Mimicking human texture classification Eva M van Rikxoort
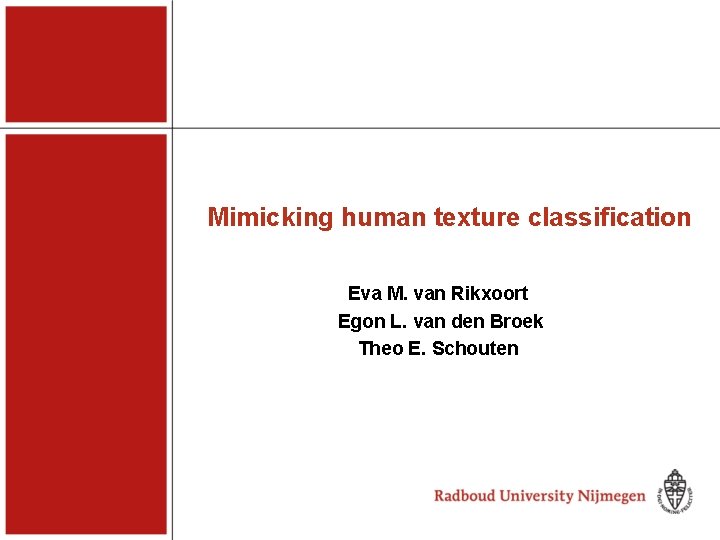
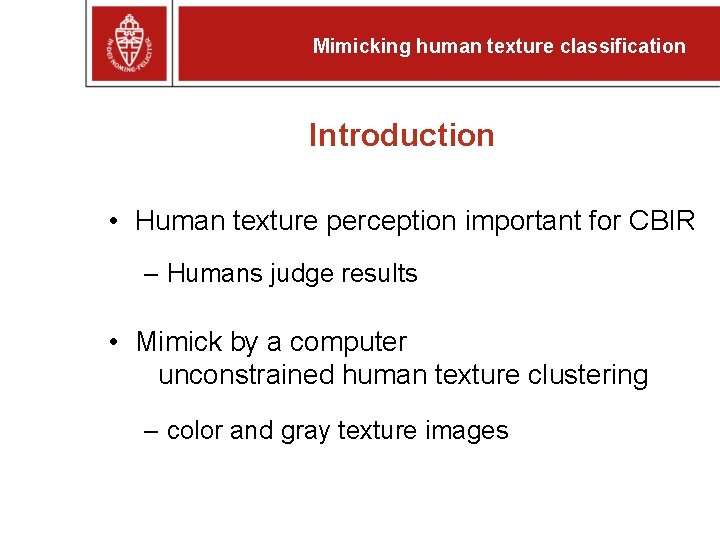
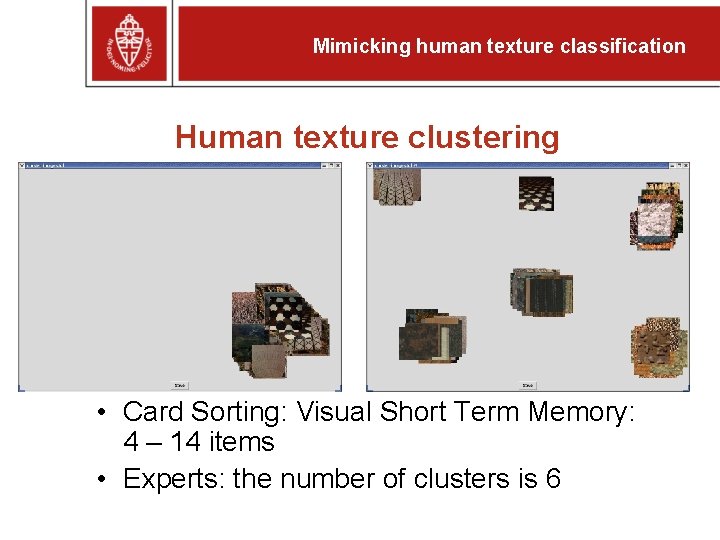
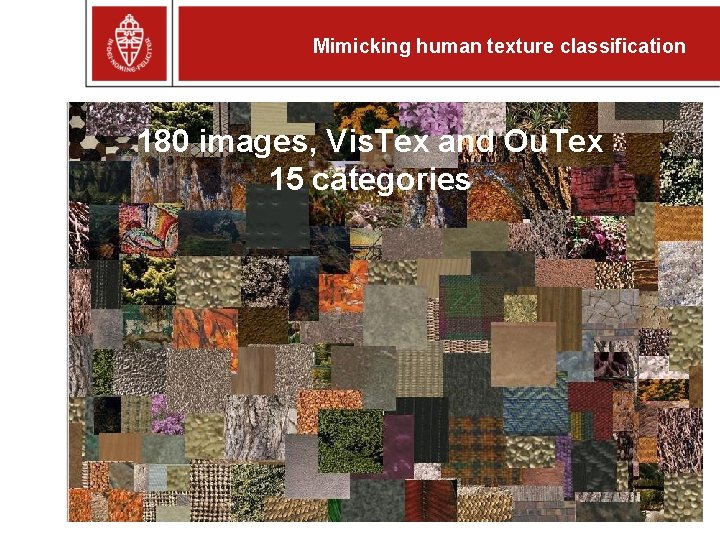
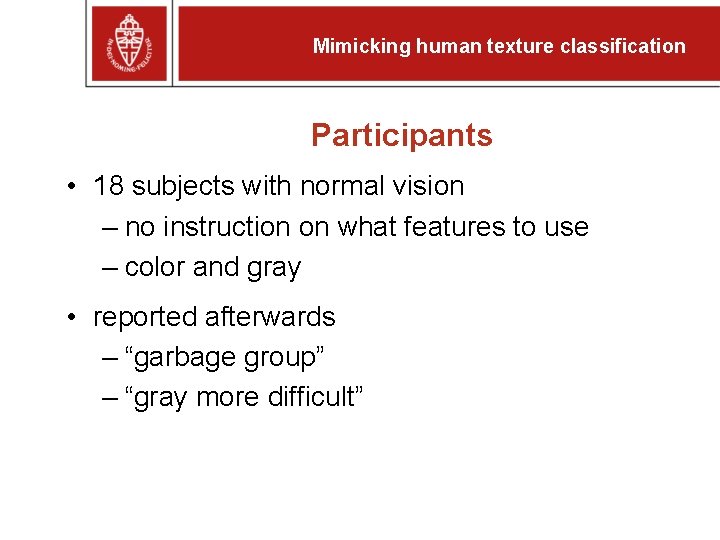
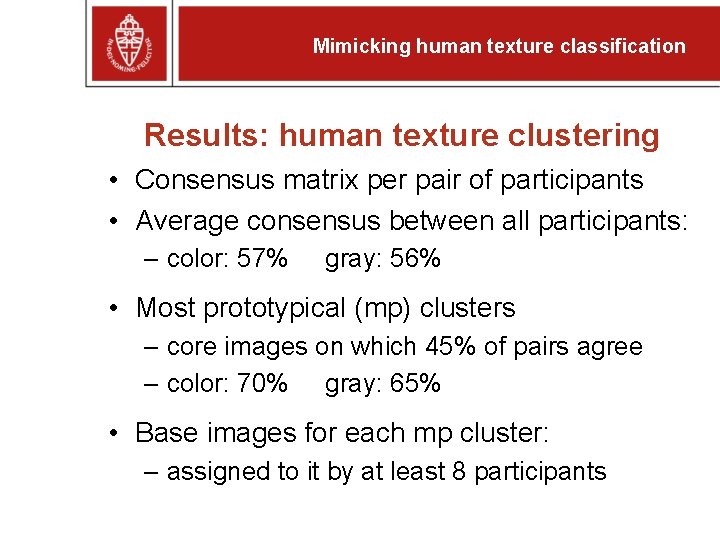
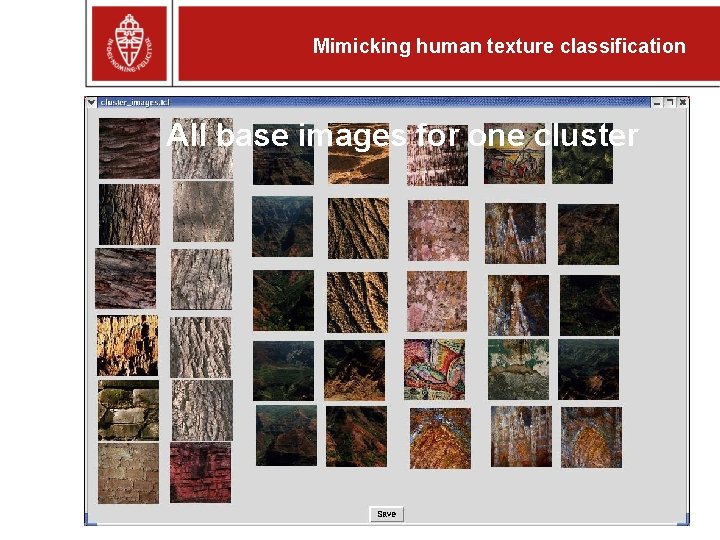
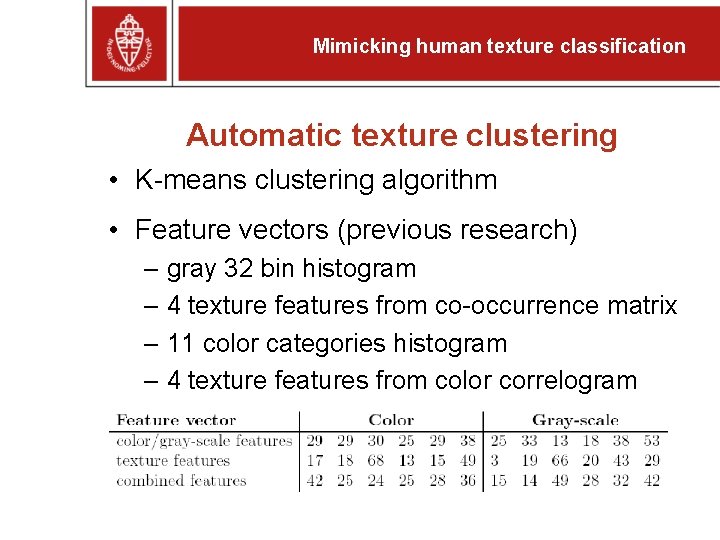
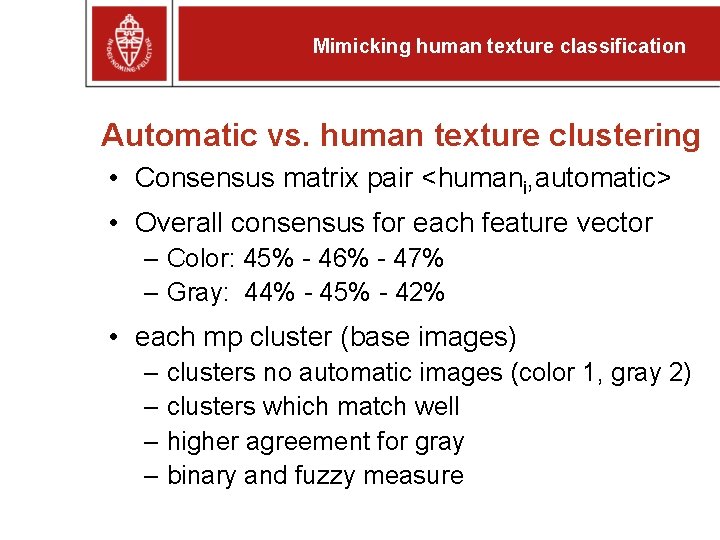
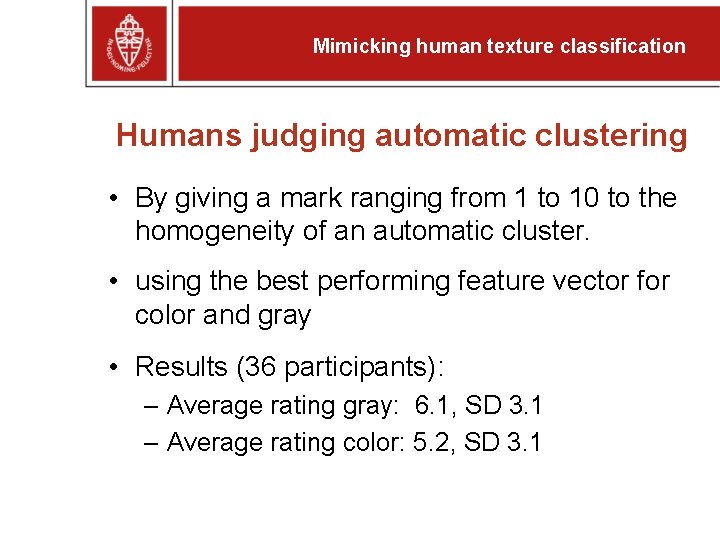
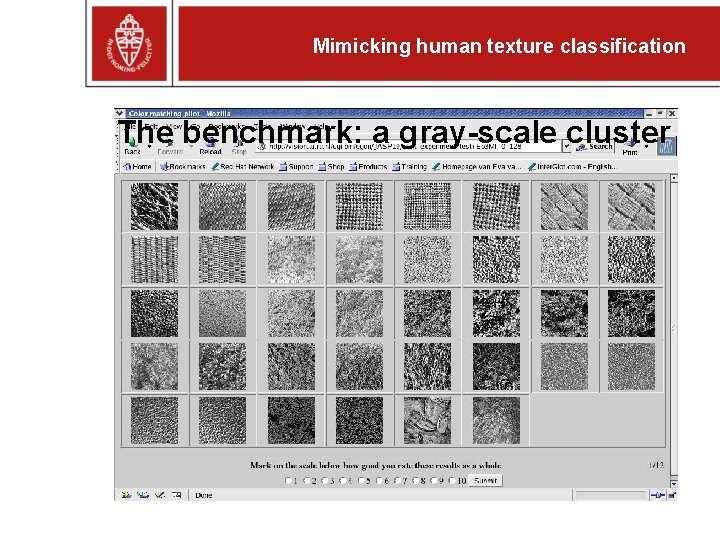
