Variations in MIK Class Means Jeremy Vincent Variations
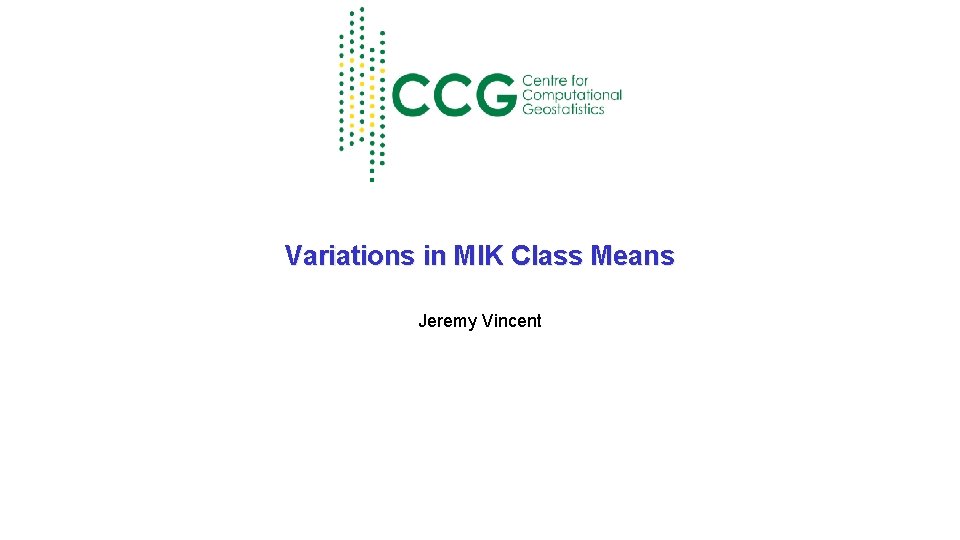
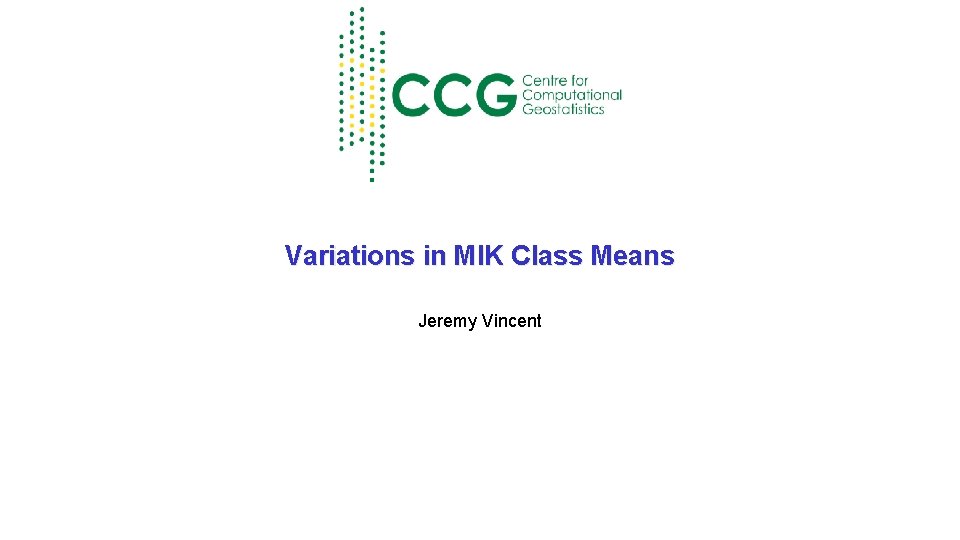
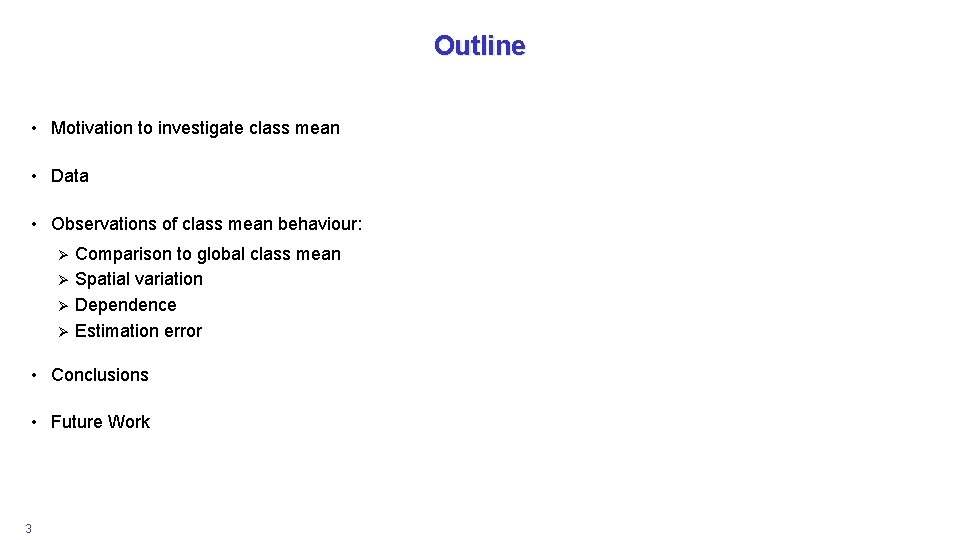
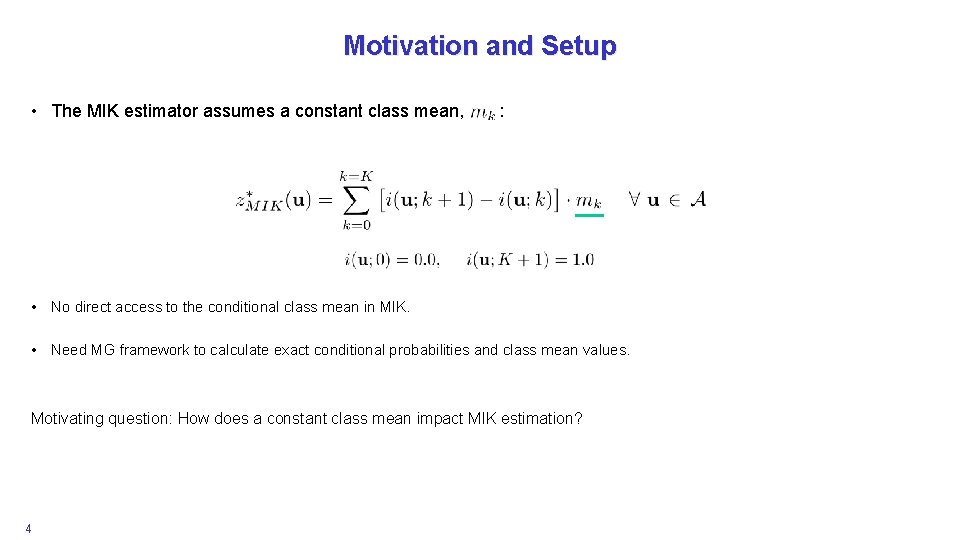
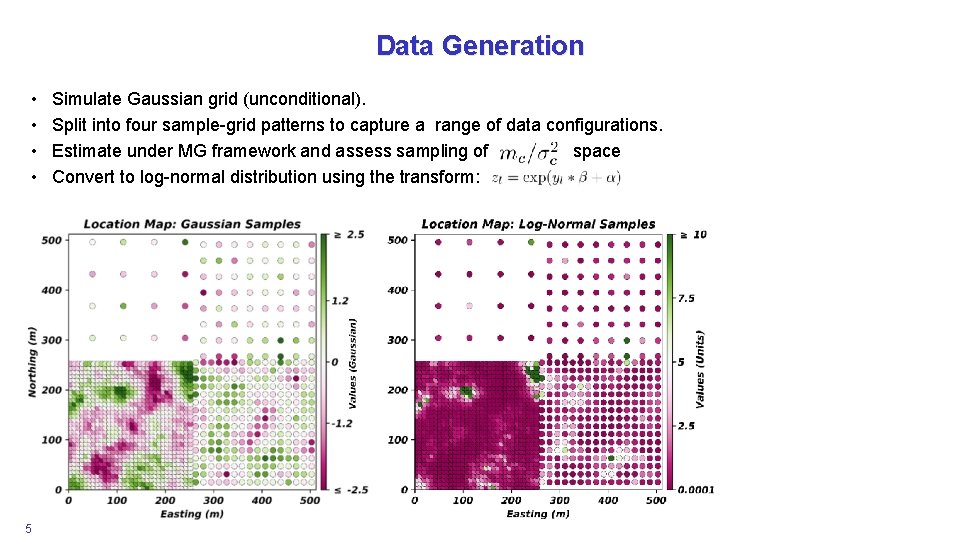
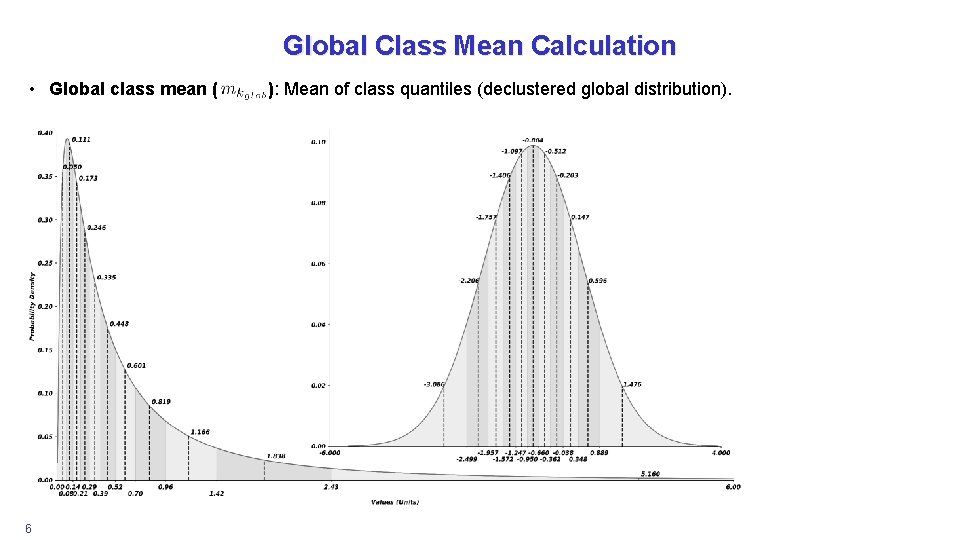
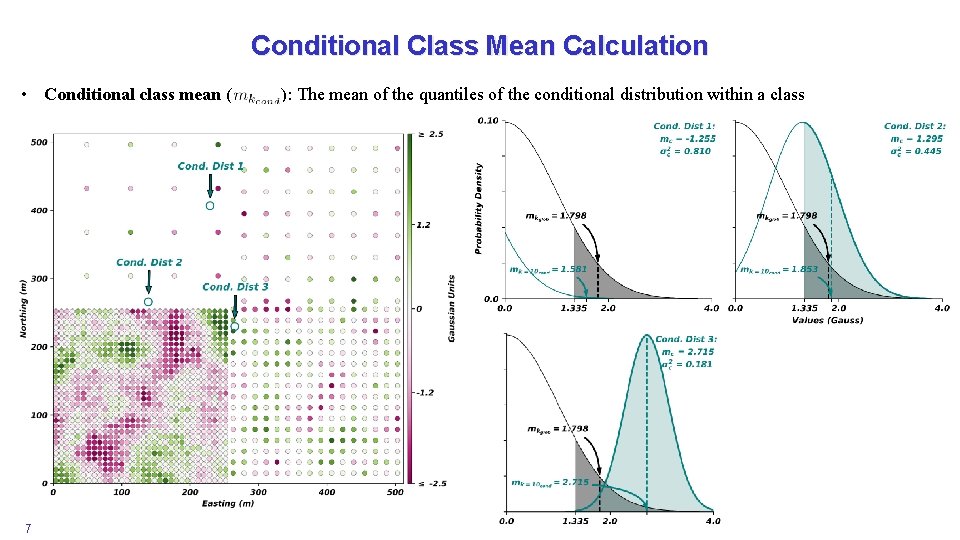
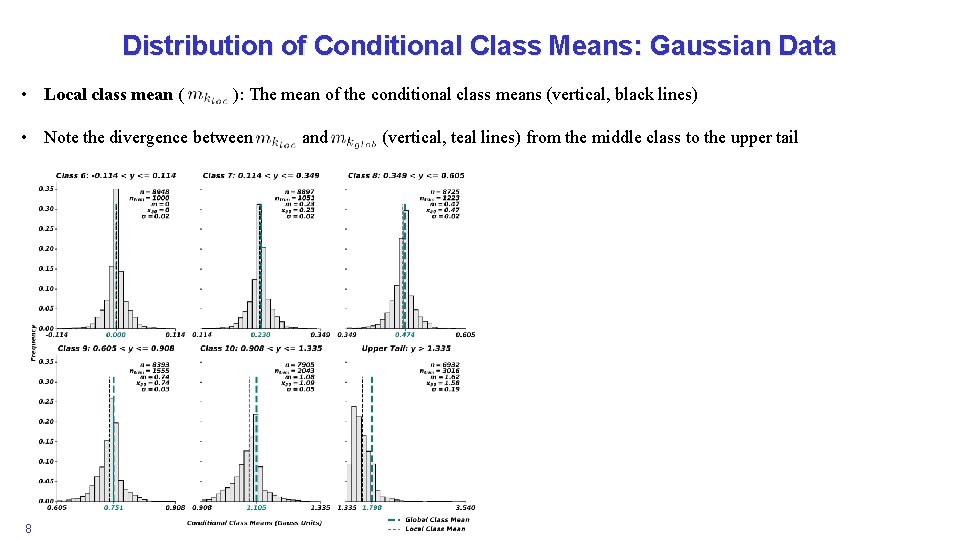
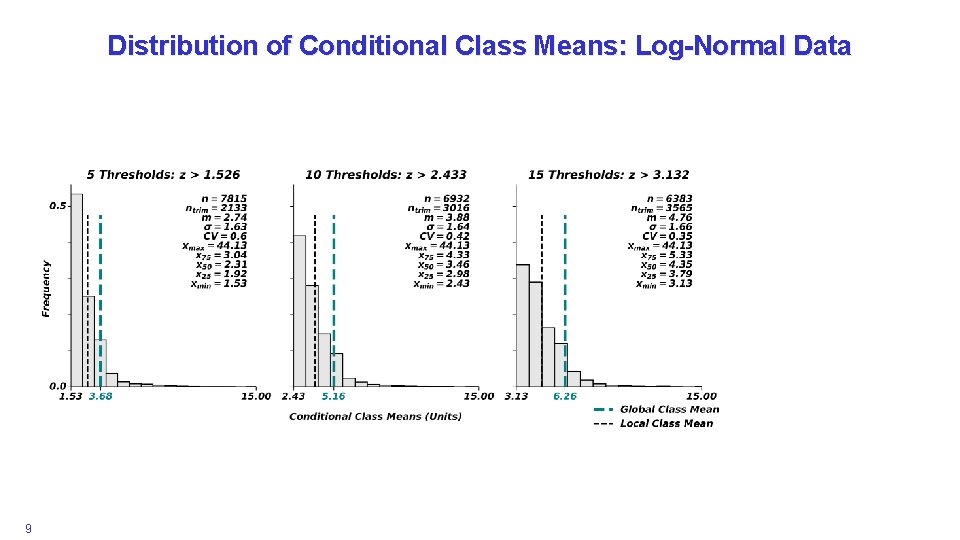
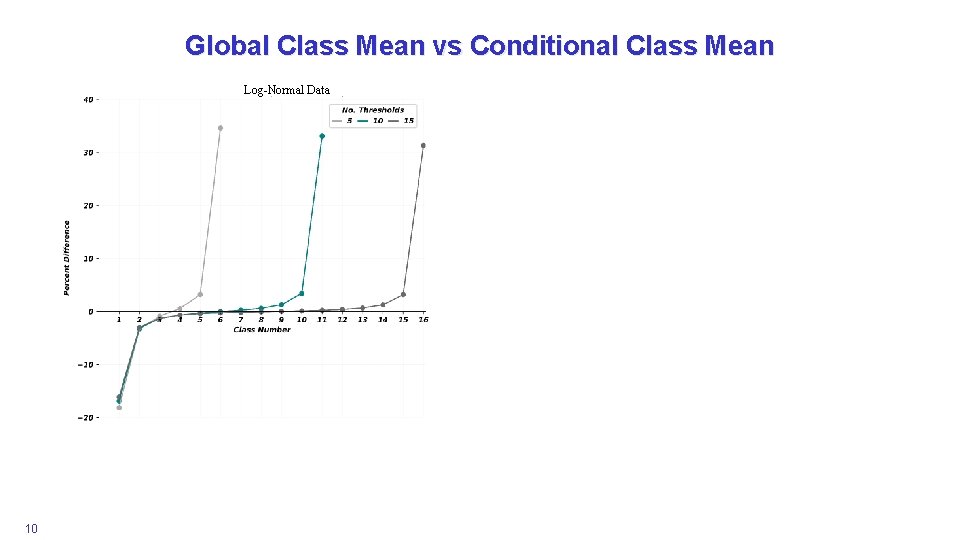
