Seasonal Climate Prediction Li Xu Department of Meteorology
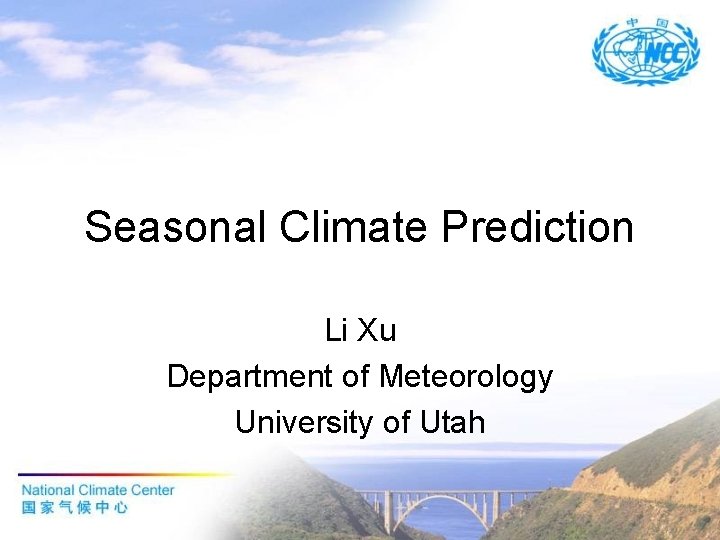
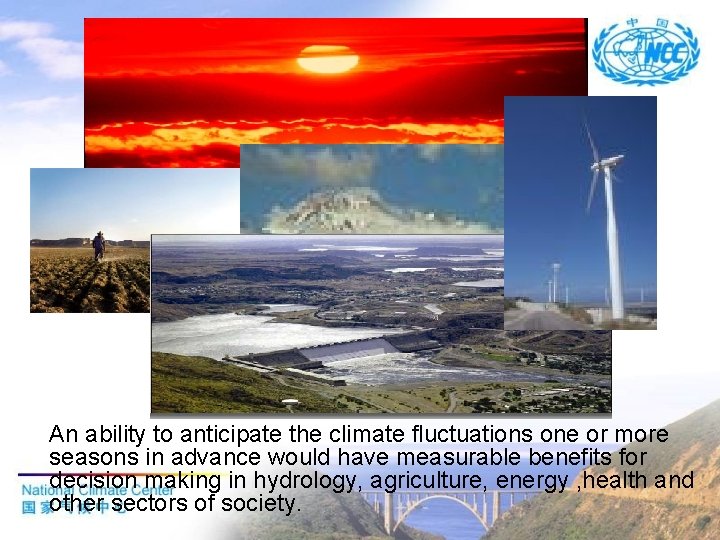
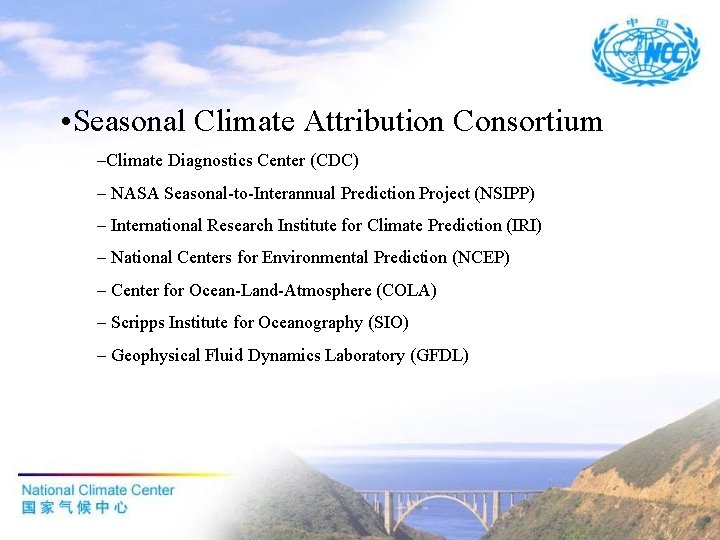
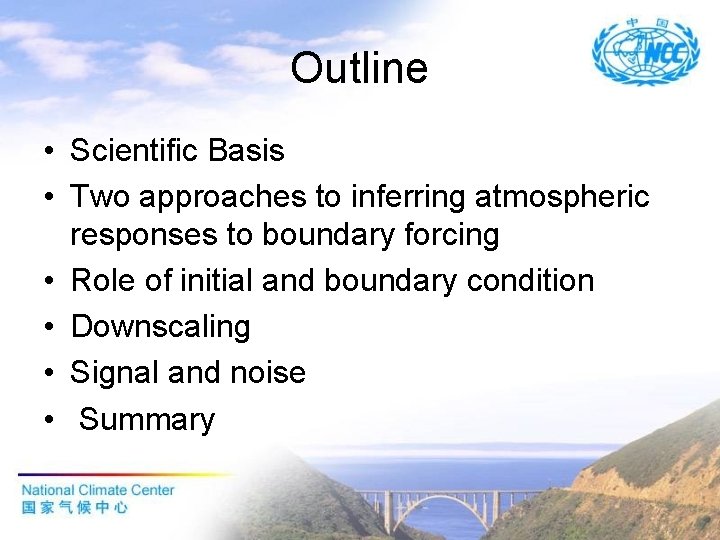
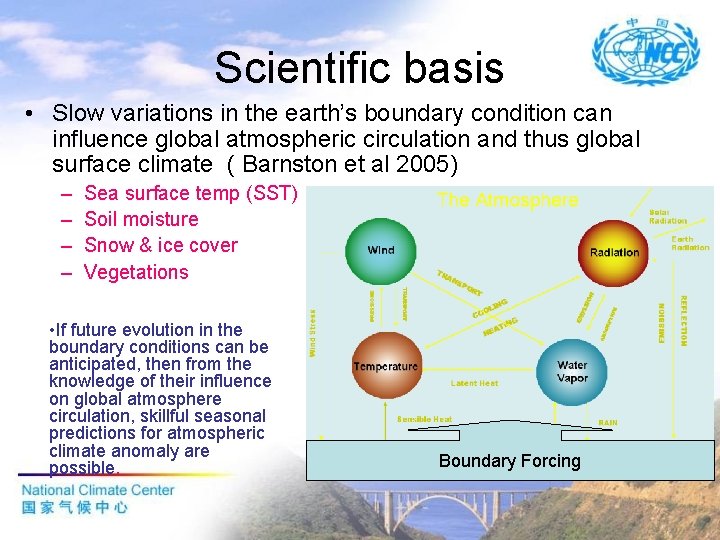
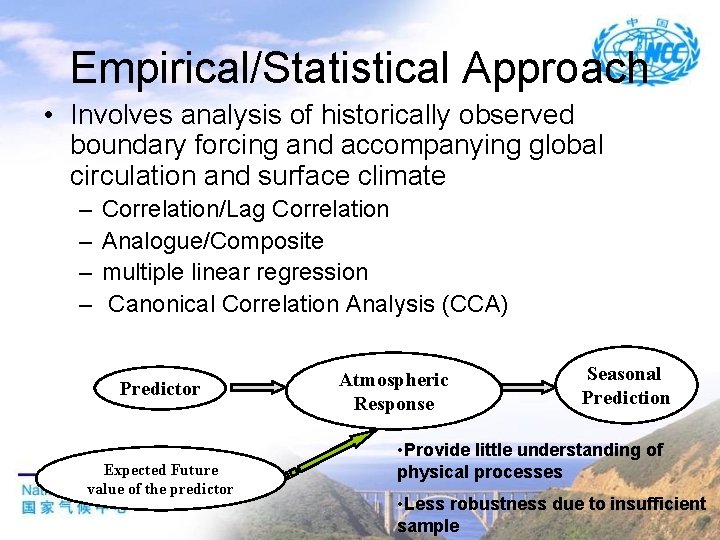
