The HWRF No Vortex Initialization HNVI realtime experiment
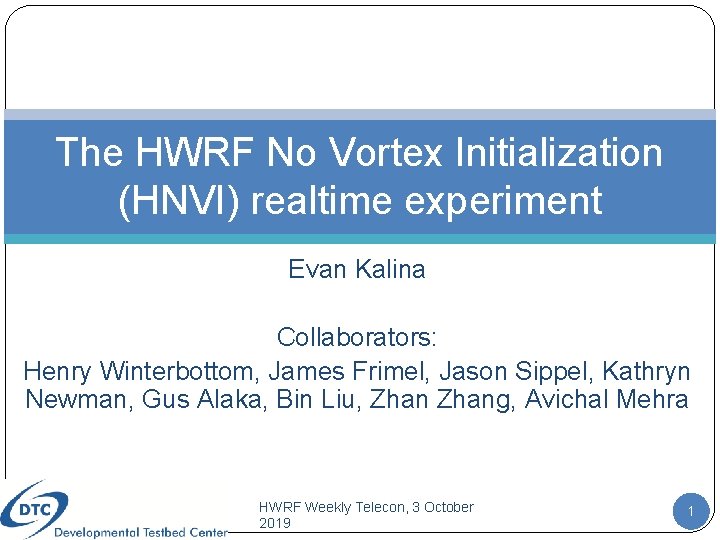
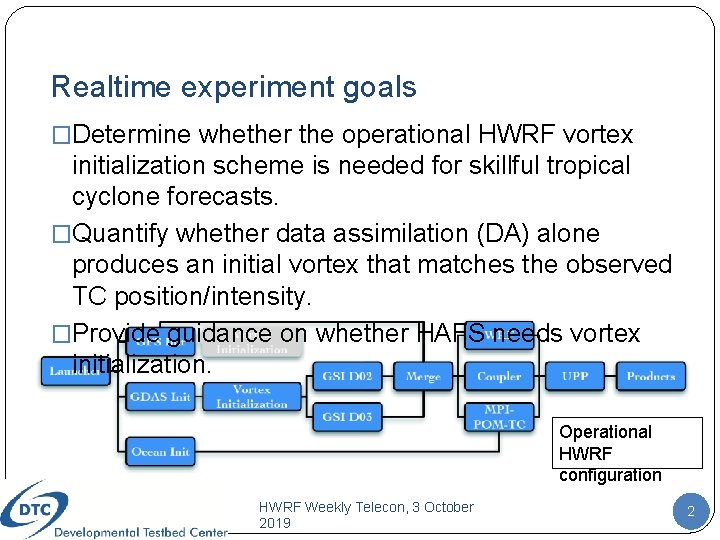
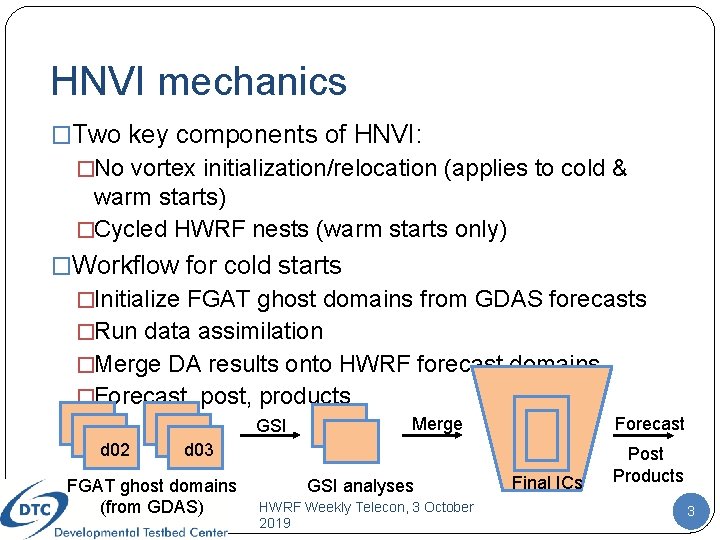
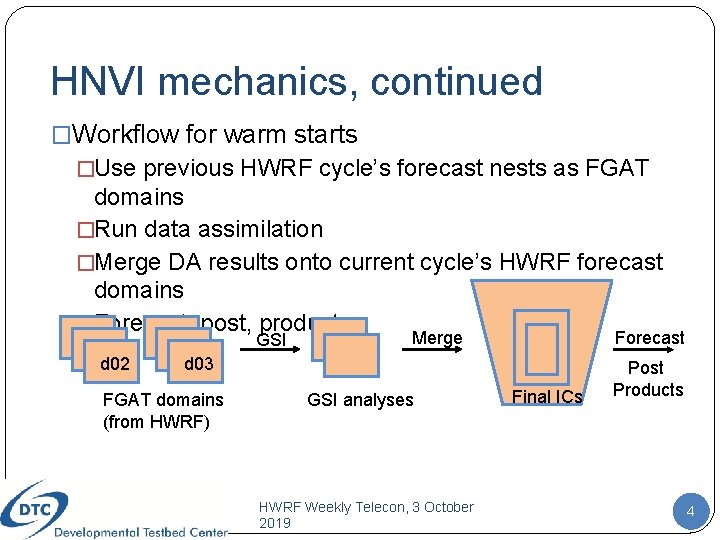
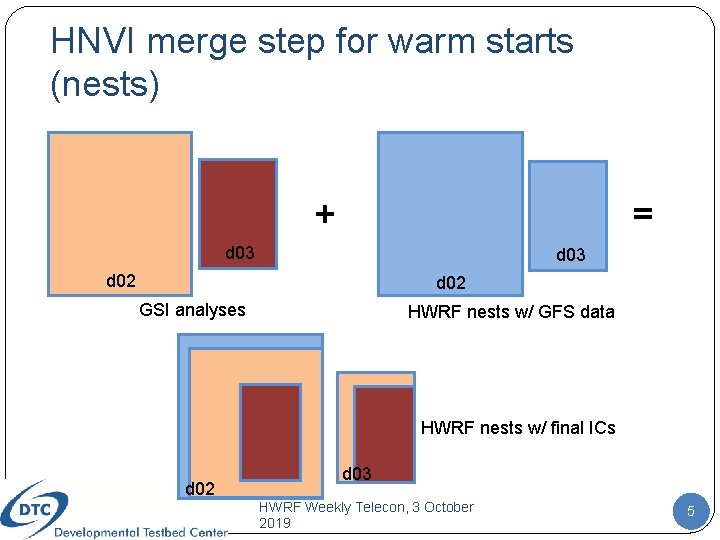
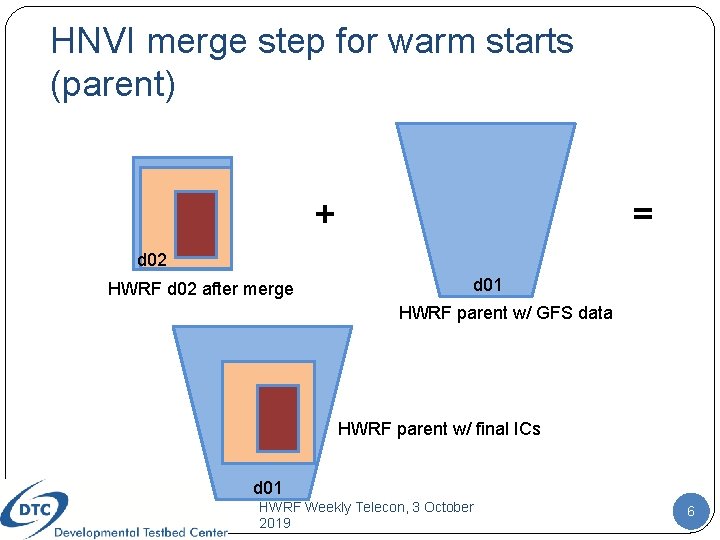
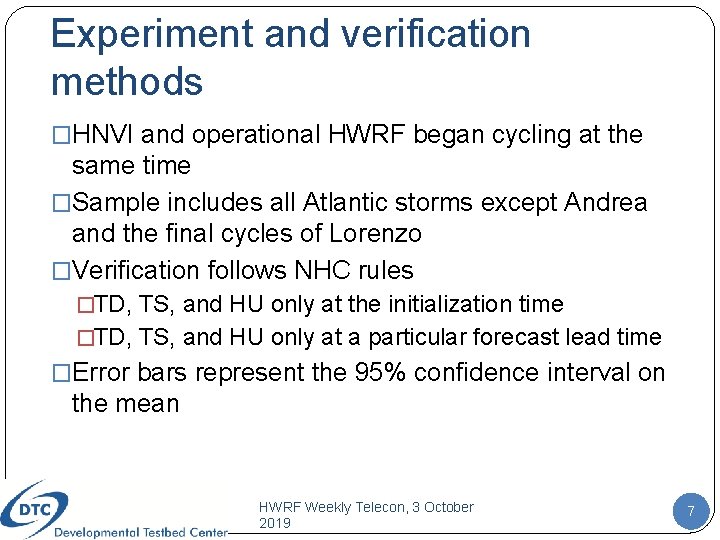
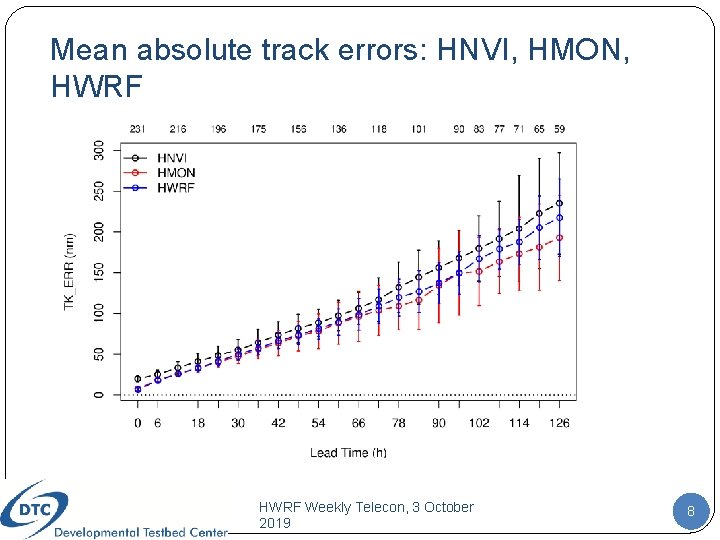
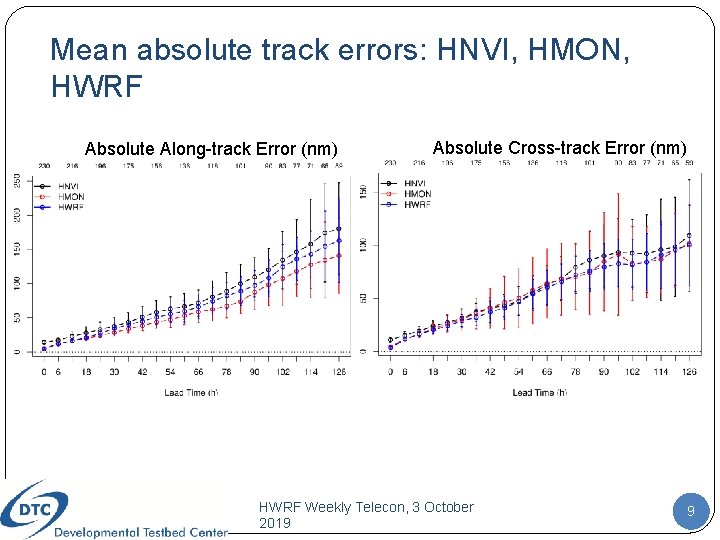
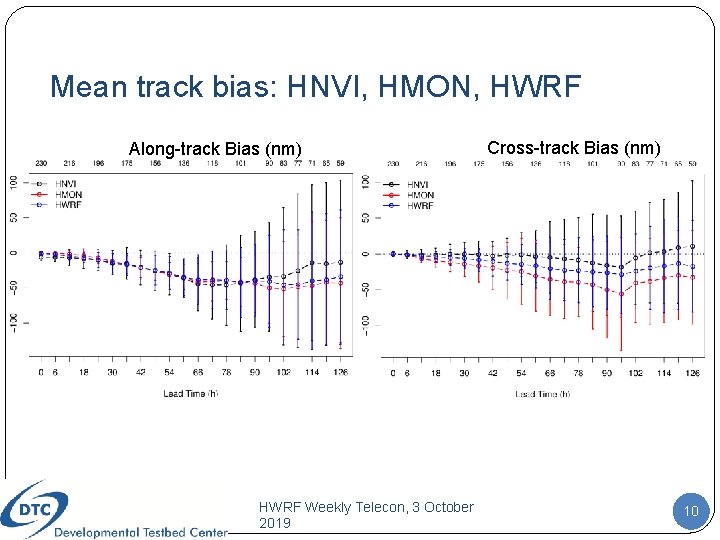
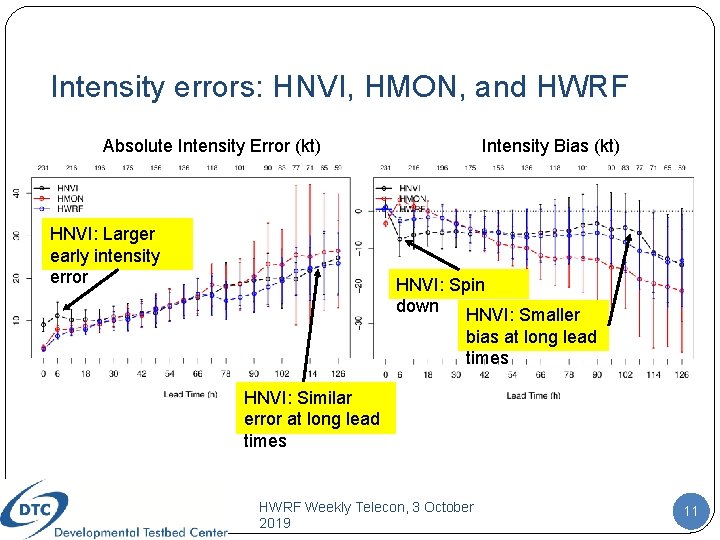
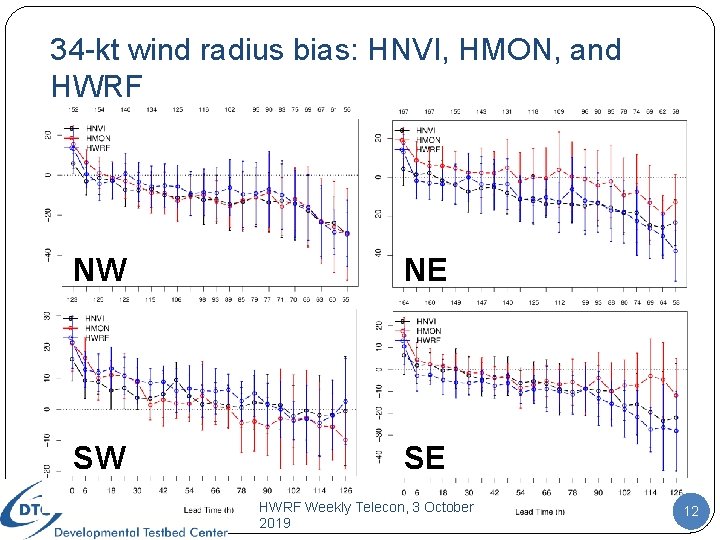
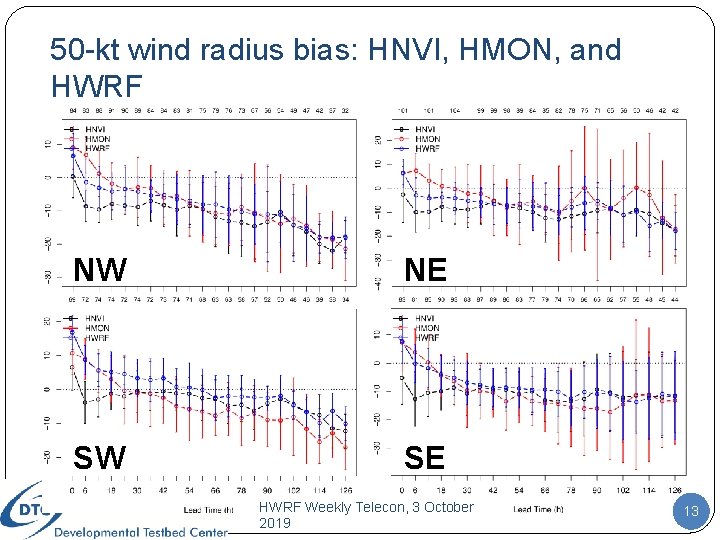
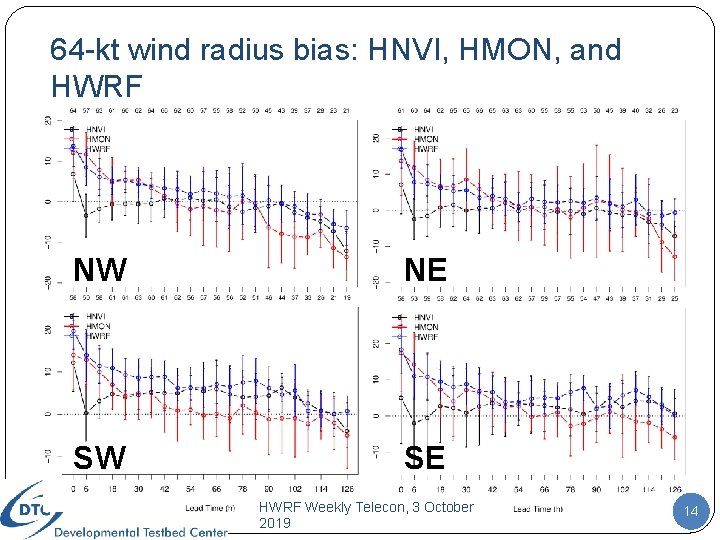
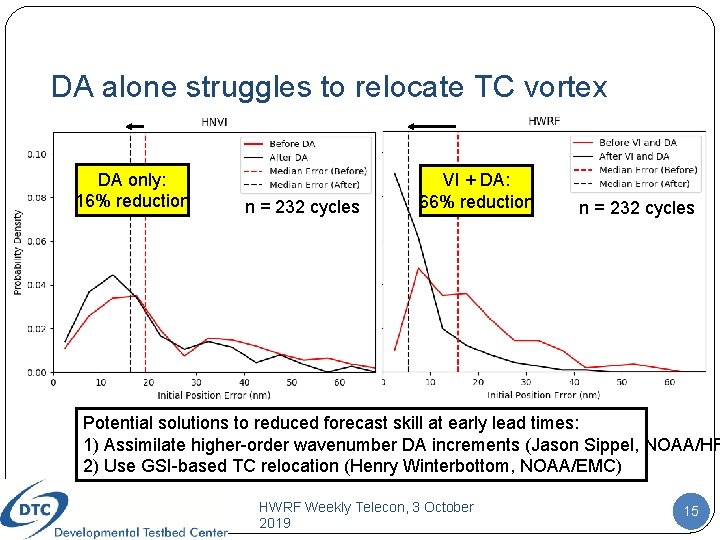
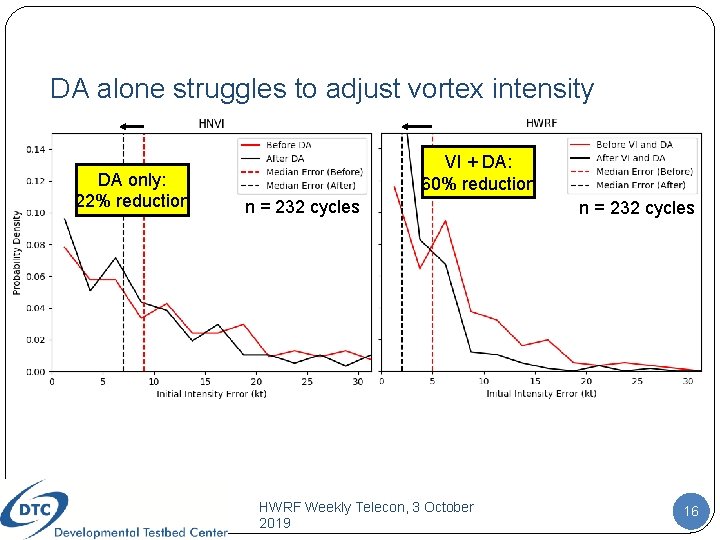
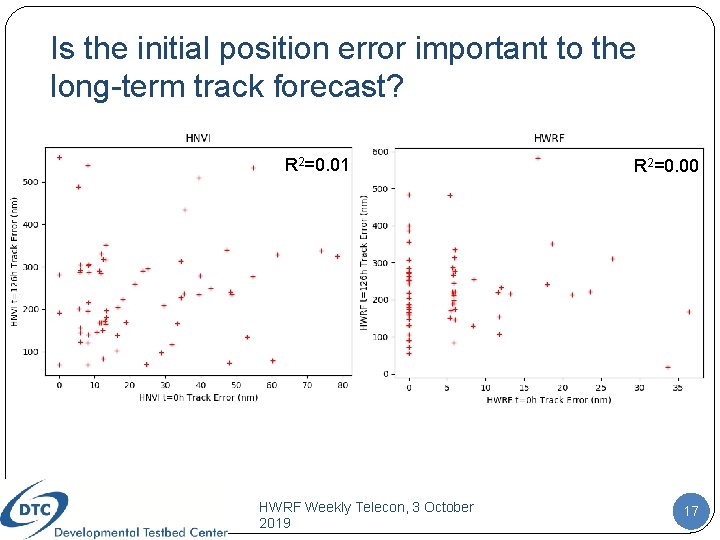
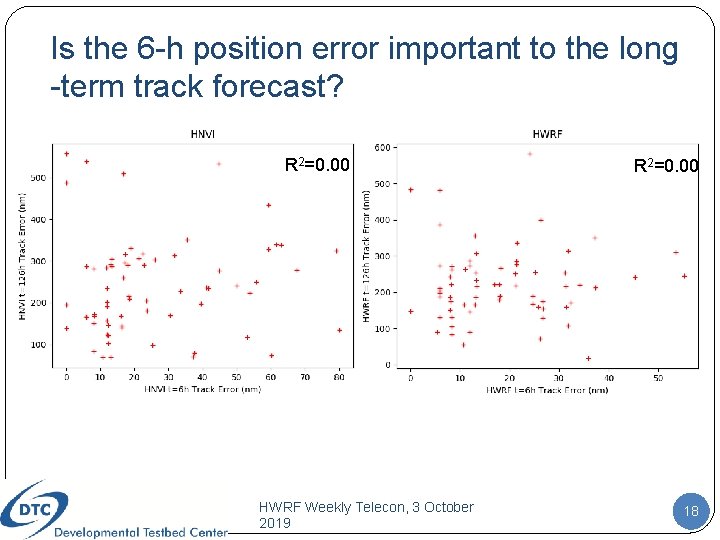
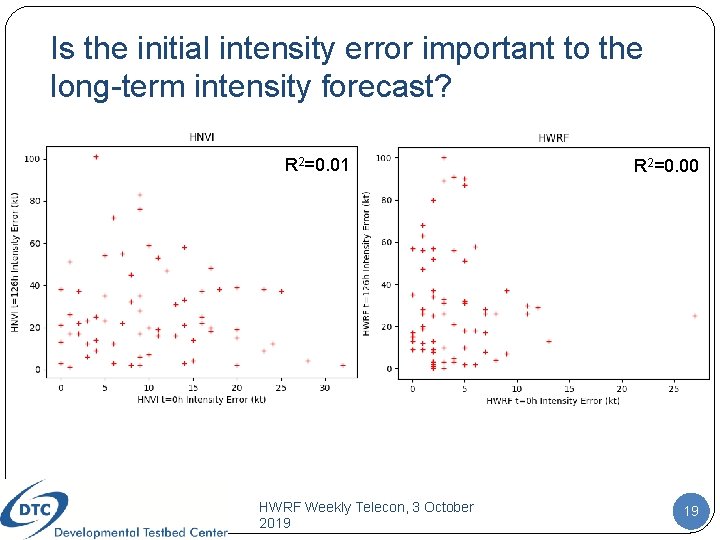
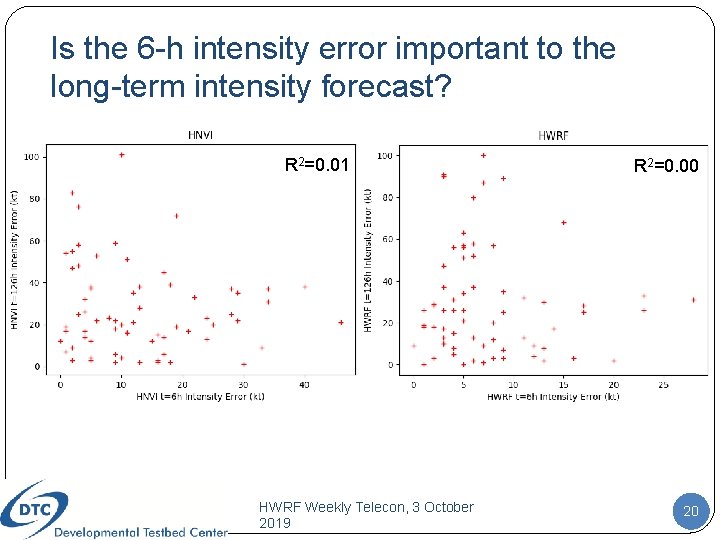