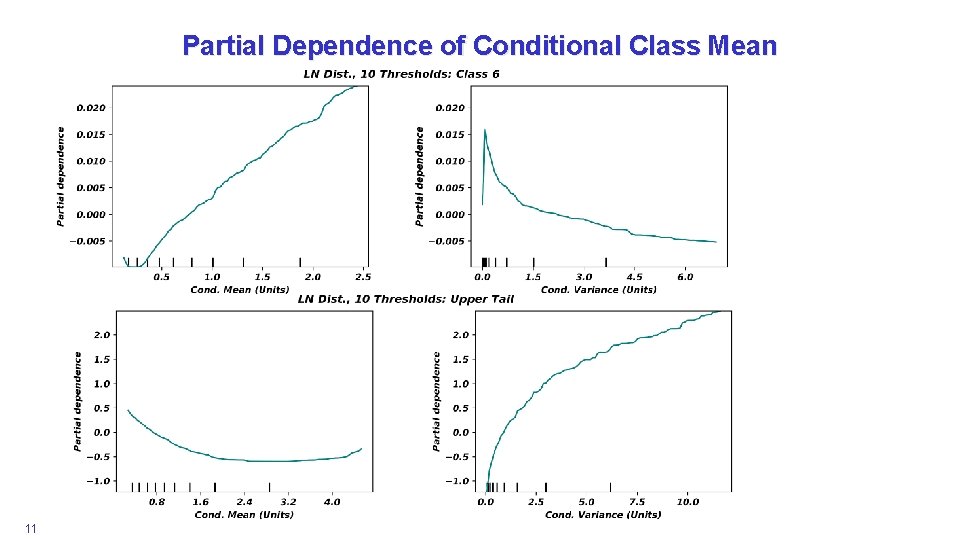
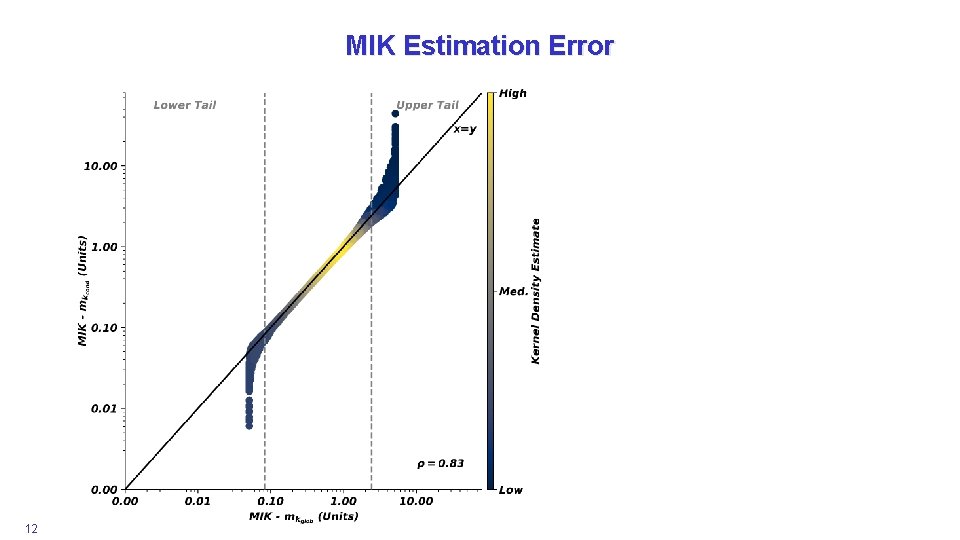
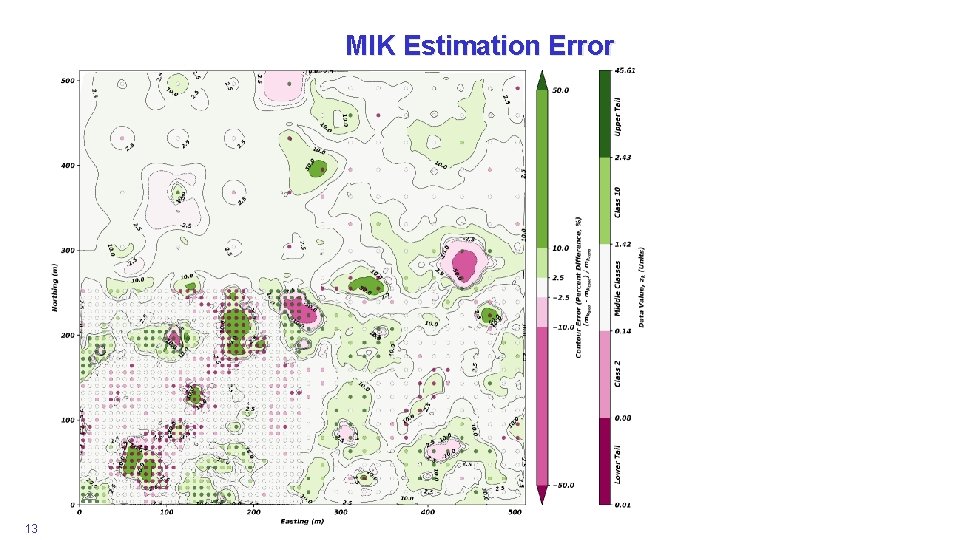
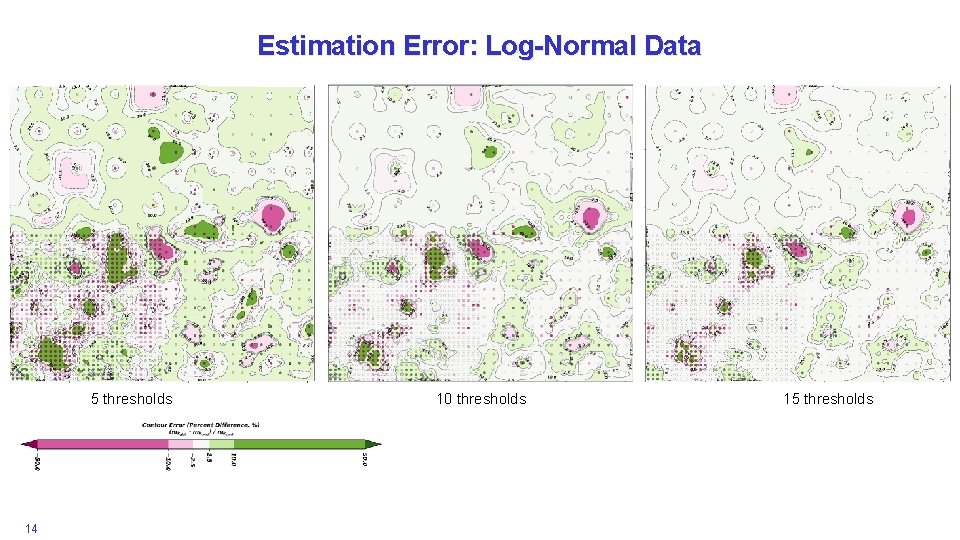
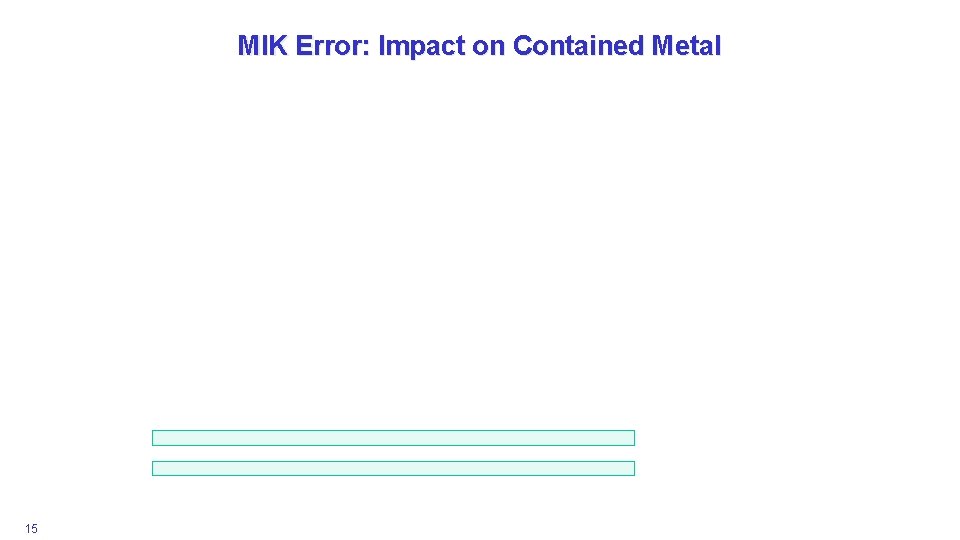
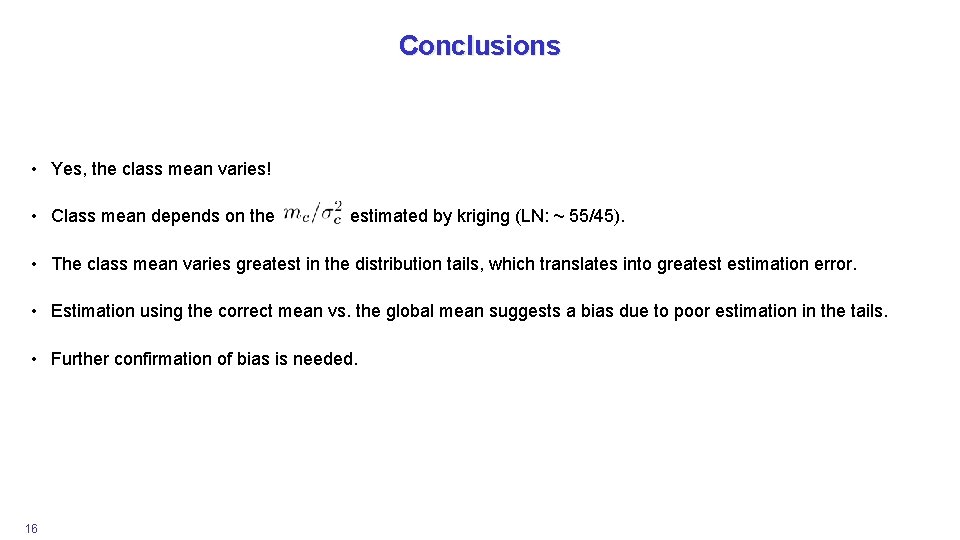
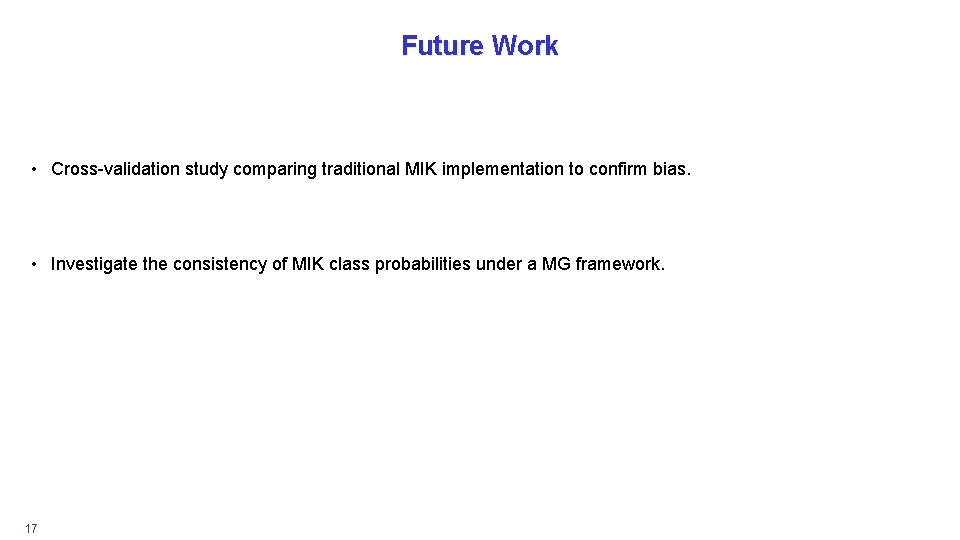
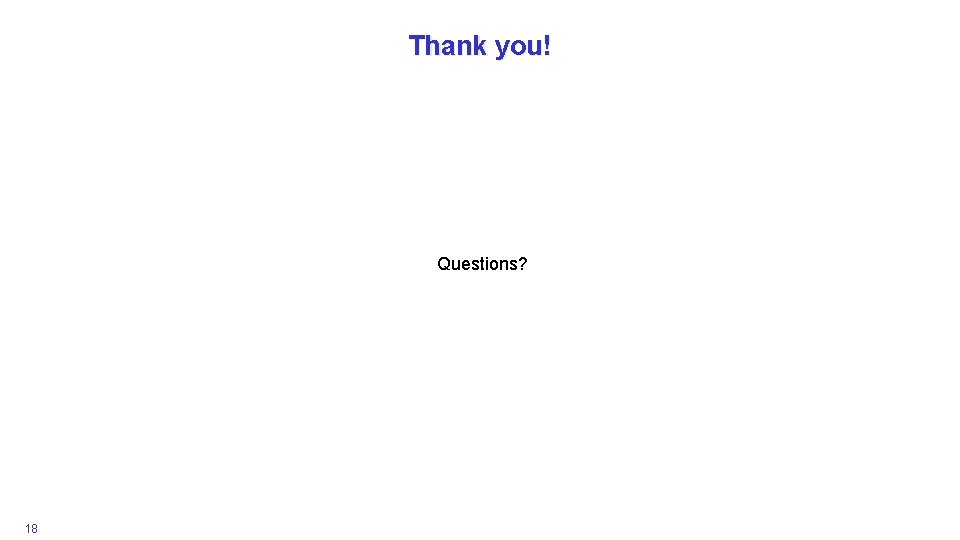
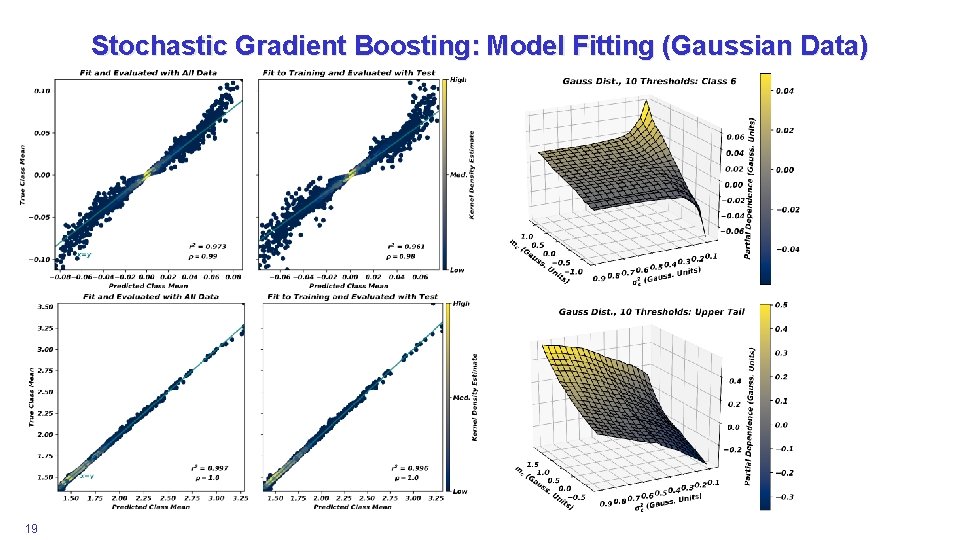
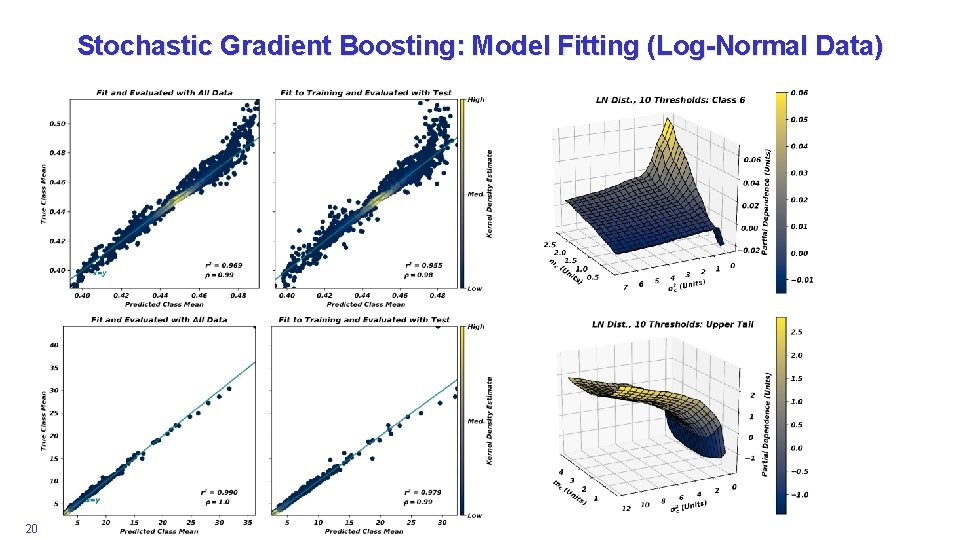
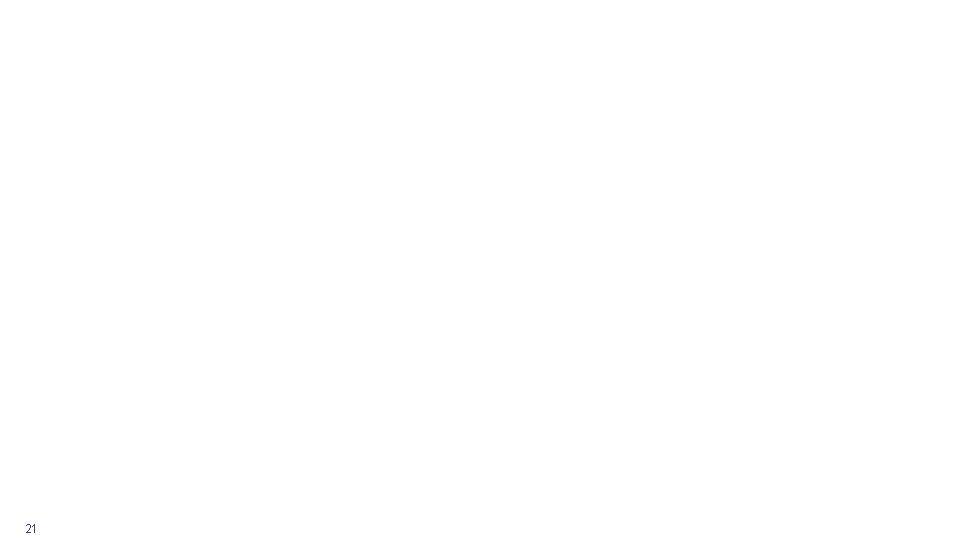
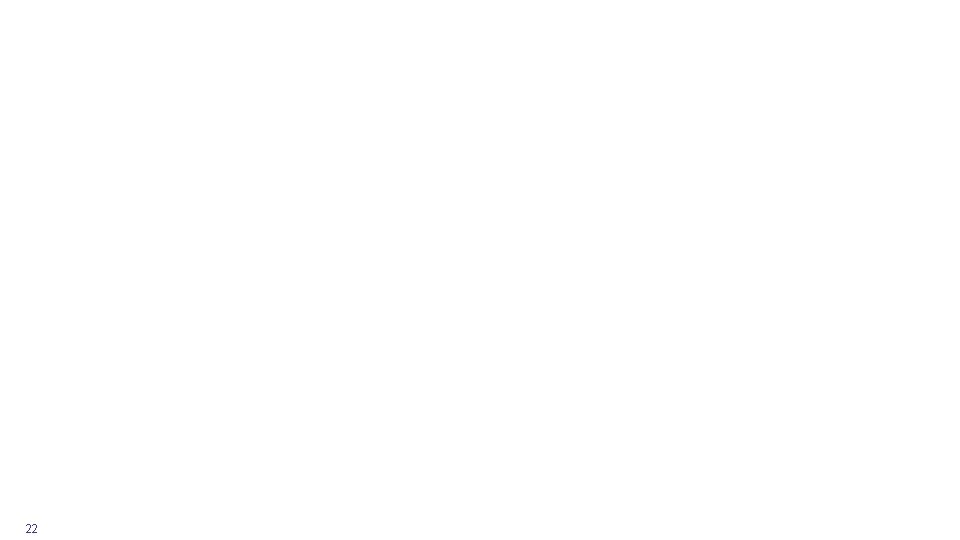
