Sample image MODELING DIVERSE INTERACTIONS IN MULTIMODAL CORRIDORS
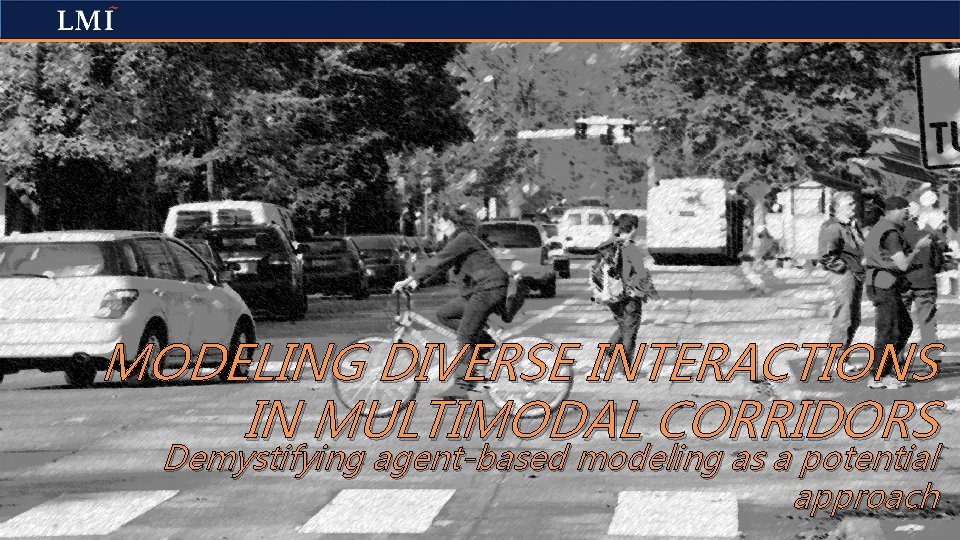
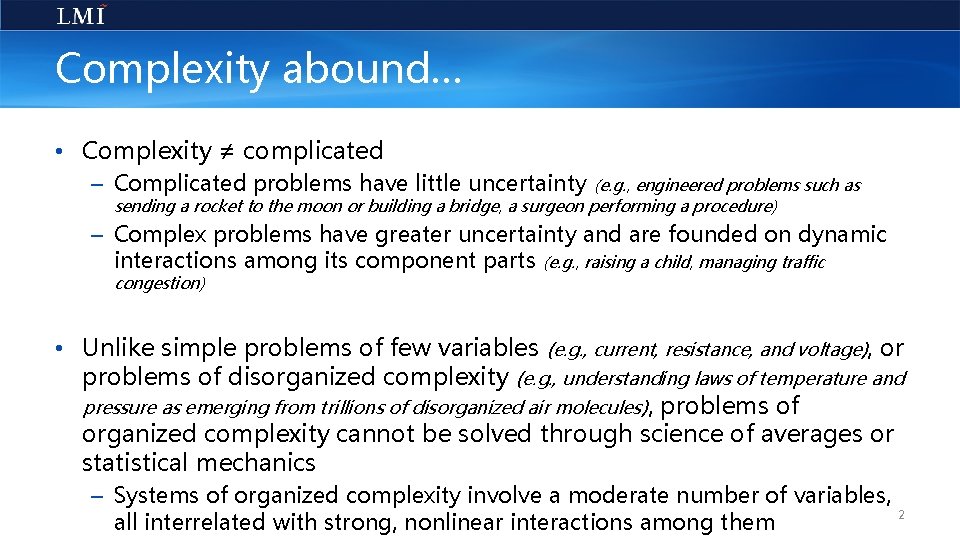
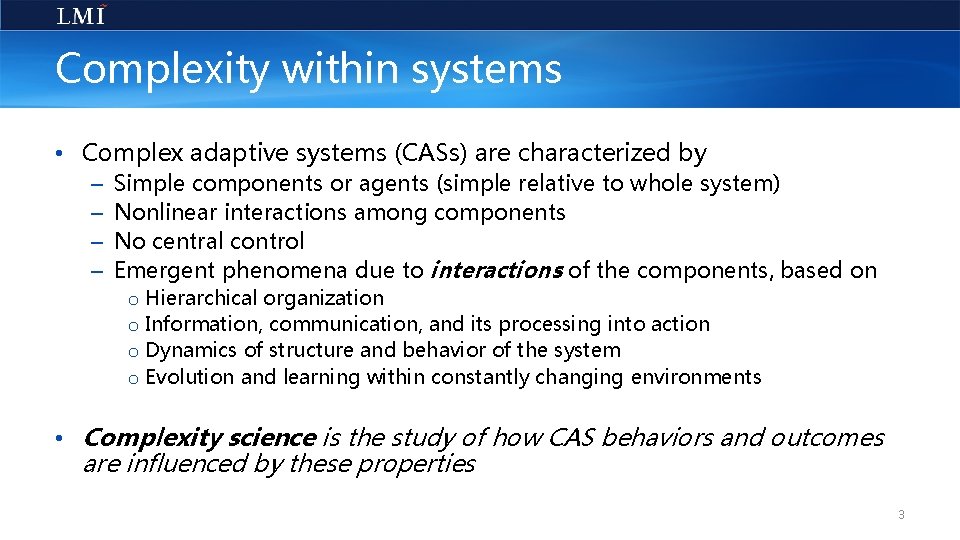
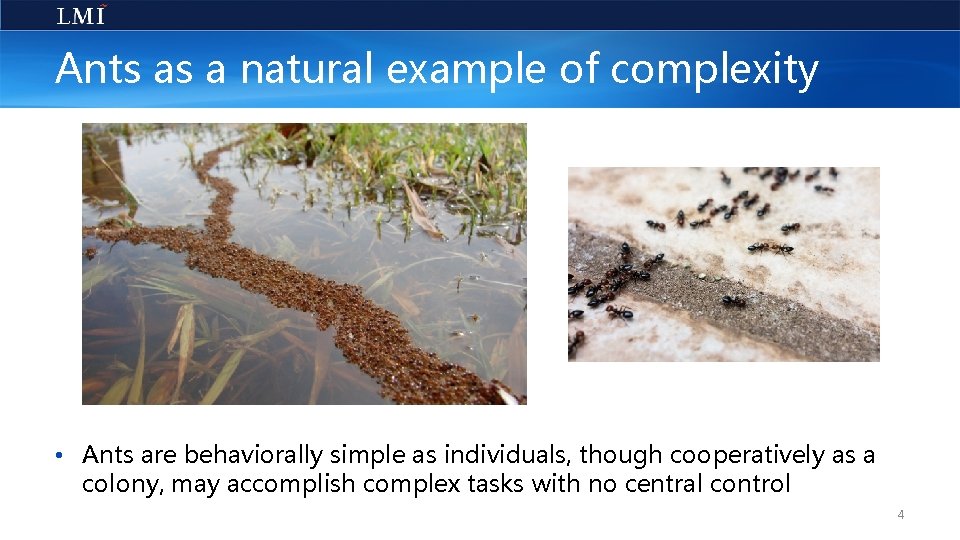
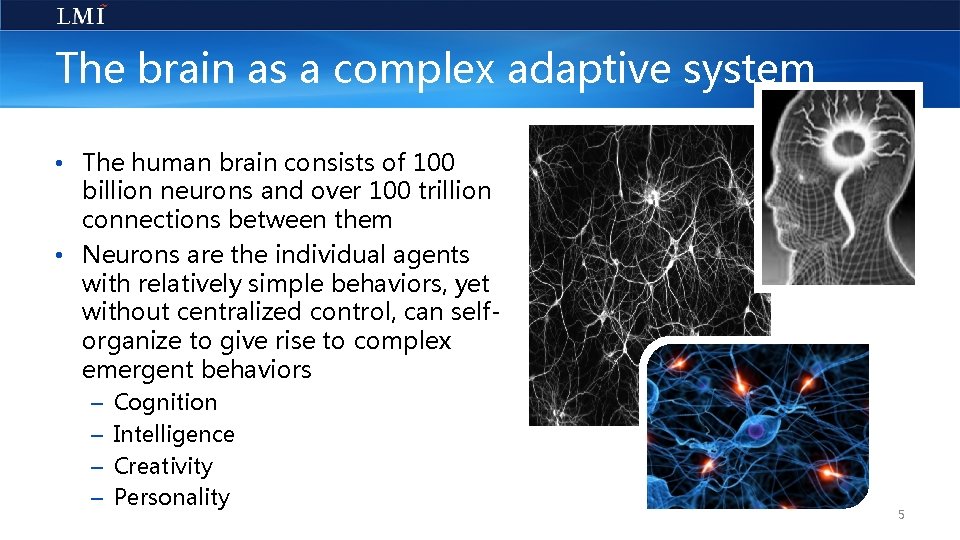
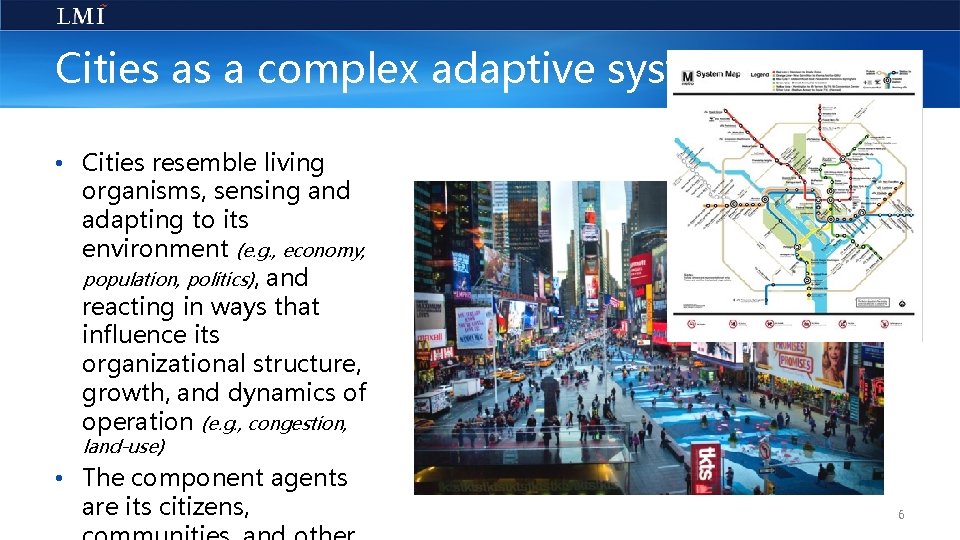
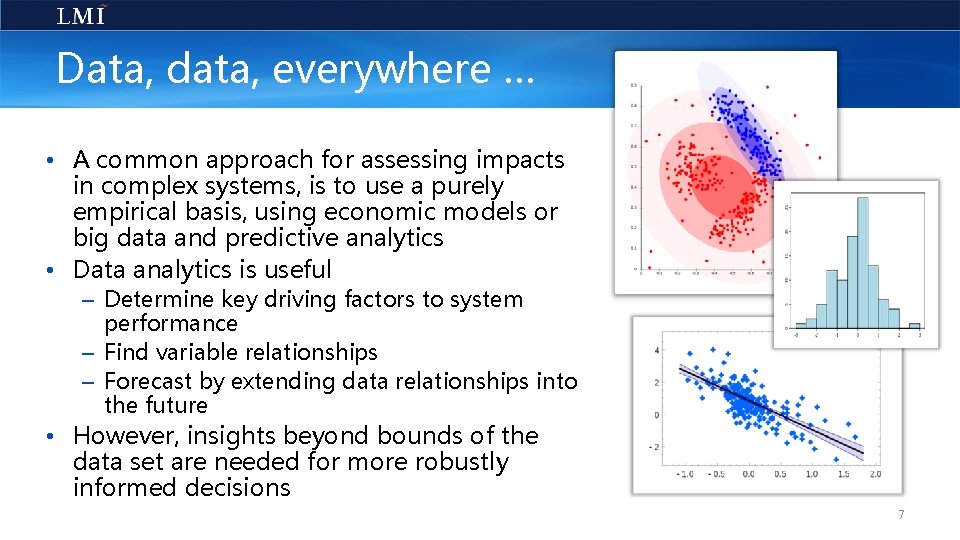
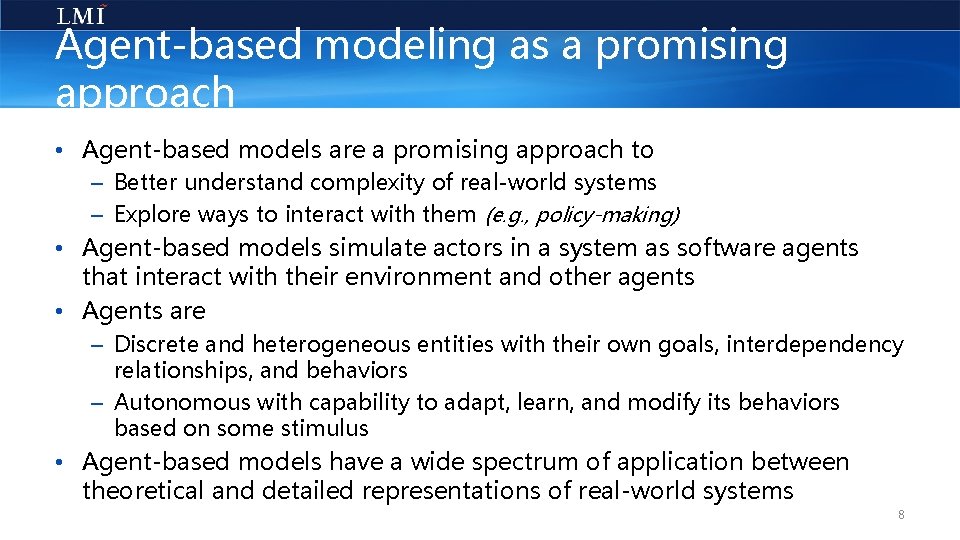
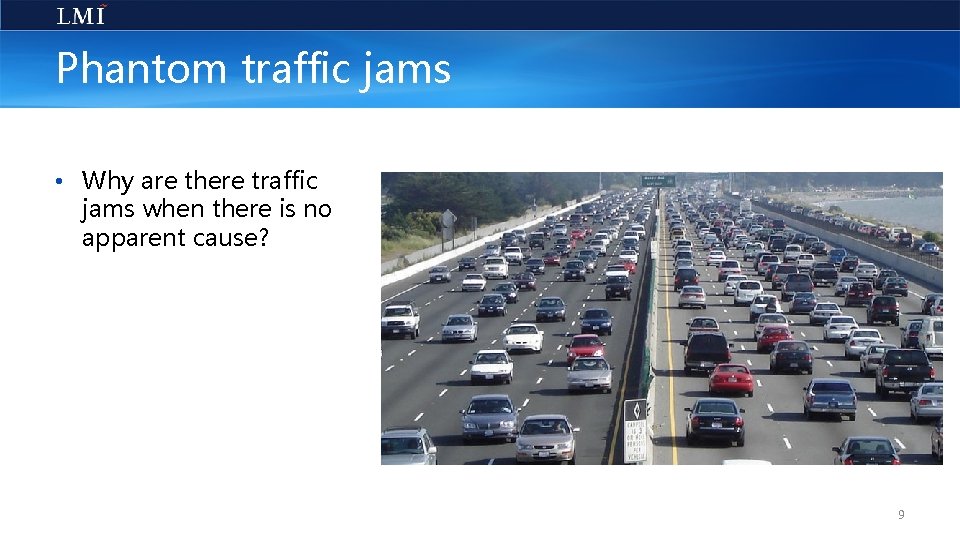
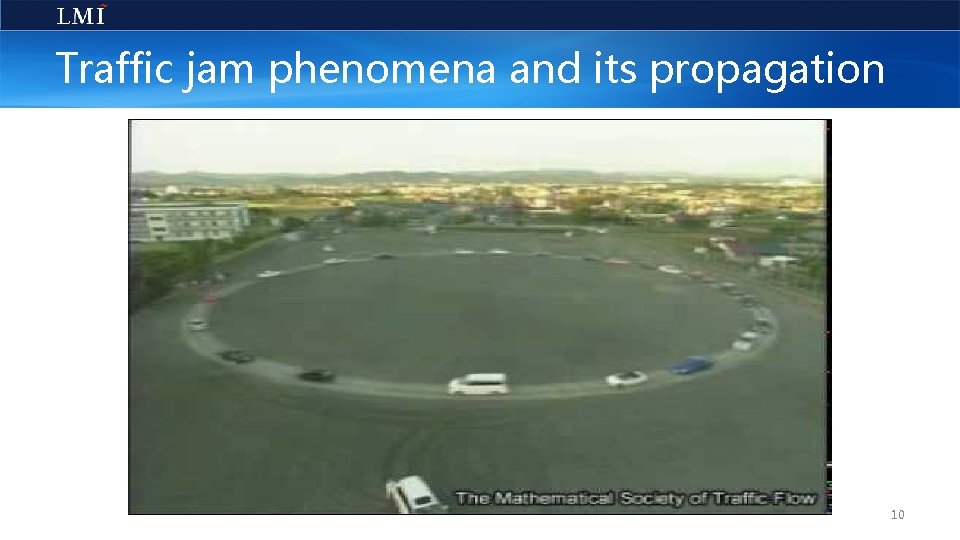
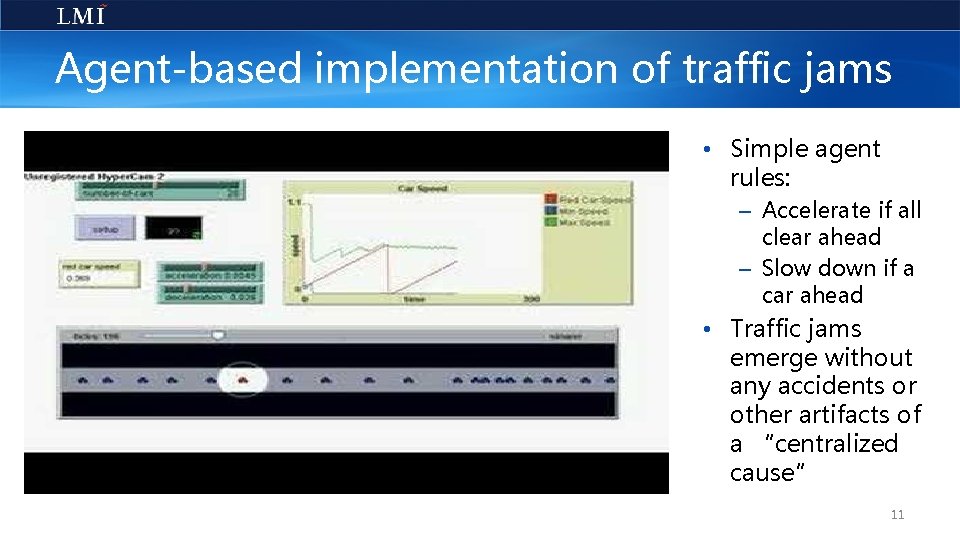
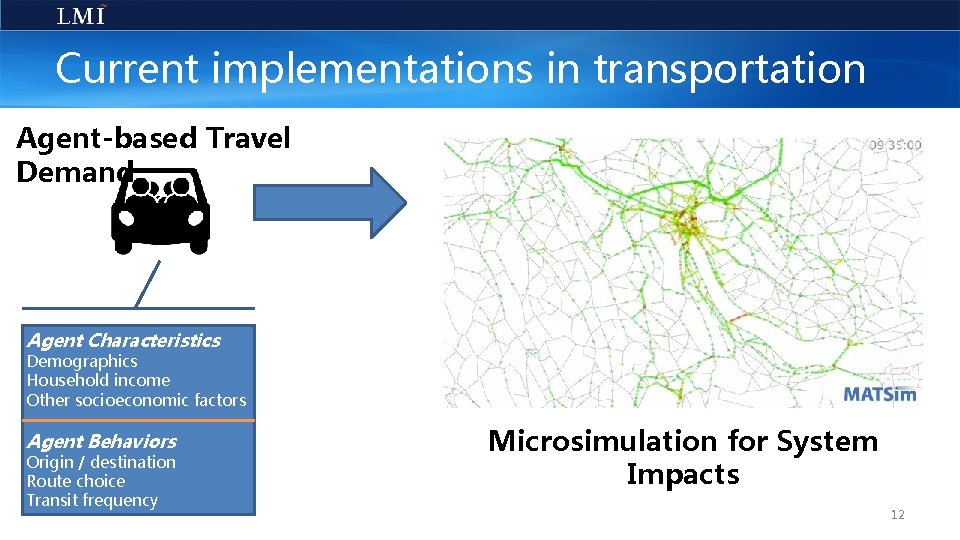
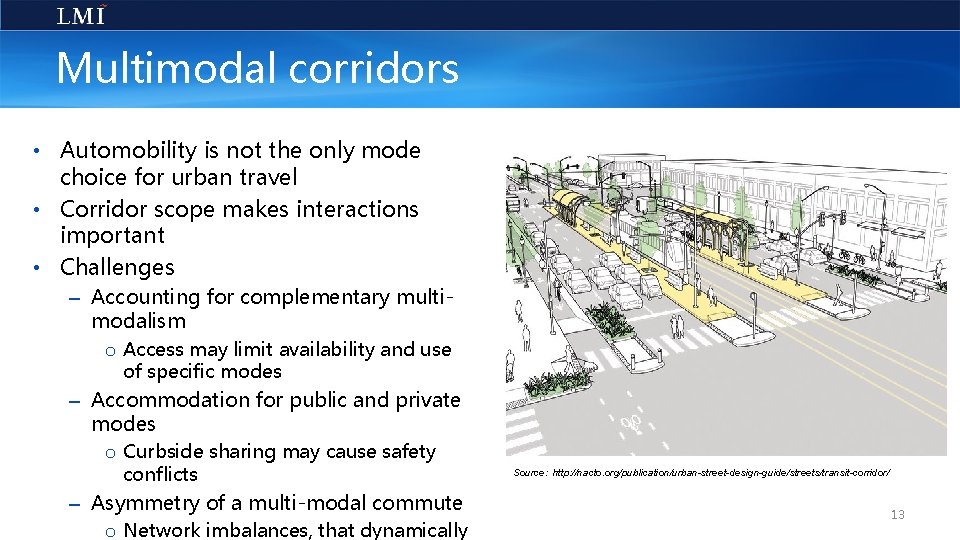
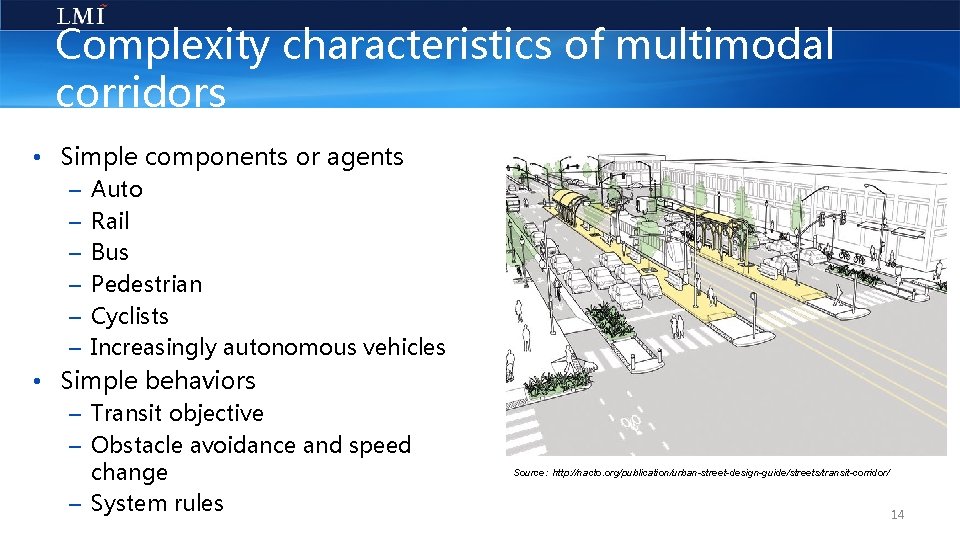
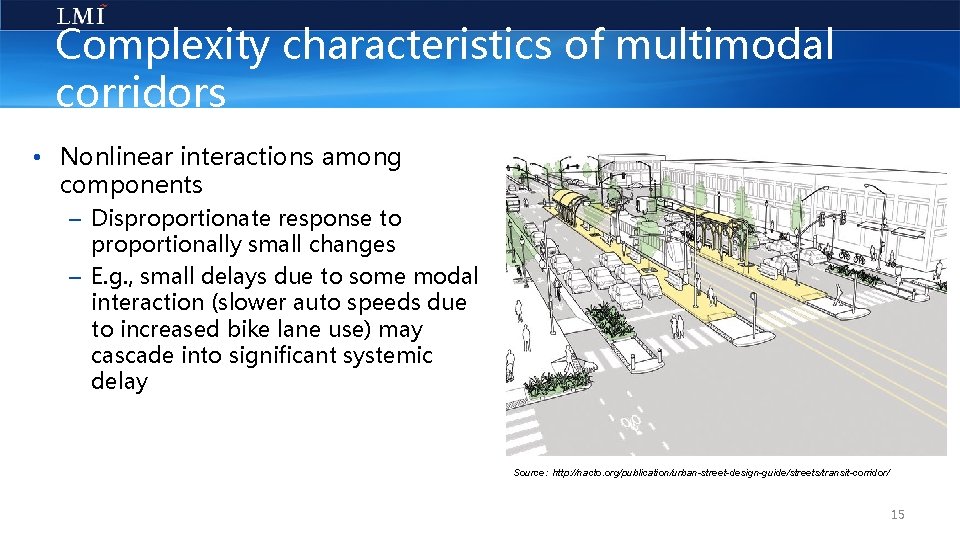
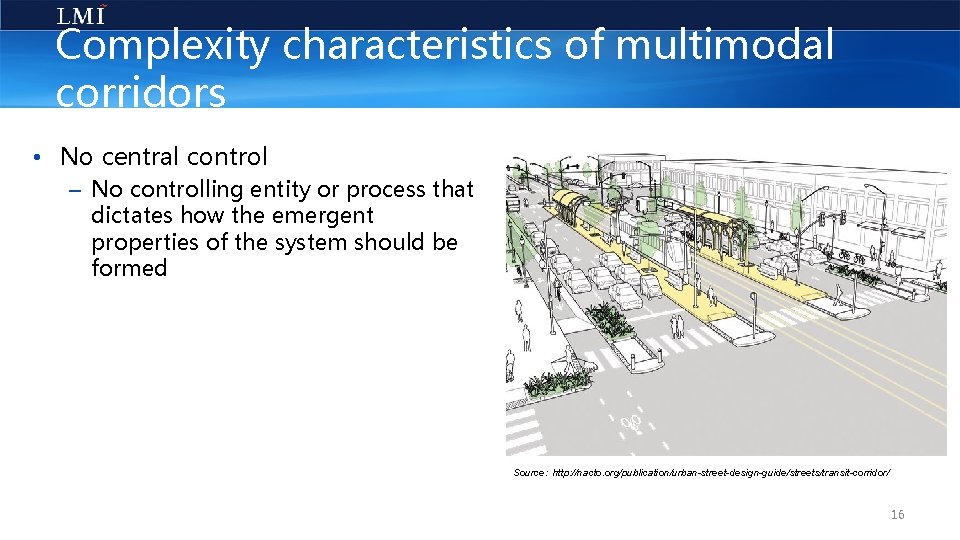
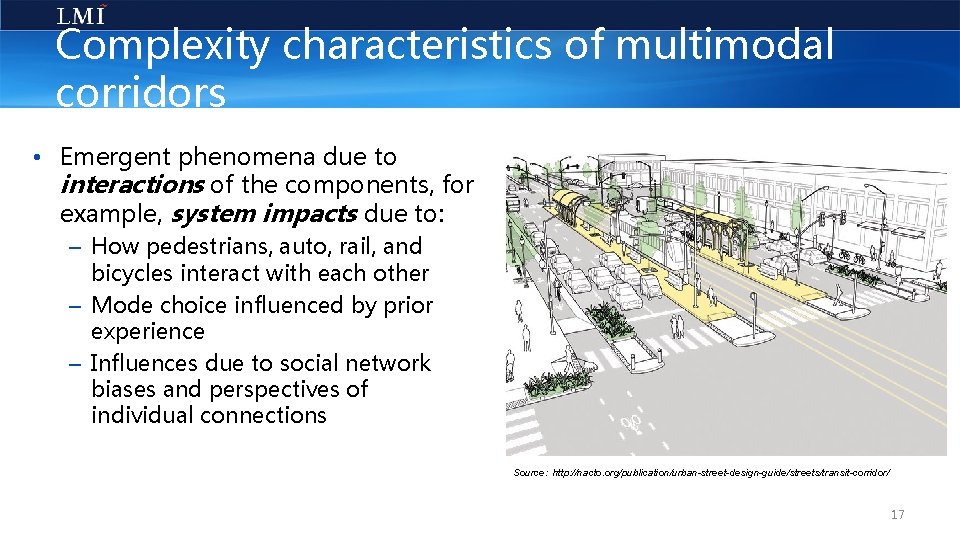
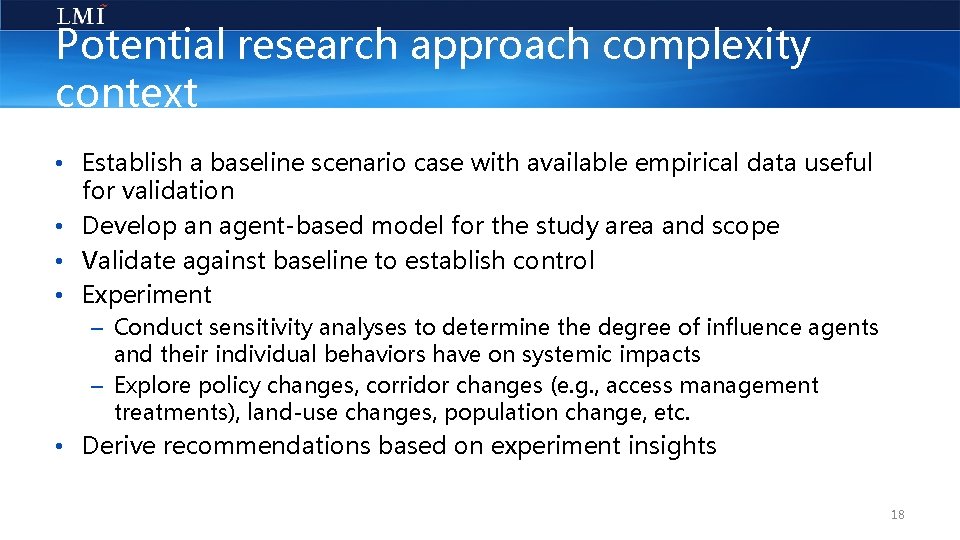
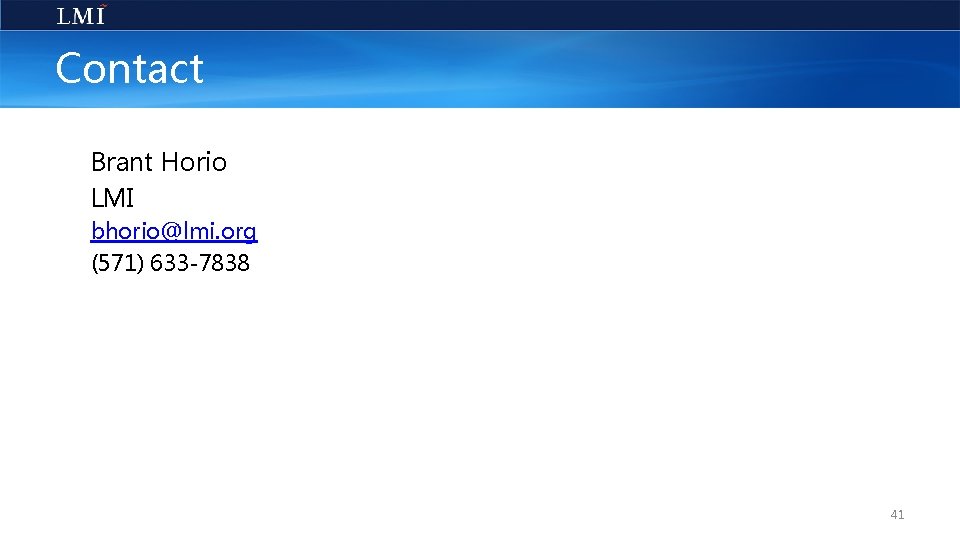
- Slides: 19
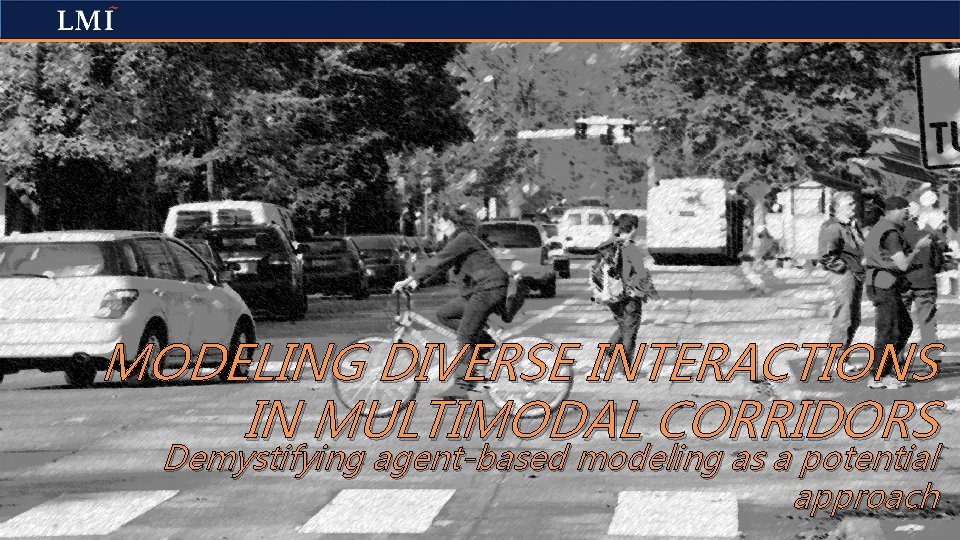
Sample image MODELING DIVERSE INTERACTIONS IN MULTIMODAL CORRIDORS Demystifying agent-based modeling as a potential approach
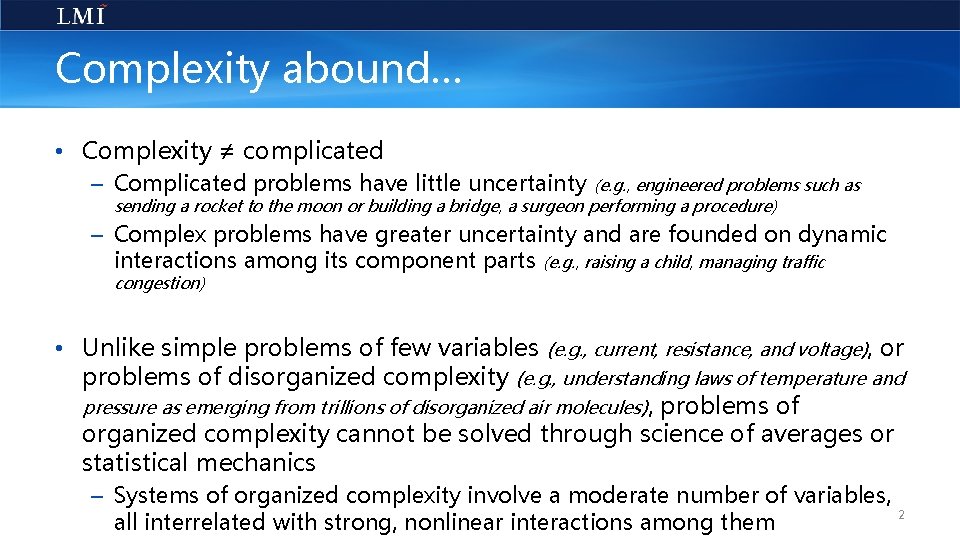