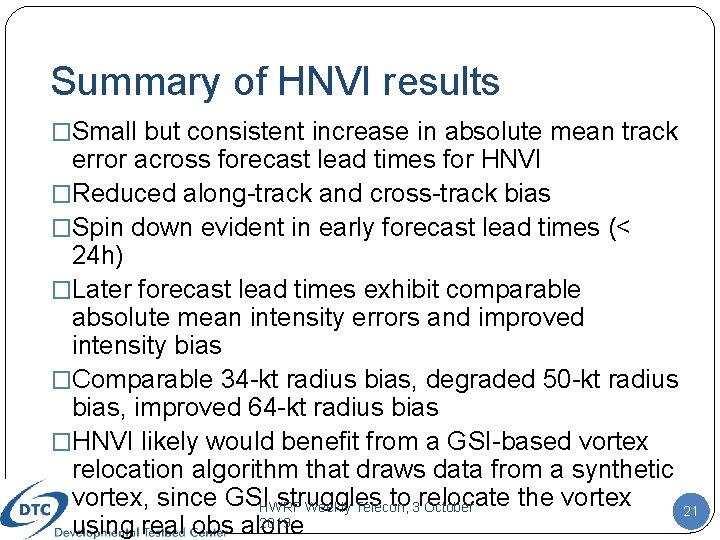
- Slides: 21
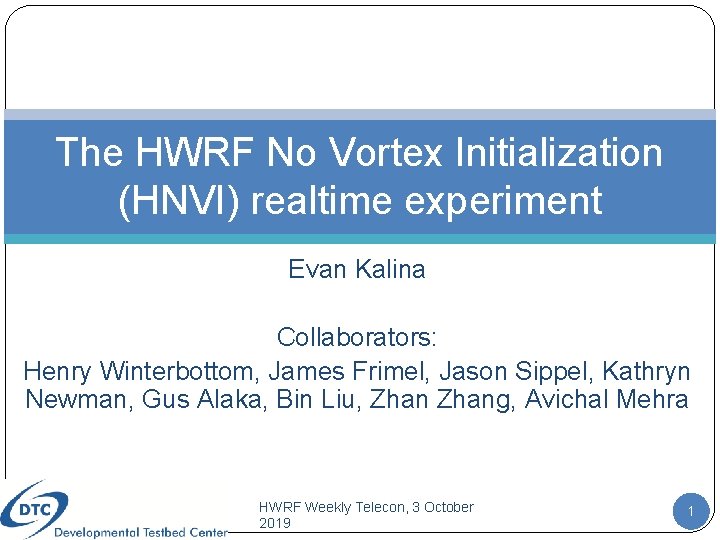
The HWRF No Vortex Initialization (HNVI) realtime experiment Evan Kalina Collaborators: Henry Winterbottom, James Frimel, Jason Sippel, Kathryn Newman, Gus Alaka, Bin Liu, Zhang, Avichal Mehra HWRF Weekly Telecon, 3 October 2019 1
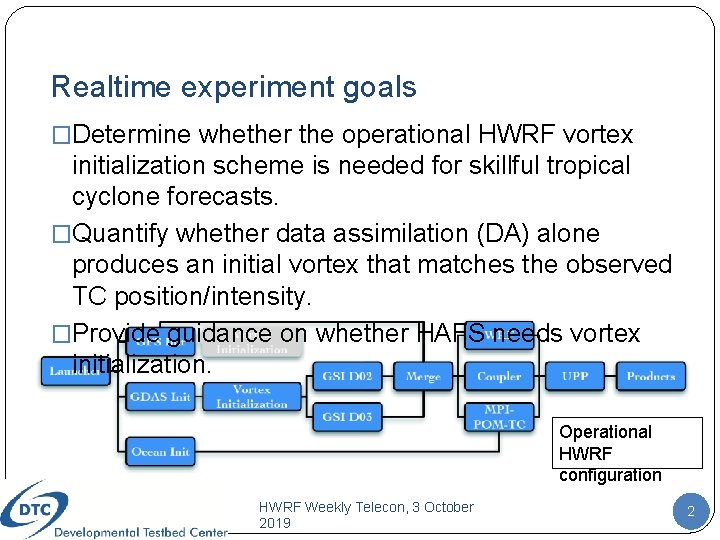
Realtime experiment goals �Determine whether the operational HWRF vortex initialization scheme is needed for skillful tropical cyclone forecasts. �Quantify whether data assimilation (DA) alone produces an initial vortex that matches the observed TC position/intensity. �Provide guidance on whether HAFS needs vortex initialization. Operational HWRF configuration HWRF Weekly Telecon, 3 October 2019 2
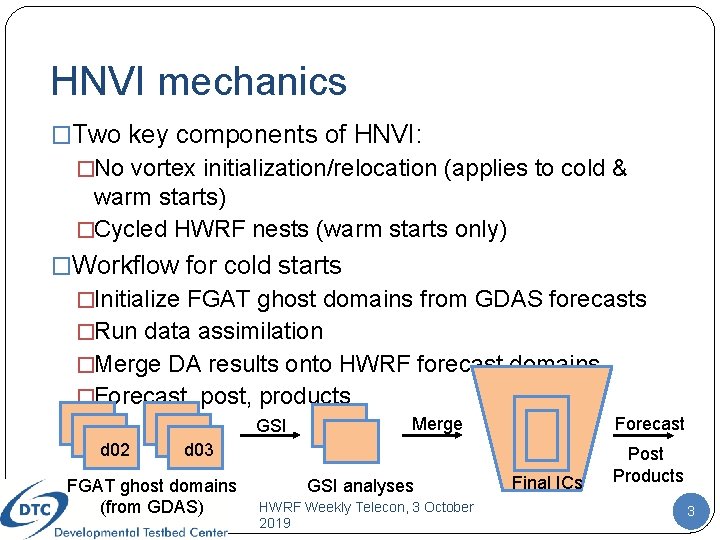
