QVals and False Discovery Rates Made Easy Dennis
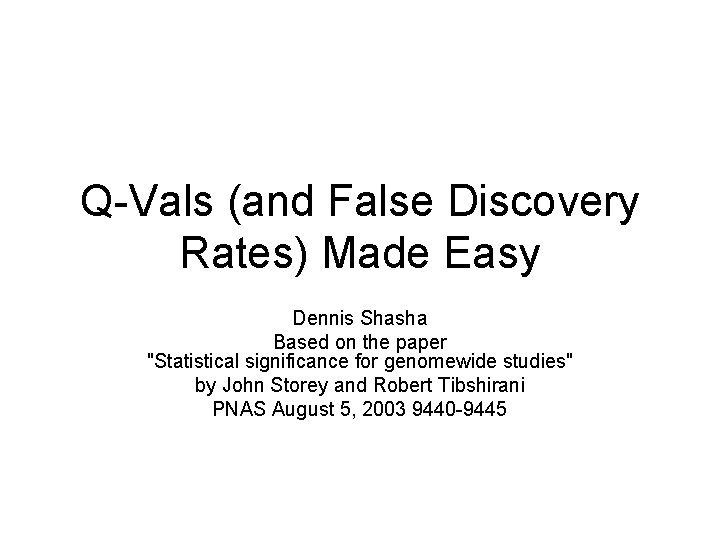
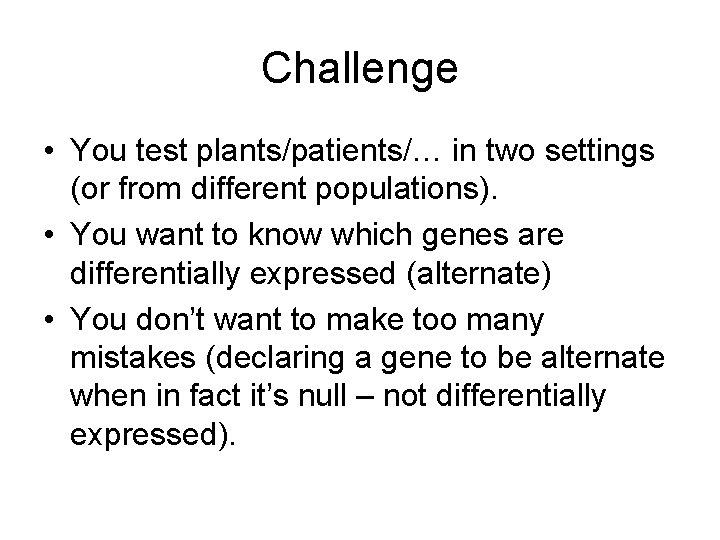
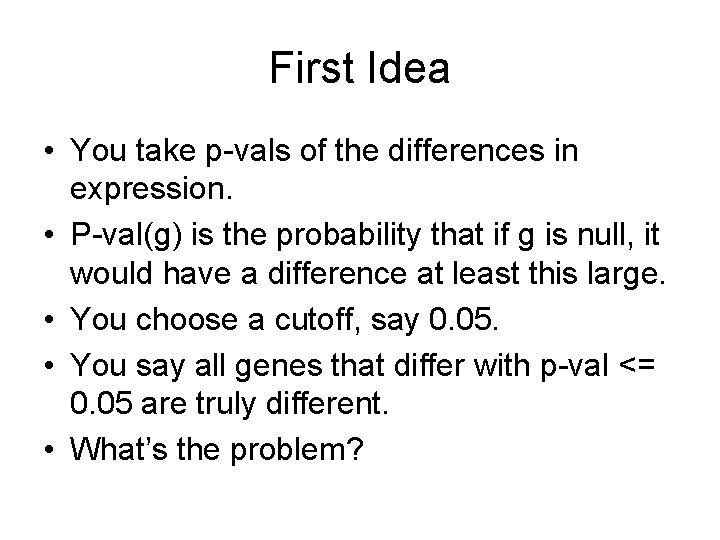
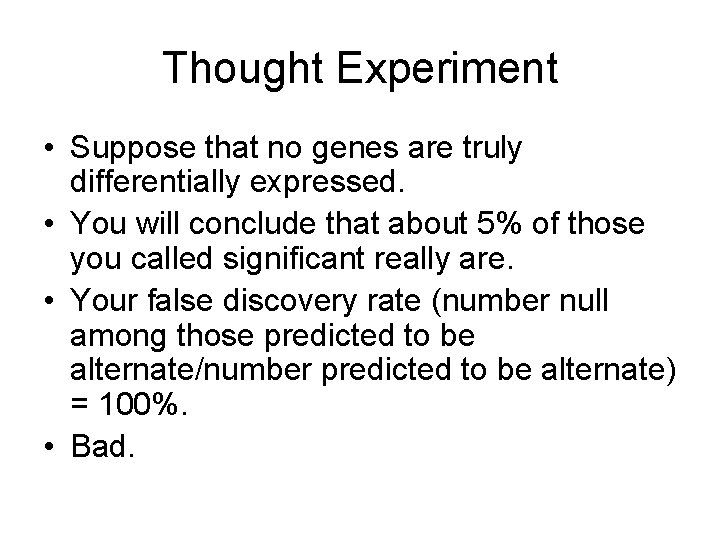
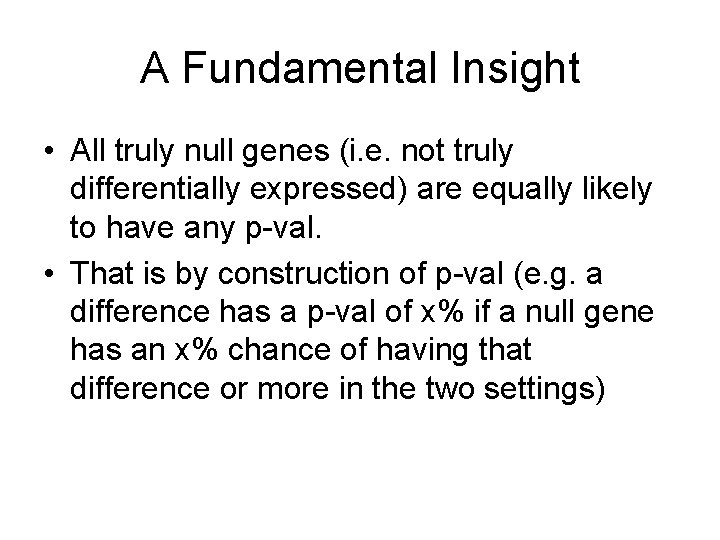
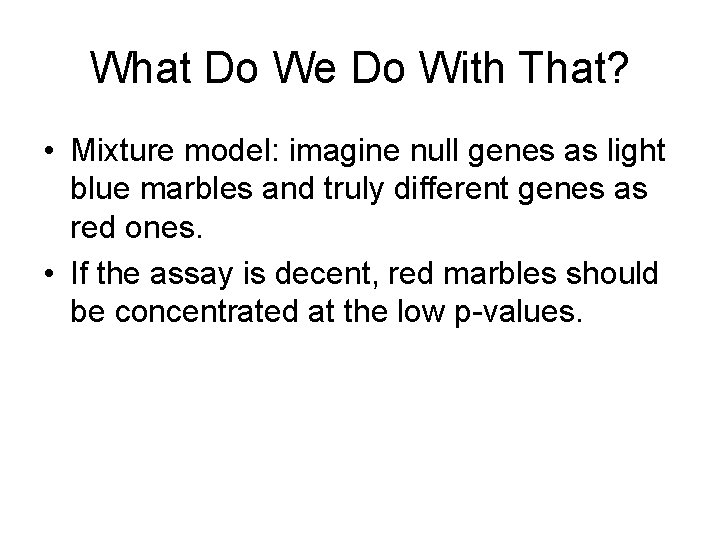
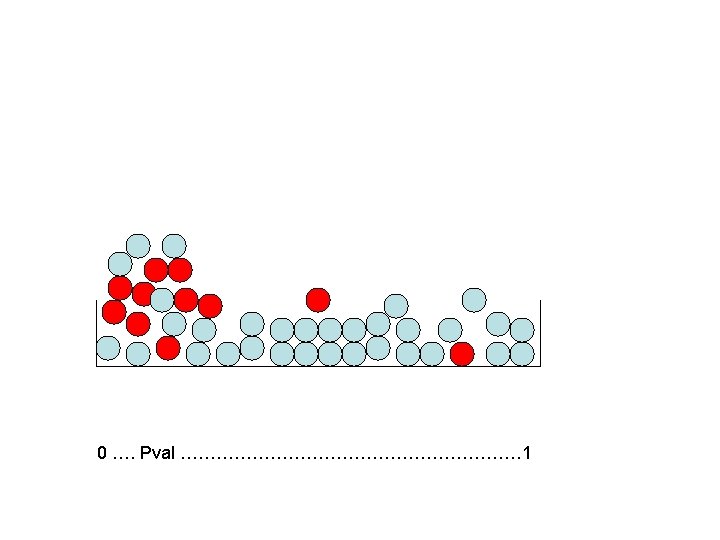
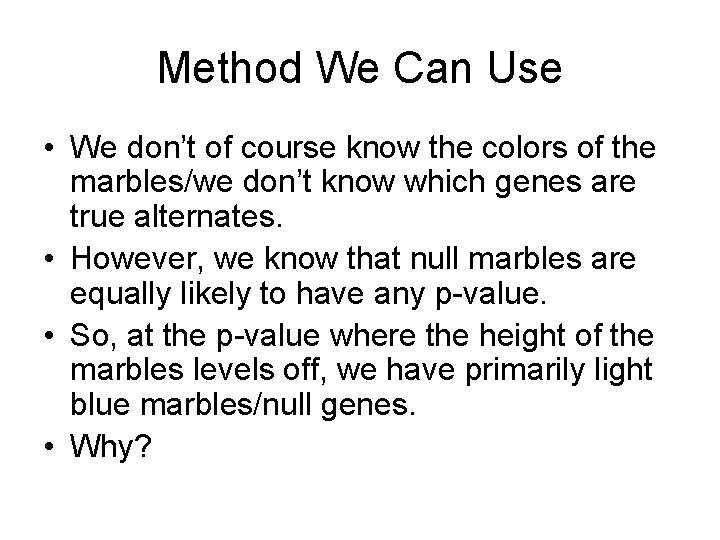
