Silver Medallion CRI Poster Review W Ed Hammond
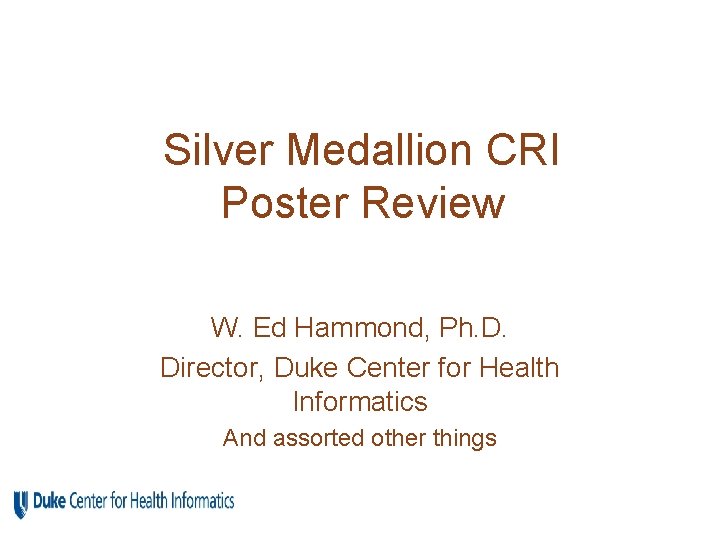
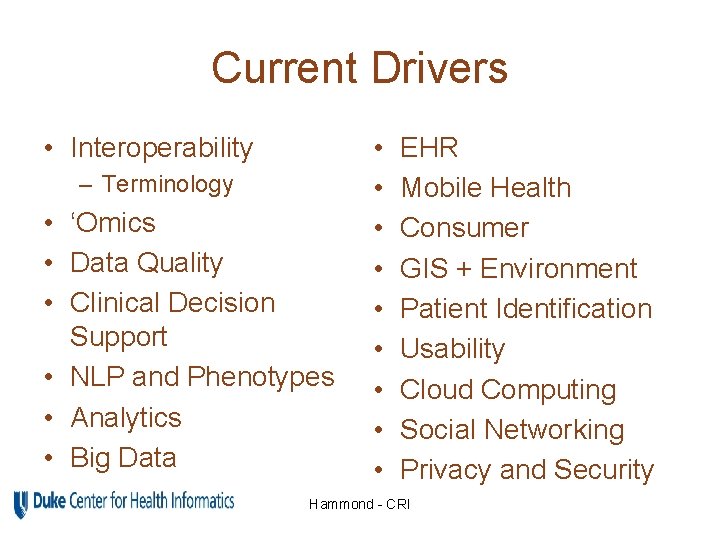
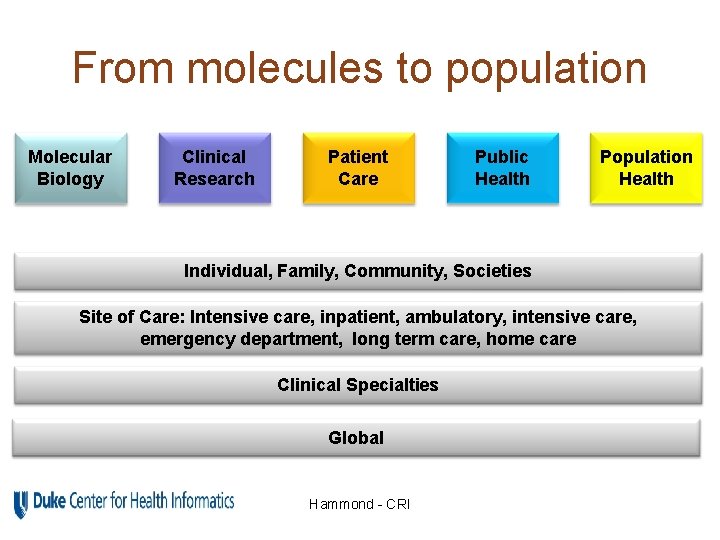
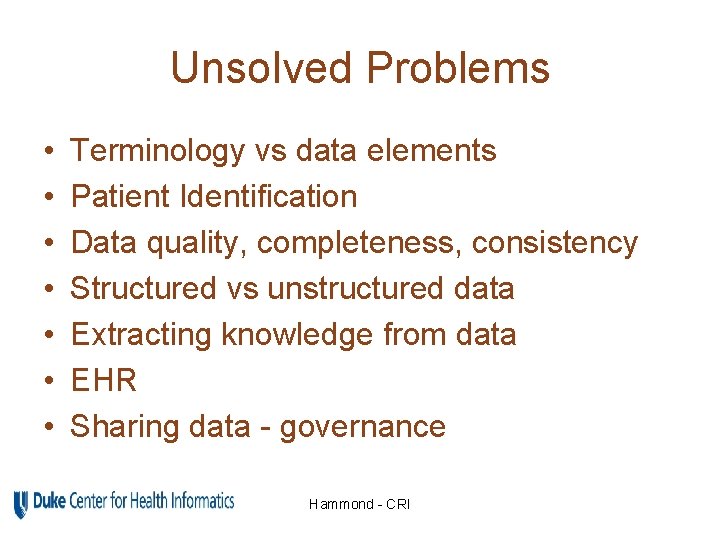
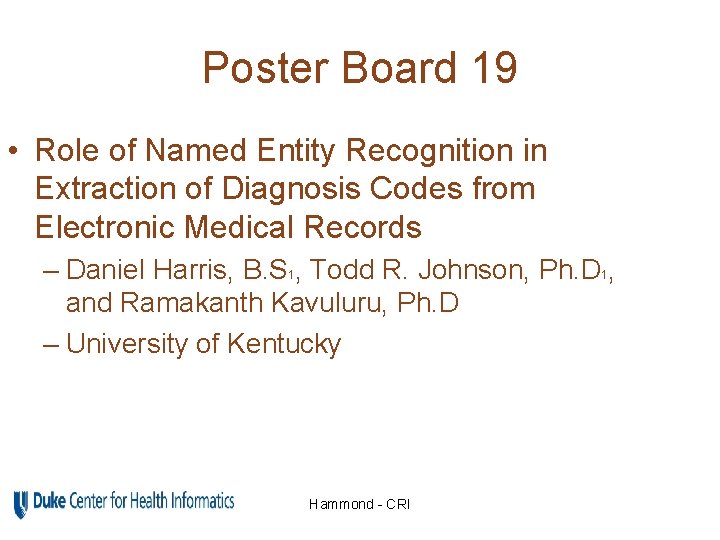
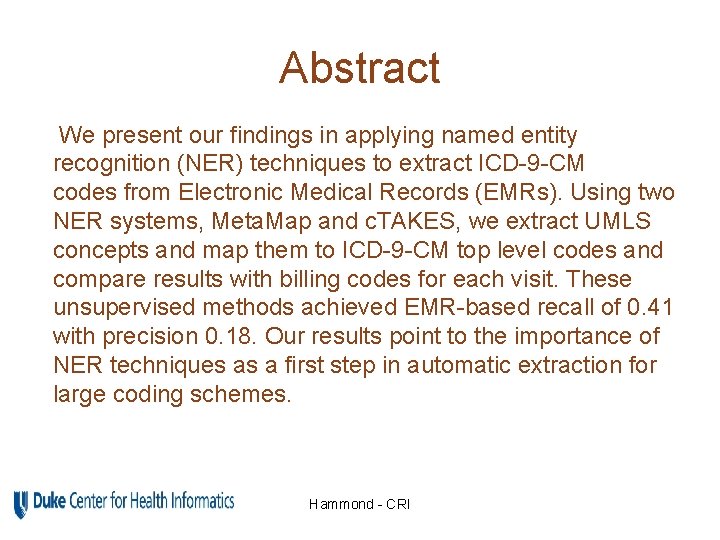
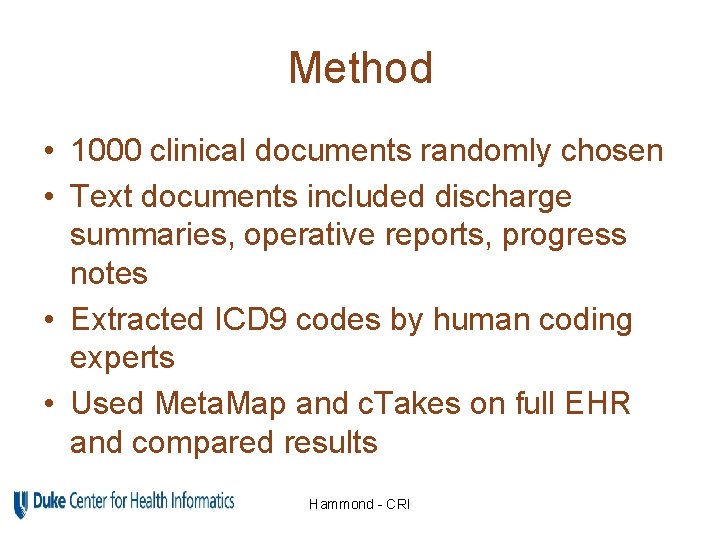
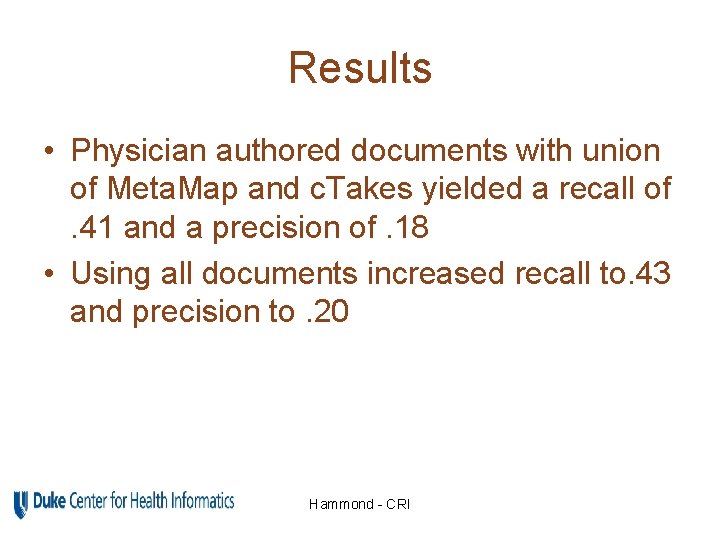
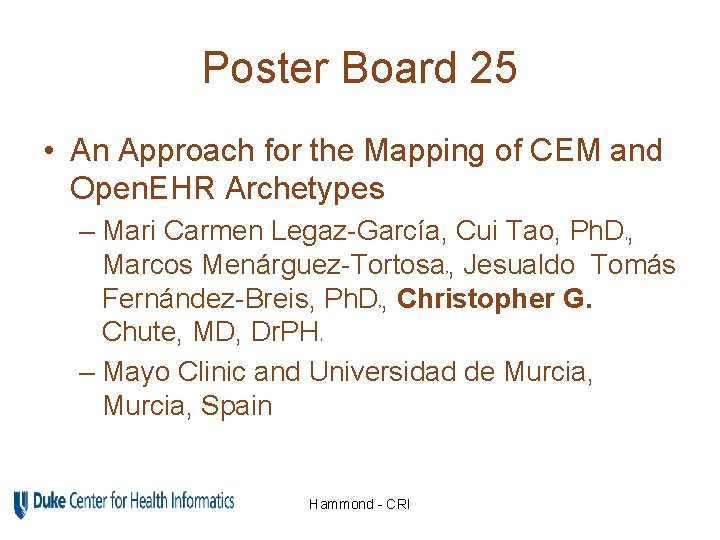
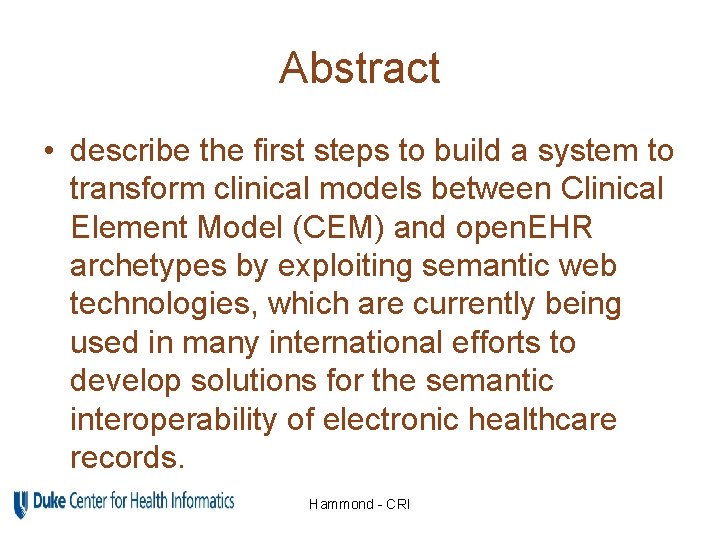
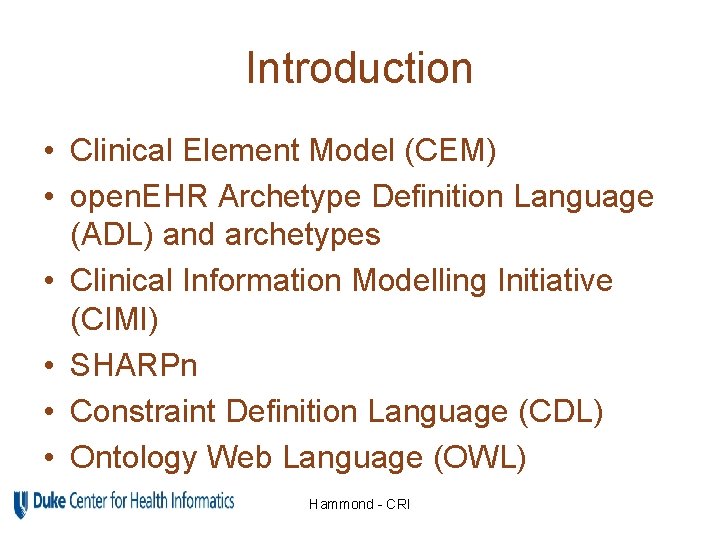
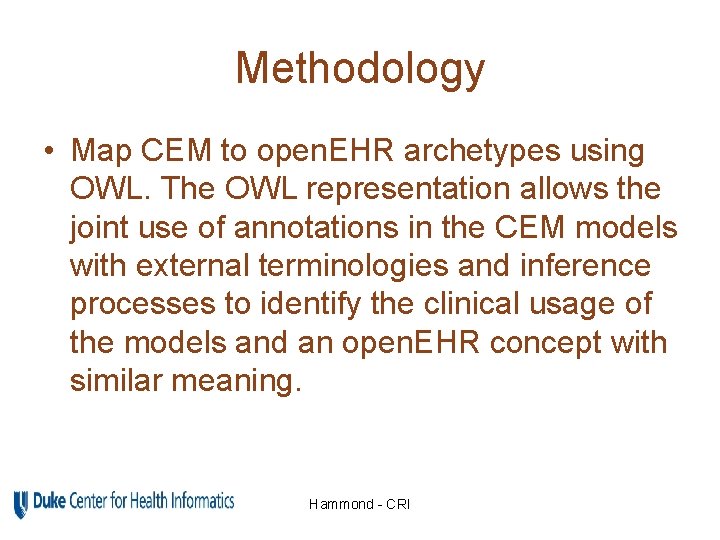
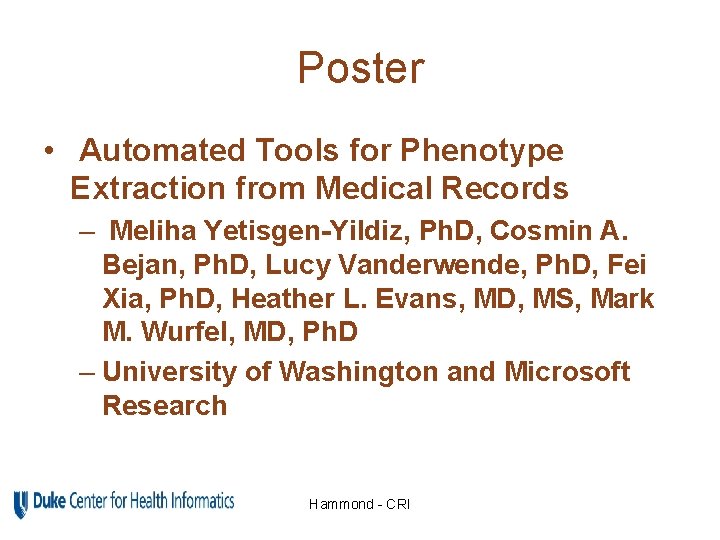
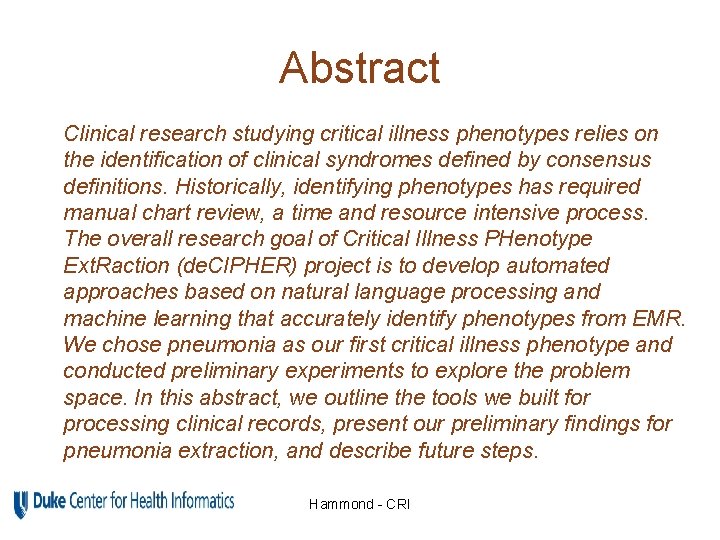
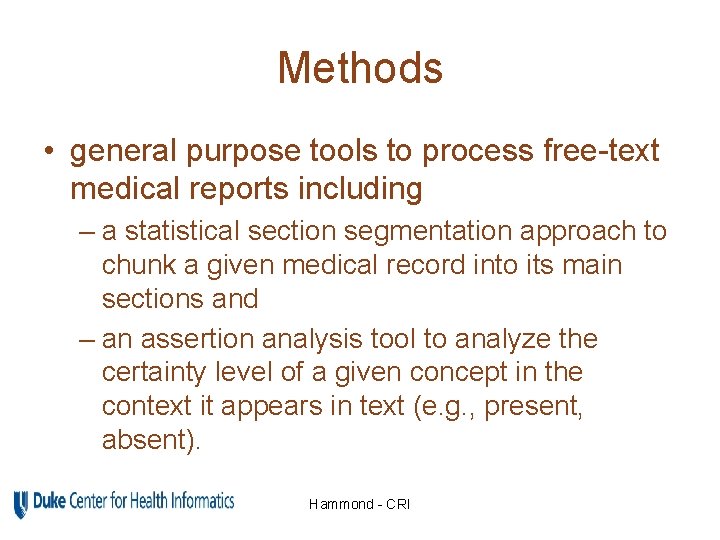
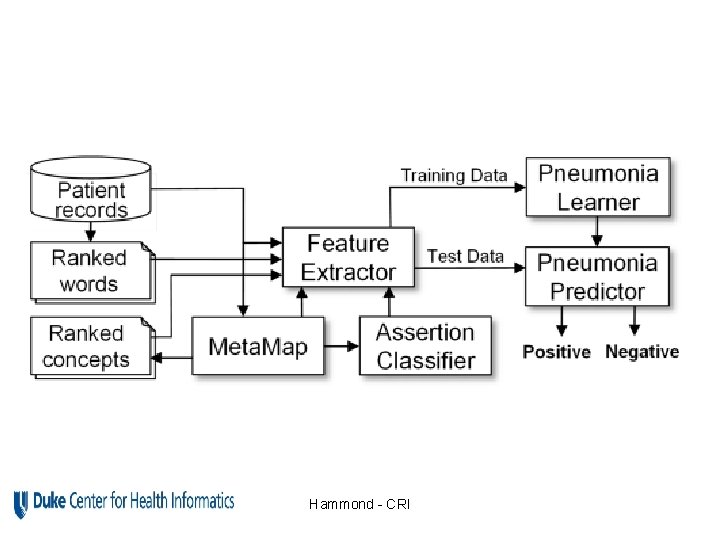
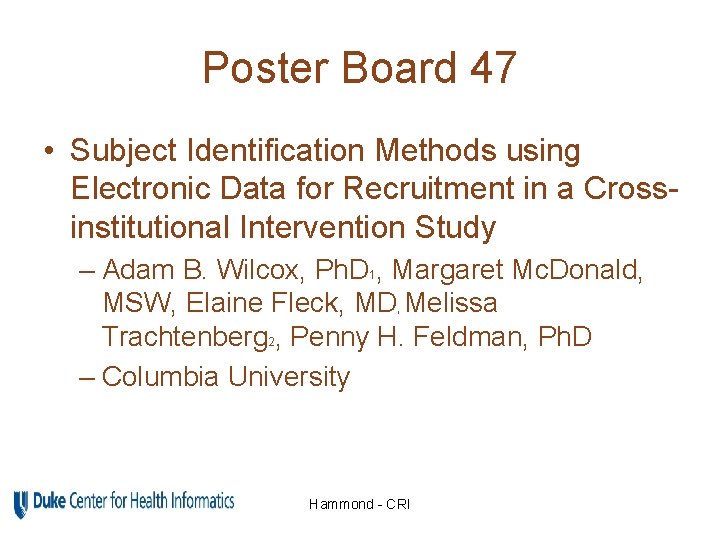
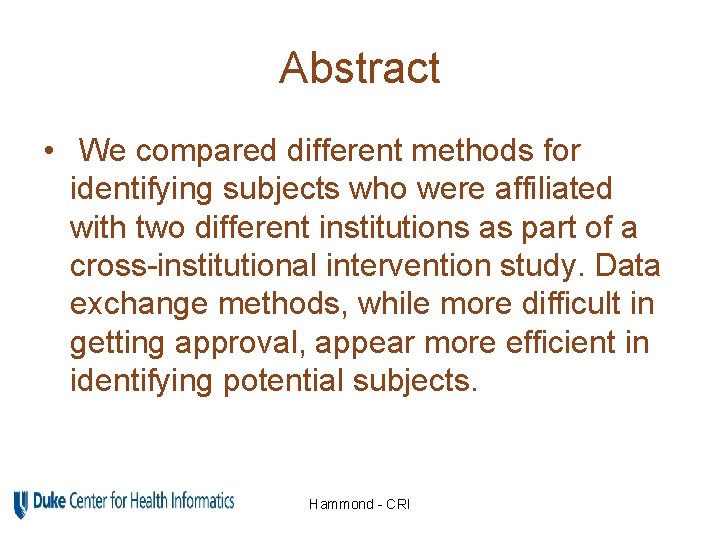
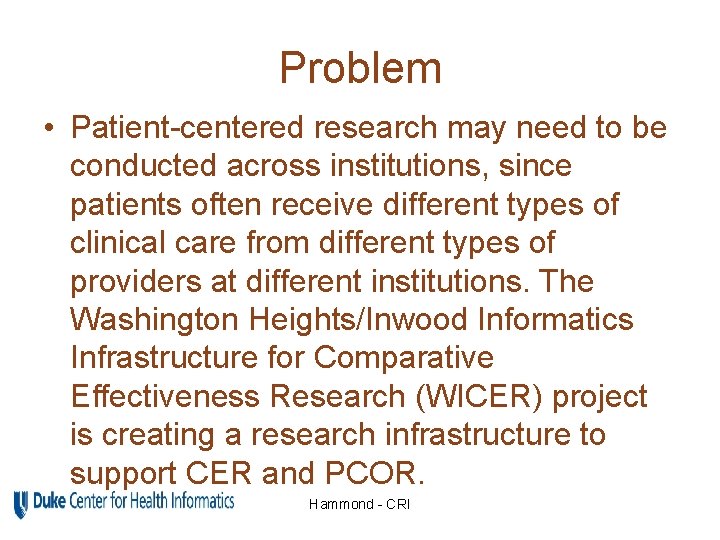
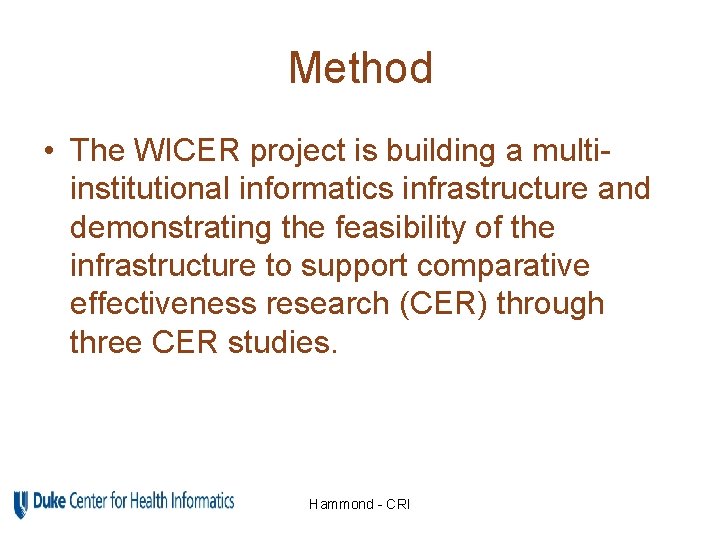
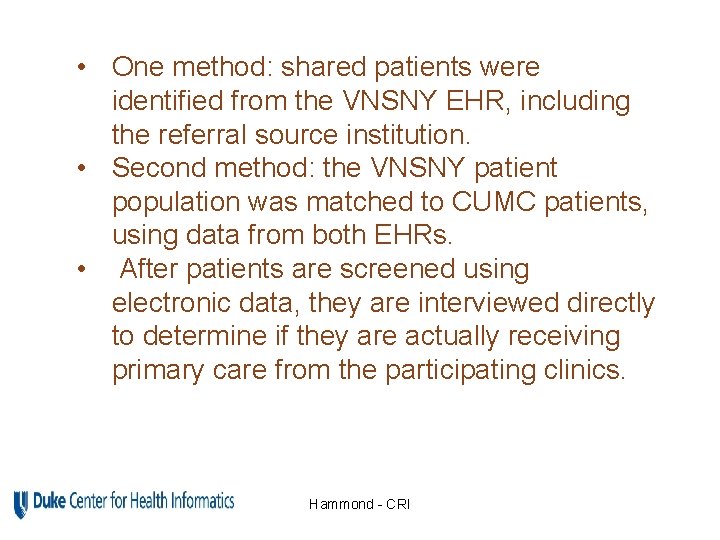
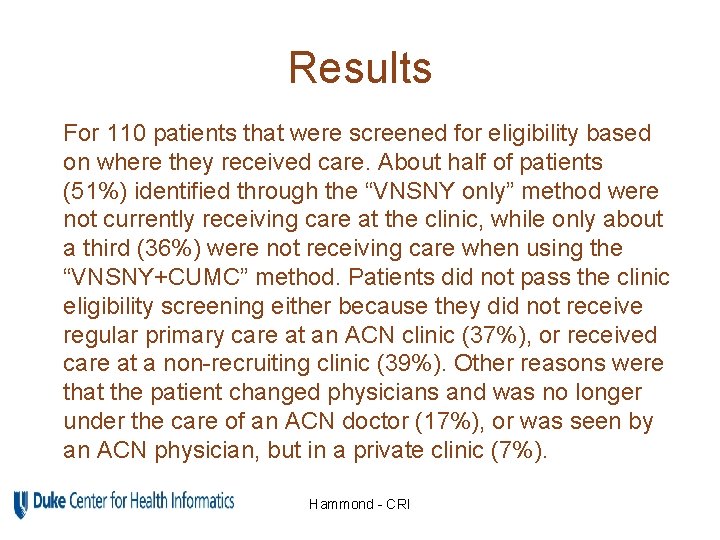
- Slides: 22
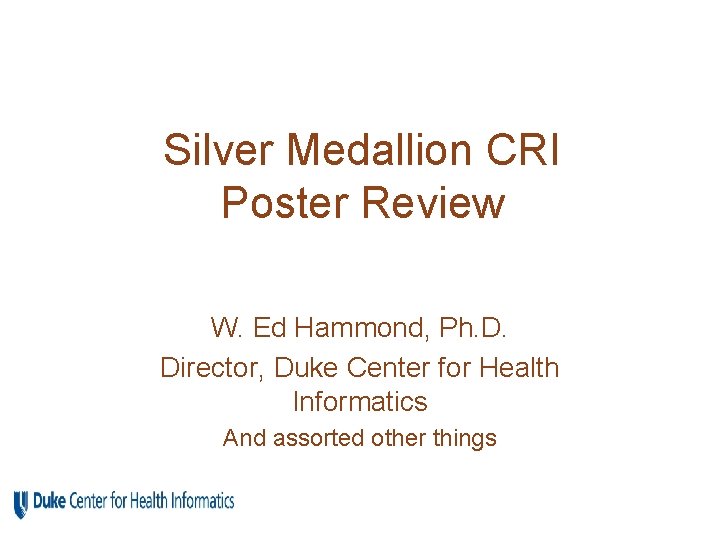
Silver Medallion CRI Poster Review W. Ed Hammond, Ph. D. Director, Duke Center for Health Informatics And assorted other things
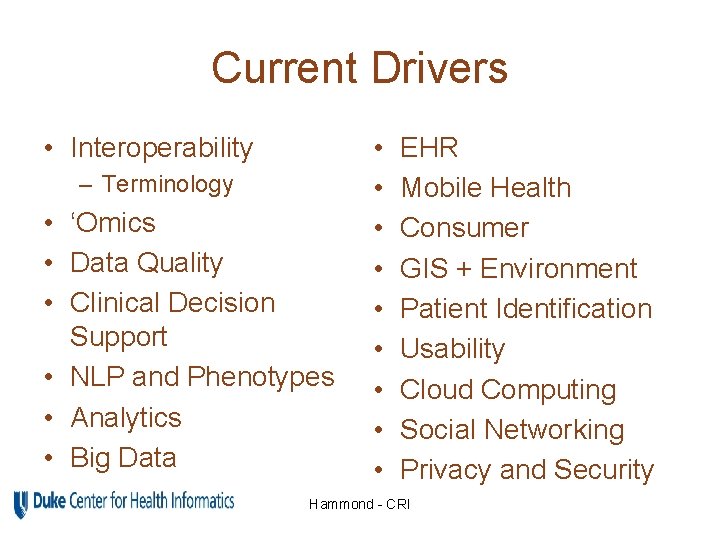
