Towards TaskOriented Computing for Pervasive Computing Environments Presenter
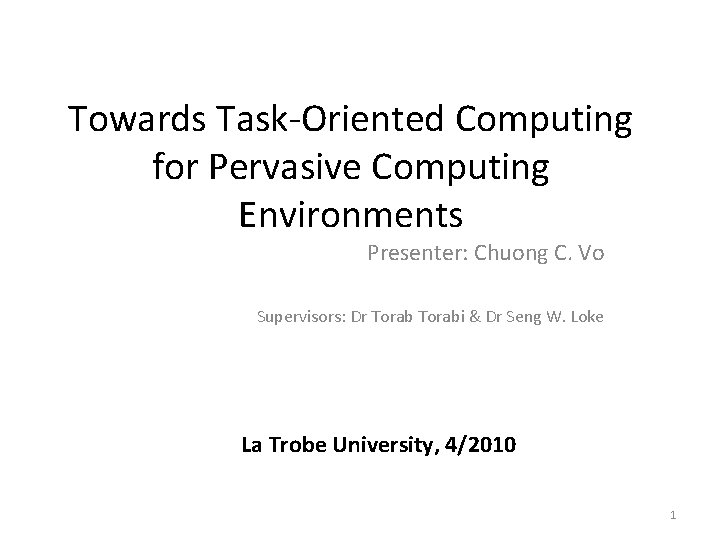
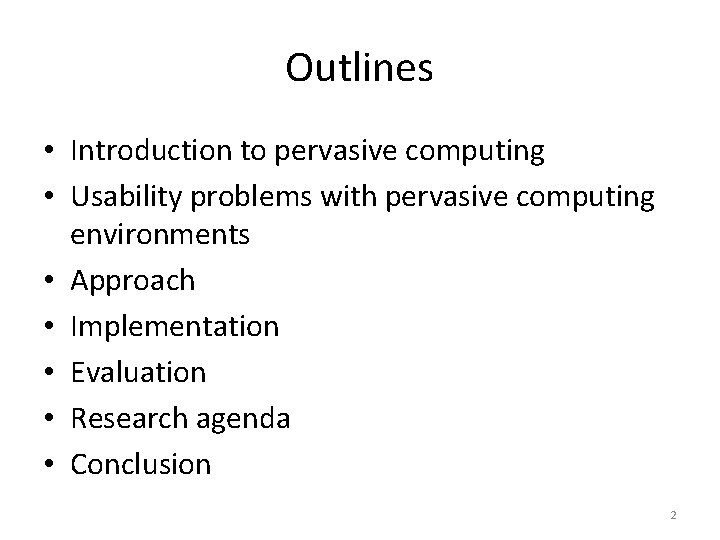
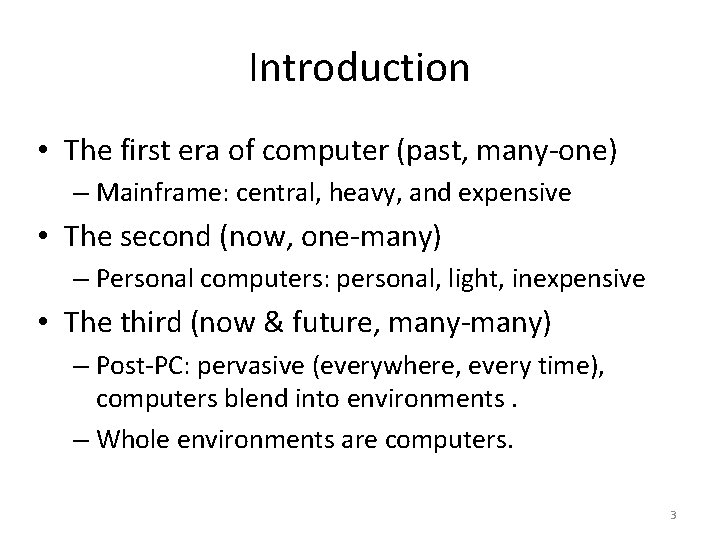
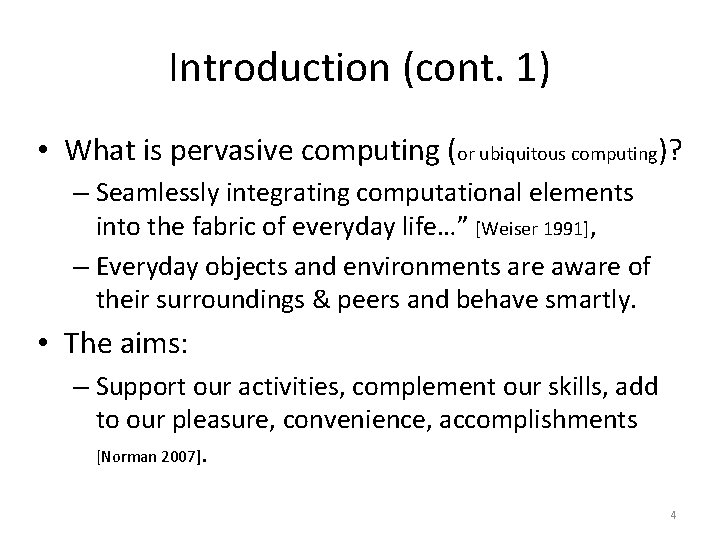
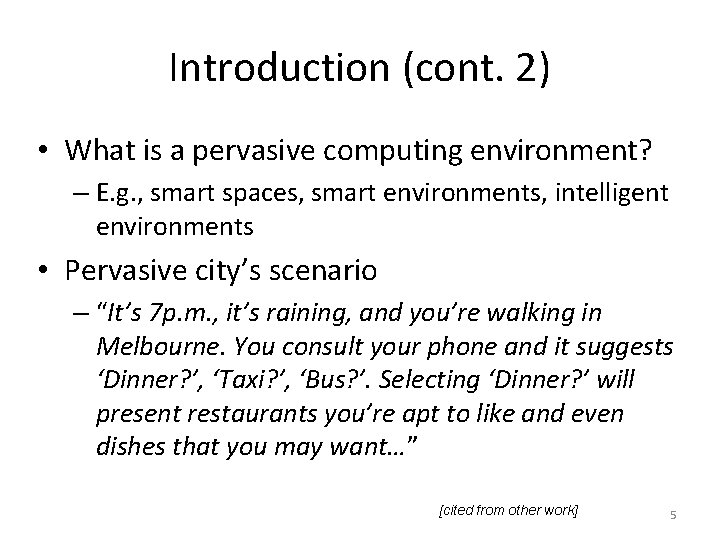
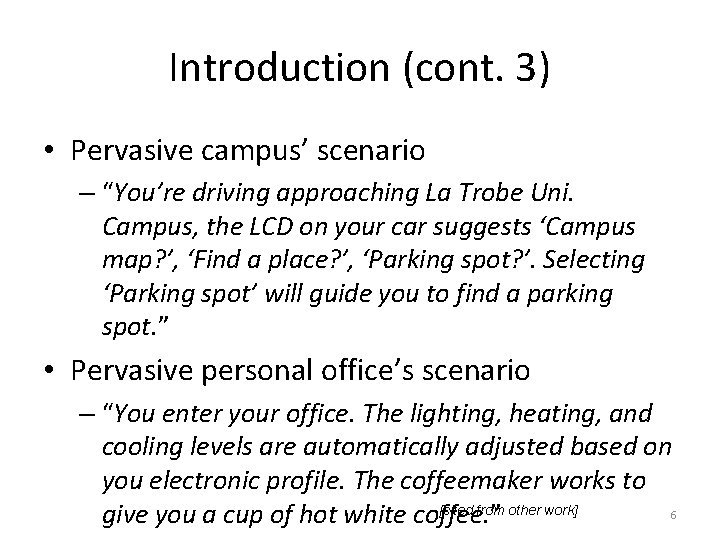
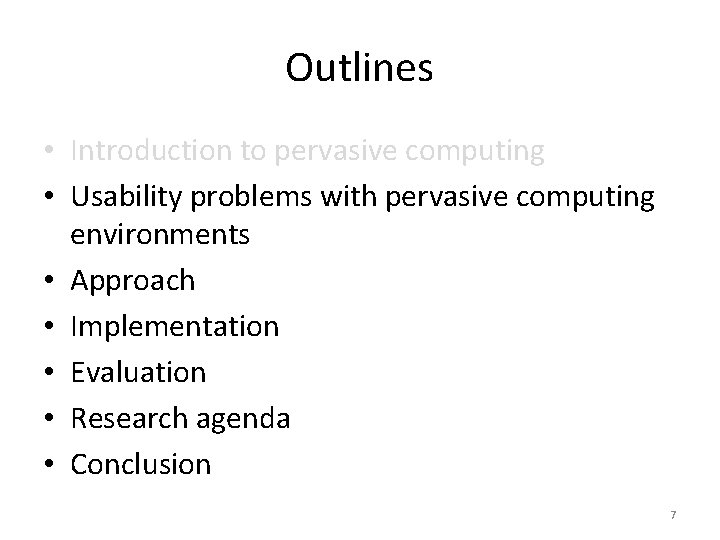
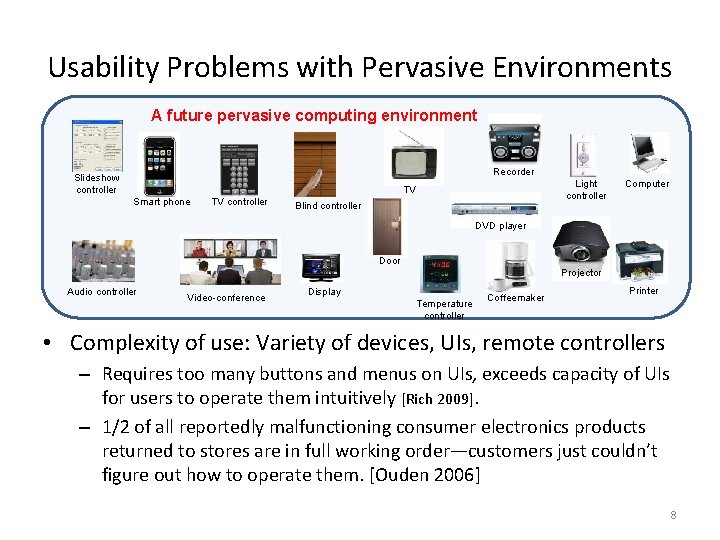
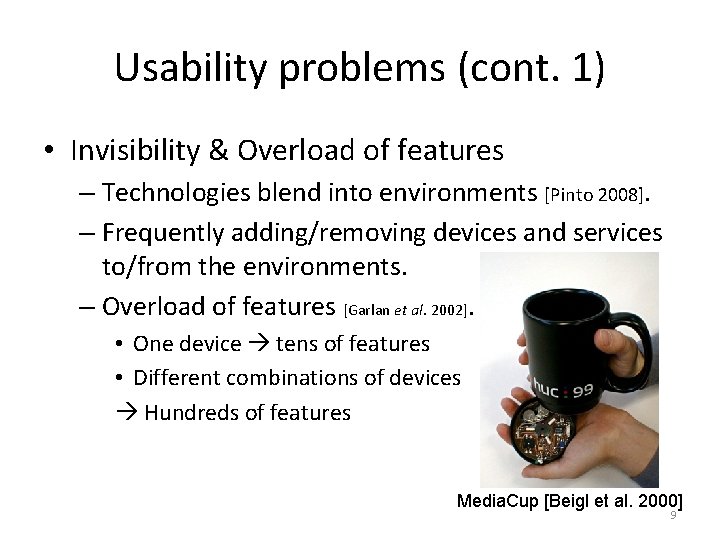
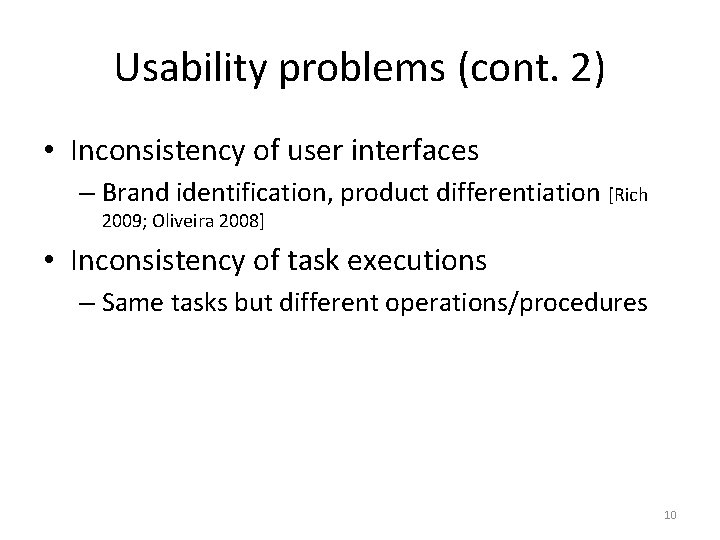
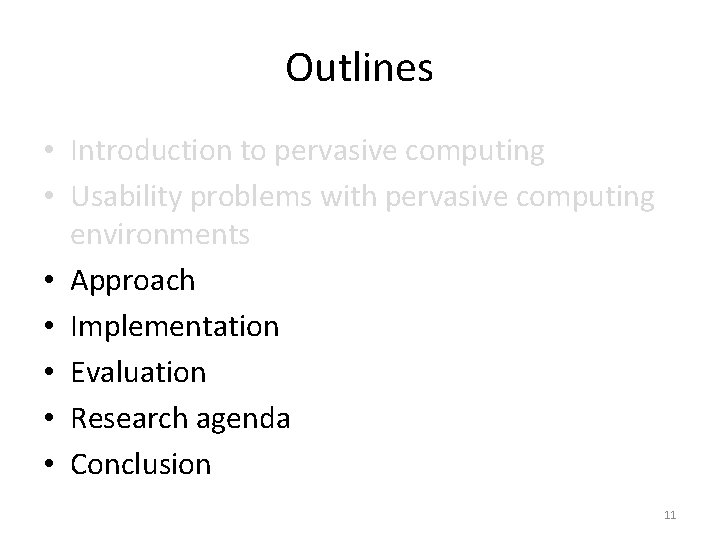
![Research hypothesis • Our approach is based on task-driven computing [Wang et al. 2000]: Research hypothesis • Our approach is based on task-driven computing [Wang et al. 2000]:](https://slidetodoc.com/presentation_image_h2/4336a2be504e1dd9670431e076641350/image-12.jpg)
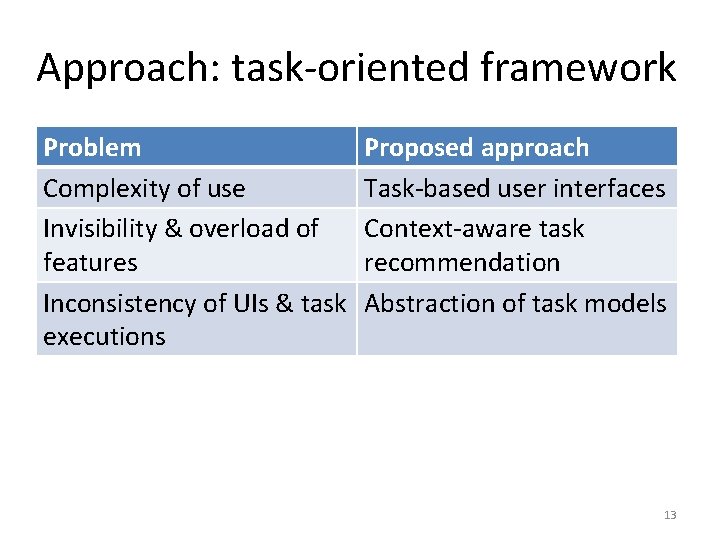
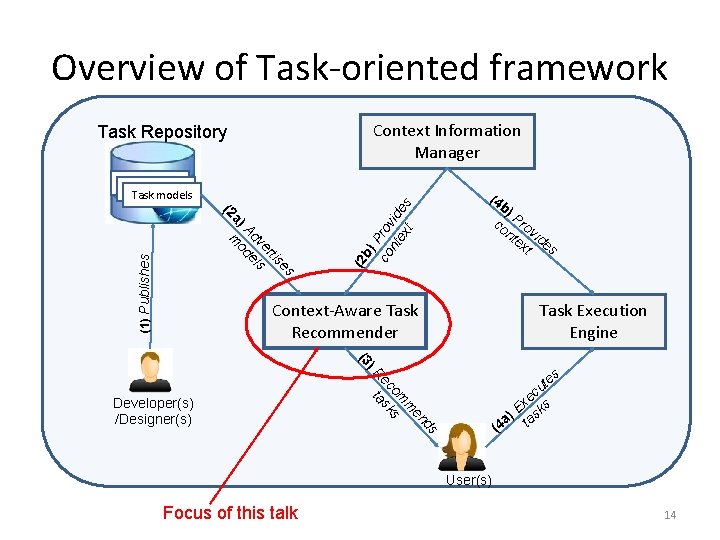
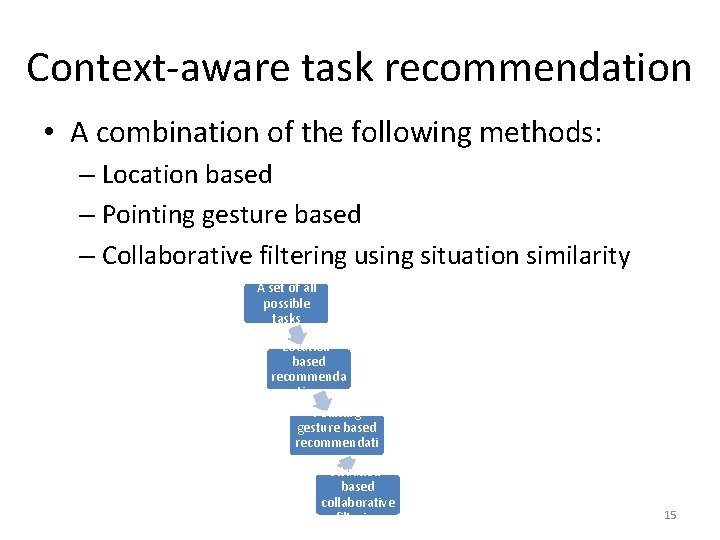
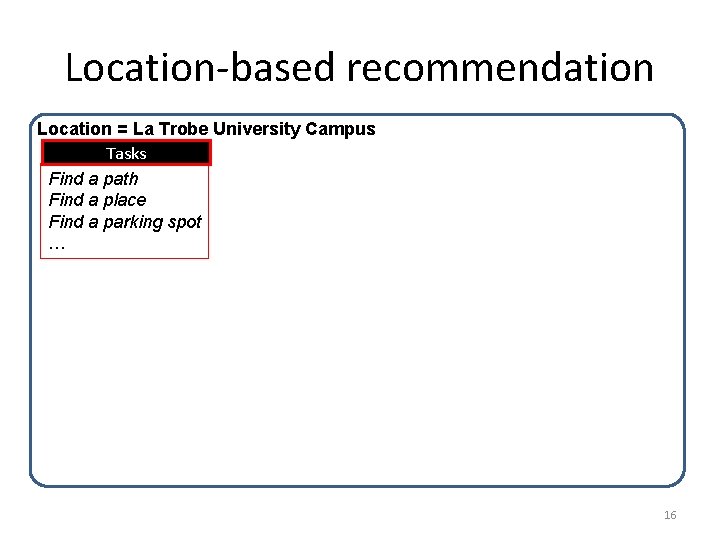
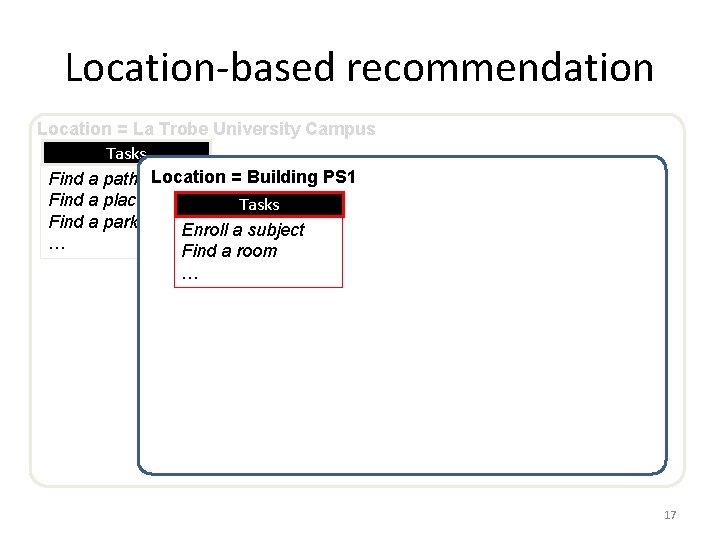
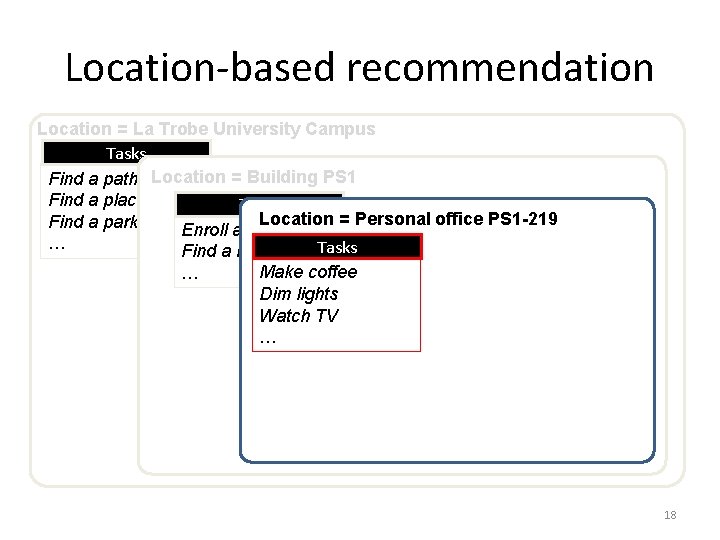
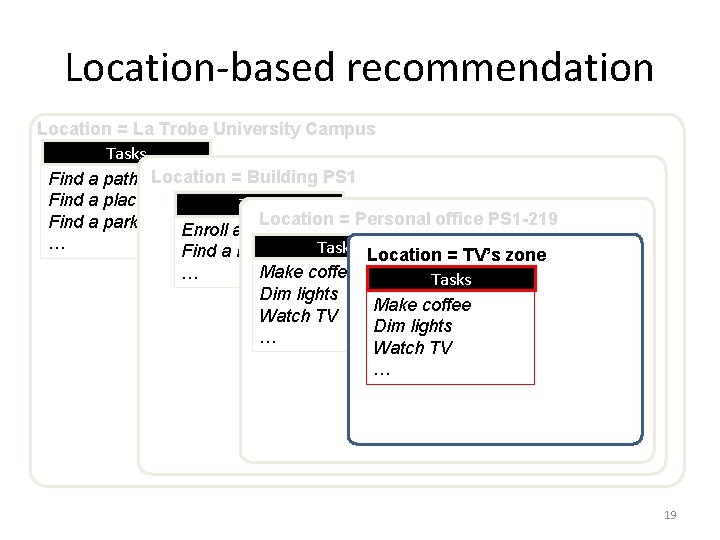
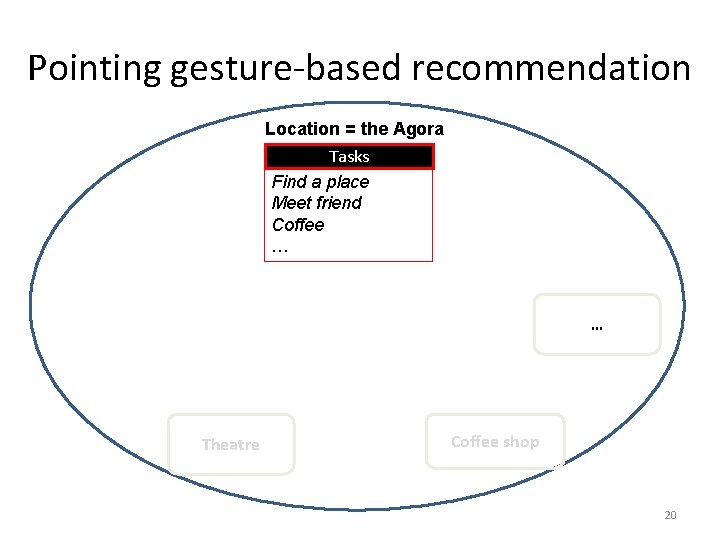
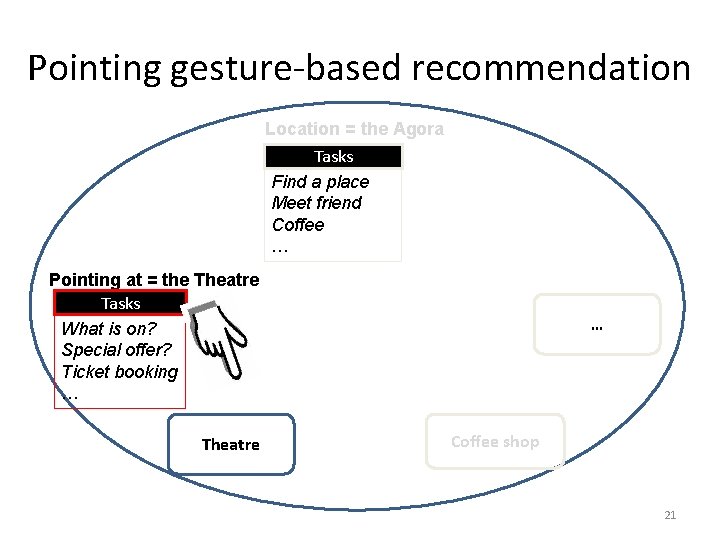
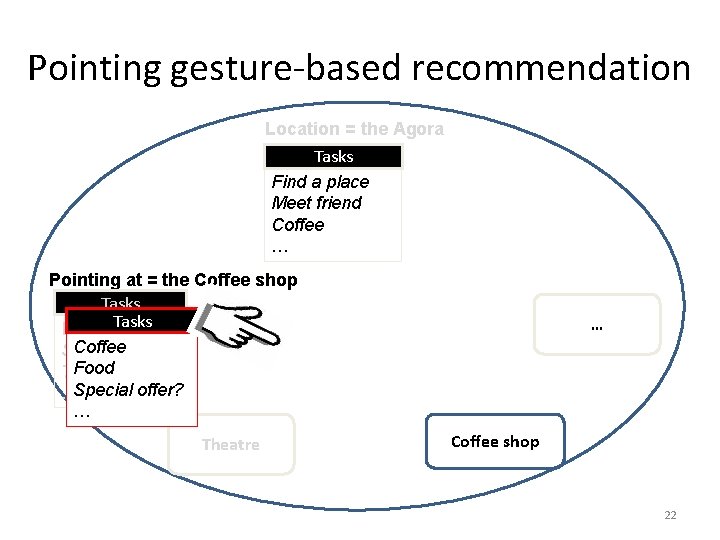
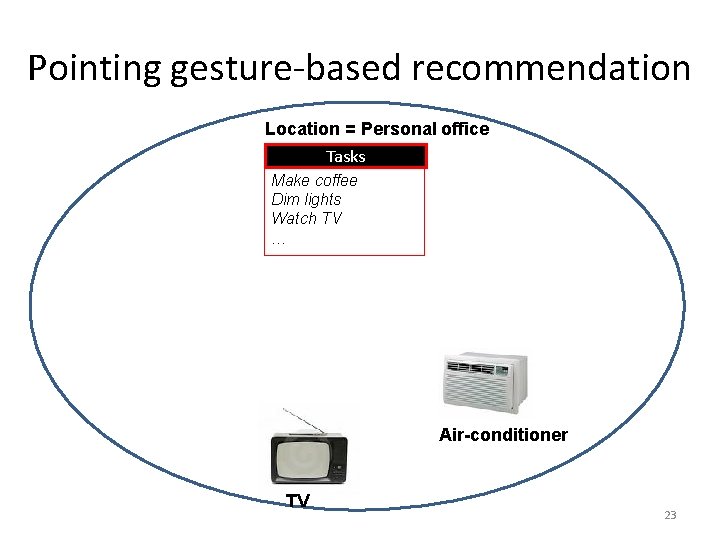
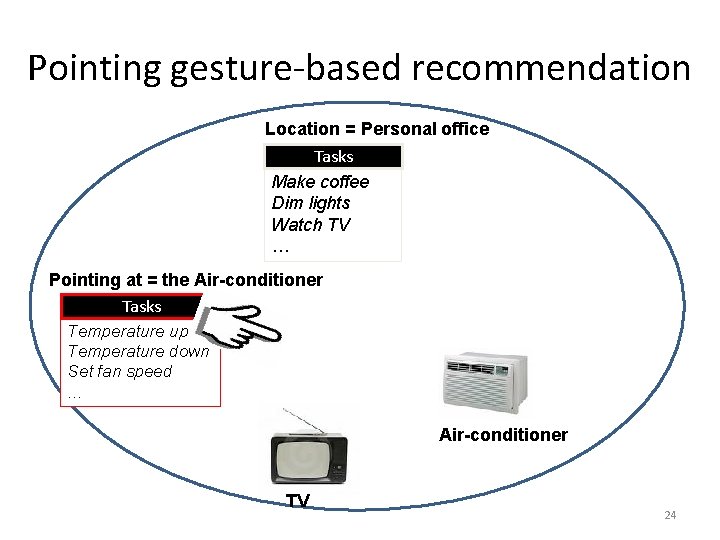
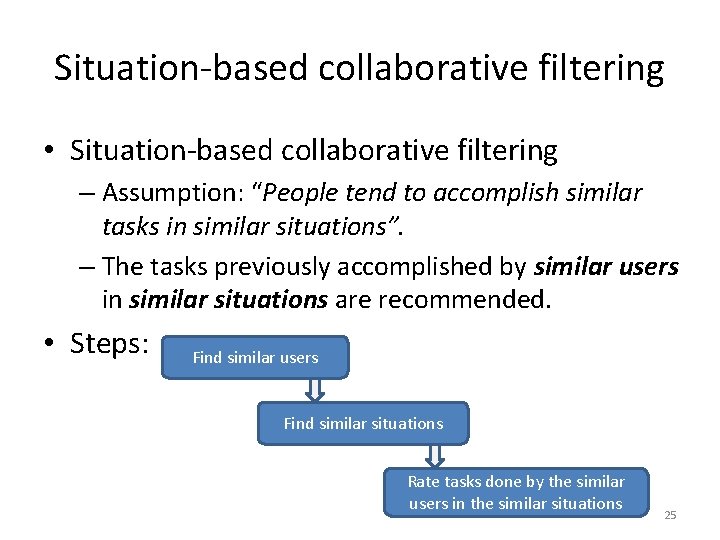
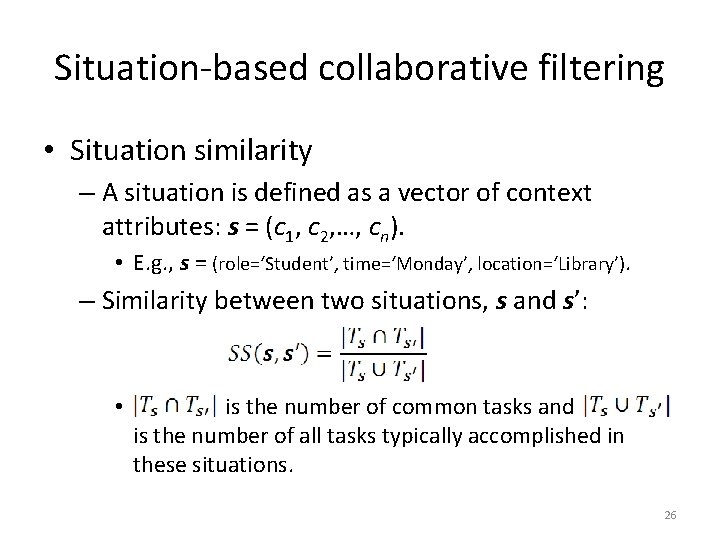
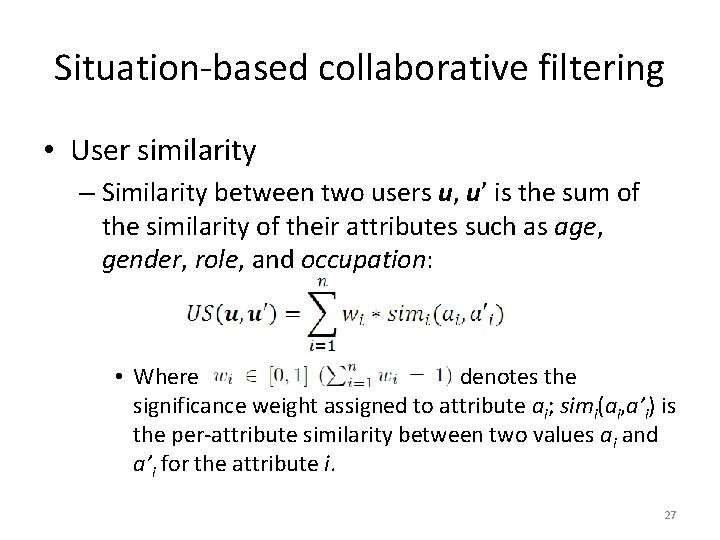
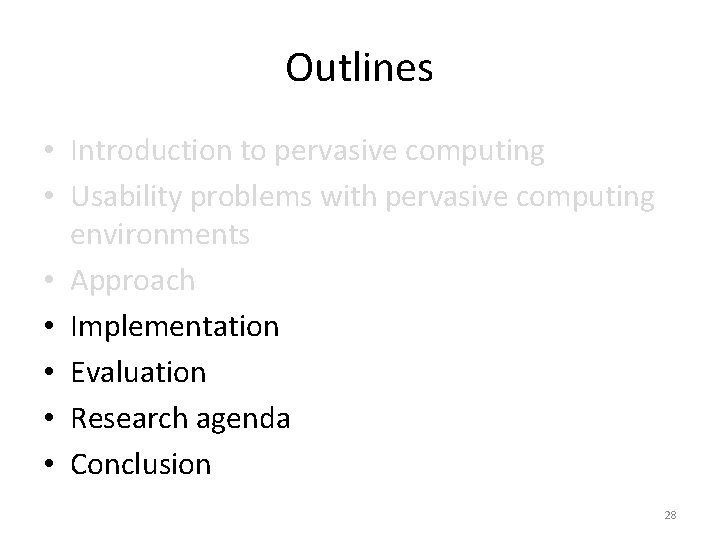
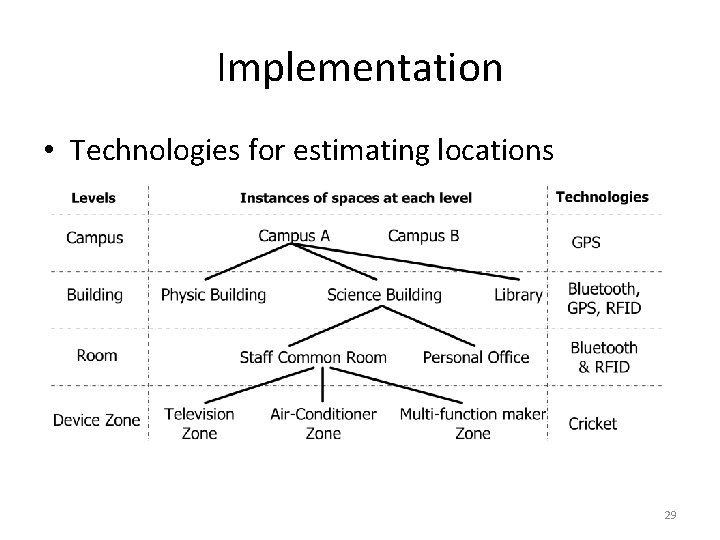
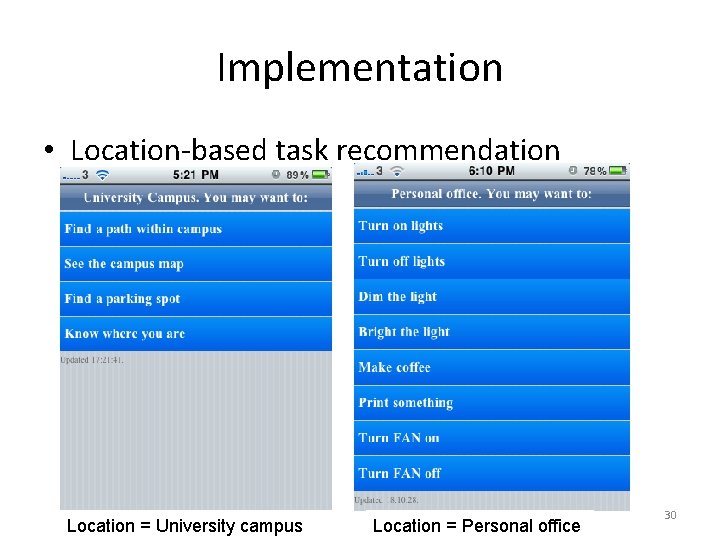
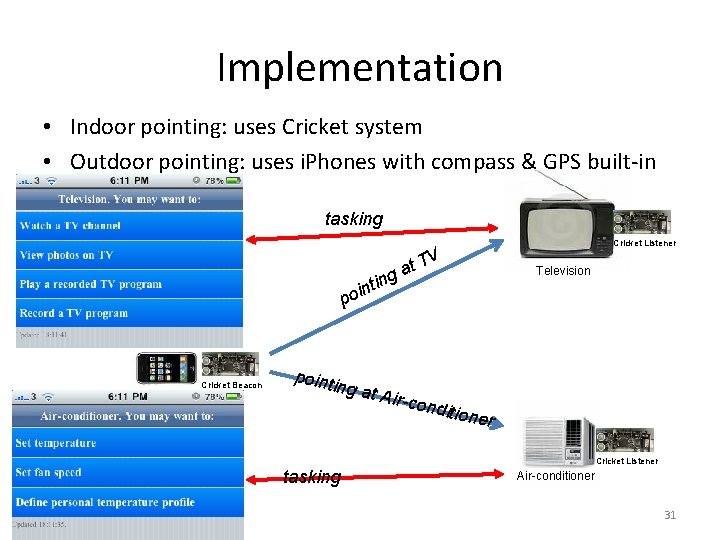
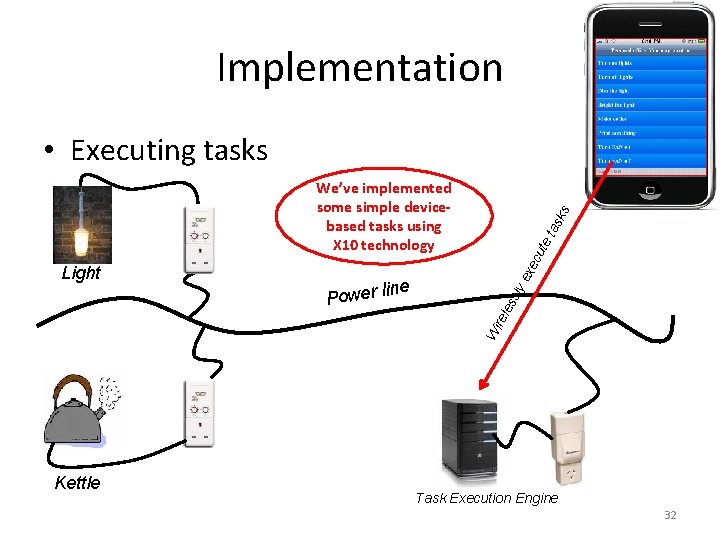
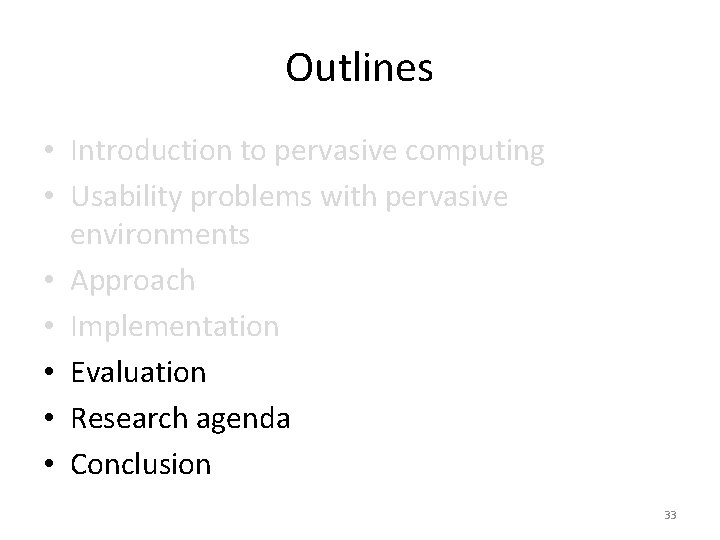
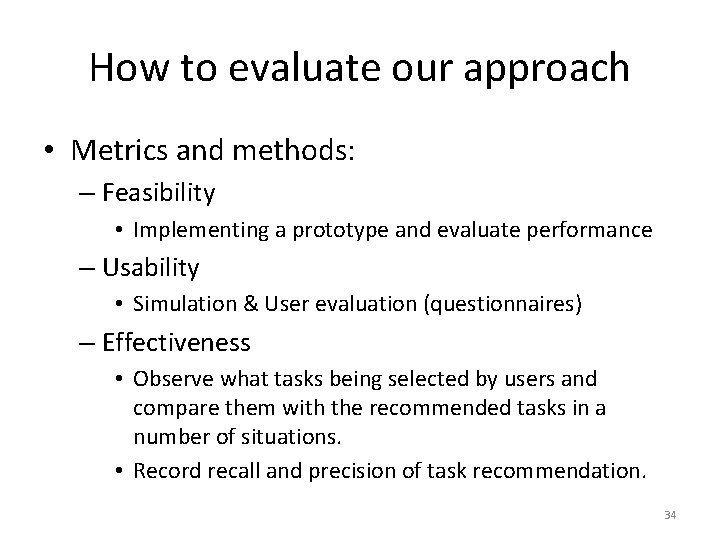
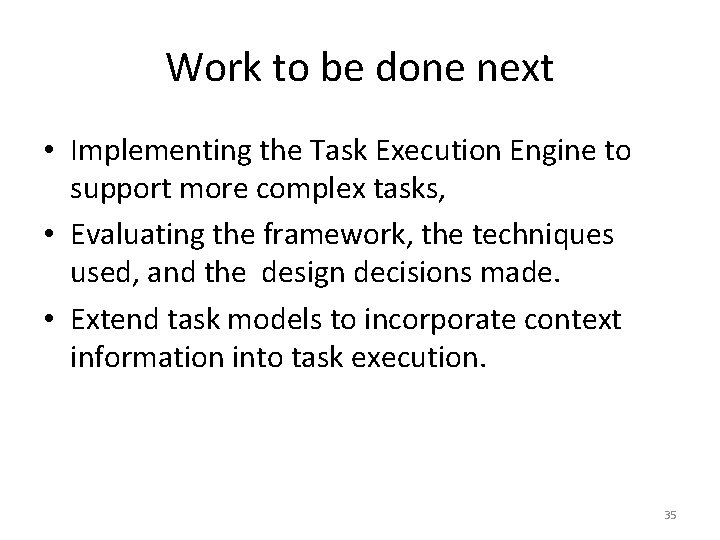
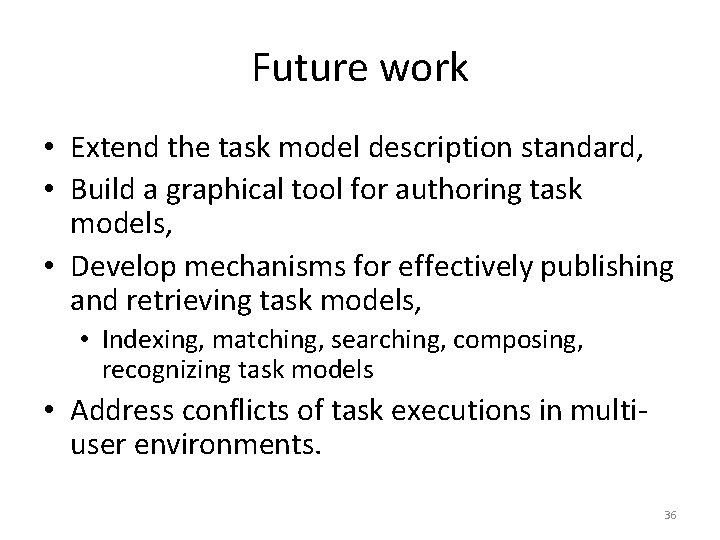
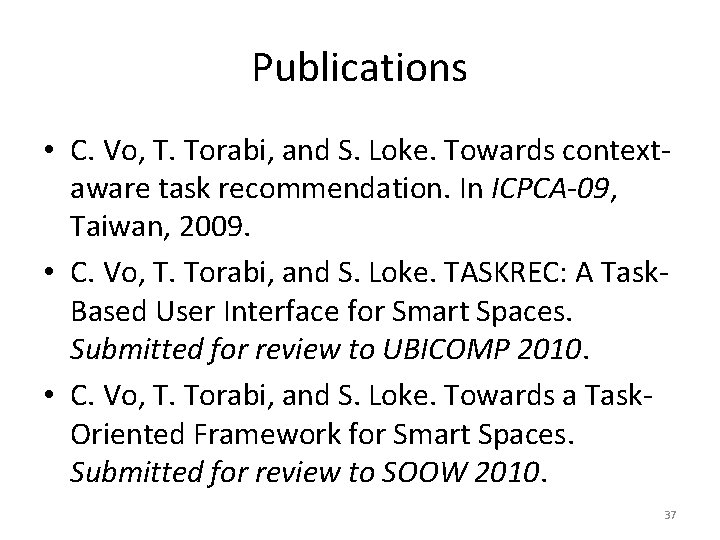
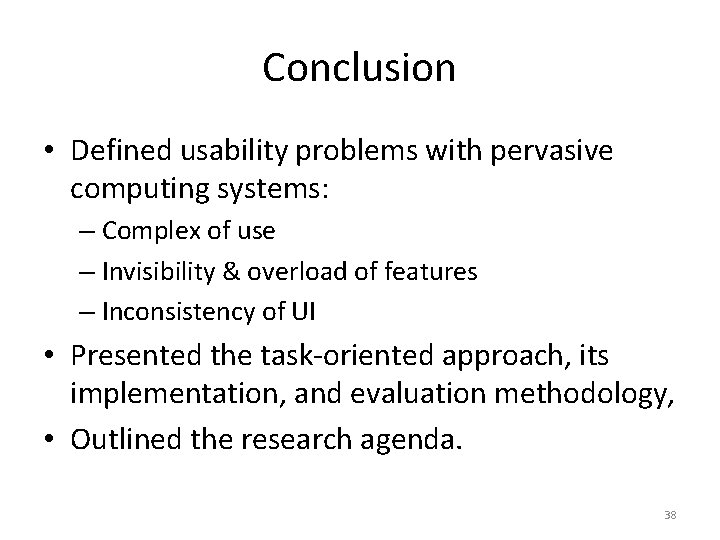
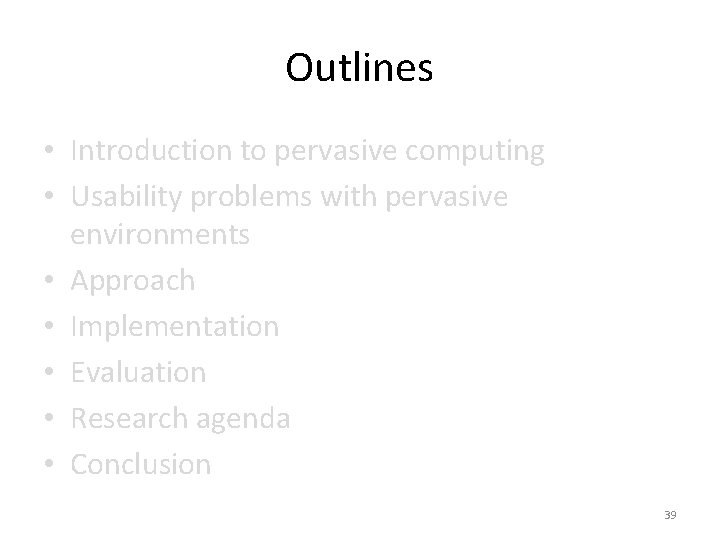
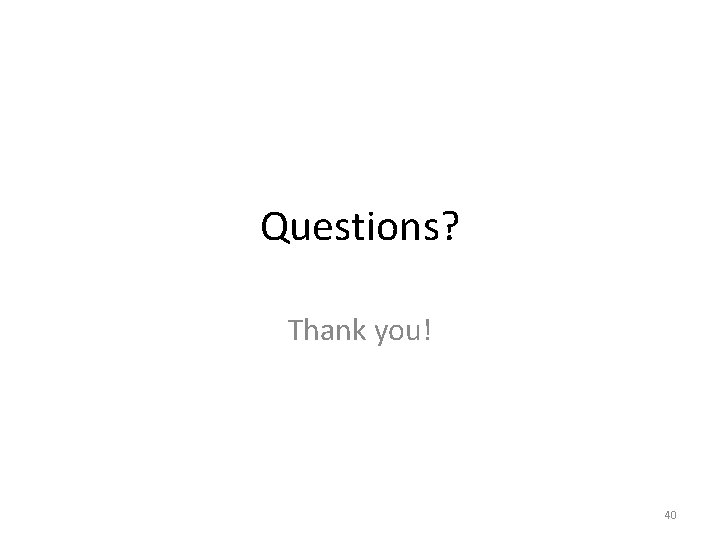
![Related work • Situation-aware application recommendation [Cheng et al. 2008] – They recommend applications Related work • Situation-aware application recommendation [Cheng et al. 2008] – They recommend applications](https://slidetodoc.com/presentation_image_h2/4336a2be504e1dd9670431e076641350/image-41.jpg)
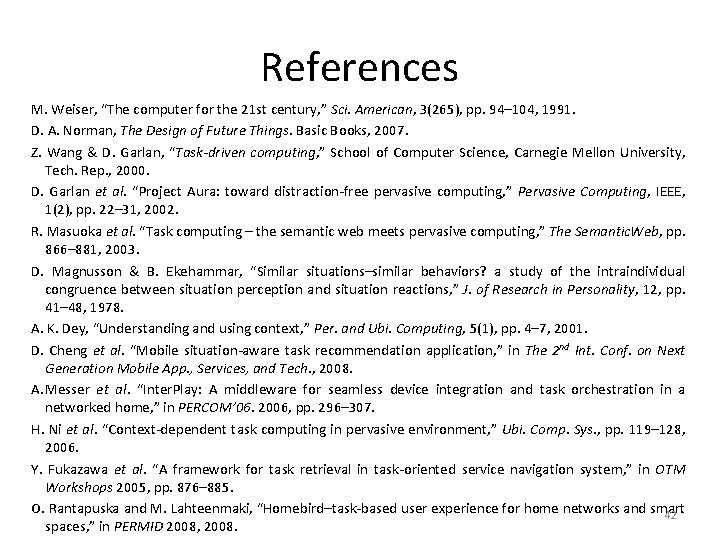
- Slides: 42
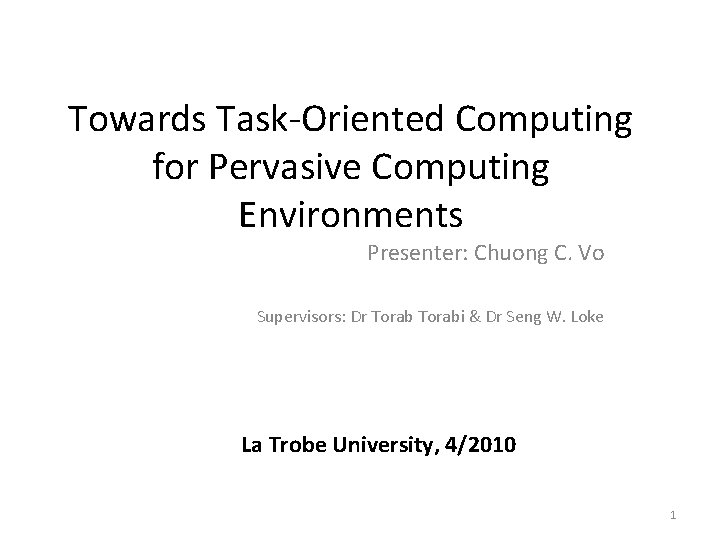
