Towards Optimal Operation State Scheduling in RFPowered Internet
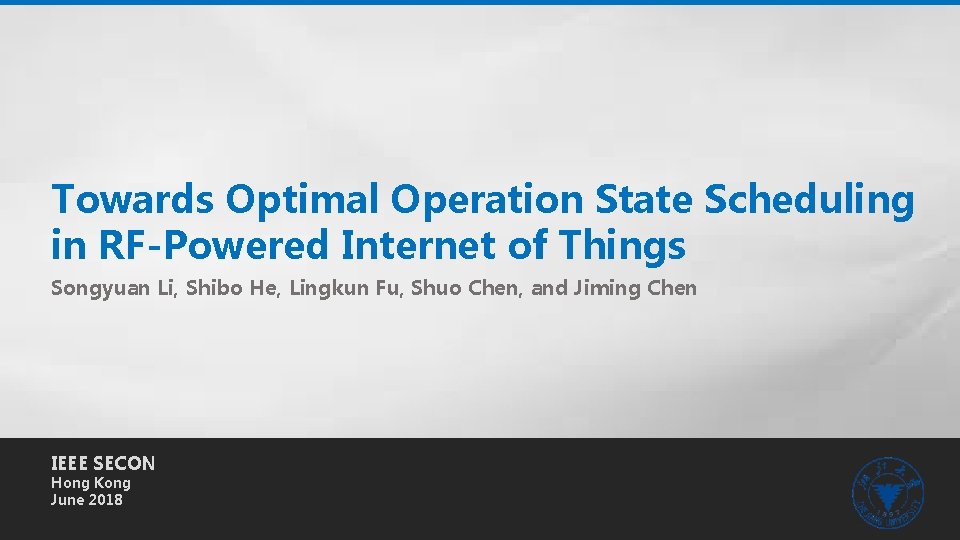
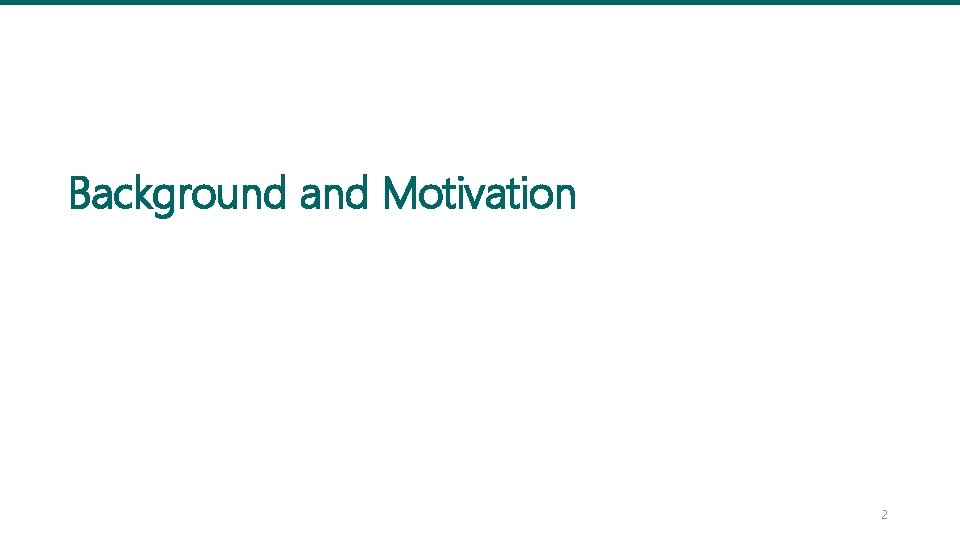
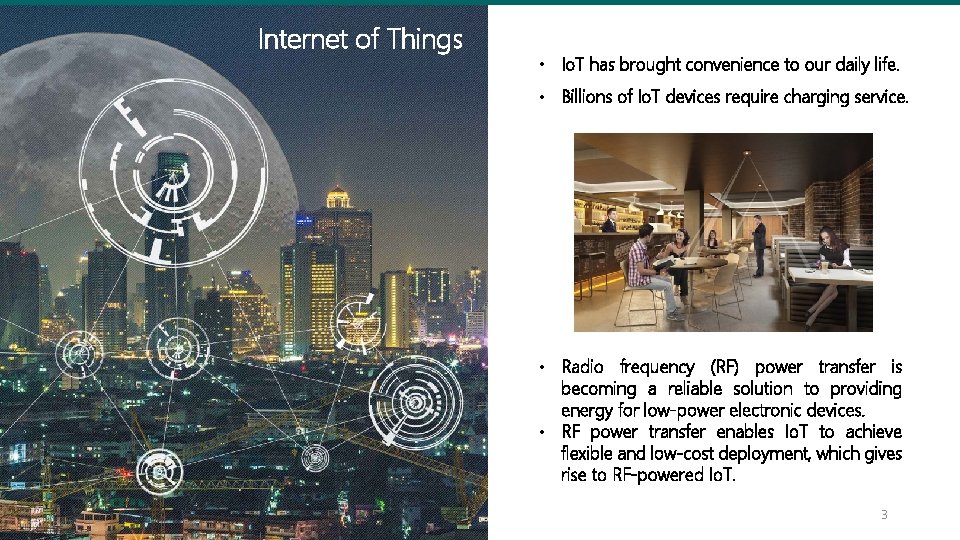
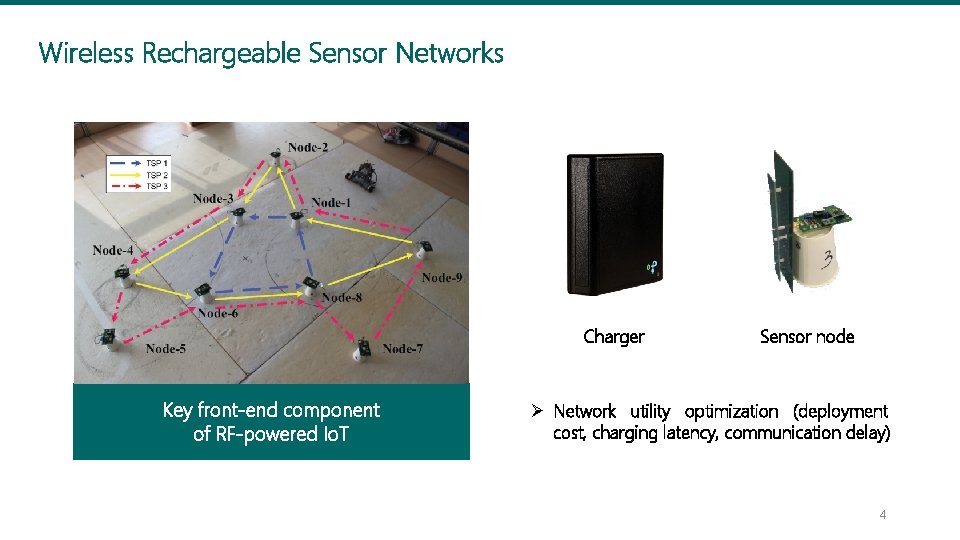
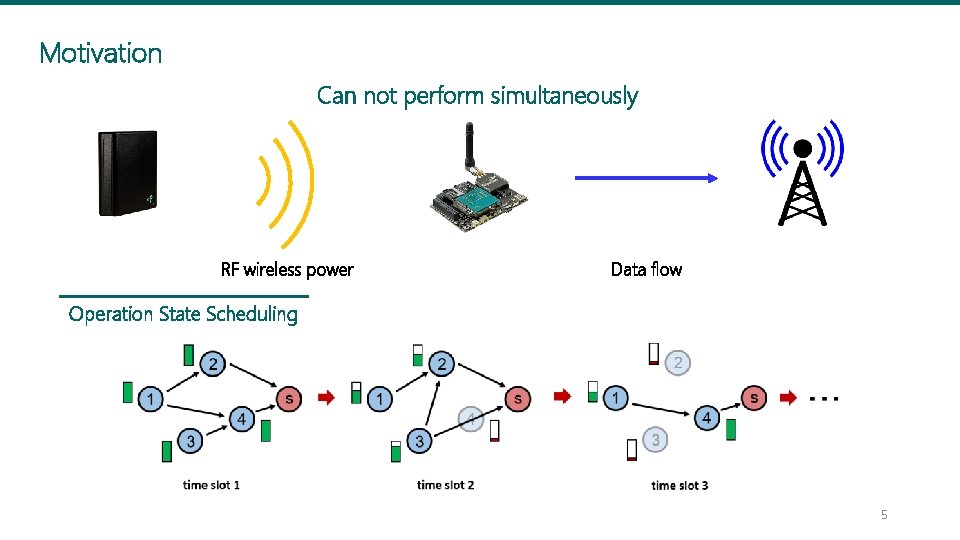
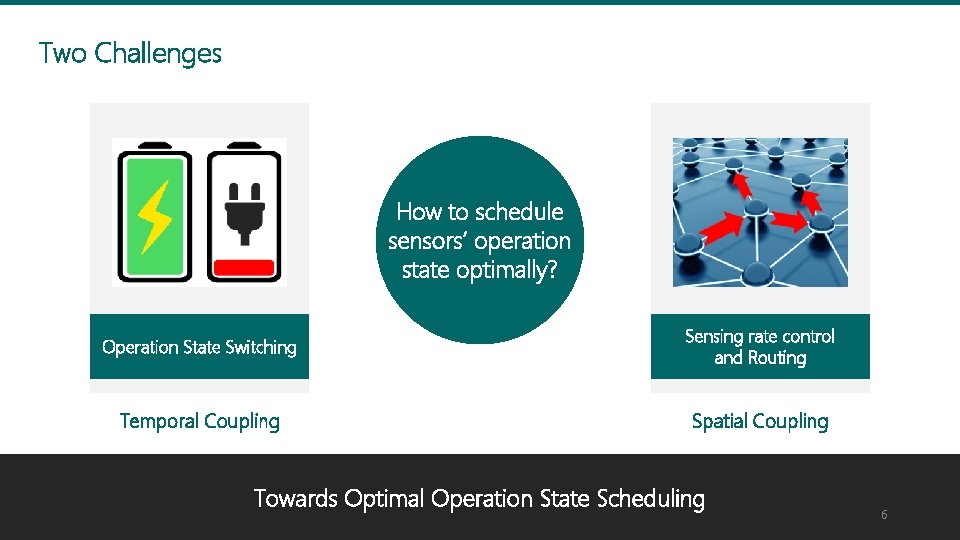
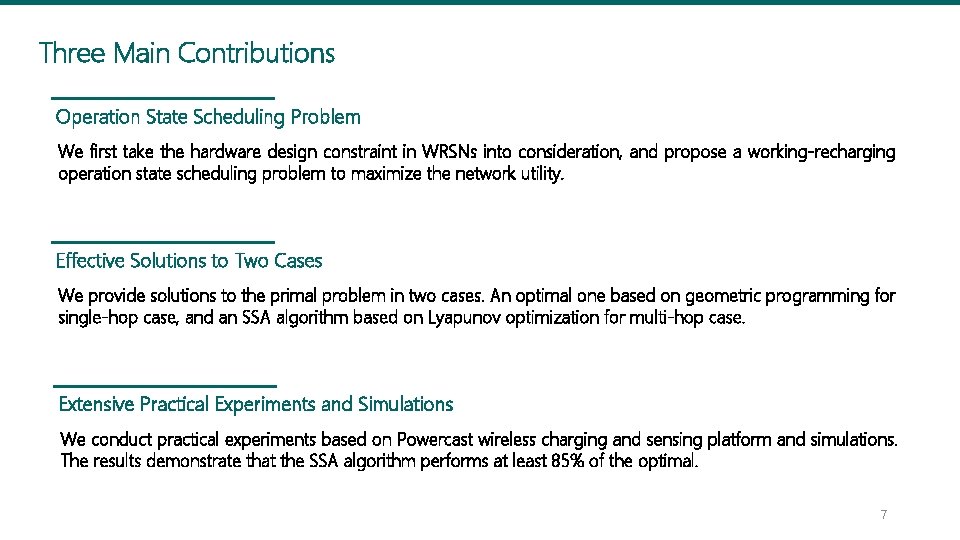
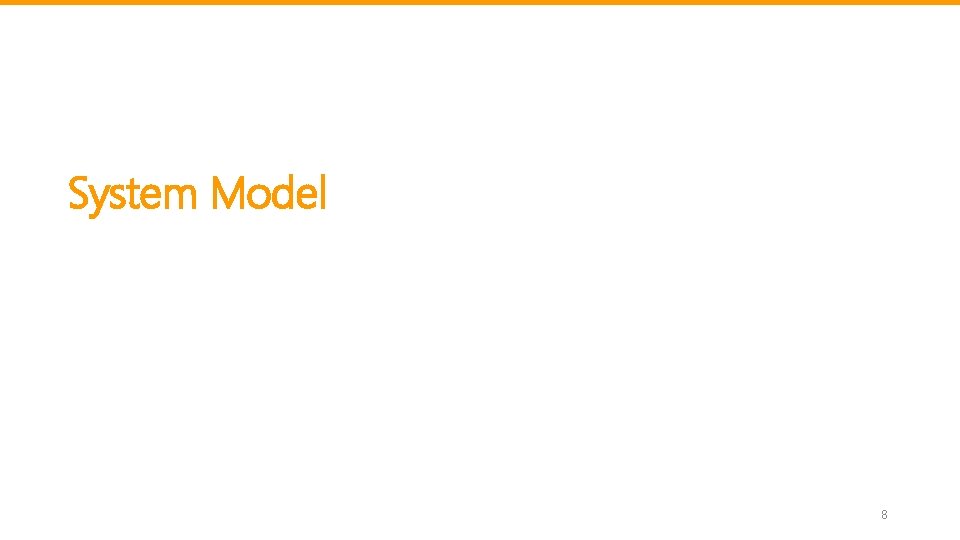
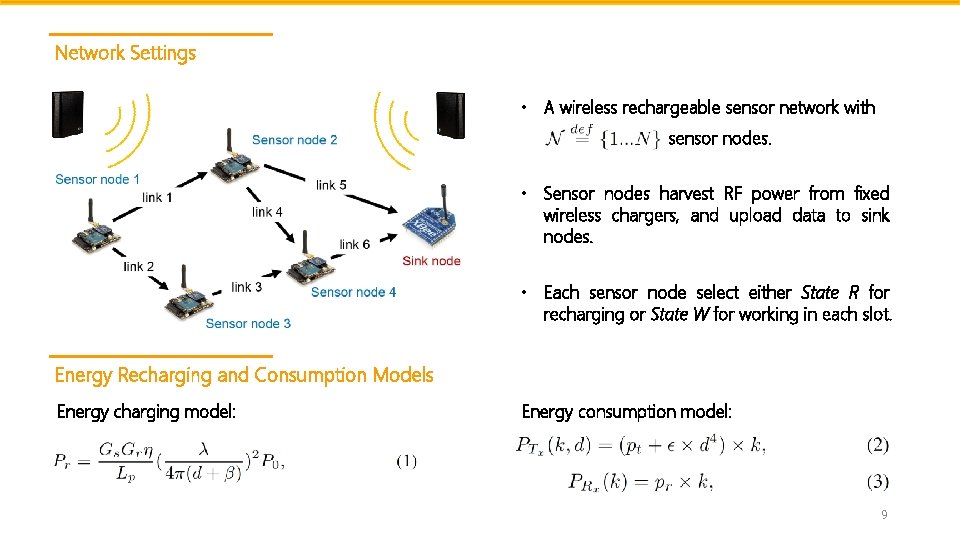
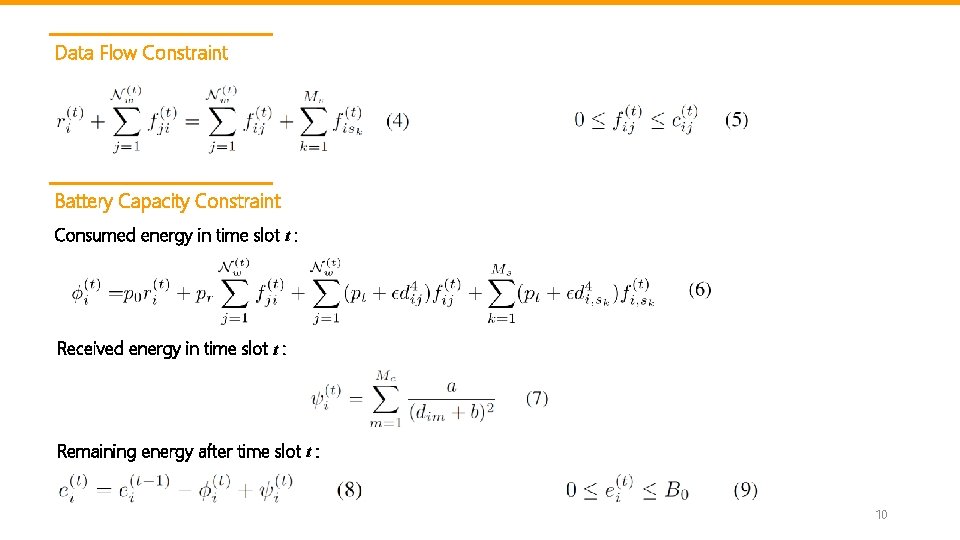
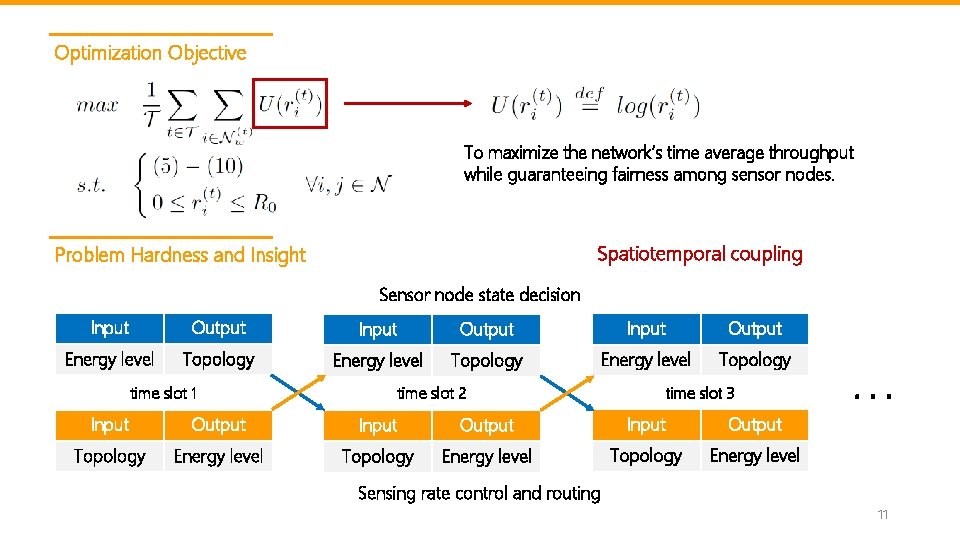
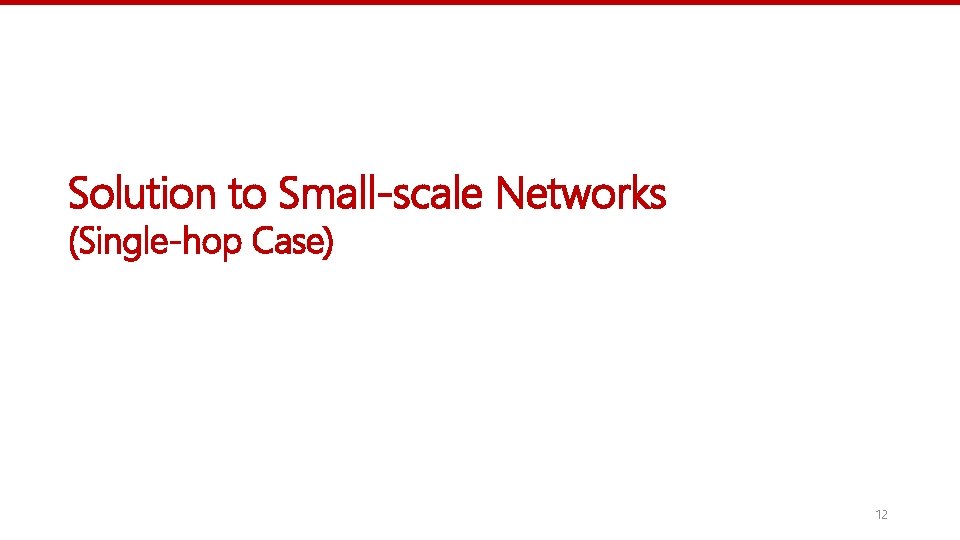
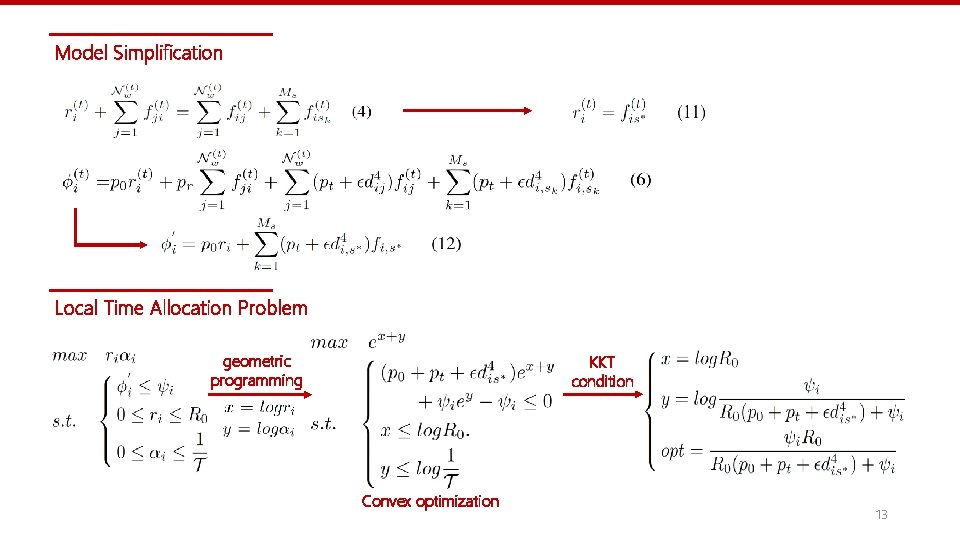
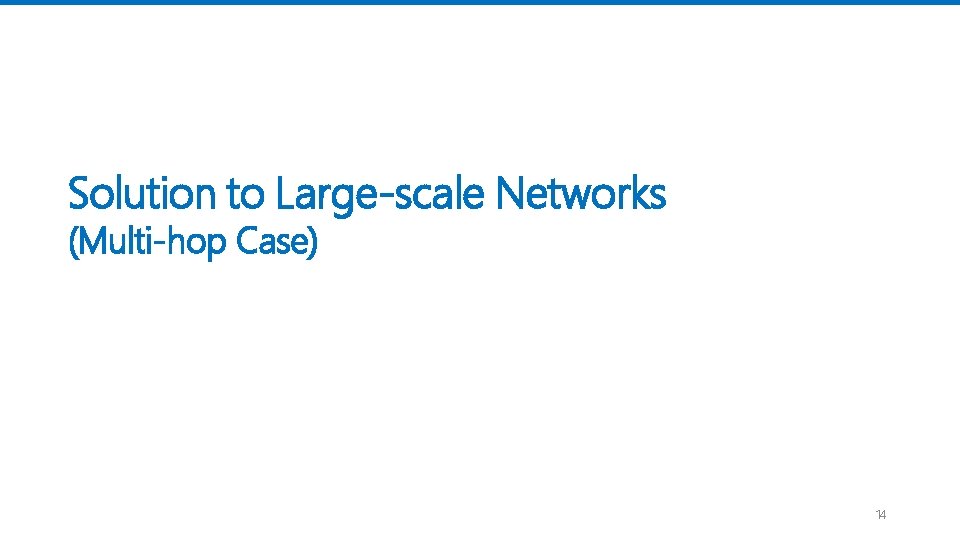
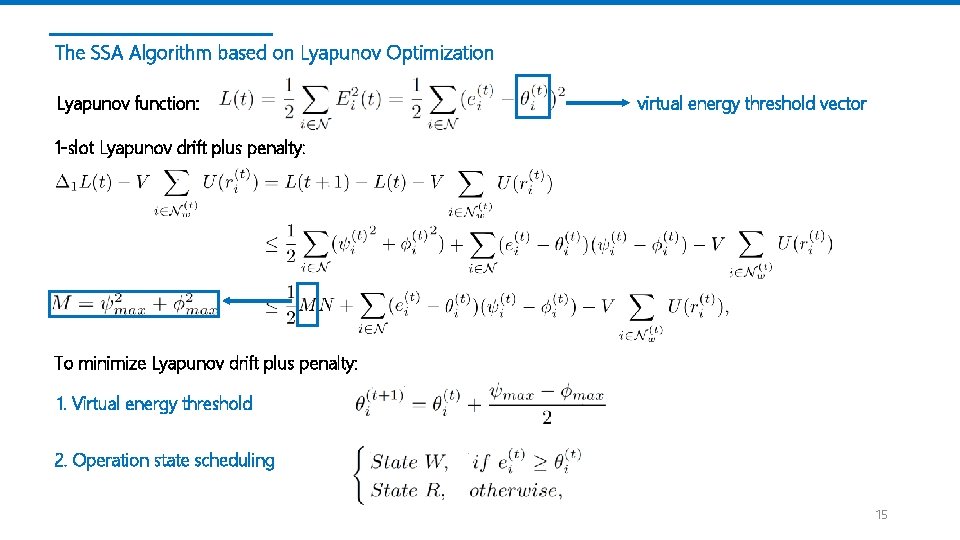
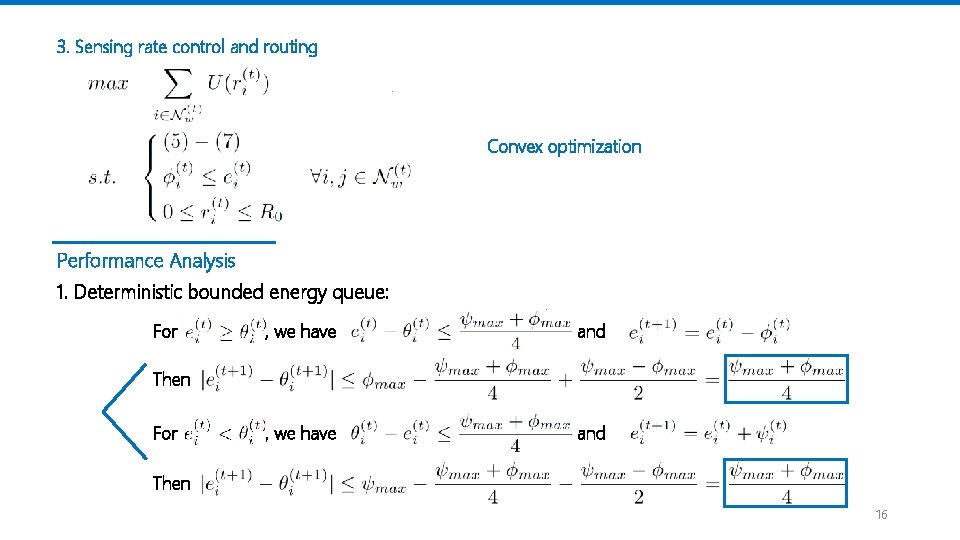
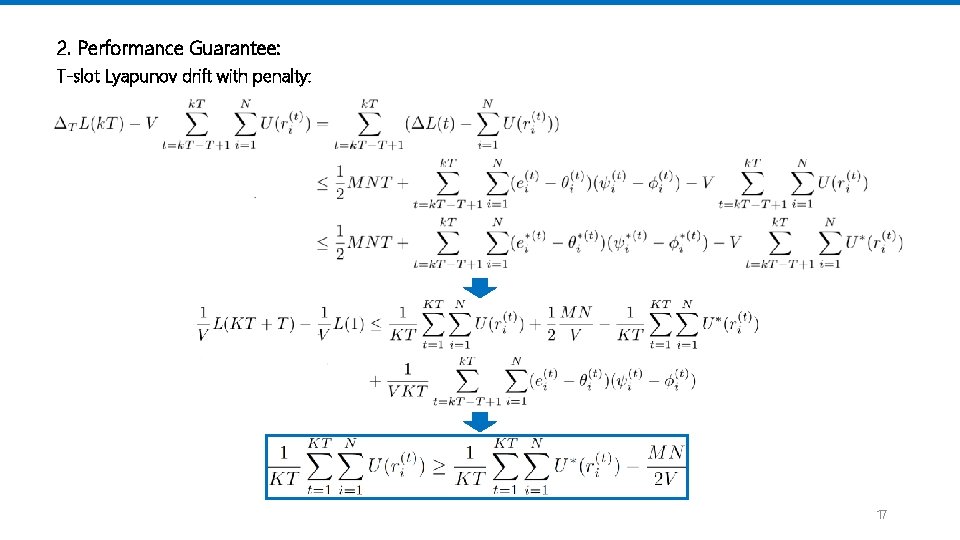
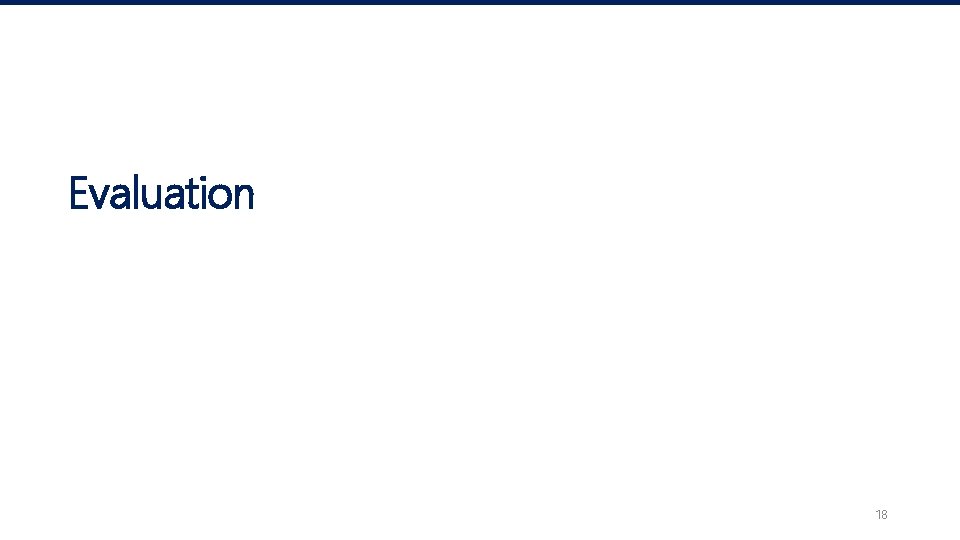
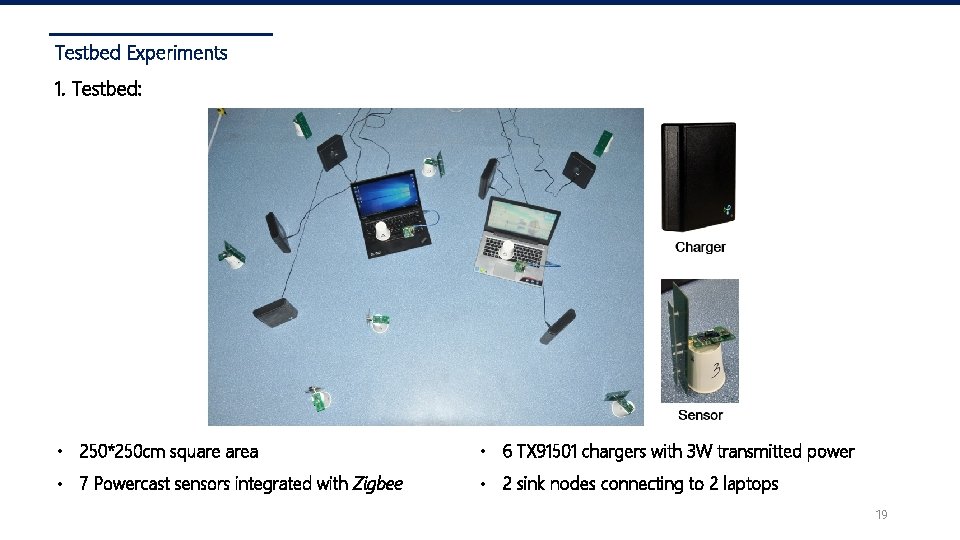
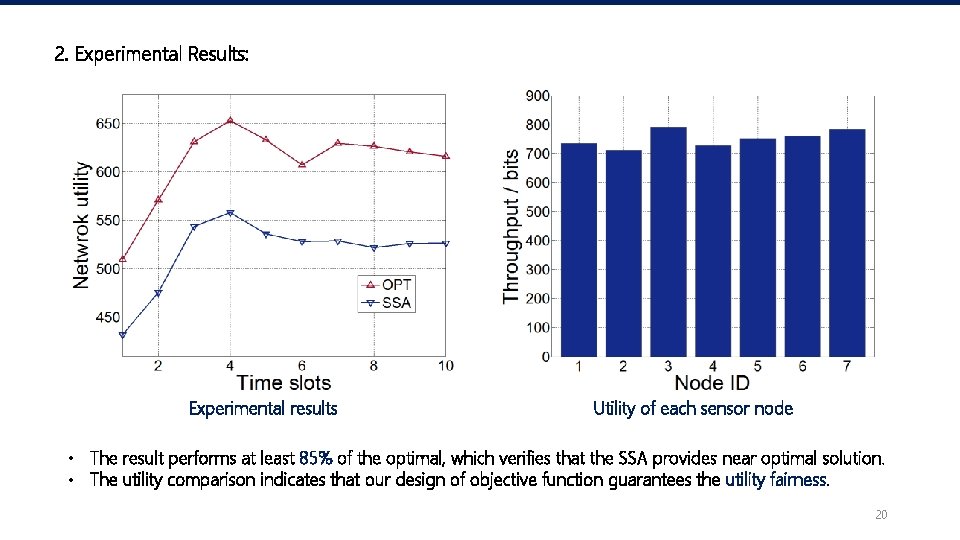
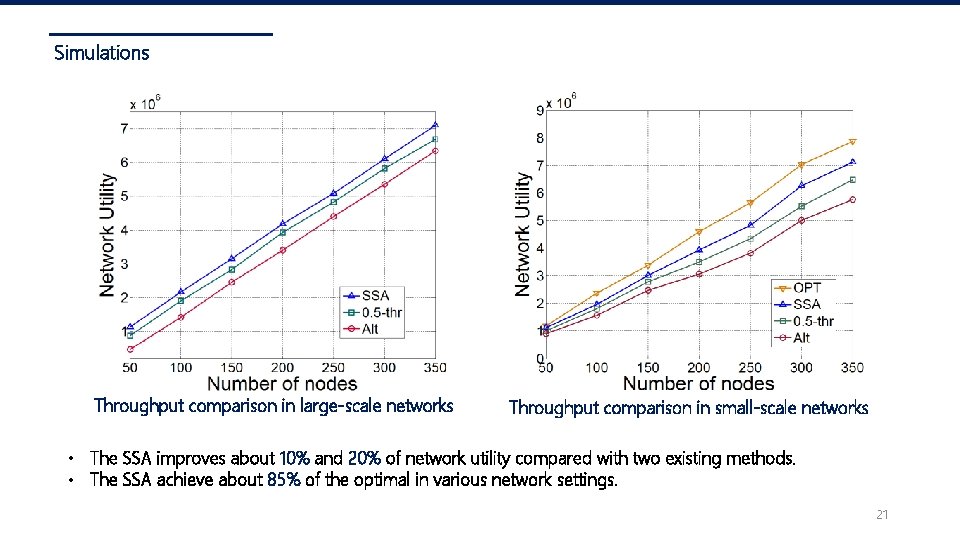
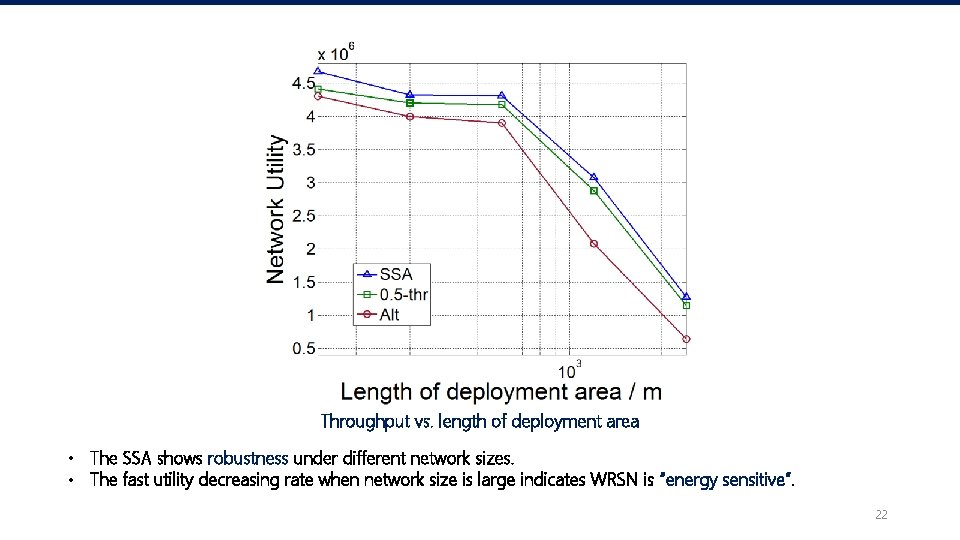
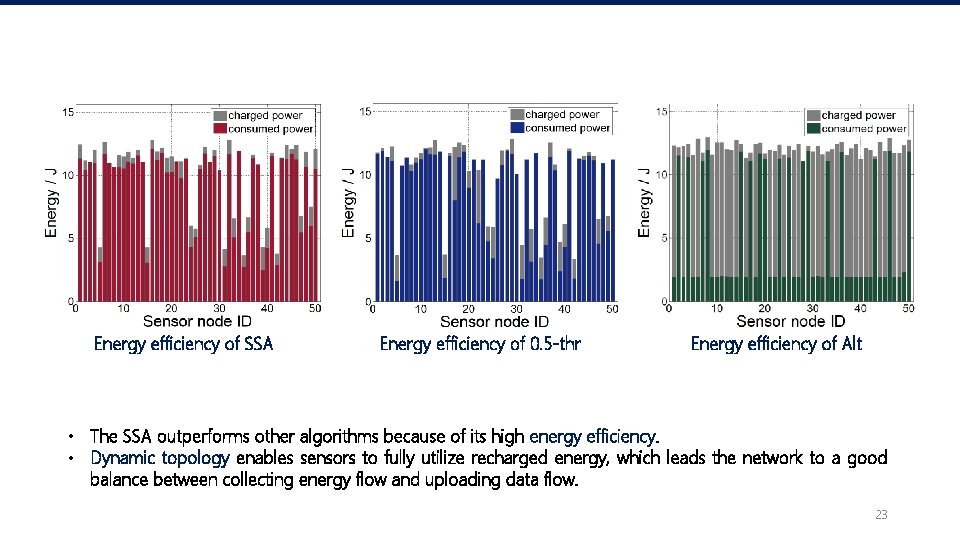
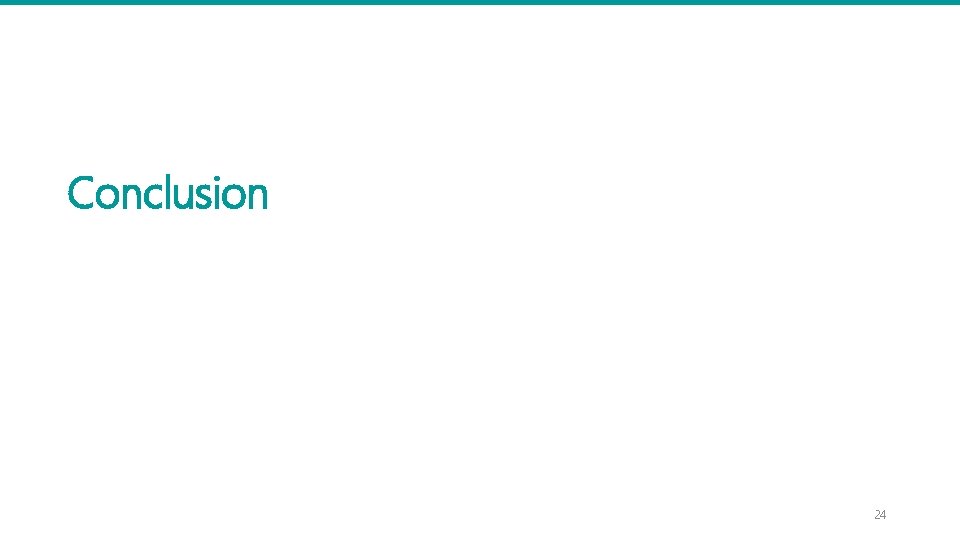
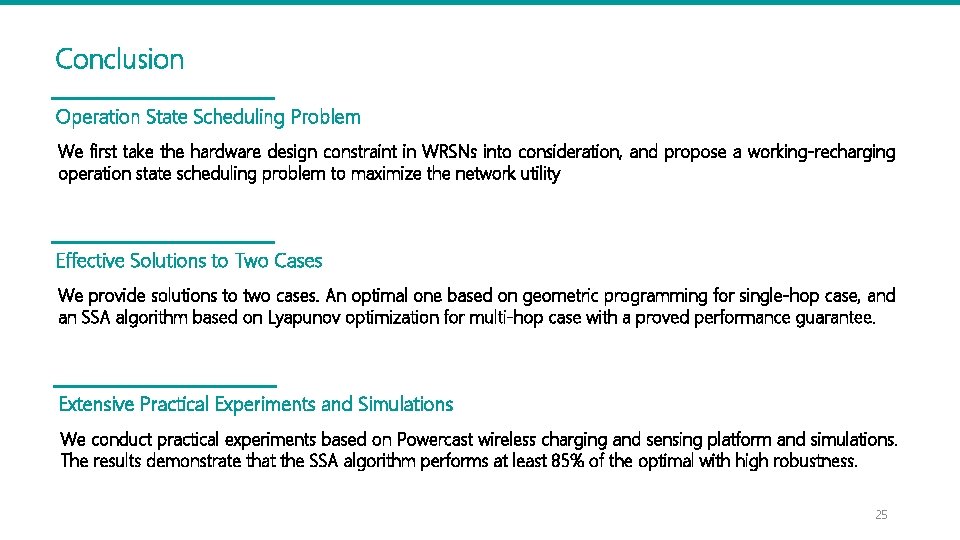
