CHAPTER 2 Decision Making Systems Modeling and Support
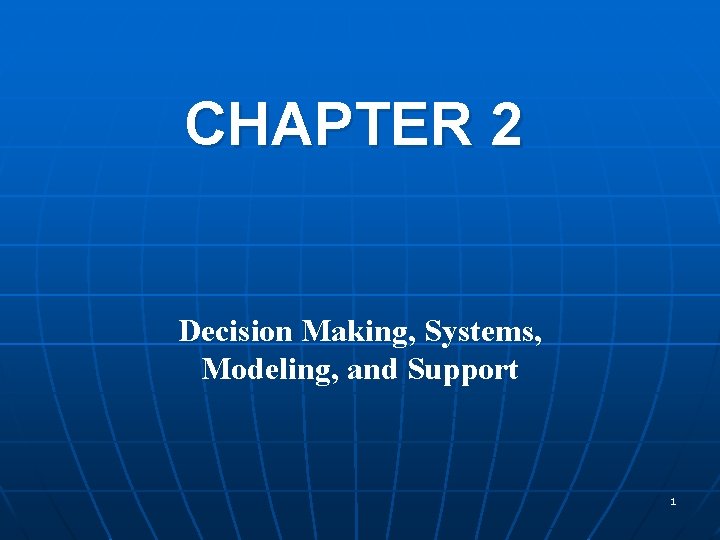
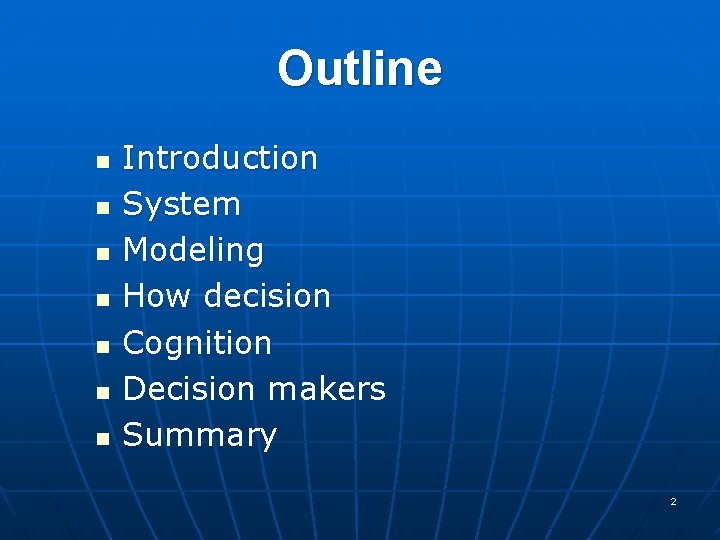
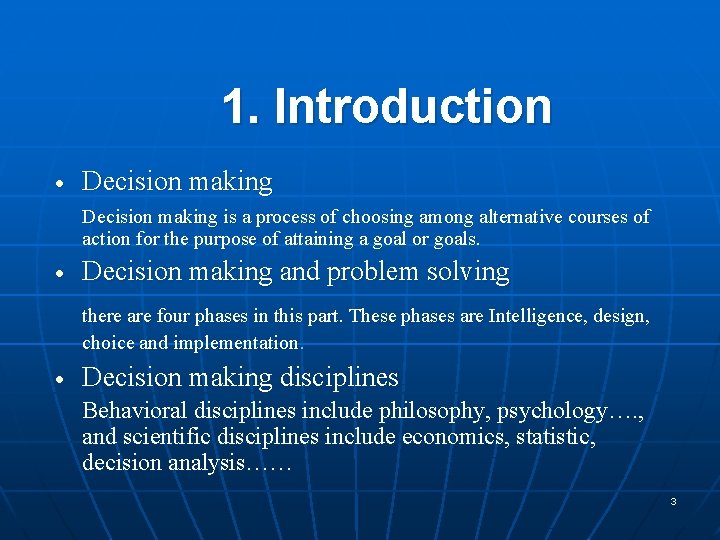
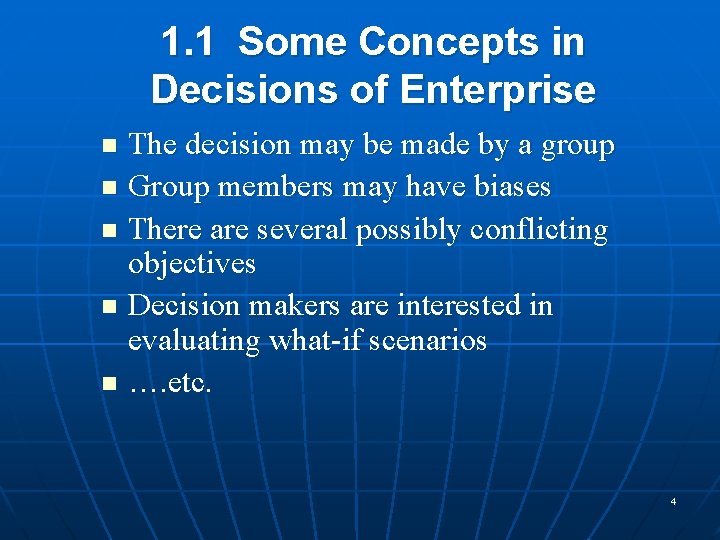
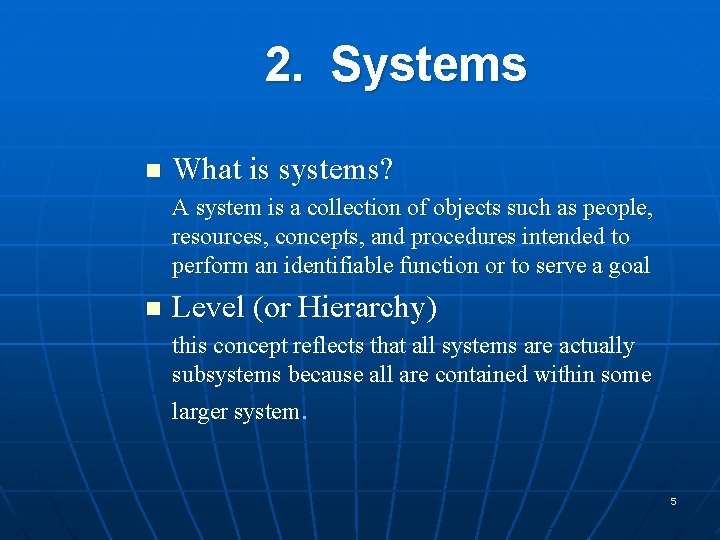
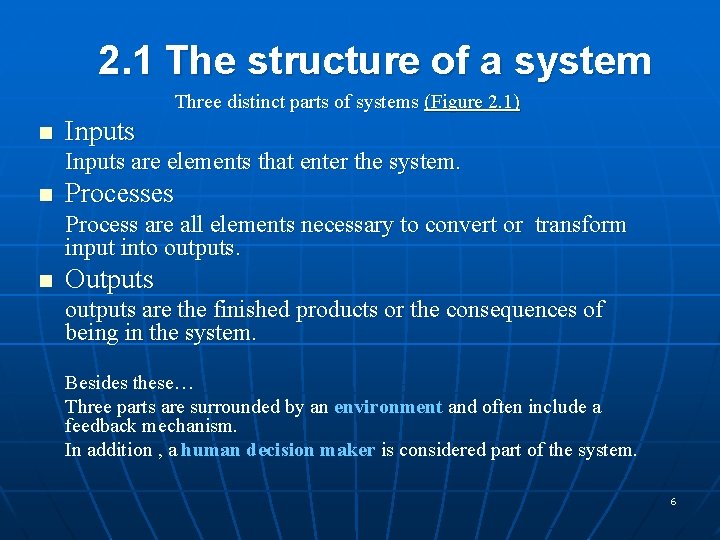
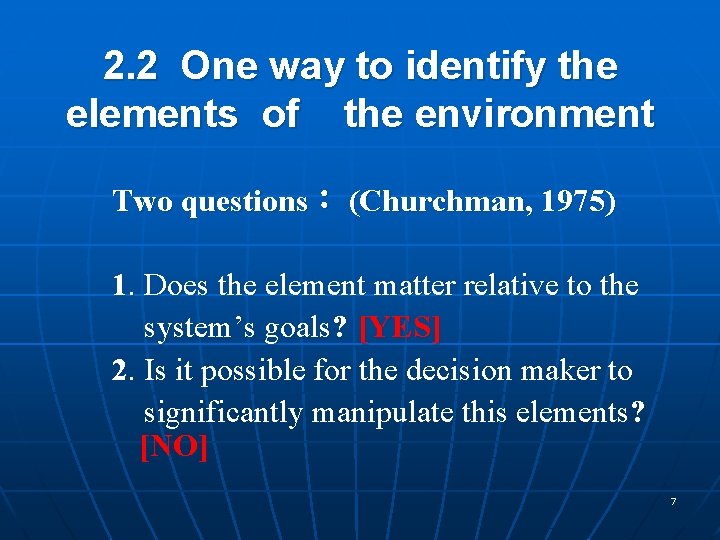
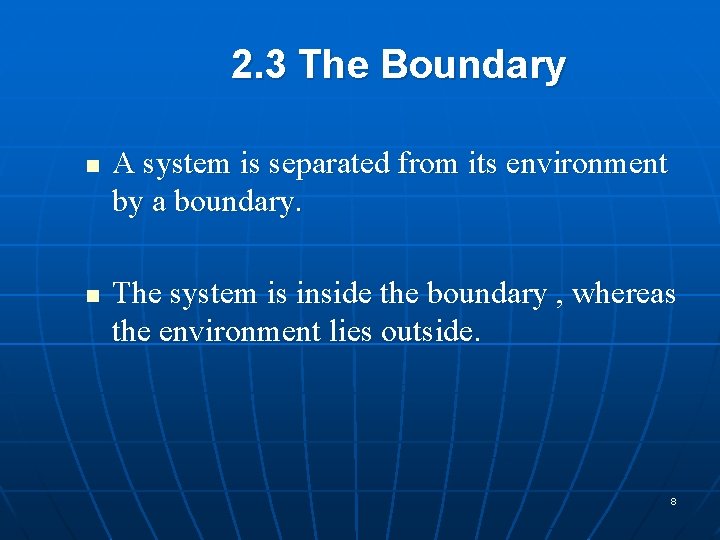
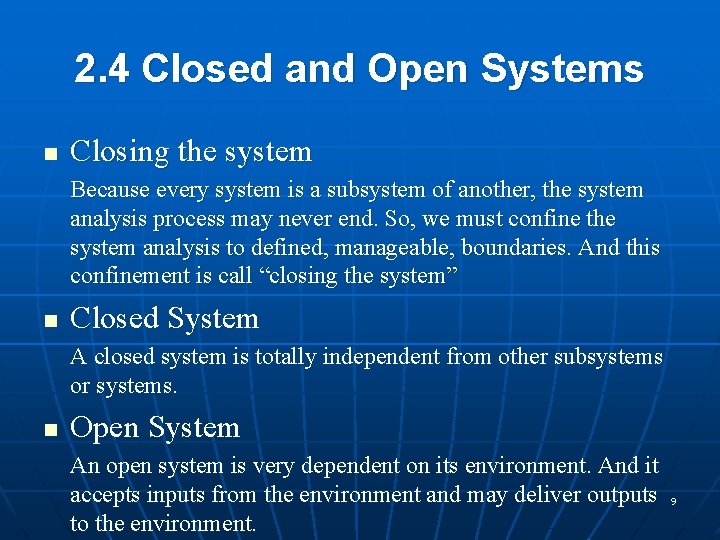
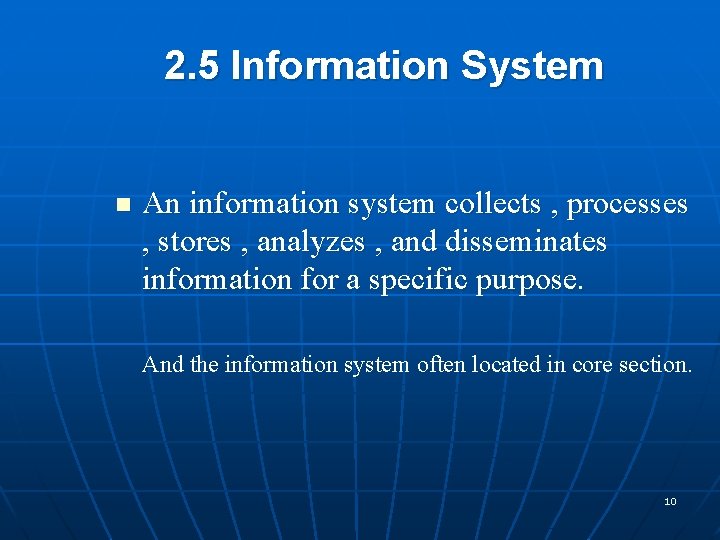
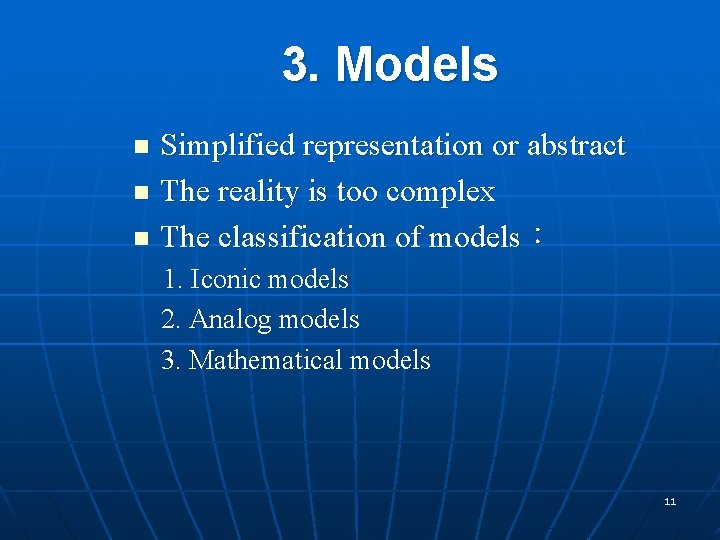
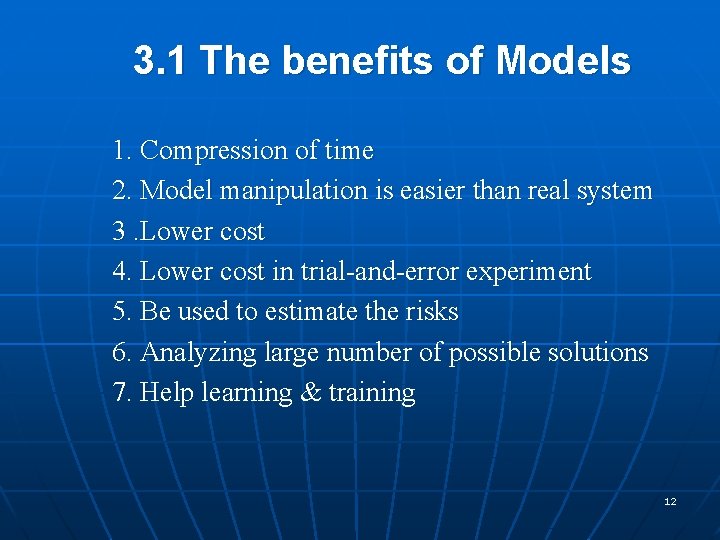
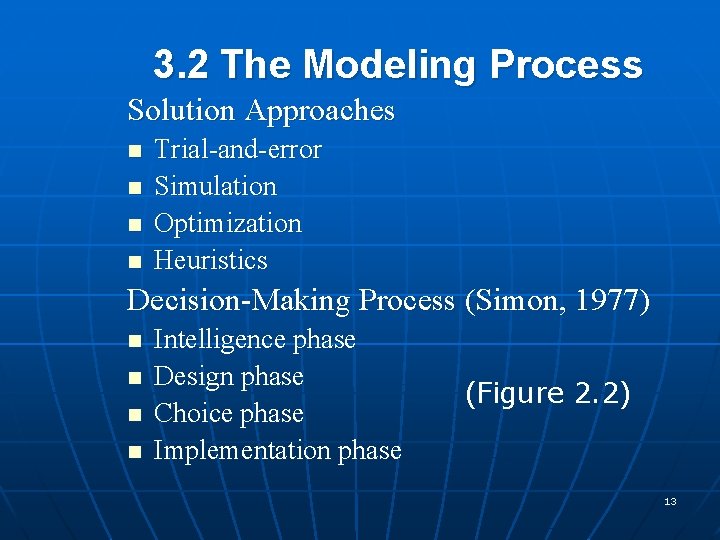
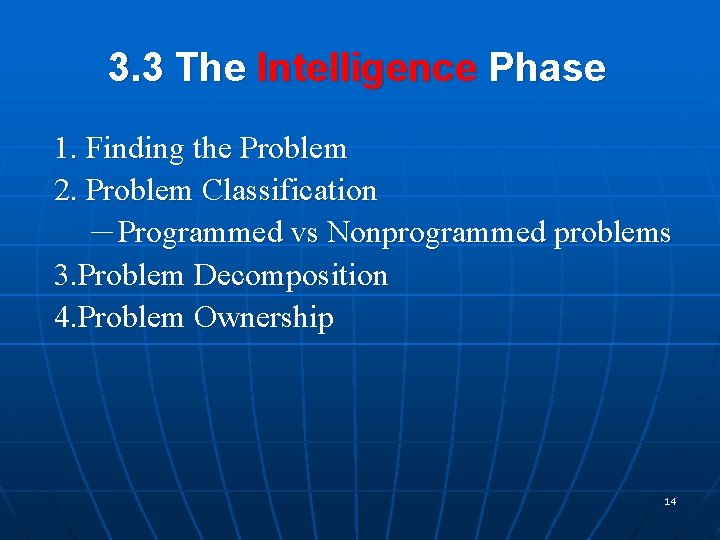
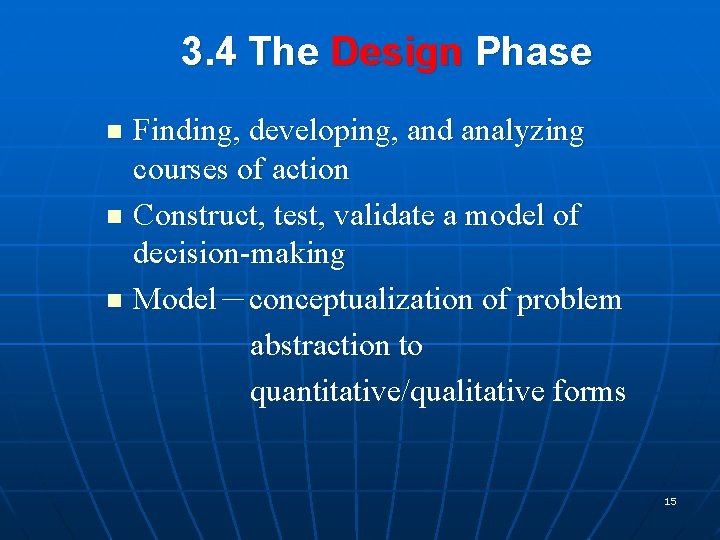
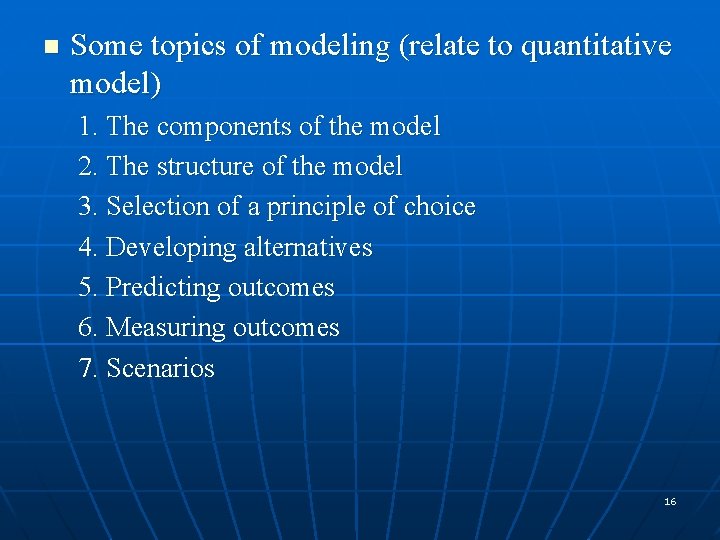
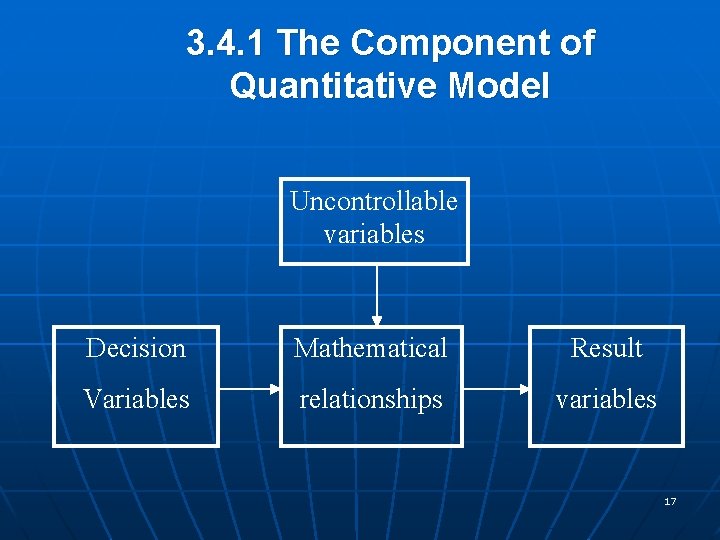
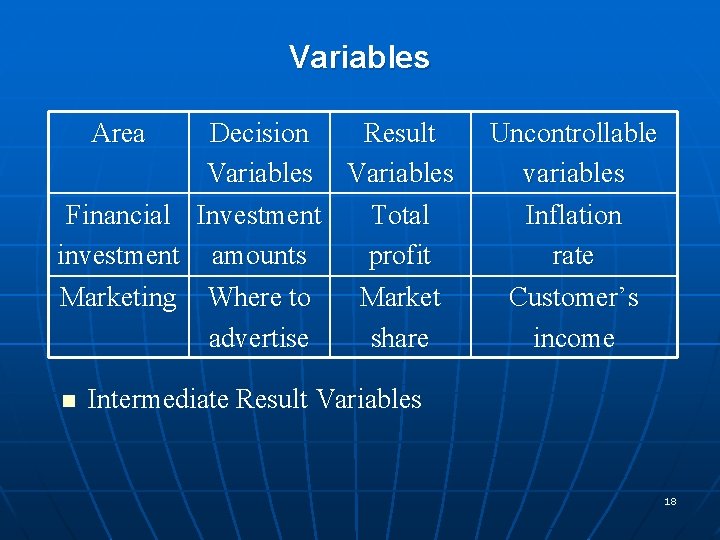
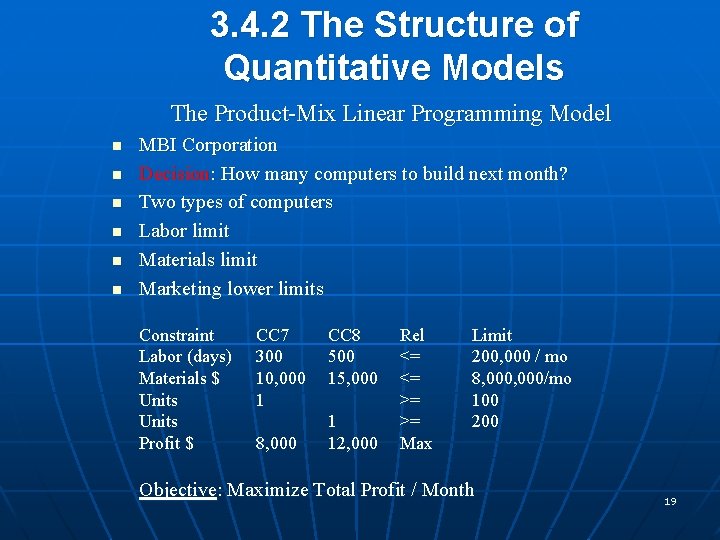
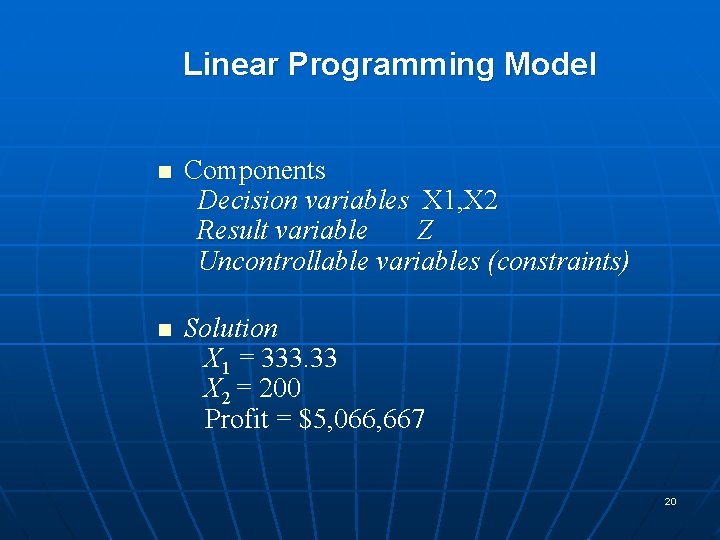
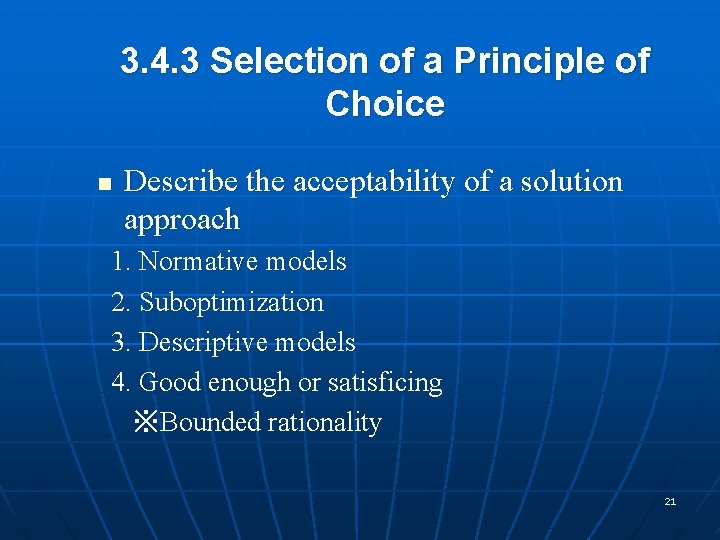
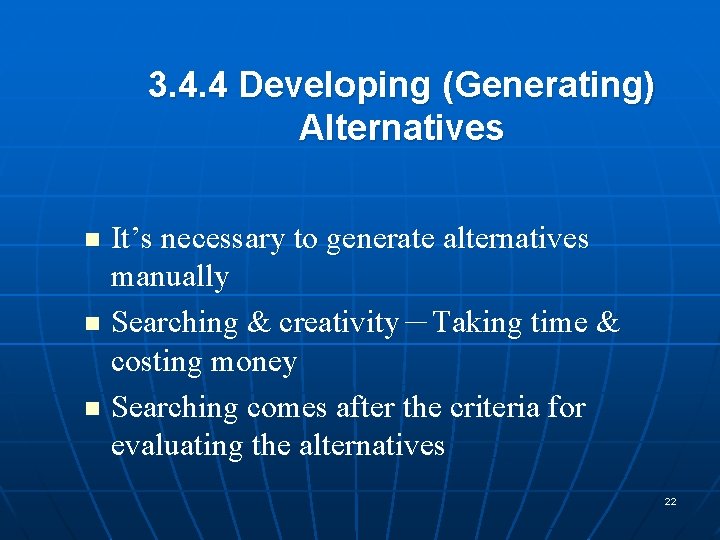
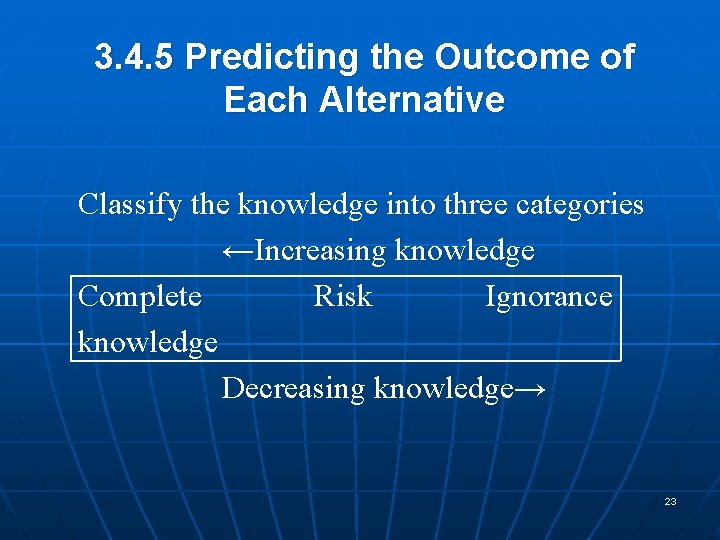
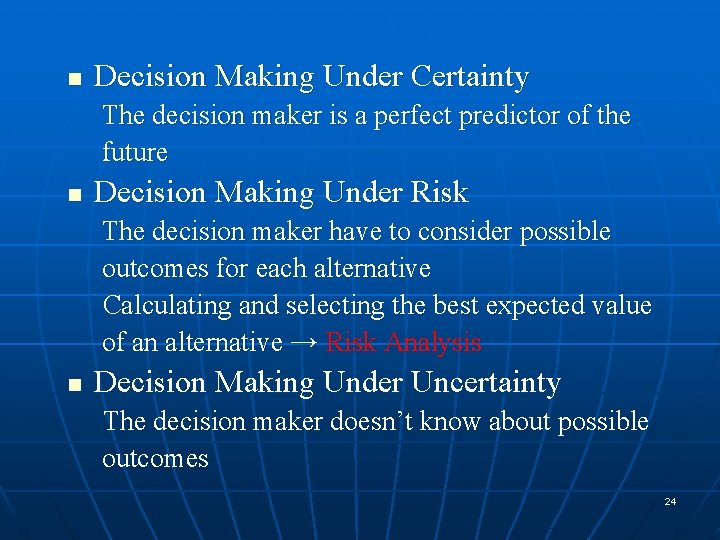
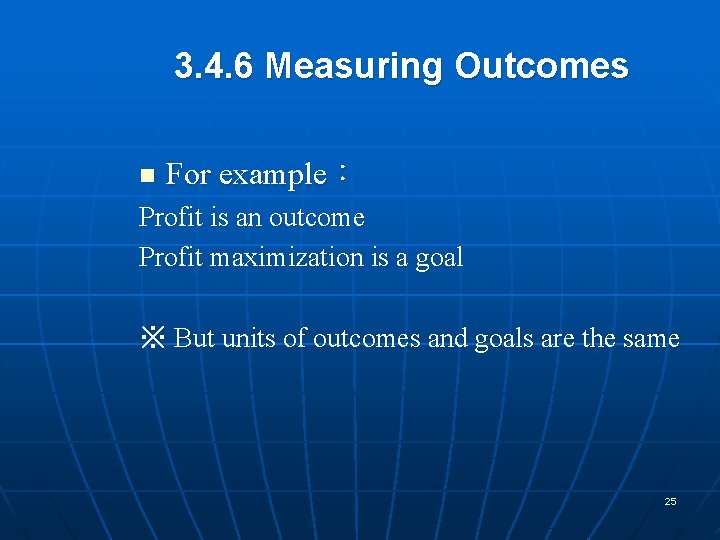
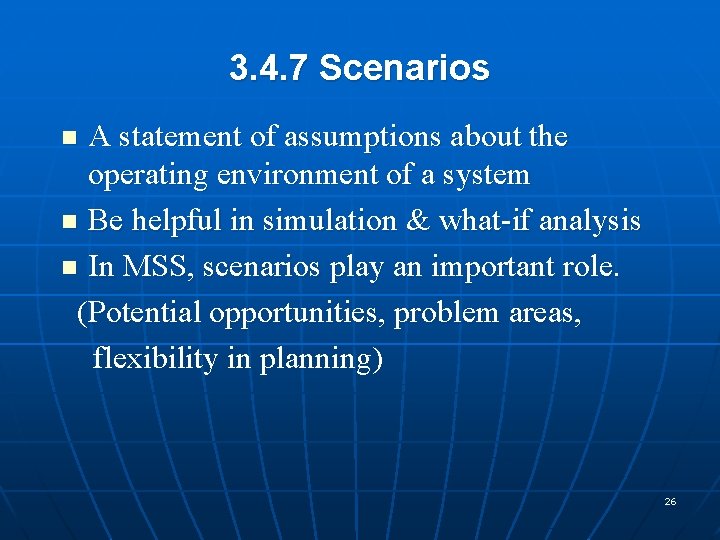
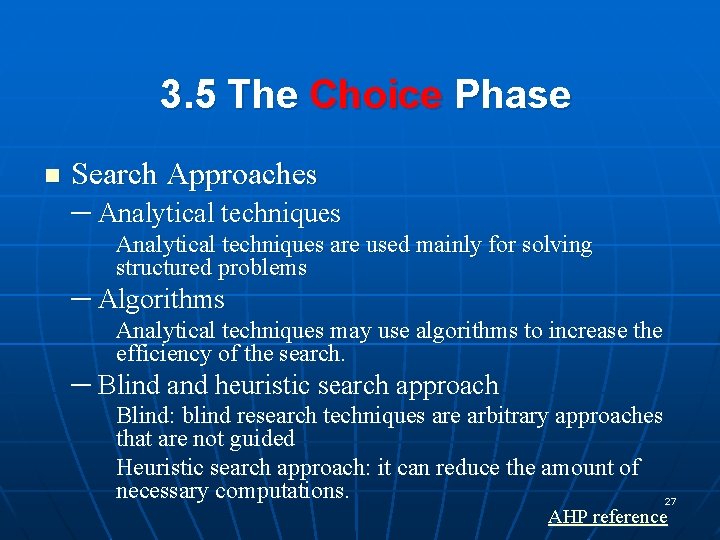
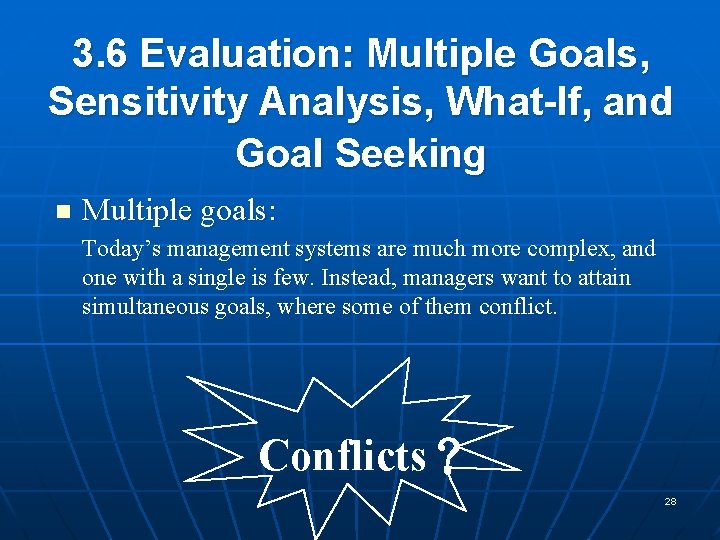
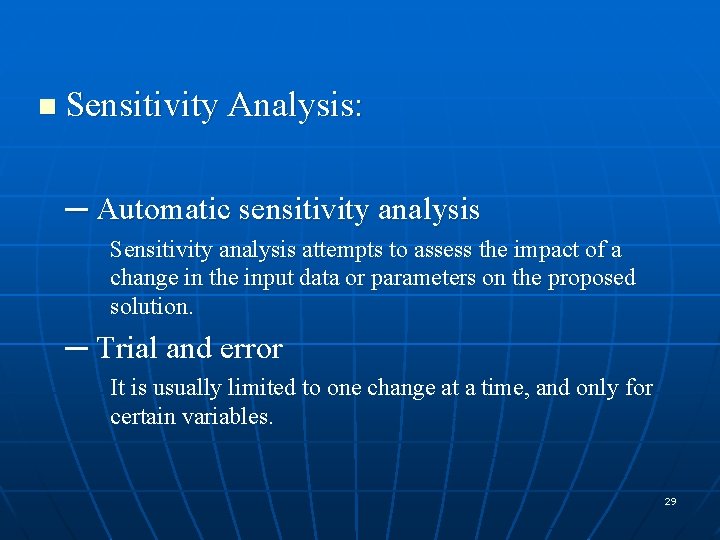
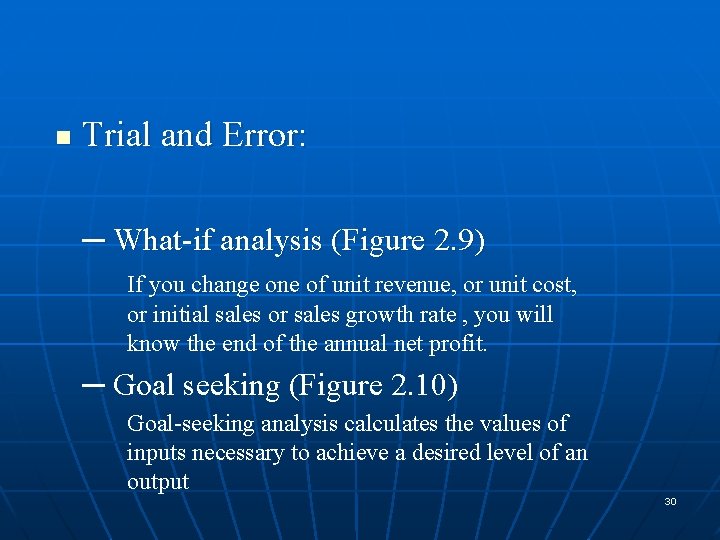
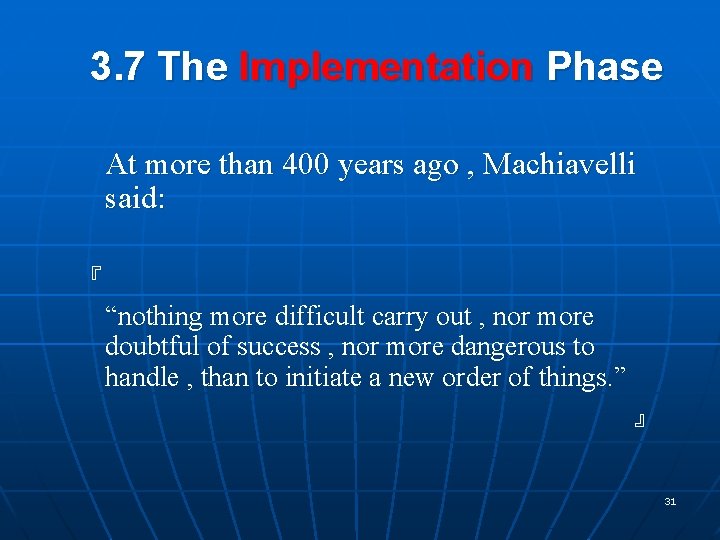
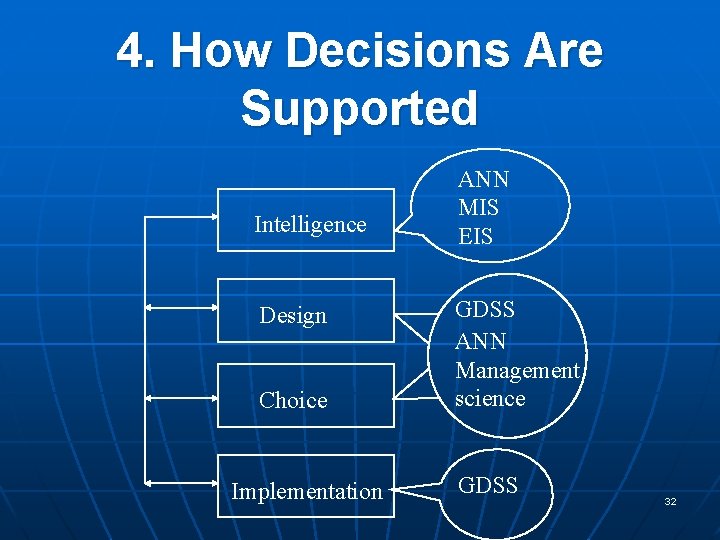
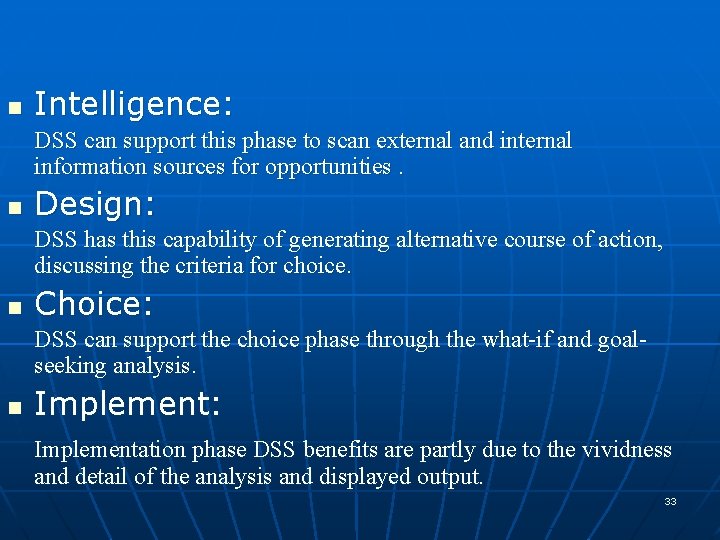
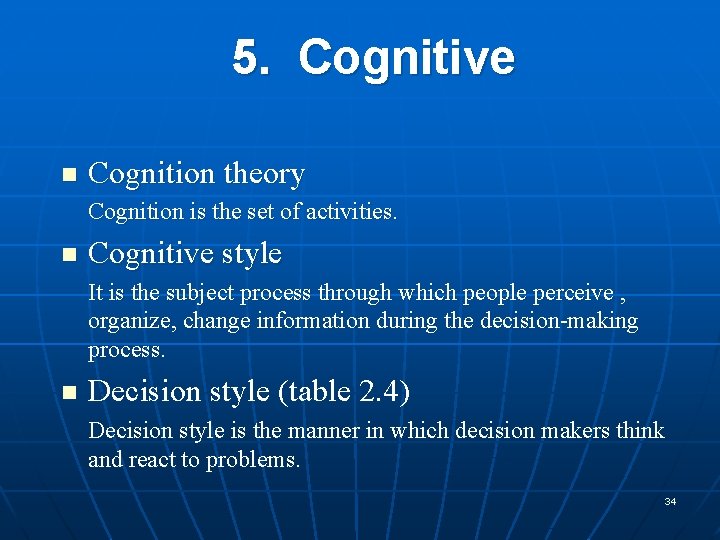
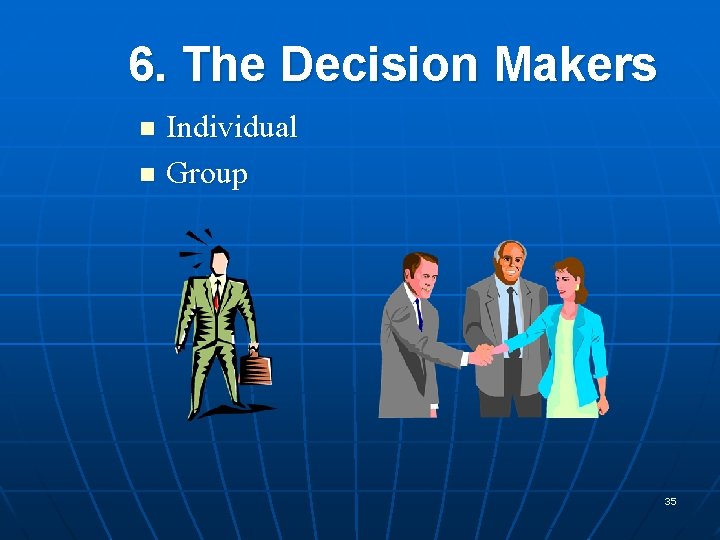
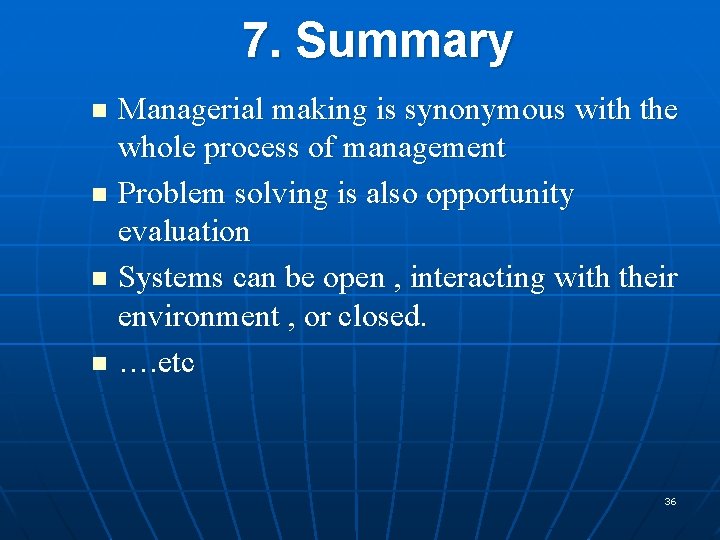
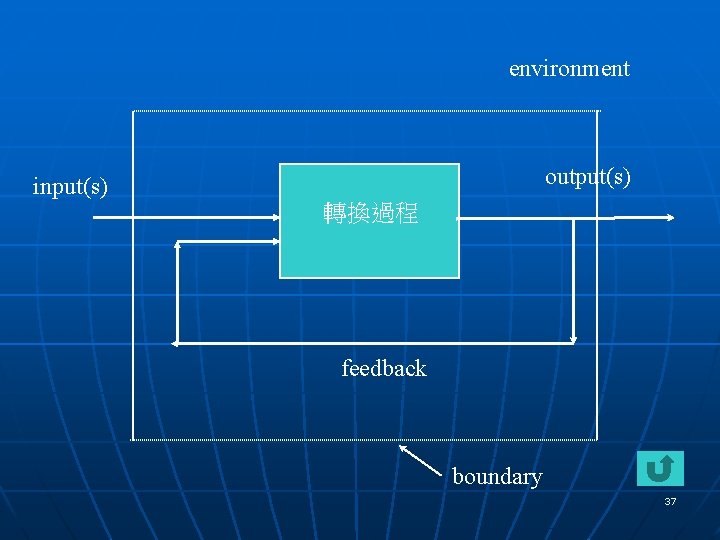
- Slides: 37
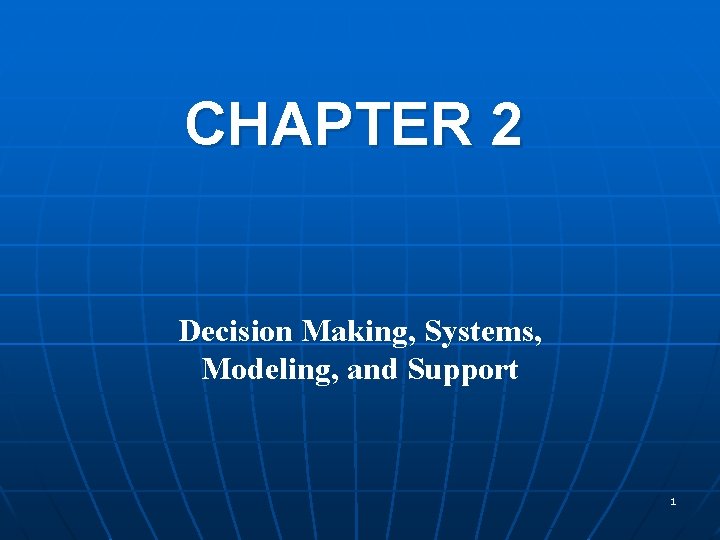
CHAPTER 2 Decision Making, Systems, Modeling, and Support 1
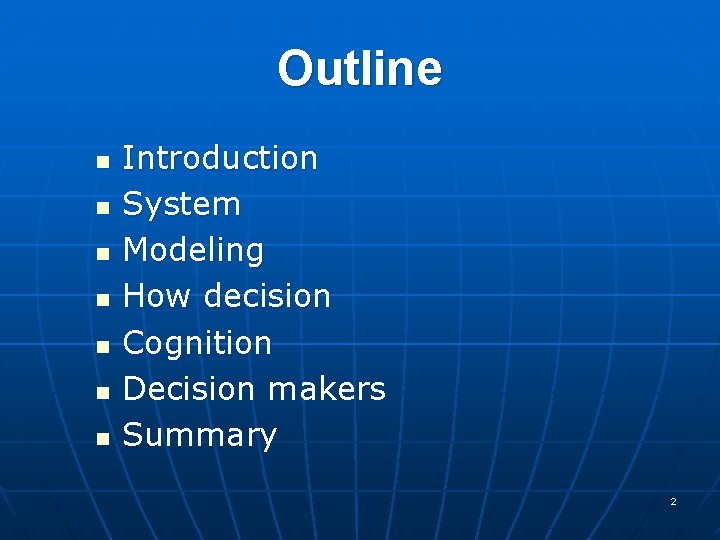