Complexity abound… • Complexity ≠ complicated – Complicated problems have little uncertainty (e. g. , engineered problems such as sending a rocket to the moon or building a bridge, a surgeon performing a procedure) – Complex problems have greater uncertainty and are founded on dynamic interactions among its component parts (e. g. , raising a child, managing traffic congestion) • Unlike simple problems of few variables (e. g. , current, resistance, and voltage), or problems of disorganized complexity (e. g. , understanding laws of temperature and pressure as emerging from trillions of disorganized air molecules) , problems of organized complexity cannot be solved through science of averages or statistical mechanics – Systems of organized complexity involve a moderate number of variables, 2 all interrelated with strong, nonlinear interactions among them
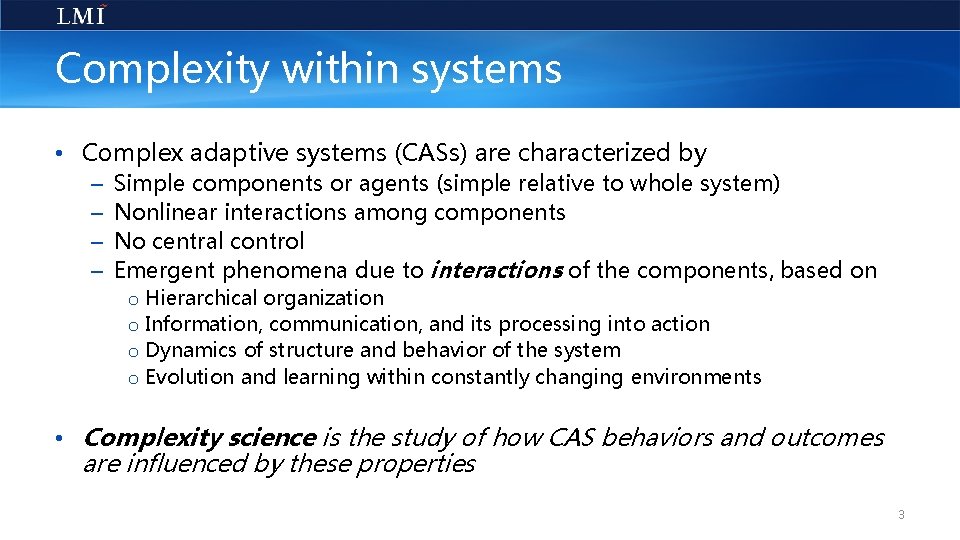
Complexity within systems • Complex adaptive systems (CASs) are characterized by – – Simple components or agents (simple relative to whole system) Nonlinear interactions among components No central control Emergent phenomena due to interactions of the components, based