Occlusion Aware 3 D Human Pose Estimation Cheng
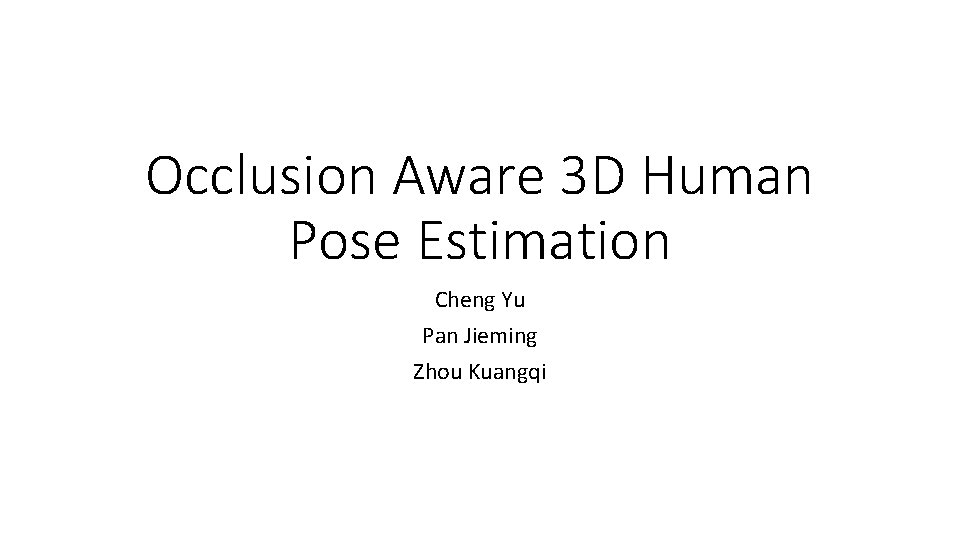
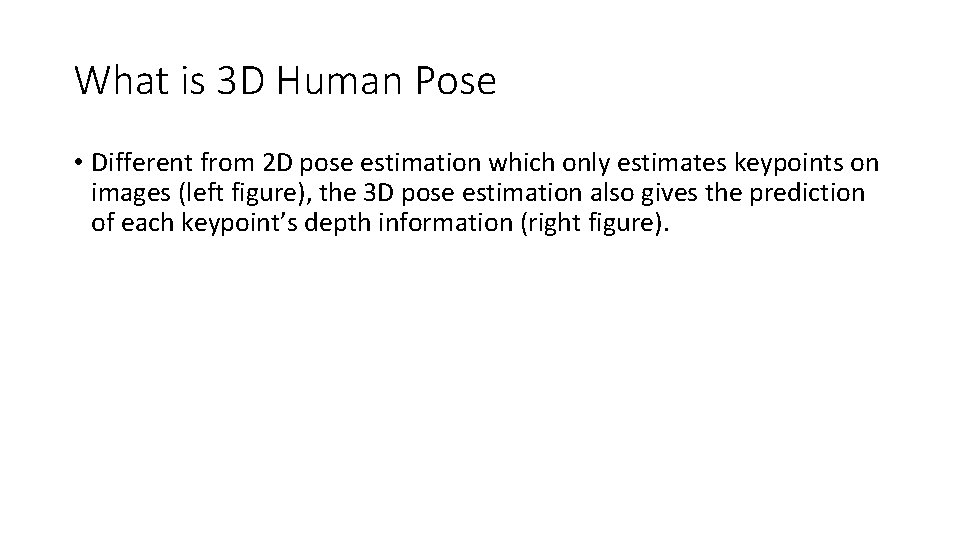
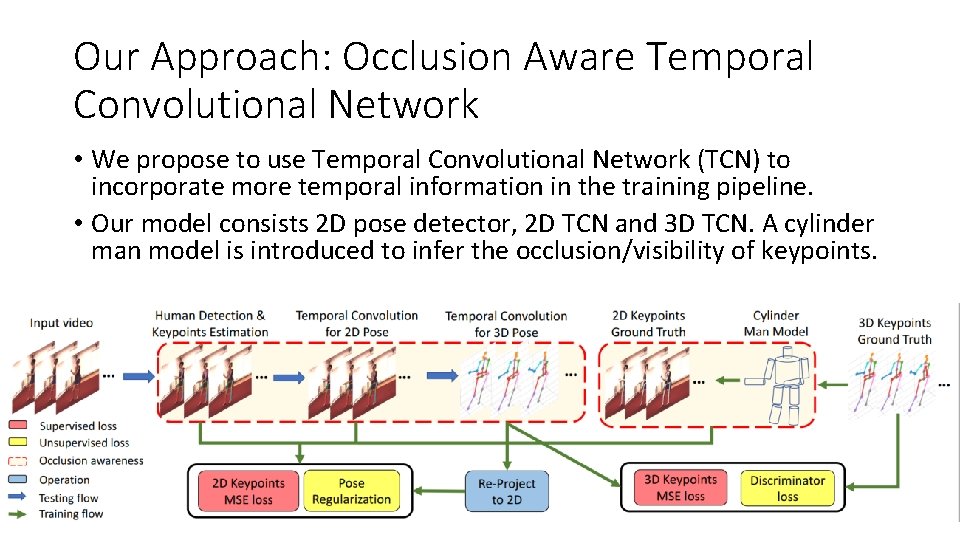
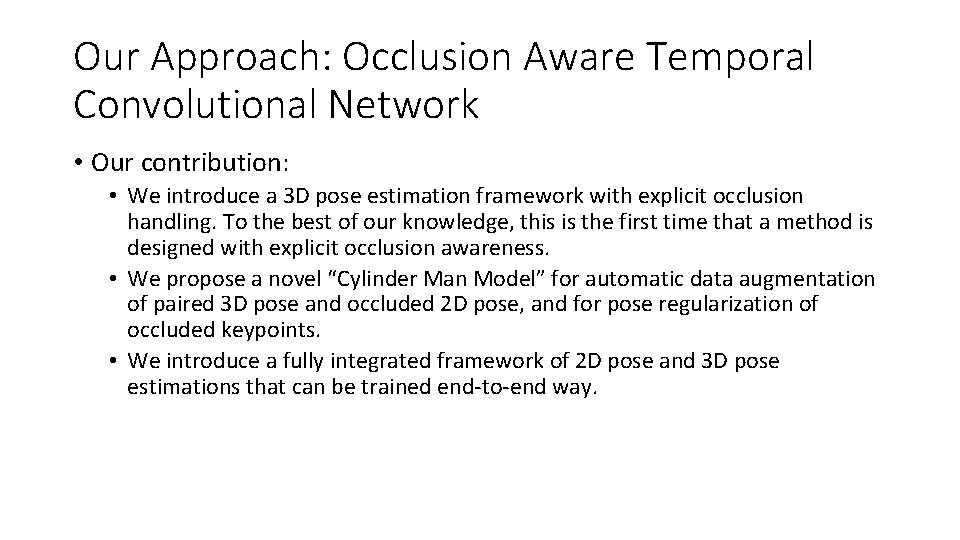
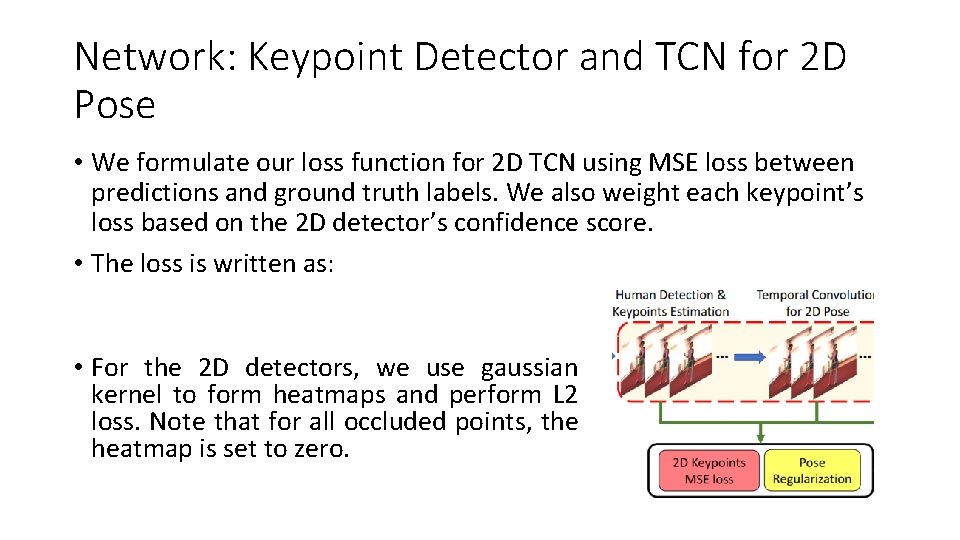