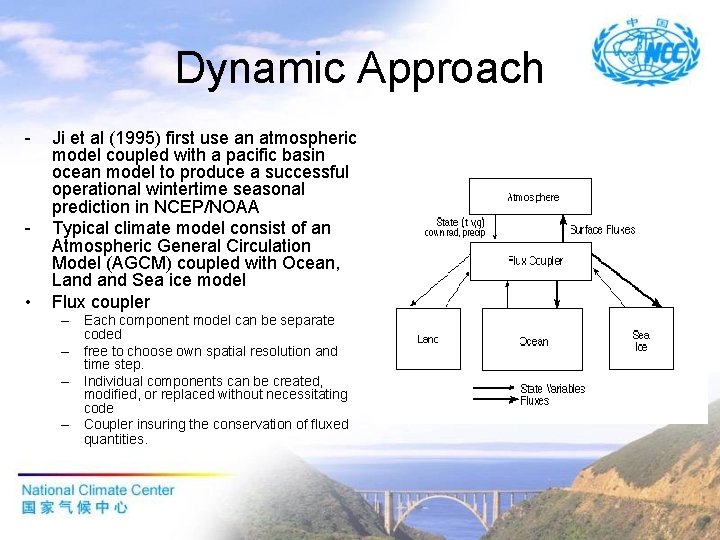
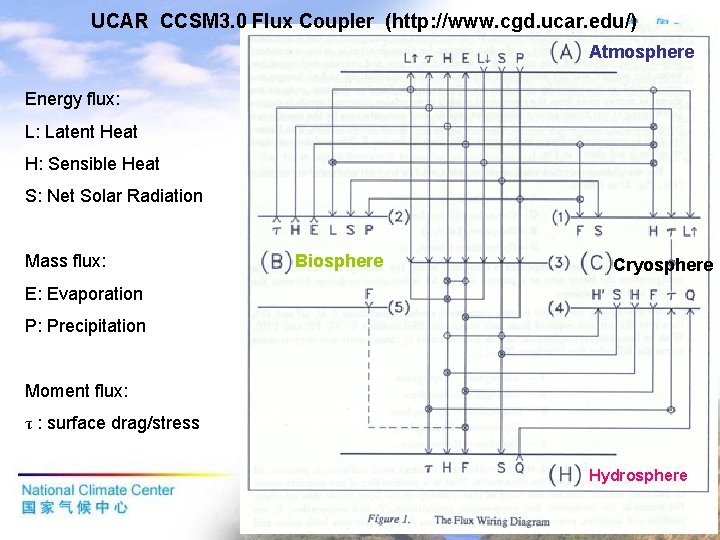
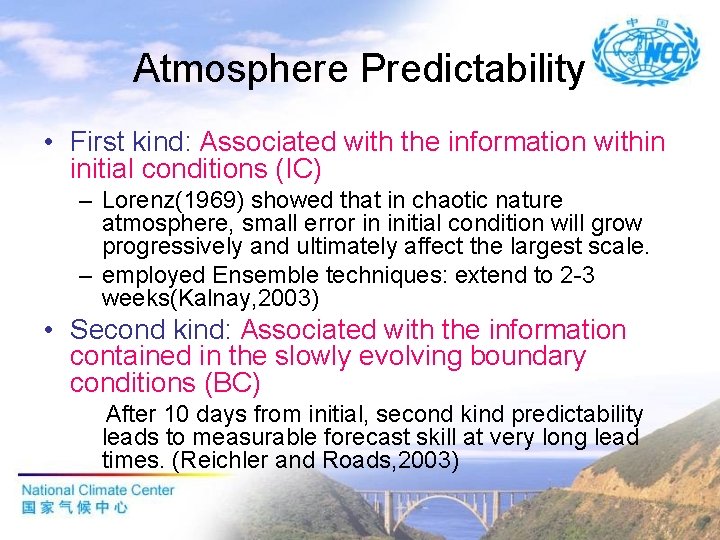
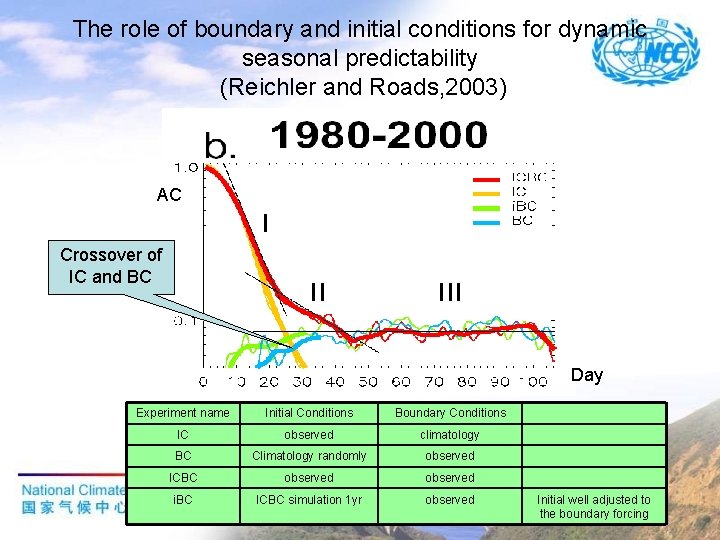
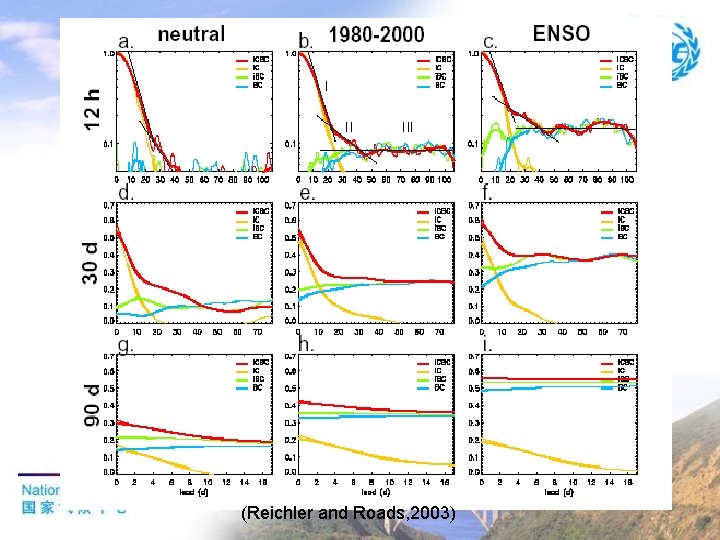
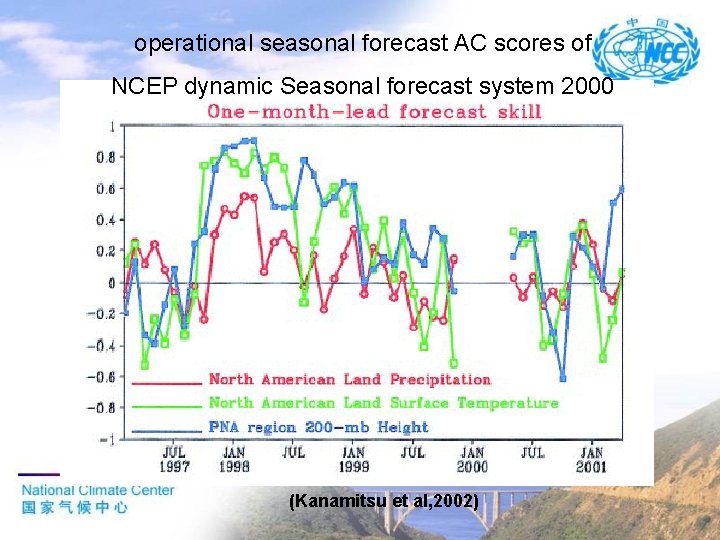
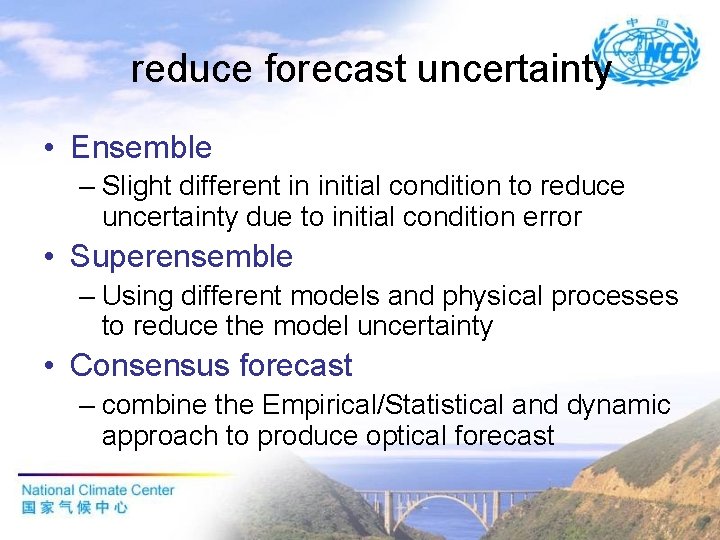
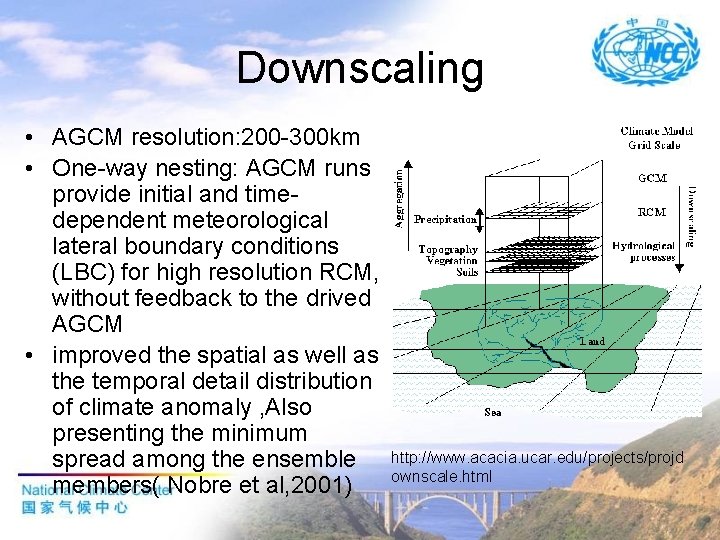