Outline n n n n Introduction System Modeling How decision Cognition Decision makers Summary 2
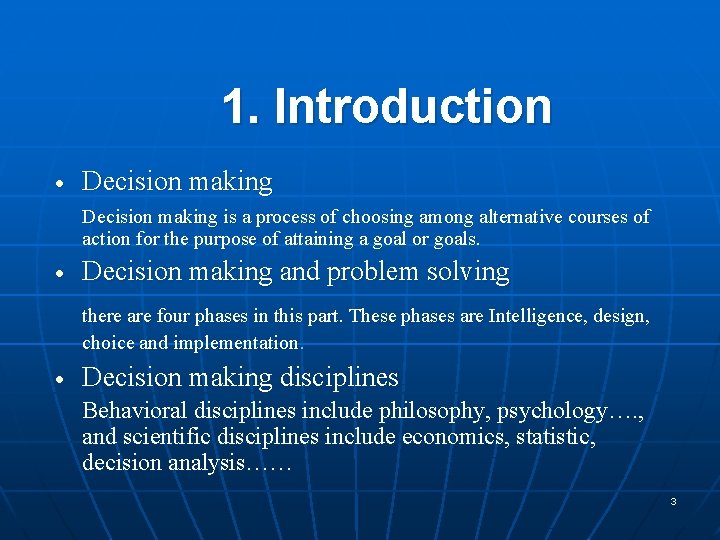
1. Introduction · Decision making is a process of choosing among alternative courses of action for the purpose of attaining a goal or goals. · Decision making and problem solving there are four phases in this part. These phases are Intelligence, design, choice and implementation. · Decision making disciplines Behavioral disciplines include philosophy, psychology…. , and scientific disciplines include economics, statistic, decision analysis…… 3
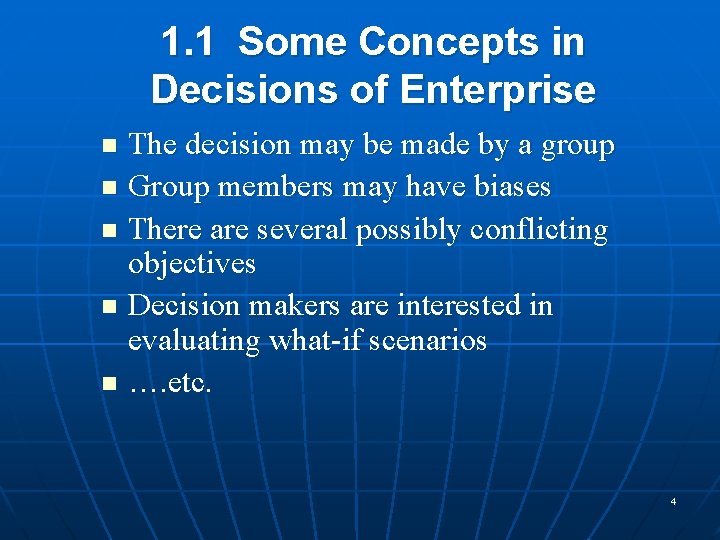
1. 1 Some Concepts in Decisions of Enterprise The decision may be made by a group n Group members may have biases n There are several possibly conflicting objectives n Decision makers are interested in evaluating what-if scenarios n …. etc. n 4
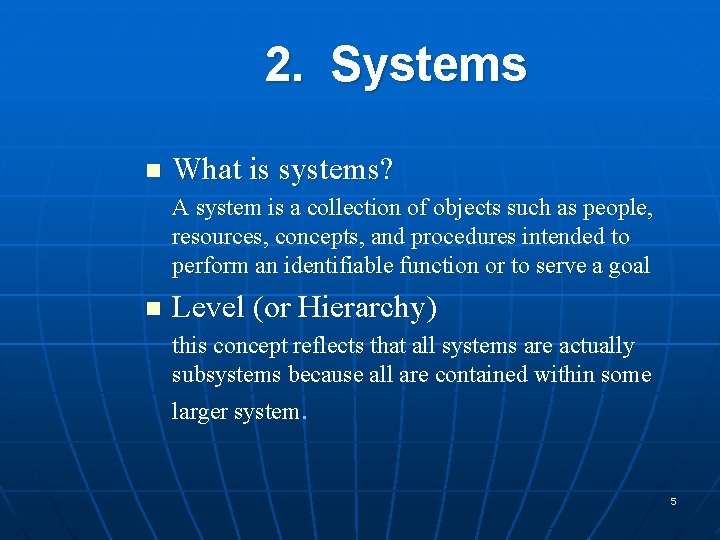
2. Systems n What is systems? A system is a collection of objects such as people, resources, concepts, and procedures intended to perform an identifiable function or to serve a goal n Level (or Hierarchy) this concept reflects that all systems are actually subsystems because all are contained within some larger system. 5
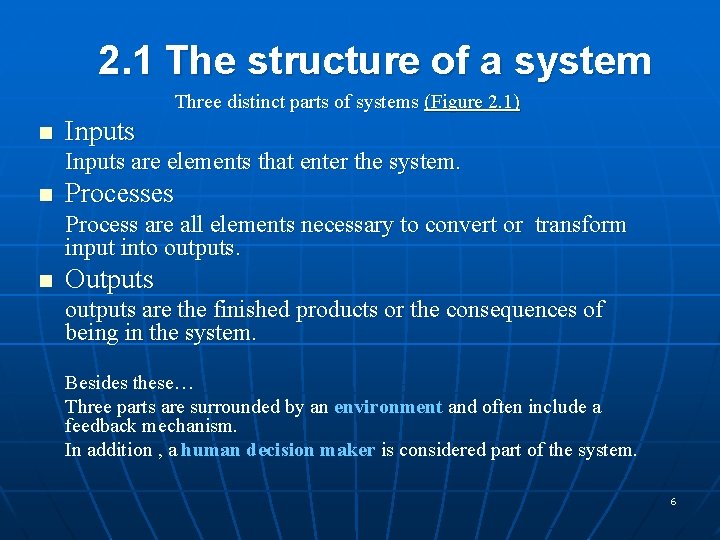
2. 1 The structure of a system Three distinct parts of systems (Figure 2. 1) n Inputs are elements that enter the system. n Processes Process are all elements necessary to convert or transform input into outputs. n Outputs outputs are the finished products or the consequences of being in the system. Besides these… Three parts are surrounded by an environment and often include a feedback mechanism. In addition , a human decision maker is considered part of the system. 6