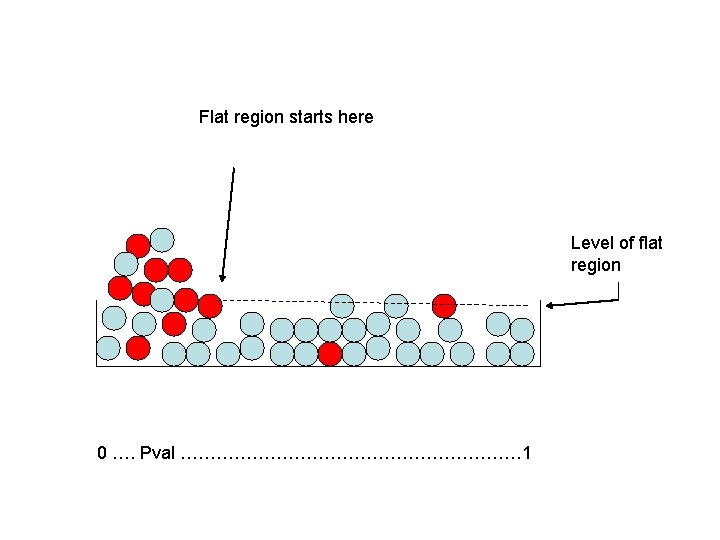
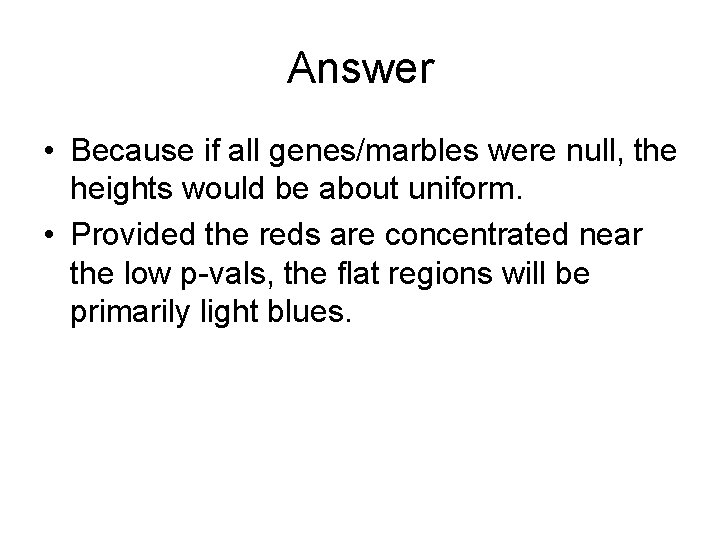
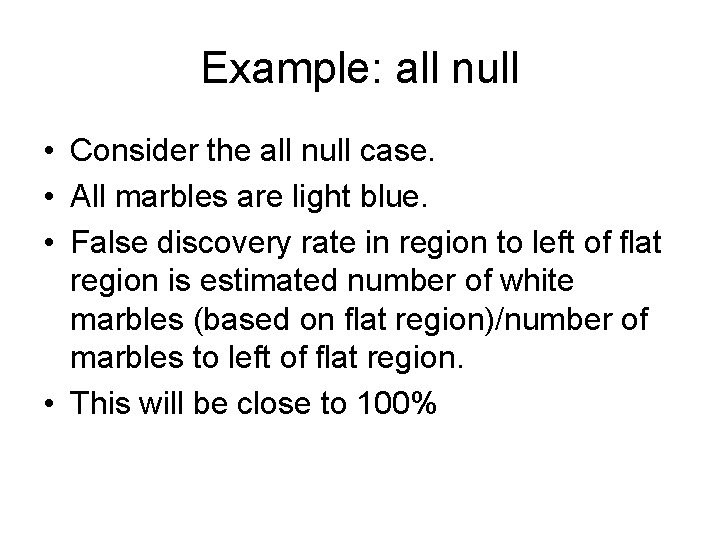
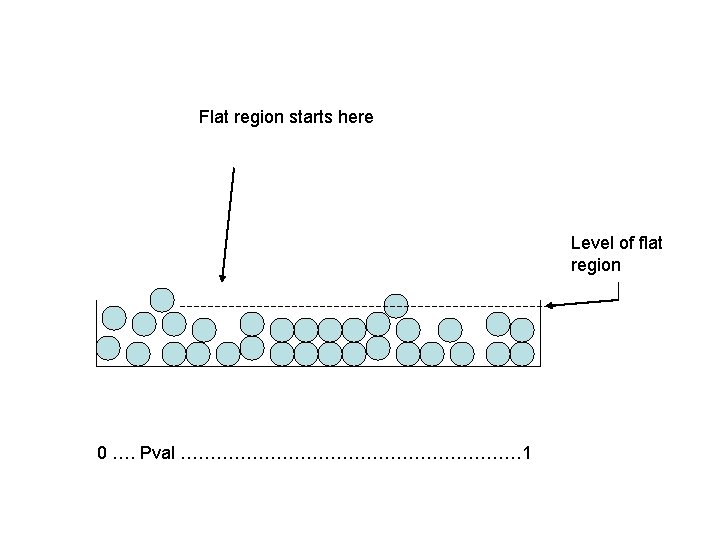
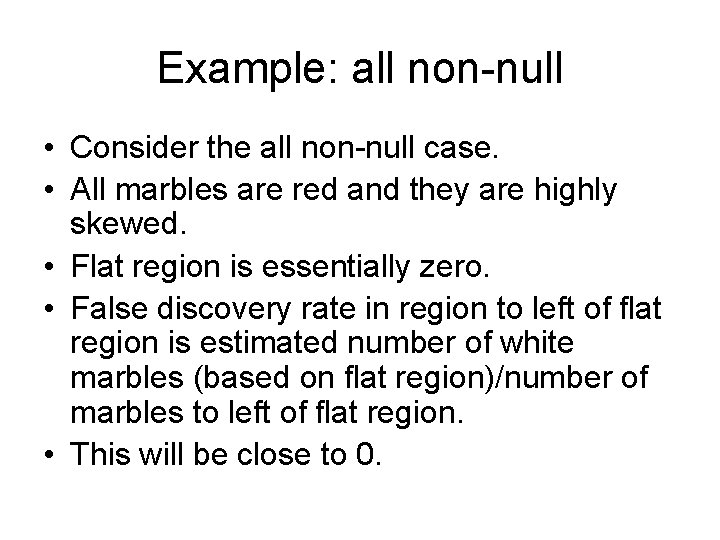
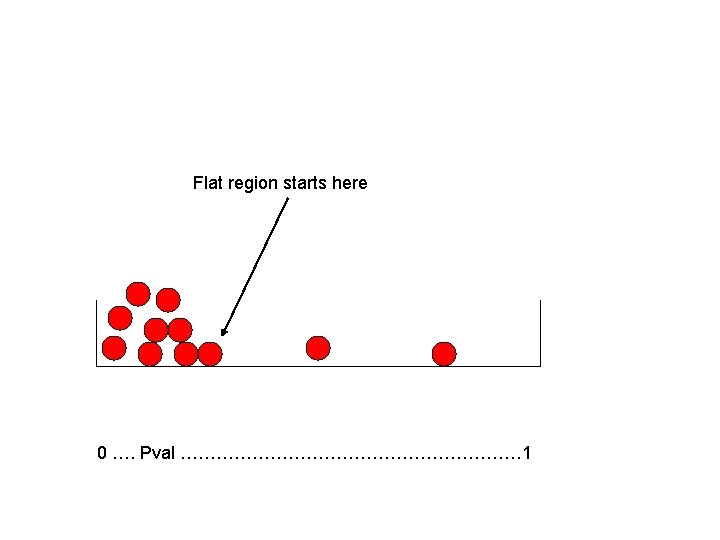
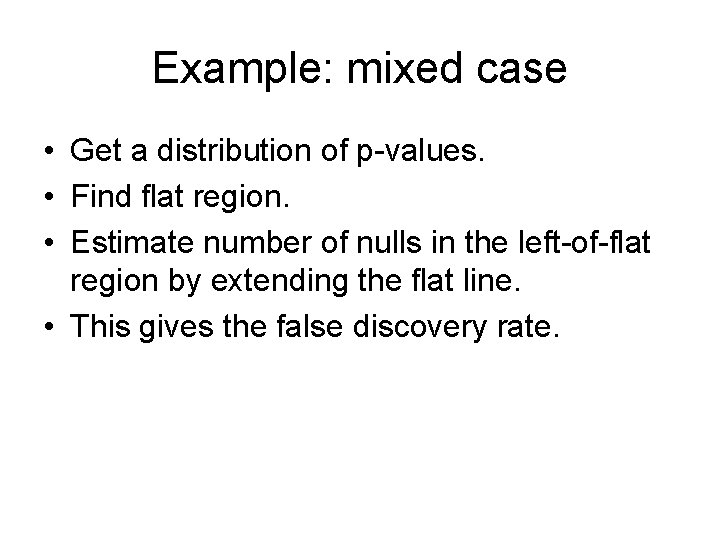
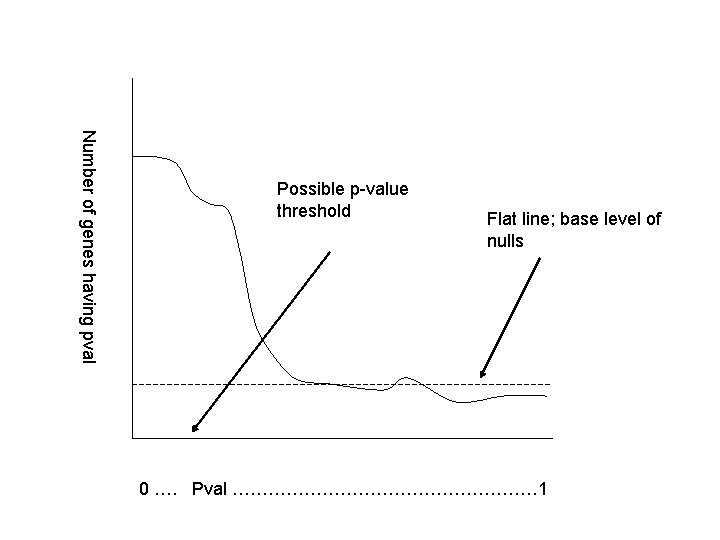
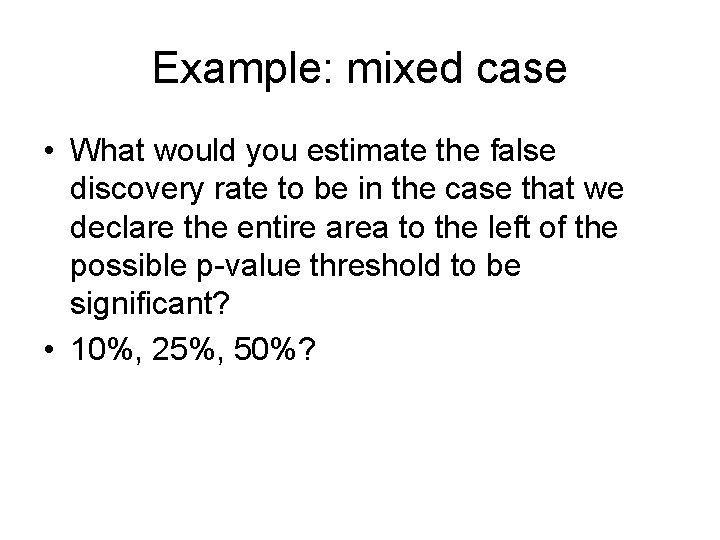
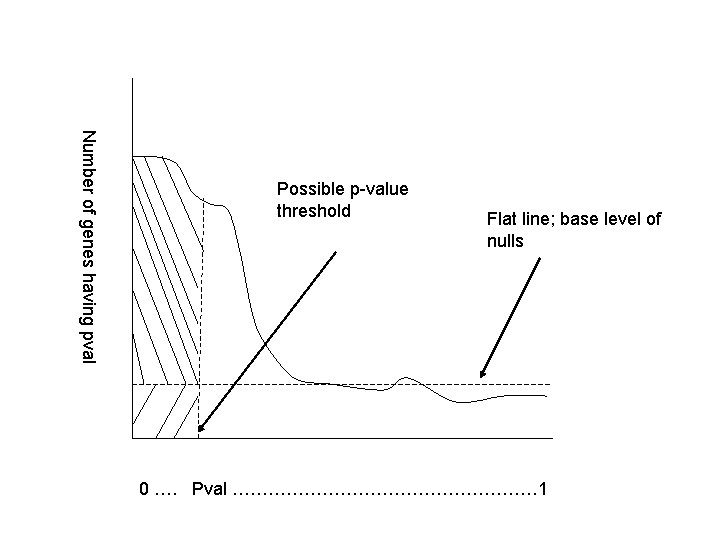