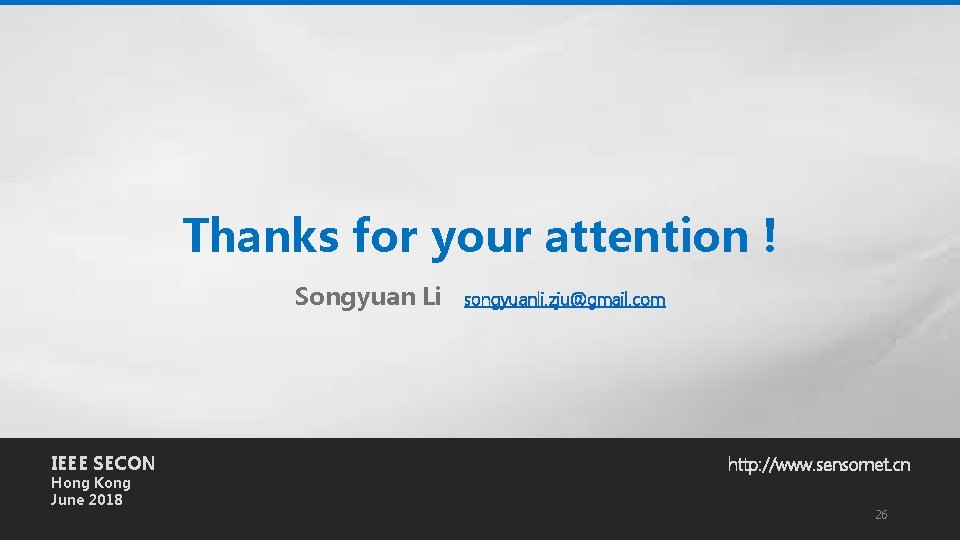
- Slides: 26
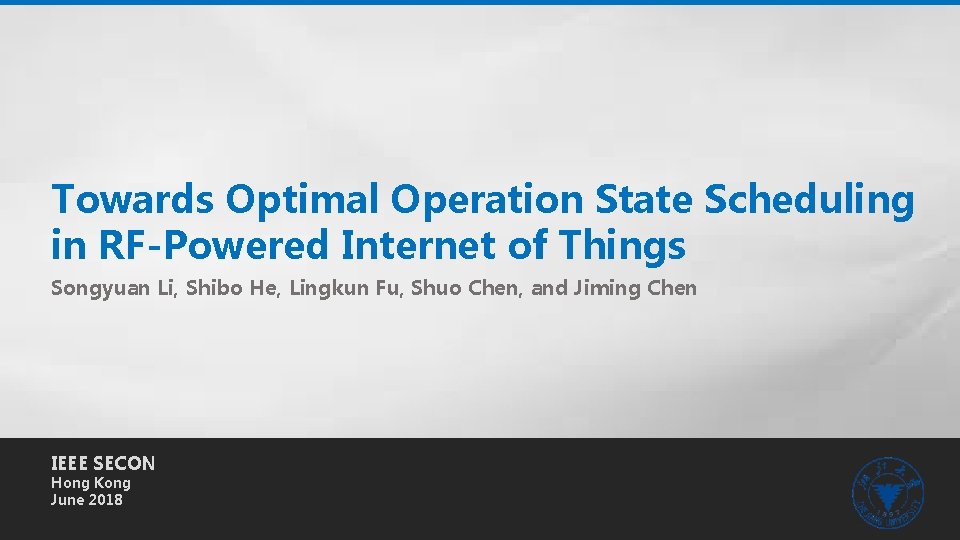
Towards Optimal Operation State Scheduling in RF-Powered Internet of Things Songyuan Li, Shibo He, Lingkun Fu, Shuo Chen, and Jiming Chen IEEE SECON Hong Kong June 2018
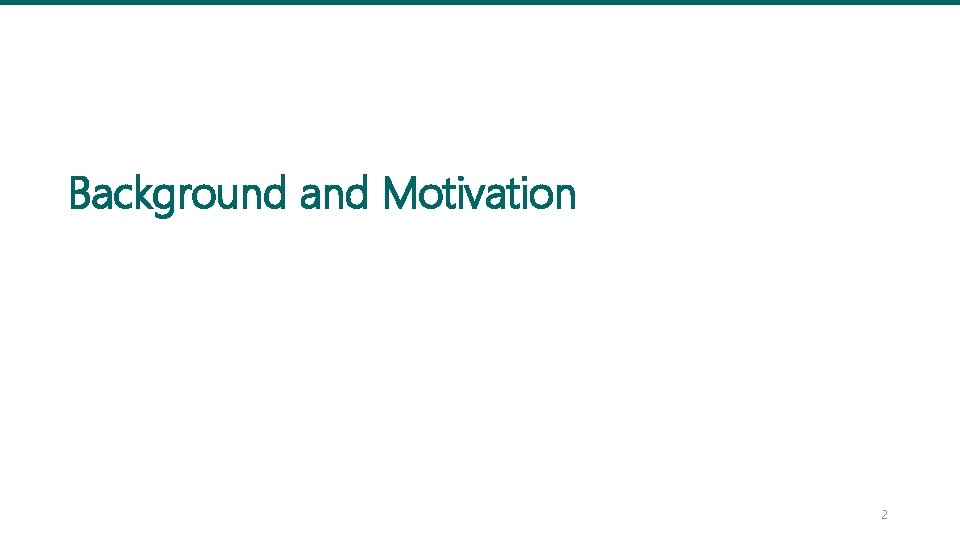
Background and Motivation 2
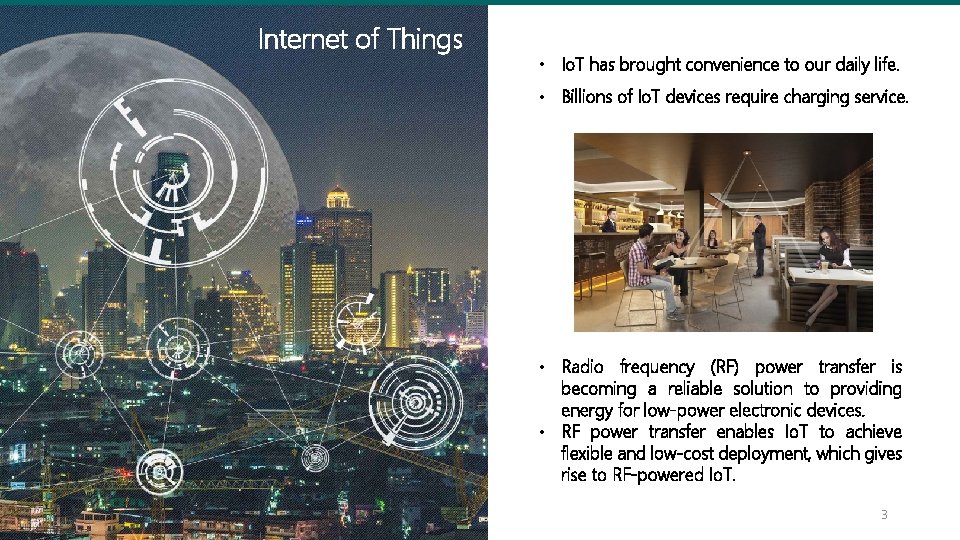
Internet of Things • Io. T has brought convenience to our daily life. • Billions of Io. T devices require charging service. • Radio frequency (RF) power transfer is becoming a reliable solution to providing energy for low-power electronic devices. • RF power transfer enables Io. T to achieve flexible and low-cost deployment, which gives rise to RF-powered Io. T. 3
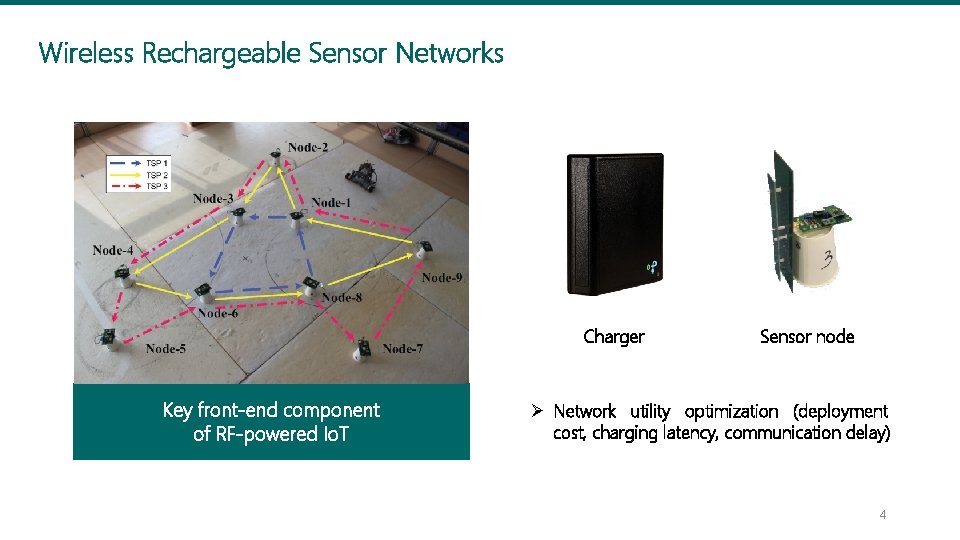
Wireless Rechargeable Sensor Networks Charger Key front-end component of RF-powered Io. T Sensor node Ø Network utility optimization (deployment cost, charging latency, communication delay) 4
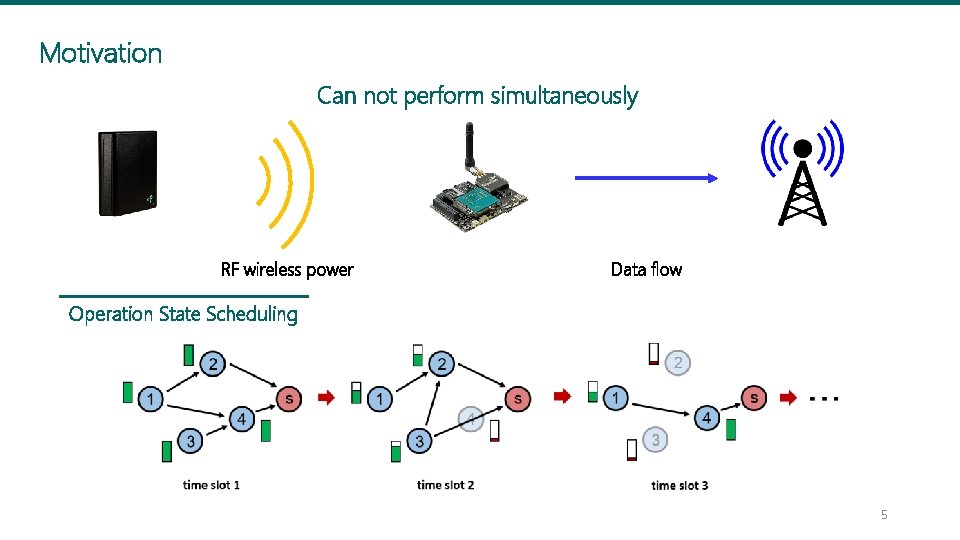
Motivation Can not perform simultaneously RF wireless power Data flow Operation State Scheduling 5
