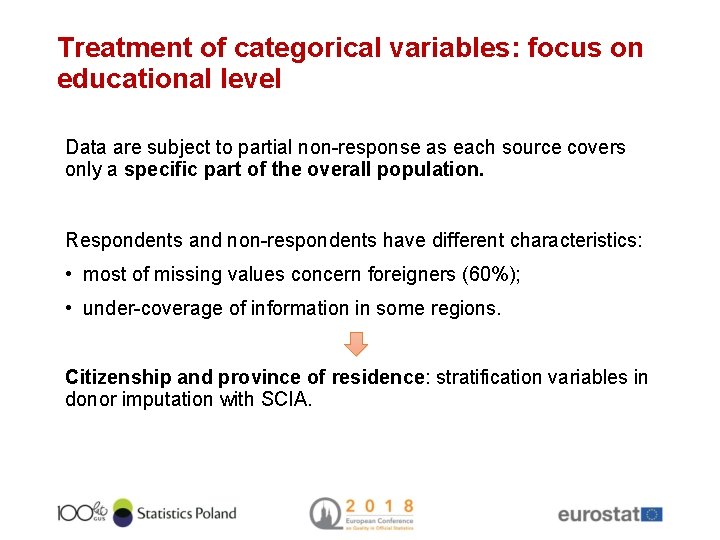
Treatment of categorical variables: focus on educational level Data are subject to partial non-response as each source covers only a specific part of the overall population. Respondents and non-respondents have different characteristics: • most of missing values concern foreigners (60%); • under-coverage of information in some regions. Citizenship and province of residence: stratification variables in donor imputation with SCIA.
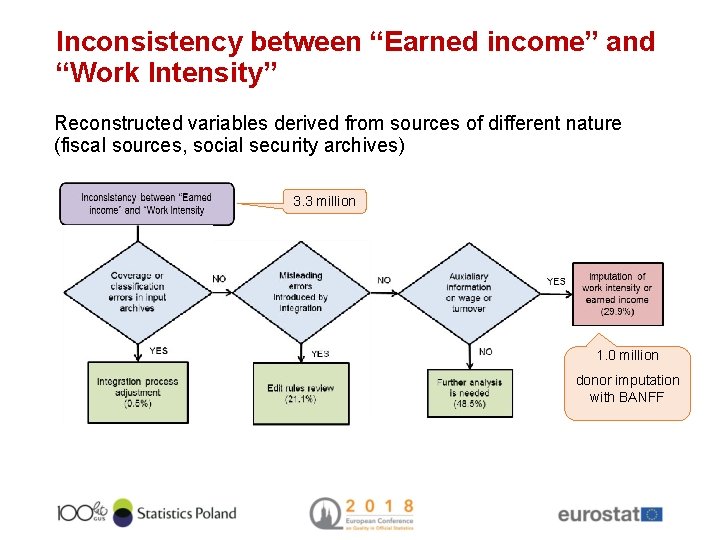
Inconsistency between “Earned income” and “Work Intensity” Reconstructed variables derived from sources of different nature (fiscal sources, social security archives) 3. 3 million 1. 0 million donor imputation with BANFF
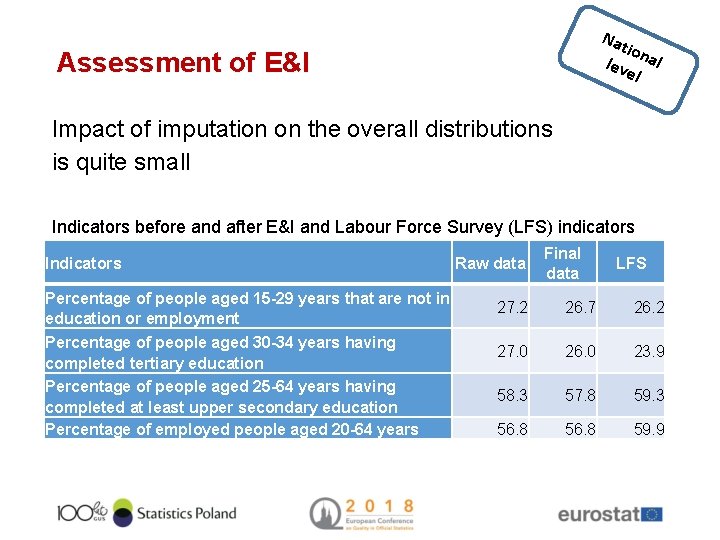
Nat io lev nal el Assessment of E&I Impact of imputation on the overall distributions is quite small Indicators before and after E&I and Labour Force Survey (LFS) indicators Indicators Percentage of people aged 15 -29 years that are not in education or employment Percentage of people aged 30 -34 years having completed tertiary education Percentage of people aged 25 -64 years having completed at least upper secondary education Percentage of employed people aged 20 -64 years Raw data Final data LFS 27. 2 26. 7 26. 2 27. 0 26. 0 23. 9 58. 3 57. 8 59. 3 56. 8 59. 9
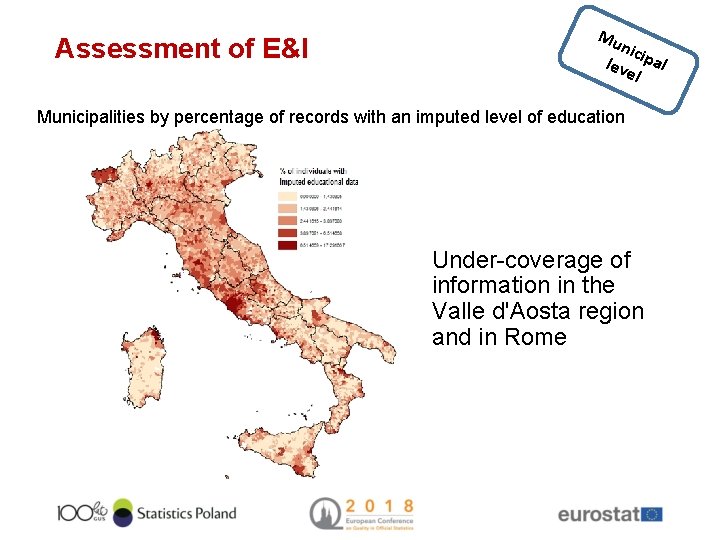