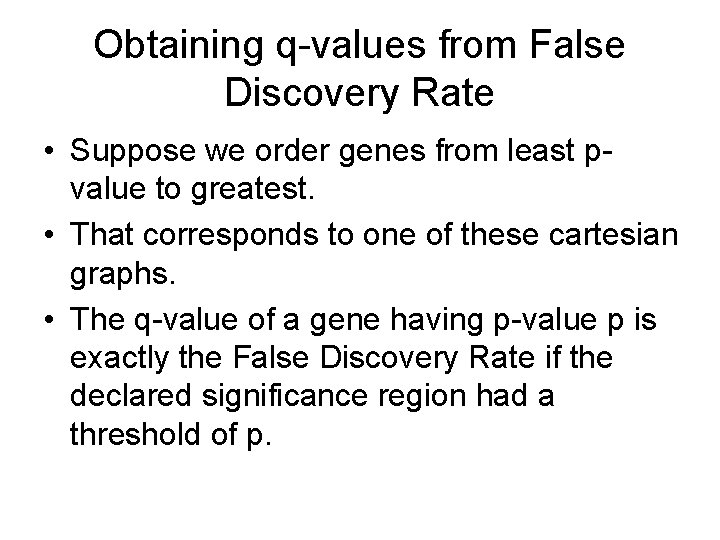
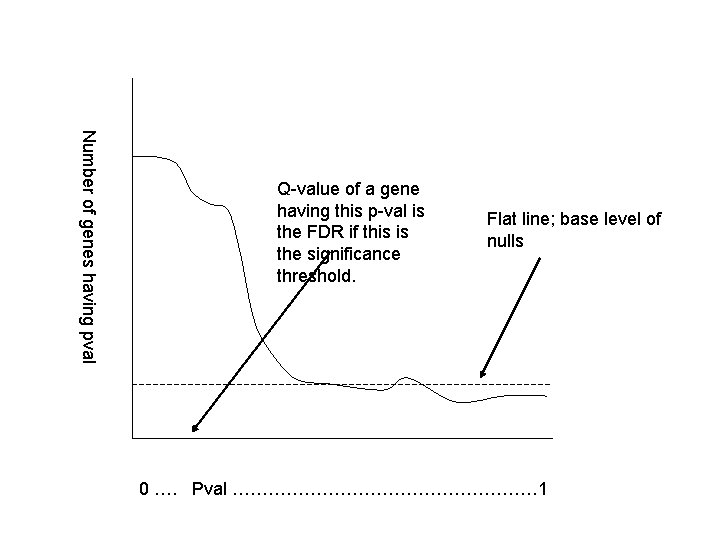
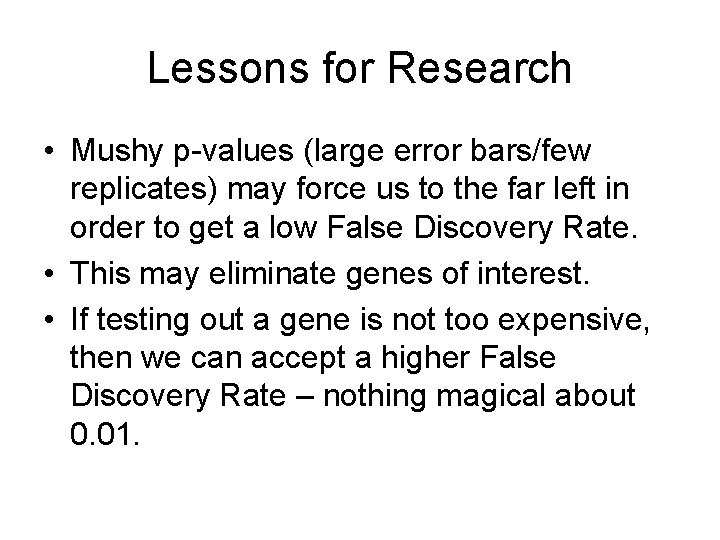
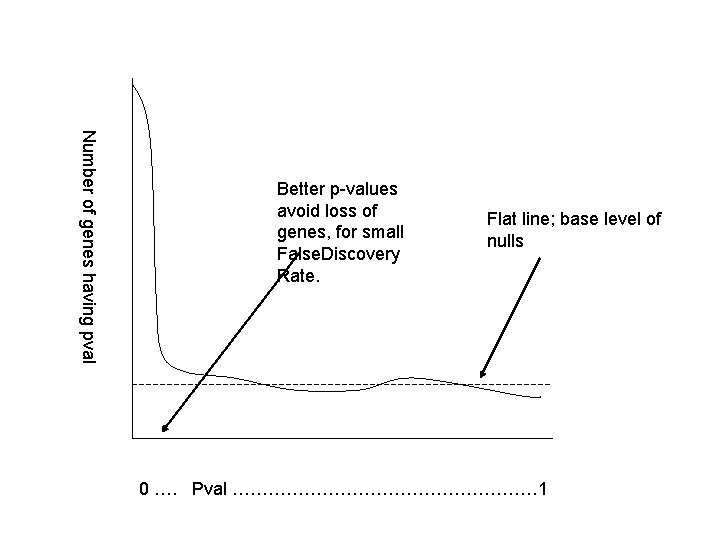
- Slides: 22
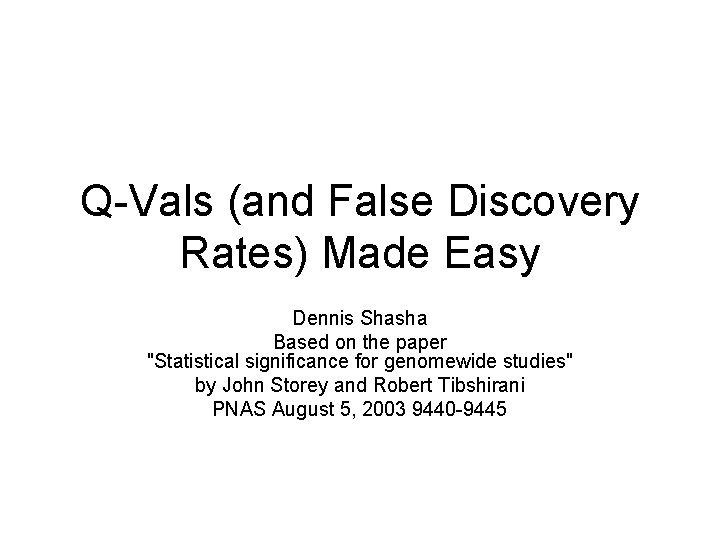
Q-Vals (and False Discovery Rates) Made Easy Dennis Shasha Based on the paper "Statistical significance for genomewide studies" by John Storey and Robert Tibshirani PNAS August 5, 2003 9440 -9445
