Multiple Treatment Comparisons and Network Metaanalyses SYRIUS II
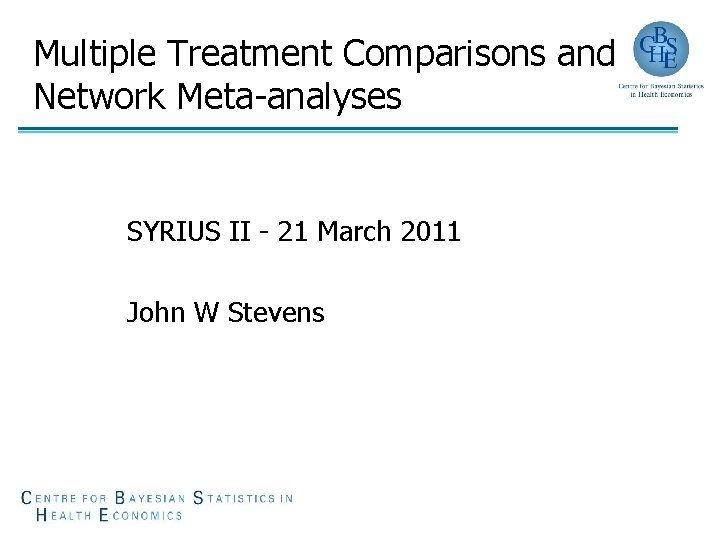
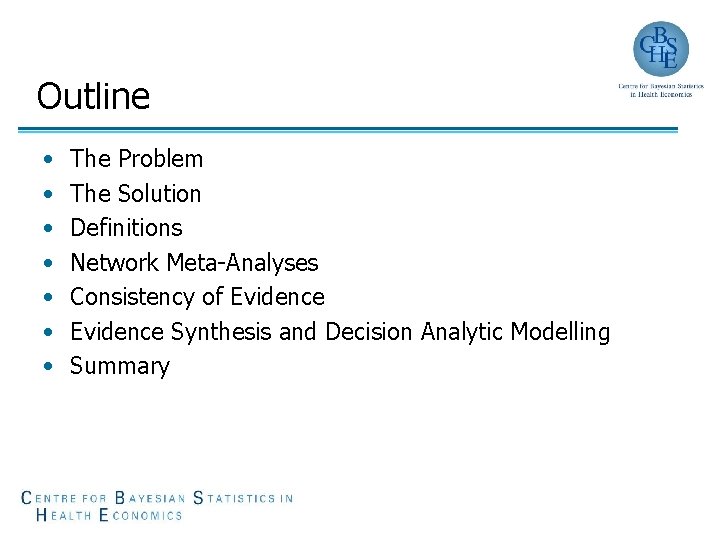
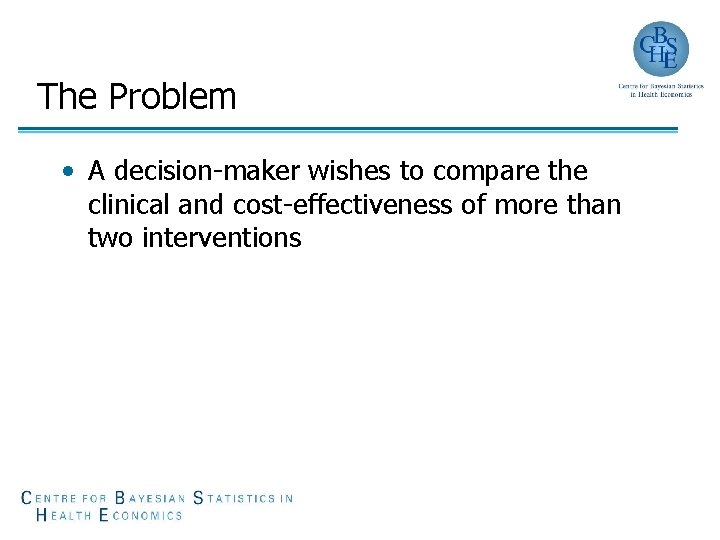
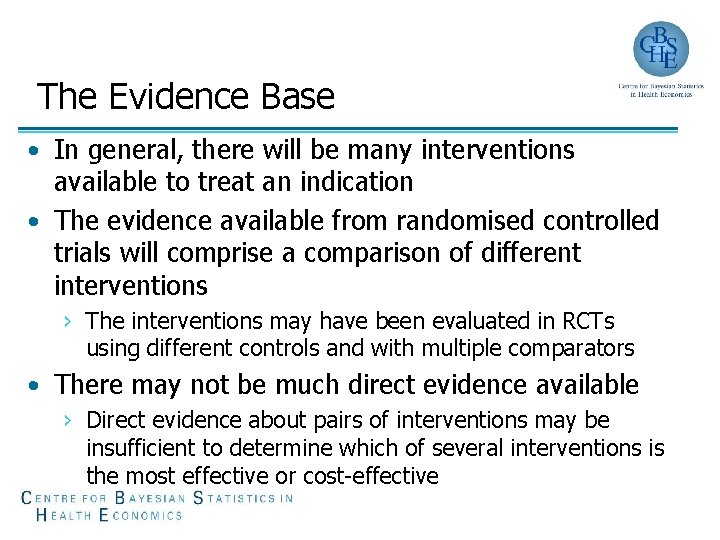
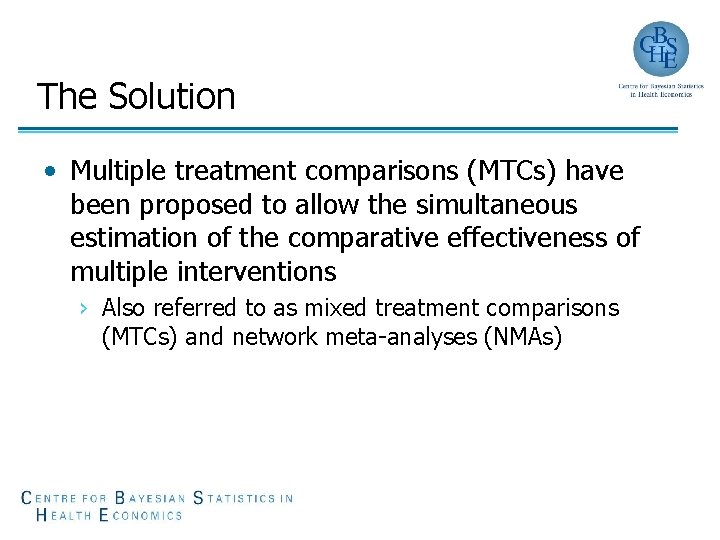
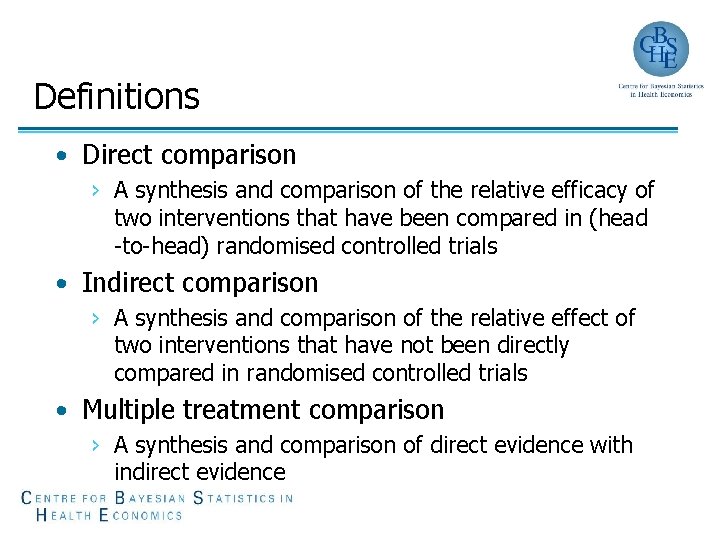
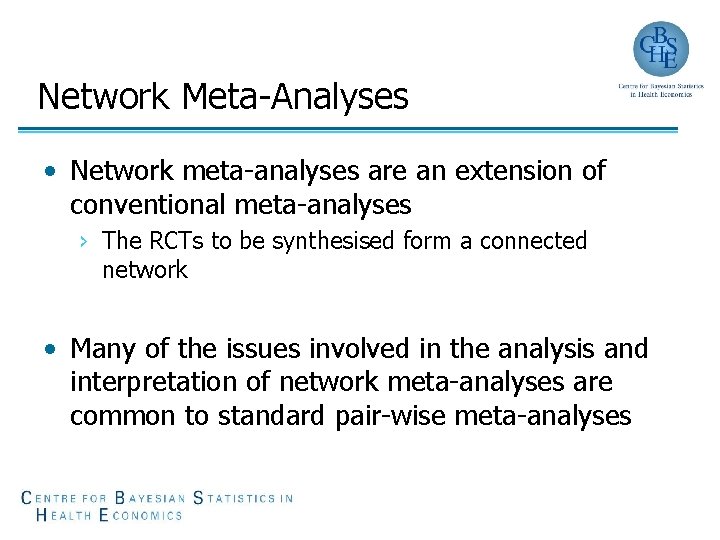
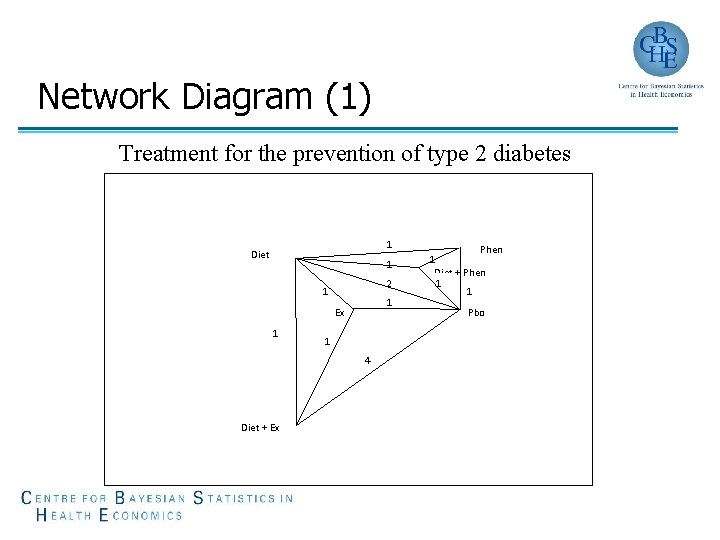
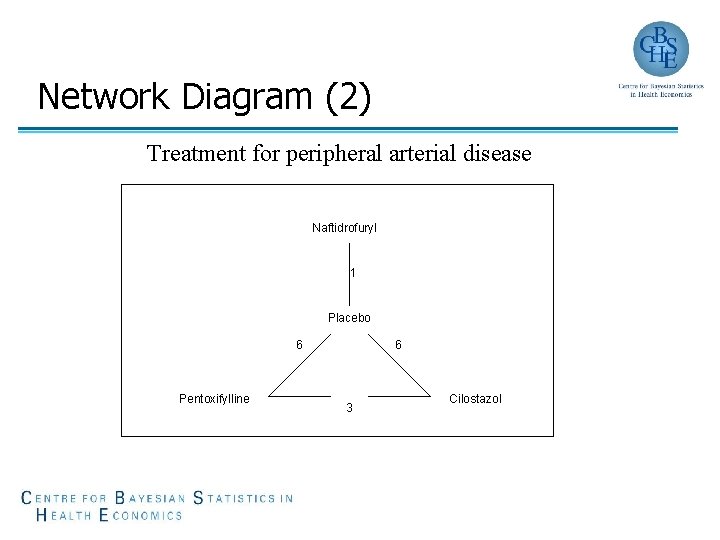
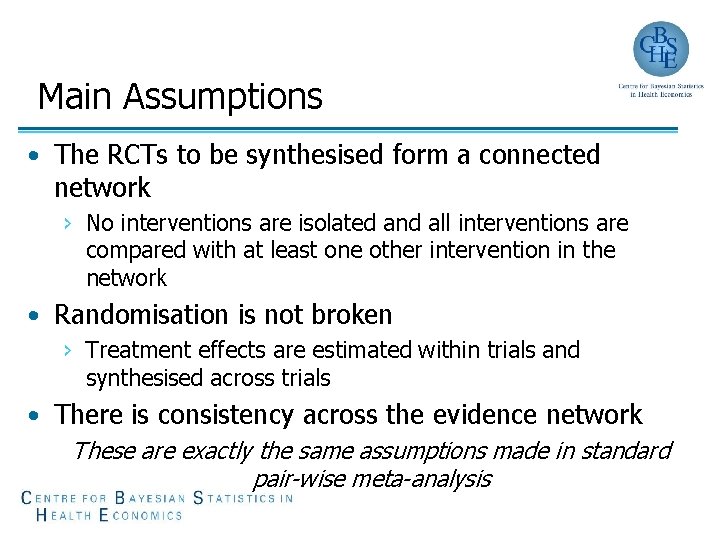
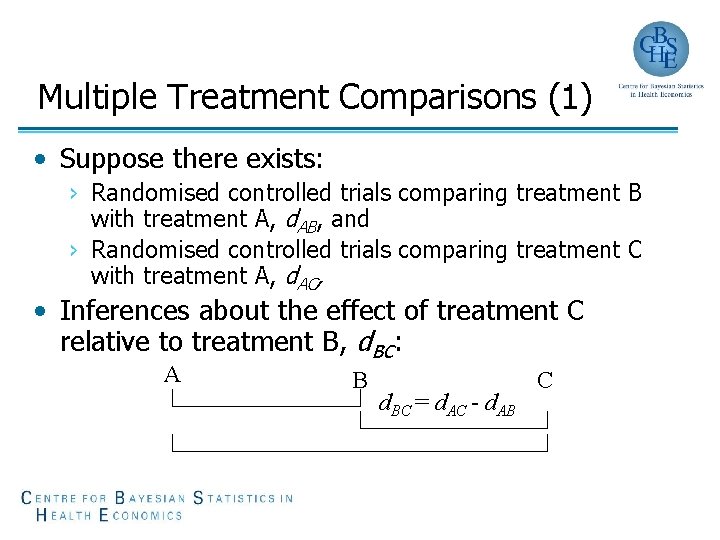
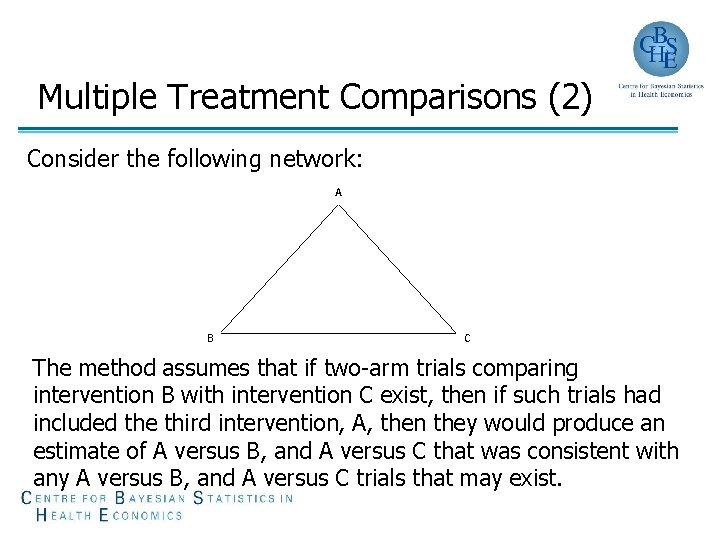
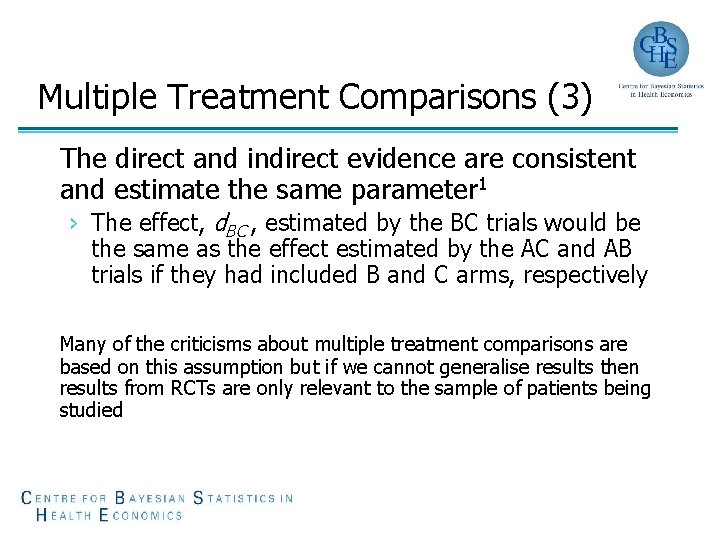
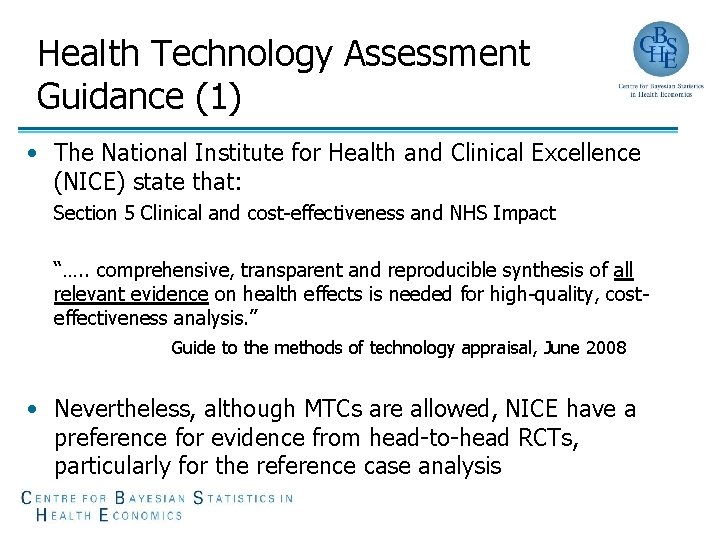
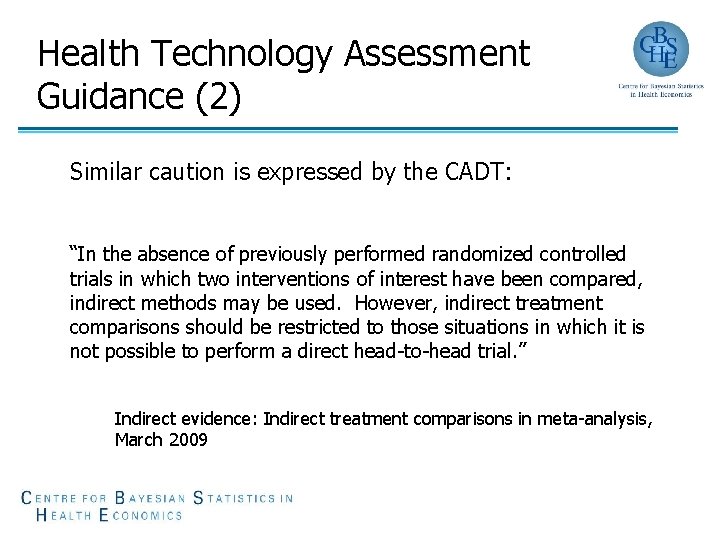
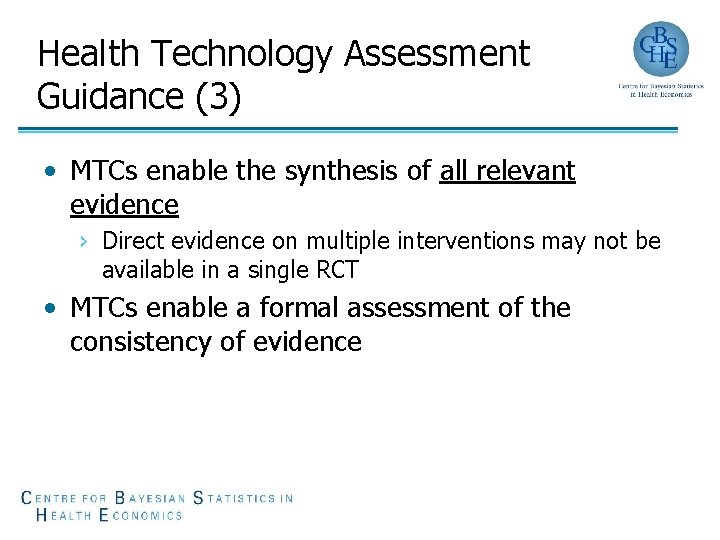
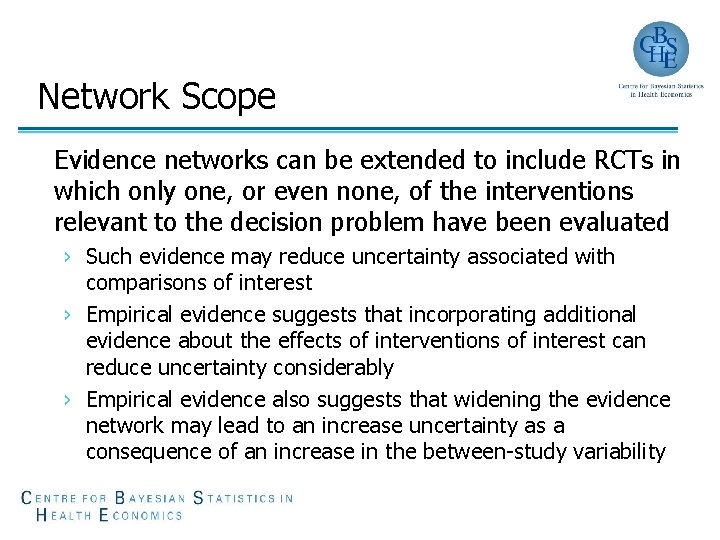
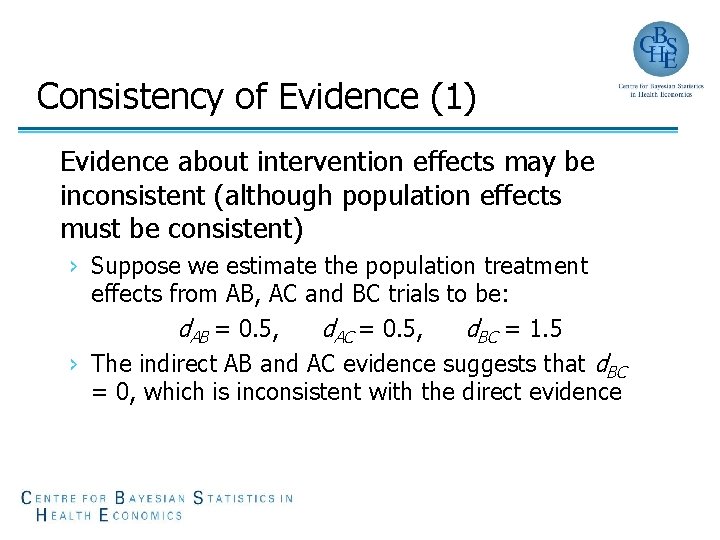
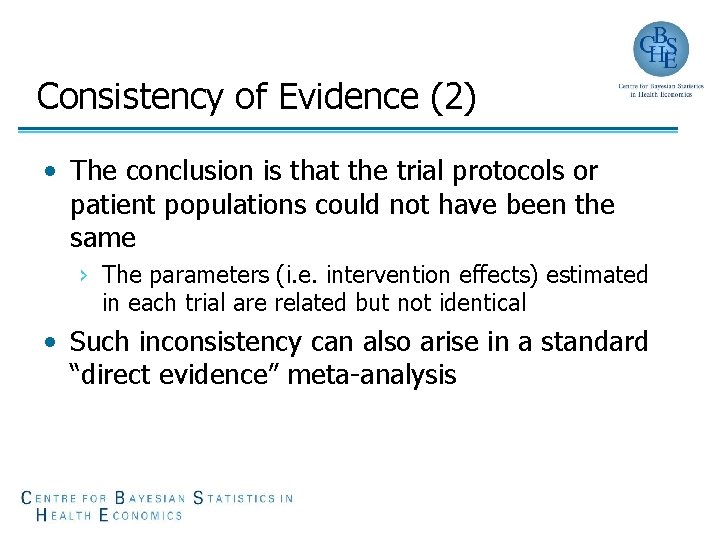
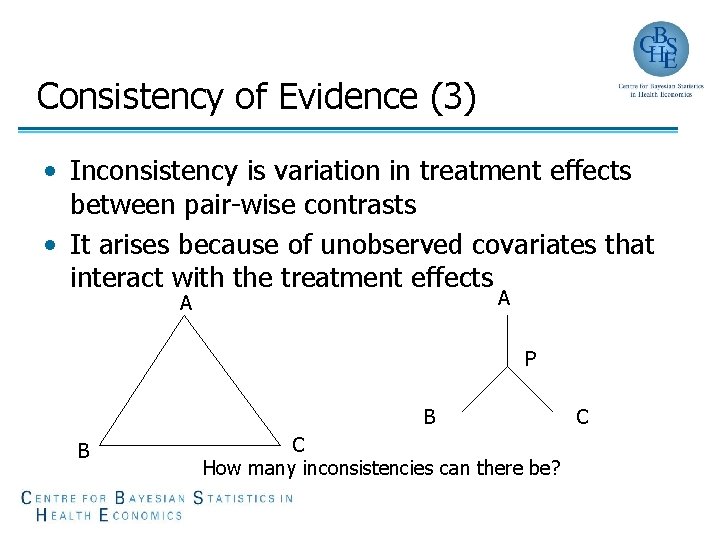
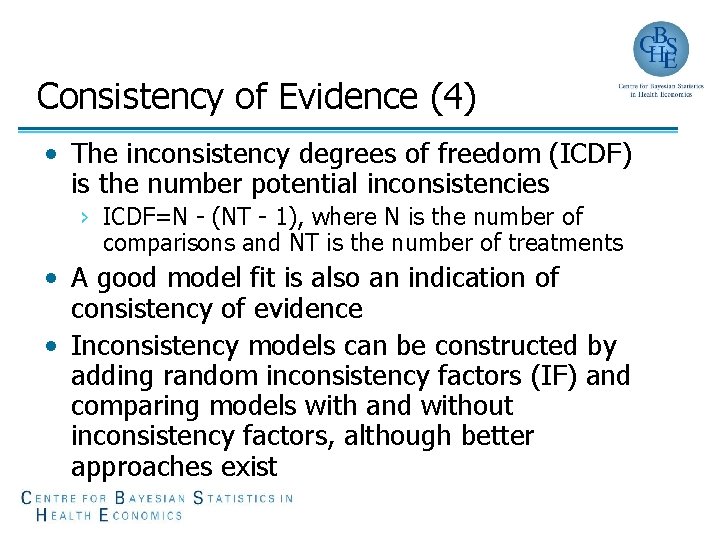
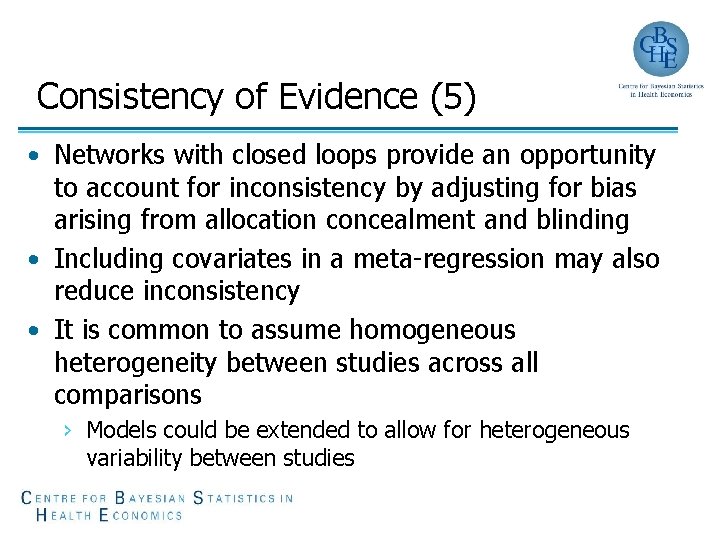
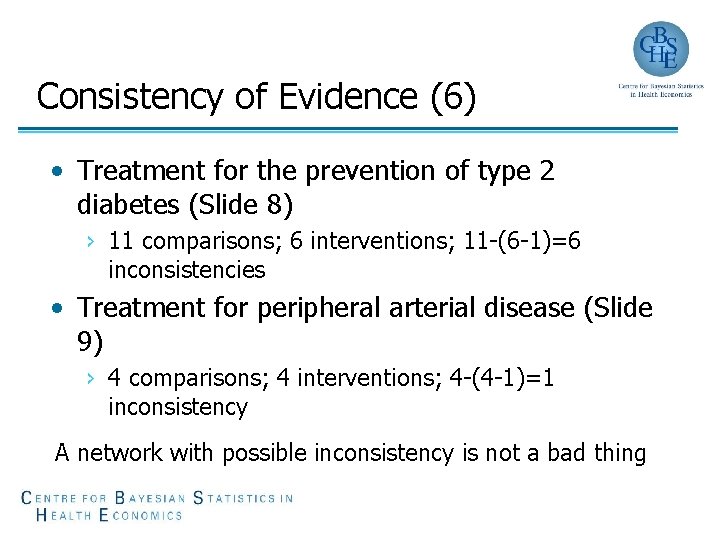
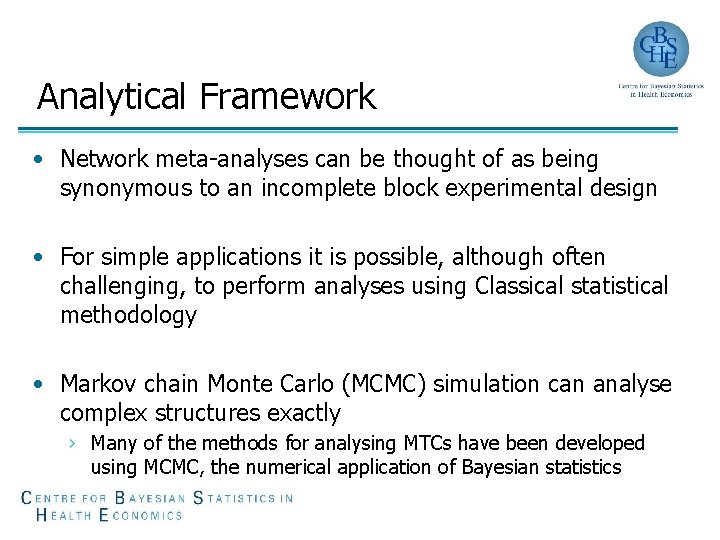