HNVI mechanics �Two key components of HNVI: �No vortex initialization/relocation (applies to cold & warm starts) �Cycled HWRF nests (warm starts only) �Workflow for cold starts �Initialize FGAT ghost domains from GDAS forecasts �Run data assimilation �Merge DA results onto HWRF forecast domains �Forecast, post, products GSI d 02 Merge Forecast d 03 FGAT ghost domains (from GDAS) GSI analyses HWRF Weekly Telecon, 3 October 2019 Final ICs Post Products 3
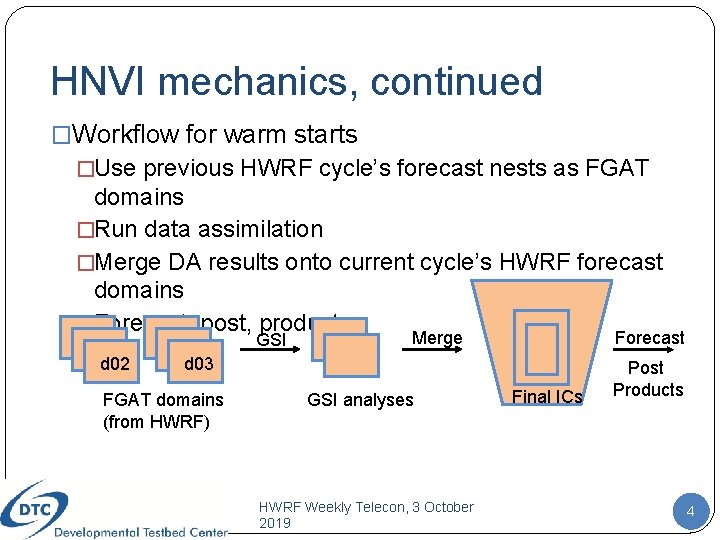
HNVI mechanics, continued �Workflow for warm starts �Use previous HWRF cycle’s forecast nests as FGAT domains �Run data assimilation �Merge DA results onto current cycle’s HWRF forecast domains �Forecast, post, products GSI d 02 Merge Forecast d 03 FGAT domains (from HWRF) GSI analyses HWRF Weekly Telecon, 3 October 2019 Final ICs Post Products 4
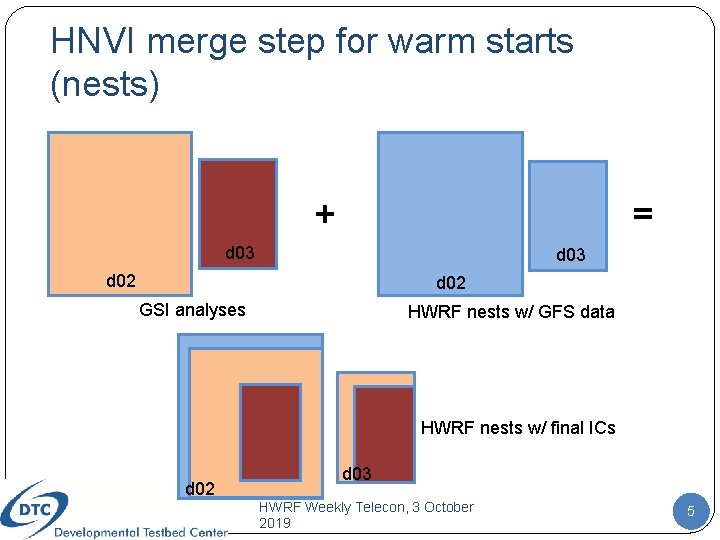
HNVI merge step for warm starts (nests) + = d 03 d 02 GSI analyses HWRF nests w/ GFS data HWRF nests w/ final ICs d 02 d 03 HWRF Weekly Telecon, 3 October 2019 5