Current Drivers • Interoperability – Terminology • ‘Omics • Data Quality • Clinical Decision Support • NLP and Phenotypes • Analytics • Big Data • • • EHR Mobile Health Consumer GIS + Environment Patient Identification Usability Cloud Computing Social Networking Privacy and Security Hammond - CRI
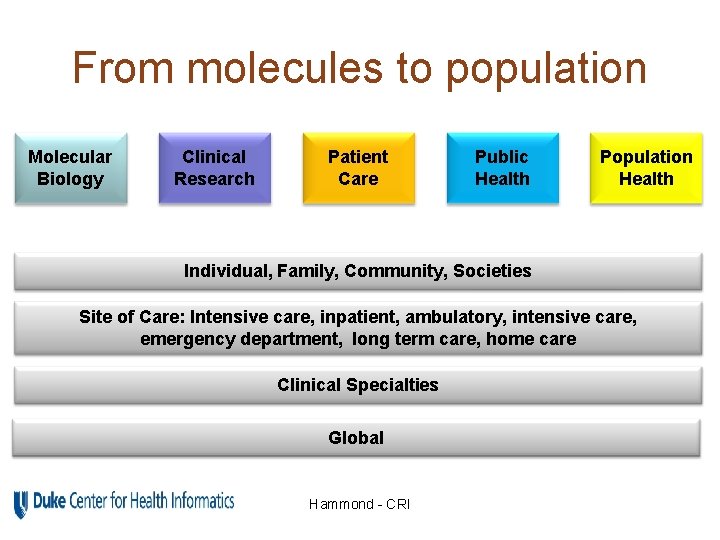
From molecules to population Molecular Biology Clinical Research Patient Care Public Health Population Health Individual, Family, Community, Societies Site of Care: Intensive care, inpatient, ambulatory, intensive care, emergency department, long term care, home care Clinical Specialties Global Hammond - CRI
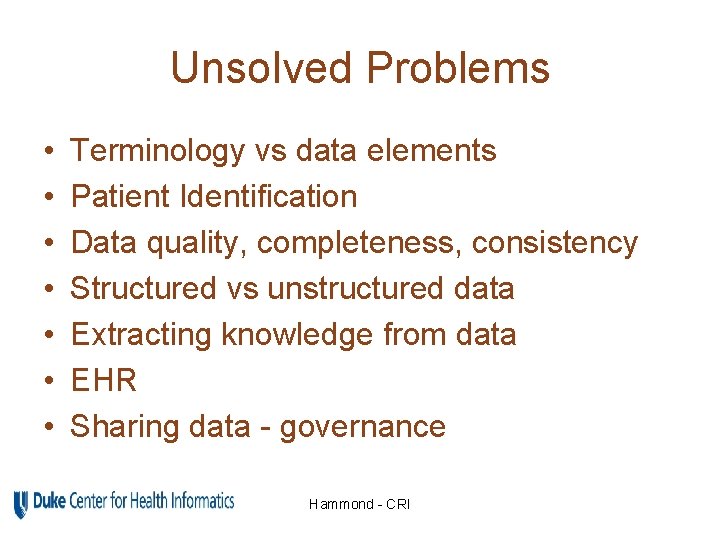
Unsolved Problems • • Terminology vs data elements Patient Identification Data quality, completeness, consistency Structured vs unstructured data Extracting knowledge from data EHR Sharing data - governance Hammond - CRI
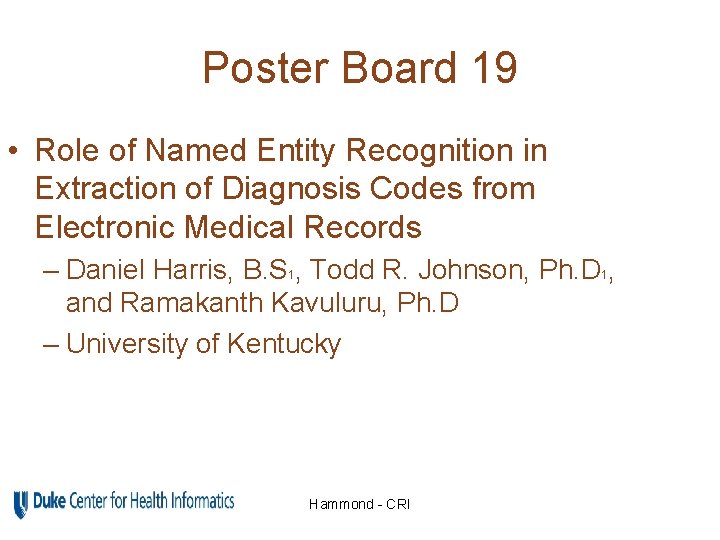
Poster Board 19 • Role of Named Entity Recognition in Extraction of Diagnosis Codes from Electronic Medical Records – Daniel Harris, B. S 1, Todd R. Johnson, Ph. D 1, and Ramakanth Kavuluru, Ph. D – University of Kentucky Hammond - CRI