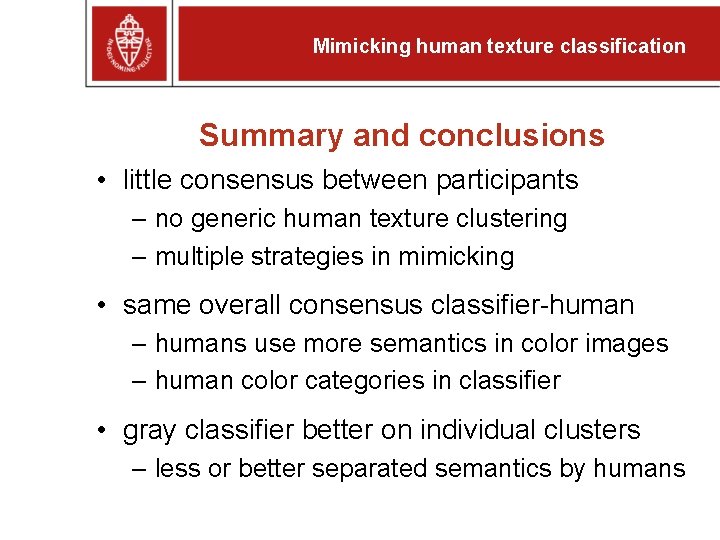
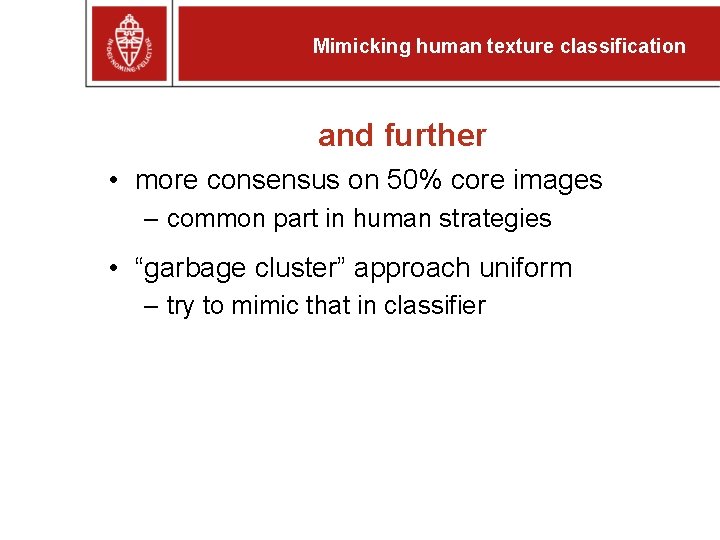
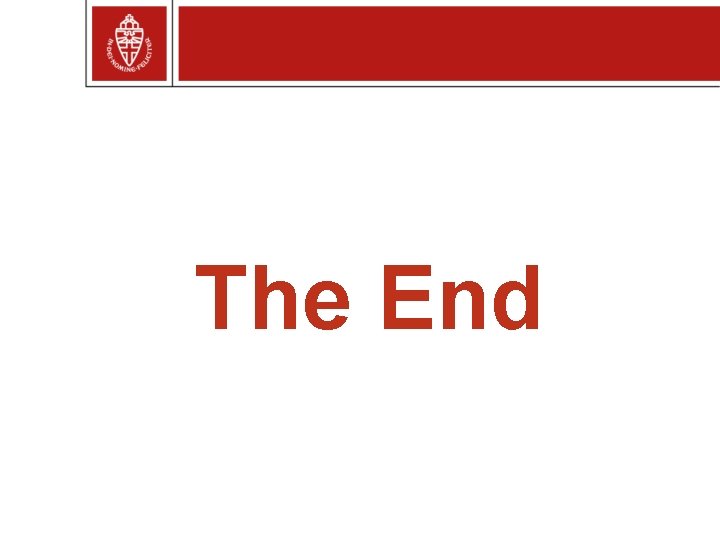
- Slides: 14
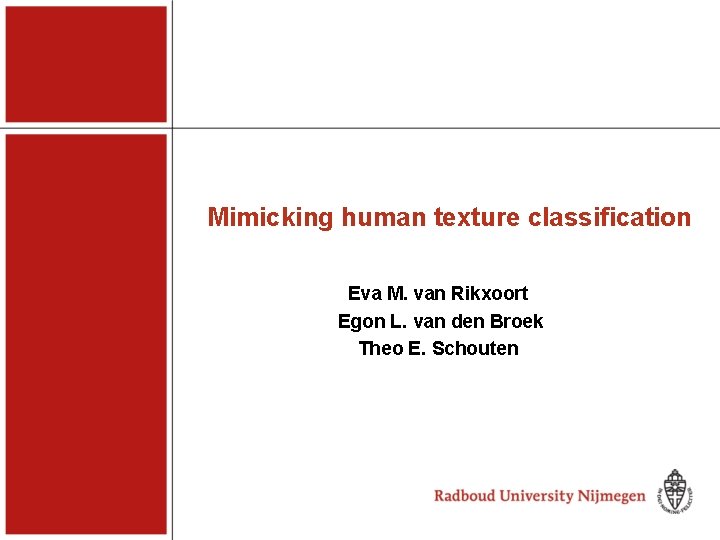
Mimicking human texture classification Eva M. van Rikxoort Egon L. van den Broek Theo E. Schouten
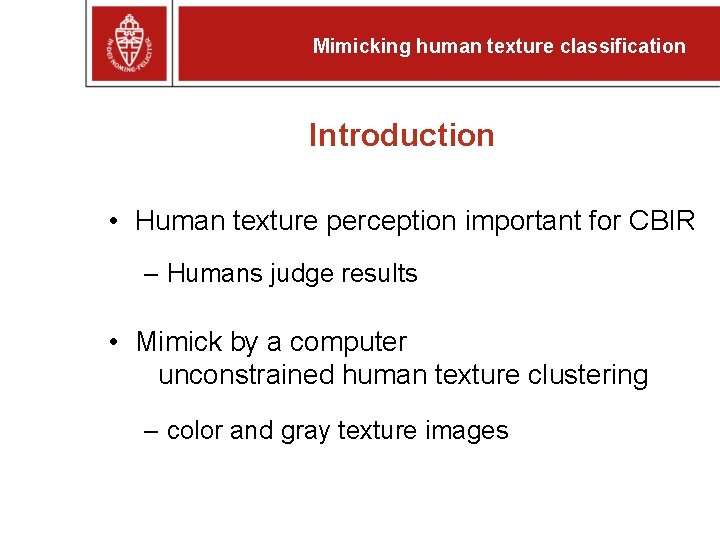
Mimicking human texture classification Introduction • Human texture perception important for CBIR – Humans judge results • Mimick by a computer unconstrained human texture clustering – color and gray texture images