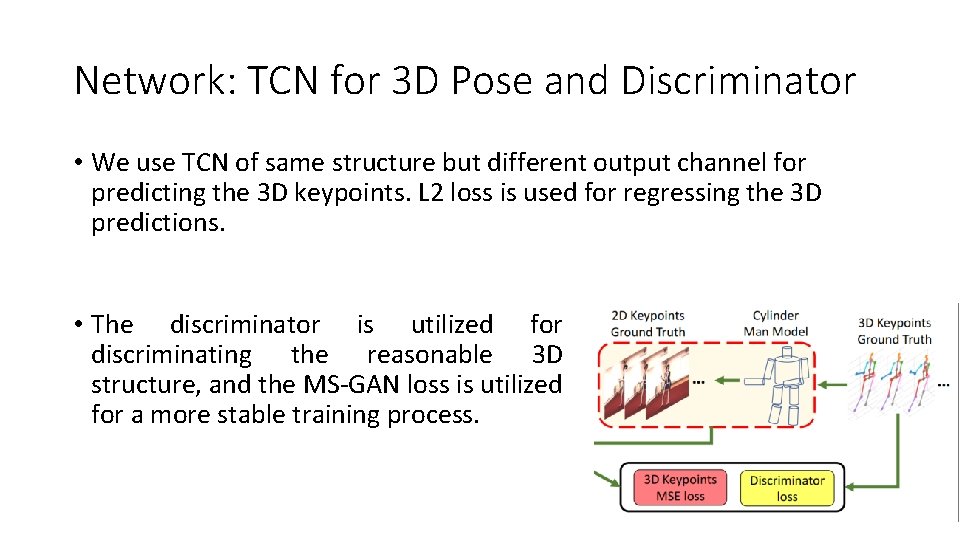
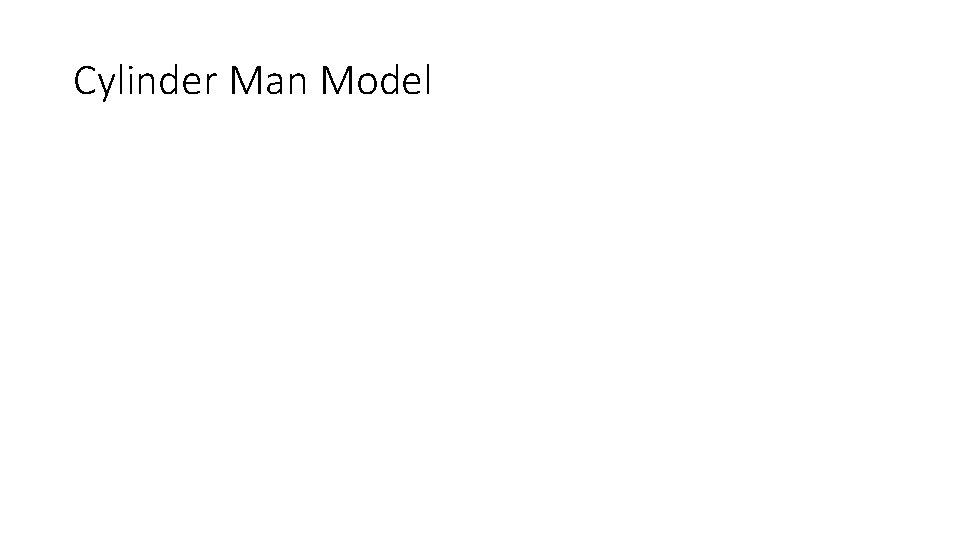
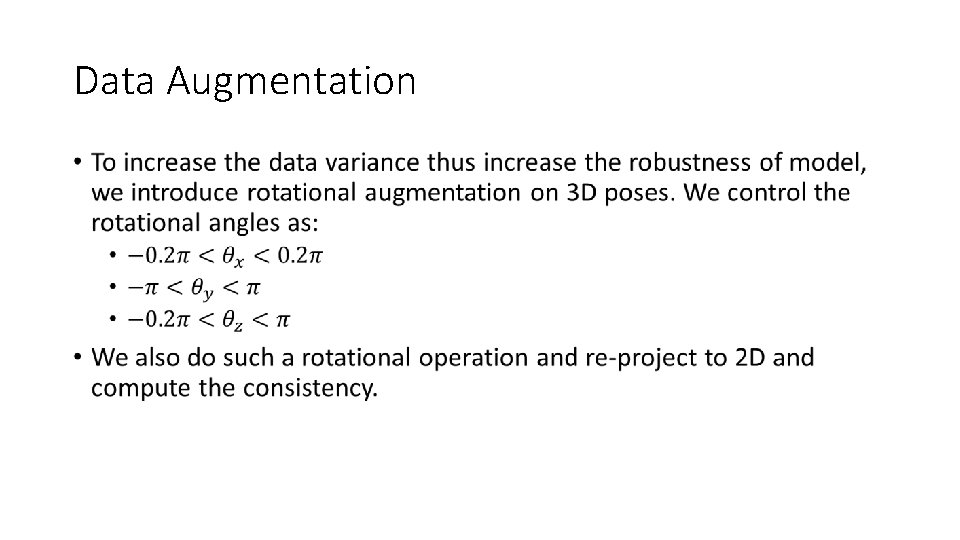
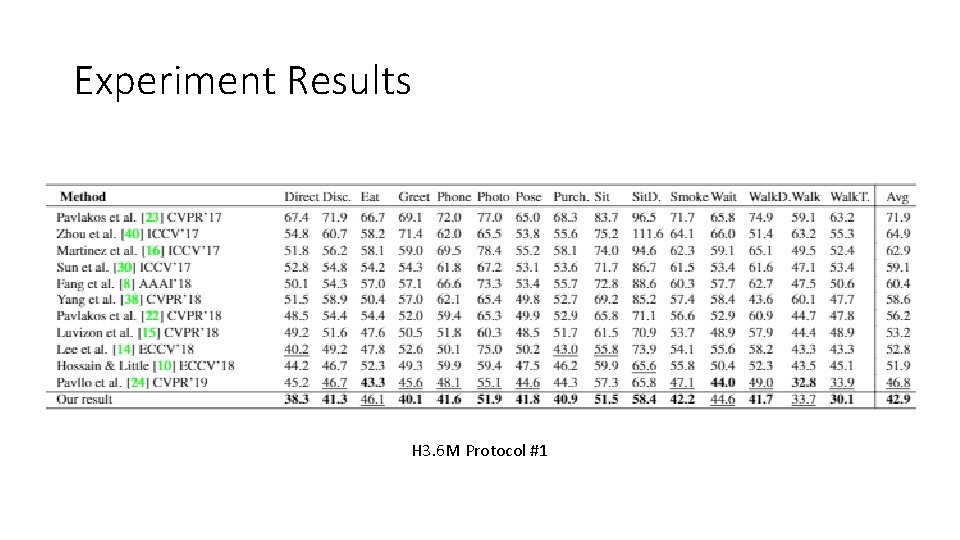
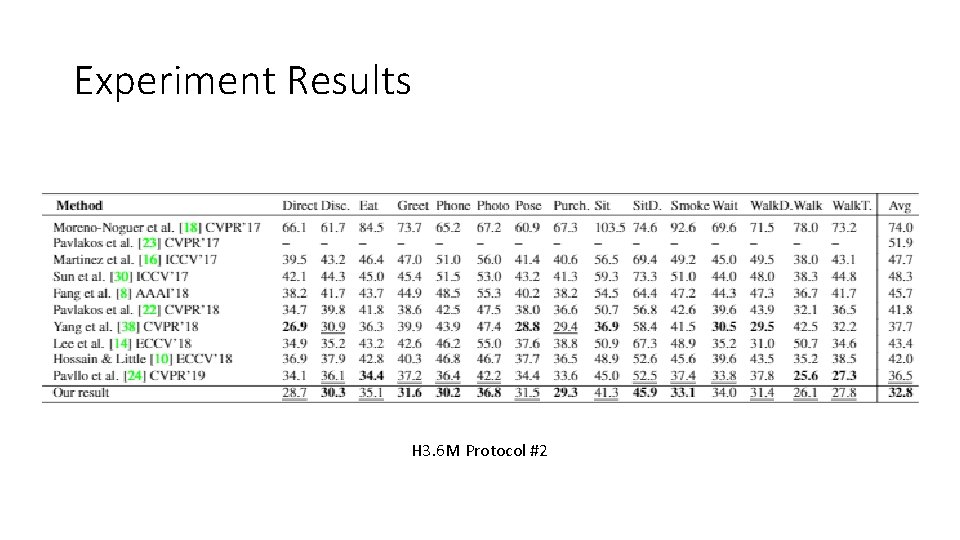
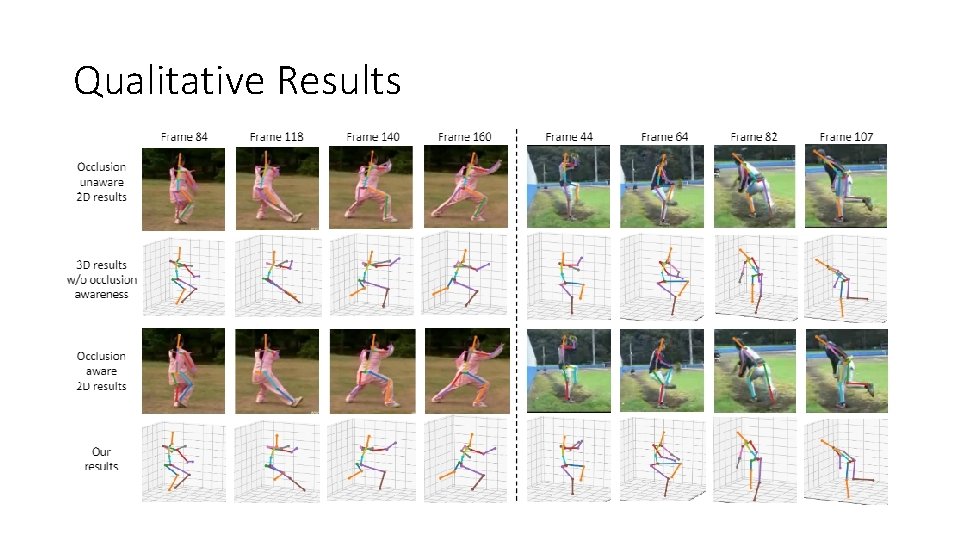
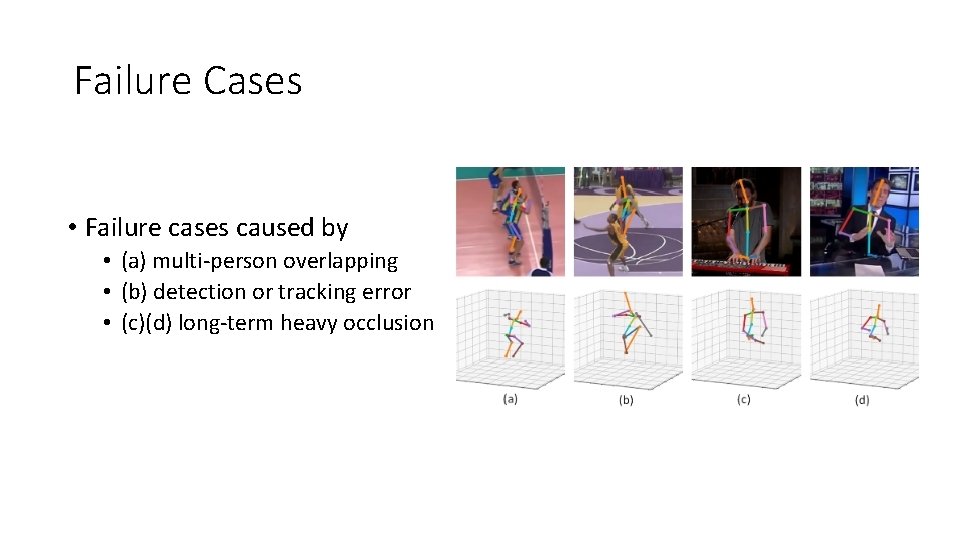