Towards Task-Oriented Computing for Pervasive Computing Environments Presenter: Chuong C. Vo Supervisors: Dr Torabi & Dr Seng W. Loke La Trobe University, 4/2010 1
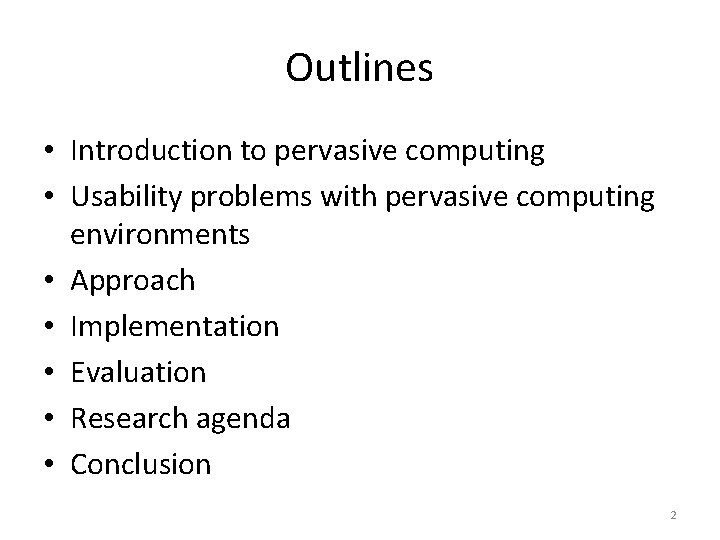
Outlines • Introduction to pervasive computing • Usability problems with pervasive computing environments • Approach • Implementation • Evaluation • Research agenda • Conclusion 2
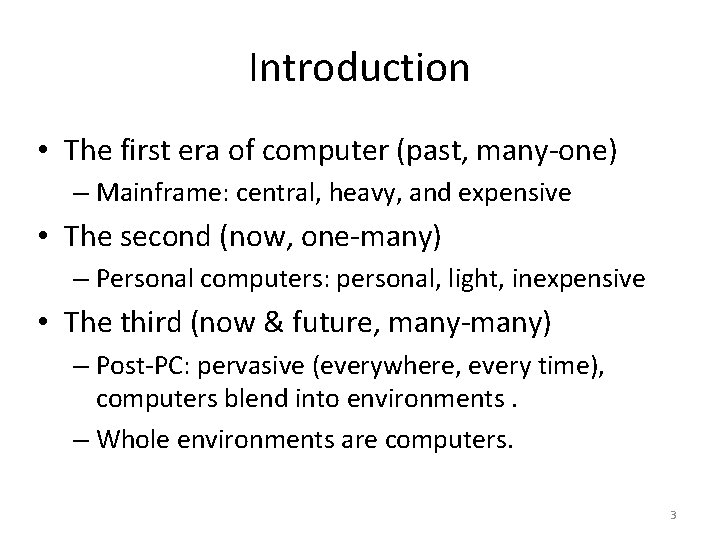
Introduction • The first era of computer (past, many-one) – Mainframe: central, heavy, and expensive • The second (now, one-many) – Personal computers: personal, light, inexpensive • The third (now & future, many-many) – Post-PC: pervasive (everywhere, every time), computers blend into environments. – Whole environments are computers. 3
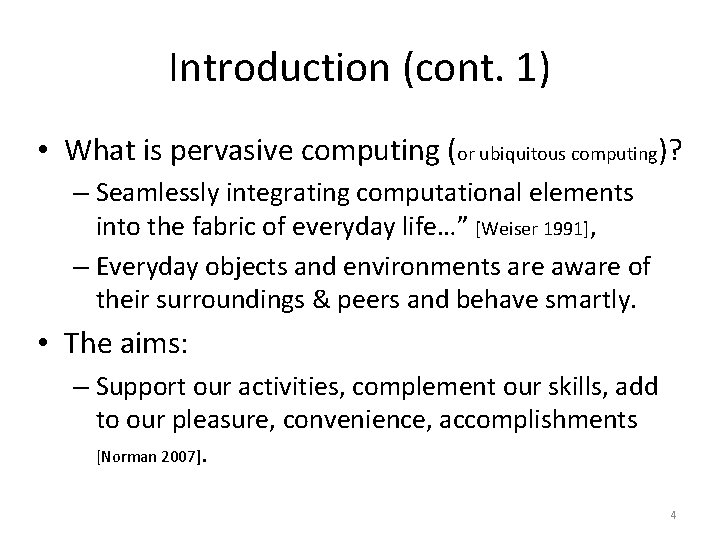
Introduction (cont. 1) • What is pervasive computing (or ubiquitous computing)? – Seamlessly integrating computational elements into the fabric of everyday life…” [Weiser 1991], – Everyday objects and environments are aware of their surroundings & peers and behave smartly. • The aims: – Support our activities, complement our skills, add to our pleasure, convenience, accomplishments [Norman 2007]. 4
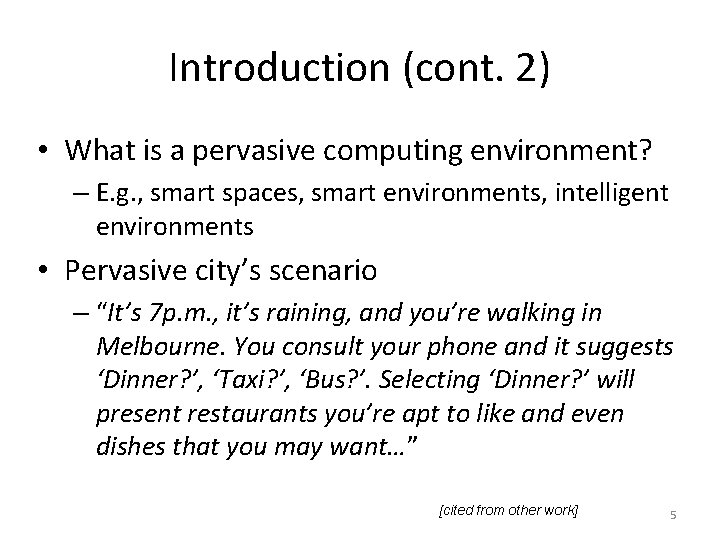
Introduction (cont. 2) • What is a pervasive computing environment? – E. g. , smart spaces, smart environments, intelligent environments • Pervasive city’s scenario – “It’s 7 p. m. , it’s raining, and you’re walking in Melbourne. You consult your phone and it suggests ‘Dinner? ’, ‘Taxi? ’, ‘Bus? ’. Selecting ‘Dinner? ’ will present restaurants you’re apt to like and even dishes that you may want…” [cited from other work] 5
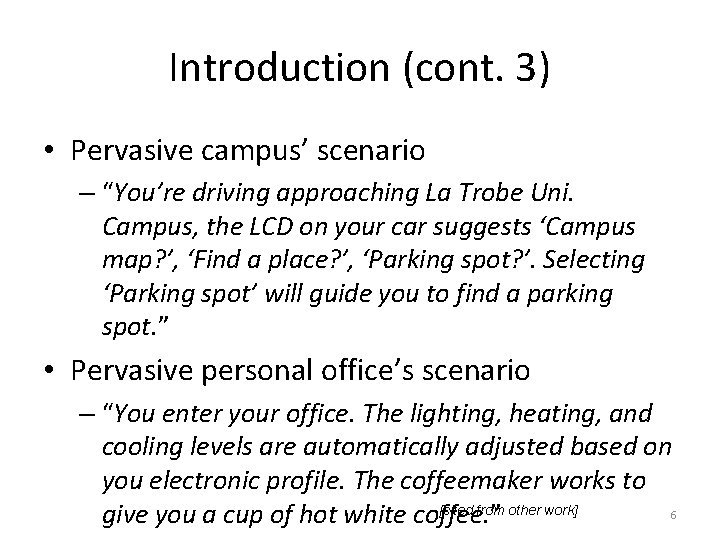
Introduction (cont. 3) • Pervasive campus’ scenario – “You’re driving approaching La Trobe Uni. Campus, the LCD on your car suggests ‘Campus map? ’, ‘Find a place? ’, ‘Parking spot? ’. Selecting ‘Parking spot’ will guide you to find a parking spot. ” • Pervasive personal office’s scenario – “You enter your office. The lighting, heating, and cooling levels are automatically adjusted based on you electronic profile. The coffeemaker works to [cited from other work] 6 give you a cup of hot white coffee. ”
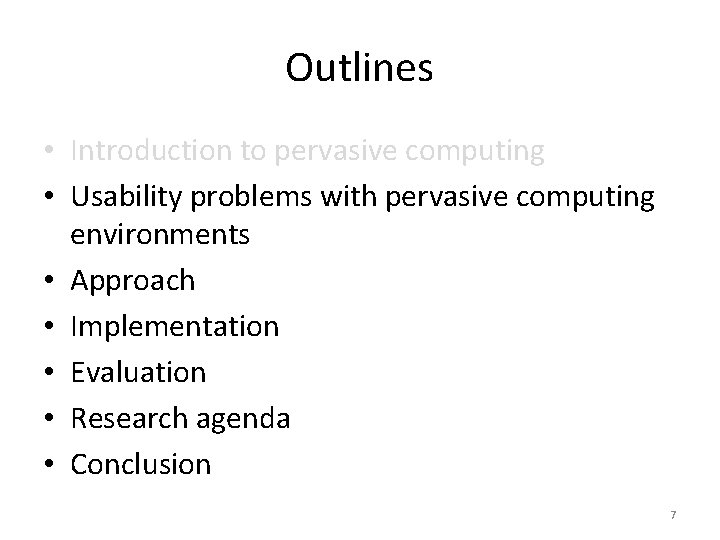
Outlines • Introduction to pervasive computing • Usability problems with pervasive computing environments • Approach • Implementation • Evaluation • Research agenda • Conclusion 7
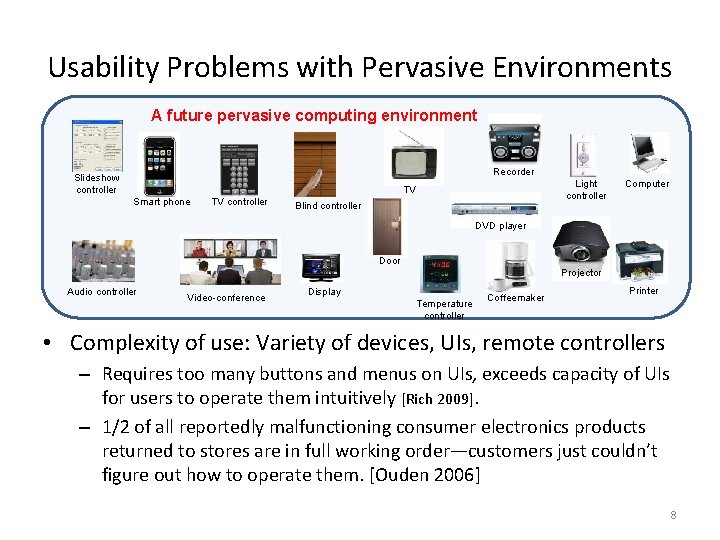