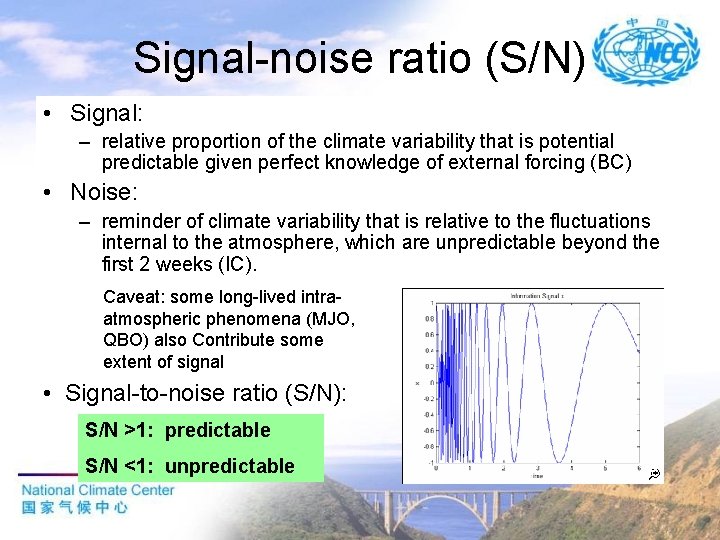
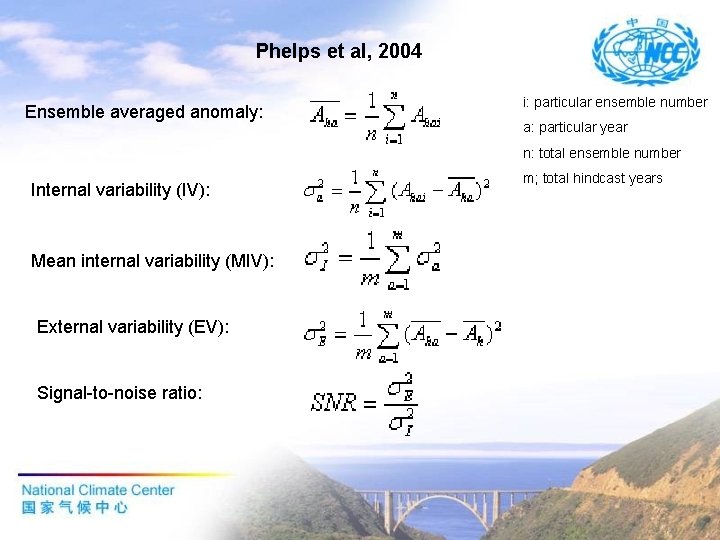
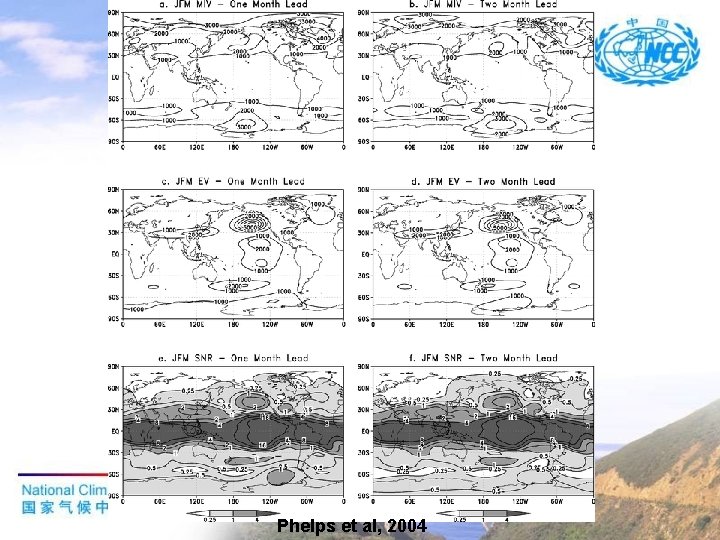
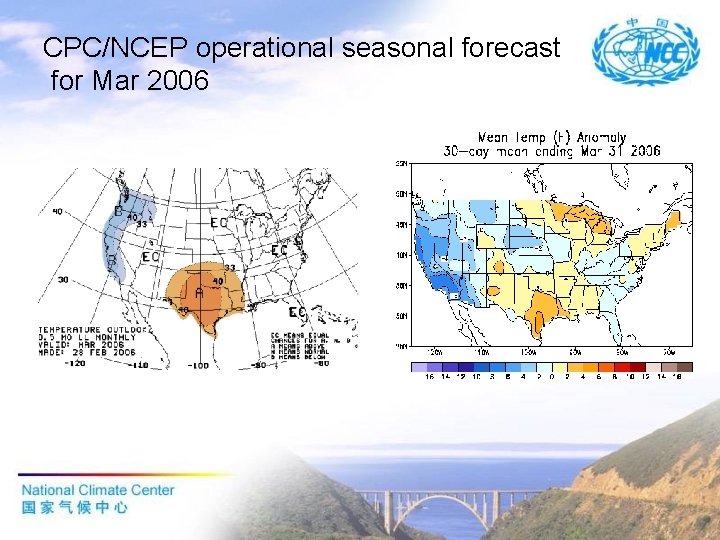
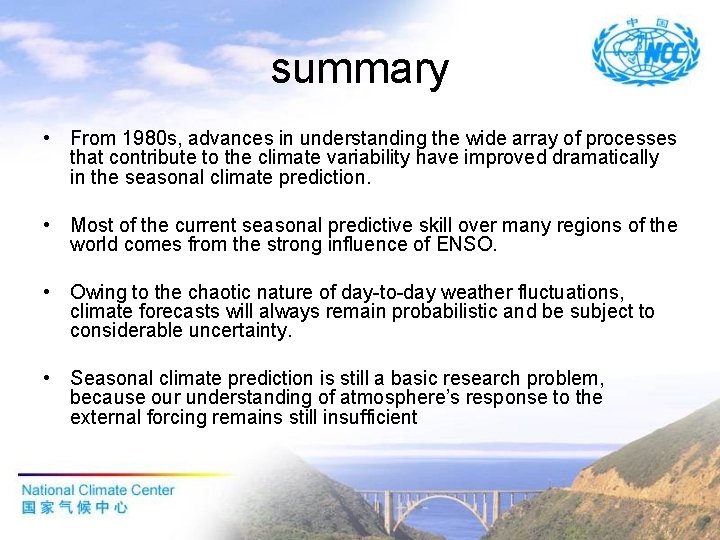
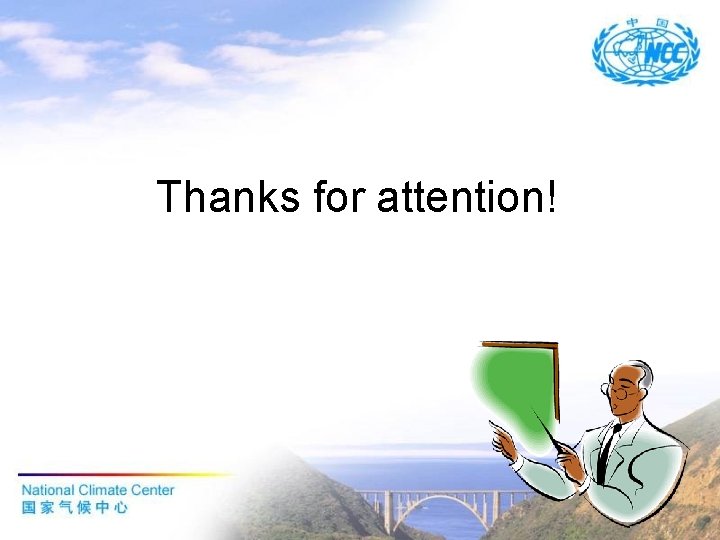
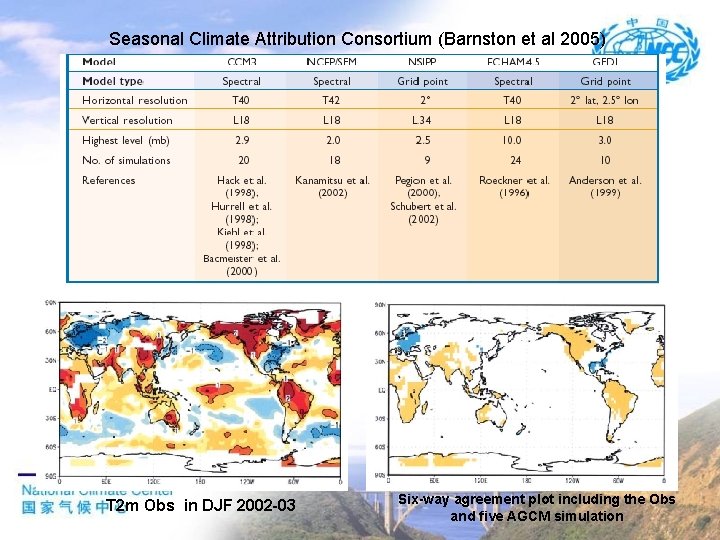
- Slides: 21
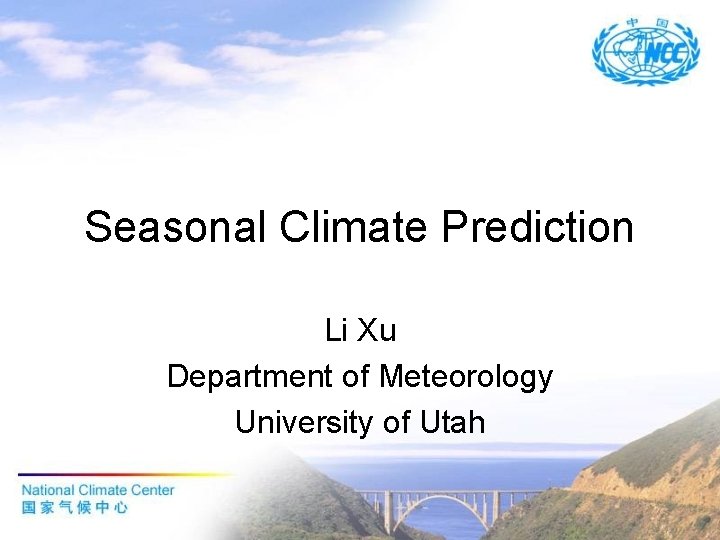