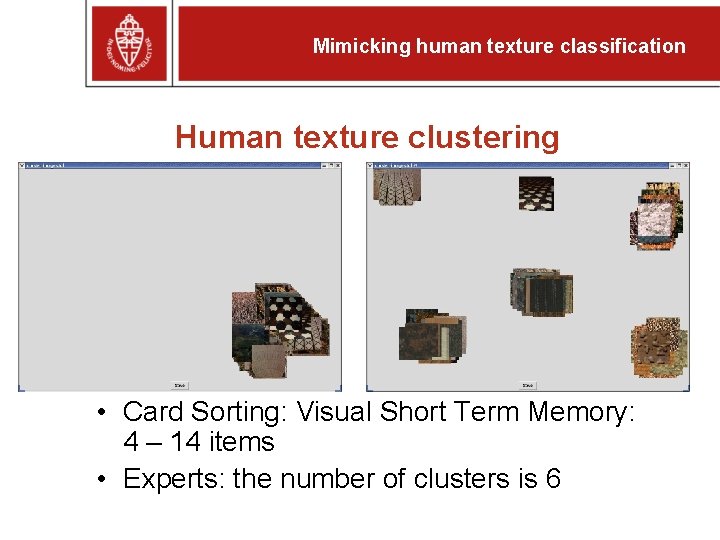
Mimicking human texture classification Human texture clustering • Card Sorting: Visual Short Term Memory: 4 – 14 items • Experts: the number of clusters is 6
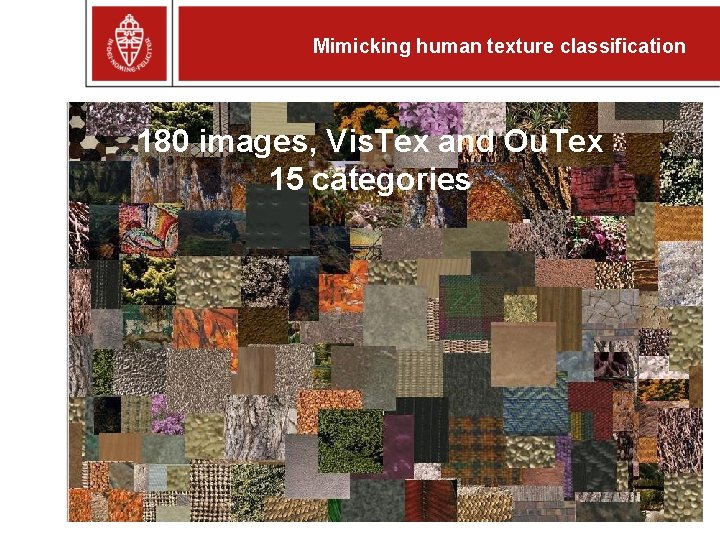
Mimicking human texture classification 180 images, Vis. Tex and Ou. Tex 15 categories
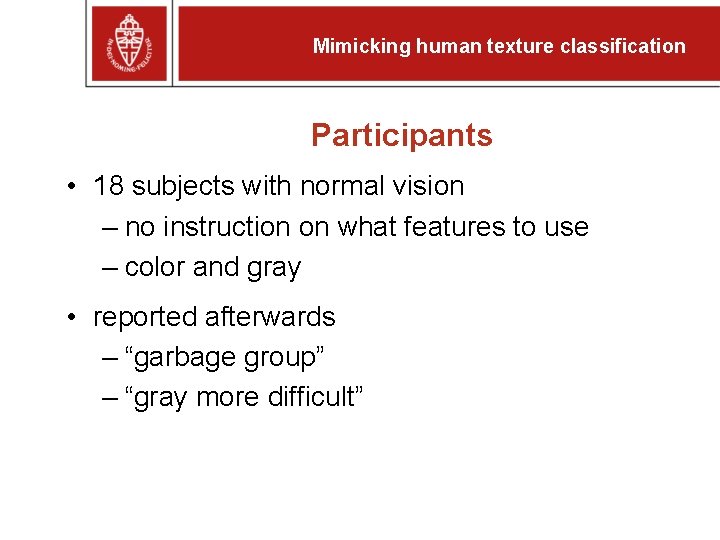
Mimicking human texture classification Participants • 18 subjects with normal vision – no instruction