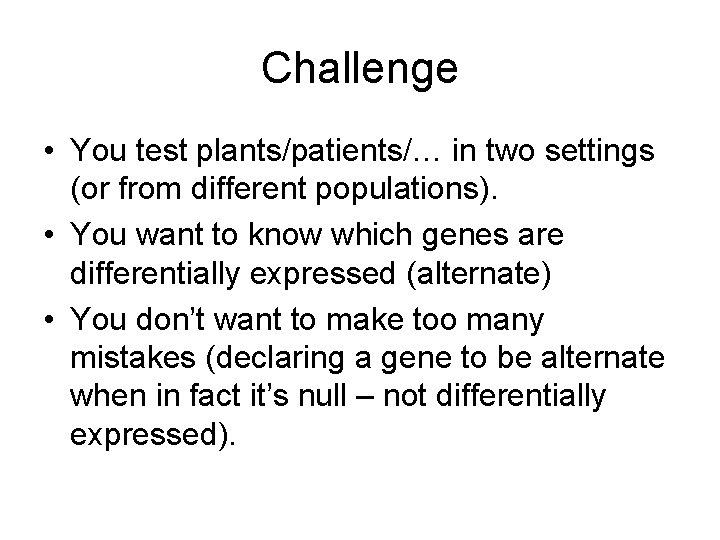
Challenge • You test plants/patients/… in two settings (or from different populations). • You want to know which genes are differentially expressed (alternate) • You don’t want to make too many mistakes (declaring a gene to be alternate when in fact it’s null – not differentially expressed).