![Model Structure: Example – Peripheral Arterial Disease (1) Observation i is trial s[i] with Model Structure: Example – Peripheral Arterial Disease (1) Observation i is trial s[i] with](https://slidetodoc.com/presentation_image/7a5a89a59497c13f59f5682d6804856d/image-25.jpg)
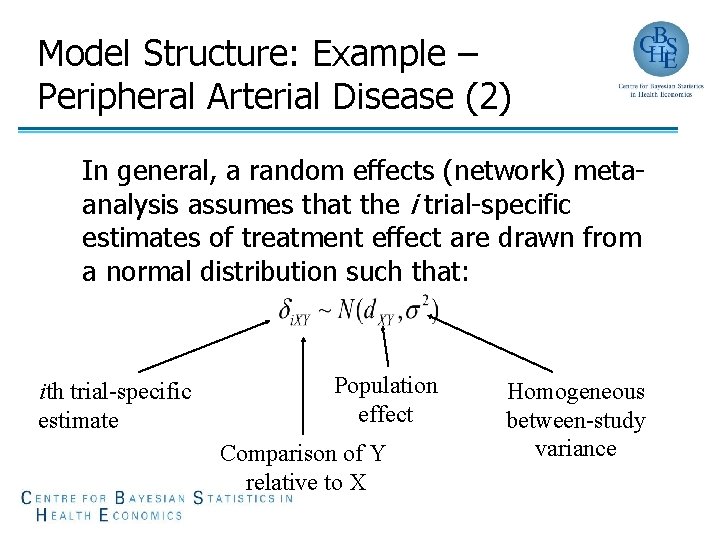
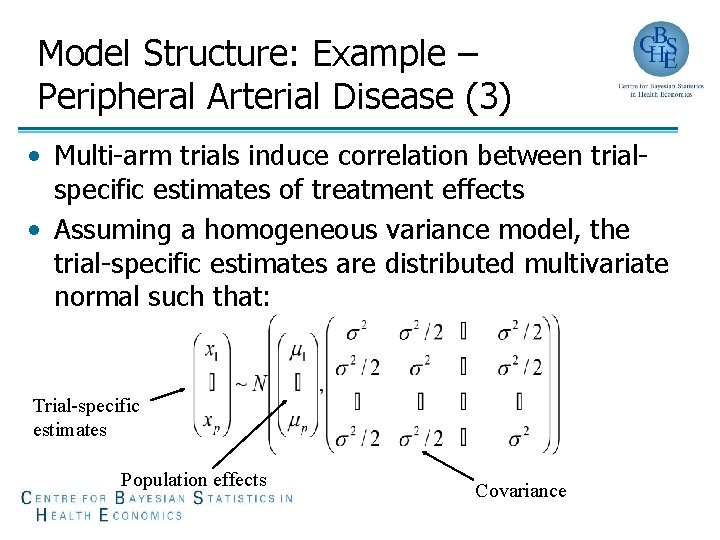
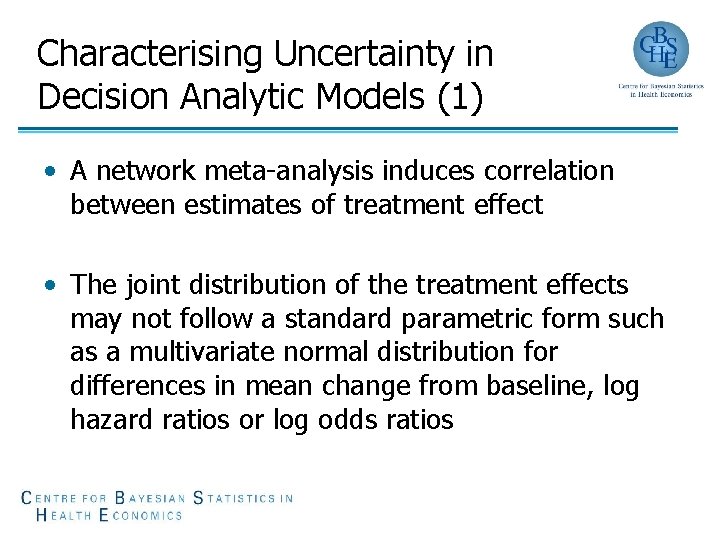
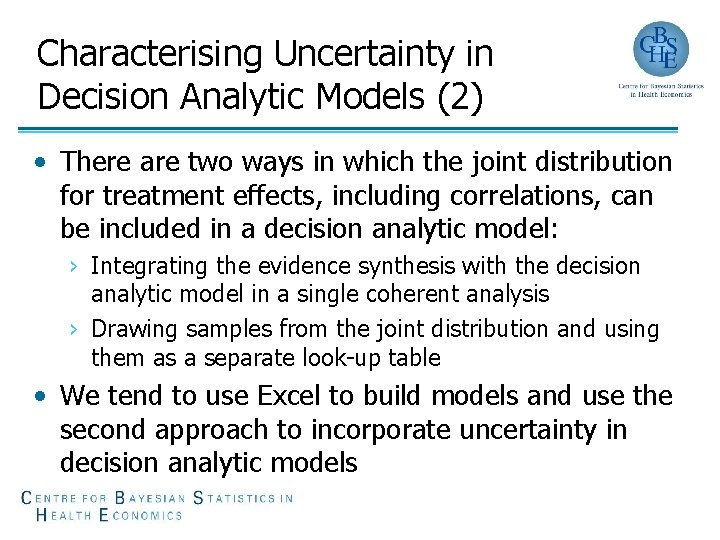
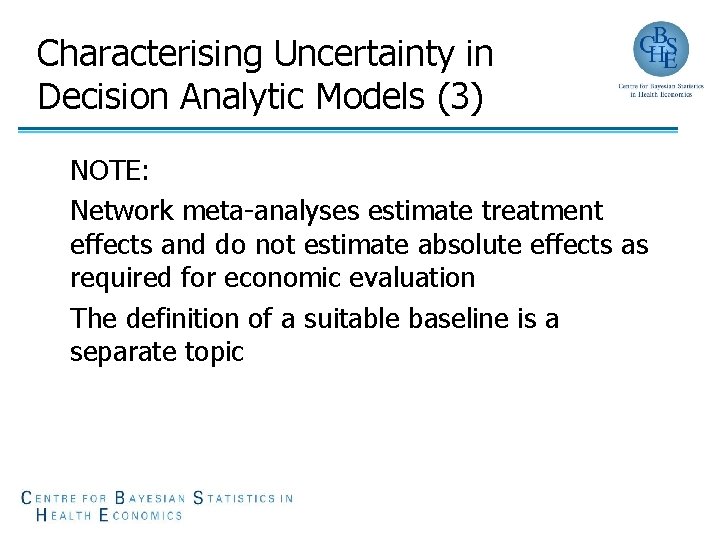
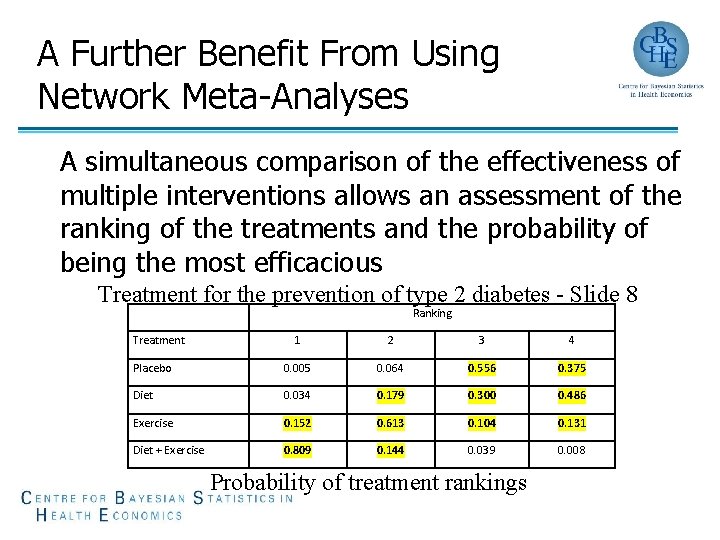
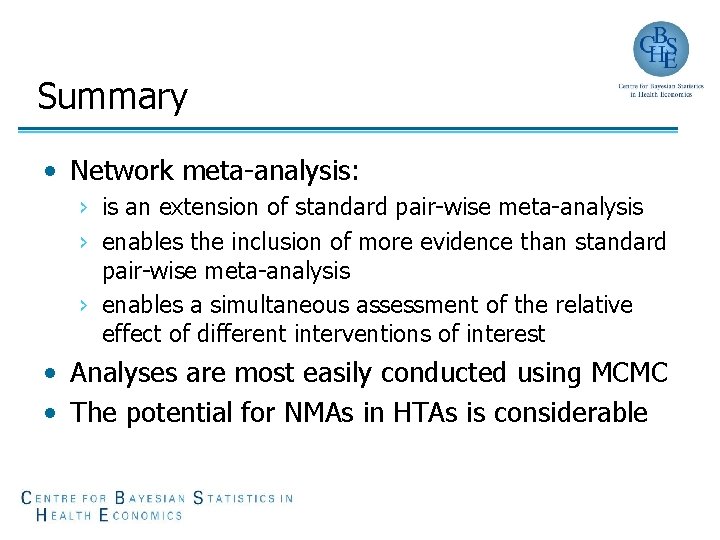
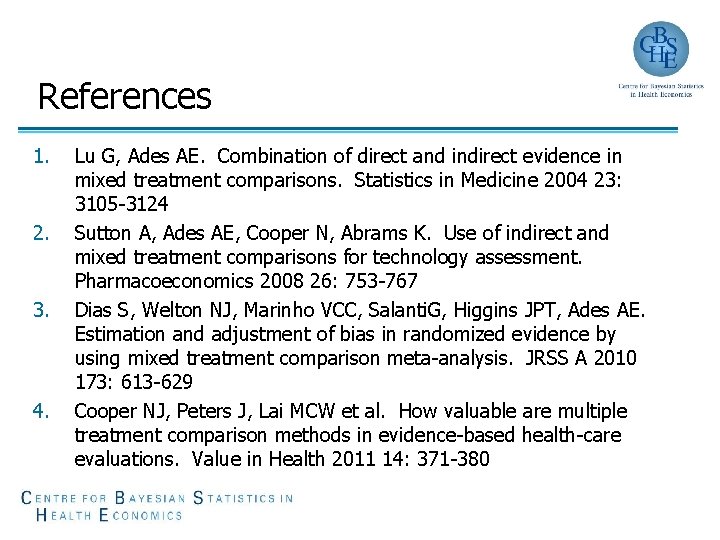
- Slides: 33
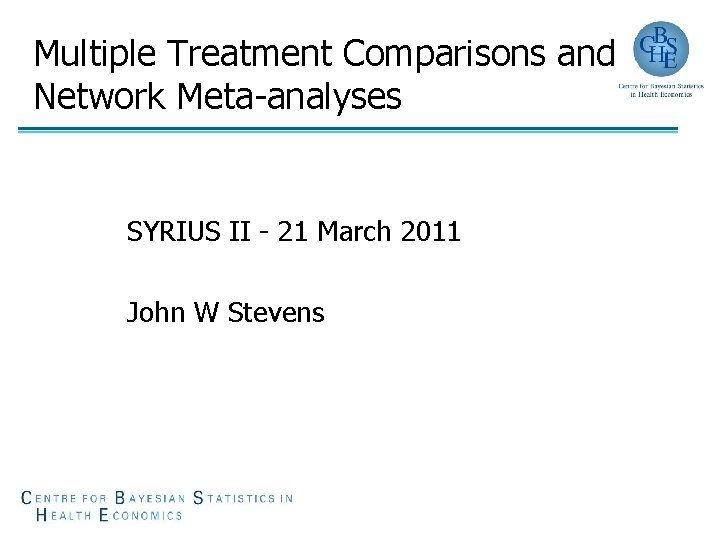
Multiple Treatment Comparisons and Network Meta-analyses SYRIUS II - 21 March 2011 John W Stevens
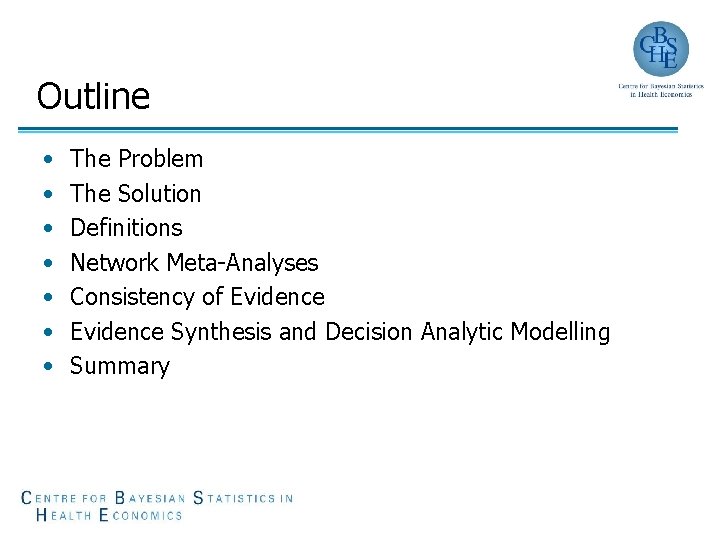
Outline • • The Problem The Solution Definitions Network Meta-Analyses Consistency of Evidence Synthesis and Decision Analytic Modelling Summary
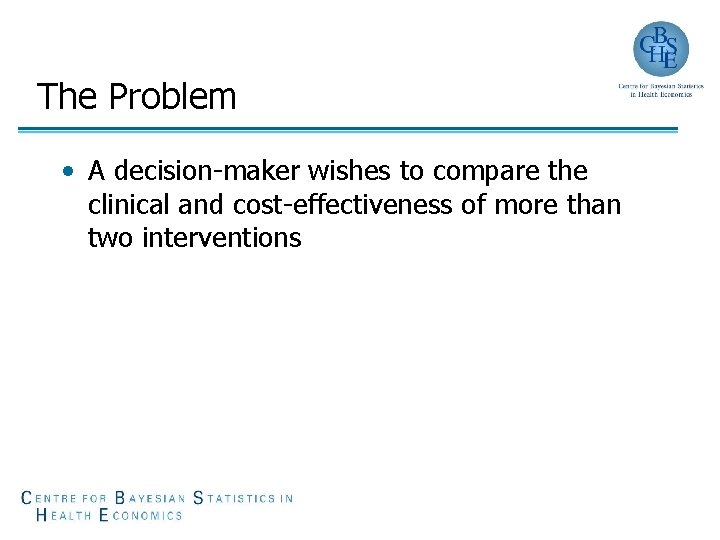
The Problem • A decision-maker wishes to compare the clinical and cost-effectiveness of more than two interventions