on o Hierarchical organization o Information, communication, and its processing into action o Dynamics of structure and behavior of the system o Evolution and learning within constantly changing environments • Complexity science is the study of how CAS behaviors and outcomes are influenced by these properties 3
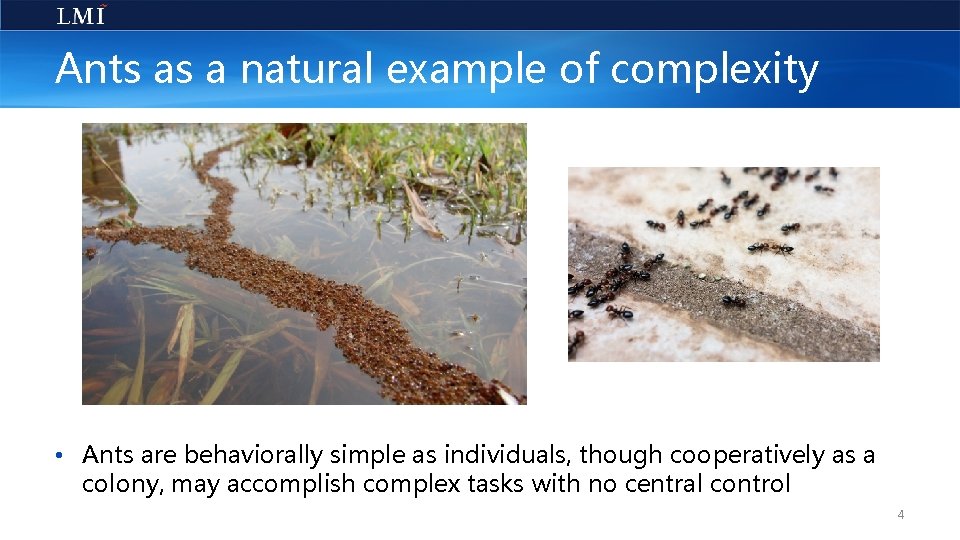
Ants as a natural example of complexity • Ants are behaviorally simple as individuals, though cooperatively as a colony, may accomplish complex tasks with no central control 4
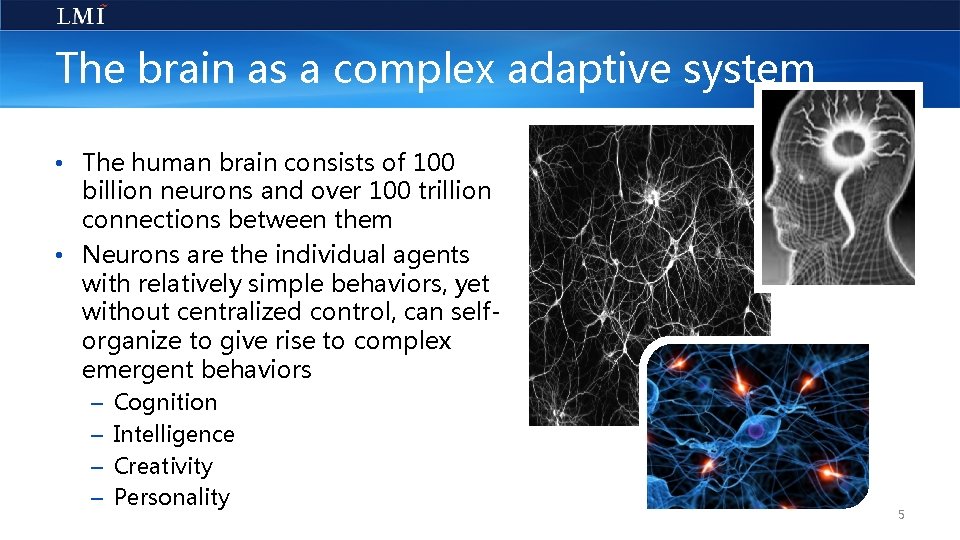
The brain as a complex adaptive system • The human brain consists of 100 billion neurons and over 100 trillion connections between them • Neurons are the individual agents with relatively simple behaviors, yet without centralized control, can selforganize to give rise to complex emergent behaviors – – Cognition Intelligence Creativity Personality 5
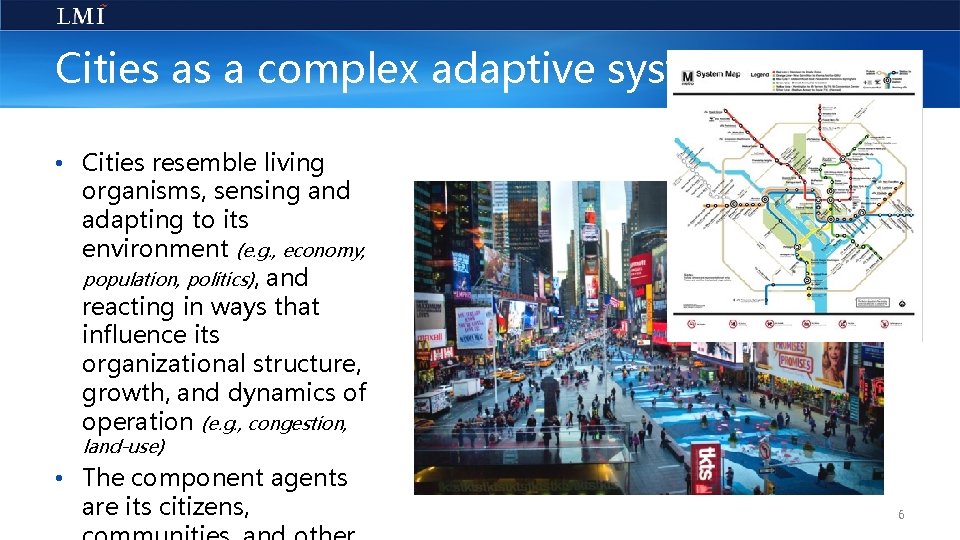