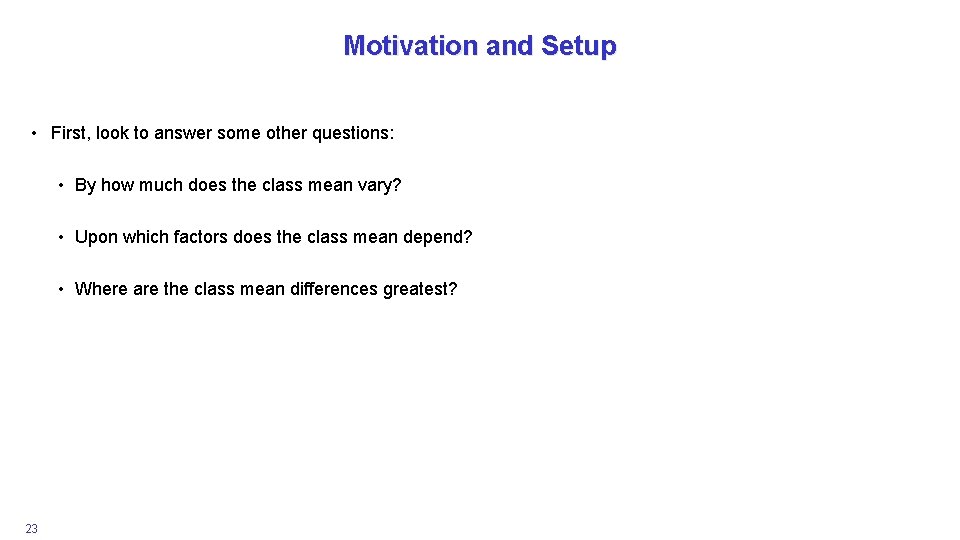
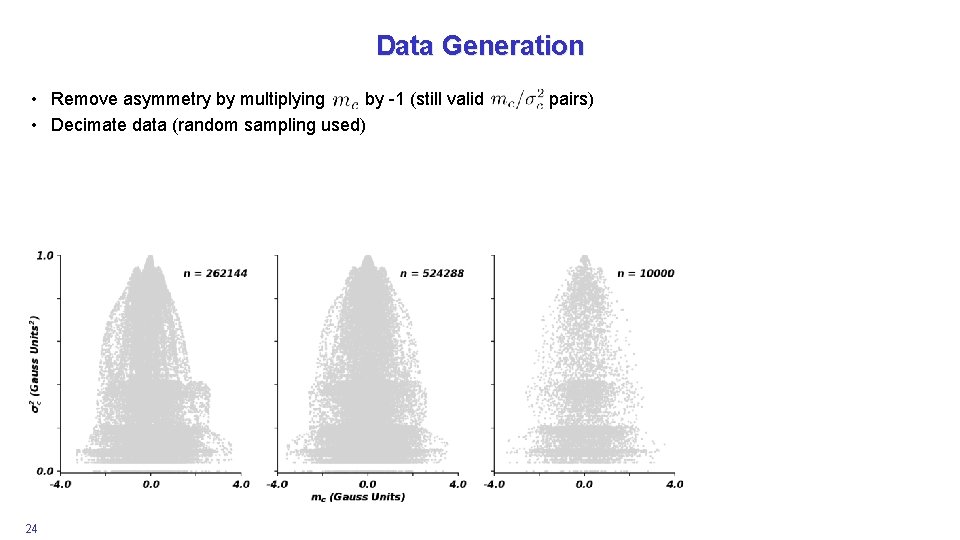
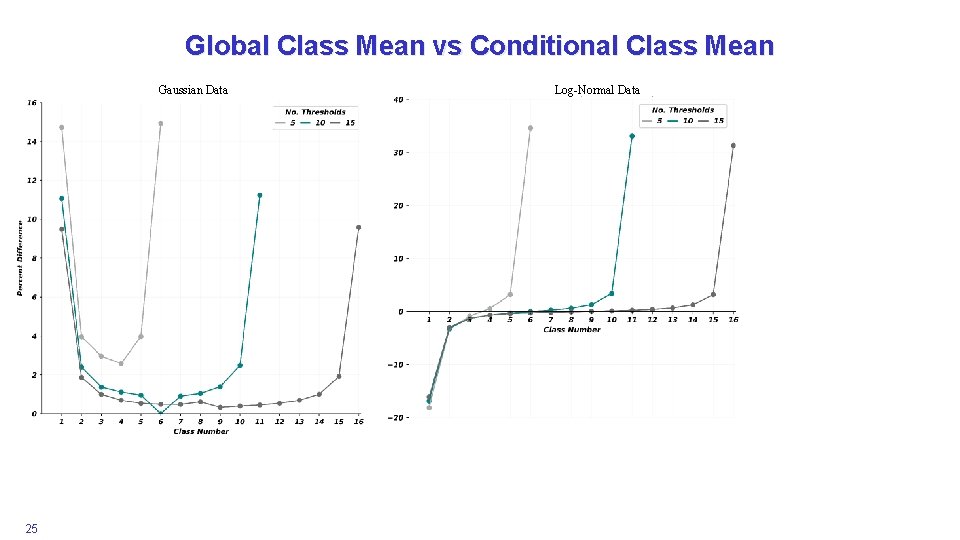
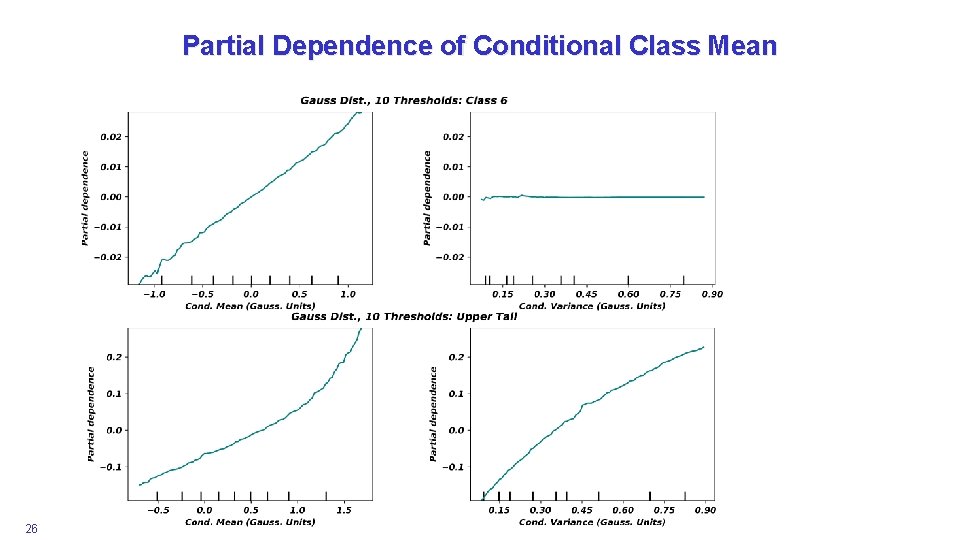
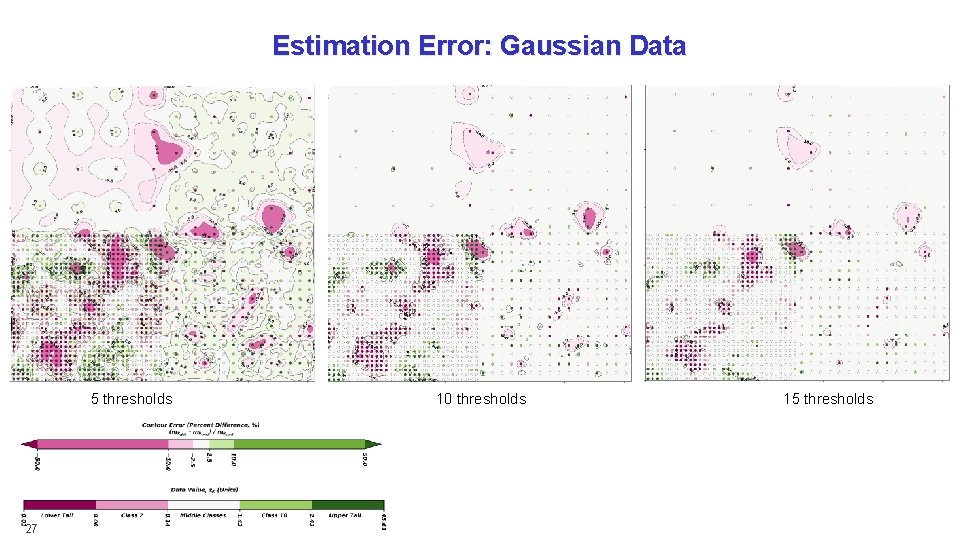
- Slides: 27
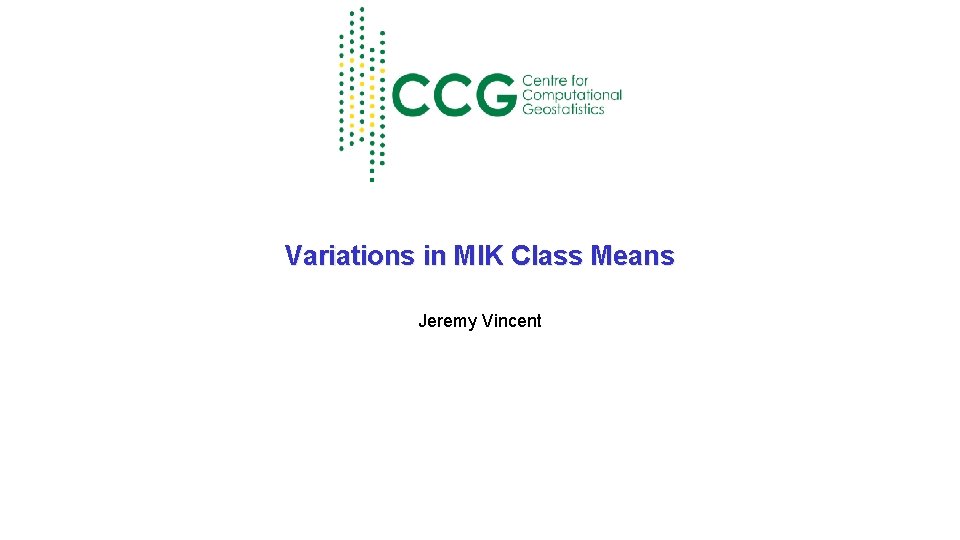
Variations in MIK Class Means Jeremy Vincent
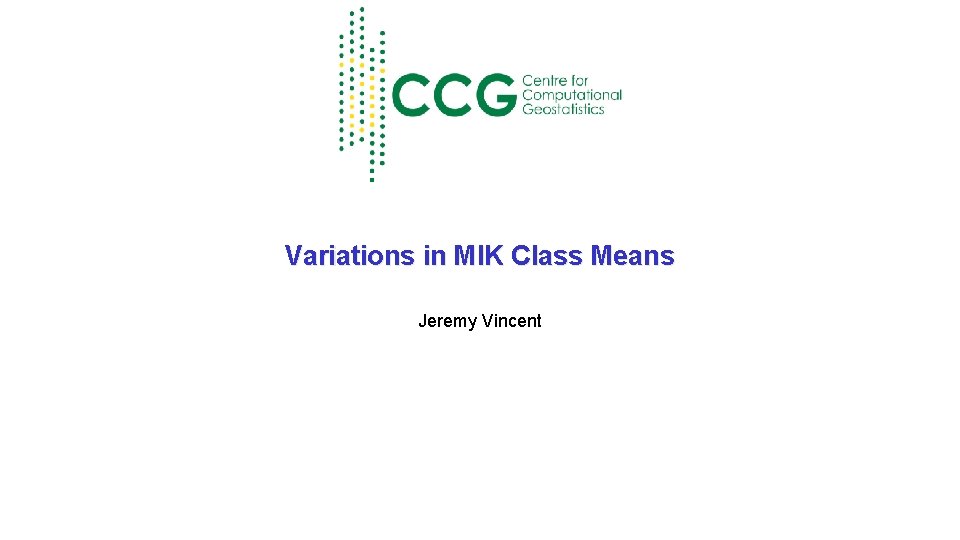
Variations in MIK Class Means Jeremy Vincent
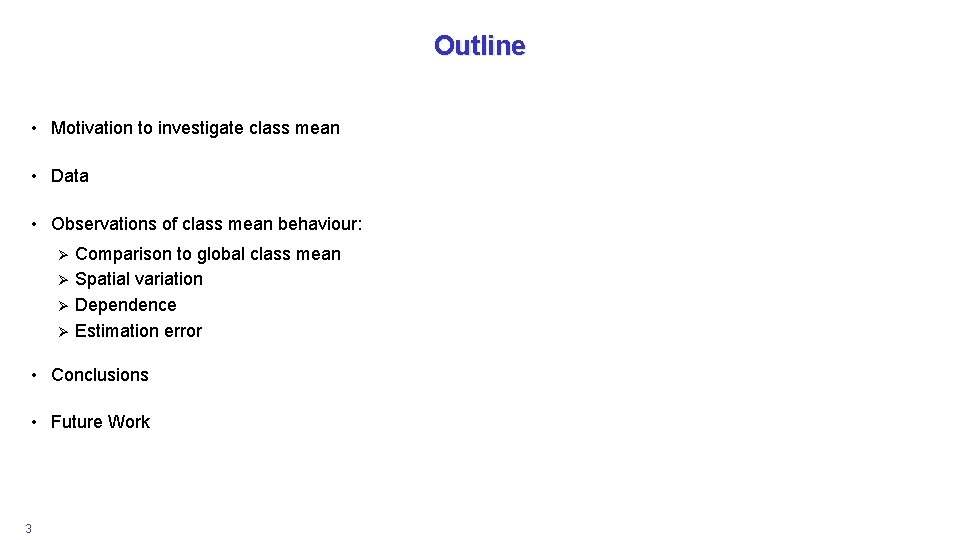