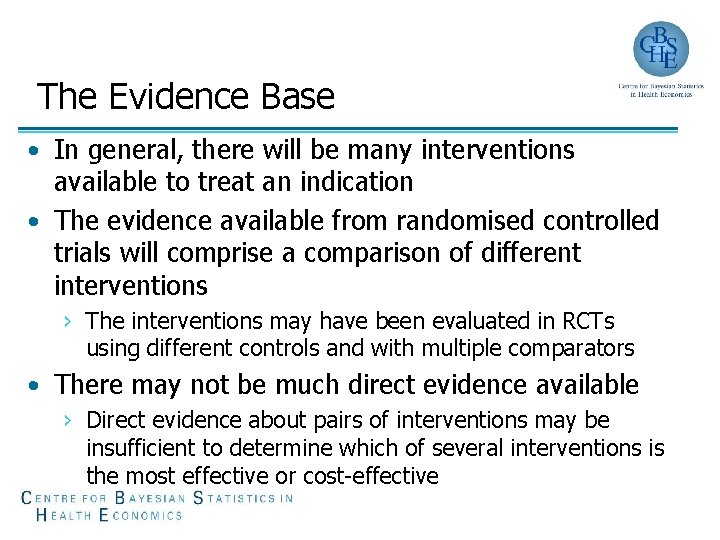
The Evidence Base • In general, there will be many interventions available to treat an indication • The evidence available from randomised controlled trials will comprise a comparison of different interventions › The interventions may have been evaluated in RCTs using different controls and with multiple comparators • There may not be much direct evidence available › Direct evidence about pairs of interventions may be insufficient to determine which of several interventions is the most effective or cost-effective
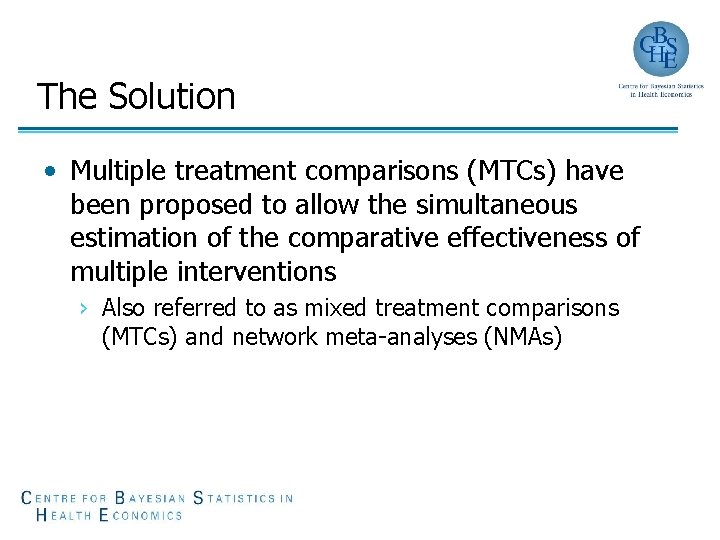
The Solution • Multiple treatment comparisons (MTCs) have been proposed to allow the simultaneous estimation of the comparative effectiveness of multiple interventions › Also referred to as mixed treatment comparisons (MTCs) and network meta-analyses (NMAs)
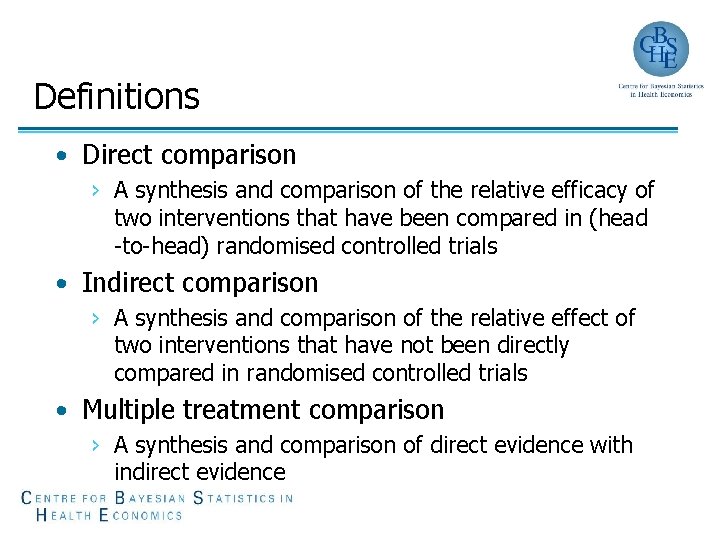
Definitions • Direct comparison › A synthesis and comparison of the relative efficacy of two interventions that have been compared in (head -to-head) randomised controlled trials • Indirect