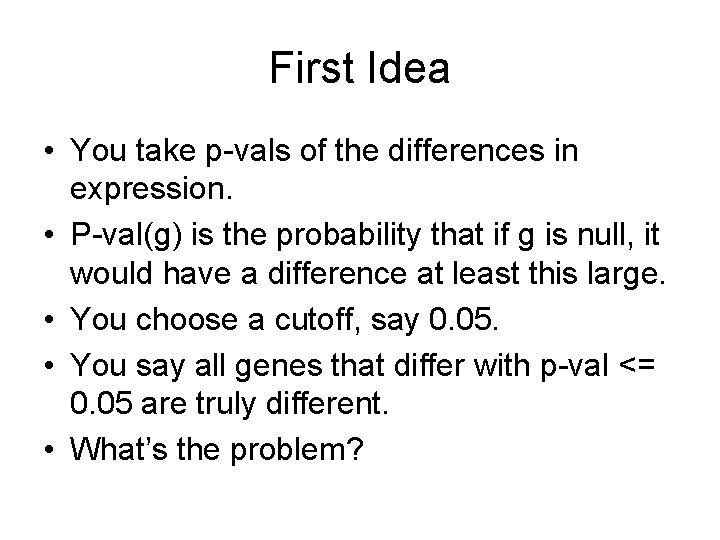
First Idea • You take p-vals of the differences in expression. • P-val(g) is the probability that if g is null, it would have a difference at least this large. • You choose a cutoff, say 0. 05. • You say all genes that differ with p-val <= 0. 05 are truly different. • What’s the problem?
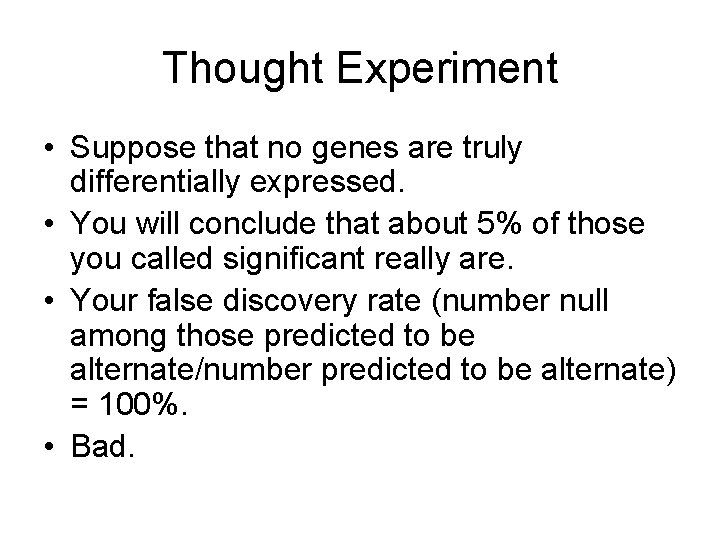
Thought Experiment • Suppose that no genes are truly differentially expressed. • You will conclude that about 5% of those you called significant really are. • Your false discovery rate (number null among those predicted to be alternate/number predicted to be alternate) = 100%. • Bad.
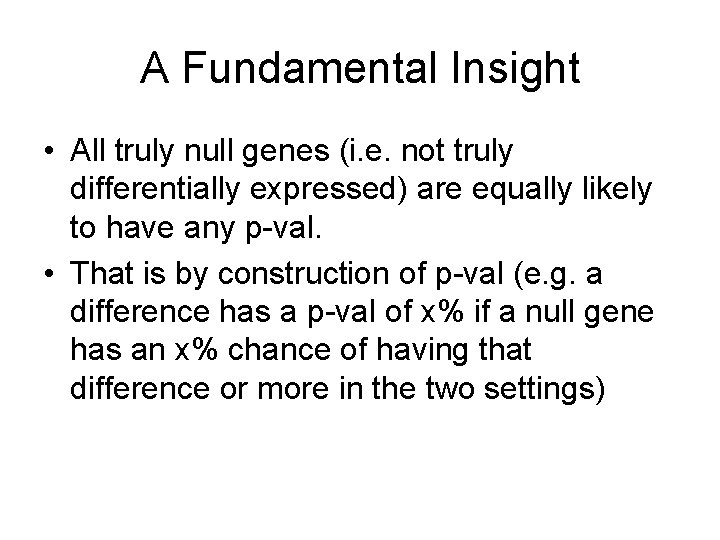
A Fundamental Insight • All truly null genes (i. e. not truly differentially expressed) are equally likely to have any p-val. • That is by construction of p-val (e. g. a difference has a p-val of x% if a null gene has an x% chance of having that difference or more in the two settings)
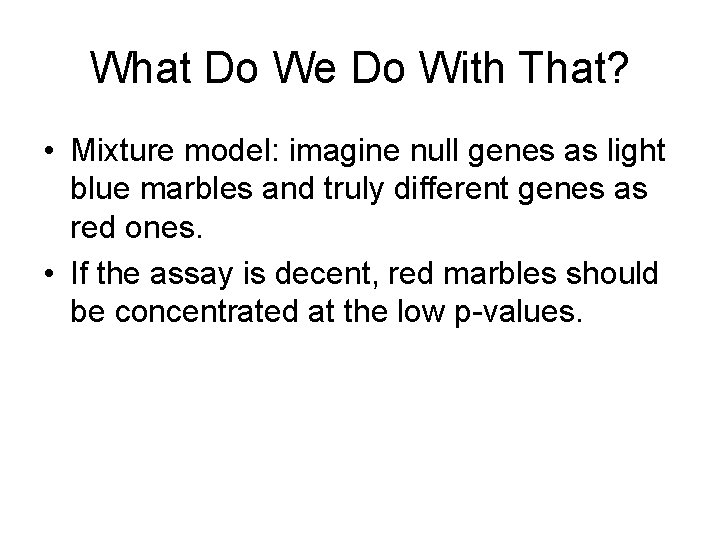
What Do We Do With That? • Mixture model: imagine null genes as light blue marbles and truly different genes as red ones. • If the assay is decent, red marbles should be concentrated at the low p-values.