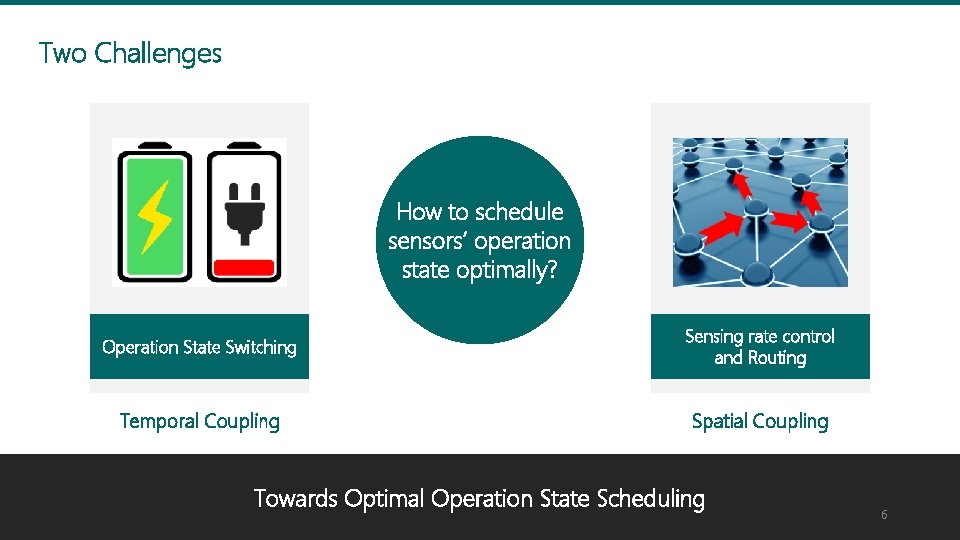
Two Challenges How to schedule sensors’ operation state optimally? Operation State Switching Sensing rate control and Routing Temporal Coupling Spatial Coupling Towards Optimal Operation State Scheduling 6
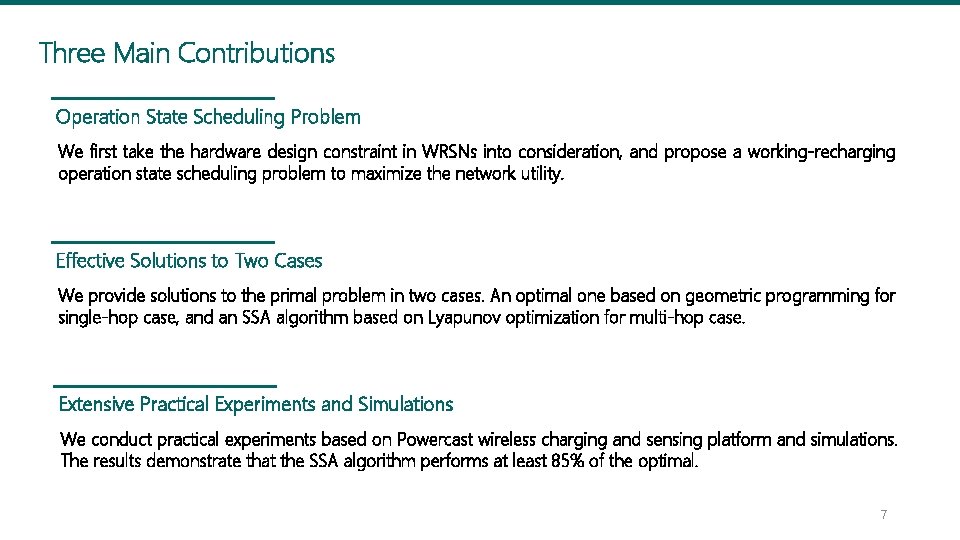
Three Main Contributions Operation State Scheduling Problem We first take the hardware design constraint in WRSNs into consideration, and propose a working-recharging operation state scheduling problem to maximize the network utility. Effective Solutions to Two Cases We provide solutions to the primal problem in two cases. An optimal one based on geometric programming for single-hop case, and an SSA algorithm based on Lyapunov optimization for multi-hop case. Extensive Practical Experiments and Simulations We conduct practical experiments based on Powercast wireless charging and sensing platform and simulations. The results demonstrate that the SSA algorithm performs at least 85% of the optimal. 7
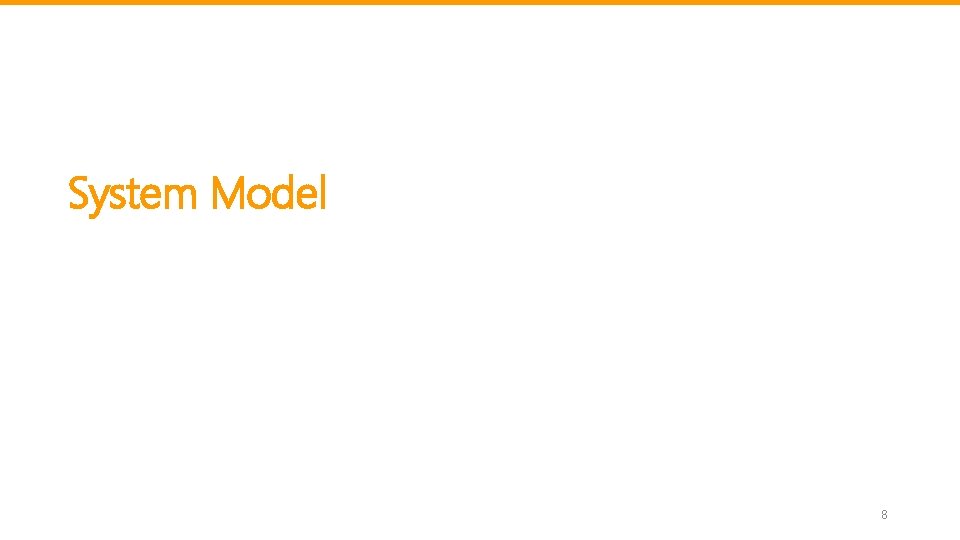
System Model 8