comparison › A synthesis and comparison of the relative effect of two interventions that have not been directly compared in randomised controlled trials • Multiple treatment comparison › A synthesis and comparison of direct evidence with indirect evidence
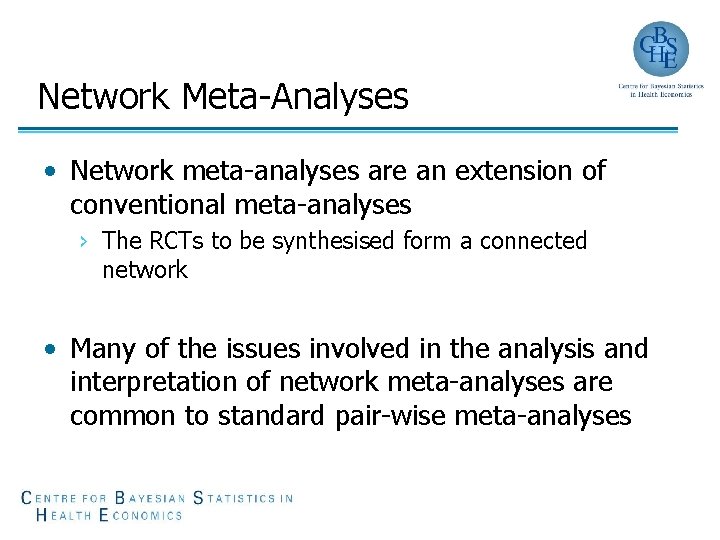
Network Meta-Analyses • Network meta-analyses are an extension of conventional meta-analyses › The RCTs to be synthesised form a connected network • Many of the issues involved in the analysis and interpretation of network meta-analyses are common to standard pair-wise meta-analyses
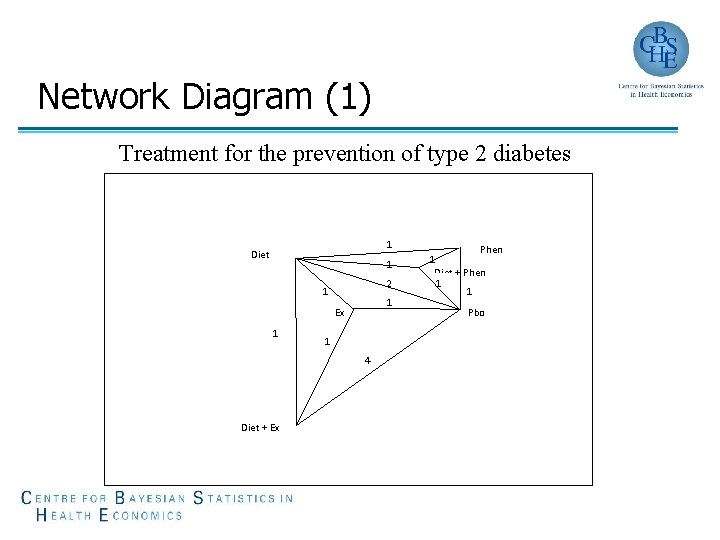
Network Diagram (1) Treatment for the prevention of type 2 diabetes 1 Diet 1 2 1 1 Ex 1 1 4 Diet + Ex 1 Phen Diet + Phen 1 1 Pbo
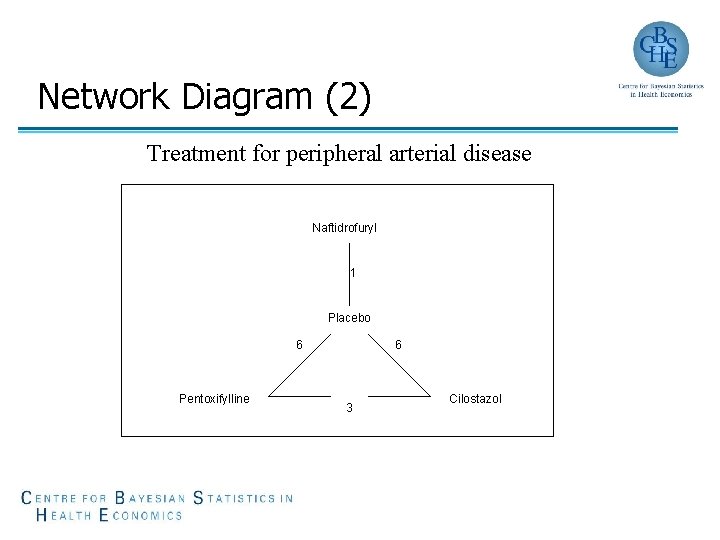
Network Diagram (2) Treatment for peripheral arterial disease Naftidrofuryl 1 Placebo 6 Pentoxifylline 6 3 Cilostazol
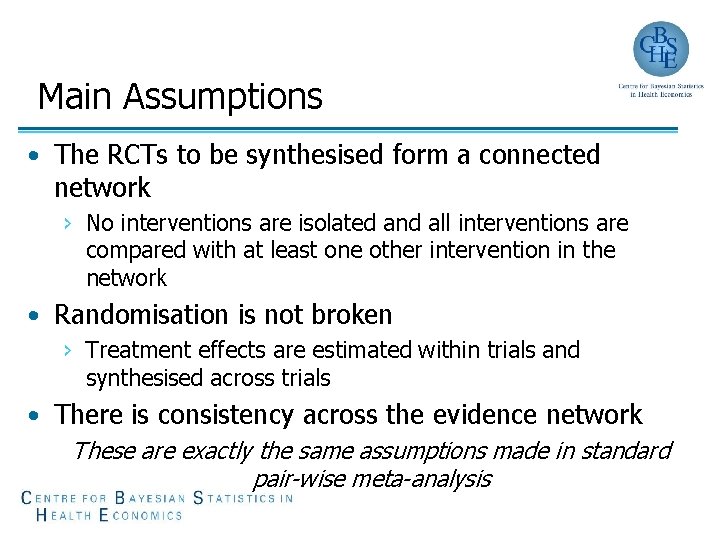