on what features to use – color and gray • reported afterwards – “garbage group” – “gray more difficult”
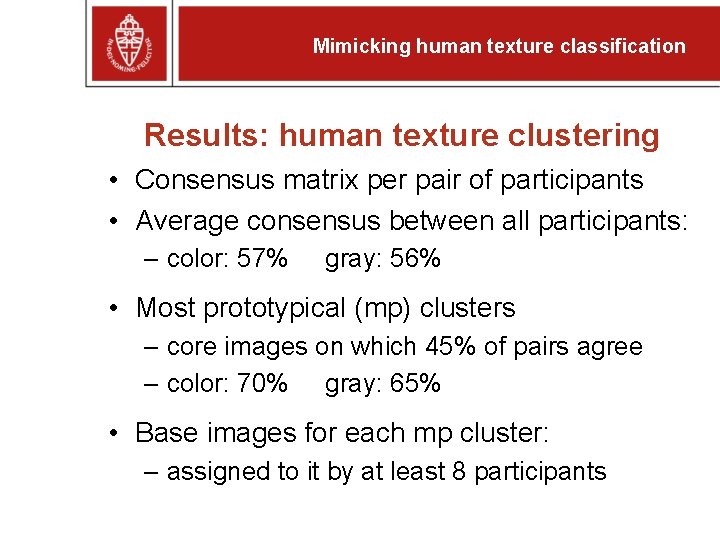
Mimicking human texture classification Results: human texture clustering • Consensus matrix per pair of participants • Average consensus between all participants: – color: 57% gray: 56% • Most prototypical (mp) clusters – core images on which 45% of pairs agree – color: 70% gray: 65% • Base images for each mp cluster: – assigned to it by at least 8 participants