Assessment of E&I Mu nic lev ipal el Municipalities by percentage of records with an imputed level of education Under-coverage of information in the Valle d'Aosta region and in Rome
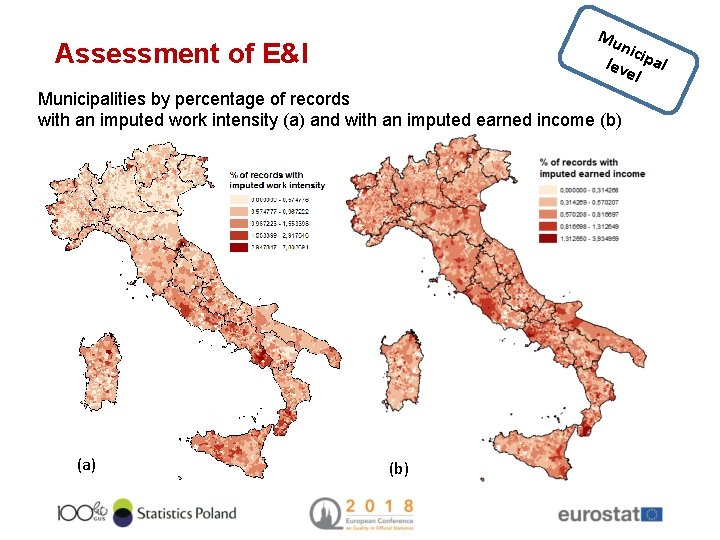
Mu nic lev ipal el Assessment of E&I Municipalities by percentage of records with an imputed work intensity (a) and with an imputed earned income (b) (a) (b)
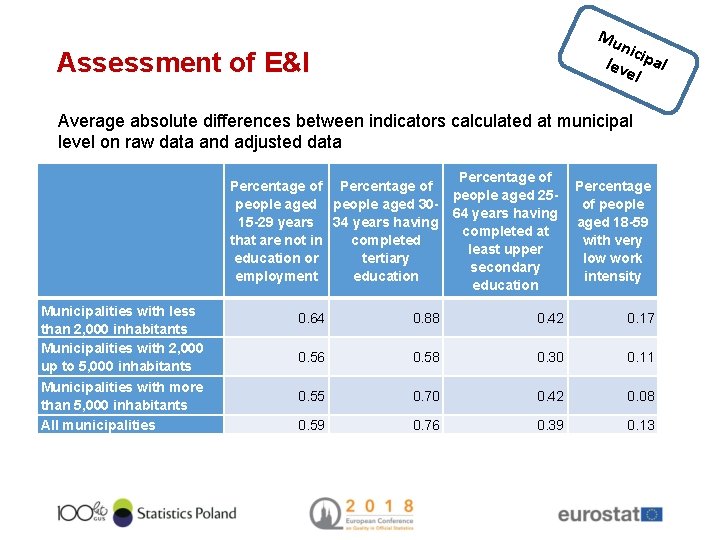
Mu nic lev ipal el Assessment of E&I Average absolute differences between indicators calculated at municipal level on raw data and adjusted data Municipalities with less than 2, 000 inhabitants Municipalities with 2, 000 up to 5, 000 inhabitants Municipalities with more than 5, 000 inhabitants All municipalities Percentage of Percentage people aged 25 people aged 30 of people 64 years having 15 -29 years 34 years having aged 18 -59 completed at that are not in completed with very least upper education or tertiary low work secondary employment education intensity education 0. 64 0. 88 0. 42 0. 17 0. 56 0. 58 0. 30 0. 11 0. 55 0. 70 0. 42 0. 08 0. 59 0. 76 0. 39 0. 13
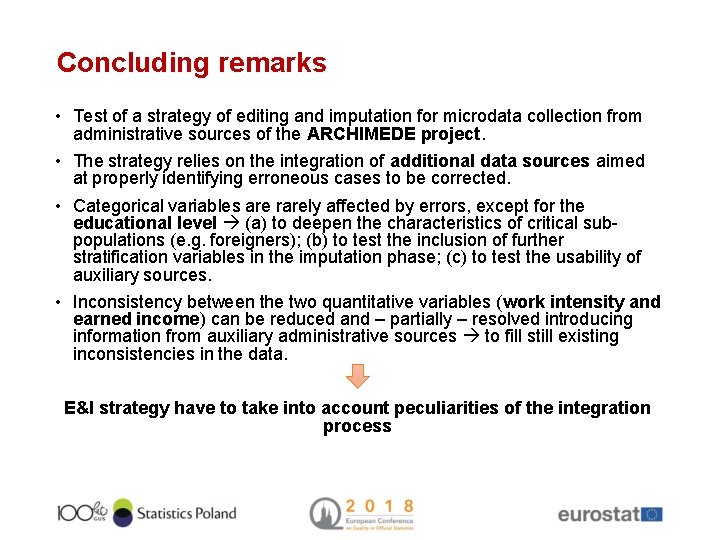
Concluding remarks • Test of a strategy of editing and imputation for microdata collection from administrative sources of the ARCHIMEDE project. • The strategy relies on the integration of additional data sources aimed at properly identifying erroneous cases to be