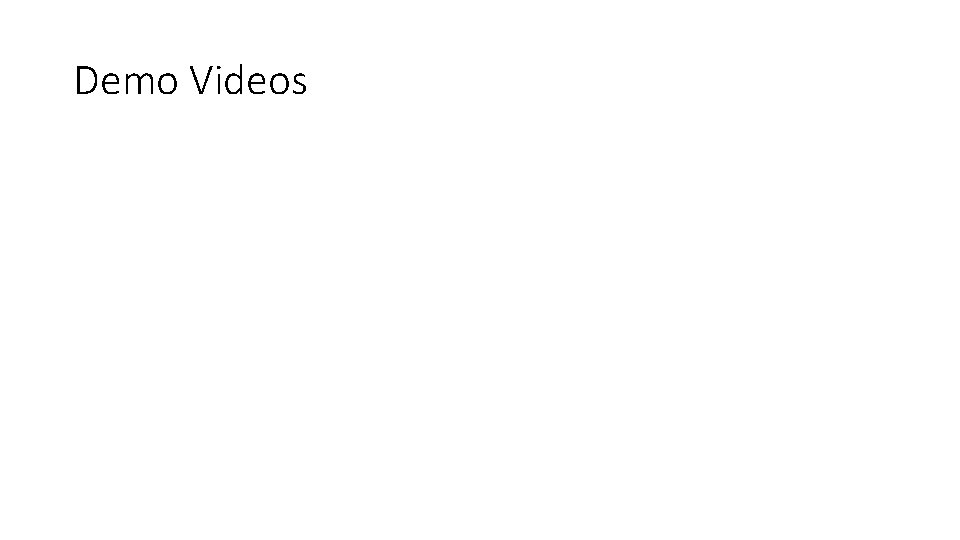
- Slides: 13
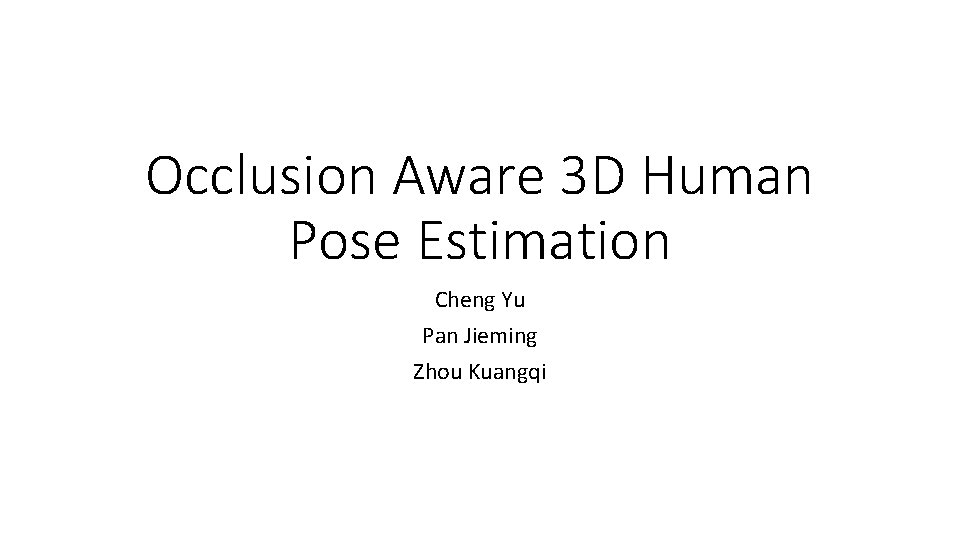
Occlusion Aware 3 D Human Pose Estimation Cheng Yu Pan Jieming Zhou Kuangqi
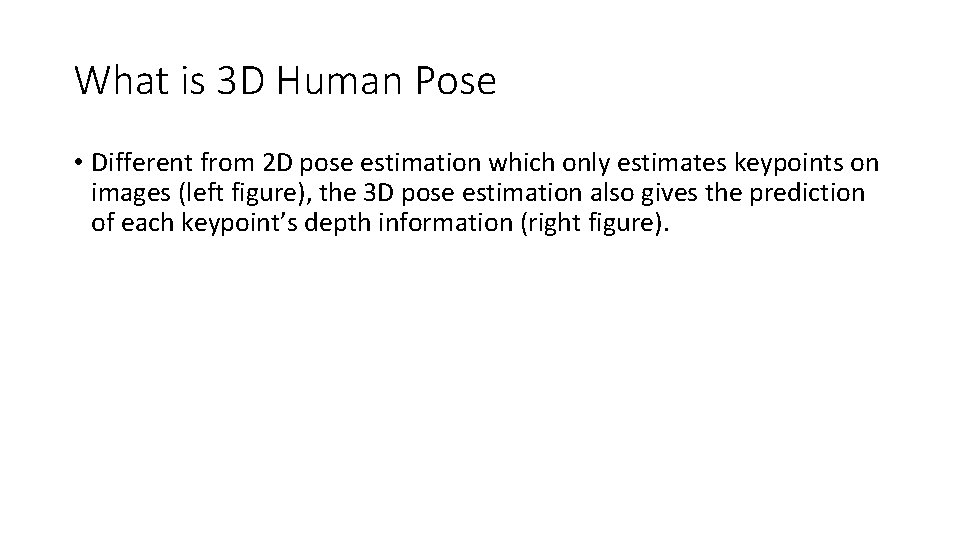
What is 3 D Human Pose • Different from 2 D pose