Usability Problems with Pervasive Environments A future pervasive computing environment Recorder Slideshow controller Light controller TV Smart phone TV controller Blind controller Computer DVD player Door Projector Audio controller Video-conference Display Temperature controller Coffeemaker Printer • Complexity of use: Variety of devices, UIs, remote controllers – Requires too many buttons and menus on UIs, exceeds capacity of UIs for users to operate them intuitively [Rich 2009]. – 1/2 of all reportedly malfunctioning consumer electronics products returned to stores are in full working order—customers just couldn’t figure out how to operate them. [Ouden 2006] 8
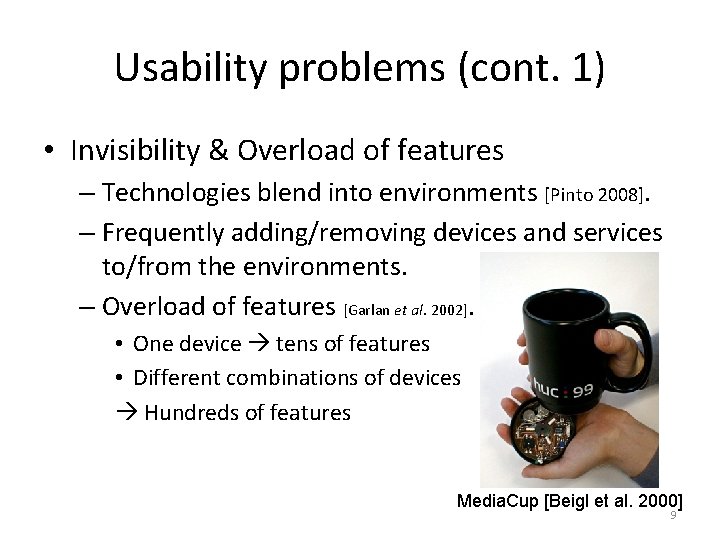
Usability problems (cont. 1) • Invisibility & Overload of features – Technologies blend into environments [Pinto 2008]. – Frequently adding/removing devices and services to/from the environments. – Overload of features [Garlan et al. 2002]. • One device tens of features • Different combinations of devices Hundreds of features Media. Cup [Beigl et al. 2000] 9
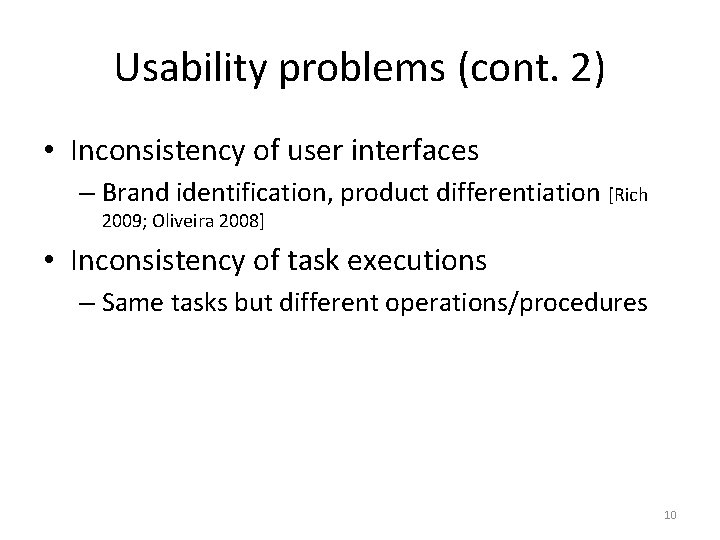
Usability problems (cont. 2) • Inconsistency of user interfaces – Brand identification, product differentiation [Rich 2009; Oliveira 2008] • Inconsistency of task executions – Same tasks but different operations/procedures 10
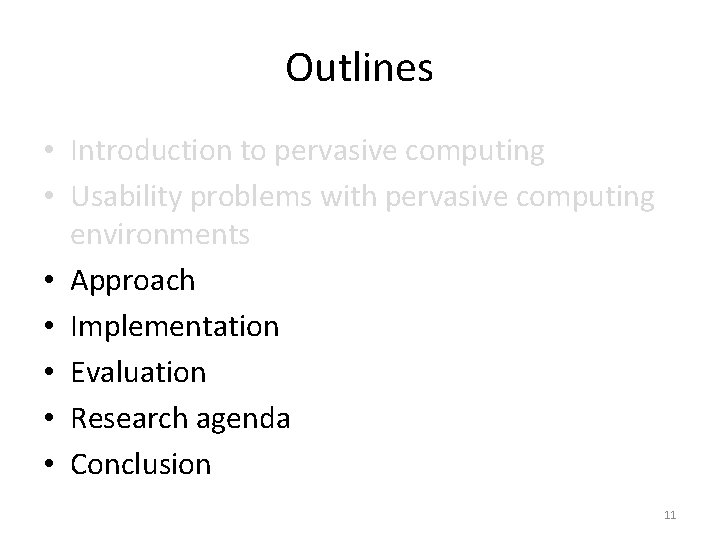
Outlines • Introduction to pervasive computing • Usability problems with pervasive computing environments • Approach • Implementation • Evaluation • Research agenda • Conclusion 11
![Research hypothesis Our approach is based on taskdriven computing Wang et al 2000 Research hypothesis • Our approach is based on task-driven computing [Wang et al. 2000]:](https://slidetodoc.com/presentation_image_h2/4336a2be504e1dd9670431e076641350/image-12.jpg)
Research hypothesis • Our approach is based on task-driven computing [Wang et al. 2000]: – A task is a user’s goal or objective [Loke 2009]. – Users interact with/think of the computing in terms of tasks instead of applications/devices. – Users focus on the tasks at hand rather than on the means for achieving those tasks [Masuoka 2003]. – Application function is modeled as tasks and subtasks. • Our research investigates the FEASIBILITY, USABILITY, and EFFECTIVENESS of the task-oriented approach to the mentioned problems. 12
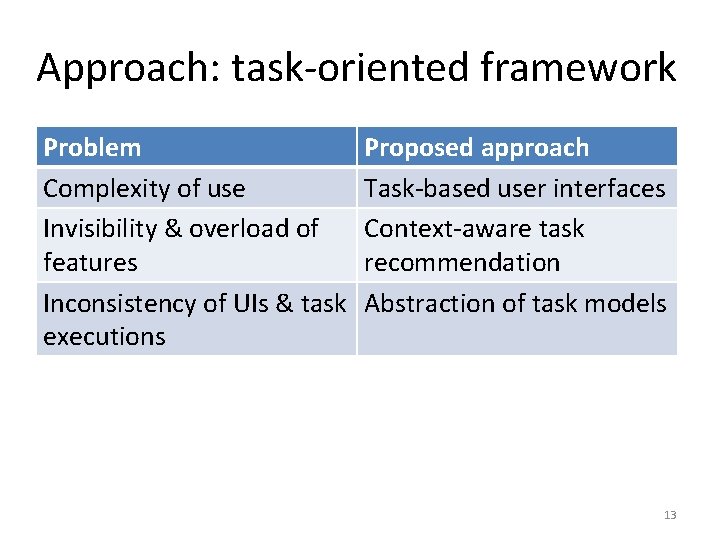