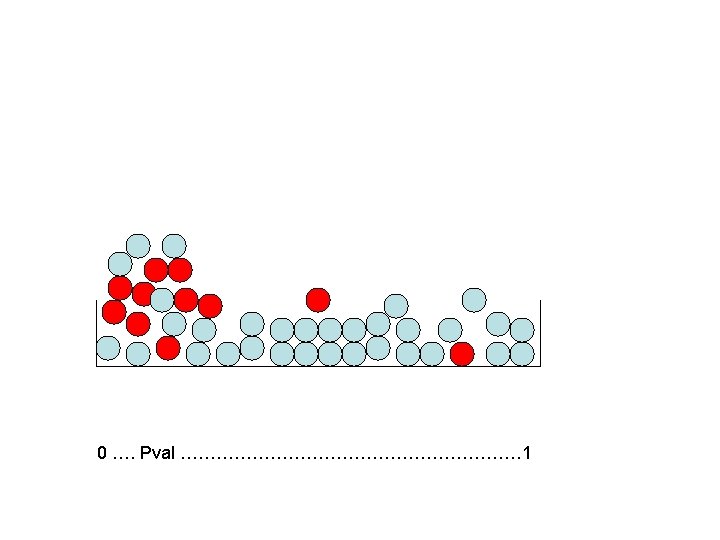
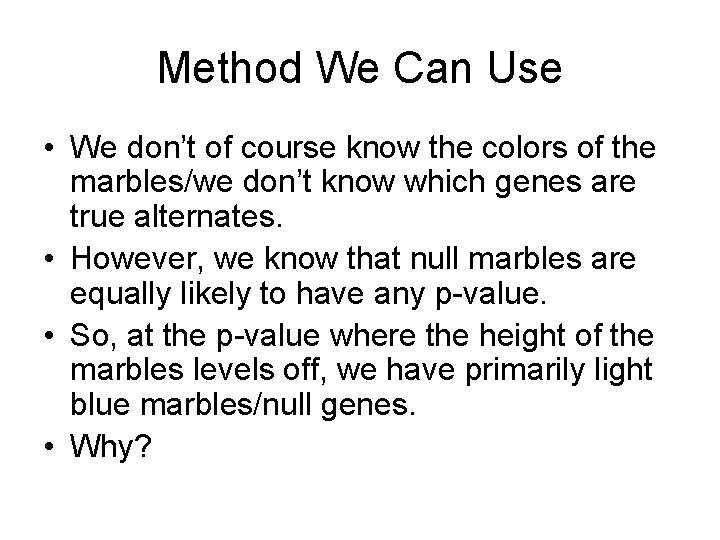
Method We Can Use • We don’t of course know the colors of the marbles/we don’t know which genes are true alternates. • However, we know that null marbles are equally likely to have any p-value. • So, at the p-value where the height of the marbles levels off, we have primarily light blue marbles/null genes. • Why?
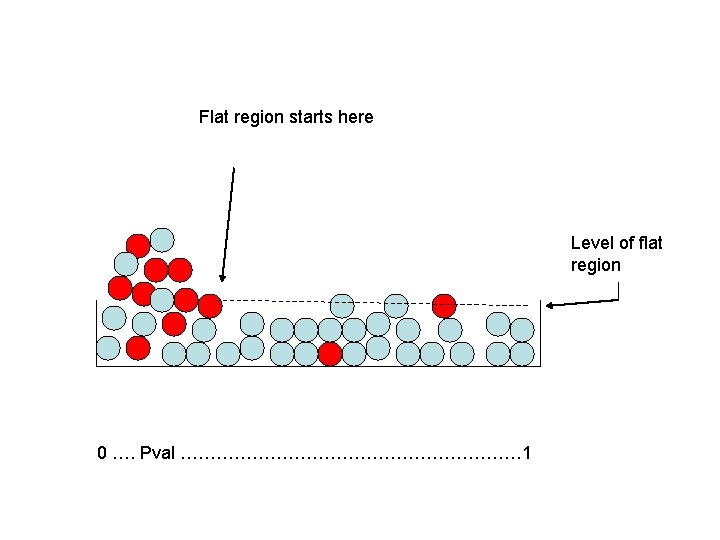
Flat region starts here Level of flat region 0 …. Pval ………………………… 1
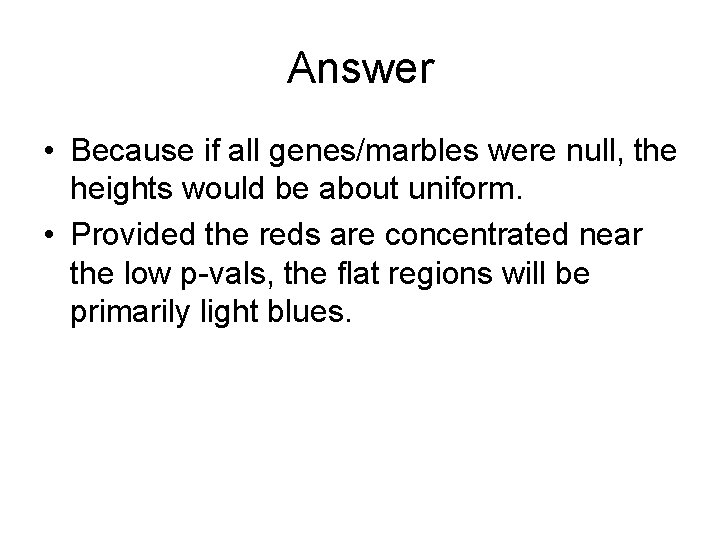
Answer • Because if all genes/marbles were null, the heights would be about uniform. • Provided the reds are concentrated near the low p-vals, the flat regions will be primarily light blues.
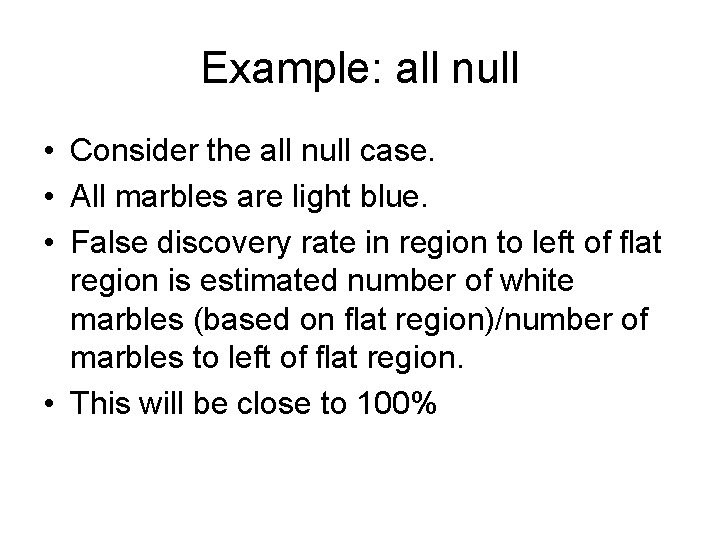
Example: all null • Consider the all null case. • All marbles are light blue. • False discovery rate in region to left of flat region is estimated number of white marbles (based on flat region)/number of marbles to left of flat region. • This will be close to 100%
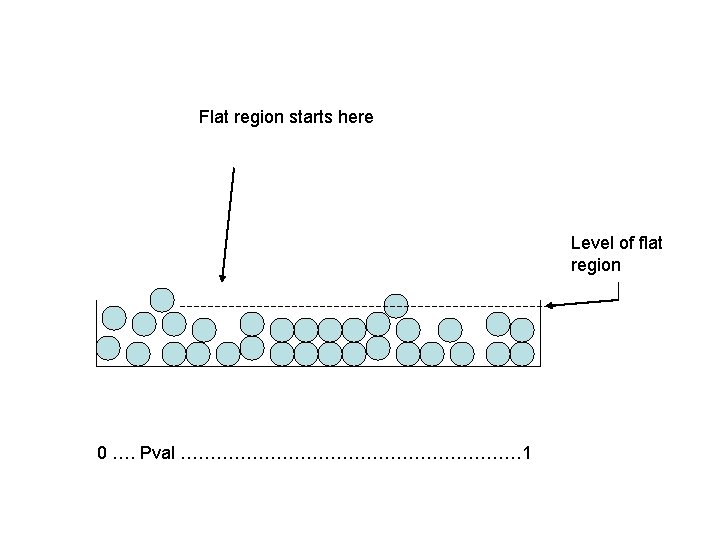
Flat region starts here Level of flat region 0 …. Pval ………………………… 1
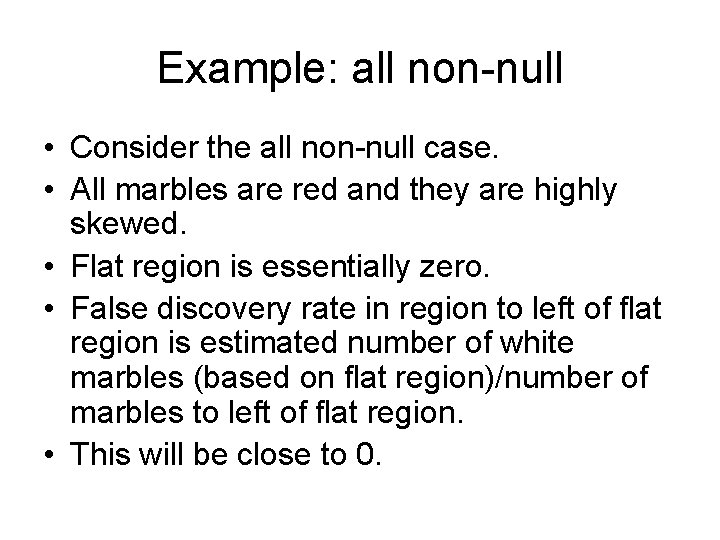
Example: all non-null • Consider the all non-null case. • All marbles are red and they are highly skewed. • Flat region is essentially zero. • False discovery rate in region to left of flat region is estimated number of white marbles (based on flat region)/number of marbles to left of flat region. • This will be close to 0.