Seasonal Climate Prediction Li Xu Department of Meteorology University of Utah
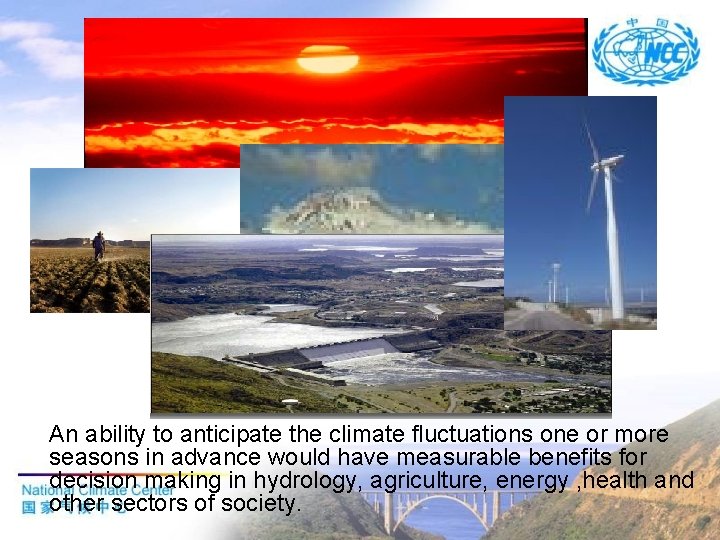
An ability to anticipate the climate fluctuations one or more seasons in advance would have measurable benefits for decision making in hydrology, agriculture, energy , health and other sectors of society.