Approach: task-oriented framework Problem Complexity of use Invisibility & overload of features Inconsistency of UIs & task executions Proposed approach Task-based user interfaces Context-aware task recommendation Abstraction of task models 13
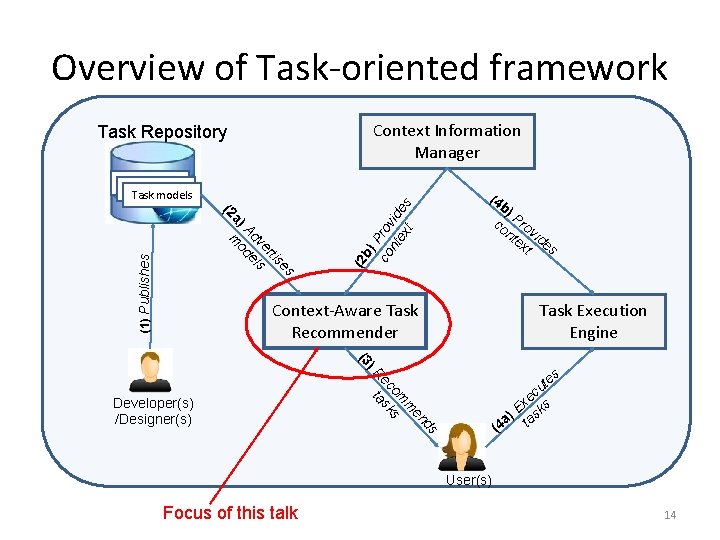
Overview of Task-oriented framework Context Information Manager Task Repository Taskmodel Task models b) P co rov nt id ex es t b) co Pro nt vid ex e t s (2 s se rti ve ls Ad de a) mo (2 (1) Publishes (4 Context-Aware Task Recommender Task Execution Engine )R (3 ds en m om s ec ask t Developer(s) /Designer(s) s te u ec x E sks ) a ta (4 User(s) Focus of this talk 14
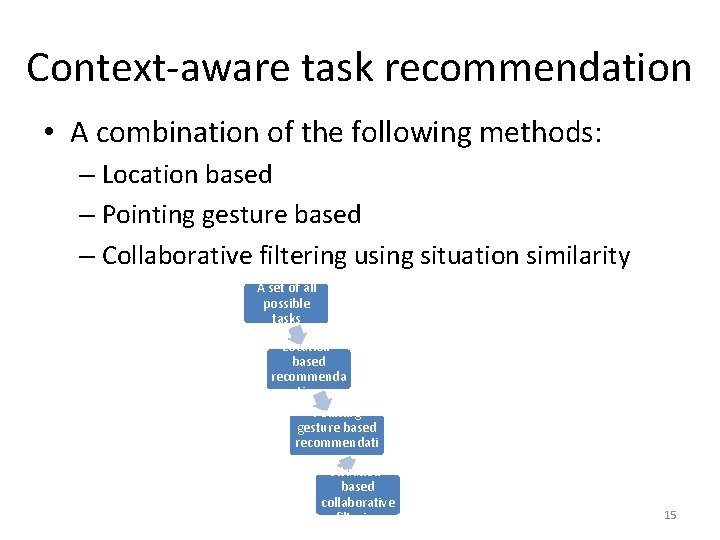
Context-aware task recommendation • A combination of the following methods: – Location based – Pointing gesture based – Collaborative filtering using situation similarity A set of all possible tasks Locationbased recommenda tion Pointing gesture based recommendati on Situationbased collaborative filtering 15
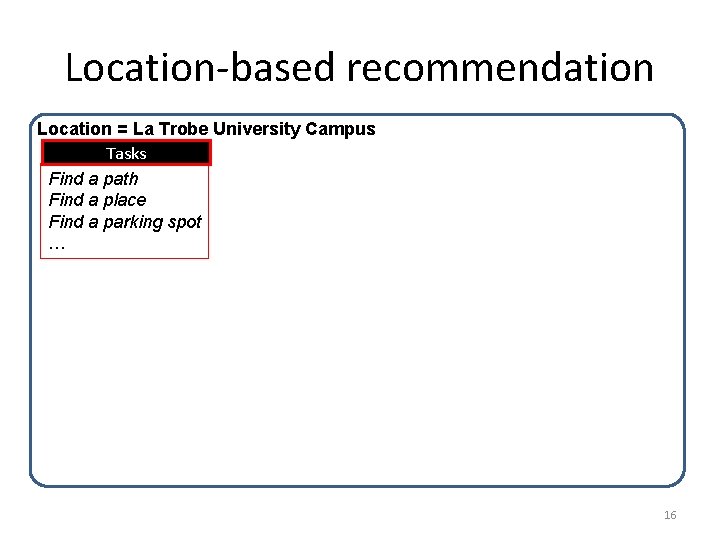
Location-based recommendation Location = La Trobe University Campus Tasks Find a path Find a place Find a parking spot … 16
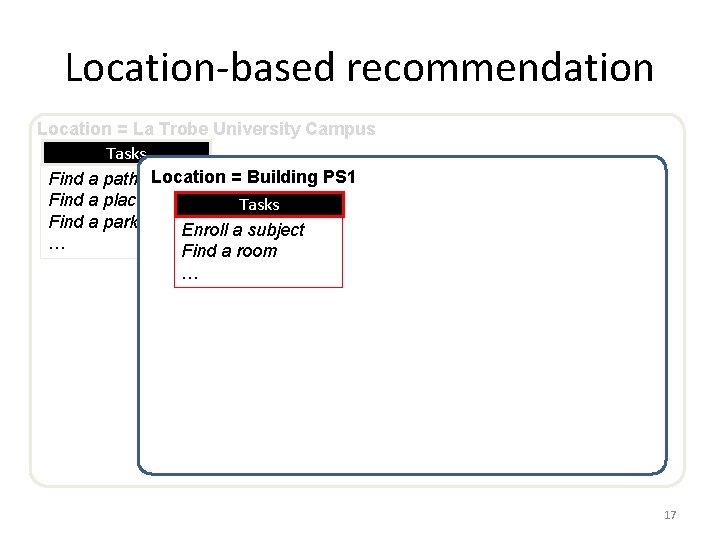
Location-based recommendation Location = La Trobe University Campus Tasks Find a path Location = Building PS 1 Find a place Tasks Find a parking spot Enroll a subject … Find a room … 17
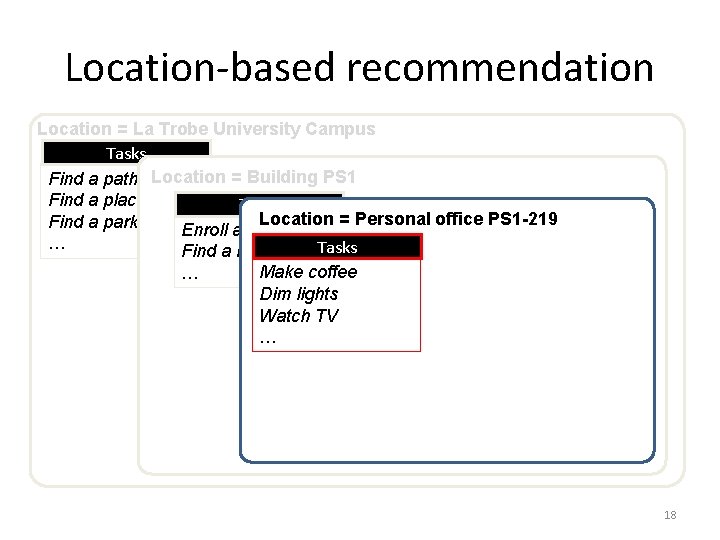
Location-based recommendation Location = La Trobe University Campus Tasks Find a path Location = Building PS 1 Find a place Tasks Location = Personal office PS 1 -219 Find a parking spot Enroll a subject … Tasks Find a room Make coffee … Dim lights Watch TV … 18
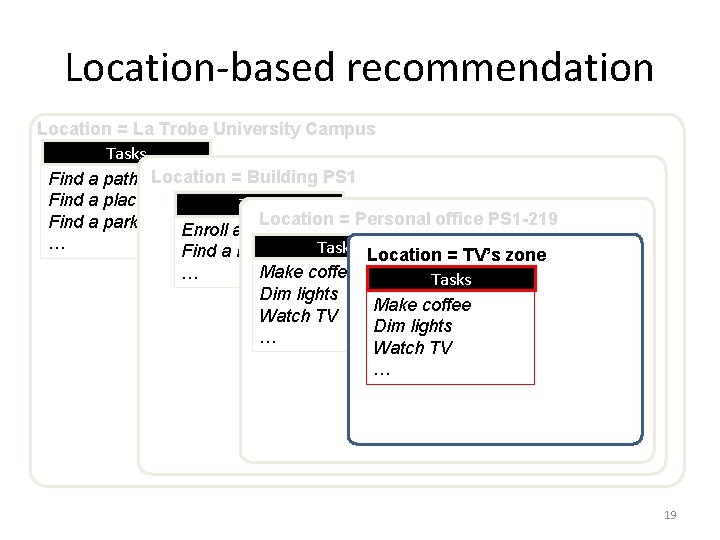
Location-based recommendation Location = La Trobe University Campus Tasks Find a path Location = Building PS 1 Find a place Tasks Location = Personal office PS 1 -219 Find a parking spot Enroll a subject … Tasks Location = TV’s zone Find a room Make coffee … Tasks Dim lights Make coffee Watch TV Dim lights … Watch TV … 19
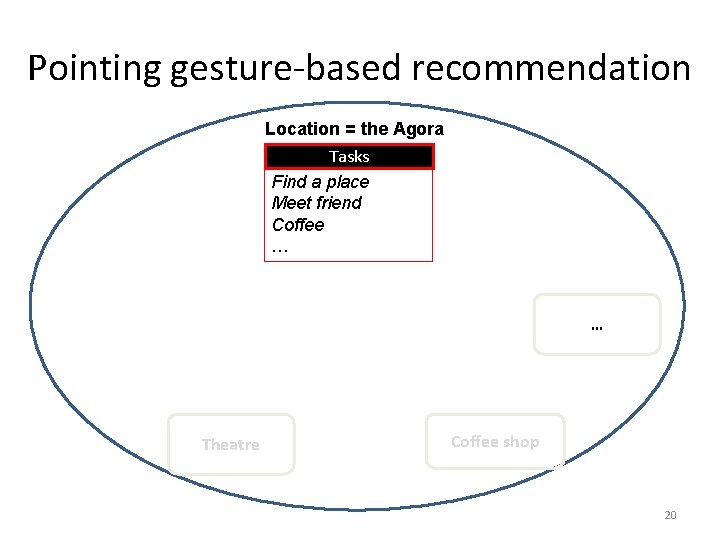
Pointing gesture-based recommendation Location = the Agora Tasks Find a place Meet friend Coffee … … Theatre Coffee shop 20
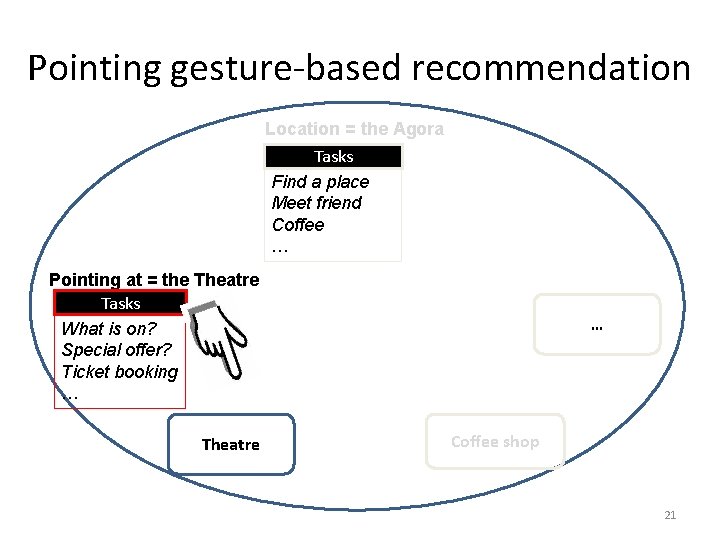
Pointing gesture-based recommendation Location = the Agora Tasks Find a place Meet friend Coffee … Pointing at = the Theatre Tasks What is on? Special offer? Ticket booking … Theatre … Coffee shop 21