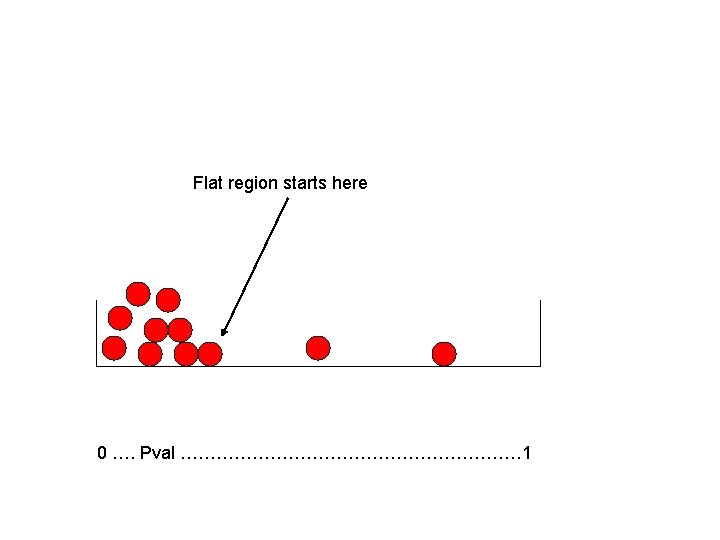
Flat region starts here 0 …. Pval ………………………… 1
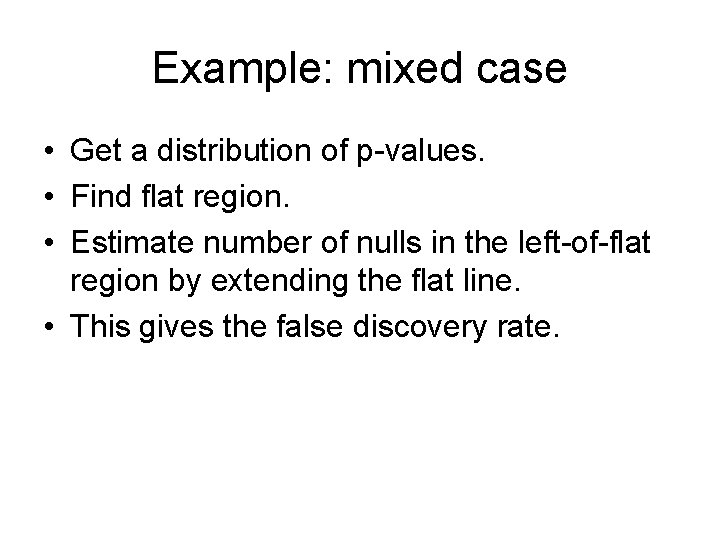
Example: mixed case • Get a distribution of p-values. • Find flat region. • Estimate number of nulls in the left-of-flat region by extending the flat line. • This gives the false discovery rate.
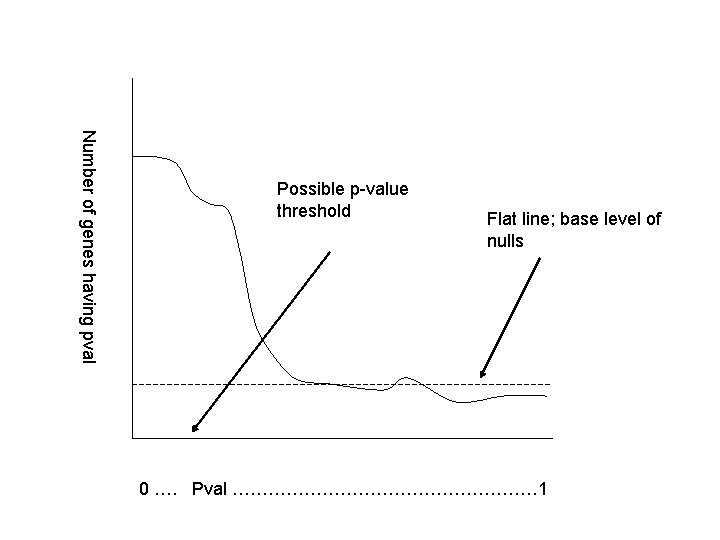
Number of genes having pval Possible p-value threshold Flat line; base level of nulls 0 …. Pval ……………………… 1
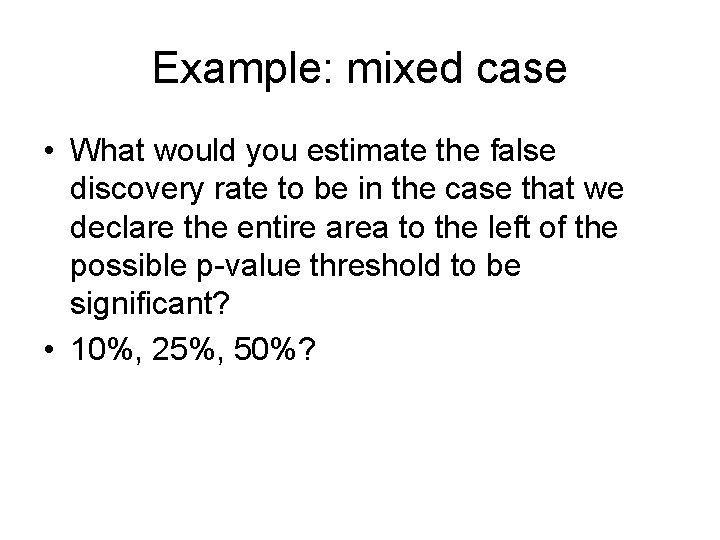
Example: mixed case • What would you estimate the false discovery rate to be in the case that we declare the entire area to the left of the possible p-value threshold to be significant? • 10%, 25%, 50%?