Cities as a complex adaptive system • Cities resemble living organisms, sensing and adapting to its environment (e. g. , economy, population, politics), and reacting in ways that influence its organizational structure, growth, and dynamics of operation (e. g. , congestion, land-use) • The component agents are its citizens, 6
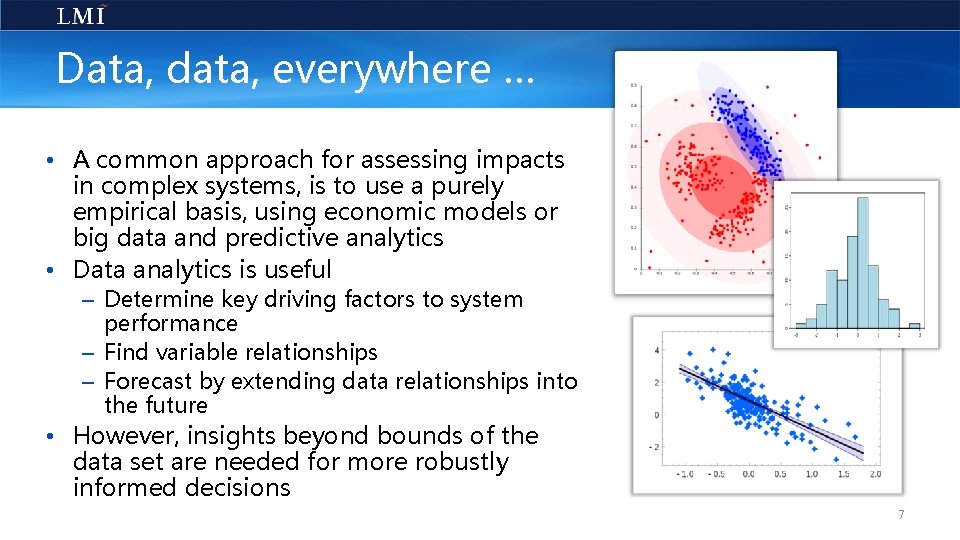
Data, data, everywhere … • A common approach for assessing impacts in complex systems, is to use a purely empirical basis, using economic models or big data and predictive analytics • Data analytics is useful – Determine key driving factors to system performance – Find variable relationships – Forecast by extending data relationships into the future • However, insights beyond bounds of the data set are needed for more robustly informed decisions 7
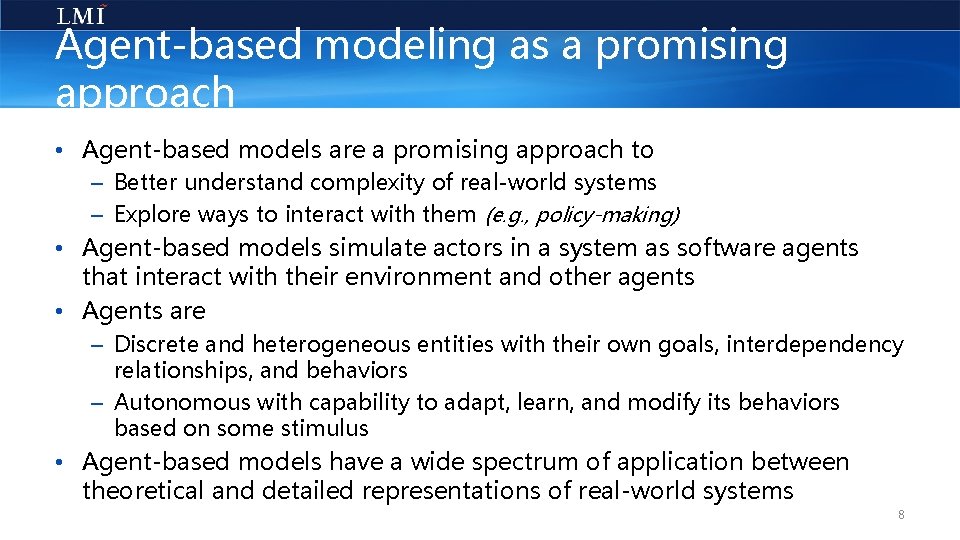
Agent-based modeling as a promising approach • Agent-based models are a promising approach to – Better understand complexity of real-world systems – Explore ways to interact with them (e. g. , policy-making) • Agent-based models simulate actors in a system as software agents that interact with their environment and other agents • Agents are – Discrete and heterogeneous entities with their own