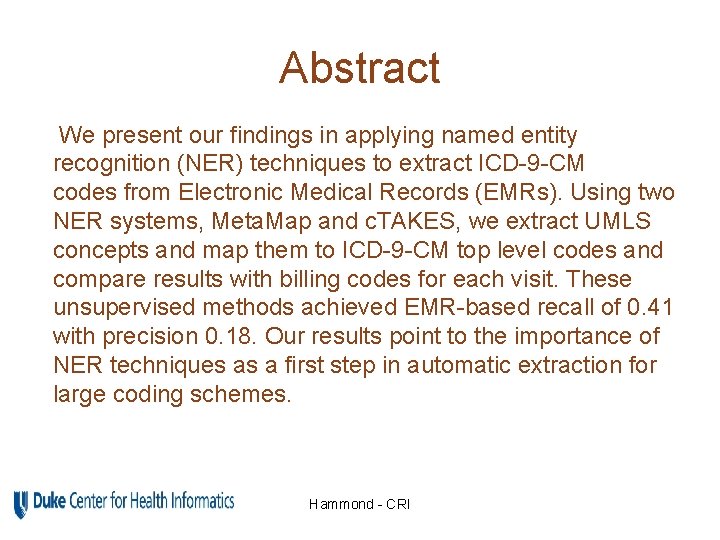
Abstract We present our findings in applying named entity recognition (NER) techniques to extract ICD-9 -CM codes from Electronic Medical Records (EMRs). Using two NER systems, Meta. Map and c. TAKES, we extract UMLS concepts and map them to ICD-9 -CM top level codes and compare results with billing codes for each visit. These unsupervised methods achieved EMR-based recall of 0. 41 with precision 0. 18. Our results point to the importance of NER techniques as a first step in automatic extraction for large coding schemes. Hammond - CRI
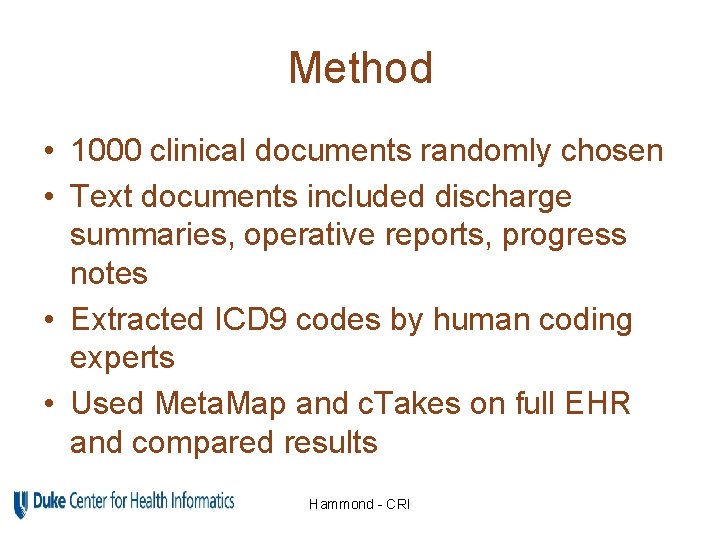
Method • 1000 clinical documents randomly chosen • Text documents included discharge summaries, operative reports, progress notes • Extracted ICD 9 codes by human coding experts • Used Meta. Map and c. Takes on full EHR and compared results Hammond - CRI
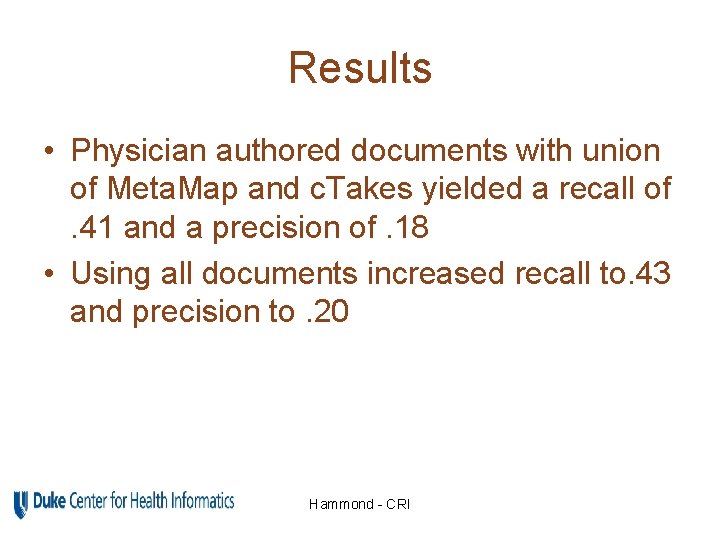
Results • Physician authored documents with union of Meta. Map and c. Takes yielded a recall of. 41 and a precision of. 18 • Using all documents increased recall to. 43 and precision to. 20 Hammond - CRI
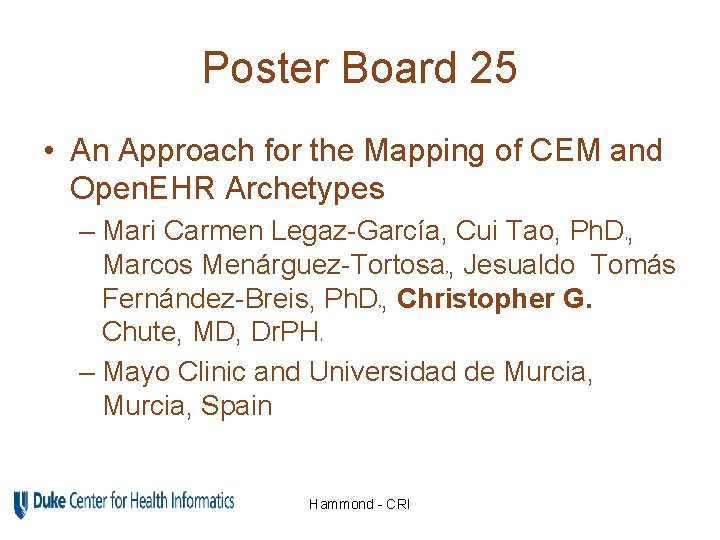