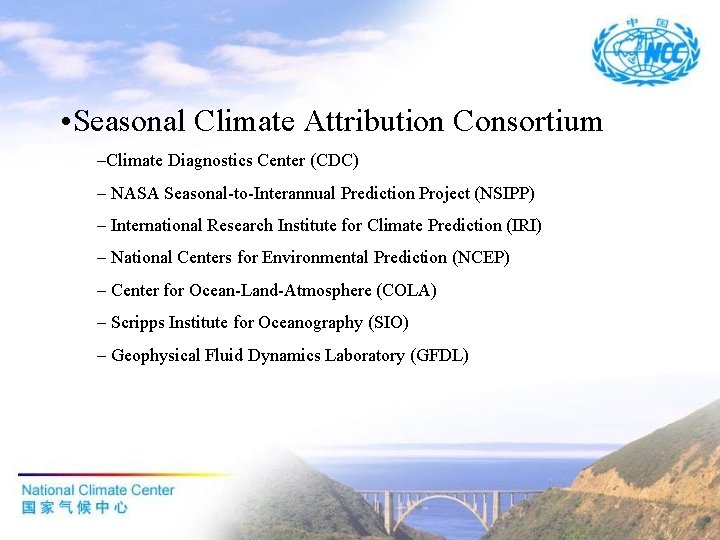
• Seasonal Climate Attribution Consortium –Climate Diagnostics Center (CDC) – NASA Seasonal-to-Interannual Prediction Project (NSIPP) – International Research Institute for Climate Prediction (IRI) – National Centers for Environmental Prediction (NCEP) – Center for Ocean-Land-Atmosphere (COLA) – Scripps Institute for Oceanography (SIO) – Geophysical Fluid Dynamics Laboratory (GFDL)
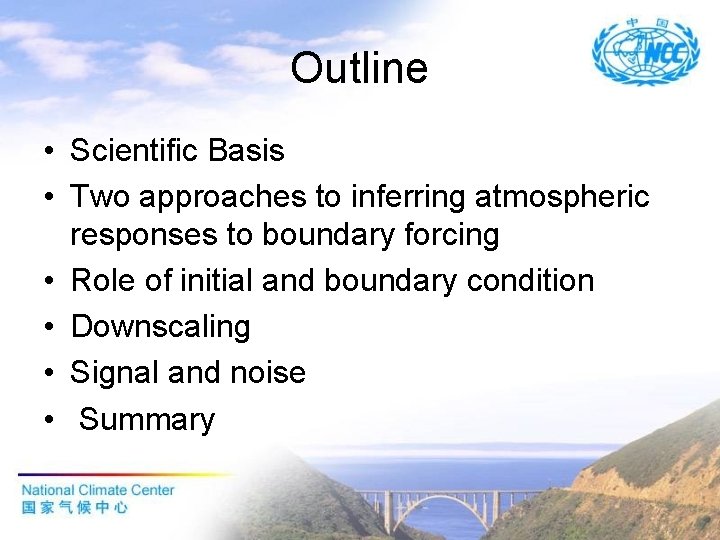
Outline • Scientific Basis • Two approaches to inferring atmospheric responses to boundary forcing • Role of initial and boundary condition • Downscaling • Signal and noise • Summary
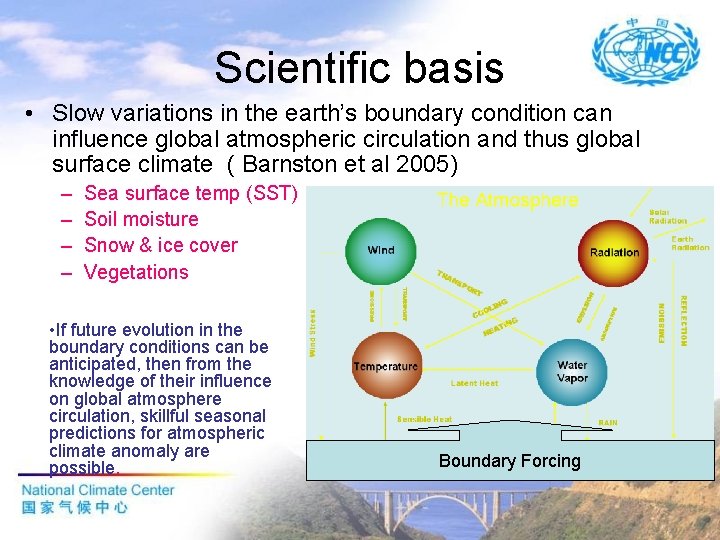
Scientific basis • Slow variations in the earth’s boundary condition can influence global atmospheric circulation and thus global surface climate ( Barnston et al 2005) – – Sea surface temp (SST) Soil moisture Snow & ice cover Vegetations • If future evolution in the boundary conditions can be anticipated, then from the knowledge of their influence on global atmosphere circulation, skillful seasonal predictions for atmospheric climate anomaly are possible. Boundary Forcing
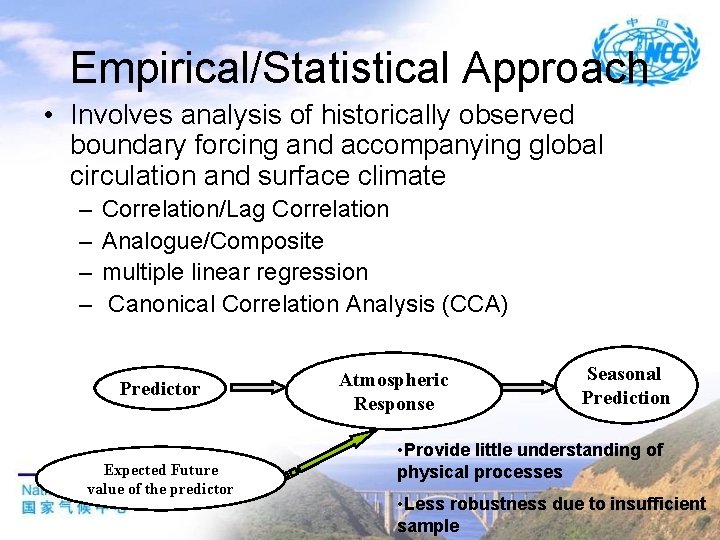
Empirical/Statistical Approach • Involves analysis of historically observed boundary forcing and accompanying global circulation and surface climate – – Correlation/Lag Correlation Analogue/Composite multiple linear regression Canonical Correlation Analysis (CCA) Predictor Expected Future value of the predictor Atmospheric Response Seasonal Prediction • Provide little understanding of physical processes • Less robustness due to insufficient sample
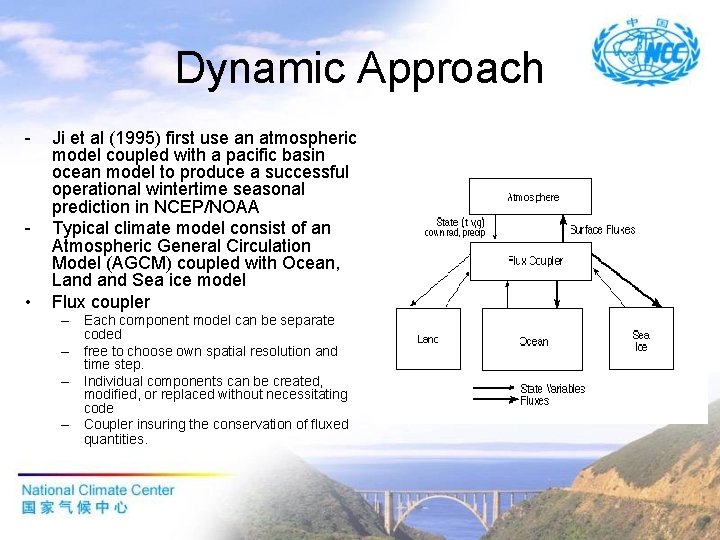
Dynamic Approach - - • Ji et al (1995) first use an atmospheric model coupled with a pacific basin ocean model to produce a successful operational wintertime seasonal prediction in NCEP/NOAA Typical climate model consist of an Atmospheric General Circulation Model (AGCM) coupled with Ocean, Land Sea ice model Flux coupler – Each component model can be separate coded – free to choose own spatial resolution and time step. – Individual components can be created, modified, or replaced without necessitating code – Coupler insuring the conservation of fluxed quantities.