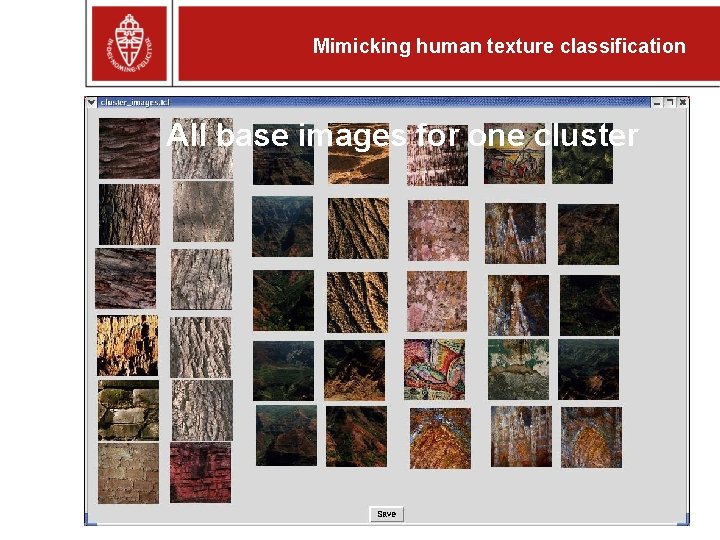
Mimicking human texture classification All base images for one cluster
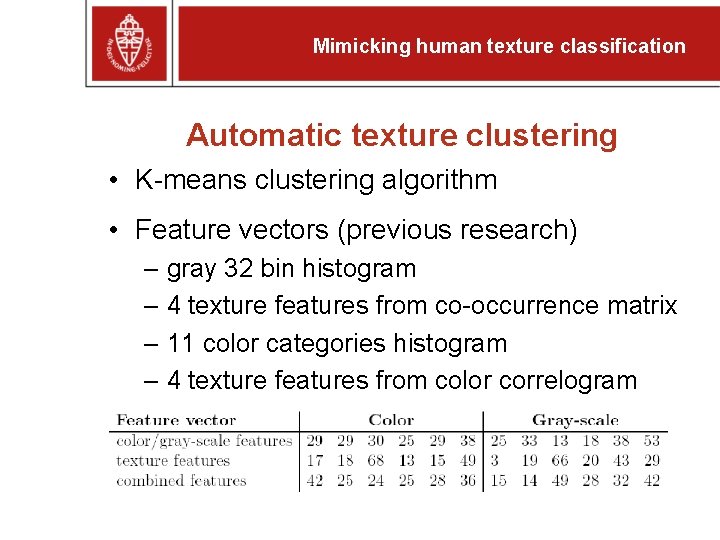
Mimicking human texture classification Automatic texture clustering • K-means clustering algorithm • Feature vectors (previous research) – gray 32 bin histogram – 4 texture features from co-occurrence matrix – 11 color categories histogram – 4 texture features from color correlogram
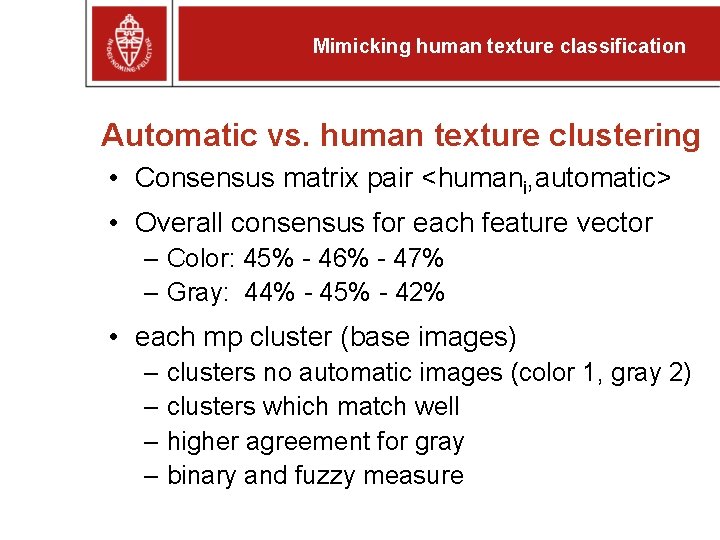