Poster Board 25 • An Approach for the Mapping of CEM and Open. EHR Archetypes – Mari Carmen Legaz-García, Cui Tao, Ph. D , Marcos Menárguez-Tortosa , Jesualdo Tomás Fernández-Breis, Ph. D , Christopher G. Chute, MD, Dr. PH – Mayo Clinic and Universidad de Murcia, Spain 1 2 2 1 Hammond - CRI
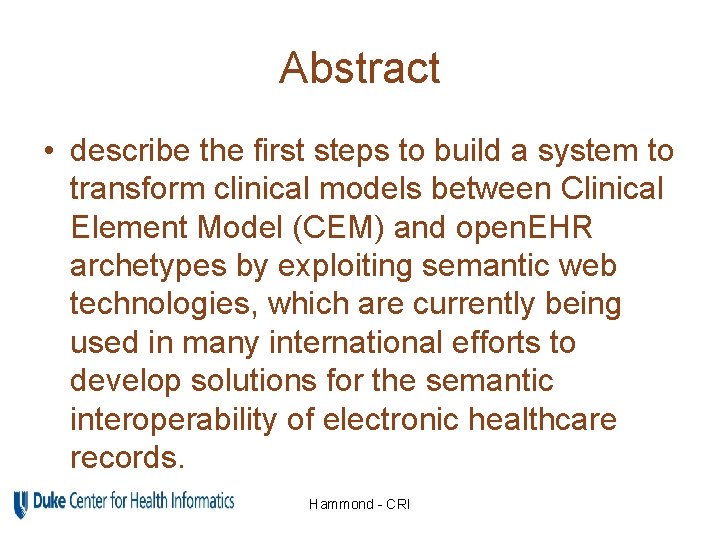
Abstract • describe the first steps to build a system to transform clinical models between Clinical Element Model (CEM) and open. EHR archetypes by exploiting semantic web technologies, which are currently being used in many international efforts to develop solutions for the semantic interoperability of electronic healthcare records. Hammond - CRI
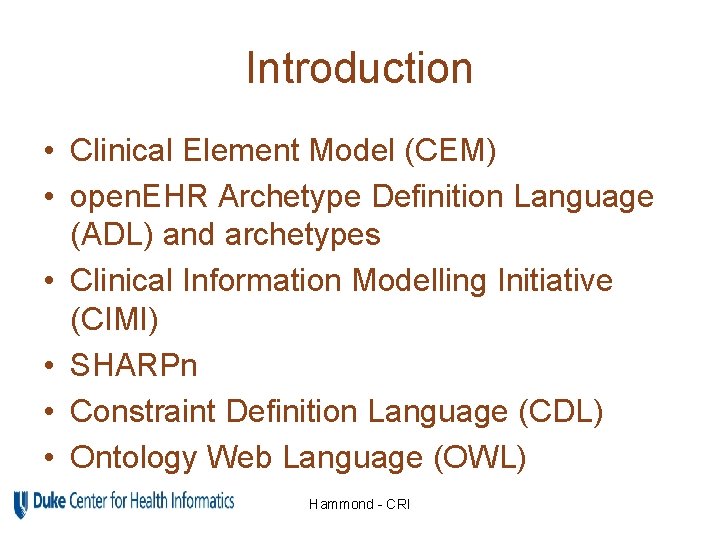
Introduction • Clinical Element Model (CEM) • open. EHR Archetype Definition Language (ADL) and archetypes • Clinical Information Modelling Initiative (CIMI) • SHARPn • Constraint Definition Language (CDL) • Ontology Web Language (OWL) Hammond - CRI
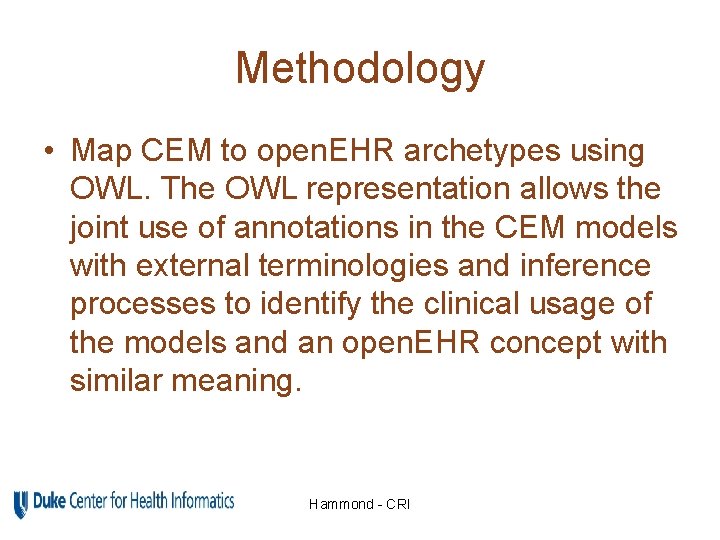
Methodology • Map CEM to open. EHR archetypes using OWL. The OWL representation allows the joint use of annotations in the CEM models with external terminologies and inference processes to identify the clinical usage of the models and an open. EHR concept with similar meaning. Hammond - CRI