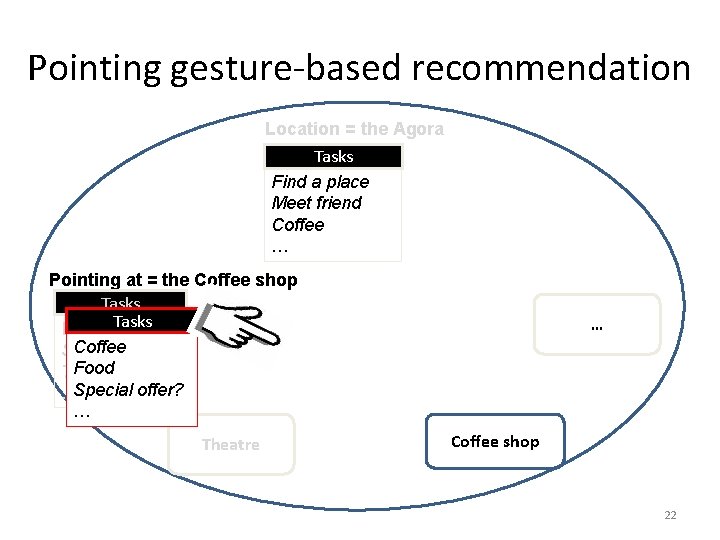
Pointing gesture-based recommendation Location = the Agora Tasks Find a place Meet friend Coffee … Pointing at = the Coffee shop Tasks What is. Tasks on? Coffeeoffer? Special Foodbooking Ticket …Special offer? … Theatre … Coffee shop 22
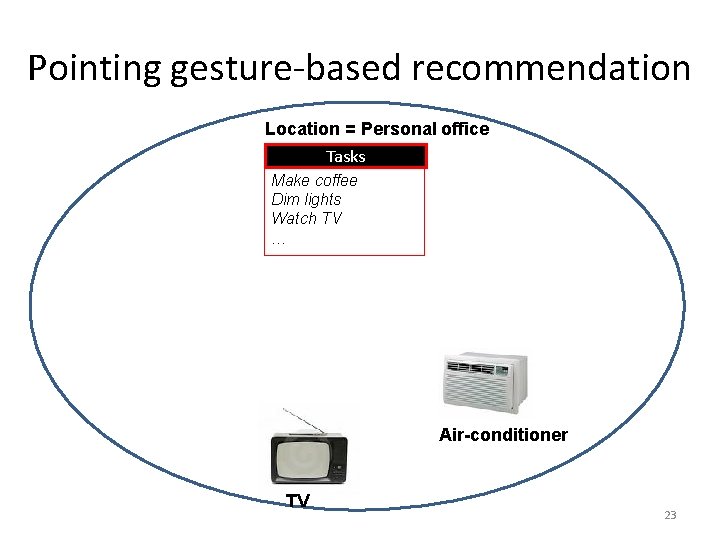
Pointing gesture-based recommendation Location = Personal office Tasks Make coffee Dim lights Watch TV … Air-conditioner TV 23
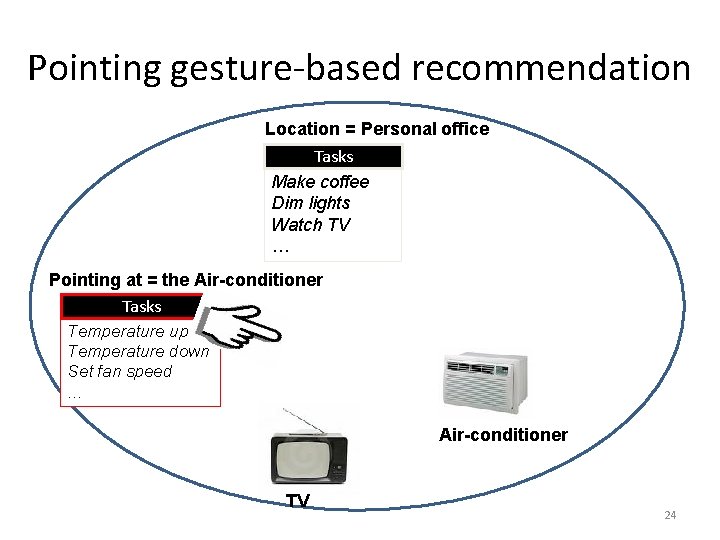
Pointing gesture-based recommendation Location = Personal office Tasks Make coffee Dim lights Watch TV … Pointing at = the Air-conditioner Tasks Temperature up Temperature down Set fan speed … Air-conditioner TV 24
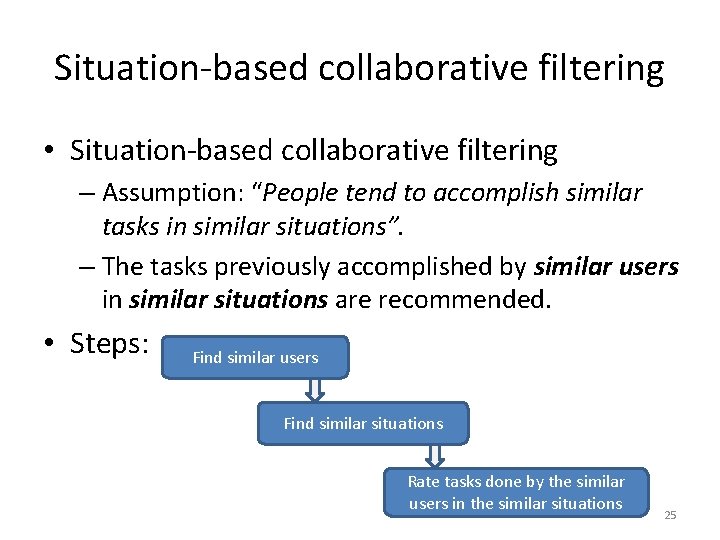
Situation-based collaborative filtering • Situation-based collaborative filtering – Assumption: “People tend to accomplish similar tasks in similar situations”. – The tasks previously accomplished by similar users in similar situations are recommended. • Steps: Find similar users Find similar situations Rate tasks done by the similar users in the similar situations 25
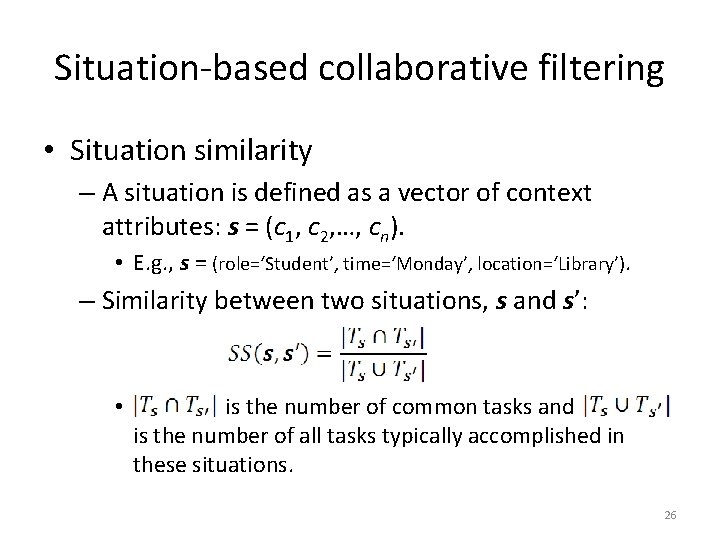
Situation-based collaborative filtering • Situation similarity – A situation is defined as a vector of context attributes: s = (c 1, c 2, …, cn). • E. g. , s = (role=‘Student’, time=‘Monday’, location=‘Library’). – Similarity between two situations, s and s’: • is the number of common tasks and is the number of all tasks typically accomplished in these situations. 26
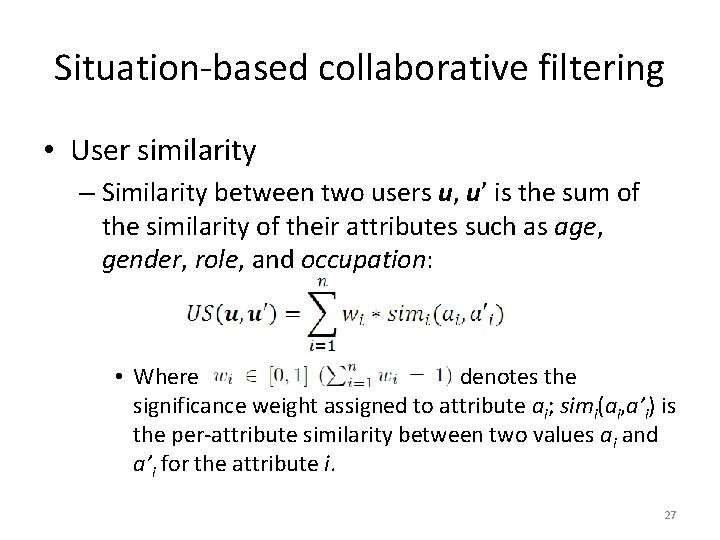
Situation-based collaborative filtering • User similarity – Similarity between two users u, u’ is the sum of the similarity of their attributes such as age, gender, role, and occupation: • Where denotes the significance weight assigned to attribute ai; simi(ai, a’i) is the per-attribute similarity between two values ai and a’i for the attribute i. 27
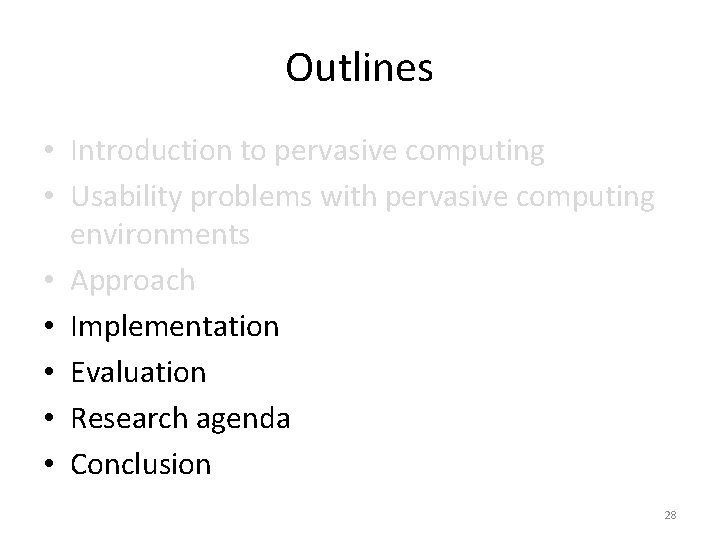
Outlines • Introduction to pervasive computing • Usability problems with pervasive computing environments • Approach • Implementation • Evaluation • Research agenda • Conclusion 28
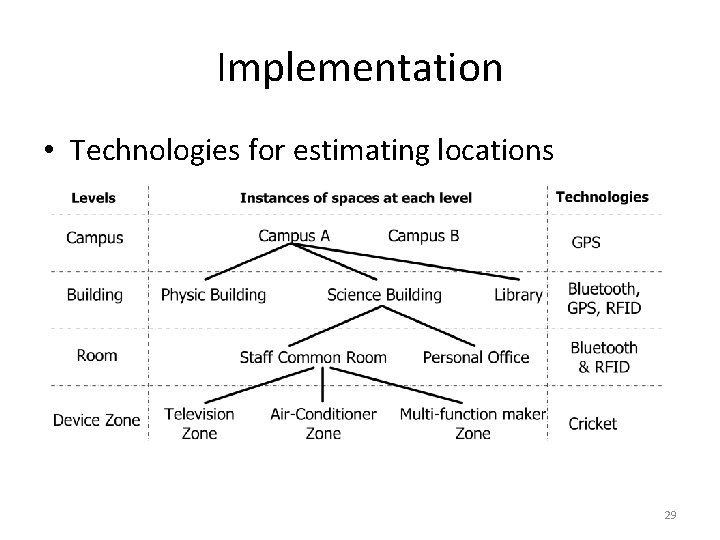
Implementation • Technologies for estimating locations 29
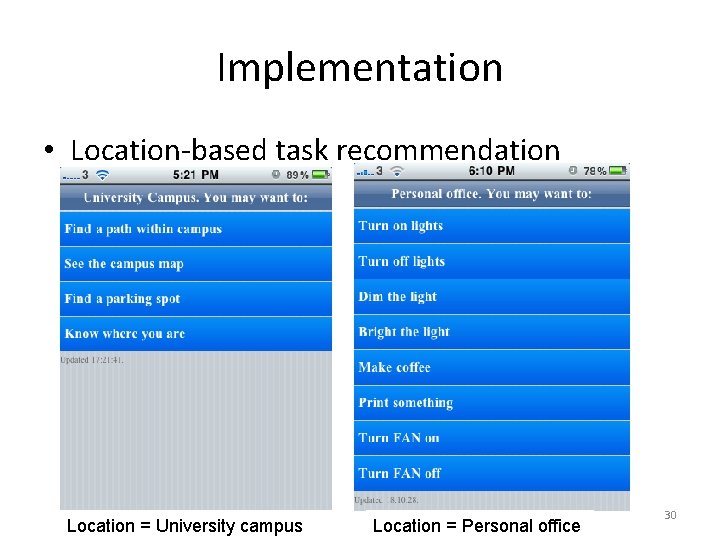
Implementation • Location-based task recommendation Location = University campus Location = Personal office 30
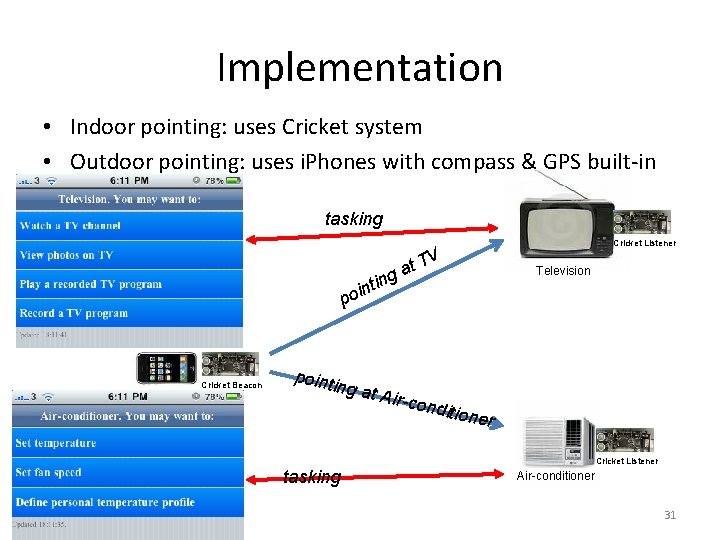