Mimicking human texture classification Automatic vs. human texture clustering • Consensus matrix pair <humani, automatic> • Overall consensus for each feature vector – Color: 45% - 46% - 47% – Gray: 44% - 45% - 42% • each mp cluster (base images) – clusters no automatic images (color 1, gray 2) – clusters which match well – higher agreement for gray – binary and fuzzy measure
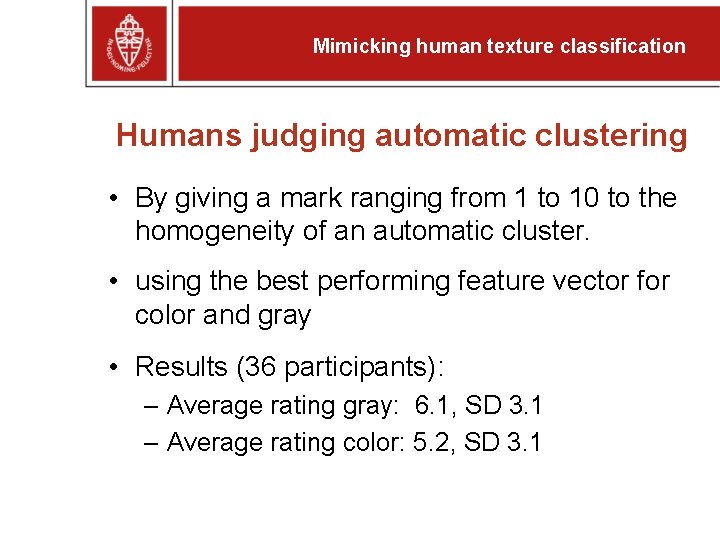
Mimicking human texture classification Humans judging automatic clustering • By giving a mark ranging