Main Assumptions • The RCTs to be synthesised form a connected network › No interventions are isolated and all interventions are compared with at least one other intervention in the network • Randomisation is not broken › Treatment effects are estimated within trials and synthesised across trials • There is consistency across the evidence network These are exactly the same assumptions made in standard pair-wise meta-analysis
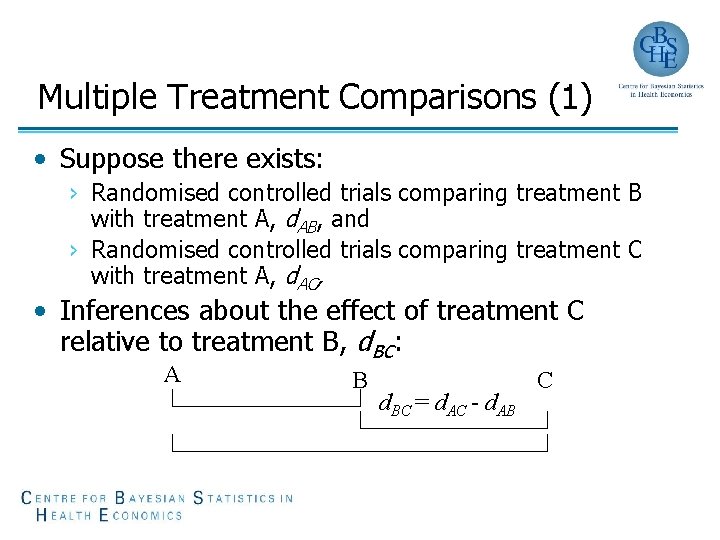
Multiple Treatment Comparisons (1) • Suppose there exists: › Randomised controlled trials comparing treatment B with treatment A, d. AB, and › Randomised controlled trials comparing treatment C with treatment A, d. AC, • Inferences about the effect of treatment C relative to treatment B, d. BC: A B d. BC = d. AC - d. AB C
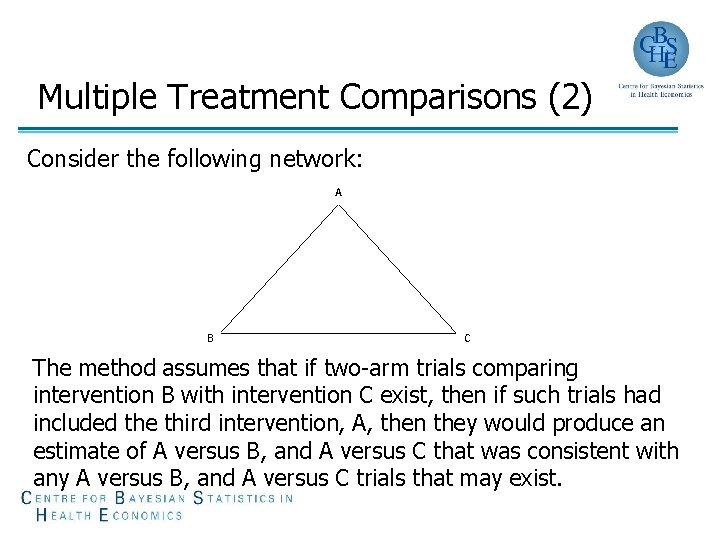
Multiple Treatment Comparisons (2) Consider the following network: A B C The method assumes that if two-arm trials comparing intervention B with intervention C exist, then if such trials had included the third intervention, A, then they would produce an estimate of A versus B, and A versus C that was consistent with any A versus B, and A versus C trials that may exist.