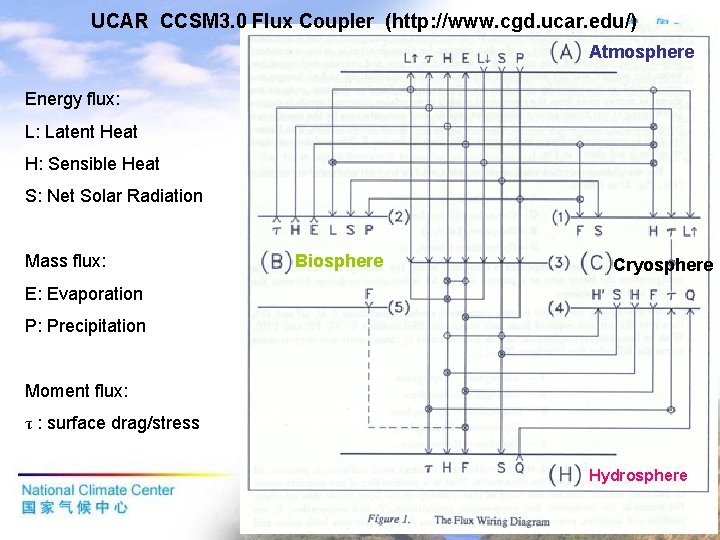
UCAR CCSM 3. 0 Flux Coupler (http: //www. cgd. ucar. edu/) Atmosphere Energy flux: L: Latent Heat H: Sensible Heat S: Net Solar Radiation Mass flux: Biosphere Cryosphere E: Evaporation P: Precipitation Moment flux: τ : surface drag/stress Hydrosphere
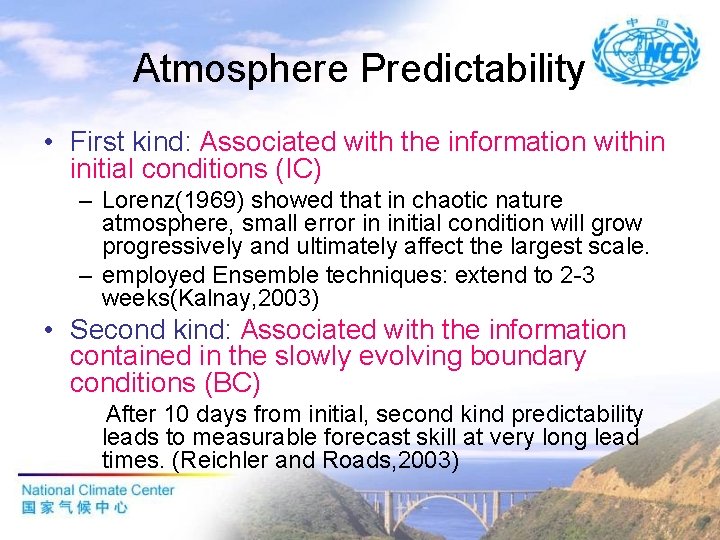
Atmosphere Predictability • First kind: Associated with the information within initial conditions (IC) – Lorenz(1969) showed that in chaotic nature atmosphere, small error in initial condition will grow progressively and ultimately affect the largest scale. – employed Ensemble techniques: extend to 2 -3 weeks(Kalnay, 2003) • Second kind: Associated with the information contained in the slowly evolving boundary conditions (BC) After 10 days from initial, second kind predictability leads to measurable forecast skill at very long lead times. (Reichler and Roads, 2003)
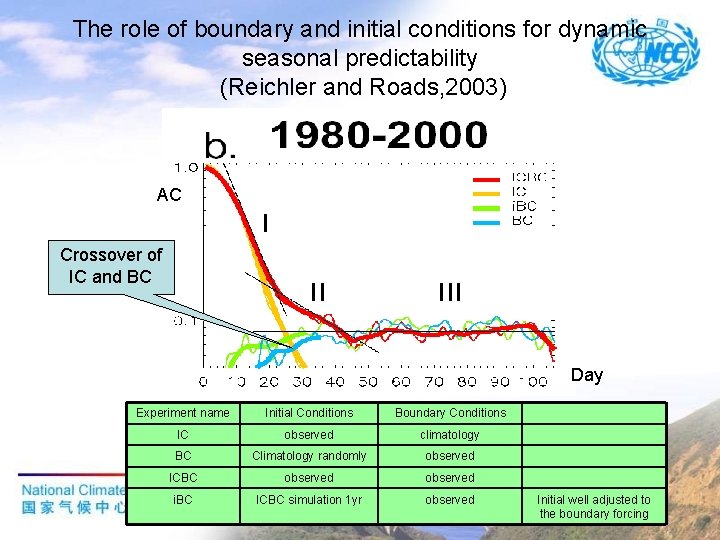
The role of boundary and initial conditions for dynamic seasonal predictability (Reichler and Roads, 2003) AC Crossover of IC and BC Day Experiment name Initial Conditions Boundary Conditions IC observed climatology BC Climatology randomly observed ICBC observed i. BC ICBC simulation 1 yr observed Initial well adjusted to the boundary forcing
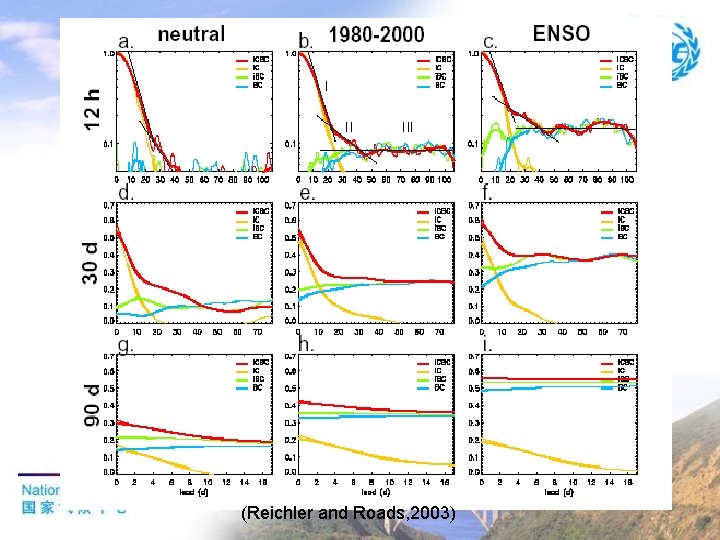
(Reichler and Roads, 2003)
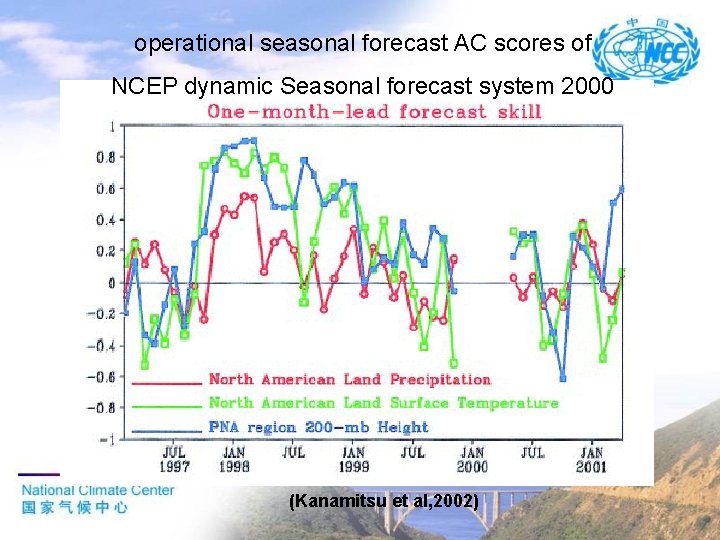
operational seasonal forecast AC scores of NCEP dynamic Seasonal forecast system 2000 (Kanamitsu et al, 2002)
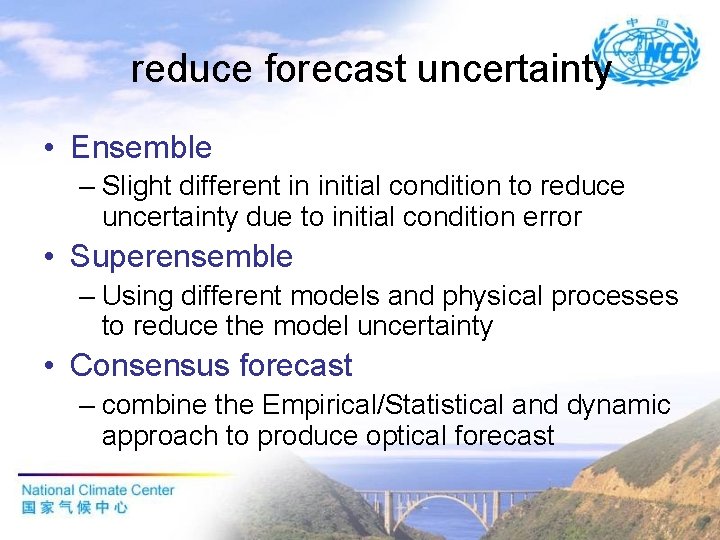