from 1 to 10 to the homogeneity of an automatic cluster. • using the best performing feature vector for color and gray • Results (36 participants): – Average rating gray: 6. 1, SD 3. 1 – Average rating color: 5. 2, SD 3. 1
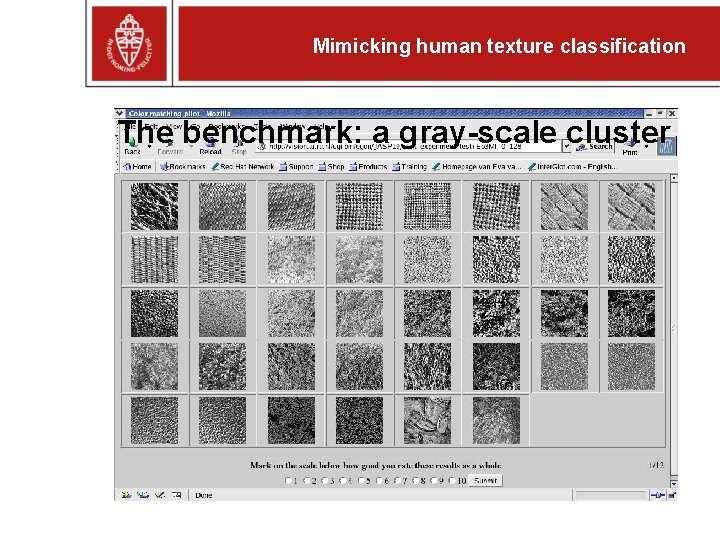
Mimicking human texture classification The benchmark: a gray-scale cluster
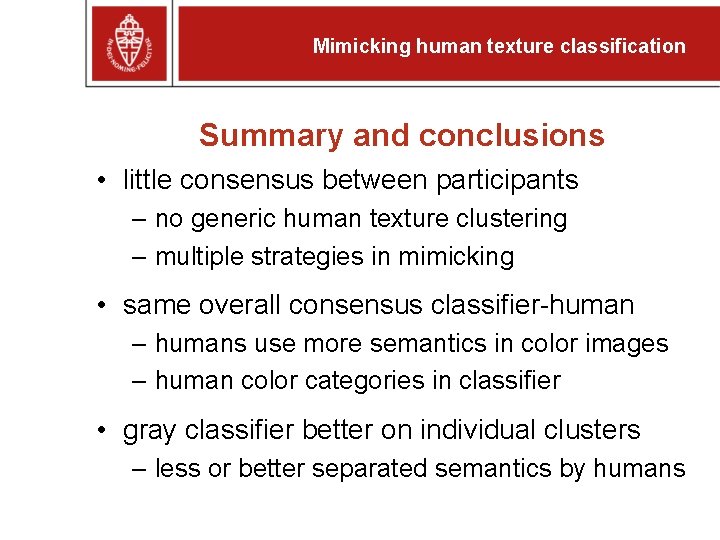
Mimicking human texture classification Summary and conclusions • little consensus between participants – no generic human