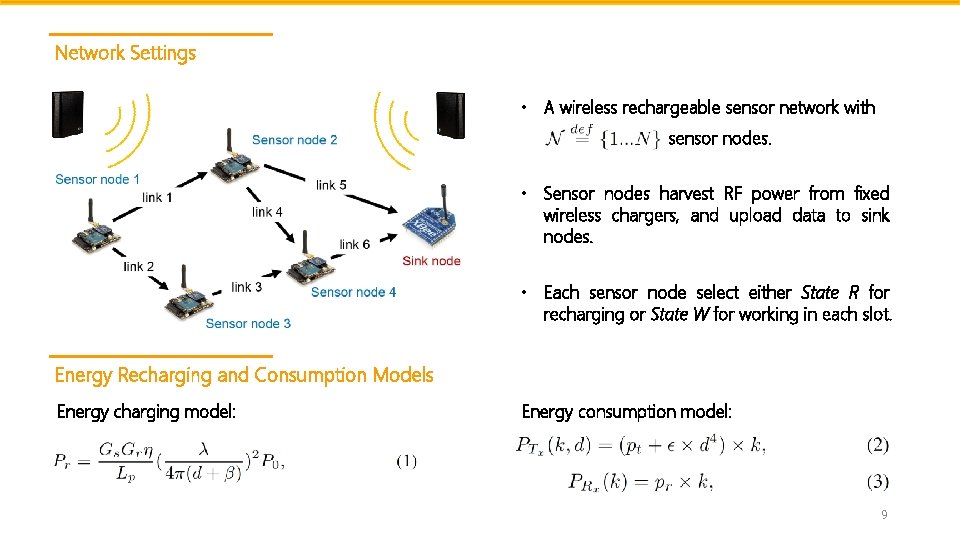
Network Settings • A wireless rechargeable sensor network with sensor nodes. • Sensor nodes harvest RF power from fixed wireless chargers, and upload data to sink nodes. • Each sensor node select either State R for recharging or State W for working in each slot. Energy Recharging and Consumption Models Energy charging model: Energy consumption model: 9
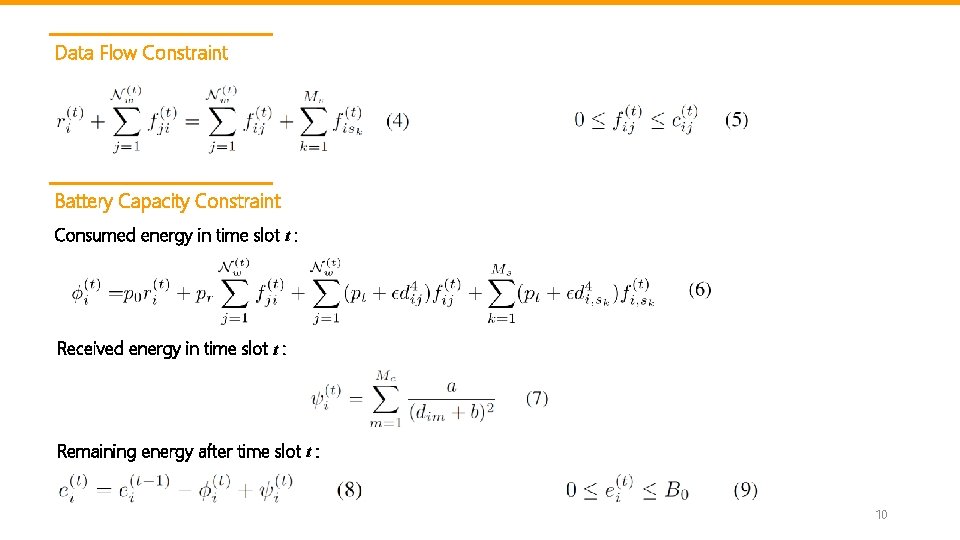
Data Flow Constraint Battery Capacity Constraint Consumed energy in time slot t : Received energy in time slot t : Remaining energy after time slot t : 10
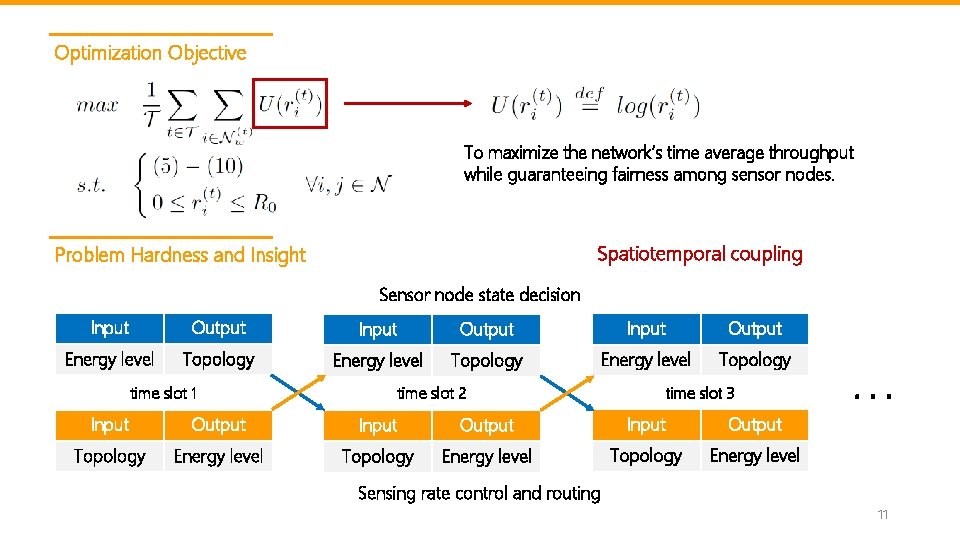
Optimization Objective To maximize the network’s time average throughput while guaranteeing fairness among sensor nodes. Spatiotemporal coupling Problem Hardness and Insight Sensor node state decision Input Output Energy level Topology time slot 1 time slot 2 time slot 3 Input Output Topology Energy level . . . Sensing rate control and routing 11
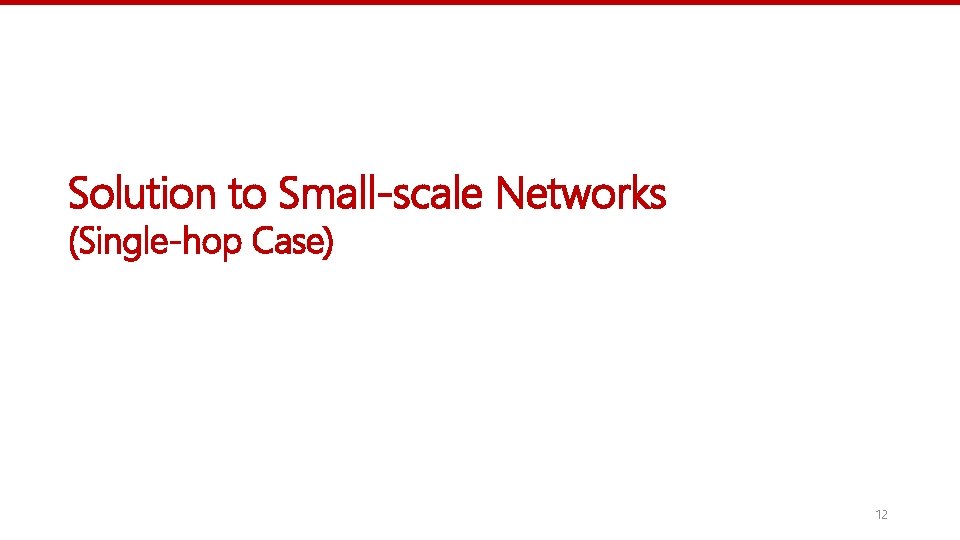
Solution to Small-scale Networks (Single-hop Case) 12
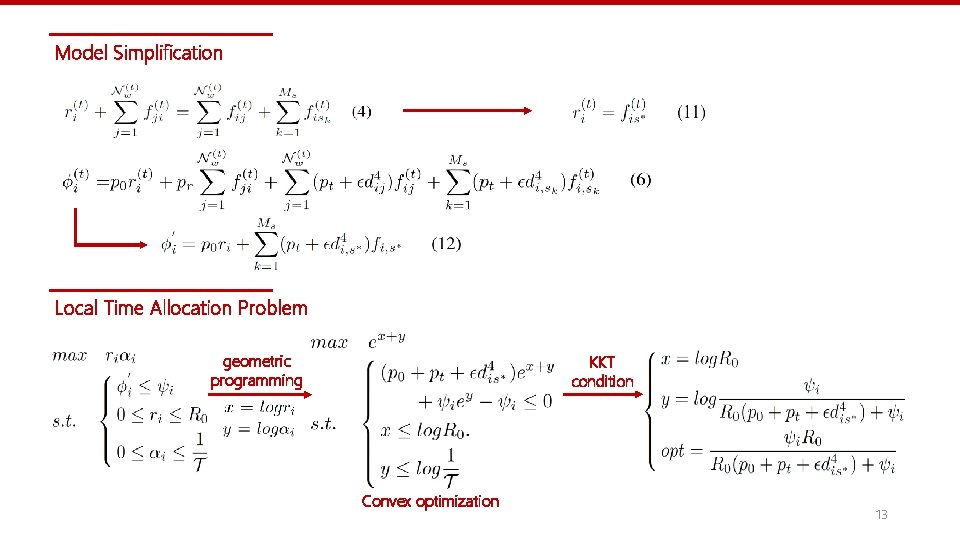