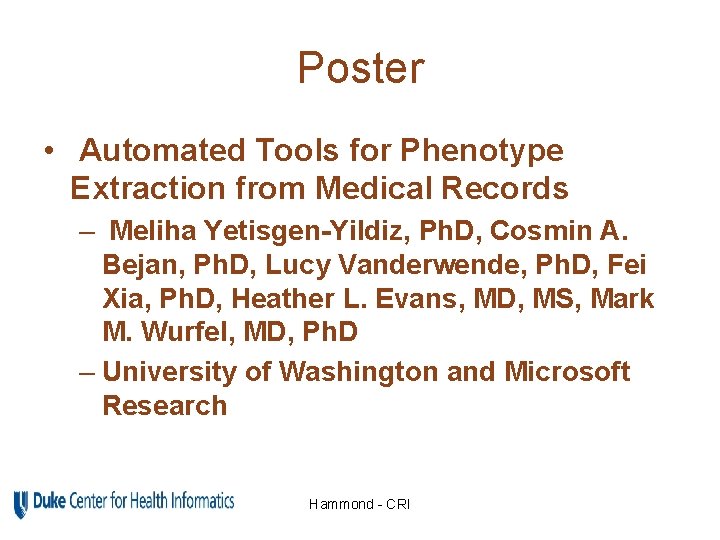
Poster • Automated Tools for Phenotype Extraction from Medical Records – Meliha Yetisgen-Yildiz, Ph. D, Cosmin A. Bejan, Ph. D, Lucy Vanderwende, Ph. D, Fei Xia, Ph. D, Heather L. Evans, MD, MS, Mark M. Wurfel, MD, Ph. D – University of Washington and Microsoft Research Hammond - CRI
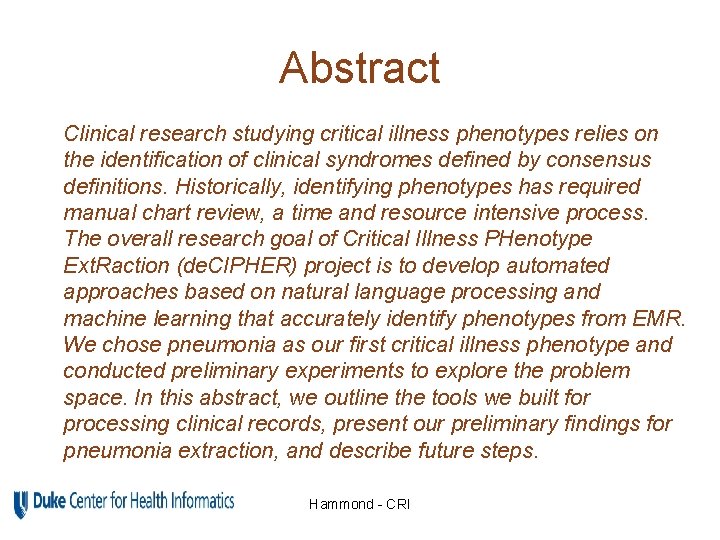
Abstract Clinical research studying critical illness phenotypes relies on the identification of clinical syndromes defined by consensus definitions. Historically, identifying phenotypes has required manual chart review, a time and resource intensive process. The overall research goal of Critical Illness PHenotype Ext. Raction (de. CIPHER) project is to develop automated approaches based on natural language processing and machine learning that accurately identify phenotypes from EMR. We chose pneumonia as our first critical illness phenotype and conducted preliminary experiments to explore the problem space. In this abstract, we outline the tools we built for processing clinical records, present our preliminary findings for pneumonia extraction, and describe future steps. Hammond - CRI