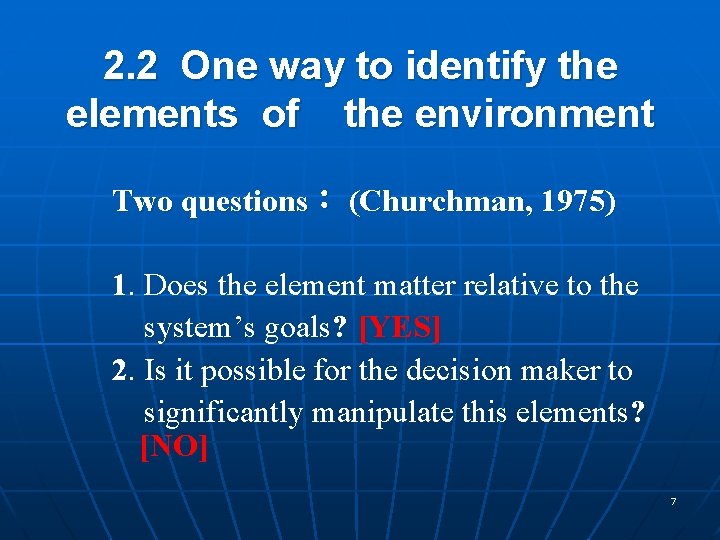
2. 2 One way to identify the elements of the environment Two questions: (Churchman, 1975) 1. Does the element matter relative to the system’s goals? [YES] 2. Is it possible for the decision maker to significantly manipulate this elements? [NO] 7
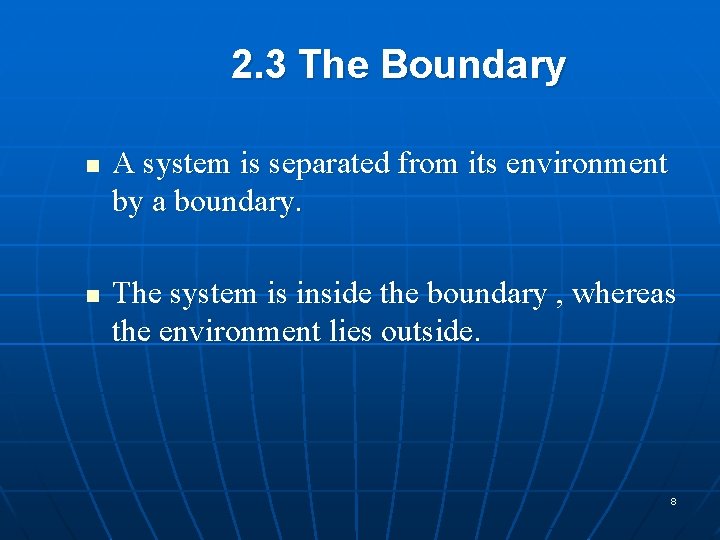
2. 3 The Boundary n n A system is separated from its environment by a boundary. The system is inside the boundary , whereas the environment lies outside. 8
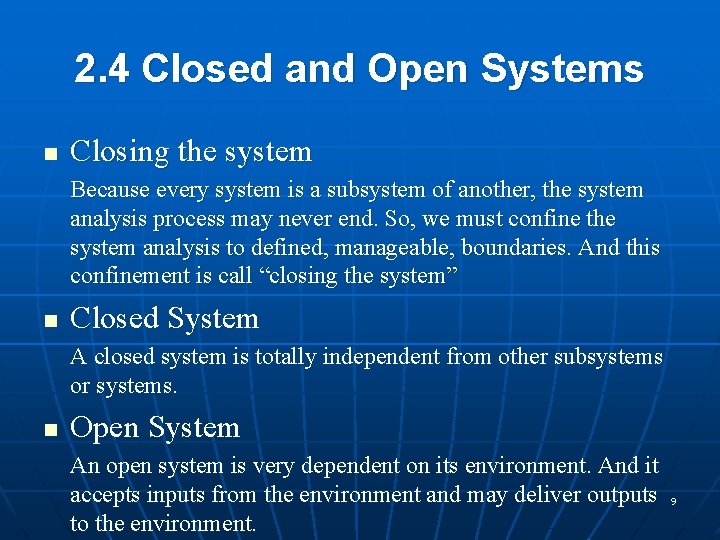
2. 4 Closed and Open Systems n Closing the system Because every system is a subsystem of another, the system analysis process may never end. So, we must confine the system analysis to defined, manageable, boundaries. And this confinement is call “closing the system” n Closed System A closed system is totally independent from other subsystems or systems. n Open System An open system is very dependent on its environment. And it accepts inputs from the environment and may deliver outputs to the environment. 9
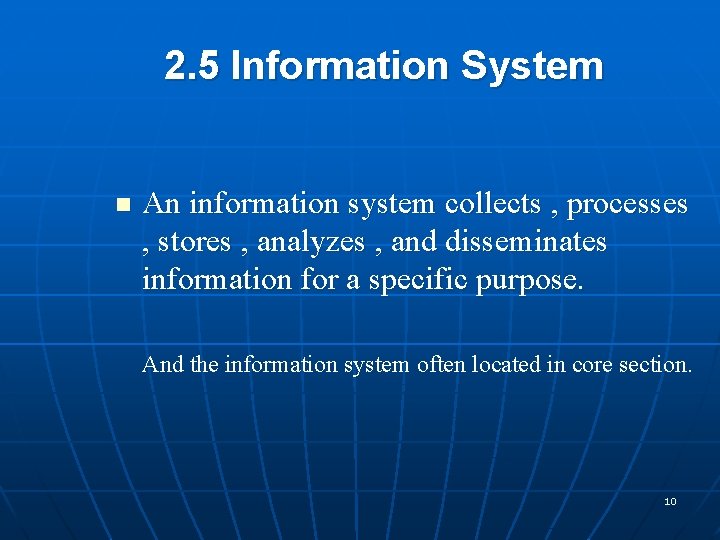
2. 5 Information System n An information system collects , processes , stores , analyzes , and disseminates information for a specific purpose. And the information system often located in core section. 10
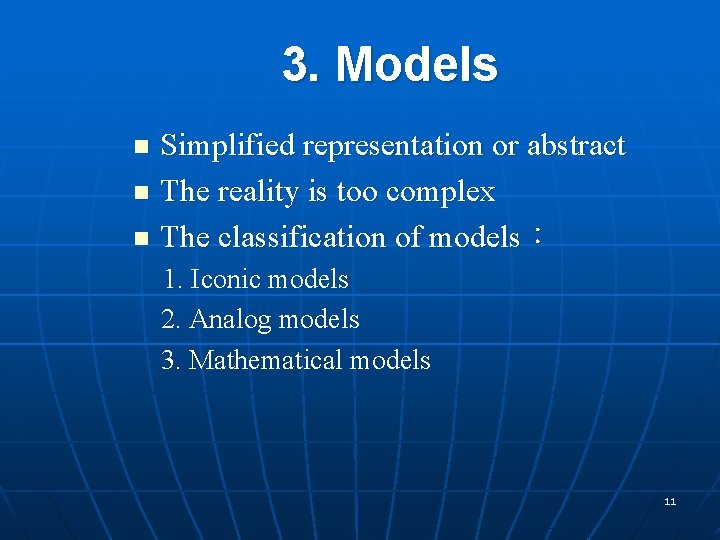
3. Models Simplified representation or abstract n The reality is too complex n The classification of models: n 1. Iconic models 2. Analog models 3. Mathematical models 11
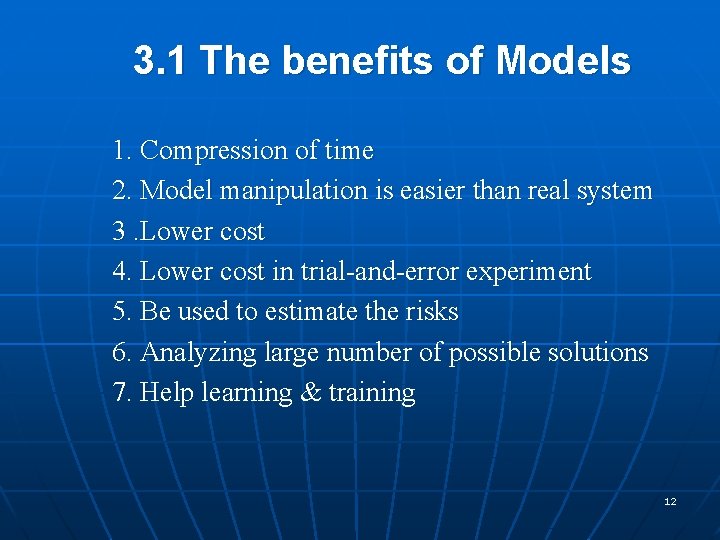
3. 1 The benefits of Models 1. Compression of time 2. Model manipulation is easier than real system 3. Lower cost 4. Lower cost in trial-and-error experiment 5. Be used to estimate the risks 6. Analyzing large number of possible solutions 7. Help learning & training 12