estimation which only estimates keypoints on images (left figure), the 3 D pose estimation also gives the prediction of each keypoint’s depth information (right figure).
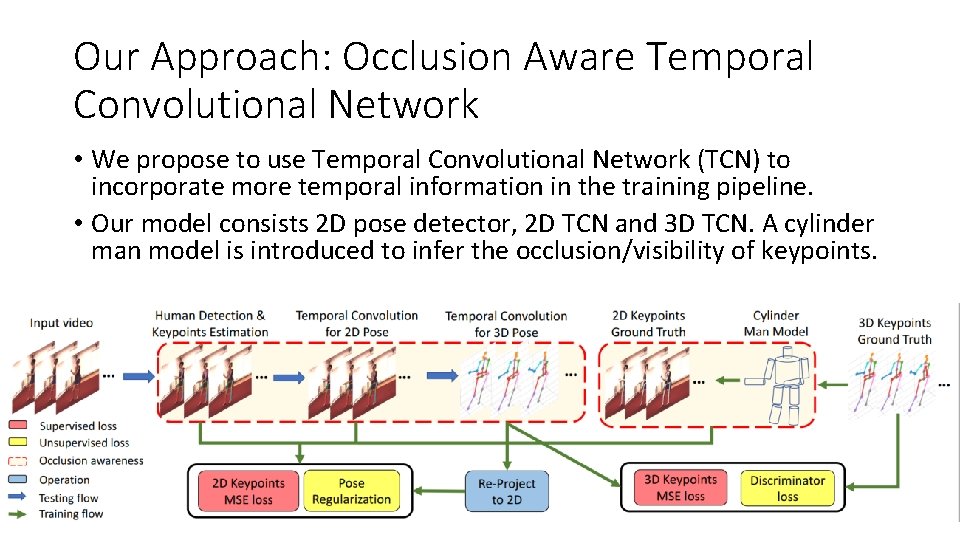
Our Approach: Occlusion Aware Temporal Convolutional Network • We propose to use Temporal Convolutional Network (TCN) to incorporate more temporal information in the training pipeline. • Our model consists 2 D pose detector, 2 D TCN and 3 D TCN. A cylinder man model is introduced to infer the occlusion/visibility of keypoints.