Model Simplification Local Time Allocation Problem geometric programming KKT condition Convex optimization 13
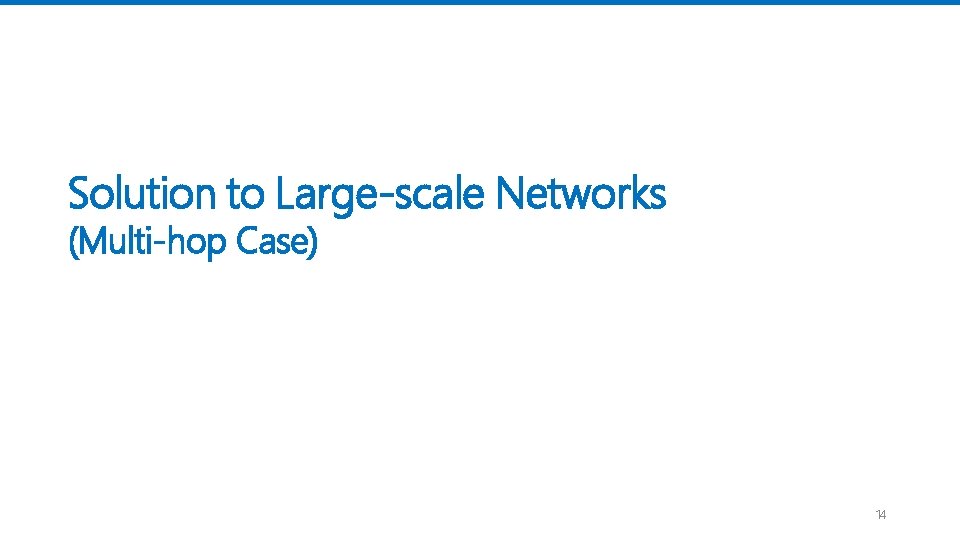
Solution to Large-scale Networks (Multi-hop Case) 14
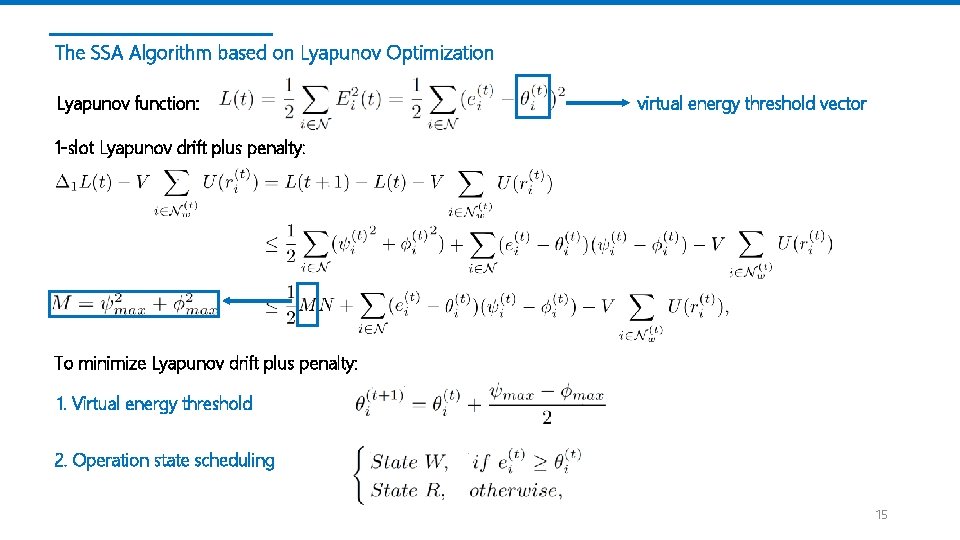
The SSA Algorithm based on Lyapunov Optimization Lyapunov function: virtual energy threshold vector 1 -slot Lyapunov drift plus penalty: To minimize Lyapunov drift plus penalty: 1. Virtual energy threshold 2. Operation state scheduling 15
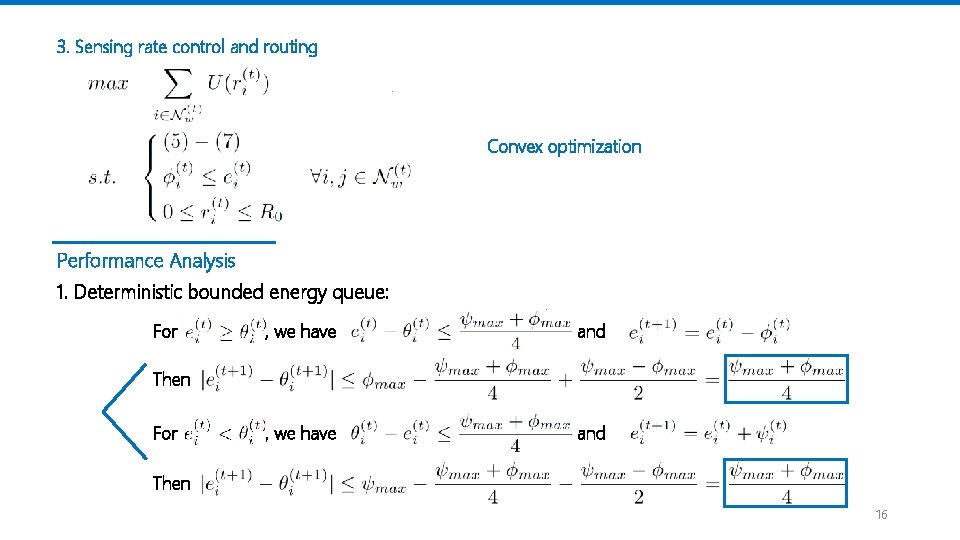
3. Sensing rate control and routing Convex optimization Performance Analysis 1. Deterministic bounded energy queue: For , we have and Then For Then 16
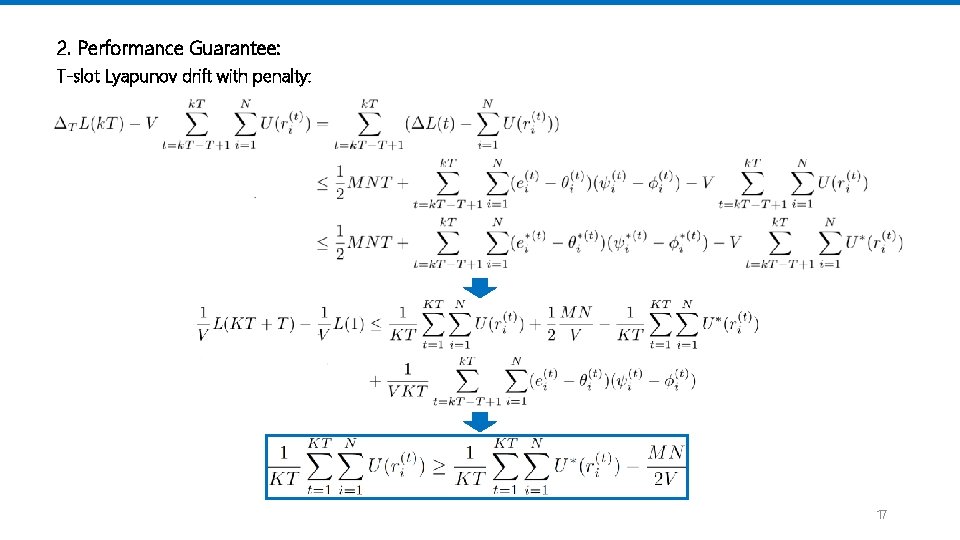
2. Performance Guarantee: T-slot Lyapunov drift with penalty: 17
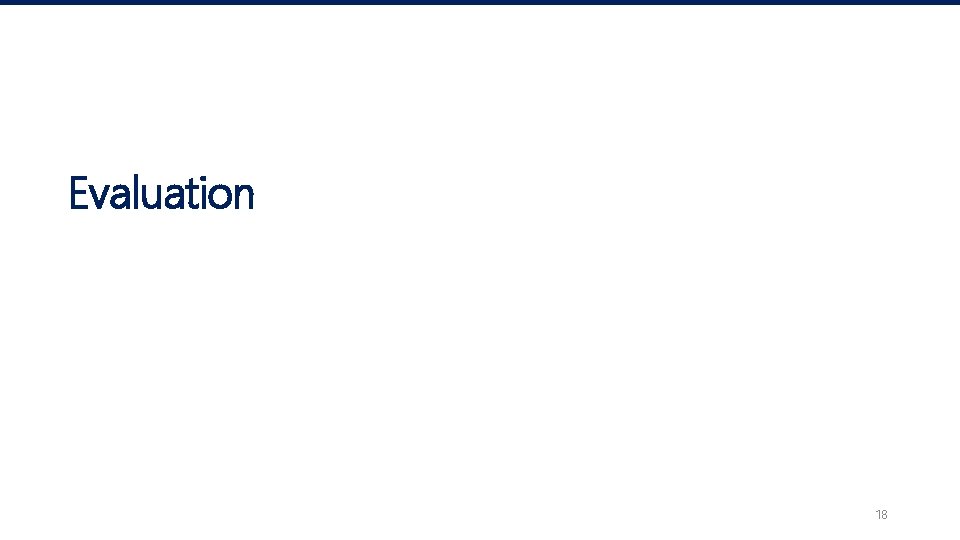
Evaluation 18
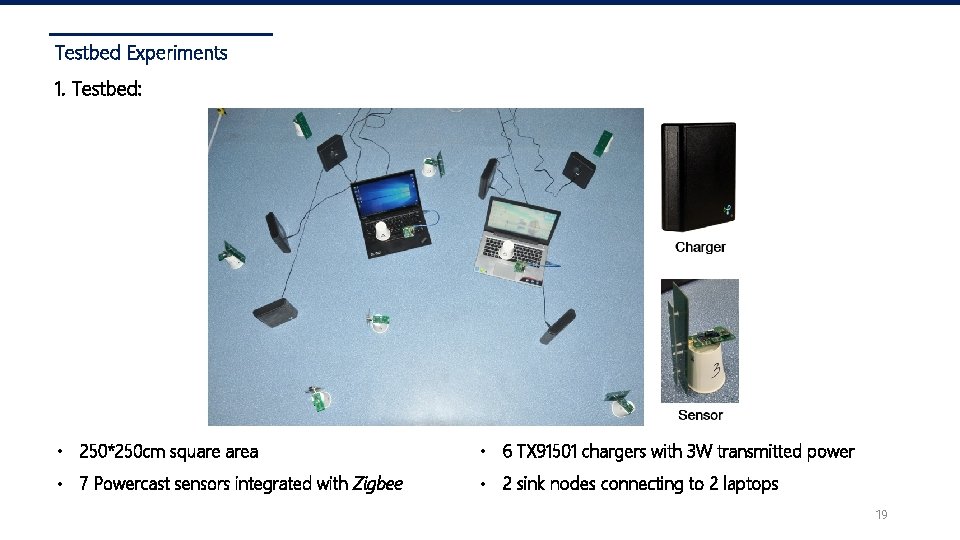
Testbed Experiments 1. Testbed: • 250*250 cm square area • 6 TX 91501 chargers with 3 W transmitted power • 7 Powercast sensors integrated with Zigbee • 2 sink nodes connecting to 2 laptops 19