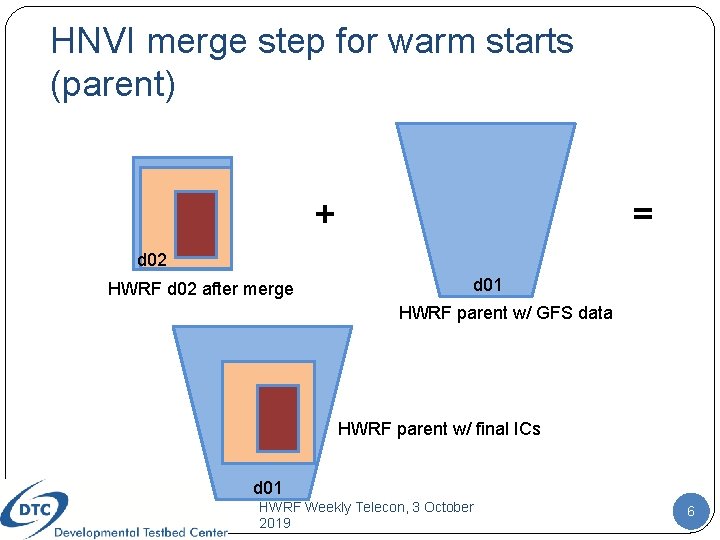
HNVI merge step for warm starts (parent) + = d 02 HWRF d 02 after merge d 01 HWRF parent w/ GFS data HWRF parent w/ final ICs d 01 HWRF Weekly Telecon, 3 October 2019 6
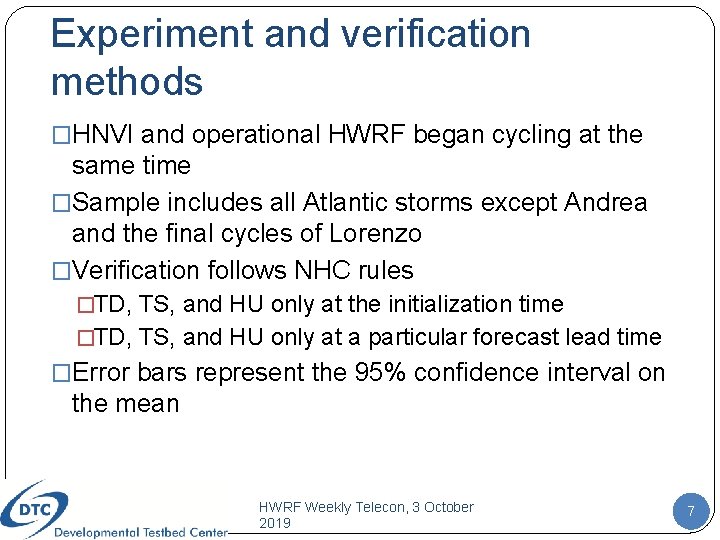
Experiment and verification methods �HNVI and operational HWRF began cycling at the same time �Sample includes all Atlantic storms except Andrea and the final cycles of Lorenzo �Verification follows NHC rules �TD, TS, and HU only at the initialization time �TD, TS, and HU only at a particular forecast lead time �Error bars represent the 95% confidence interval on the mean HWRF Weekly Telecon, 3 October 2019 7
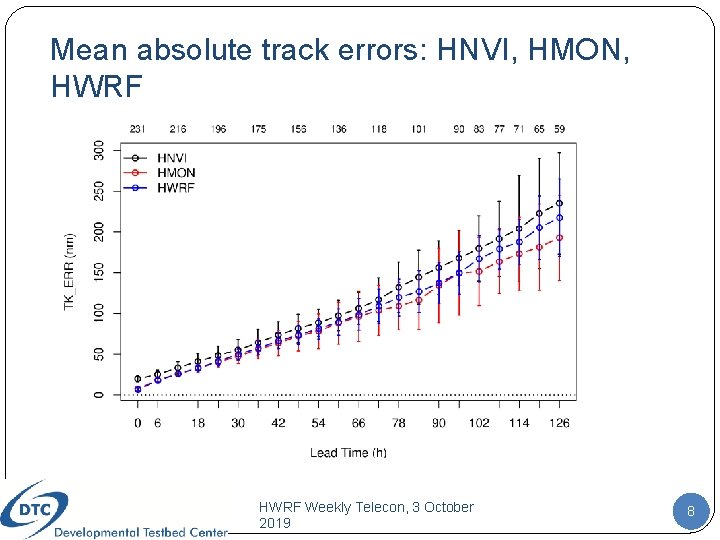
Mean absolute track errors: HNVI, HMON, HWRF Weekly Telecon, 3 October 2019 8
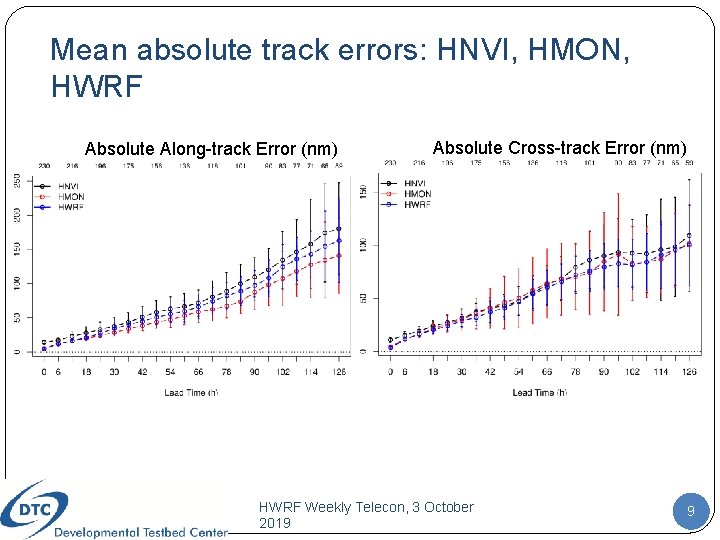