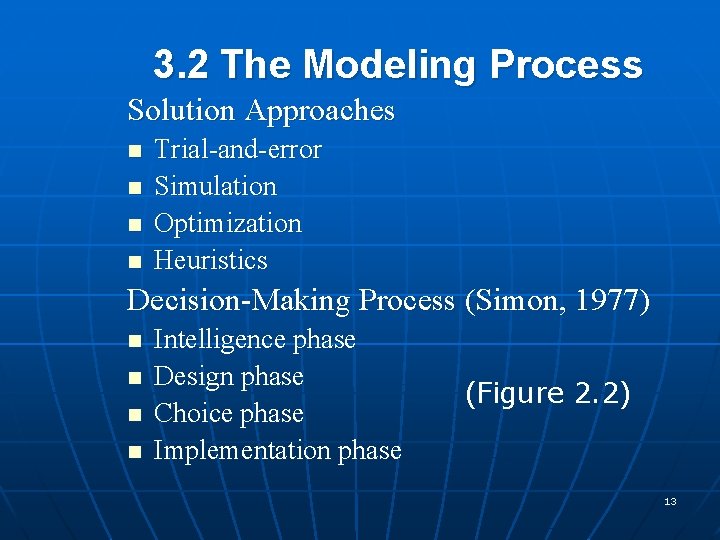
3. 2 The Modeling Process Solution Approaches n n Trial-and-error Simulation Optimization Heuristics Decision-Making Process (Simon, 1977) n n Intelligence phase Design phase Choice phase Implementation phase (Figure 2. 2) 13
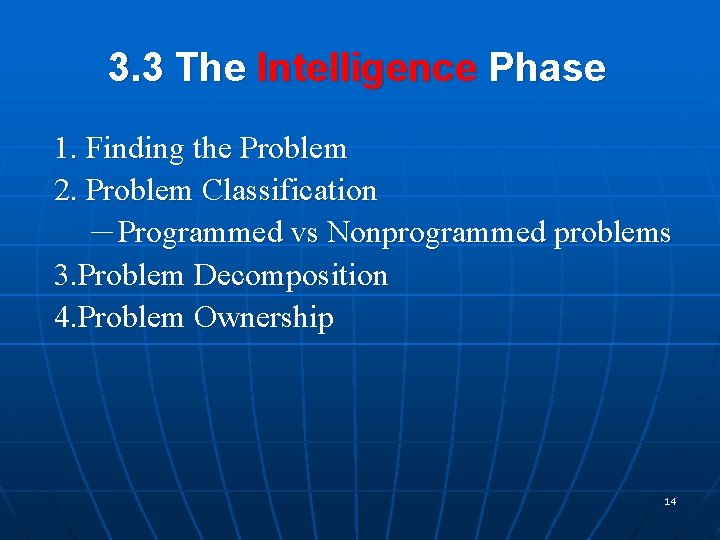
3. 3 The Intelligence Phase 1. Finding the Problem 2. Problem Classification -Programmed vs Nonprogrammed problems 3. Problem Decomposition 4. Problem Ownership 14
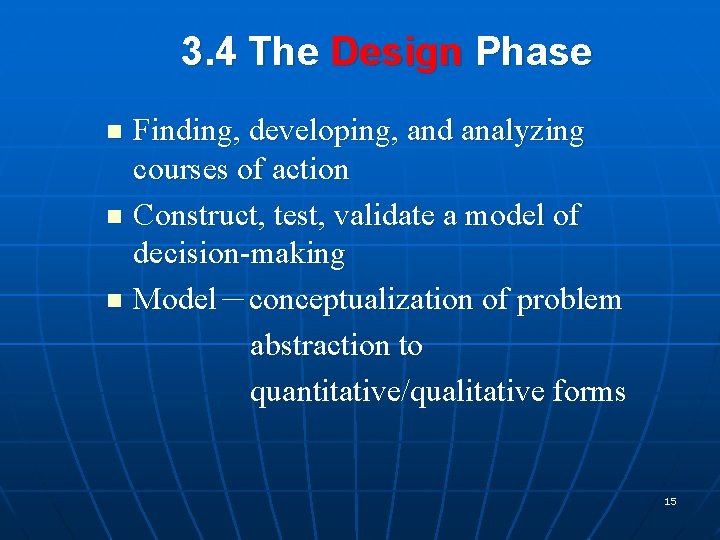
3. 4 The Design Phase Finding, developing, and analyzing courses of action n Construct, test, validate a model of decision-making n Model-conceptualization of problem abstraction to quantitative/qualitative forms n 15
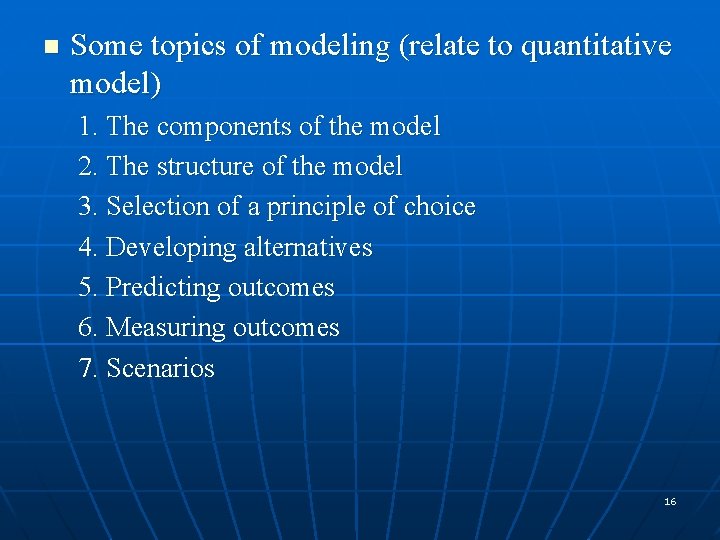
n Some topics of modeling (relate to quantitative model) 1. The components of the model 2. The structure of the model 3. Selection of a principle of choice 4. Developing alternatives 5. Predicting outcomes 6. Measuring outcomes 7. Scenarios 16
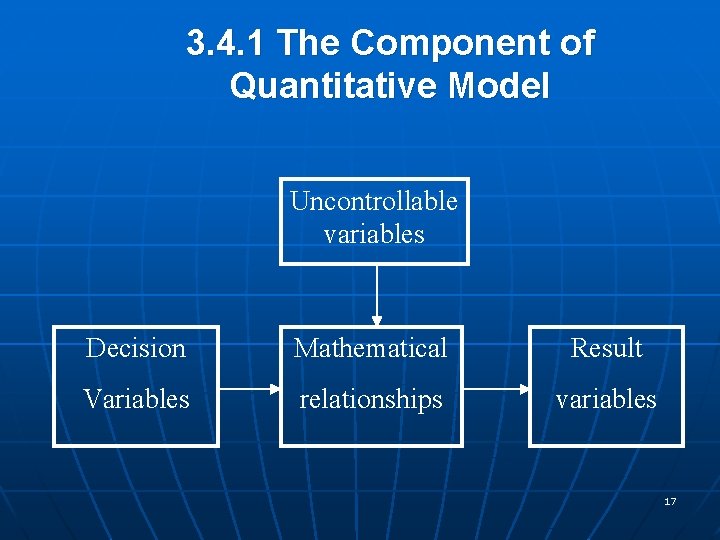
3. 4. 1 The Component of Quantitative Model Uncontrollable variables Decision Mathematical Result Variables relationships variables 17
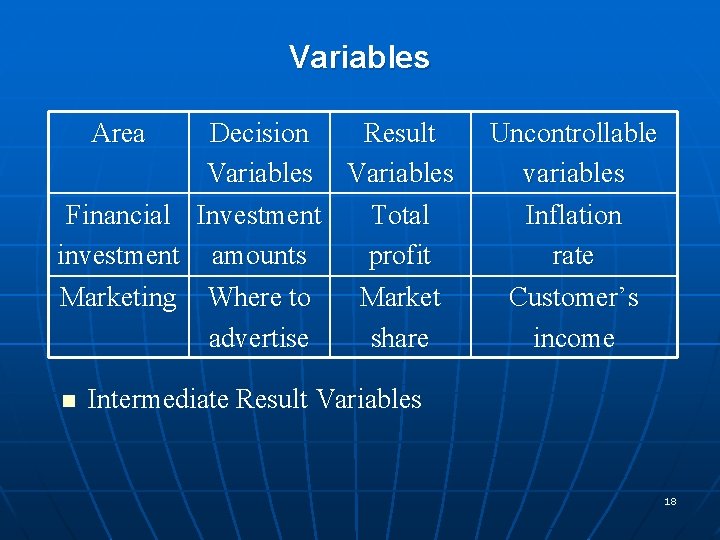
Variables Area Financial investment Marketing n Decision Result Variables Investment Total amounts profit Where to Market advertise share Uncontrollable variables Inflation rate Customer’s income Intermediate Result Variables 18
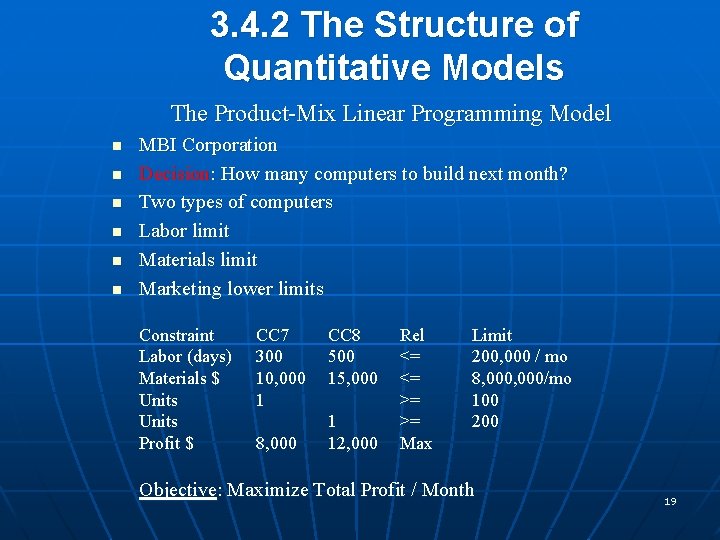