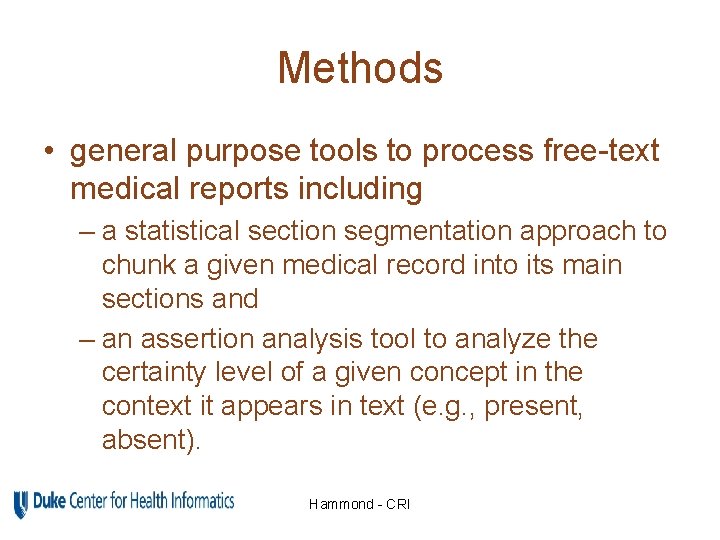
Methods • general purpose tools to process free-text medical reports including – a statistical section segmentation approach to chunk a given medical record into its main sections and – an assertion analysis tool to analyze the certainty level of a given concept in the context it appears in text (e. g. , present, absent). Hammond - CRI
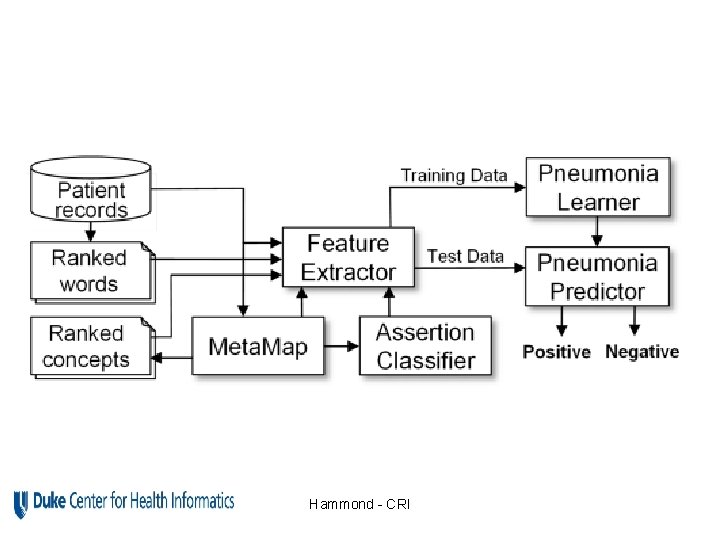
Hammond - CRI
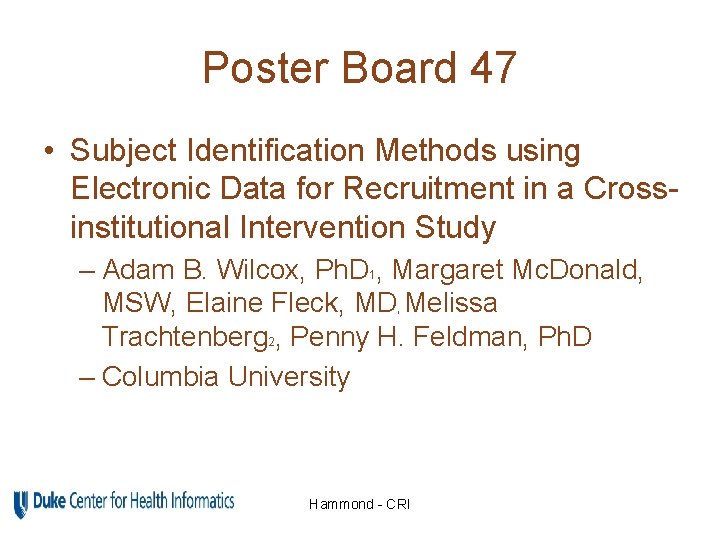
Poster Board 47 • Subject Identification Methods using Electronic Data for Recruitment in a Crossinstitutional Intervention Study – Adam B. Wilcox, Ph. D 1, Margaret Mc. Donald, MSW, Elaine Fleck, MD, Melissa Trachtenberg 2, Penny H. Feldman, Ph. D – Columbia University Hammond - CRI
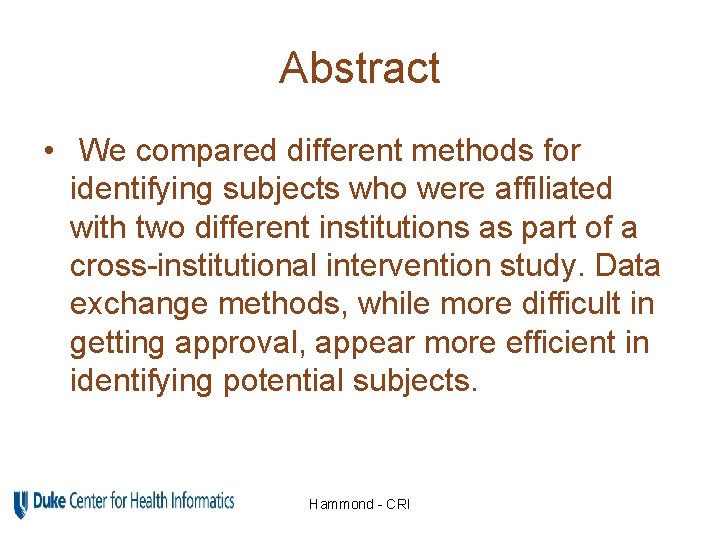
Abstract • We compared different methods for identifying subjects who were affiliated with two different institutions as part of a cross-institutional intervention study. Data exchange methods, while more difficult in getting approval, appear more efficient in identifying potential subjects. Hammond - CRI