Implementation • Indoor pointing: uses Cricket system • Outdoor pointing: uses i. Phones with compass & GPS built-in tasking V g ntin at T Cricket Listener Television poi Cricket Beacon pointi n g at A ir-con dition er Cricket Listener tasking Air-conditioner 31
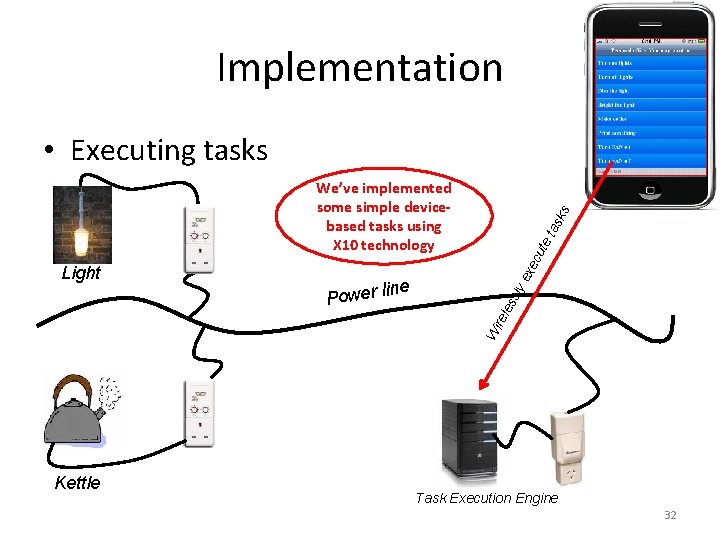
Implementation • Executing tasks ute ec ex sly les ine Power l Wi re Light tas ks We’ve implemented some simple devicebased tasks using X 10 technology Kettle Task Execution Engine 32
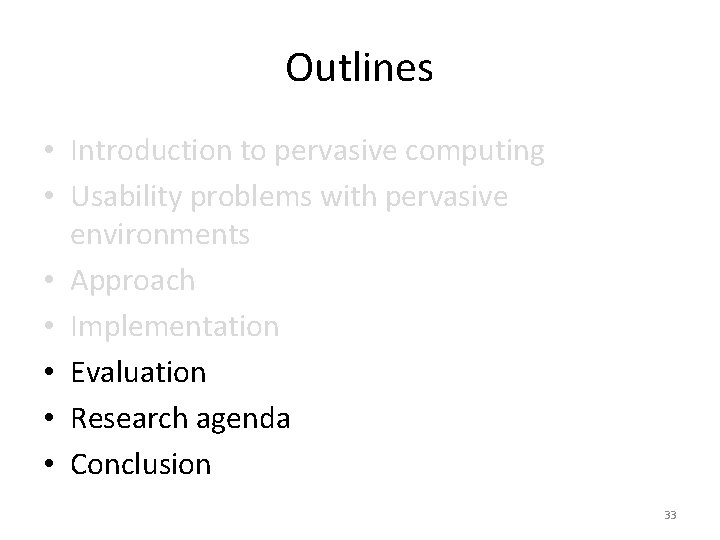
Outlines • Introduction to pervasive computing • Usability problems with pervasive environments • Approach • Implementation • Evaluation • Research agenda • Conclusion 33
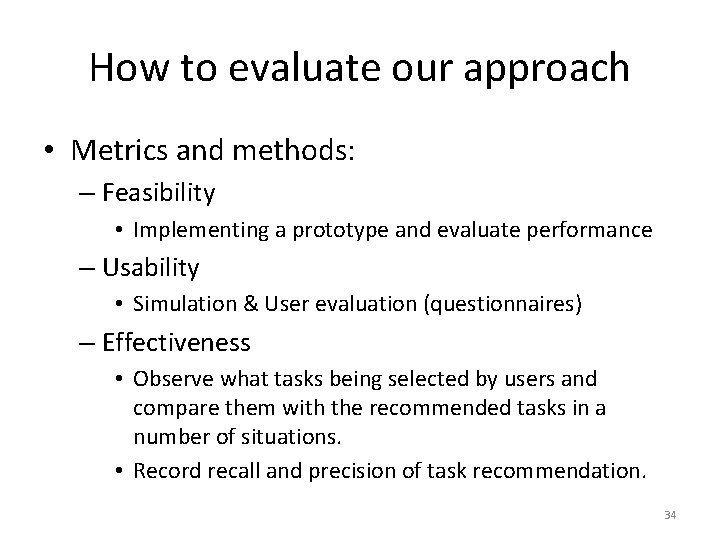
How to evaluate our approach • Metrics and methods: – Feasibility • Implementing a prototype and evaluate performance – Usability • Simulation & User evaluation (questionnaires) – Effectiveness • Observe what tasks being selected by users and compare them with the recommended tasks in a number of situations. • Record recall and precision of task recommendation. 34
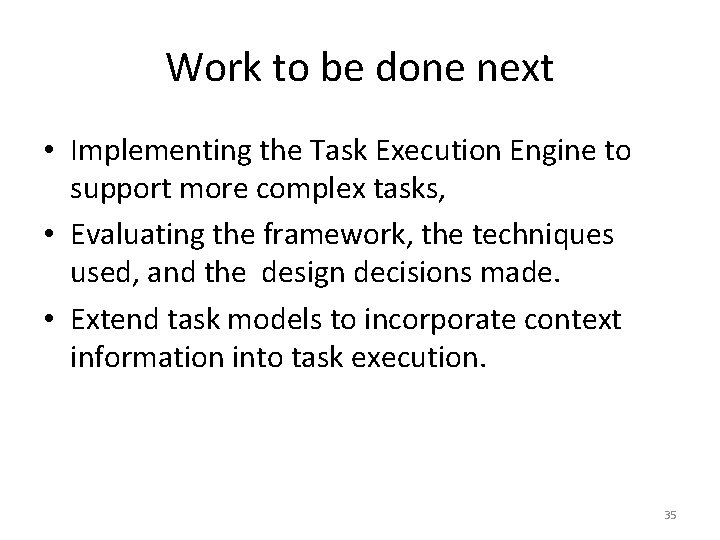
Work to be done next • Implementing the Task Execution Engine to support more complex tasks, • Evaluating the framework, the techniques used, and the design decisions made. • Extend task models to incorporate context information into task execution. 35
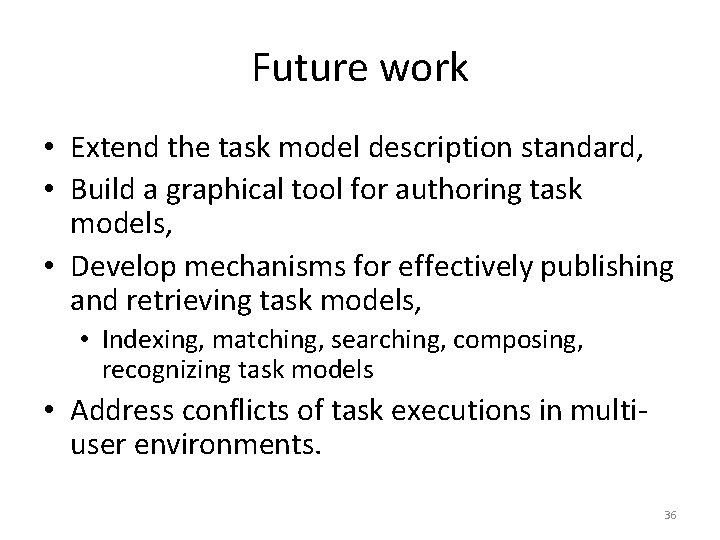
Future work • Extend the task model description standard, • Build a graphical tool for authoring task models, • Develop mechanisms for effectively publishing and retrieving task models, • Indexing, matching, searching, composing, recognizing task models • Address conflicts of task executions in multiuser environments. 36
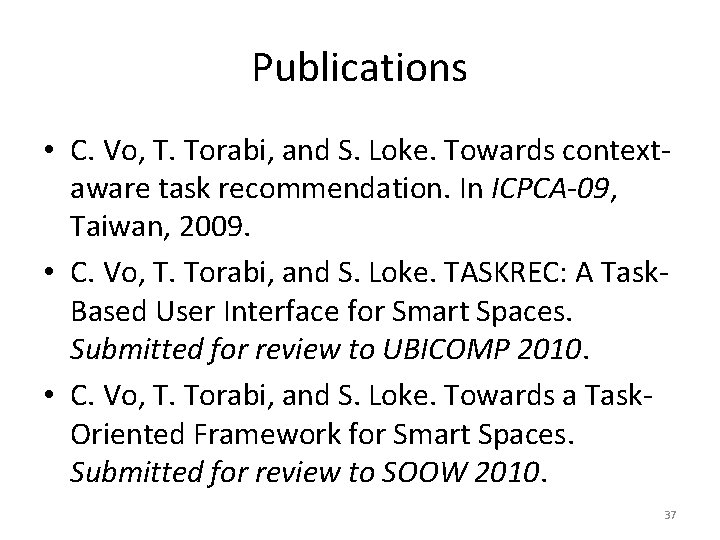
Publications • C. Vo, T. Torabi, and S. Loke. Towards contextaware task recommendation. In ICPCA-09, Taiwan, 2009. • C. Vo, T. Torabi, and S. Loke. TASKREC: A Task. Based User Interface for Smart Spaces. Submitted for review to UBICOMP 2010. • C. Vo, T. Torabi, and S. Loke. Towards a Task. Oriented Framework for Smart Spaces. Submitted for review to SOOW 2010. 37
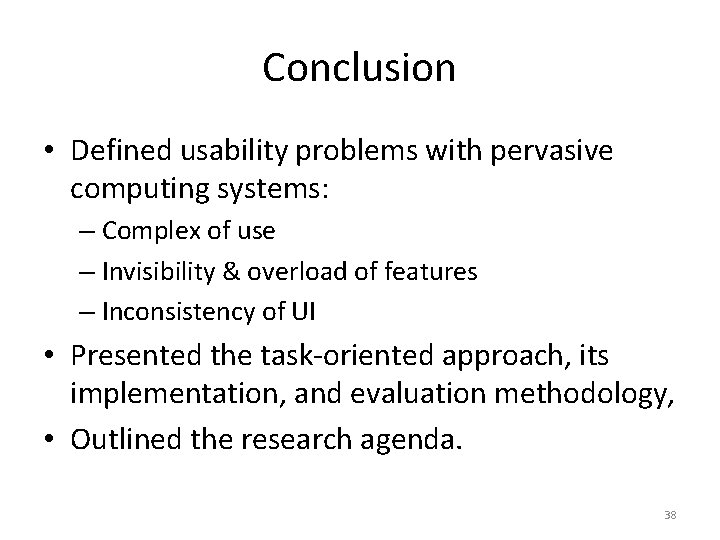
Conclusion • Defined usability problems with pervasive computing systems: – Complex of use – Invisibility & overload of features – Inconsistency of UI • Presented the task-oriented approach, its implementation, and evaluation methodology, • Outlined the research agenda. 38