3. 4. 2 The Structure of Quantitative Models The Product-Mix Linear Programming Model n n n MBI Corporation Decision: How many computers to build next month? Two types of computers Labor limit Materials limit Marketing lower limits Constraint Labor (days) Materials $ Units Profit $ CC 7 300 10, 000 1 8, 000 CC 8 500 15, 000 1 12, 000 Rel <= <= >= >= Max Limit 200, 000 / mo 8, 000/mo 100 200 Objective: Maximize Total Profit / Month 19
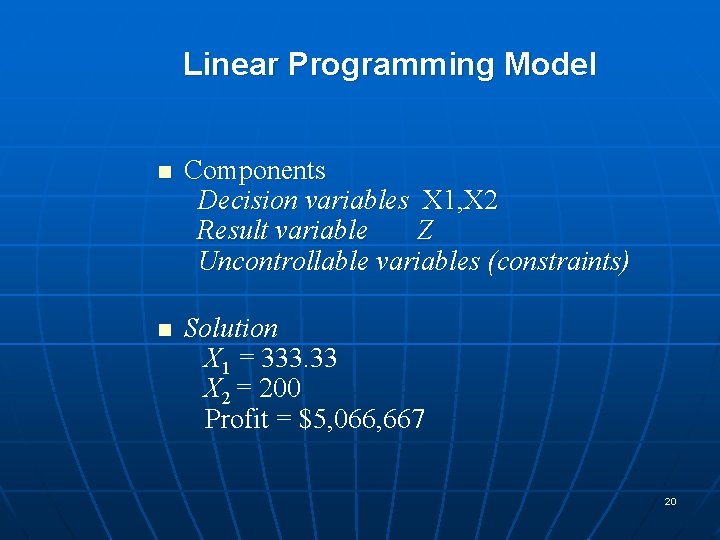
Linear Programming Model n Components Decision variables X 1, X 2 Result variable Z Uncontrollable variables (constraints) n Solution X 1 = 333. 33 X 2 = 200 Profit = $5, 066, 667 20
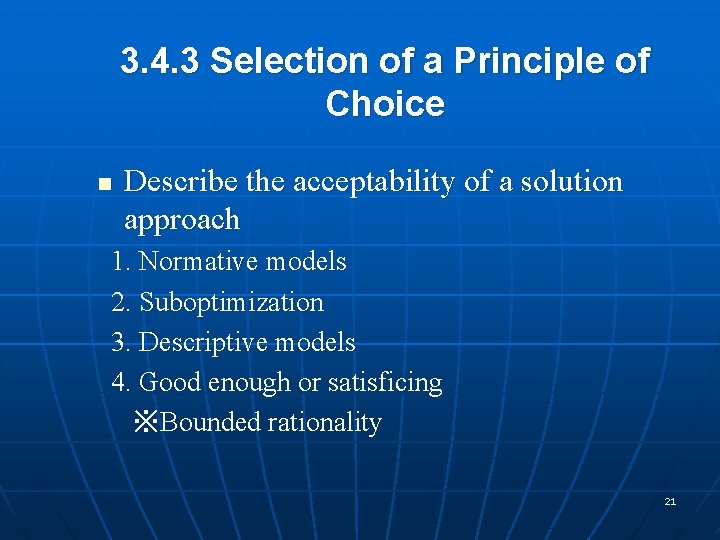
3. 4. 3 Selection of a Principle of Choice n Describe the acceptability of a solution approach 1. Normative models 2. Suboptimization 3. Descriptive models 4. Good enough or satisficing ※Bounded rationality 21
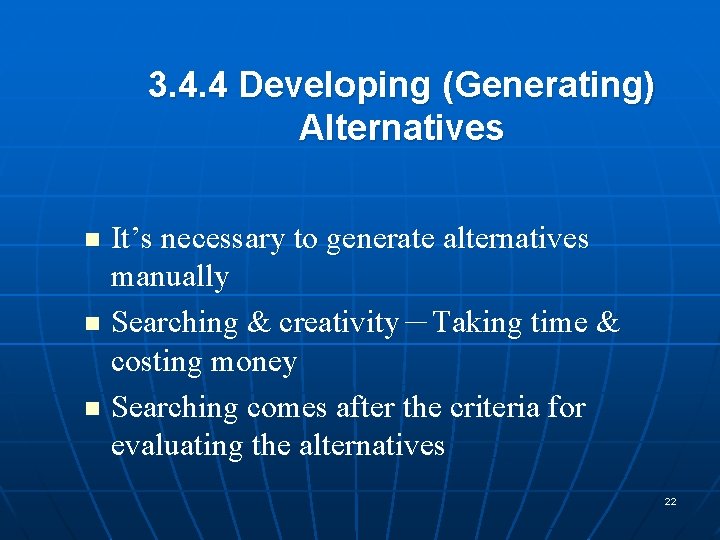
3. 4. 4 Developing (Generating) Alternatives It’s necessary to generate alternatives manually n Searching & creativity-Taking time & costing money n Searching comes after the criteria for evaluating the alternatives n 22
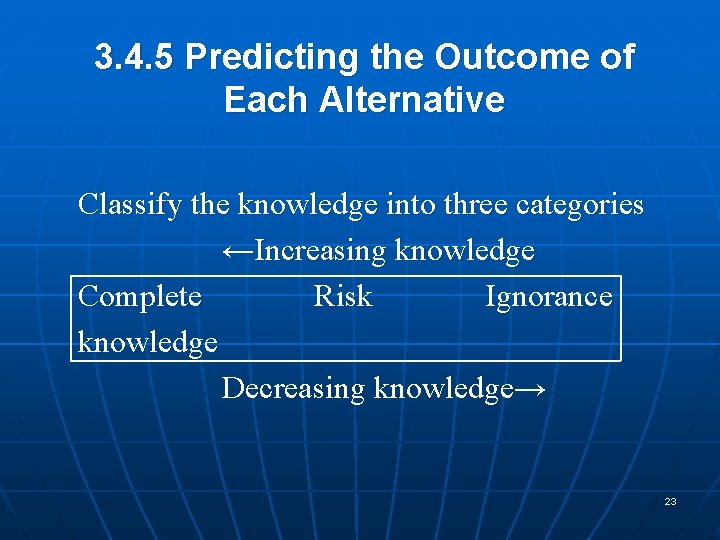
3. 4. 5 Predicting the Outcome of Each Alternative Classify the knowledge into three categories ←Increasing knowledge Complete Risk Ignorance knowledge Decreasing knowledge→ 23
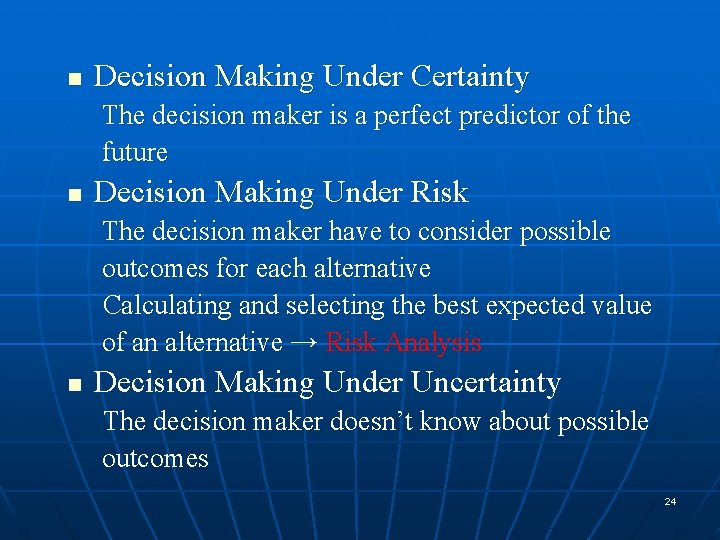
n Decision Making Under Certainty The decision maker is a perfect predictor of the future n Decision Making Under Risk The decision maker have to consider possible outcomes for each alternative Calculating and selecting the best expected value of an alternative → Risk Analysis n Decision Making Under Uncertainty The decision maker doesn’t know about possible outcomes 24
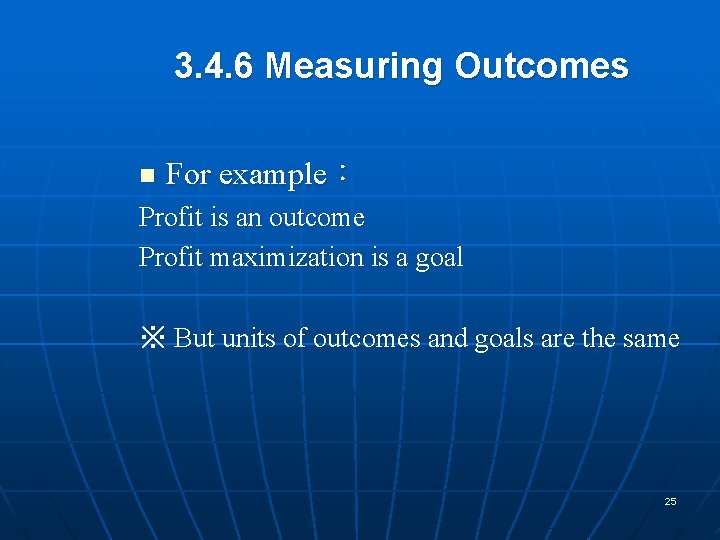