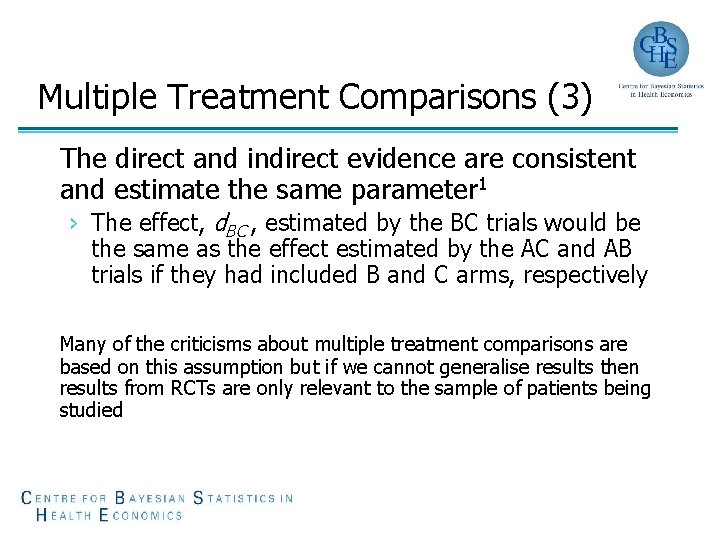
Multiple Treatment Comparisons (3) The direct and indirect evidence are consistent and estimate the same parameter 1 › The effect, d. BC , estimated by the BC trials would be the same as the effect estimated by the AC and AB trials if they had included B and C arms, respectively Many of the criticisms about multiple treatment comparisons are based on this assumption but if we cannot generalise results then results from RCTs are only relevant to the sample of patients being studied
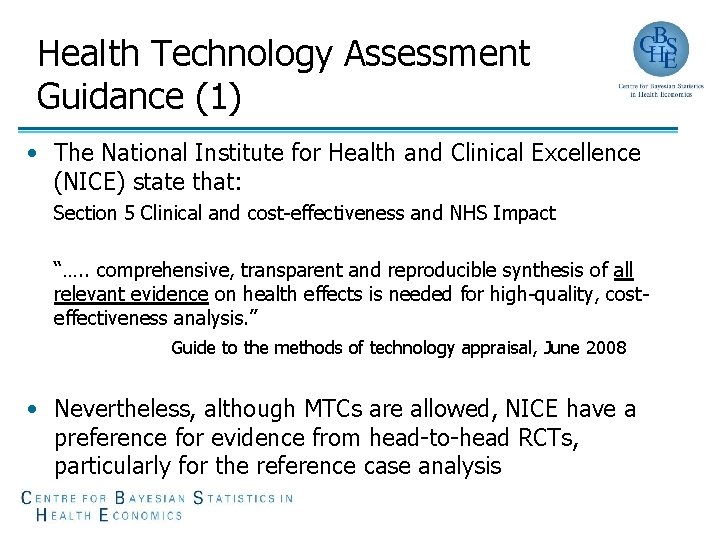
Health Technology Assessment Guidance (1) • The National Institute for Health and Clinical Excellence (NICE) state that: Section 5 Clinical and cost-effectiveness and NHS Impact “…. . comprehensive, transparent and reproducible synthesis of all relevant evidence on health effects is needed for high-quality, costeffectiveness analysis. ” Guide to the methods of technology appraisal, June 2008 • Nevertheless, although MTCs are allowed, NICE have a preference for evidence from head-to-head RCTs, particularly for the reference case analysis
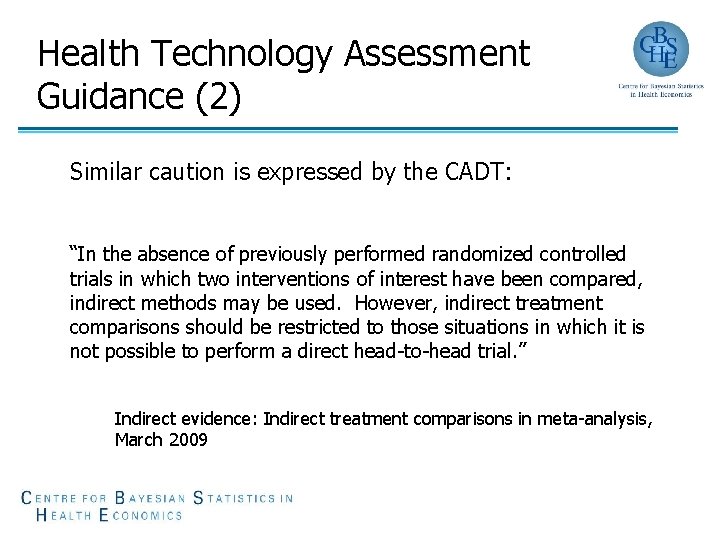
Health Technology Assessment Guidance (2) Similar caution is expressed by the CADT: “In the absence of previously performed randomized controlled trials in which two interventions of interest have been compared, indirect methods may be used. However, indirect treatment comparisons should be restricted to those situations in which it is not possible to perform a direct head-to-head trial. ” Indirect evidence: Indirect treatment comparisons in meta-analysis, March 2009
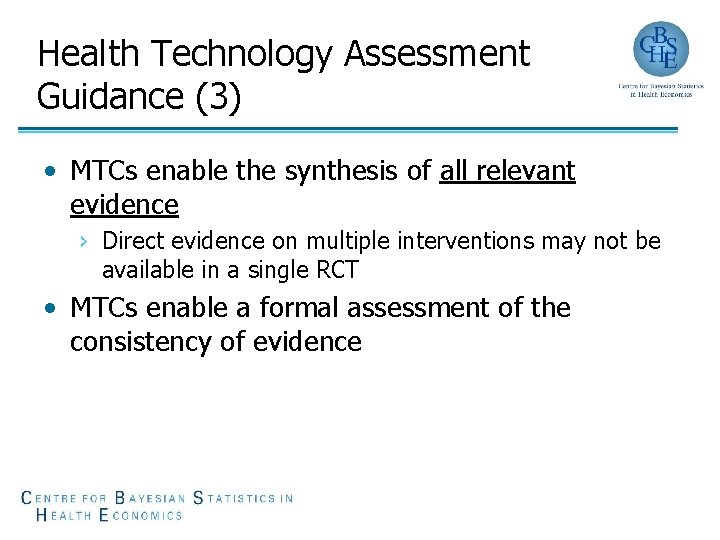
Health Technology Assessment Guidance (3) • MTCs enable the synthesis of all relevant evidence › Direct evidence on multiple interventions may not be available in a single RCT • MTCs enable a formal assessment of the consistency of evidence
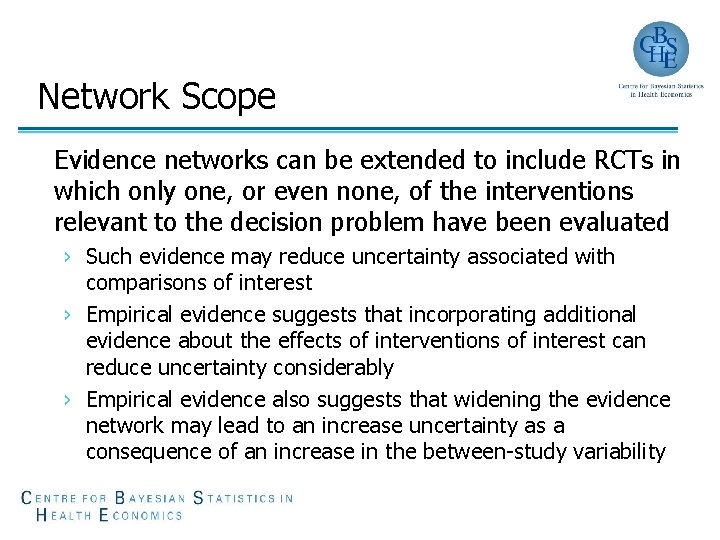
Network Scope Evidence networks can be extended to include RCTs in which only one, or even none, of the interventions relevant to the decision problem have been evaluated › Such evidence may reduce uncertainty associated with comparisons of interest › Empirical evidence suggests that incorporating additional evidence about the effects of interventions of interest can reduce uncertainty considerably › Empirical evidence also suggests that widening the evidence network may lead to an increase uncertainty as a consequence of an increase in the between-study variability
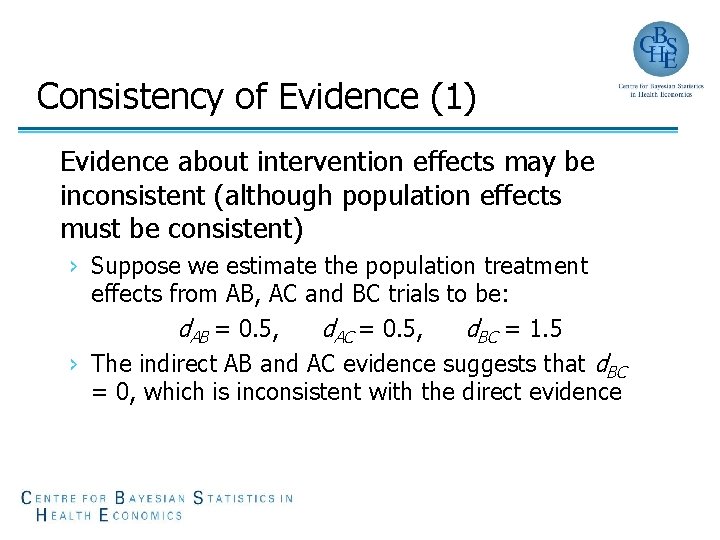
Consistency of Evidence (1) Evidence about intervention effects may be inconsistent (although population effects must be consistent) › Suppose we estimate the population treatment effects from AB, AC and BC trials to be: d. AB = 0. 5, d. AC = 0. 5, d. BC = 1. 5 › The indirect AB and AC evidence suggests that d. BC = 0, which is inconsistent with the direct evidence
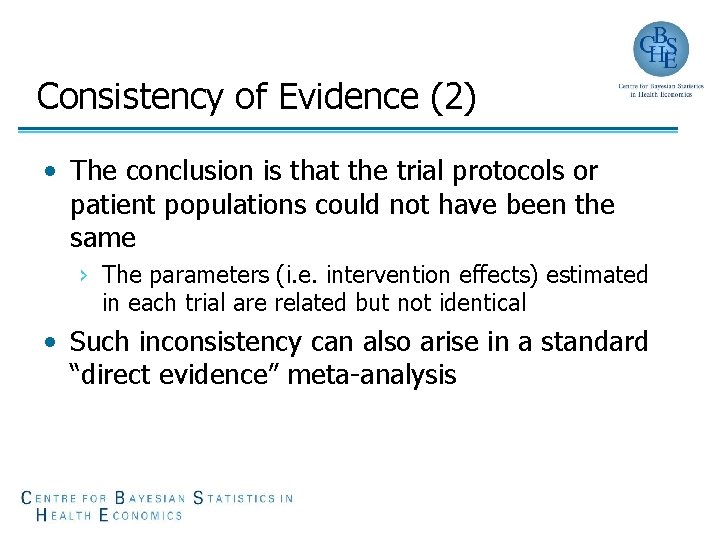
Consistency of Evidence (2) • The conclusion is that the trial protocols or patient populations could not have been the same › The parameters (i. e. intervention effects) estimated in each trial are related but not identical • Such inconsistency can also arise in a standard “direct evidence” meta-analysis
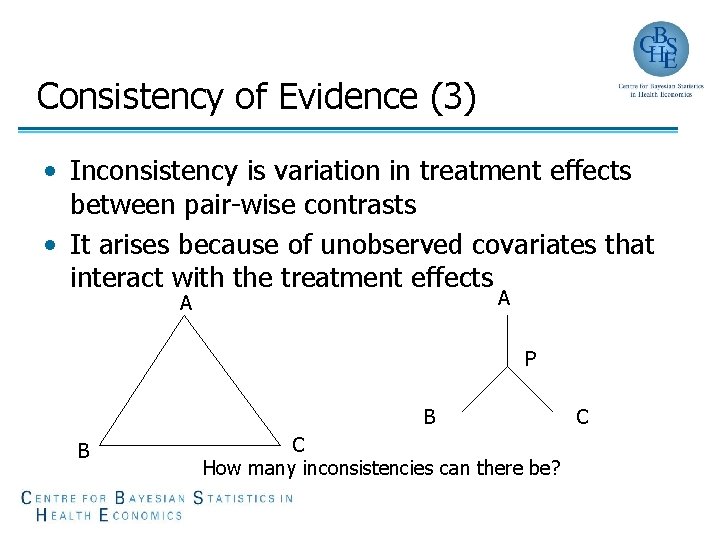
Consistency of Evidence (3) • Inconsistency is variation in treatment effects between pair-wise contrasts • It arises because of unobserved covariates that interact with the treatment effects A A P B B C How many inconsistencies can there be? C
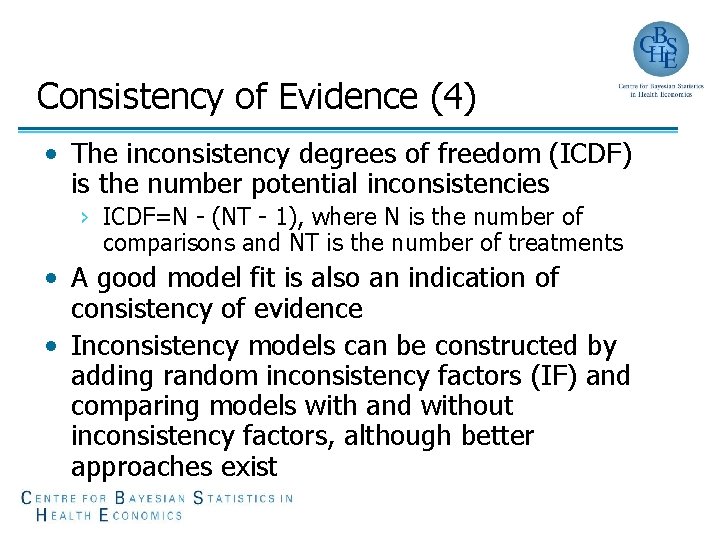