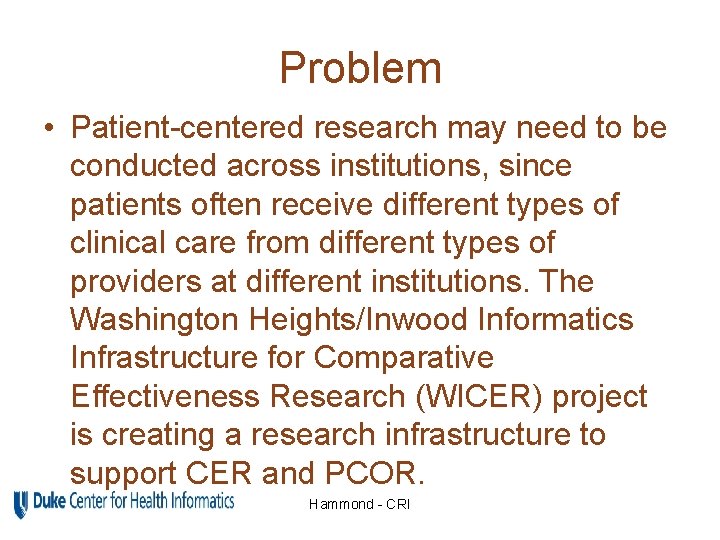
Problem • Patient-centered research may need to be conducted across institutions, since patients often receive different types of clinical care from different types of providers at different institutions. The Washington Heights/Inwood Informatics Infrastructure for Comparative Effectiveness Research (WICER) project is creating a research infrastructure to support CER and PCOR. Hammond - CRI
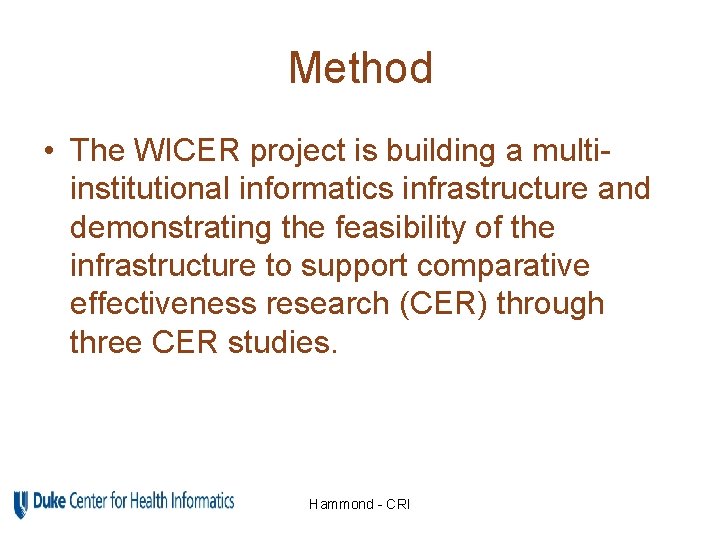
Method • The WICER project is building a multiinstitutional informatics infrastructure and demonstrating the feasibility of the infrastructure to support comparative effectiveness research (CER) through three CER studies. Hammond - CRI
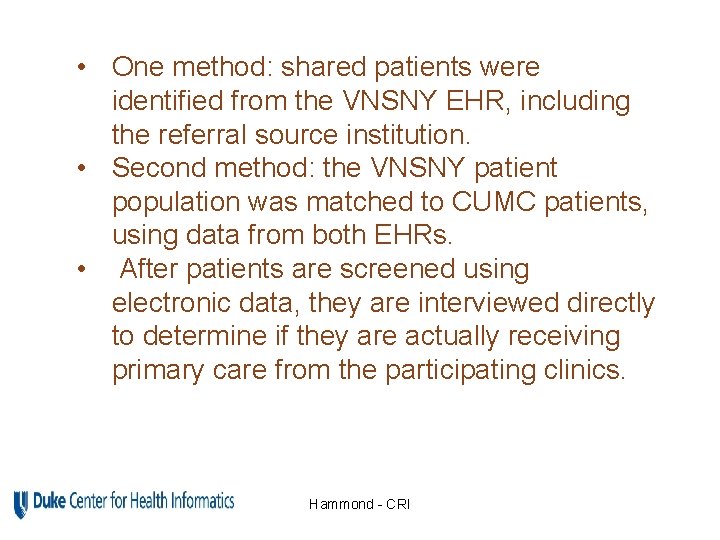
• One method: shared patients were identified from the VNSNY EHR, including the referral source institution. • Second method: the VNSNY patient population was matched to CUMC patients, using data from both EHRs. • After patients are screened using electronic data, they are interviewed directly to determine if they are actually receiving primary care from the participating clinics. Hammond - CRI