Outline • Motivation to investigate class mean • Data • Observations of class mean behaviour: Ø Ø Comparison to global class mean Spatial variation Dependence Estimation error • Conclusions • Future Work 3
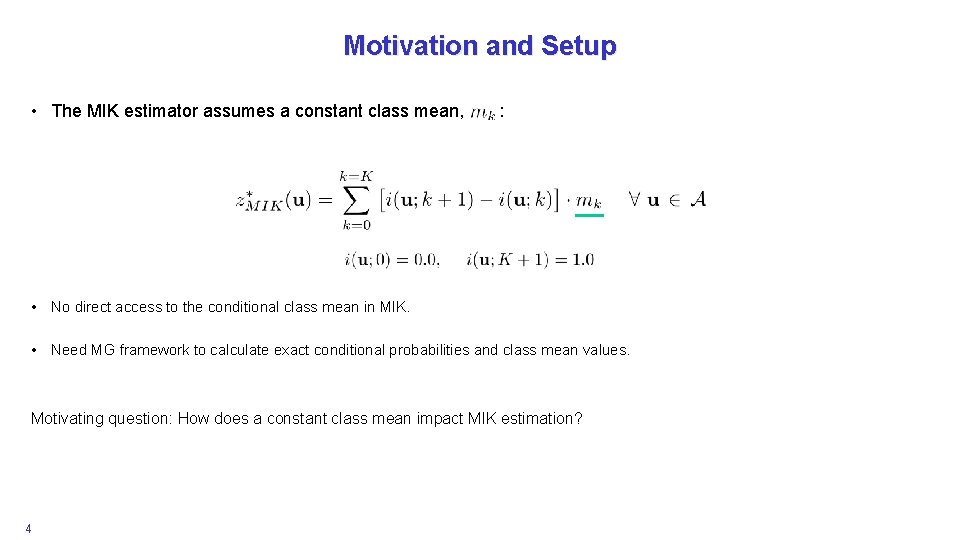
Motivation and Setup • The MIK estimator assumes a constant class mean, : • No direct access to the conditional class mean in MIK. • Need MG framework to calculate exact conditional probabilities and class mean values. Motivating question: How does a constant class mean impact MIK estimation? 4