Consistency of Evidence (4) • The inconsistency degrees of freedom (ICDF) is the number potential inconsistencies › ICDF=N - (NT - 1), where N is the number of comparisons and NT is the number of treatments • A good model fit is also an indication of consistency of evidence • Inconsistency models can be constructed by adding random inconsistency factors (IF) and comparing models with and without inconsistency factors, although better approaches exist
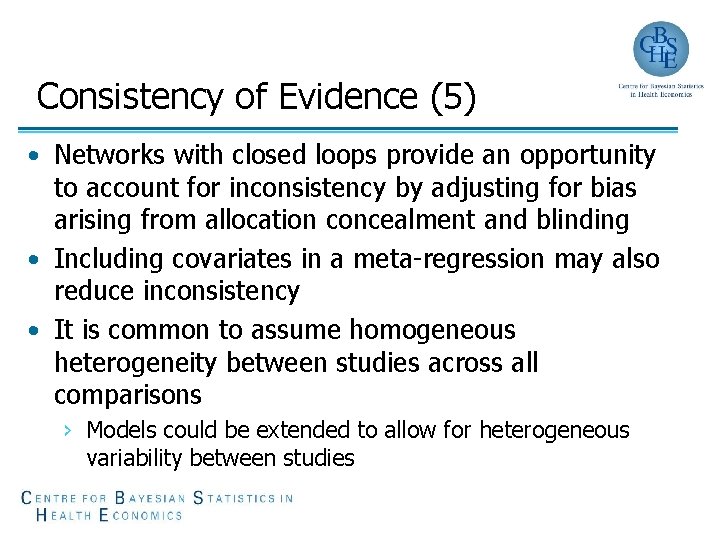
Consistency of Evidence (5) • Networks with closed loops provide an opportunity to account for inconsistency by adjusting for bias arising from allocation concealment and blinding • Including covariates in a meta-regression may also reduce inconsistency • It is common to assume homogeneous heterogeneity between studies across all comparisons › Models could be extended to allow for heterogeneous variability between studies
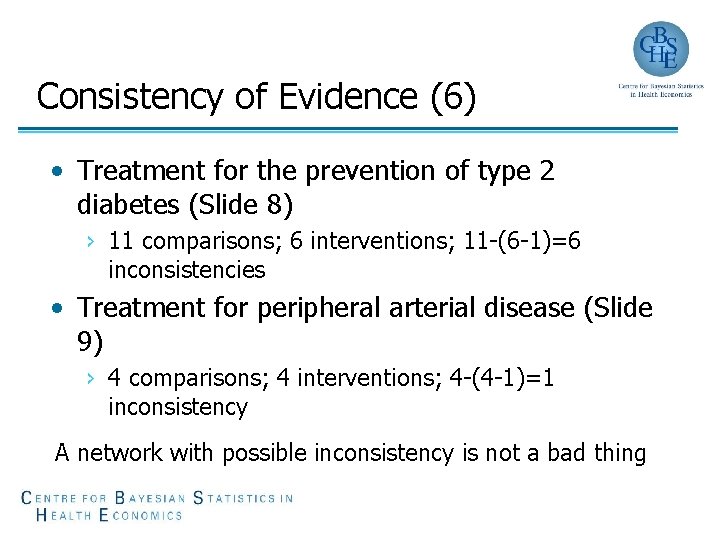
Consistency of Evidence (6) • Treatment for the prevention of type 2 diabetes (Slide 8) › 11 comparisons; 6 interventions; 11 -(6 -1)=6 inconsistencies • Treatment for peripheral arterial disease (Slide 9) › 4 comparisons; 4 interventions; 4 -(4 -1)=1 inconsistency A network with possible inconsistency is not a bad thing
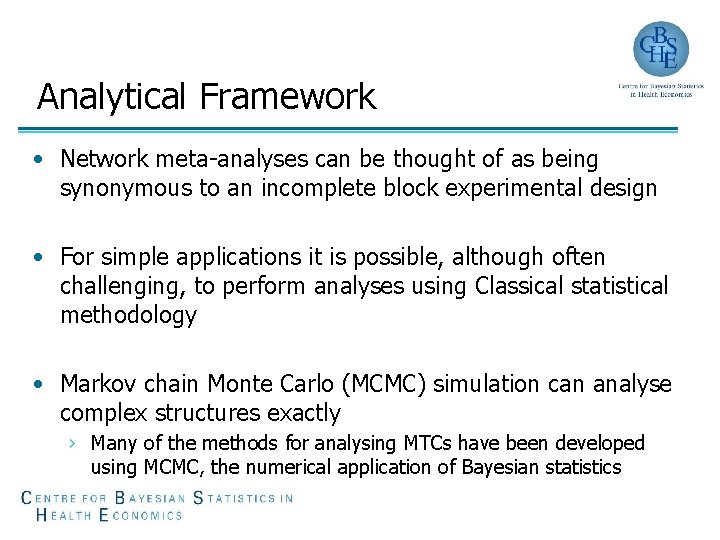
Analytical Framework • Network meta-analyses can be thought of as being synonymous to an incomplete block experimental design • For simple applications it is possible, although often challenging, to perform analyses using Classical statistical methodology • Markov chain Monte Carlo (MCMC) simulation can analyse complex structures exactly › Many of the methods for analysing MTCs have been developed using MCMC, the numerical application of Bayesian statistics
![Model Structure Example Peripheral Arterial Disease 1 Observation i is trial si with Model Structure: Example – Peripheral Arterial Disease (1) Observation i is trial s[i] with](https://slidetodoc.com/presentation_image/7a5a89a59497c13f59f5682d6804856d/image-25.jpg)
Model Structure: Example – Peripheral Arterial Disease (1) Observation i is trial s[i] with intervention t[i] compared to control treatment b[i]: x[i]) <- mu[s[i]] + d[t[i]] – d[b[i]] A v B trial Av C trial A v D trial A V B v C trial arm A d[1] – d[1] mu[s[i]] arm B d[2] – d[1] mu[s[i]] + d[2] arm A d[1] – d[1] mu[s[i]] arm C d[3] – d[1] mu[s[i]] + d[3] arm A d[1] – d[1] mu[s[i]] arm D d[4] – d[1] mu[s[i]] + d[4] arm A d[1] – d[1] mu[s[i]] arm B d[2] – d[1] mu[s[i]] + d[2] – d[1] d. AB arm c d[3] – d[1] mu[s[i]] + d[3] – d[1] d. AC d. AB d. AC d. AD
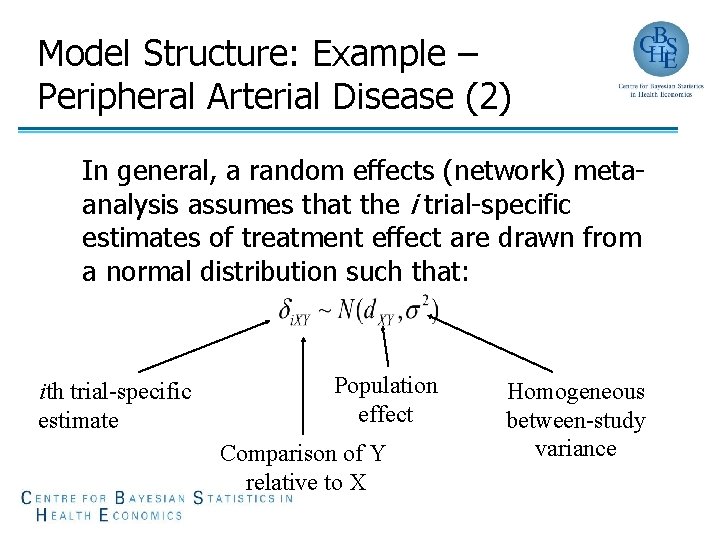
Model Structure: Example – Peripheral Arterial Disease (2) In general, a random effects (network) metaanalysis assumes that the i trial-specific estimates of treatment effect are drawn from a normal distribution such that: ith trial-specific estimate Population effect Comparison of Y relative to X Homogeneous between-study variance
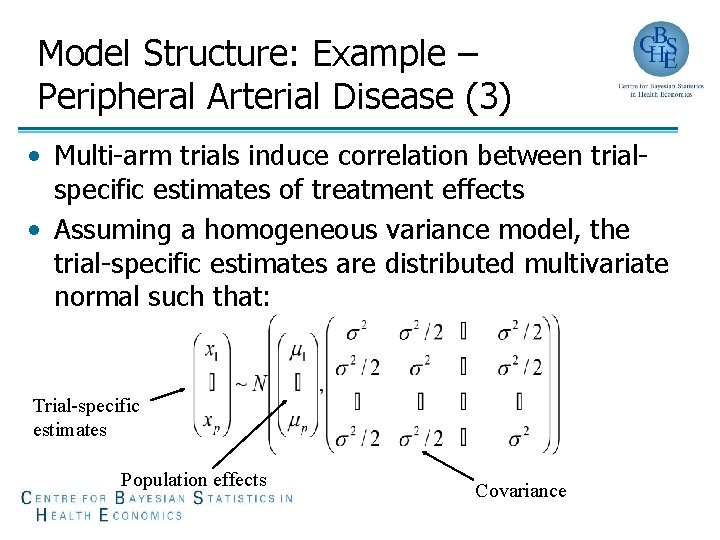
Model Structure: Example – Peripheral Arterial Disease (3) • Multi-arm trials induce correlation between trialspecific estimates of treatment effects • Assuming a homogeneous variance model, the trial-specific estimates are distributed multivariate normal such that: Trial-specific estimates Population effects Covariance
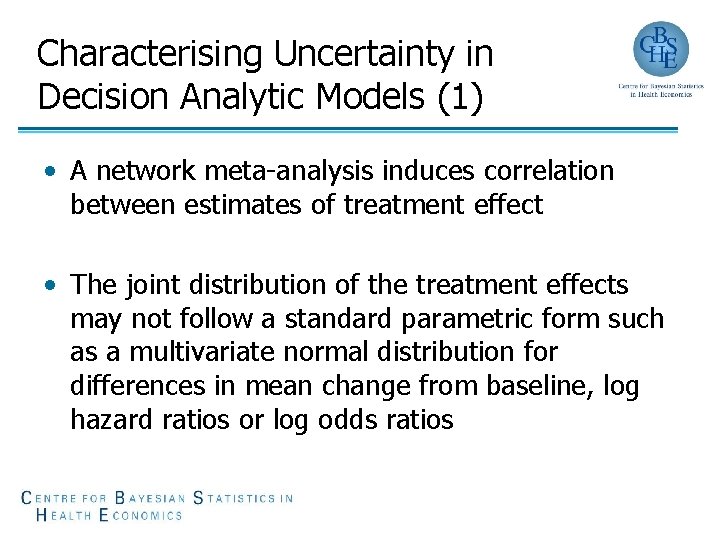
Characterising Uncertainty in Decision Analytic Models (1) • A network meta-analysis induces correlation between estimates of treatment effect • The joint distribution of the treatment effects may not follow a standard parametric form such as a multivariate normal distribution for differences in mean change from baseline, log hazard ratios or log odds ratios