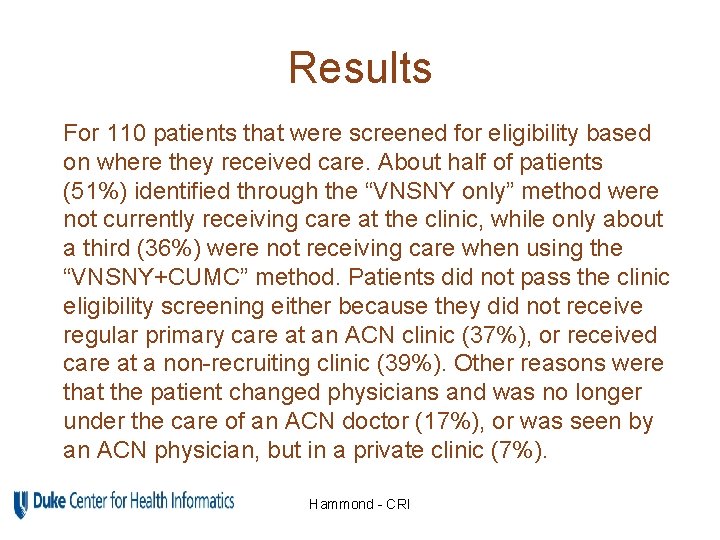
Results For 110 patients that were screened for eligibility based on where they received care. About half of patients (51%) identified through the “VNSNY only” method were not currently receiving care at the clinic, while only about a third (36%) were not receiving care when using the “VNSNY+CUMC” method. Patients did not pass the clinic eligibility screening either because they did not receive regular primary care at an ACN clinic (37%), or received care at a non-recruiting clinic (39%). Other reasons were that the patient changed physicians and was no longer under the care of an ACN doctor (17%), or was seen by an ACN physician, but in a private clinic (7%). Hammond - CRI
Dealuri zgribulite
Biografia de cri cri
Silver carpet bedroom
Chemical change examples
Megan alfonsi
Medallion group
Choose about fact x opinion, in this same order:
Silver plus medicine
Cri du chat karyotype
Sindromul cri du chat wikipedia
Cri du chat chromosomal abnormalities
Cri du chat karyotype
Does tiki barber have sickle cell
Ljubec
Cri
Sindrom cri du chat
Www.cri.or.th
Cri du chat syndrome
Corso opem cri 2021 slide
Mutasi gen
Cri framework
Eu fico com.a pureza
Dot