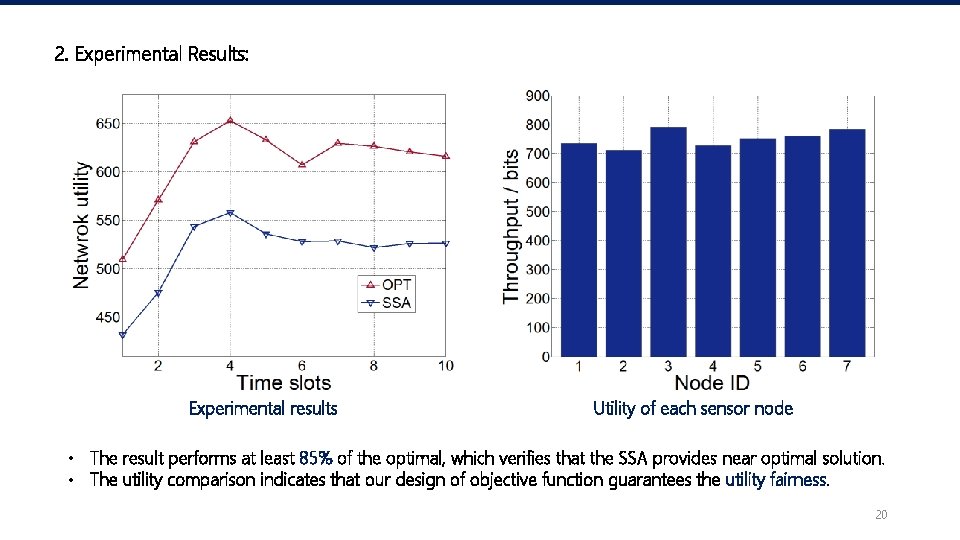
2. Experimental Results: Experimental results Utility of each sensor node • The result performs at least 85% of the optimal, which verifies that the SSA provides near optimal solution. • The utility comparison indicates that our design of objective function guarantees the utility fairness. 20
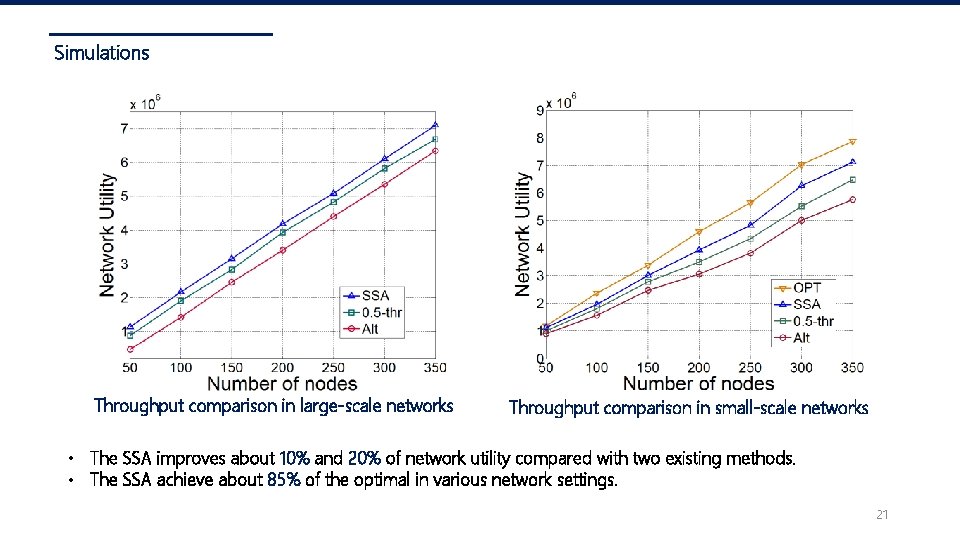
Simulations Throughput comparison in large-scale networks Throughput comparison in small-scale networks • The SSA improves about 10% and 20% of network utility compared with two existing methods. • The SSA achieve about 85% of the optimal in various network settings. 21
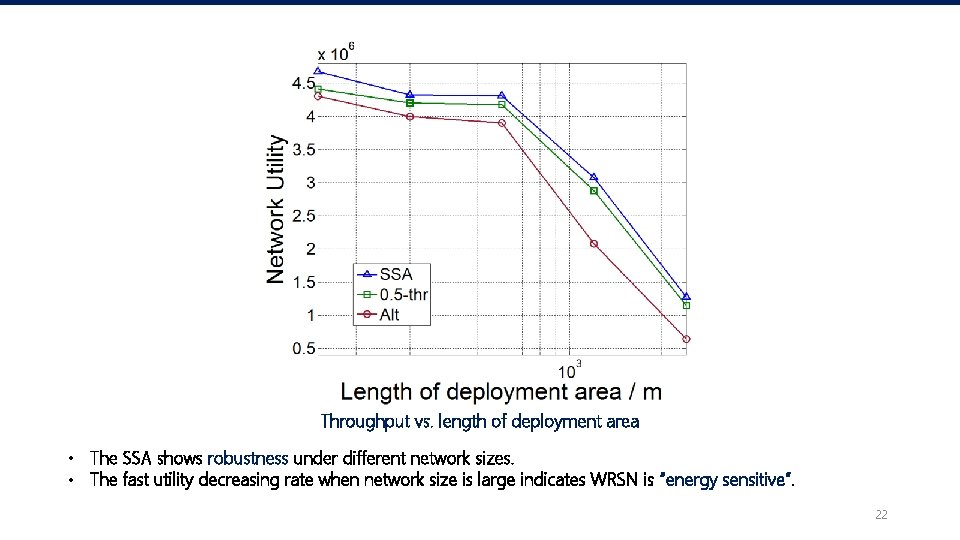
Throughput vs. length of deployment area • The SSA shows robustness under different network sizes. • The fast utility decreasing rate when network size is large indicates WRSN is “energy sensitive”. 22
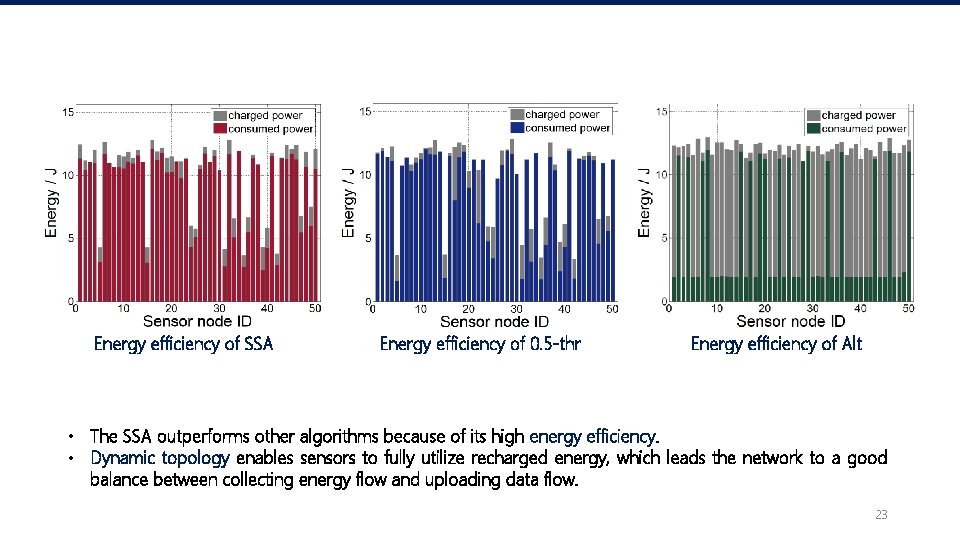