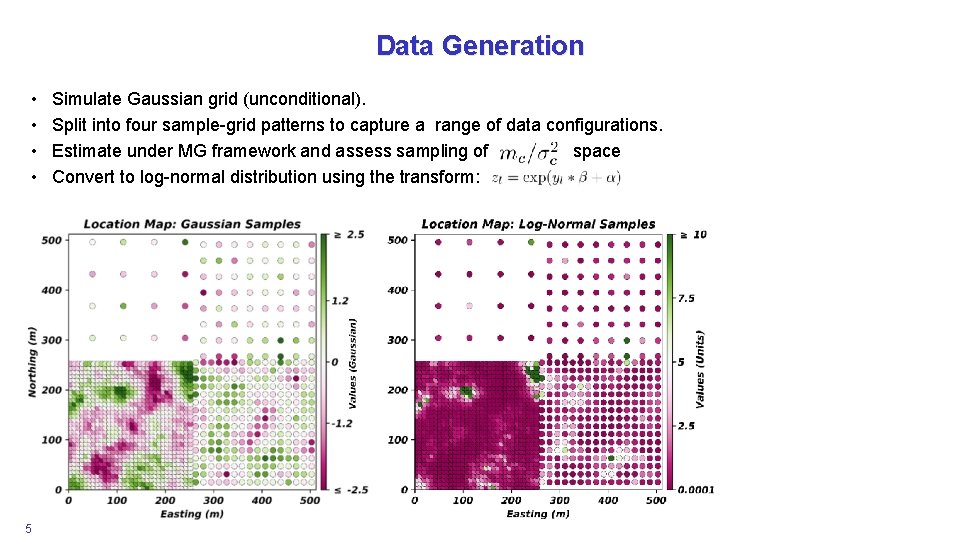
Data Generation • • 5 Simulate Gaussian grid (unconditional). Split into four sample-grid patterns to capture a range of data configurations. Estimate under MG framework and assess sampling of space Convert to log-normal distribution using the transform:
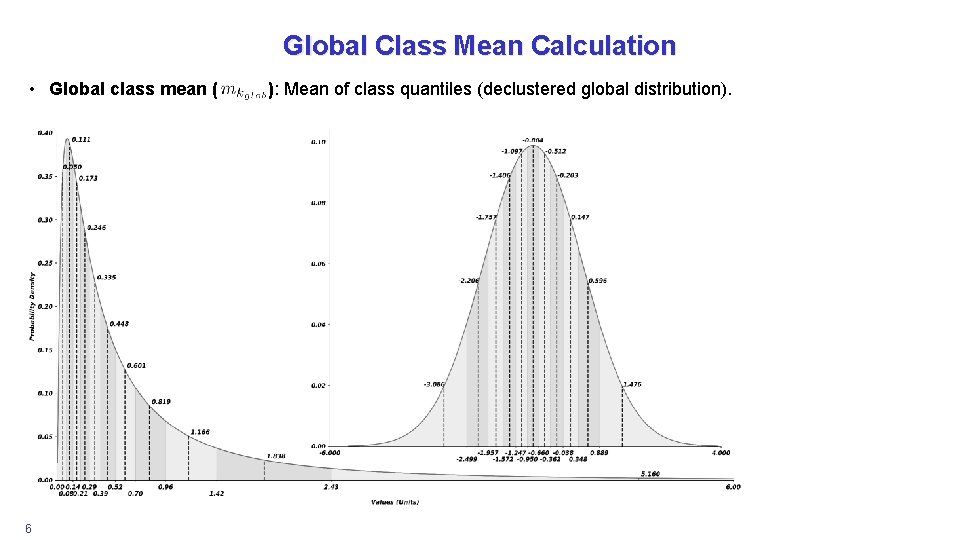
Global Class Mean Calculation • Global class mean ( 6 ): Mean of class quantiles (declustered global distribution).