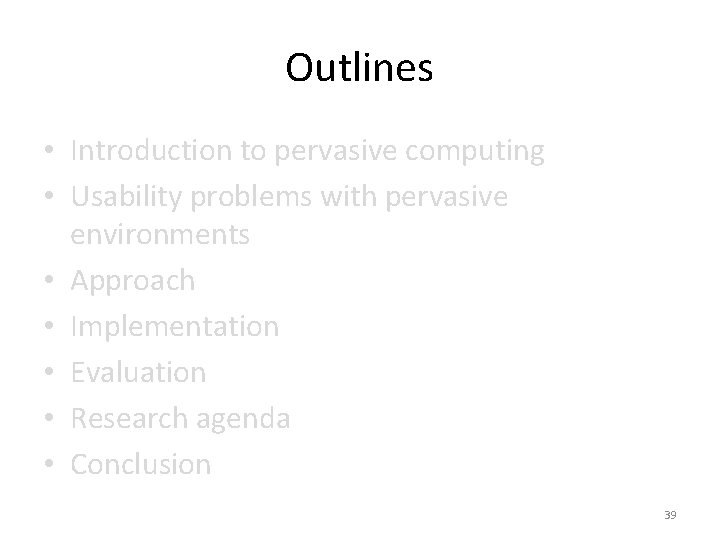
Outlines • Introduction to pervasive computing • Usability problems with pervasive environments • Approach • Implementation • Evaluation • Research agenda • Conclusion 39
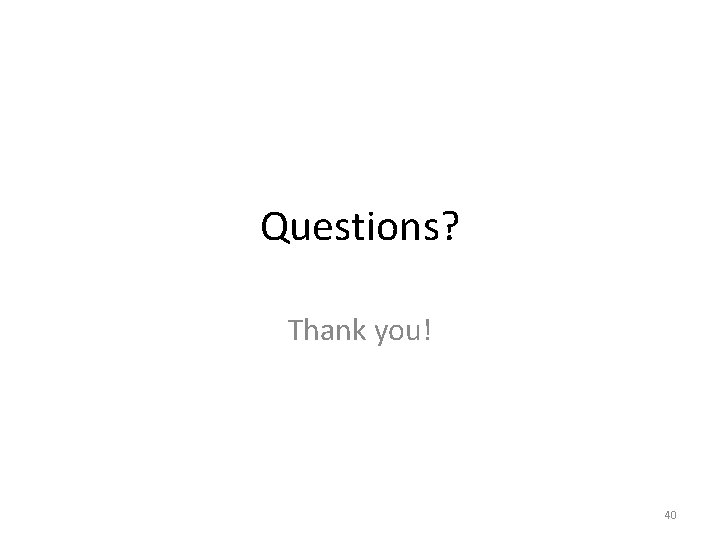
Questions? Thank you! 40
![Related work Situationaware application recommendation Cheng et al 2008 They recommend applications Related work • Situation-aware application recommendation [Cheng et al. 2008] – They recommend applications](https://slidetodoc.com/presentation_image_h2/4336a2be504e1dd9670431e076641350/image-41.jpg)
Related work • Situation-aware application recommendation [Cheng et al. 2008] – They recommend applications <> We recommend tasks (multi-apps) – They use pure situation similarity <> We use task based similarity • Homebird system [Rantapuska et al. 2008] – It recommends tasks based on features of devices discovered – However, because this approach does not consider user situation, it can recommend feasible tasks which may be not relevant. • Inter. Play [Messer et al. 2006] – For device integration and task orchestration in a networked home. – It asks user to express their intended tasks and assumes that the users have knowledge about feasible tasks. – In contrast, our approach can recommend relevant, feasible tasks without these requirements. • Context-dependent task discovery [Ni et al. 2006] – Discovering active tasks by matching current context with required context of tasks. – This can discover feasible tasks but potentially irrelevant tasks. • Task retrieval [Fukazawa et al. 2005] – Ask user to specify target names (e. g. , cafe shop, theatre) for retrieving tasks which are associated with these names. – Our system has integrated this knowledge into place/devices-related task repository. 41
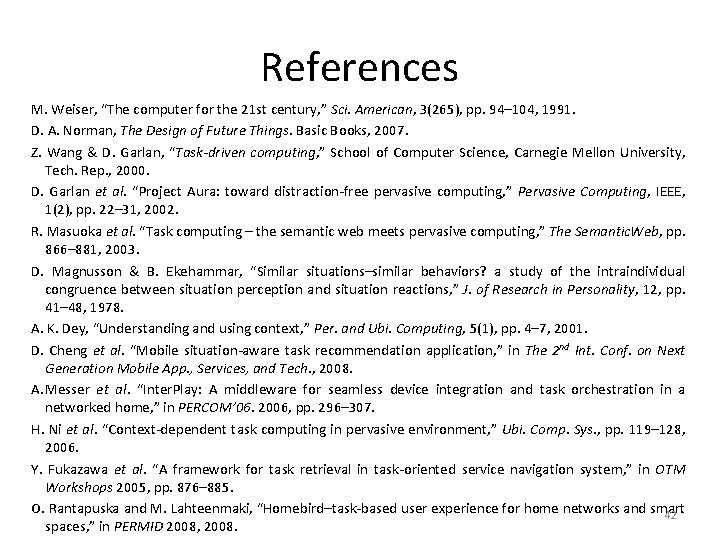
References M. Weiser, “The computer for the 21 st century, ” Sci. American, 3(265), pp. 94– 104, 1991. D. A. Norman, The Design of Future Things. Basic Books, 2007. Z. Wang & D. Garlan, “Task-driven computing, ” School of Computer Science, Carnegie Mellon University, Tech. Rep. , 2000. D. Garlan et al. “Project Aura: toward distraction-free pervasive computing, ” Pervasive Computing, IEEE, 1(2), pp. 22– 31, 2002. R. Masuoka et al. “Task computing – the semantic web meets pervasive computing, ” The Semantic. Web, pp. 866– 881, 2003. D. Magnusson & B. Ekehammar, “Similar situations–similar behaviors? a study of the intraindividual congruence between situation perception and situation reactions, ” J. of Research in Personality, 12, pp. 41– 48, 1978. A. K. Dey, “Understanding and using context, ” Per. and Ubi. Computing, 5(1), pp. 4– 7, 2001. D. Cheng et al. “Mobile situation-aware task recommendation application, ” in The 2 nd Int. Conf. on Next Generation Mobile App. , Services, and Tech. , 2008. A. Messer et al. “Inter. Play: A middleware for seamless device integration and task orchestration in a networked home, ” in PERCOM’ 06. 2006, pp. 296– 307. H. Ni et al. “Context-dependent task computing in pervasive environment, ” Ubi. Comp. Sys. , pp. 119– 128, 2006. Y. Fukazawa et al. “A framework for task retrieval in task-oriented service navigation system, ” in OTM Workshops 2005, pp. 876– 885. O. Rantapuska and M. Lahteenmaki, “Homebird–task-based user experience for home networks and smart 42 spaces, ” in PERMID 2008, 2008.
Mit mobile wireless
Pervasive and mobile computing
Pervasive computing ppt
Mobile and pervasive computing
Mobile computer wikipedia
Sand: towards high-performance serverless computing
Primary and secondary stakeholders
Pddst
X-trace: a pervasive network tracing framework
Pervasive developmental disorder
Orchastrate
Pervasive mood
Chapter 13 natural environments of europe
Stable prediction across unknown environments
Exercise in hot and cold environments
Interrelationship between micro and market environment
Implations
High quality supportive environments
International business: environments & operations
Diverse learning environments survey
Designing constructivist learning environments
Psychologically informed environments
International business environments and operations
Vmmaxvirtualdisks
Different environments
External environments and accountability of schools ppt
Characteristics of virtualization environment
Enabling environments eyfs
World geography
Psychologically informed environments
Diverse learning environments survey
Actors in marketing environment
The marketing environment
Simple reflex agents examples
Chapter 13 natural environments of europe
Business environment
International business environments and operations
Economic activity
Creating supportive environments smoking
International business environments and operations
Geometriska former i förskolan
Claes martinsson
Tillitsbaserad ledning