Energy efficiency of SSA Energy efficiency of 0. 5 -thr Energy efficiency of Alt • The SSA outperforms other algorithms because of its high energy efficiency. • Dynamic topology enables sensors to fully utilize recharged energy, which leads the network to a good balance between collecting energy flow and uploading data flow. 23
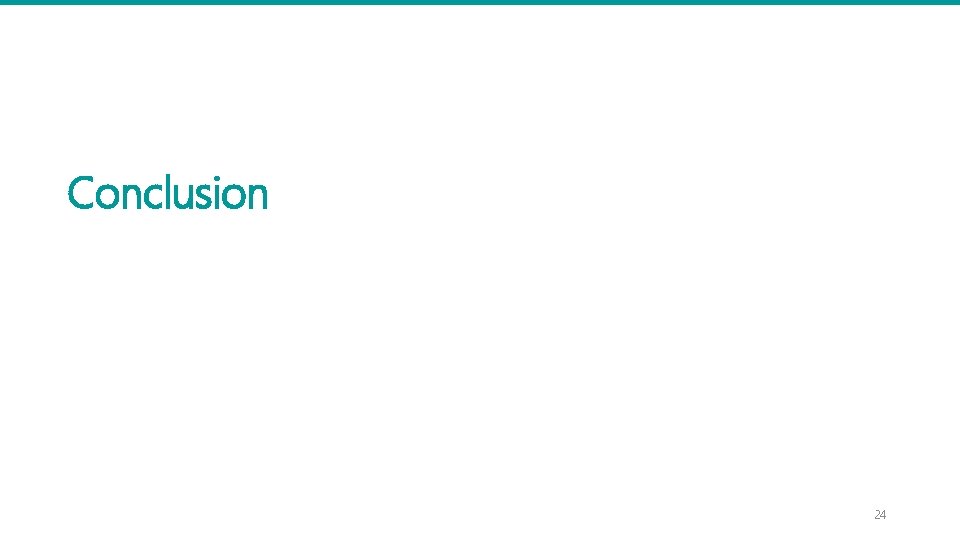
Conclusion 24
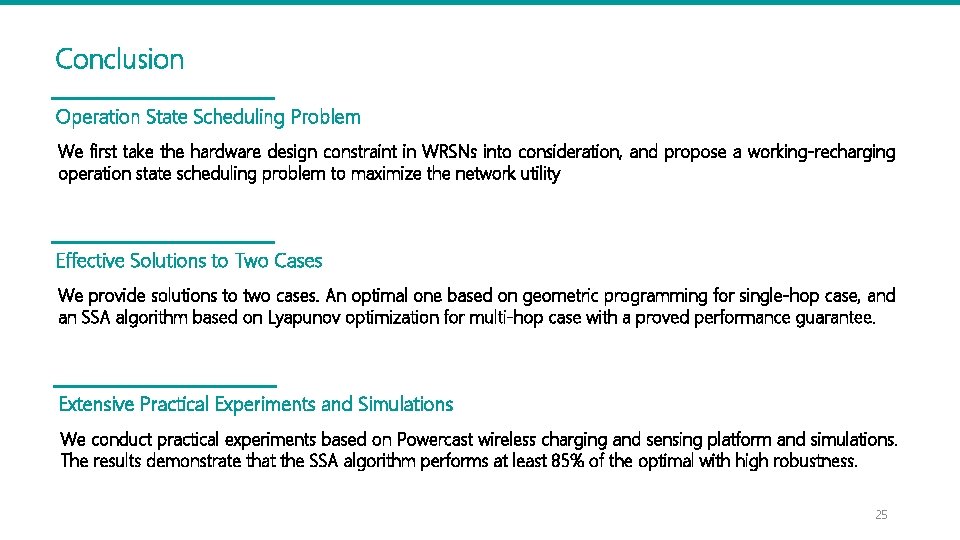
Conclusion Operation State Scheduling Problem We first take the hardware design constraint in WRSNs into consideration, and propose a working-recharging operation state scheduling problem to maximize the network utility Effective Solutions to Two Cases We provide solutions to two cases. An optimal one based on geometric programming for single-hop case, and an SSA algorithm based on Lyapunov optimization for multi-hop case with a proved performance guarantee. Extensive Practical Experiments and Simulations We conduct practical experiments based on Powercast wireless charging and sensing platform and simulations. The results demonstrate that the SSA algorithm performs at least 85% of the optimal with high robustness. 25
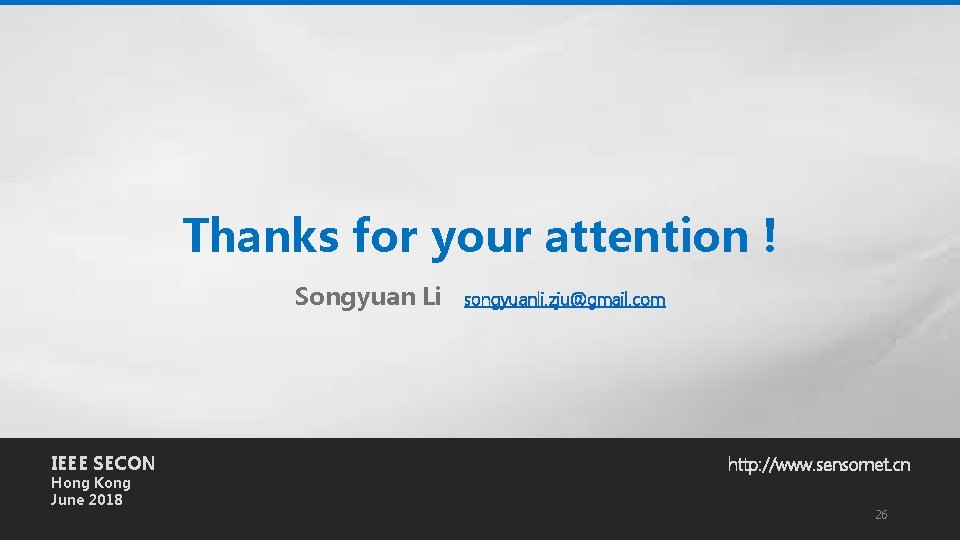
Thanks for your attention ! Songyuan Li IEEE SECON Hong Kong June 2018 songyuanli. zju@gmail. com http: //www. sensornet. cn 26
Job scheduling vs process scheduling
Internet or internet
"state of the internet"
Towards a language-based theory of learning
Compare torvald and nora's attitude towards money
The author's attitude toward the subject
Together towards improvement
Sand: towards high-performance serverless computing
Hrd strategy towards 2030
Light bending towards the normal
Divergent boundary
Feelings of hatred towards somebody
Towards deep conversational recommendations
How have attitudes towards immigrants changed over time?
Towards a theory of transcultural fandom
Sacrifice character trait
Plates that move towards each other
Towards independence commerce program
Is the author's attitude towards the subject
Why do the gamemakers dry-up the river and ponds
The path of a point moving through a space.
Free body diagram egg drop
O thou who art turning thy face towards god
Ethnocentric attitude
Rethinking education: towards a global common good?
Mechanical approach towards personnel
Expository and exploratory approach