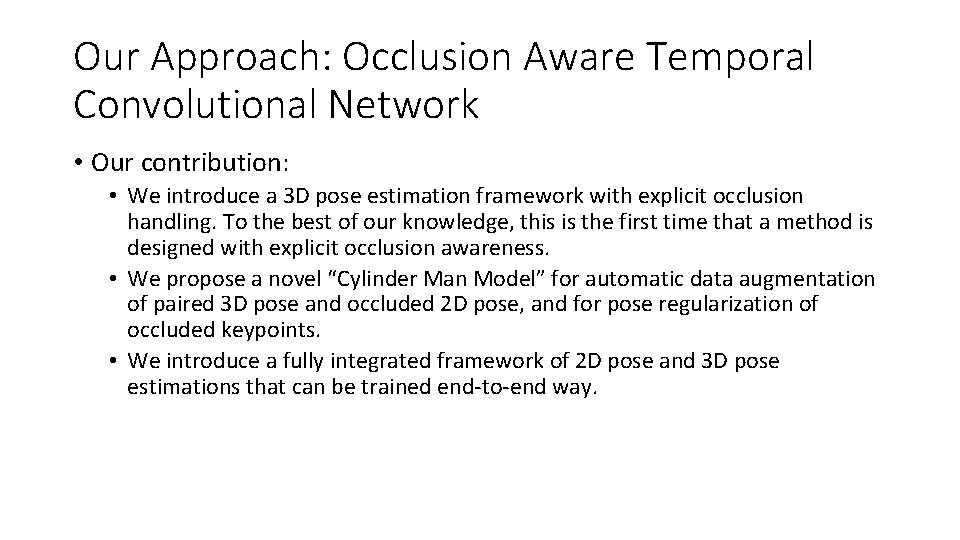
Our Approach: Occlusion Aware Temporal Convolutional Network • Our contribution: • We introduce a 3 D pose estimation framework with explicit occlusion handling. To the best of our knowledge, this is the first time that a method is designed with explicit occlusion awareness. • We propose a novel “Cylinder Man Model” for automatic data augmentation of paired 3 D pose and occluded 2 D pose, and for pose regularization of occluded keypoints. • We introduce a fully integrated framework of 2 D pose and 3 D pose estimations that can be trained end-to-end way.
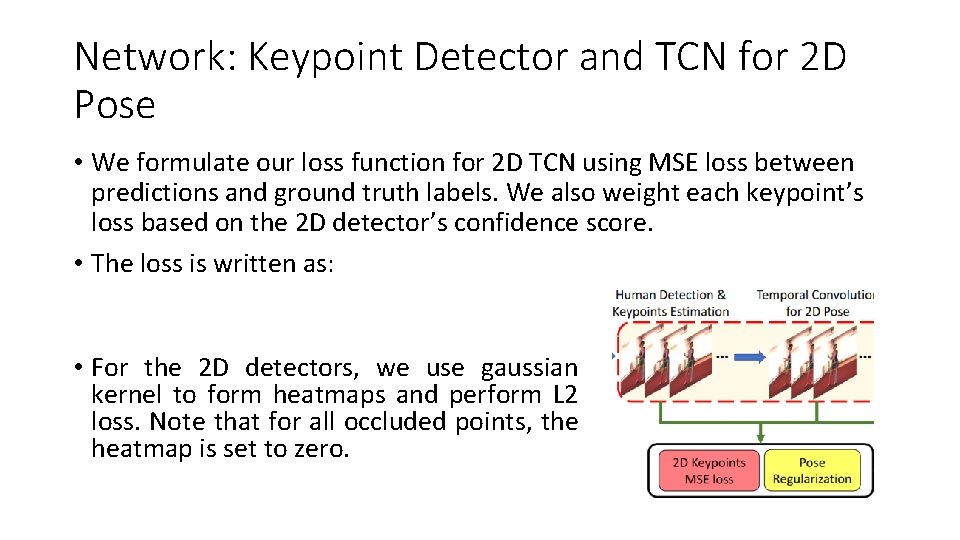
Network: Keypoint Detector and TCN for 2 D Pose • We formulate our loss function for 2 D TCN using MSE loss between predictions and ground truth labels. We also weight each keypoint’s loss based on the 2 D detector’s confidence score. • The loss is written as: • For the 2 D detectors, we use gaussian kernel to form heatmaps and perform L 2 loss. Note that for all occluded points, the heatmap is set to zero.
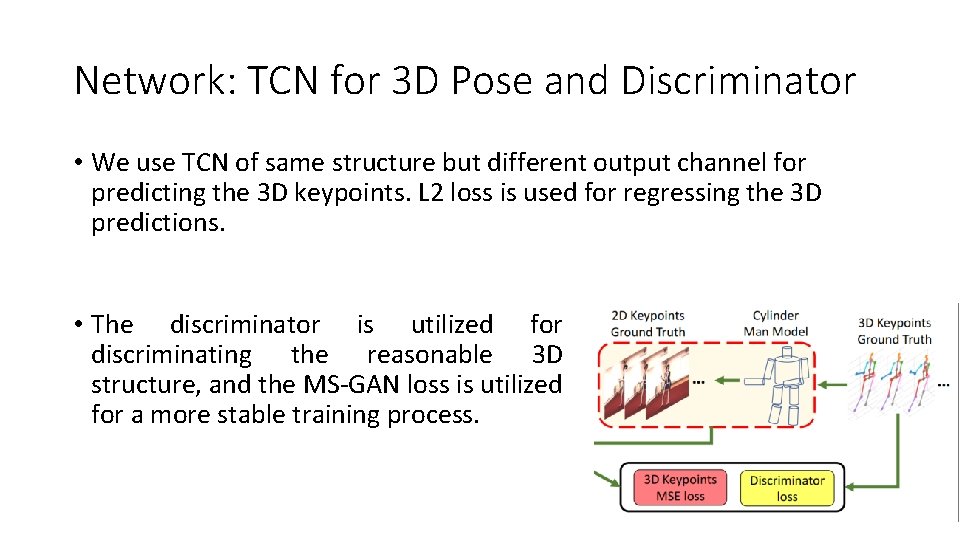
Network: TCN for 3 D Pose and Discriminator • We use TCN of same structure but different output channel for predicting the 3 D keypoints. L 2 loss is used for regressing the 3 D predictions. • The discriminator is utilized for discriminating the reasonable 3 D structure, and the MS-GAN loss is utilized for a more stable training process.