goals, interdependency relationships, and behaviors – Autonomous with capability to adapt, learn, and modify its behaviors based on some stimulus • Agent-based models have a wide spectrum of application between theoretical and detailed representations of real-world systems 8
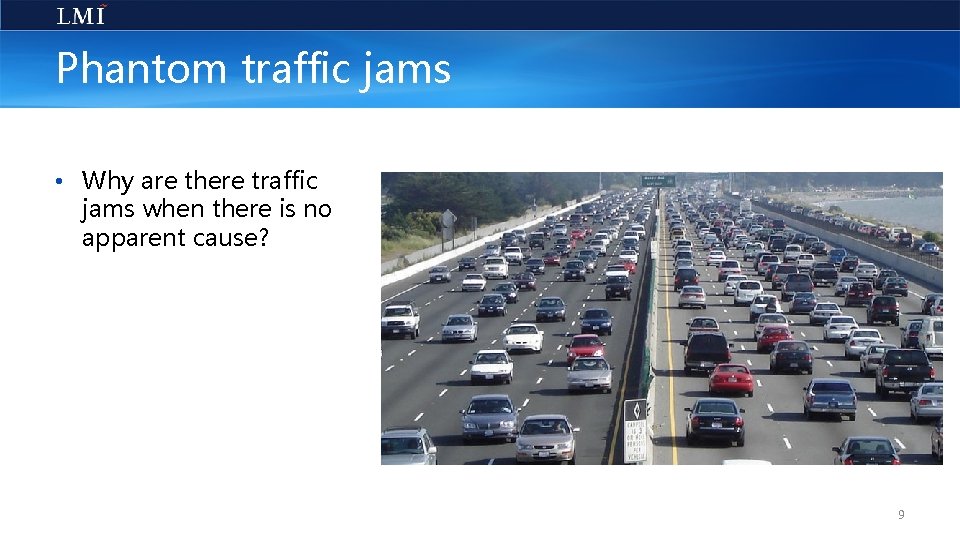
Phantom traffic jams • Why are there traffic jams when there is no apparent cause? 9
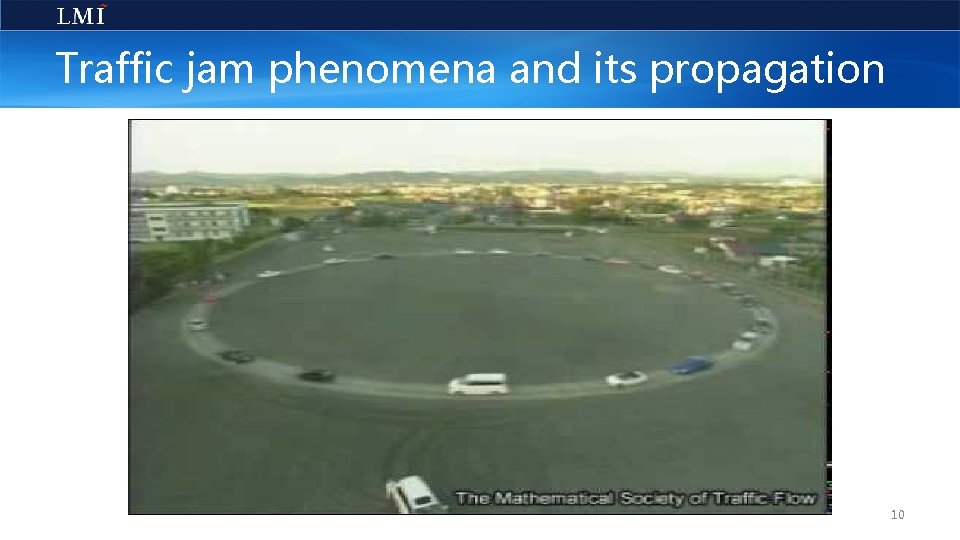
Traffic jam phenomena and its propagation 10
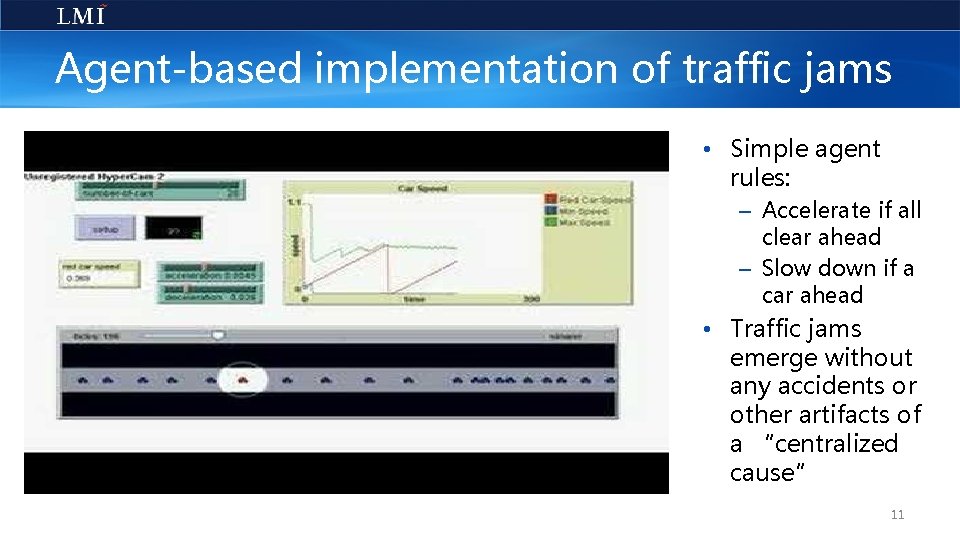
Agent-based implementation of traffic jams • Simple agent rules: – Accelerate if all clear ahead – Slow down if a car ahead • Traffic jams emerge without any accidents or other artifacts of a “centralized cause” 11
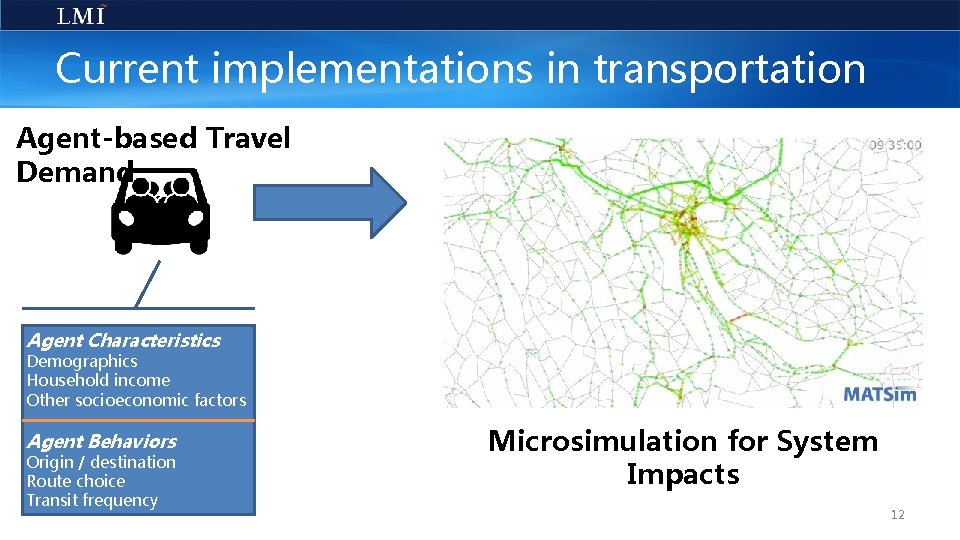
Current implementations in transportation Agent-based Travel Demand Agent Characteristics Demographics Household income Other socioeconomic factors Agent Behaviors Origin / destination Route choice Transit frequency Microsimulation for System Impacts 12
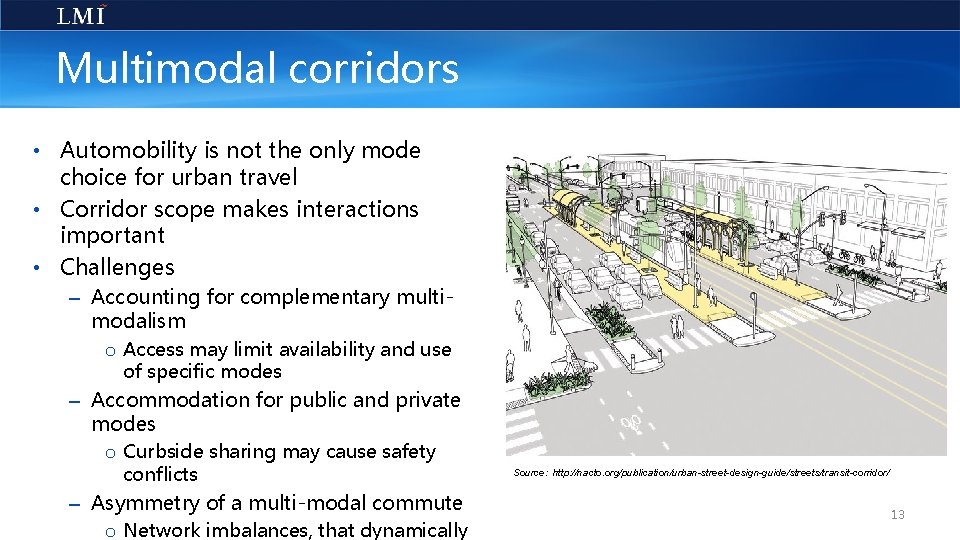