3. 4. 6 Measuring Outcomes n For example: Profit is an outcome Profit maximization is a goal ※ But units of outcomes and goals are the same 25
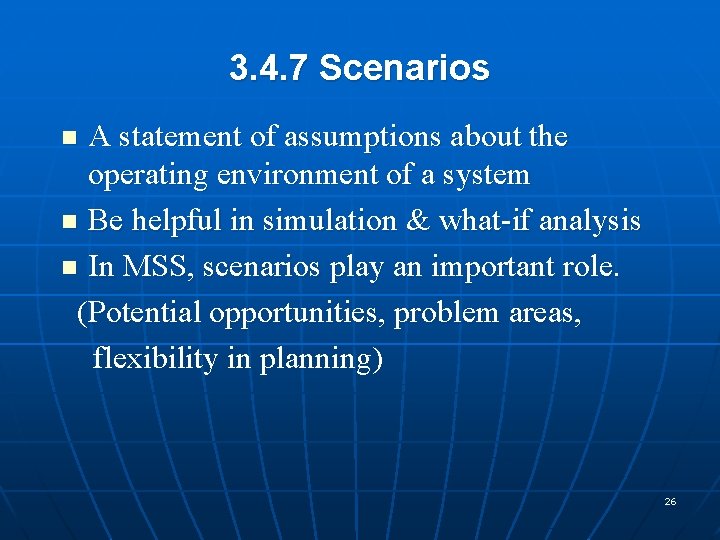
3. 4. 7 Scenarios A statement of assumptions about the operating environment of a system n Be helpful in simulation & what-if analysis n In MSS, scenarios play an important role. (Potential opportunities, problem areas, flexibility in planning) n 26
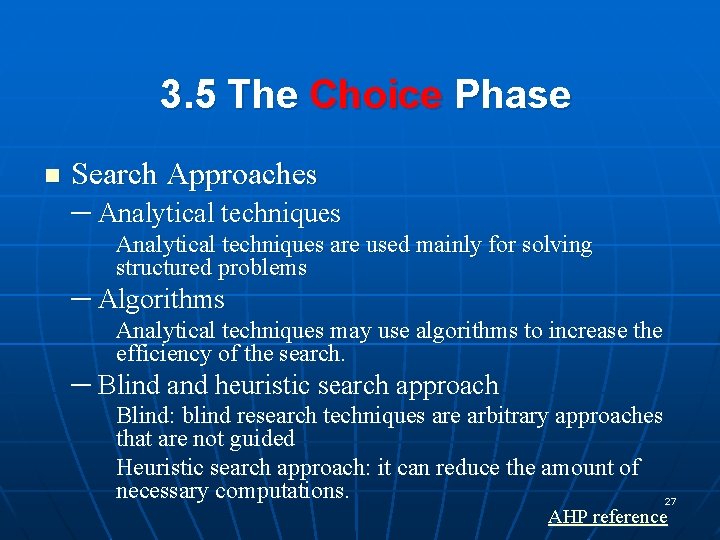
3. 5 The Choice Phase n Search Approaches ─ Analytical techniques are used mainly for solving structured problems ─ Algorithms Analytical techniques may use algorithms to increase the efficiency of the search. ─ Blind and heuristic search approach Blind: blind research techniques are arbitrary approaches that are not guided Heuristic search approach: it can reduce the amount of necessary computations. 27 AHP reference
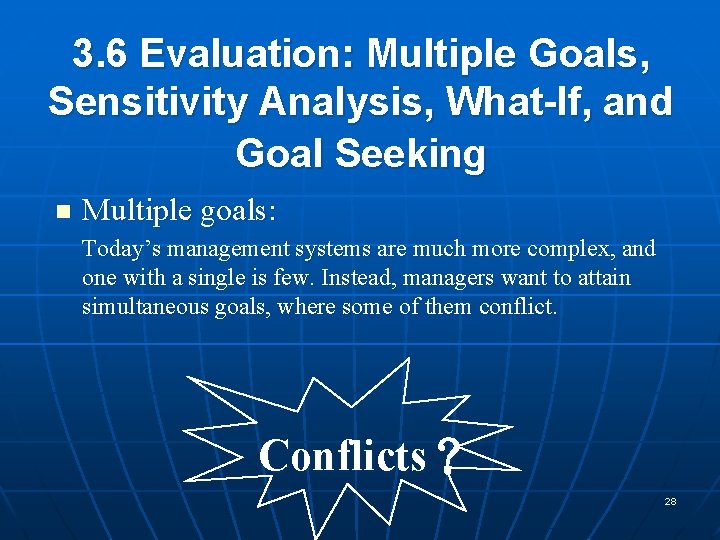
3. 6 Evaluation: Multiple Goals, Sensitivity Analysis, What-If, and Goal Seeking n Multiple goals: Today’s management systems are much more complex, and one with a single is few. Instead, managers want to attain simultaneous goals, where some of them conflict. Conflicts? 28
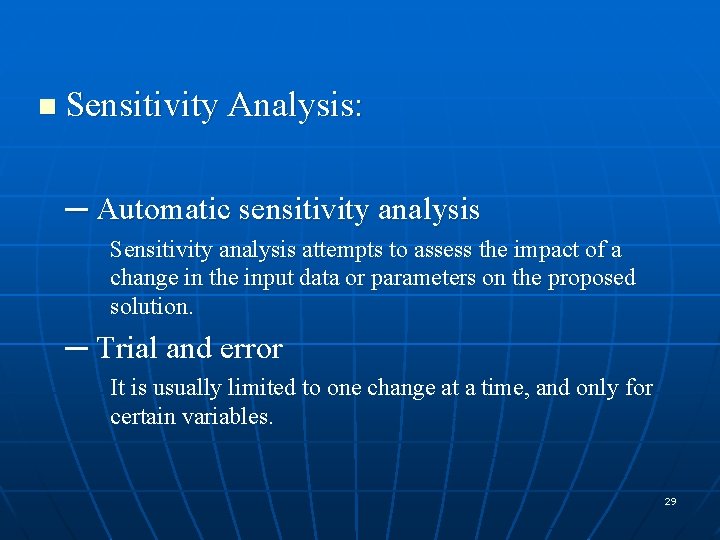
n Sensitivity Analysis: ─ Automatic sensitivity analysis Sensitivity analysis attempts to assess the impact of a change in the input data or parameters on the proposed solution. ─ Trial and error It is usually limited to one change at a time, and only for certain variables. 29
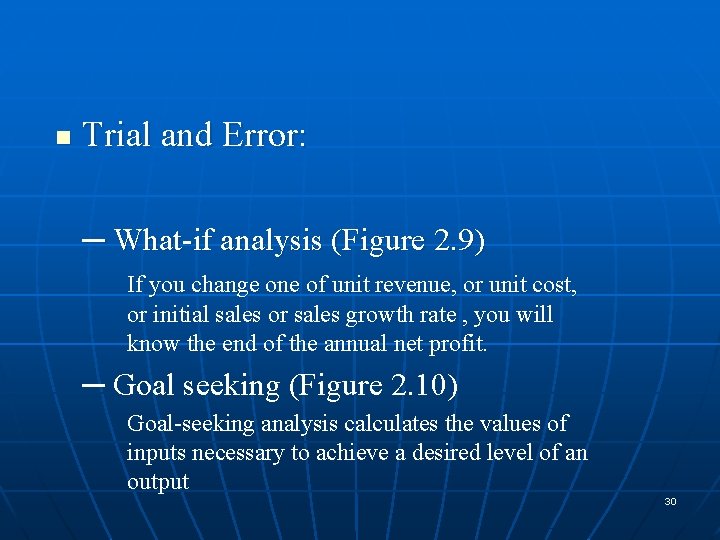
n Trial and Error: ─ What-if analysis (Figure 2. 9) If you change one of unit revenue, or unit cost, or initial sales or sales growth rate , you will know the end of the annual net profit. ─ Goal seeking (Figure 2. 10) Goal-seeking analysis calculates the values of inputs necessary to achieve a desired level of an output 30