Mean absolute track errors: HNVI, HMON, HWRF Absolute Along-track Error (nm) Absolute Cross-track Error (nm) HWRF Weekly Telecon, 3 October 2019 9
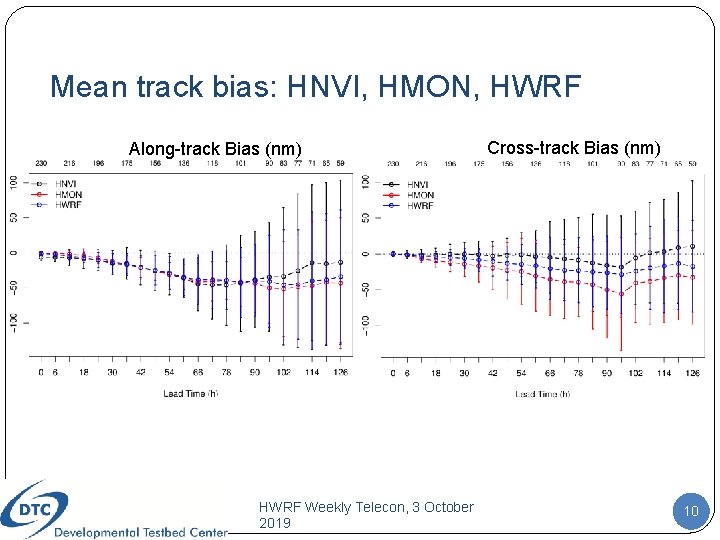
Mean track bias: HNVI, HMON, HWRF Along-track Bias (nm) HWRF Weekly Telecon, 3 October 2019 Cross-track Bias (nm) 10
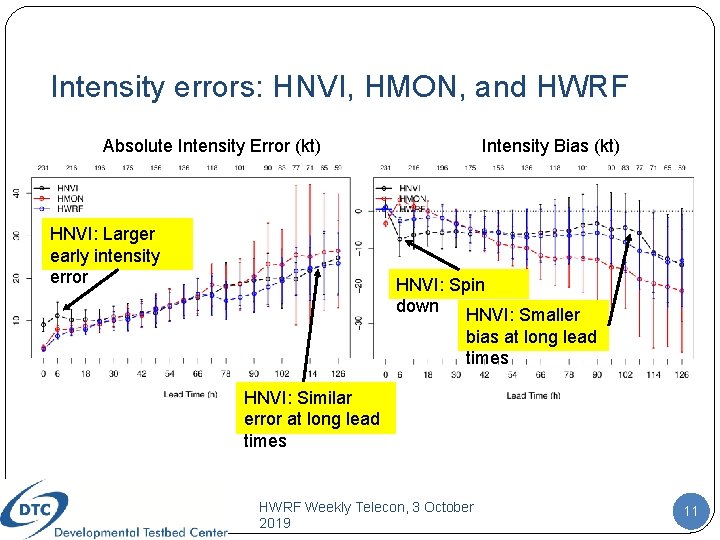
Intensity errors: HNVI, HMON, and HWRF Absolute Intensity Error (kt) HNVI: Larger early intensity error Intensity Bias (kt) HNVI: Spin down HNVI: Smaller bias at long lead times HNVI: Similar error at long lead times HWRF Weekly Telecon, 3 October 2019 11
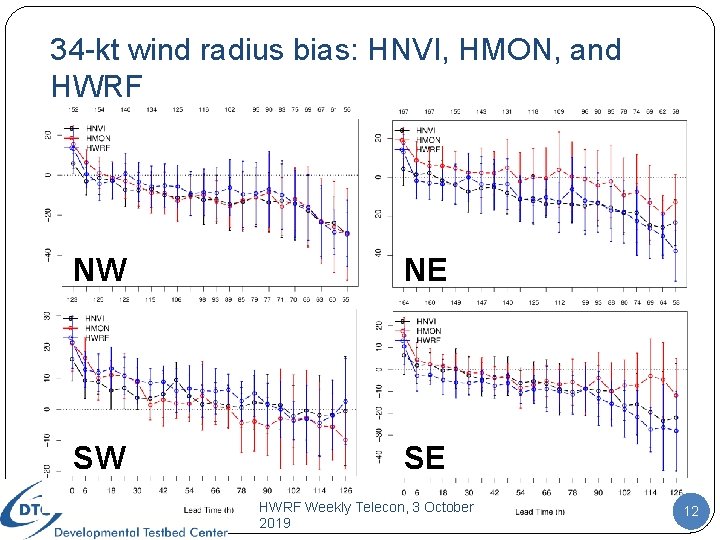
34 -kt wind radius bias: HNVI, HMON, and HWRF NW NE SW SE HWRF Weekly Telecon, 3 October 2019 12
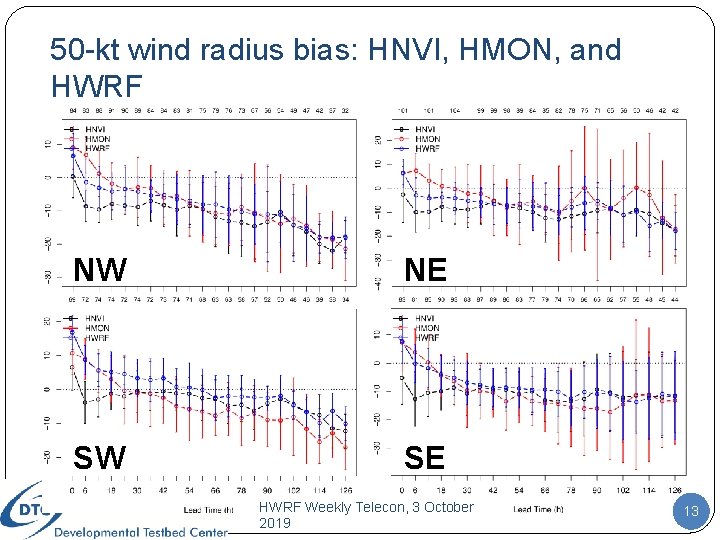
50 -kt wind radius bias: HNVI, HMON, and HWRF NW NE SW SE HWRF Weekly Telecon, 3 October 2019 13
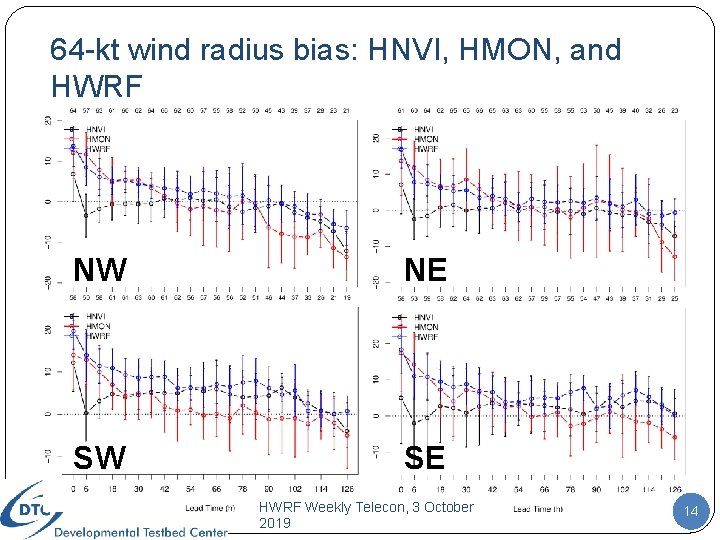