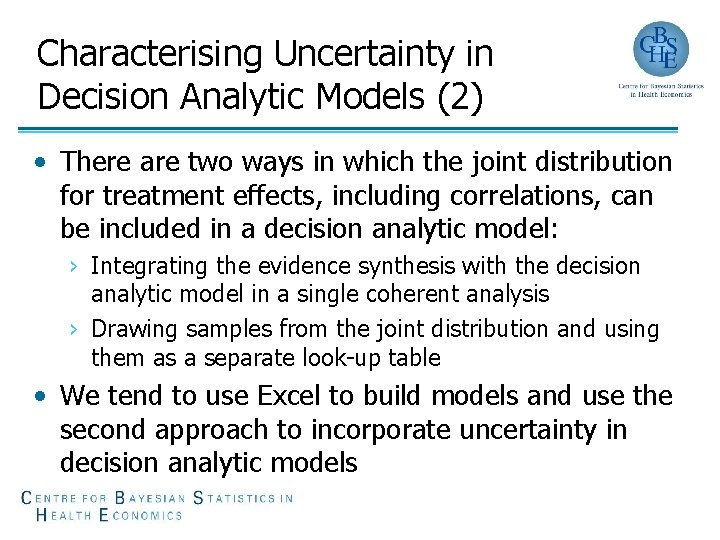
Characterising Uncertainty in Decision Analytic Models (2) • There are two ways in which the joint distribution for treatment effects, including correlations, can be included in a decision analytic model: › Integrating the evidence synthesis with the decision analytic model in a single coherent analysis › Drawing samples from the joint distribution and using them as a separate look-up table • We tend to use Excel to build models and use the second approach to incorporate uncertainty in decision analytic models
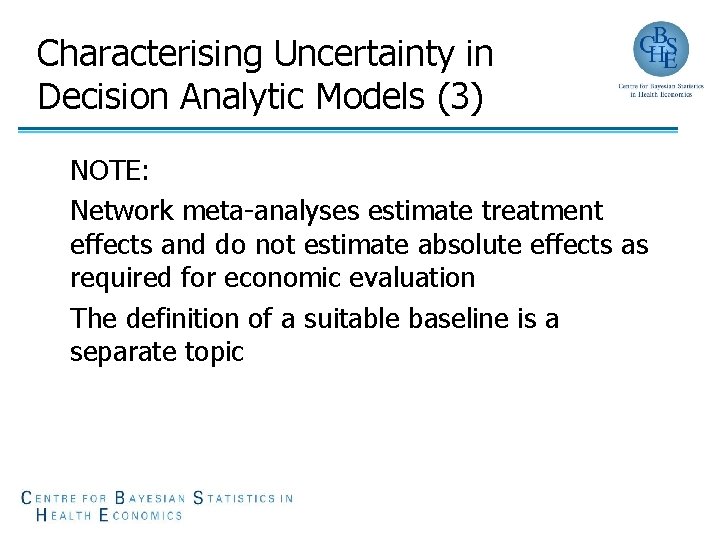
Characterising Uncertainty in Decision Analytic Models (3) NOTE: Network meta-analyses estimate treatment effects and do not estimate absolute effects as required for economic evaluation The definition of a suitable baseline is a separate topic
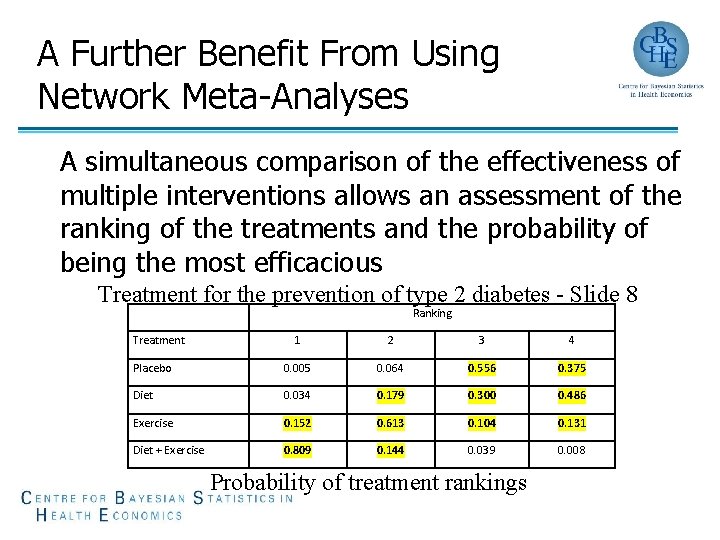
A Further Benefit From Using Network Meta-Analyses A simultaneous comparison of the effectiveness of multiple interventions allows an assessment of the ranking of the treatments and the probability of being the most efficacious Treatment for the prevention of type 2 diabetes - Slide 8 Ranking Treatment 1 2 3 4 Placebo 0. 005 0. 064 0. 556 0. 375 Diet 0. 034 0. 179 0. 300 0. 486 Exercise 0. 152 0. 613 0. 104 0. 131 Diet + Exercise 0. 809 0. 144 0. 039 0. 008 Probability of treatment rankings
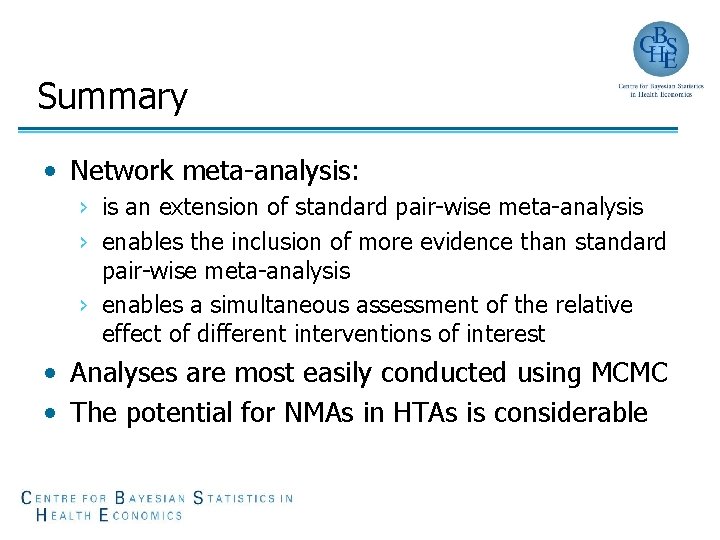
Summary • Network meta-analysis: › is an extension of standard pair-wise meta-analysis › enables the inclusion of more evidence than standard pair-wise meta-analysis › enables a simultaneous assessment of the relative effect of different interventions of interest • Analyses are most easily conducted using MCMC • The potential for NMAs in HTAs is considerable
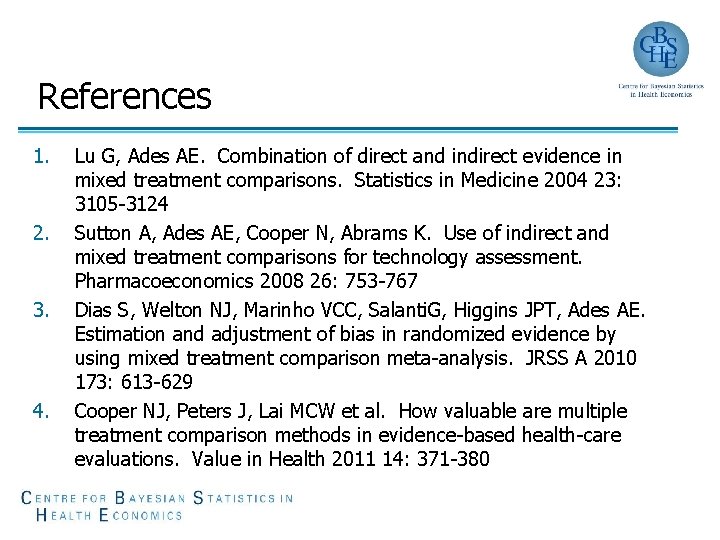
References 1. 2. 3. 4. Lu G, Ades AE. Combination of direct and indirect evidence in mixed treatment comparisons. Statistics in Medicine 2004 23: 3105 -3124 Sutton A, Ades AE, Cooper N, Abrams K. Use of indirect and mixed treatment comparisons for technology assessment. Pharmacoeconomics 2008 26: 753 -767 Dias S, Welton NJ, Marinho VCC, Salanti. G, Higgins JPT, Ades AE. Estimation and adjustment of bias in randomized evidence by using mixed treatment comparison meta-analysis. JRSS A 2010 173: 613 -629 Cooper NJ, Peters J, Lai MCW et al. How valuable are multiple treatment comparison methods in evidence-based health-care evaluations. Value in Health 2011 14: 371 -380
Max bensadon
Xkcd jellybeans
Aba reversal design
Delayed multiple baseline design
Shared memory mimd architecture
A priori and post hoc comparisons
Adjective equality
Adverbs that compare
Comparisons of adjectives and adverbs
Comparisons of equality spanish
Nucleus simile
Comparative superlative good
Making comparisons pictures
Inequality ejemplos
Ernesto mira mas television que alberto
Comparative of equality ejemplos
Selection sort number of comparisons
Equal comparisons
Comparative and superlative modifiers
Modifiers of comparisons
Realidades 2 capitulo 1b making comparisons
Comparatives equality
Insertion sort big o notation
Comparisons in french
Old comparatives
A lot far much comparative
Planned contrasts
Tú eres (less) simpático que federico.
Realidades 2 capitulo 1b making comparisons answers
Adjectives and degrees of comparison
Poems with comparisons
Quantifiers to make comparisons
Comparisons with as
Internet price comparisons