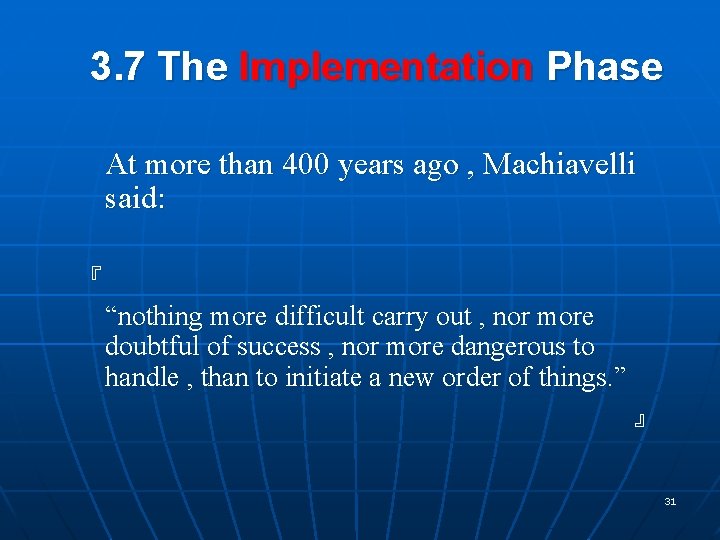
3. 7 The Implementation Phase At more than 400 years ago , Machiavelli said: 『 “nothing more difficult carry out , nor more doubtful of success , nor more dangerous to handle , than to initiate a new order of things. ” 』 31
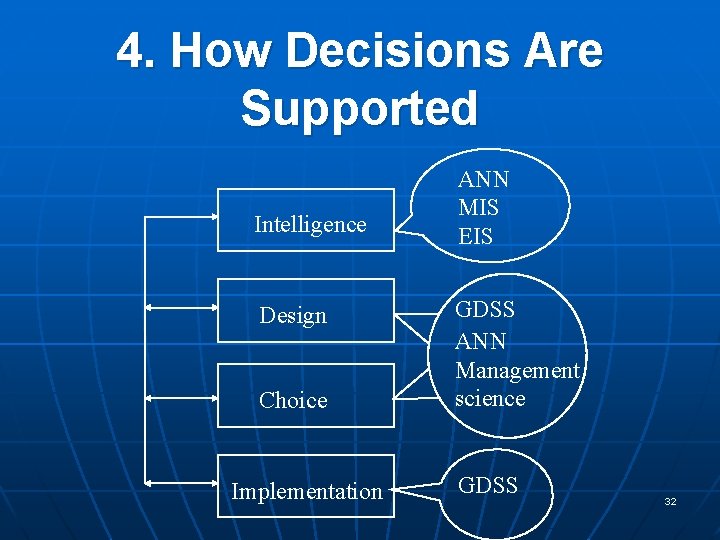
4. How Decisions Are Supported Intelligence Design Choice Implementation ANN MIS EIS GDSS ANN Management science GDSS 32
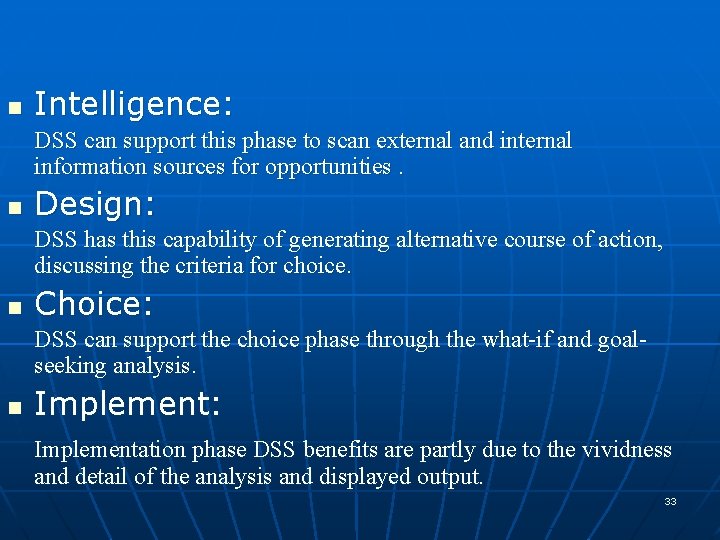
n Intelligence: DSS can support this phase to scan external and internal information sources for opportunities. n Design: DSS has this capability of generating alternative course of action, discussing the criteria for choice. n Choice: DSS can support the choice phase through the what-if and goalseeking analysis. n Implement: Implementation phase DSS benefits are partly due to the vividness and detail of the analysis and displayed output. 33
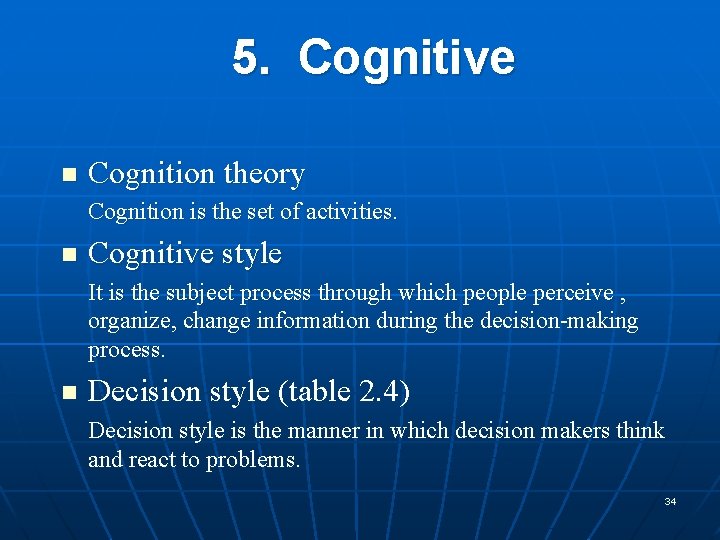
5. Cognitive n Cognition theory Cognition is the set of activities. n Cognitive style It is the subject process through which people perceive , organize, change information during the decision-making process. n Decision style (table 2. 4) Decision style is the manner in which decision makers think and react to problems. 34
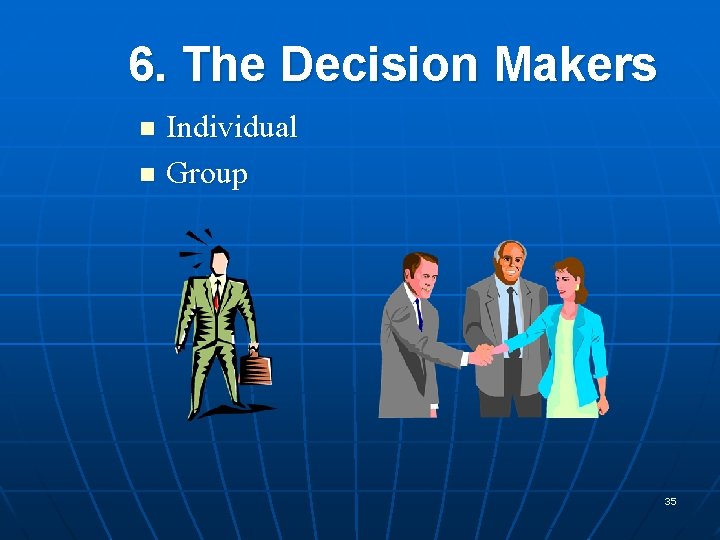
6. The Decision Makers Individual n Group n 35
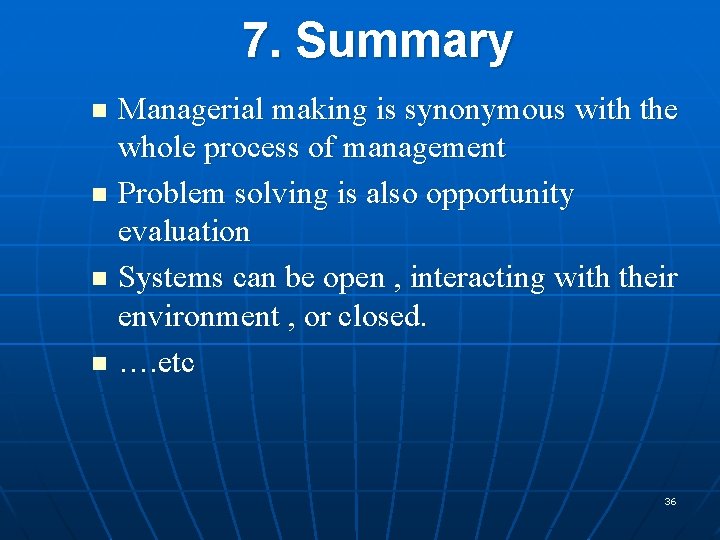
7. Summary Managerial making is synonymous with the whole process of management n Problem solving is also opportunity evaluation n Systems can be open , interacting with their environment , or closed. n …. etc n 36