Multimodal corridors • Automobility is not the only mode choice for urban travel • Corridor scope makes interactions important • Challenges – Accounting for complementary multimodalism o Access may limit availability and use of specific modes – Accommodation for public and private modes o Curbside sharing may cause safety conflicts – Asymmetry of a multi-modal commute o Network imbalances, that dynamically Source: http: //nacto. org/publication/urban-street-design-guide/streets/transit-corridor/ 13
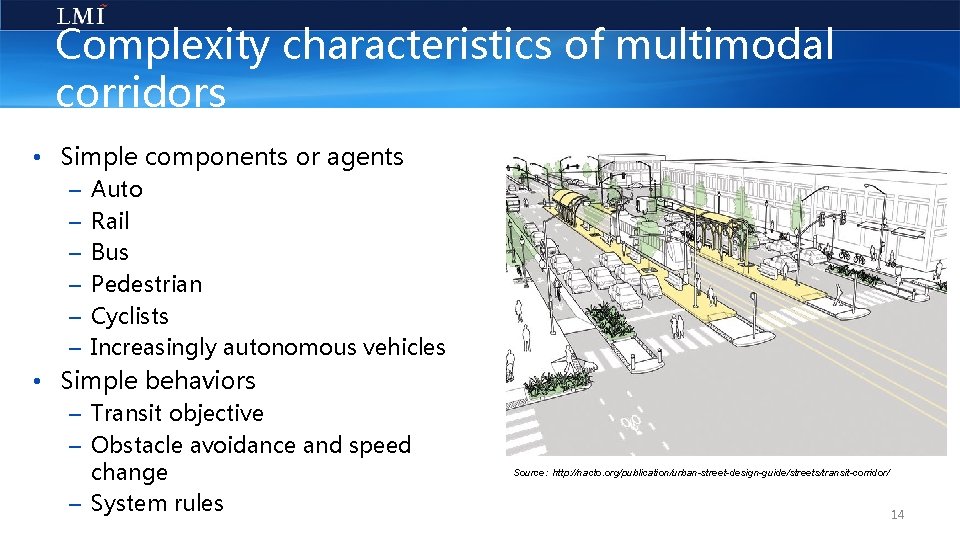
Complexity characteristics of multimodal corridors • Simple components or agents – – – Auto Rail Bus Pedestrian Cyclists Increasingly autonomous vehicles • Simple behaviors – Transit objective – Obstacle avoidance and speed change – System rules Source: http: //nacto. org/publication/urban-street-design-guide/streets/transit-corridor/ 14
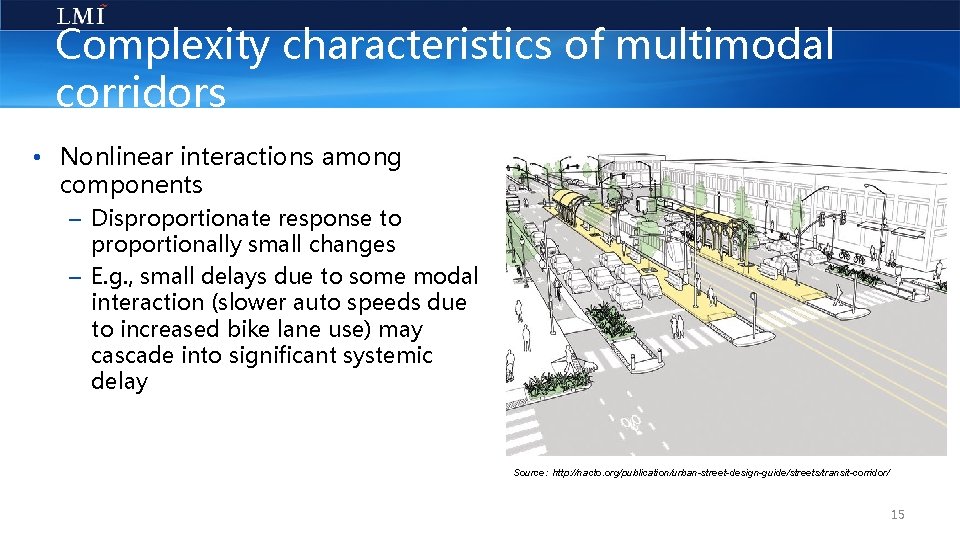
Complexity characteristics of multimodal corridors • Nonlinear interactions among components – Disproportionate response to proportionally small changes – E. g. , small delays due to some modal interaction (slower auto speeds due to increased bike lane use) may cascade into significant systemic delay Source: http: //nacto. org/publication/urban-street-design-guide/streets/transit-corridor/ 15