corrected. • Categorical variables are rarely affected by errors, except for the educational level (a) to deepen the characteristics of critical subpopulations (e. g. foreigners); (b) to test the inclusion of further stratification variables in the imputation phase; (c) to test the usability of auxiliary sources. • Inconsistency between the two quantitative variables (work intensity and earned income) can be reduced and – partially – resolved introducing information from auxiliary administrative sources to fill still existing inconsistencies in the data. E&I strategy have to take into account peculiarities of the integration process
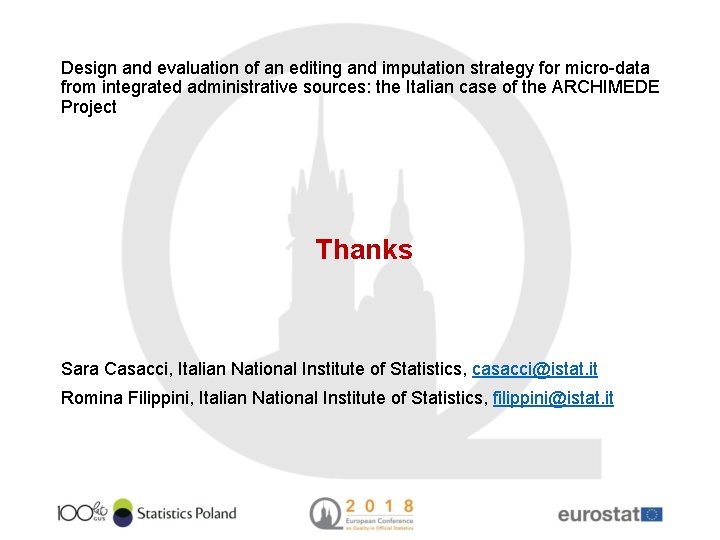
Design and evaluation of an editing and imputation strategy for micro-data from integrated administrative sources: the Italian case of the ARCHIMEDE Project Thanks Sara Casacci, Italian National Institute of Statistics, casacci@istat. it Romina Filippini, Italian National Institute of Statistics, filippini@istat. it
Central editing in research methodology
Linear editing vs non linear editing
Boni de suractivité
Fiml in mplus
Eagle imputation
Missing data imputation
Font editing and design tools in multimedia
Arrow font
Template editing instructions and feedback
What are the most common data input techniques in gis
Planning drafting revising editing
Writing process ppt
Digital vs analog signal
Revise and editing