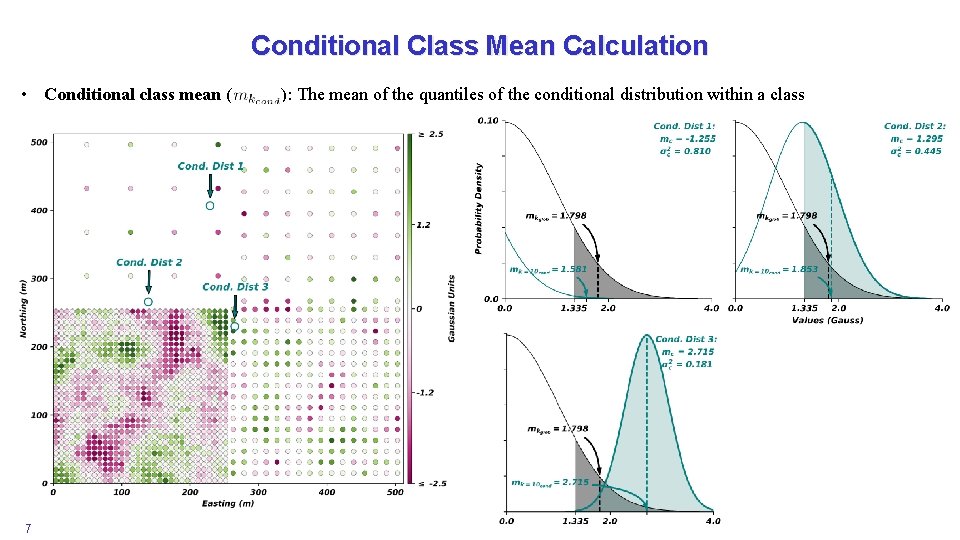
Conditional Class Mean Calculation • Conditional class mean ( 7 ): The mean of the quantiles of the conditional distribution within a class
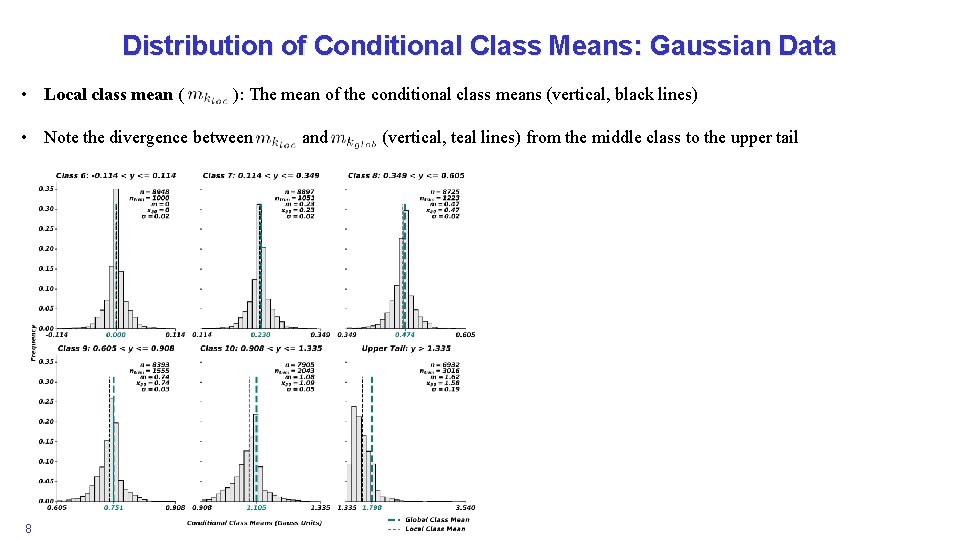
Distribution of Conditional Class Means: Gaussian Data • Local class mean ( ): The mean of the conditional class means (vertical, black lines) • Note the divergence between 8 and (vertical, teal lines) from the middle class to the upper tail
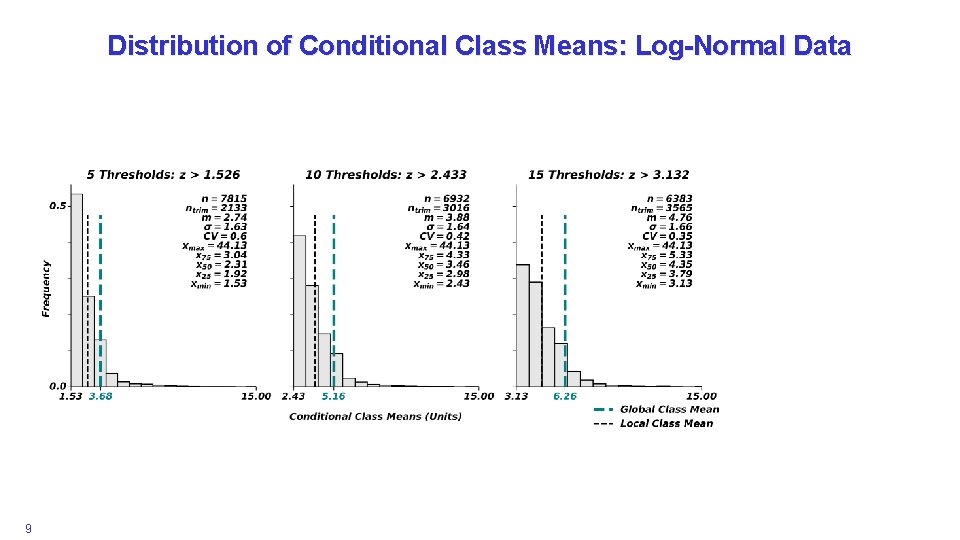
Distribution of Conditional Class Means: Log-Normal Data 9
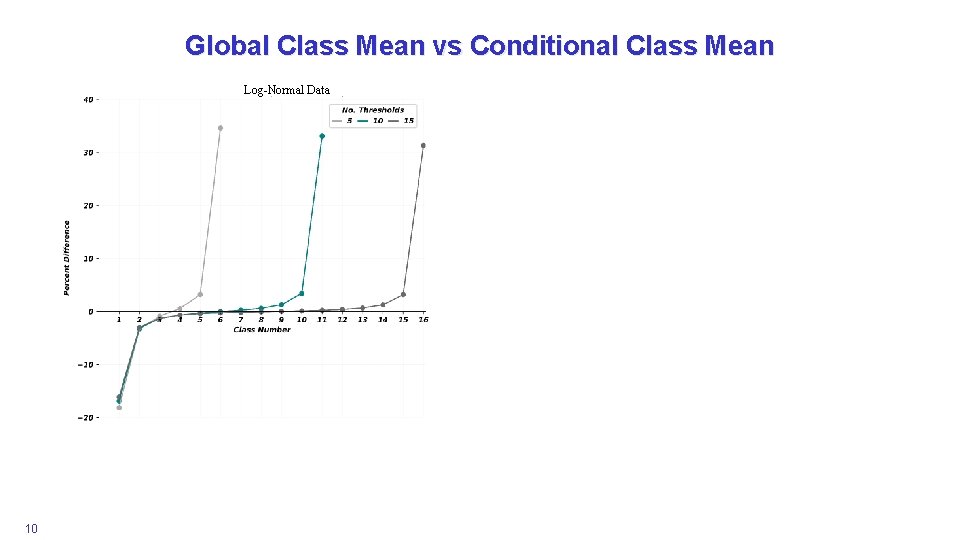
Global Class Mean vs Conditional Class Mean Log-Normal Data 10
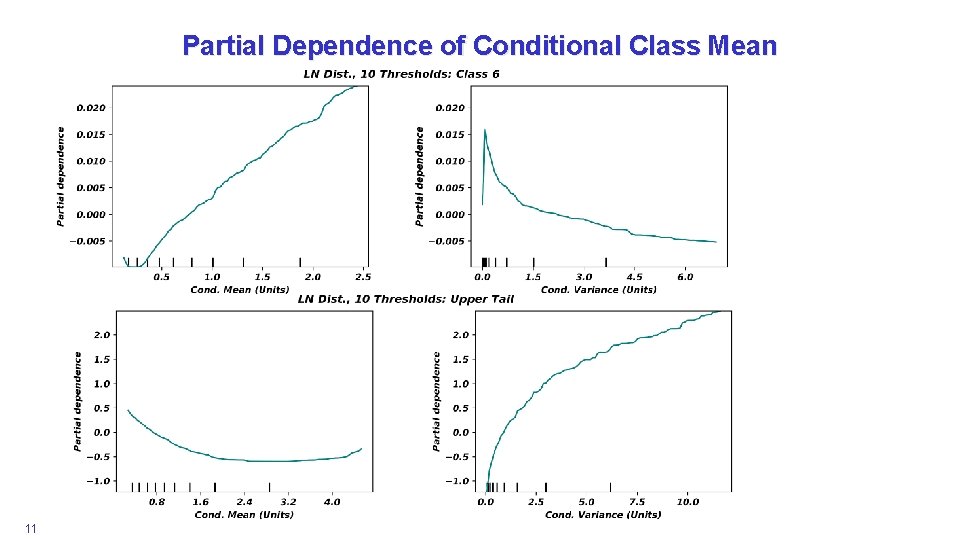
Partial Dependence of Conditional Class Mean 11
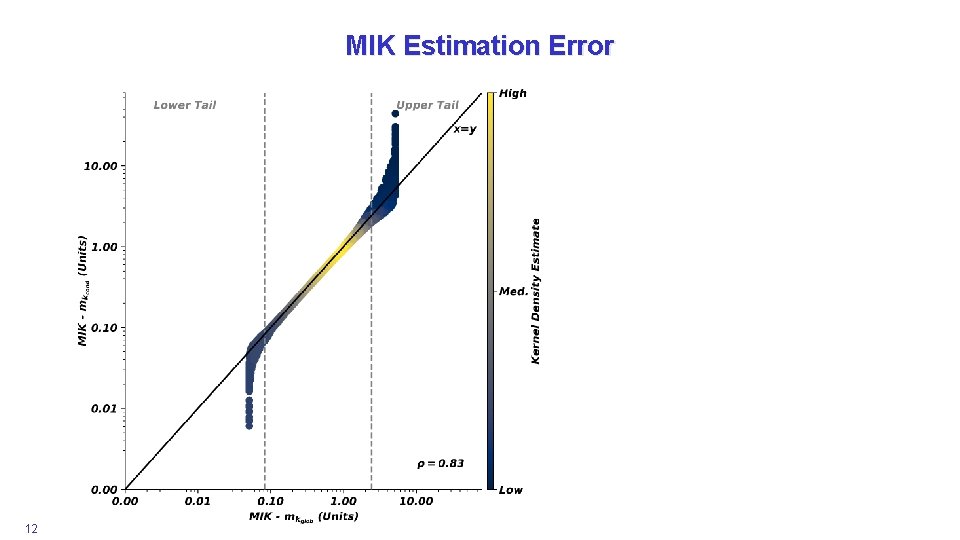
MIK Estimation Error 12
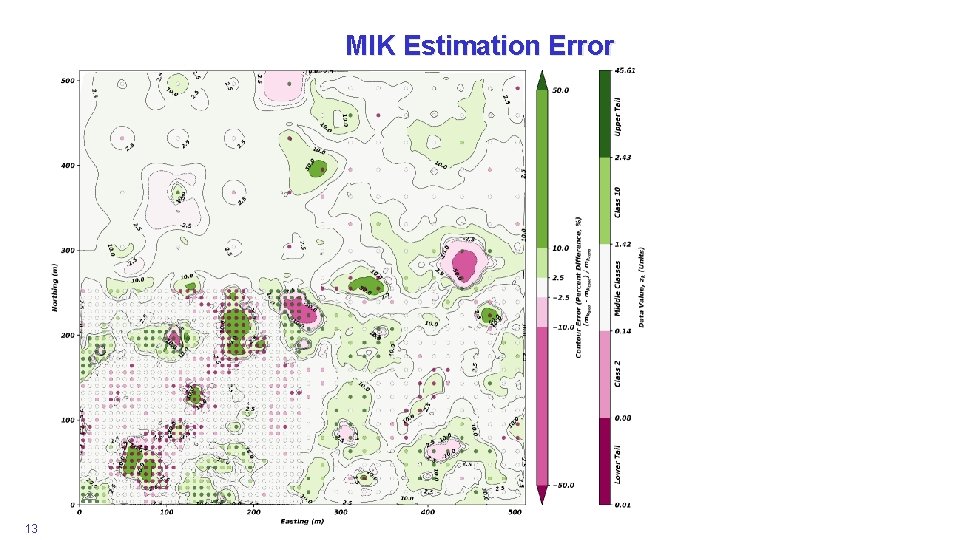
MIK Estimation Error 13
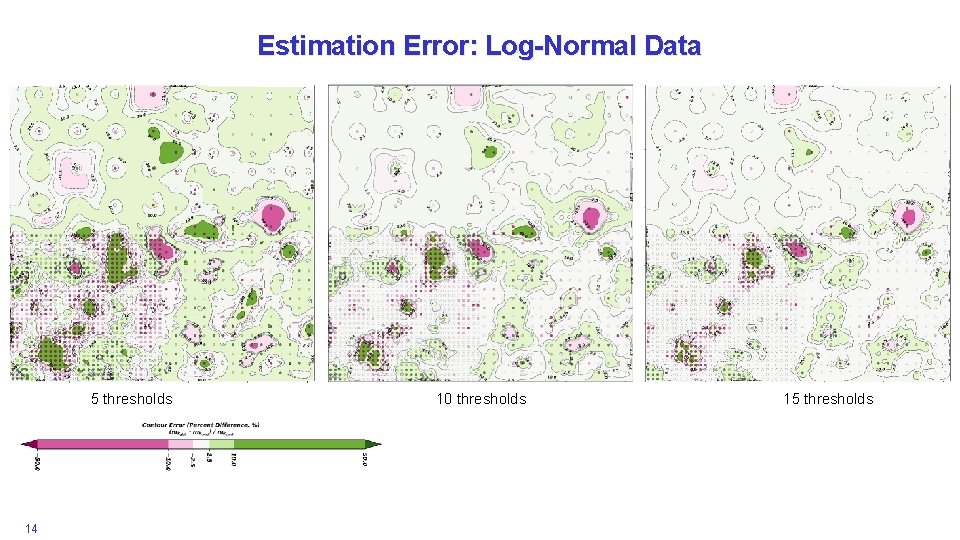
Estimation Error: Log-Normal Data 5 thresholds 14 10 thresholds 15 thresholds
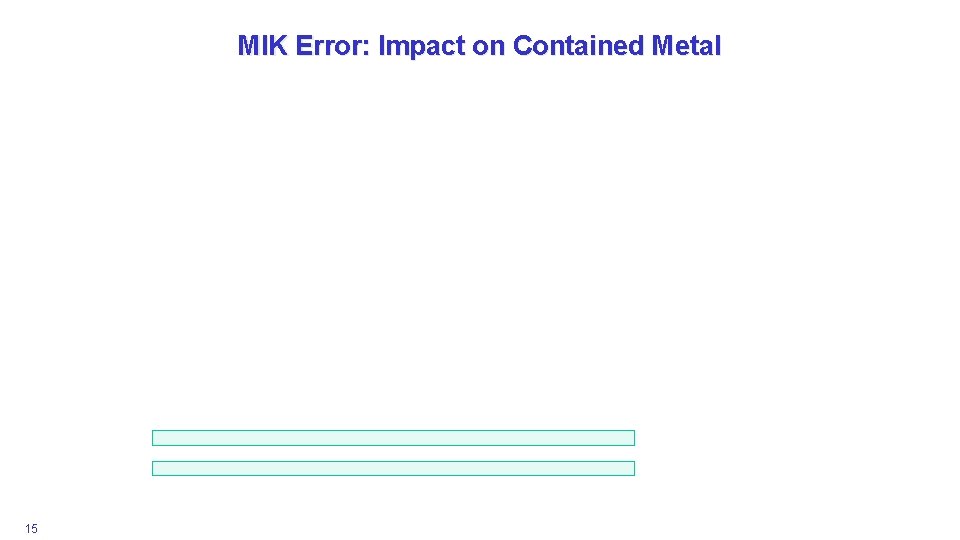
MIK Error: Impact on Contained Metal 15
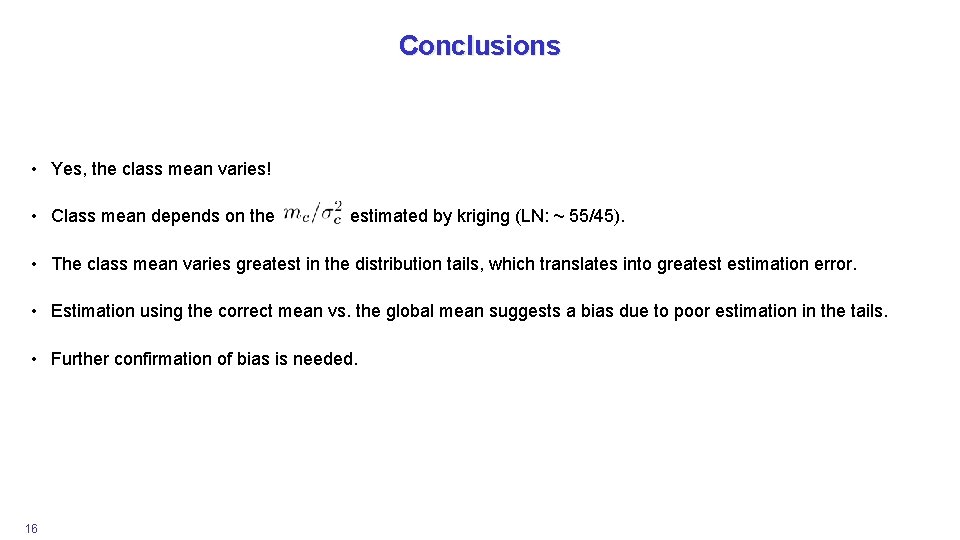