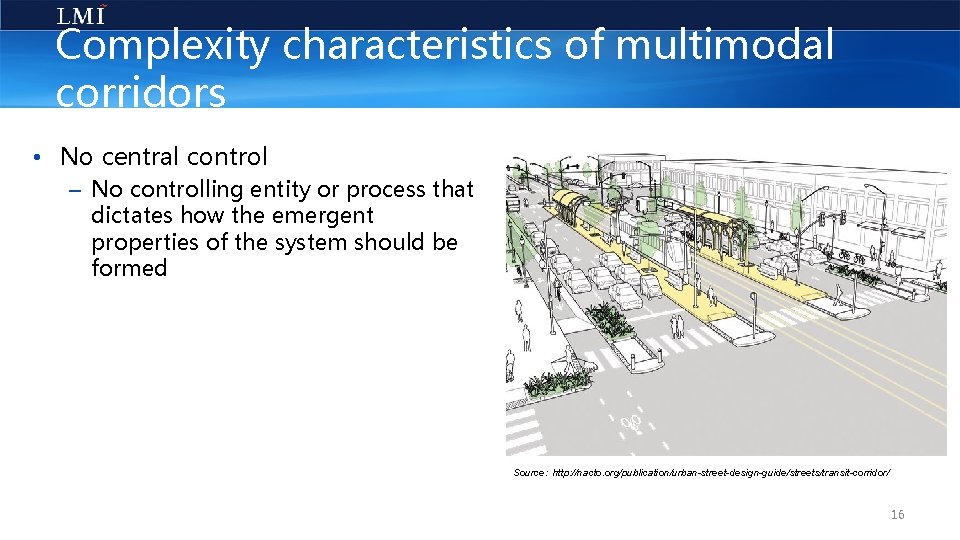
Complexity characteristics of multimodal corridors • No central control – No controlling entity or process that dictates how the emergent properties of the system should be formed Source: http: //nacto. org/publication/urban-street-design-guide/streets/transit-corridor/ 16
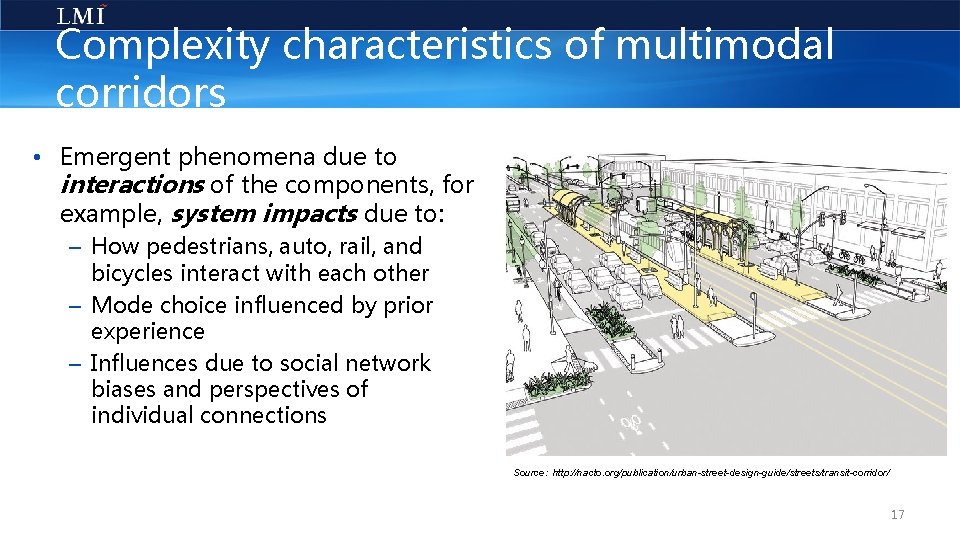
Complexity characteristics of multimodal corridors • Emergent phenomena due to interactions of the components, for example, system impacts due to: – How pedestrians, auto, rail, and bicycles interact with each other – Mode choice influenced by prior experience – Influences due to social network biases and perspectives of individual connections Source: http: //nacto. org/publication/urban-street-design-guide/streets/transit-corridor/ 17
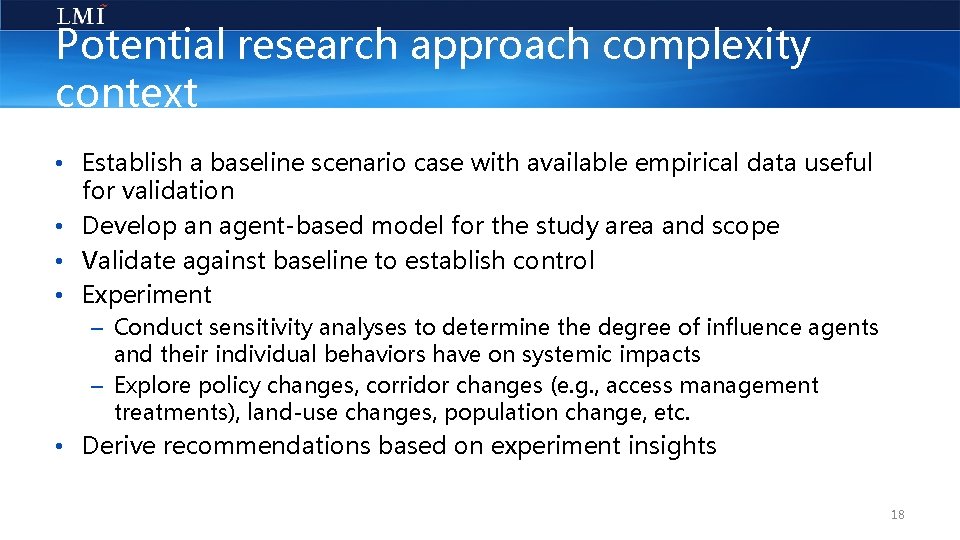