64 -kt wind radius bias: HNVI, HMON, and HWRF NW NE SW SE HWRF Weekly Telecon, 3 October 2019 14
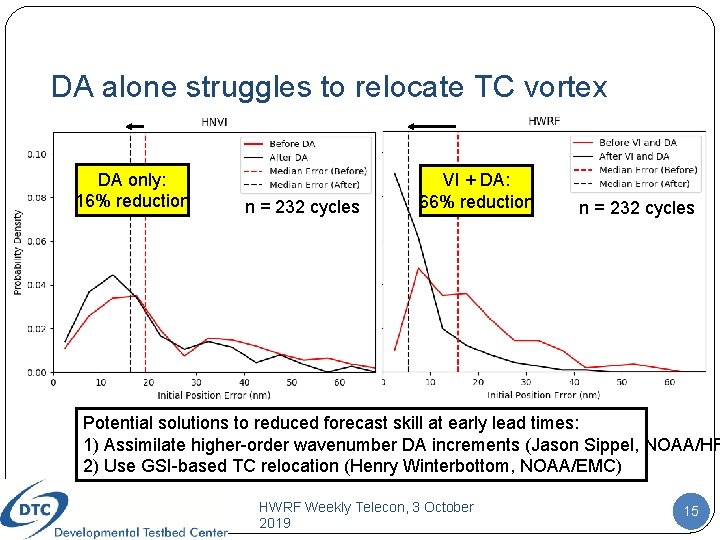
DA alone struggles to relocate TC vortex DA only: 16% reduction n = 232 cycles VI + DA: 66% reduction n = 232 cycles Potential solutions to reduced forecast skill at early lead times: 1) Assimilate higher-order wavenumber DA increments (Jason Sippel, NOAA/HR 2) Use GSI-based TC relocation (Henry Winterbottom, NOAA/EMC) HWRF Weekly Telecon, 3 October 2019 15
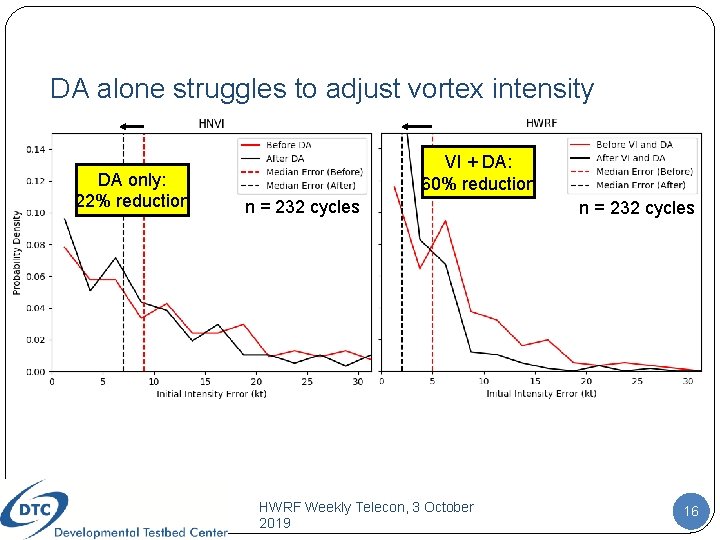
DA alone struggles to adjust vortex intensity DA only: 22% reduction VI + DA: 60% reduction n = 232 cycles HWRF Weekly Telecon, 3 October 2019 n = 232 cycles 16
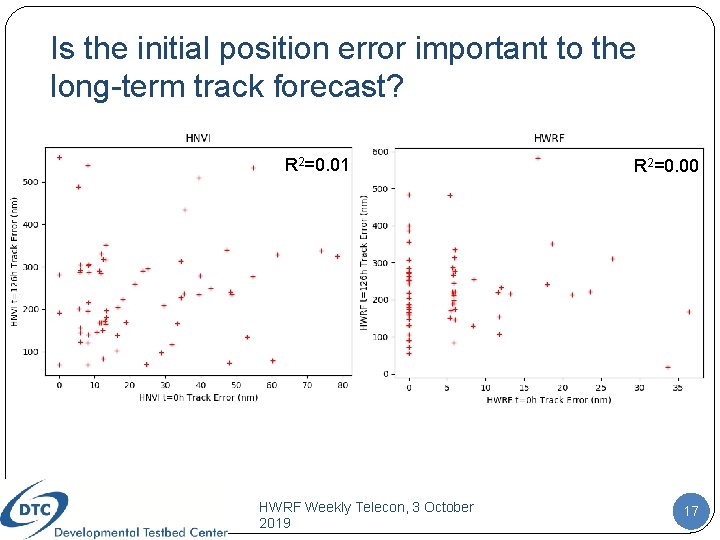
Is the initial position error important to the long-term track forecast? R 2=0. 01 HWRF Weekly Telecon, 3 October 2019 R 2=0. 00 17