reduce forecast uncertainty • Ensemble – Slight different in initial condition to reduce uncertainty due to initial condition error • Superensemble – Using different models and physical processes to reduce the model uncertainty • Consensus forecast – combine the Empirical/Statistical and dynamic approach to produce optical forecast
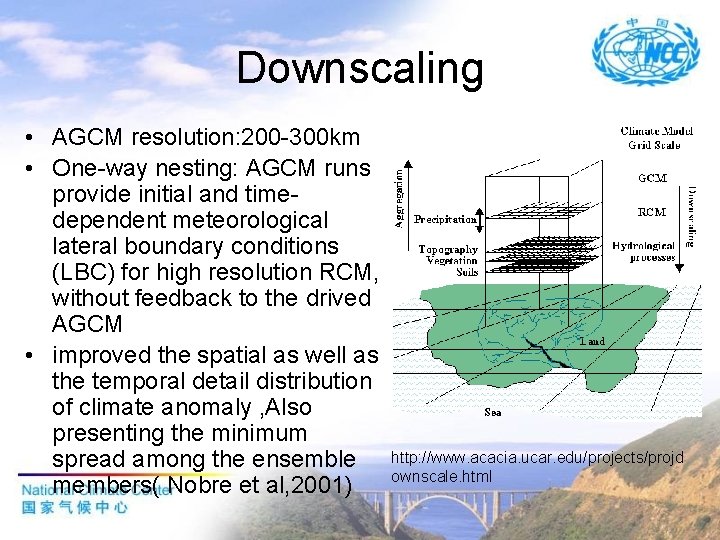
Downscaling • AGCM resolution: 200 -300 km • One-way nesting: AGCM runs provide initial and timedependent meteorological lateral boundary conditions (LBC) for high resolution RCM, without feedback to the drived AGCM • improved the spatial as well as the temporal detail distribution of climate anomaly , Also presenting the minimum spread among the ensemble members( Nobre et al, 2001) http: //www. acacia. ucar. edu/projects/projd ownscale. html
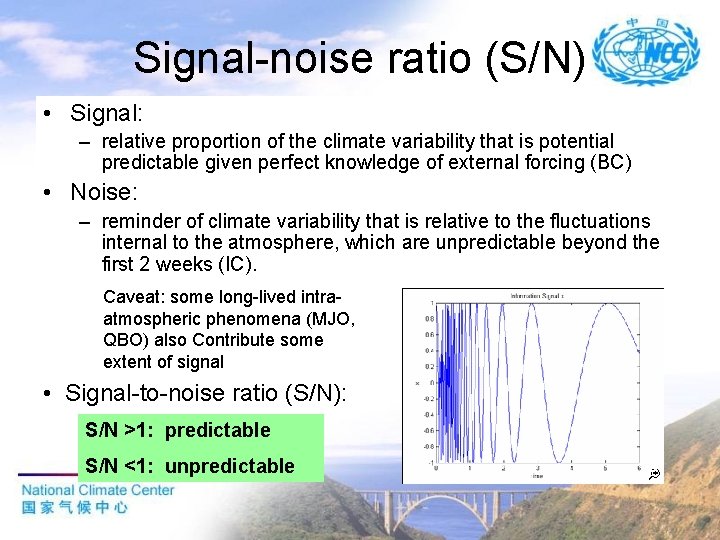
Signal-noise ratio (S/N) • Signal: – relative proportion of the climate variability that is potential predictable given perfect knowledge of external forcing (BC) • Noise: – reminder of climate variability that is relative to the fluctuations internal to the atmosphere, which are unpredictable beyond the first 2 weeks (IC). Caveat: some long-lived intraatmospheric phenomena (MJO, QBO) also Contribute some extent of signal • Signal-to-noise ratio (S/N): S/N >1: predictable S/N <1: unpredictable
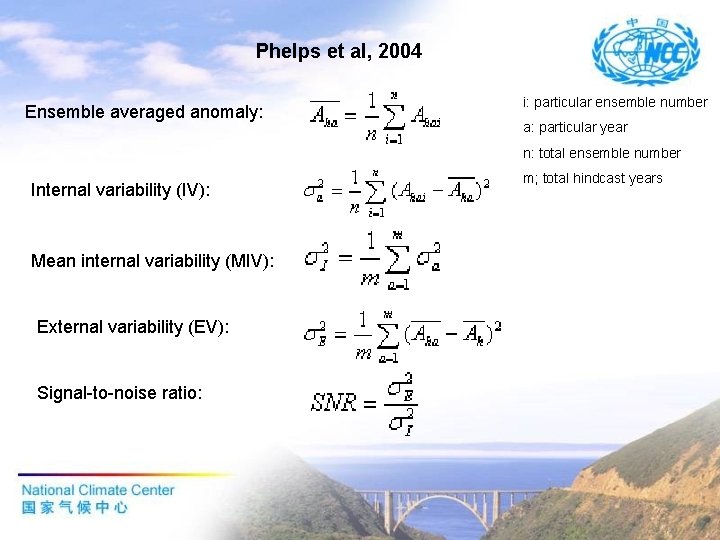
Phelps et al, 2004 Ensemble averaged anomaly: i: particular ensemble number a: particular year n: total ensemble number Internal variability (IV): Mean internal variability (MIV): External variability (EV): Signal-to-noise ratio: m; total hindcast years
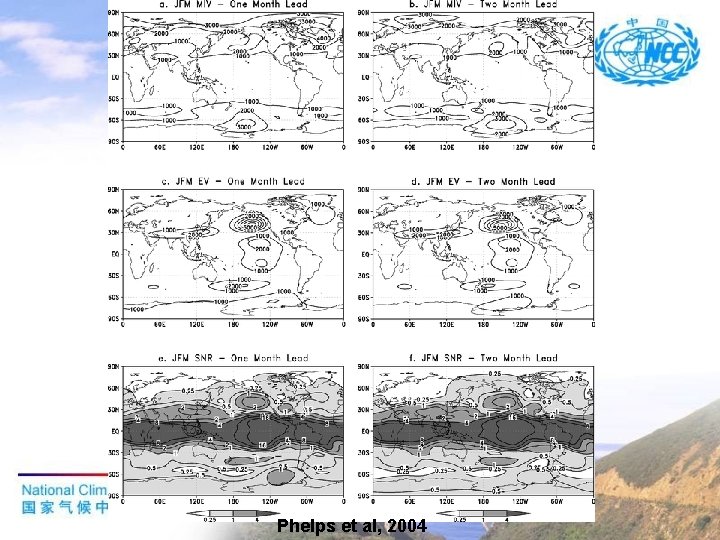
Phelps et al, 2004