Conclusions • Yes, the class mean varies! • Class mean depends on the estimated by kriging (LN: ~ 55/45). • The class mean varies greatest in the distribution tails, which translates into greatest estimation error. • Estimation using the correct mean vs. the global mean suggests a bias due to poor estimation in the tails. • Further confirmation of bias is needed. 16
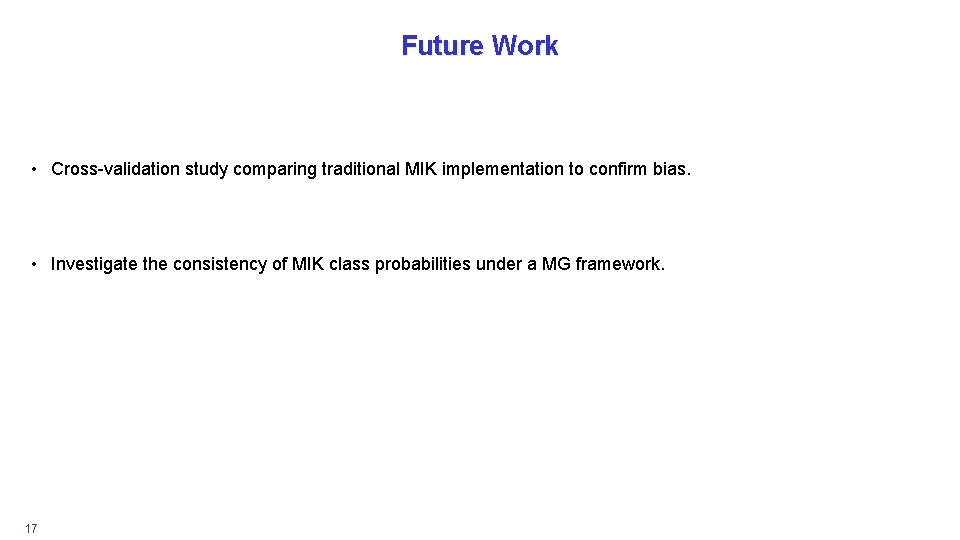
Future Work • Cross-validation study comparing traditional MIK implementation to confirm bias. • Investigate the consistency of MIK class probabilities under a MG framework. 17
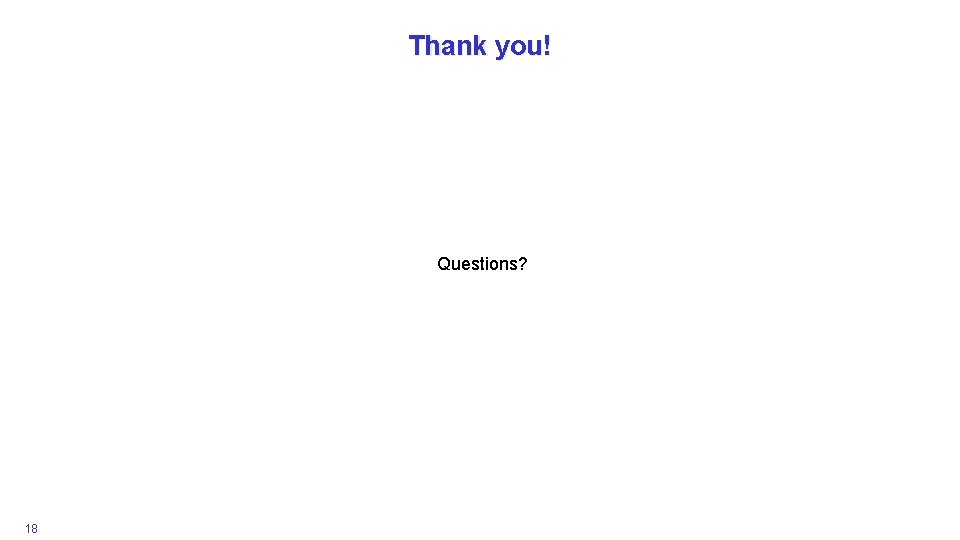
Thank you! Questions? 18
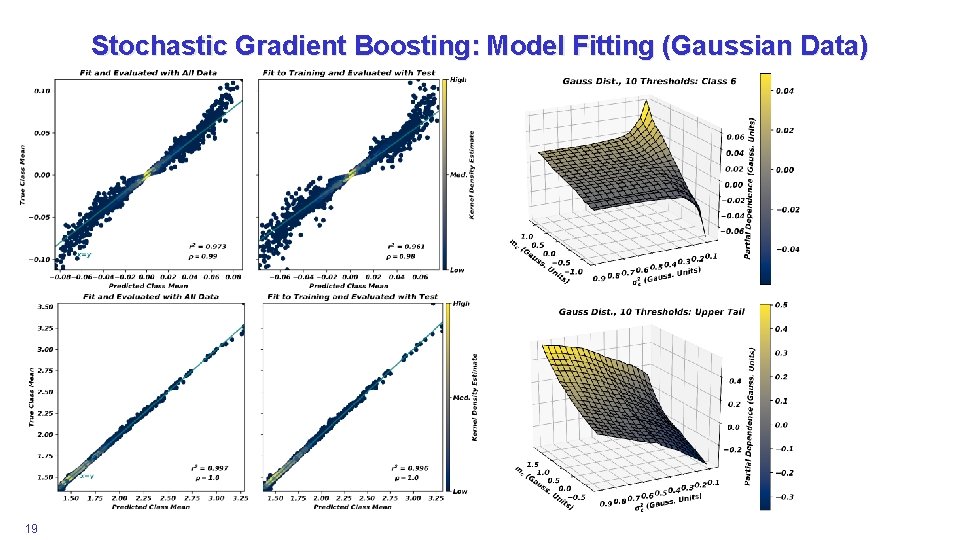
Stochastic Gradient Boosting: Model Fitting (Gaussian Data) 19
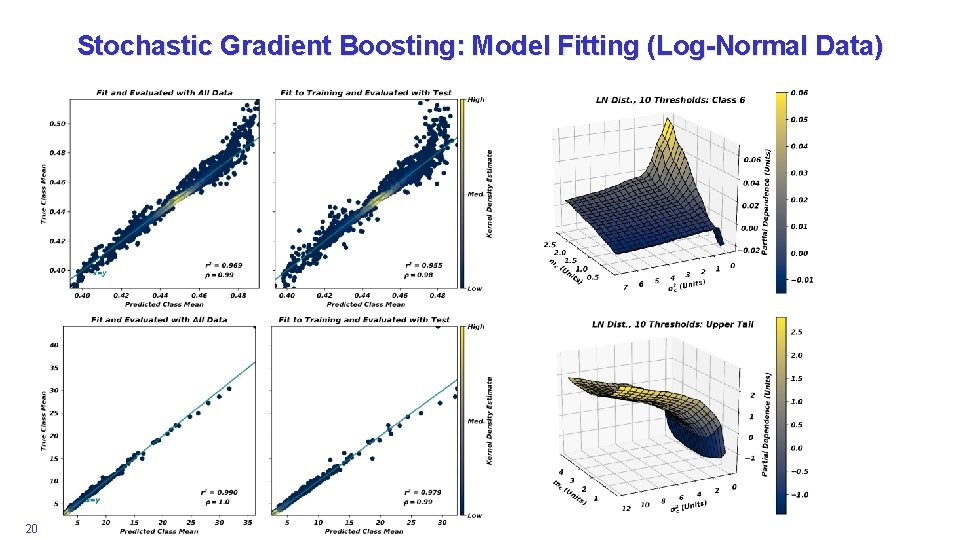
Stochastic Gradient Boosting: Model Fitting (Log-Normal Data) 20
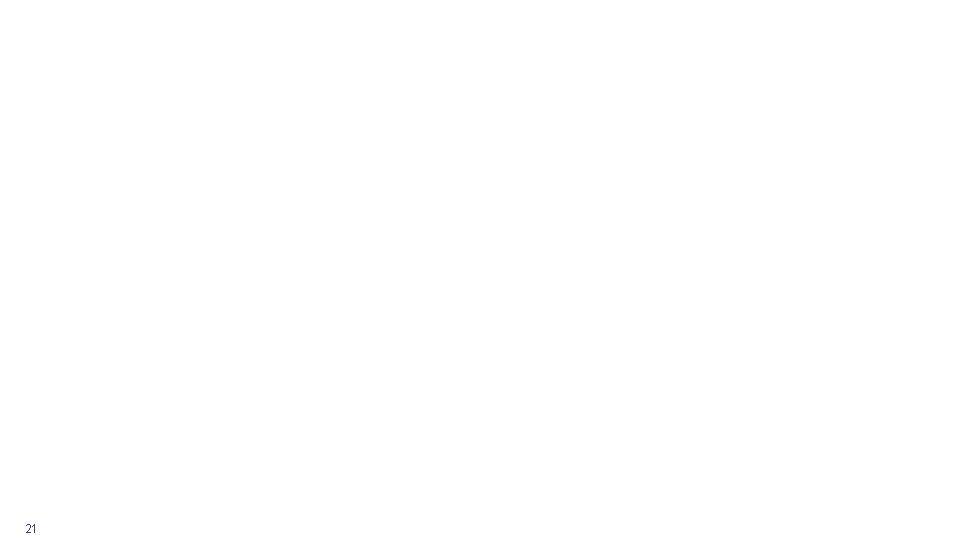
21
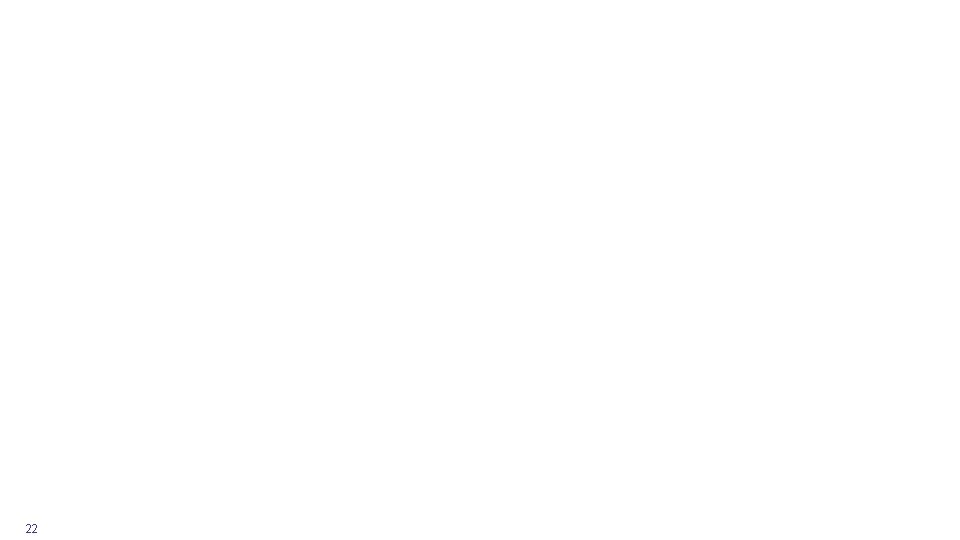
22
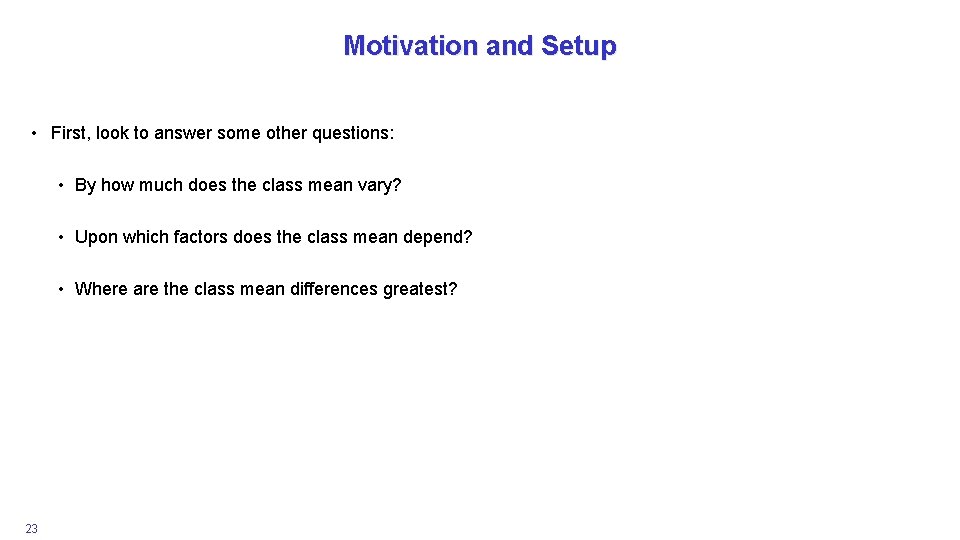
Motivation and Setup • First, look to answer some other questions: • By how much does the class mean vary? • Upon which factors does the class mean depend? • Where are the class mean differences greatest? 23