Potential research approach complexity context • Establish a baseline scenario case with available empirical data useful for validation • Develop an agent-based model for the study area and scope • Validate against baseline to establish control • Experiment – Conduct sensitivity analyses to determine the degree of influence agents and their individual behaviors have on systemic impacts – Explore policy changes, corridor changes (e. g. , access management treatments), land-use changes, population change, etc. • Derive recommendations based on experiment insights 18
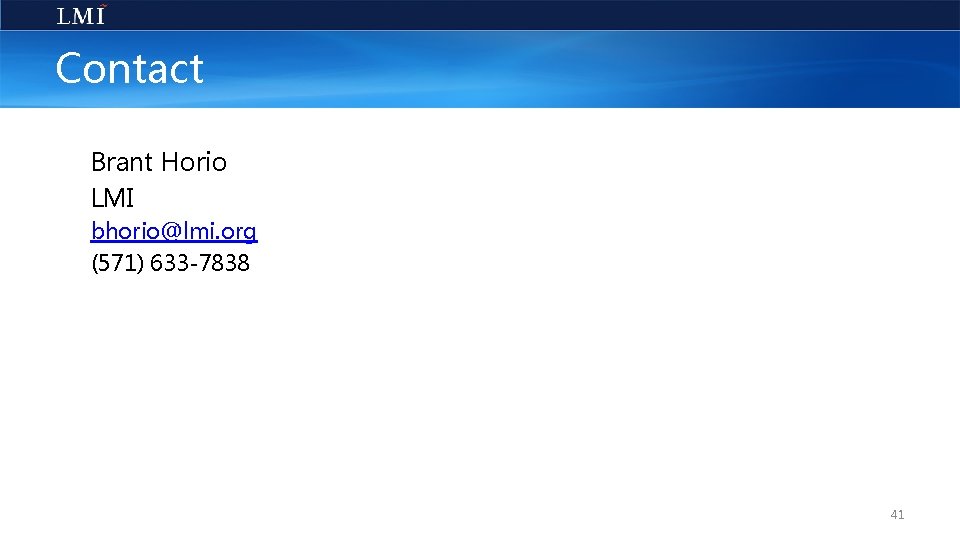
Contact Brant Horio LMI bhorio@lmi. org (571) 633 -7838 41
Diversity of protists
Tent corridors
Safe corridors law
Tent corridors
Helen c. erickson
Dimensional modeling vs relational modeling
Where can i upload images
Multimodal vs multimedia
The multimodal text big ed mona
Multimodal association areas
Lazarus multimodal therapy
Analysis of poem for my mother by jennifer davids
Cuáles son los componentes de la estrategia multimodal
Multimodal association areas
Multimodal rehabilitering
Tipos de texto multimodal
Transporte multimodal ventajas y desventajas
Multimodal integration
Multimodal composition
Multimodal tekst