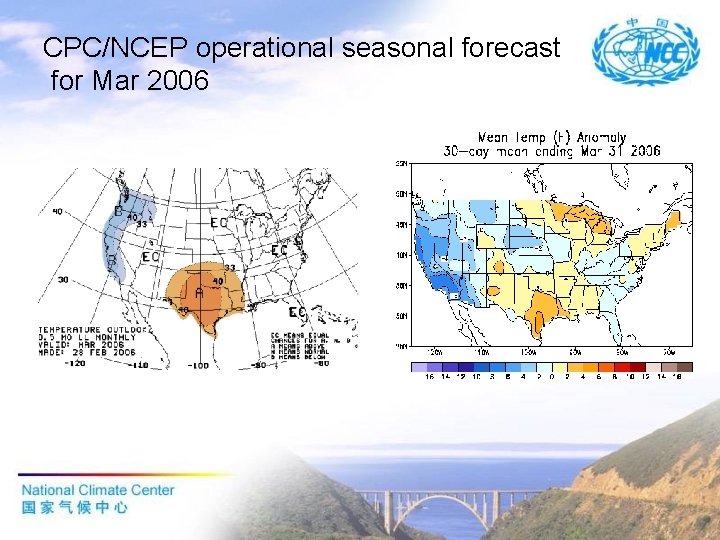
CPC/NCEP operational seasonal forecast for Mar 2006
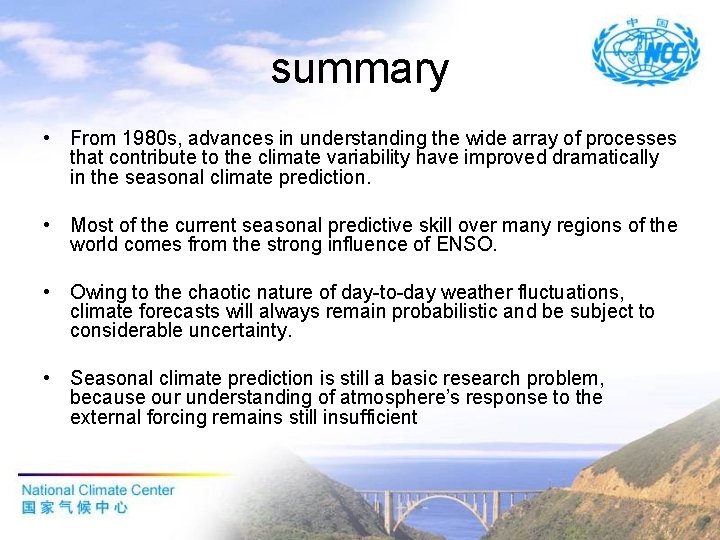
summary • From 1980 s, advances in understanding the wide array of processes that contribute to the climate variability have improved dramatically in the seasonal climate prediction. • Most of the current seasonal predictive skill over many regions of the world comes from the strong influence of ENSO. • Owing to the chaotic nature of day-to-day weather fluctuations, climate forecasts will always remain probabilistic and be subject to considerable uncertainty. • Seasonal climate prediction is still a basic research problem, because our understanding of atmosphere’s response to the external forcing remains still insufficient
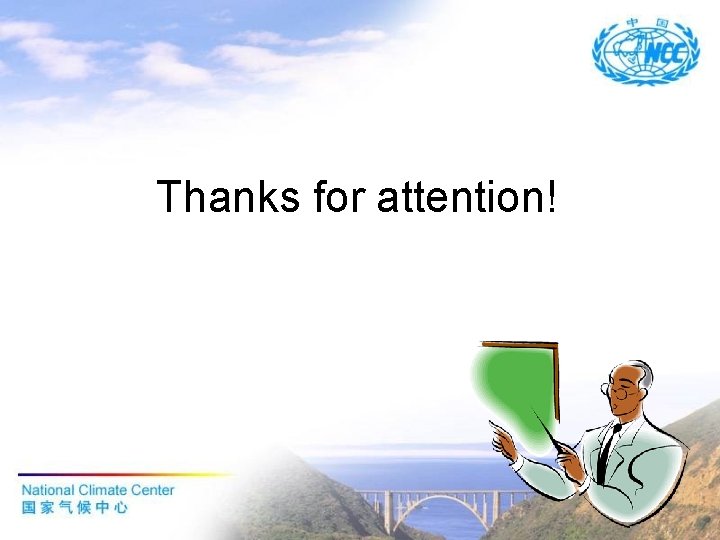
Thanks for attention!
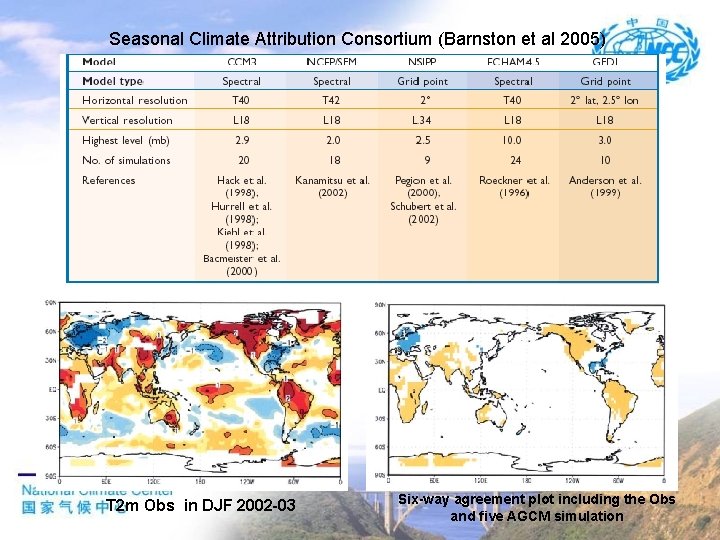
Seasonal Climate Attribution Consortium (Barnston et al 2005) T 2 m Obs in DJF 2002 -03 Six-way agreement plot including the Obs and five AGCM simulation
River valley civilizations
Penn state meteorology jobs
Vanuatu meteorology
Earthquake maldives
Department of meteorology maldives
Cpc africa desk
Climate change 2014 mitigation of climate change
Climatogram definition
Seasonal work meaning
Iri moore
Nurseries are seasonal pond
Deseasonalized demand formula
Mild persistent asthma
Seasonal movement definition
From statsmodels.tsa.seasonal import stl
Recognized seasonal employer visa
Why do globes lean sideways?
Symptomen van seasonal affective disorder
Where is the temperate forest located
Seasonal unemployment example
Seasonal round
Adaptations of deciduous forest