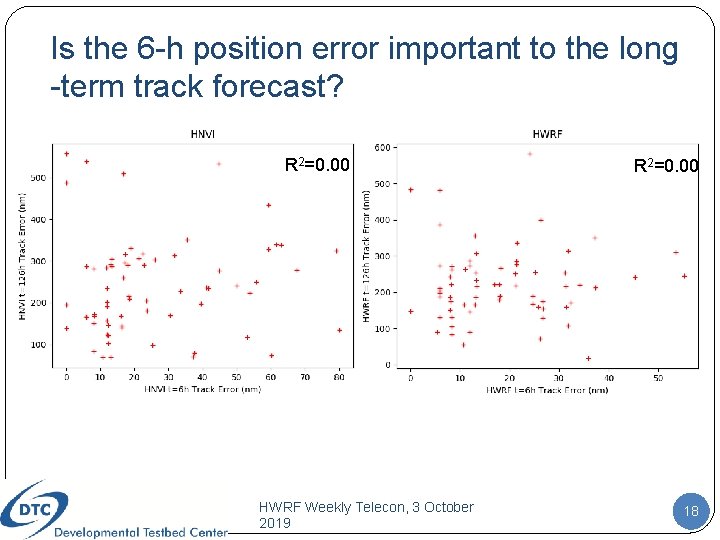
Is the 6 -h position error important to the long -term track forecast? R 2=0. 00 HWRF Weekly Telecon, 3 October 2019 R 2=0. 00 18
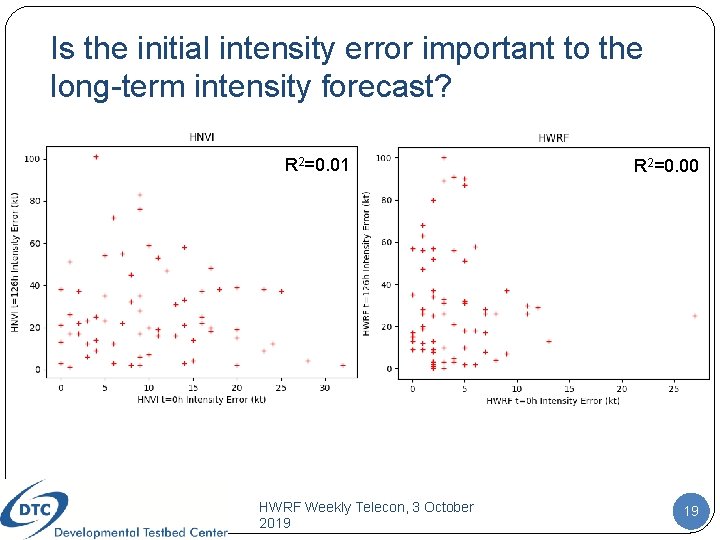
Is the initial intensity error important to the long-term intensity forecast? R 2=0. 01 HWRF Weekly Telecon, 3 October 2019 R 2=0. 00 19
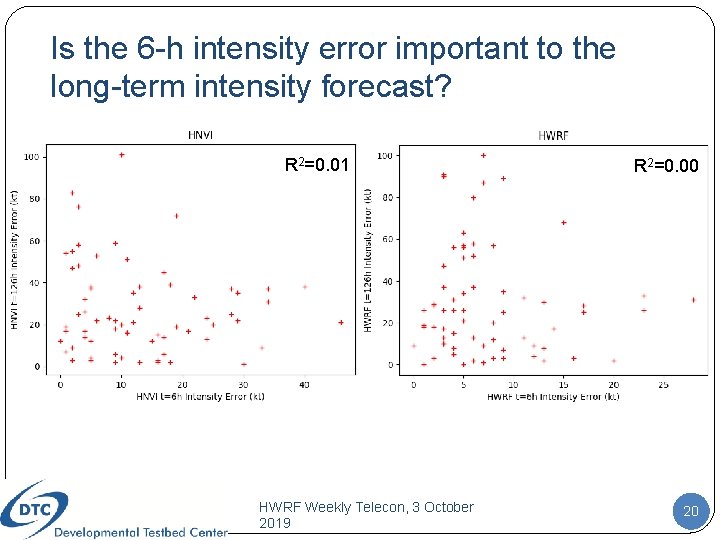
Is the 6 -h intensity error important to the long-term intensity forecast? R 2=0. 01 HWRF Weekly Telecon, 3 October 2019 R 2=0. 00 20
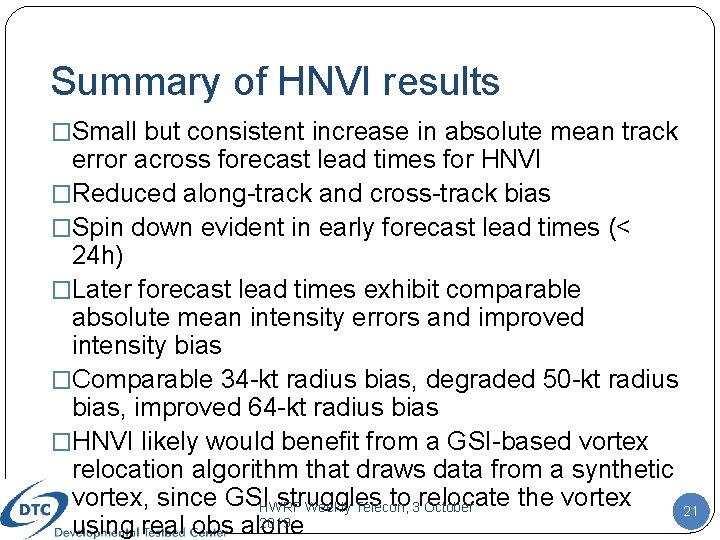
Summary of HNVI results �Small but consistent increase in absolute mean track error across forecast lead times for HNVI �Reduced along-track and cross-track bias �Spin down evident in early forecast lead times (< 24 h) �Later forecast lead times exhibit comparable absolute mean intensity errors and improved intensity bias �Comparable 34 -kt radius bias, degraded 50 -kt radius bias, improved 64 -kt radius bias �HNVI likely would benefit from a GSI-based vortex relocation algorithm that draws data from a synthetic vortex, since GSIHWRF struggles to 3 relocate the vortex Weekly Telecon, October 21 2019 using real obs alone
C++ struct partial initialization
C# multidimensional array initialization
Systemverilog multidimensional array initialization
Initialization dan increase pada penggunaan for bersifat
Matlab array initialize
Ymir character initialization
Initialization
Is array a structured data type
Bios initialization
C++ string initialization
Descartes vortex theory
Vortex keratopathy drugs
Horizontal component of lift
Vortex flow in torque converter
Cicoplan
Atmospheric vortex engine
Szlivka ferenc
Toroidal vortex cannon
Hairpin vortex
Vortex port for plasmapheresis
Karman vortex maf sensor
Translation in fluids