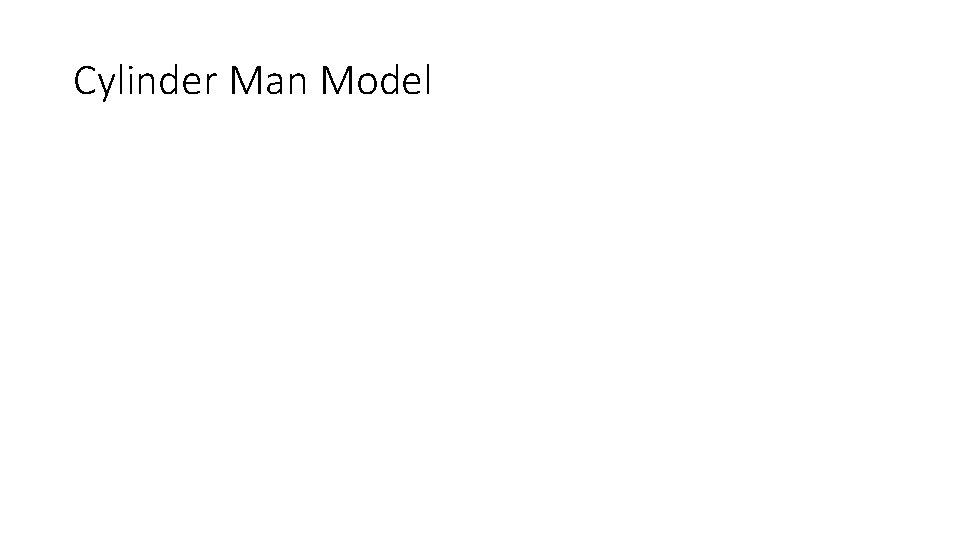
Cylinder Man Model
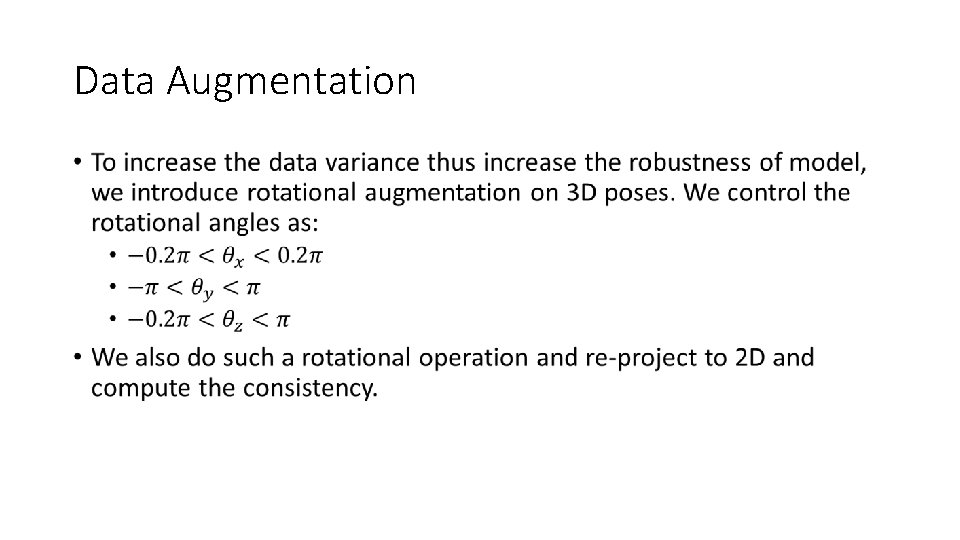
Data Augmentation •
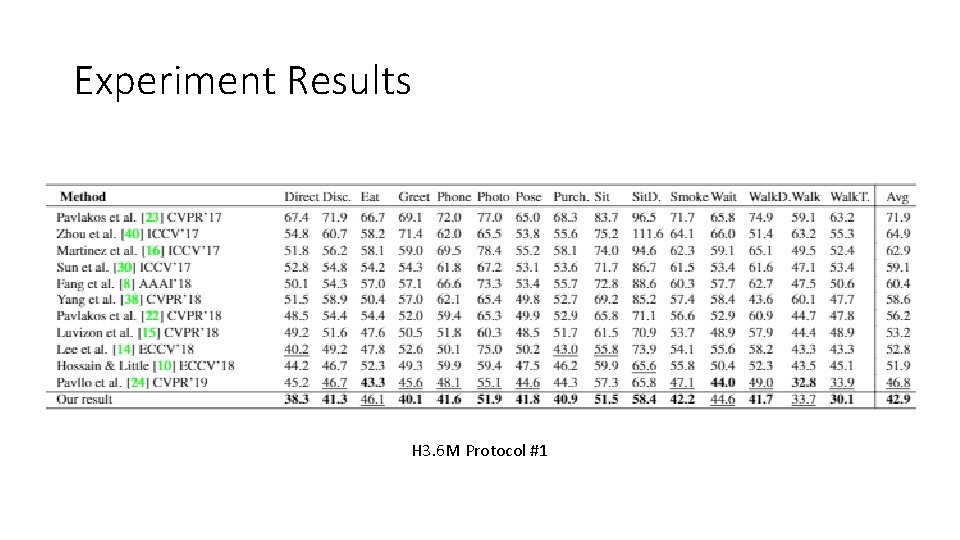
Experiment Results H 3. 6 M Protocol #1
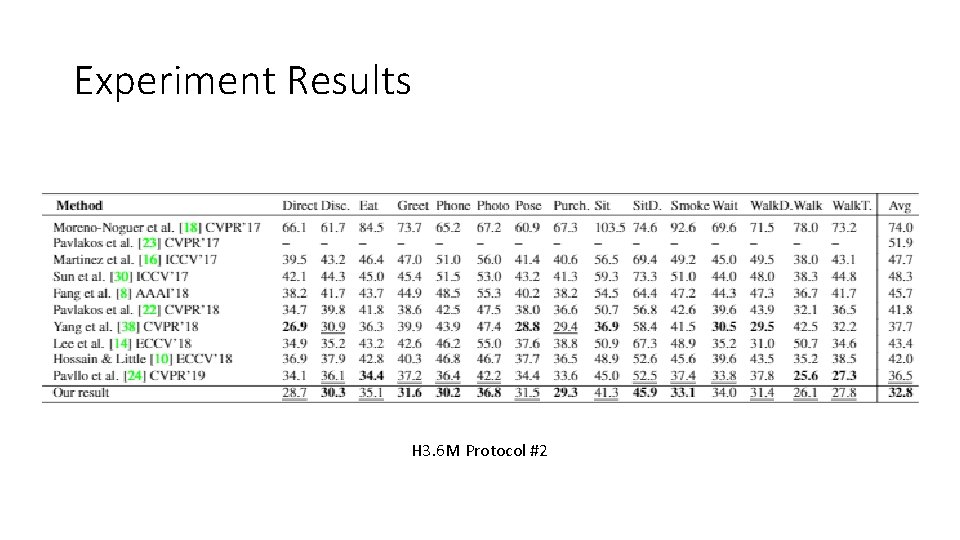
Experiment Results H 3. 6 M Protocol #2