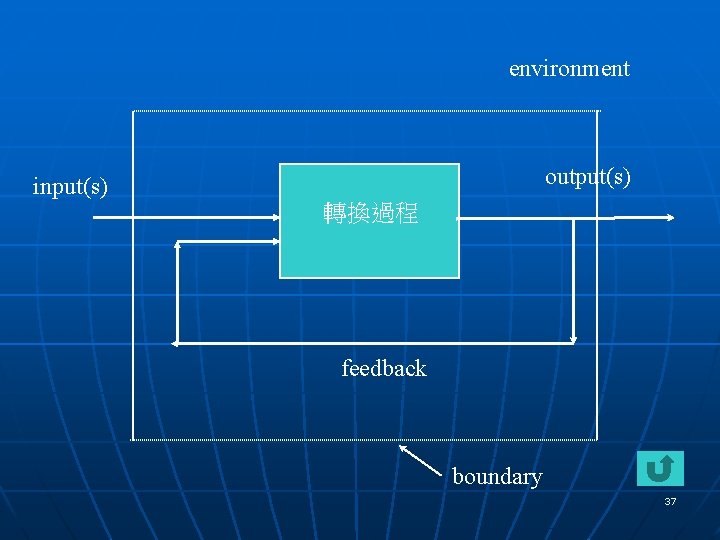
environment output(s) input(s) 轉換過程 feedback boundary 37
Decision support systems and intelligent systems
Chapter 2 economic systems and decision making
Chapter 2 economic systems and decision making
Chapter 2 economic systems and decision making
Objectives of decision making
Financial management process
Decision support and business intelligence systems
Decision support system in business intelligence
Decision support and expert systems
Modeling and role modeling theory
How do information systems aid in decision making
Developing spreadsheet-based decision support systems
Relational vs dimensional data modeling
Chapter 6 prices and decision making assessment answers
Chapter 11 decision making and relevant information
Management chapter 5 planning and decision making
Chapter 11 decision making and relevant information
Chapter 2 management supervision and decision making
Relevant information for decision making
Options-based planning
Chapter 1 section 3 economic choices and decision making
Sequential decision analytics and modeling
Financial decision making process
Copyright
Relevant cost for decision making solution chapter 13
Modeling logic with decision tables
Cnnx11
Modeling logic with decision tables
Explain the decision tree modeling for capacity expansion
Manufacturing systems modeling and analysis
Decision tree and decision table examples
Using recursion in models and decision making
Using recursion in models and decision making sheet 3
Hoy and tarter decision making model
Decision making and relevant information
Best books on problem solving and decision making
Individual and group decision making
Individual and group decision making