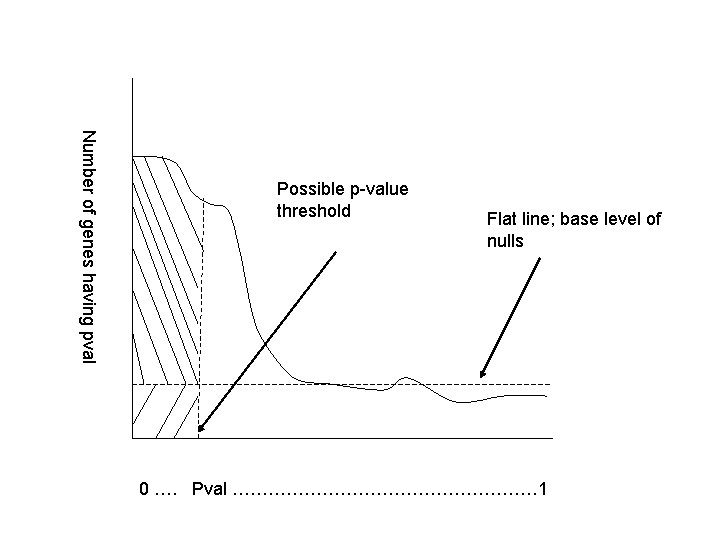
Number of genes having pval Possible p-value threshold Flat line; base level of nulls 0 …. Pval ……………………… 1
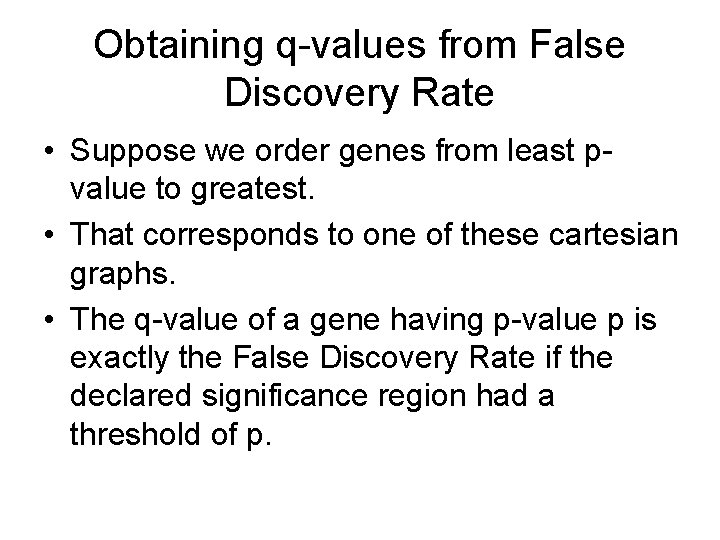
Obtaining q-values from False Discovery Rate • Suppose we order genes from least pvalue to greatest. • That corresponds to one of these cartesian graphs. • The q-value of a gene having p-value p is exactly the False Discovery Rate if the declared significance region had a threshold of p.
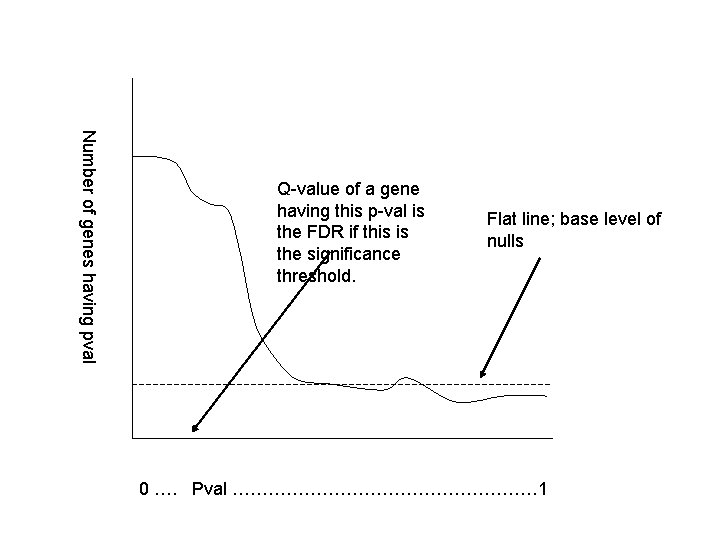
Number of genes having pval Q-value of a gene having this p-val is the FDR if this is the significance threshold. Flat line; base level of nulls 0 …. Pval ……………………… 1
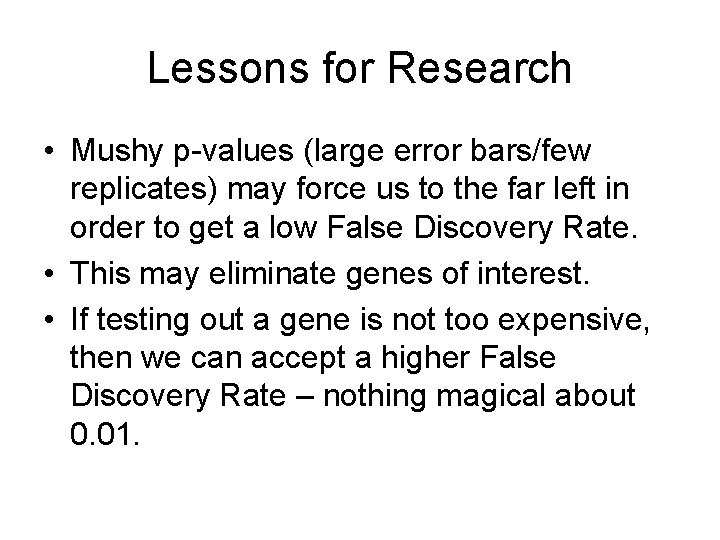
Lessons for Research • Mushy p-values (large error bars/few replicates) may force us to the far left in order to get a low False Discovery Rate. • This may eliminate genes of interest. • If testing out a gene is not too expensive, then we can accept a higher False Discovery Rate – nothing magical about 0. 01.
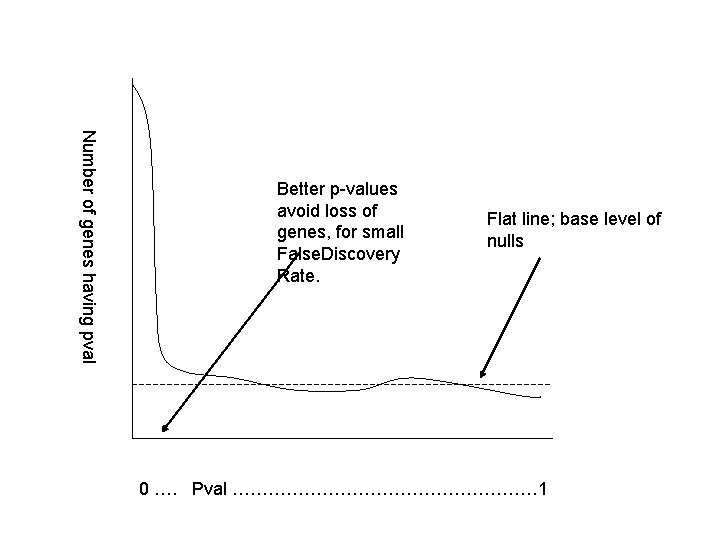
Number of genes having pval Better p-values avoid loss of genes, for small False. Discovery Rate. Flat line; base level of nulls 0 …. Pval ……………………… 1
Is a ratio a rate
Equivalent ratios definition
Ratios rates and unit rates
Ratios rates and unit rates
Slidetodoc.com
Rates of reaction quiz
False discovery rate calculator
Deductive vs inductive
Every quiz has been easy. therefore the test will be easy
Deductive reasoning
Amer rasheed
Portunus simulation
Rhonda scharf
Coagulation pathway made easy
Tasc distinguished achievement
Nepotism laws in texas
Evangelism made easy
Balancing chemical equations made easy
Significant figures made easy
Sales force mobility
Prius effect leed
Stainless steel crown charting
Essay made easy