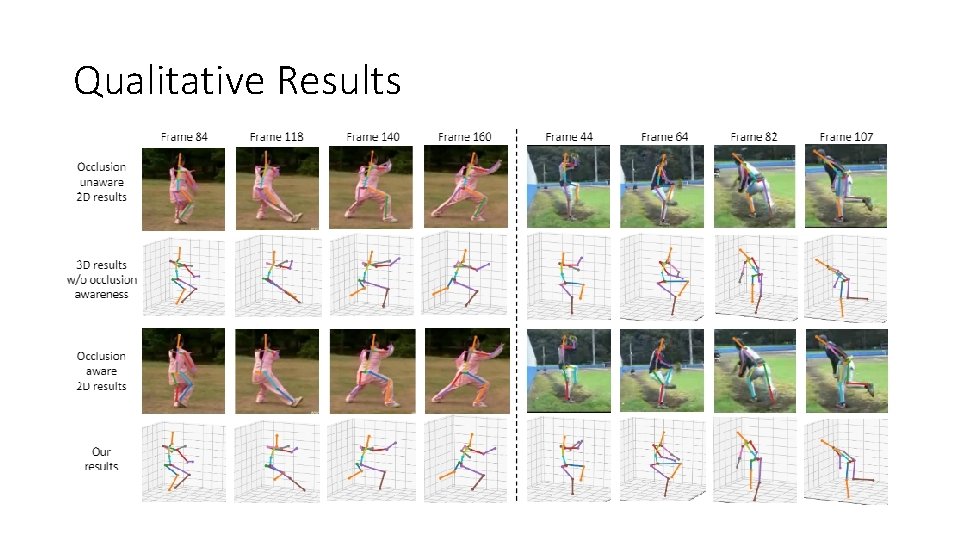
Qualitative Results
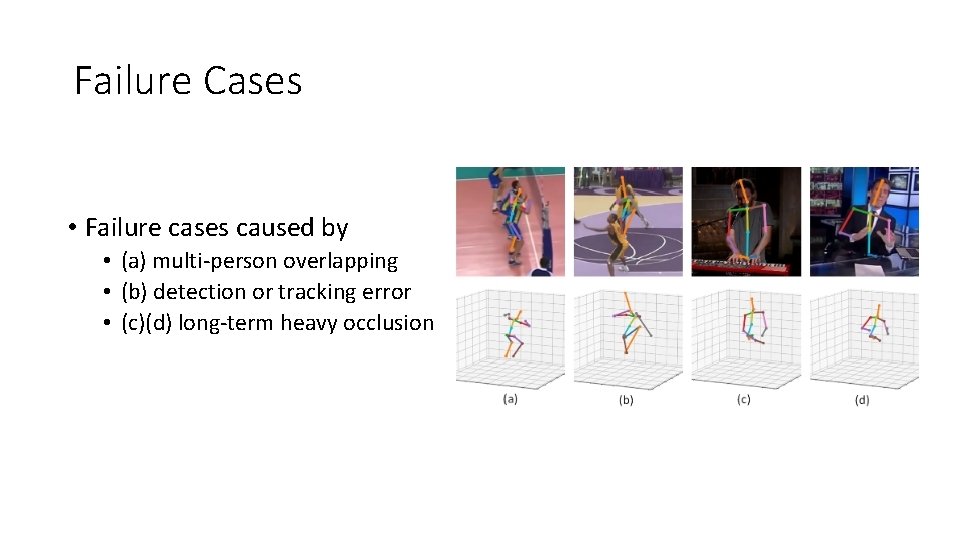
Failure Cases • Failure cases caused by • (a) multi-person overlapping • (b) detection or tracking error • (c)(d) long-term heavy occlusion
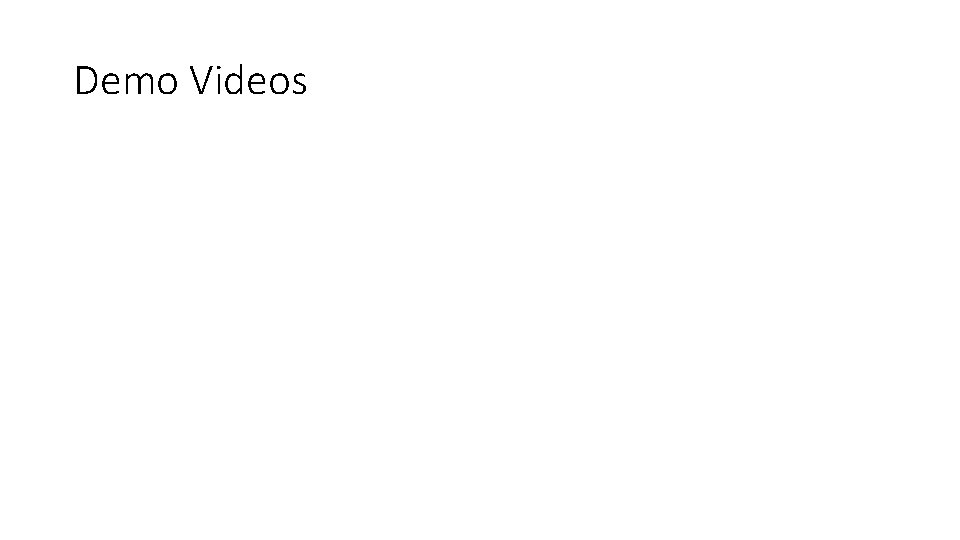
Demo Videos