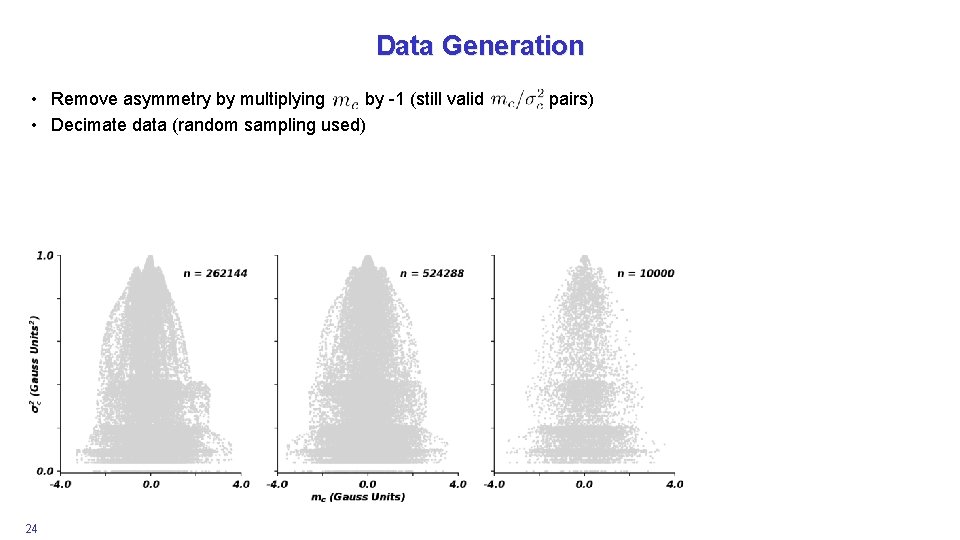
Data Generation • Remove asymmetry by multiplying by -1 (still valid • Decimate data (random sampling used) 24 pairs)
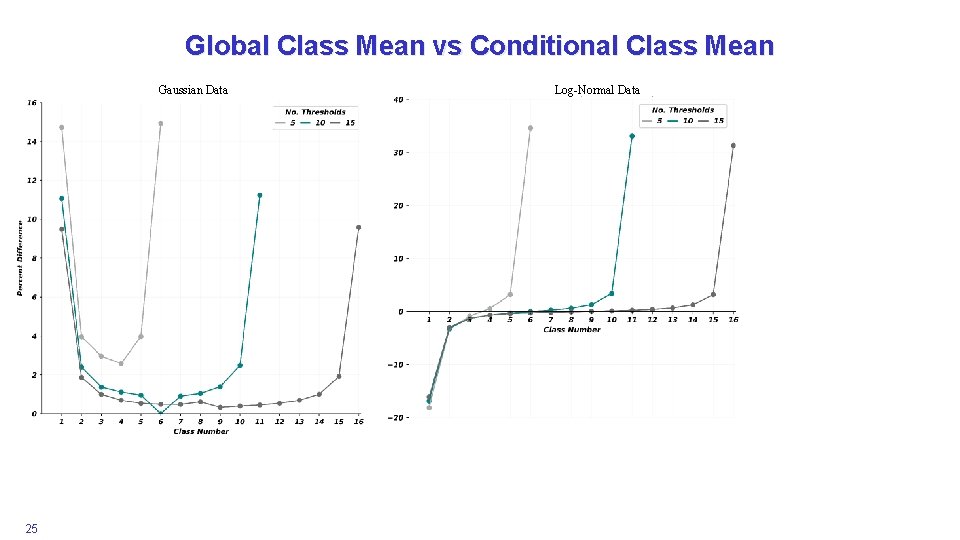
Global Class Mean vs Conditional Class Mean Gaussian Data 25 Log-Normal Data
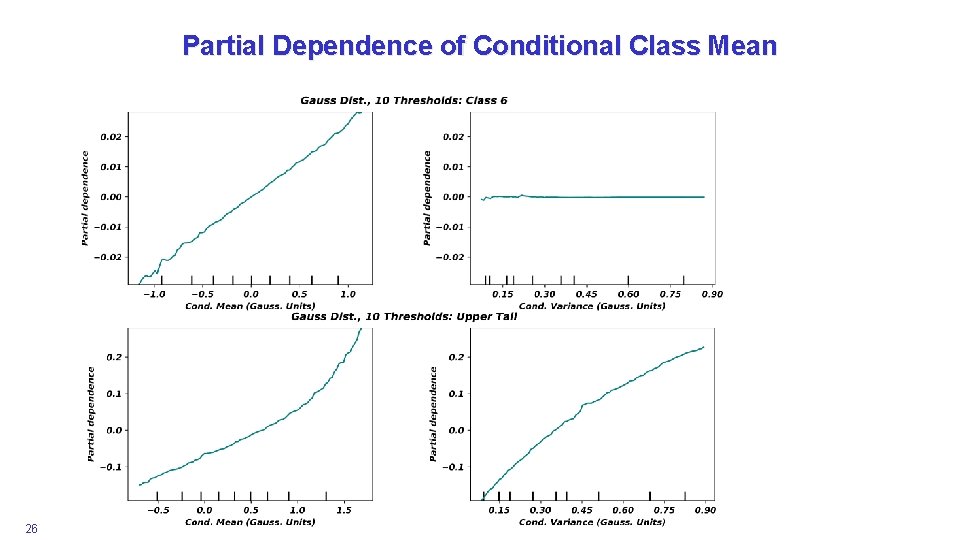
Partial Dependence of Conditional Class Mean 26
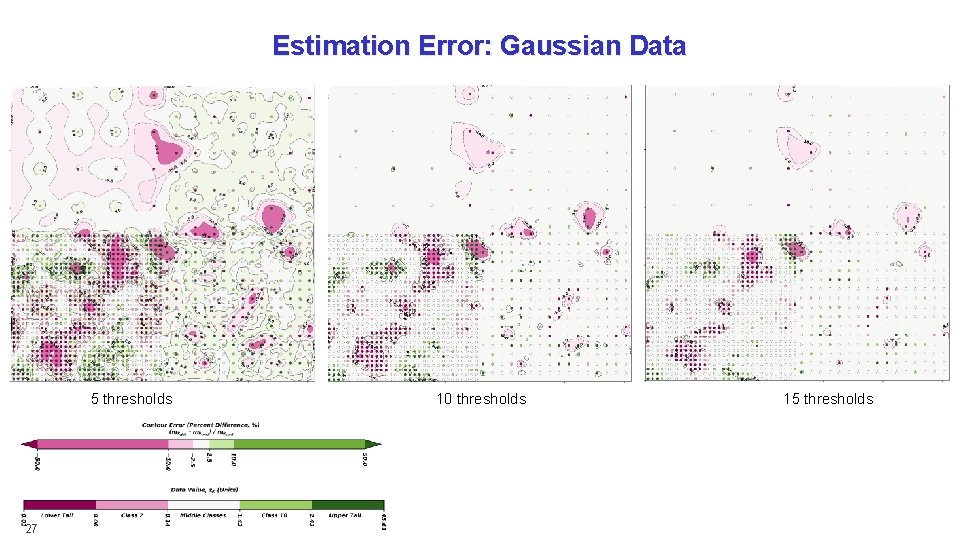
Estimation Error: Gaussian Data 5 thresholds 27 10 thresholds 15 thresholds
Kalamajka mik mik mik tanec
Jeremy jeremy
Pte mik szakdolgozat
Mik1
Aleksandra mik
Varoitusvaatetuksen suojausluokat
Látjátok feleim szümtükhel
Meteorologické prvky
Mik rekam medis
Mik rekam medis
1 mik
Mik azok a felharmonikusok
If poly means many, what is gon?
Meta means in metamorphism
Meta means change and morph means heat
Biodiversity and conservation
Bio means life
Valuation of variations
Kinds of variation
Inverse variation
Kwl plus chart
Cultural variations in attachment
Computer organization & architecture: themes and variations
Need for biasing
Super bowl squares variations
Example of joint variation
Variations pathologiques de la température
Variations in psychology attributes