texture clustering – multiple strategies in mimicking • same overall consensus classifier-human – humans use more semantics in color images – human color categories in classifier • gray classifier better on individual clusters – less or better separated semantics by humans
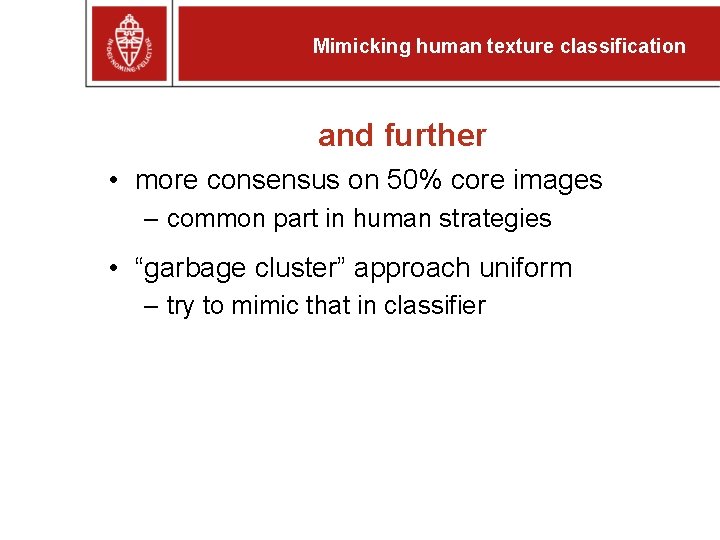
Mimicking human texture classification and further • more consensus on 50% core images – common part in human strategies • “garbage cluster” approach uniform – try to mimic that in classifier
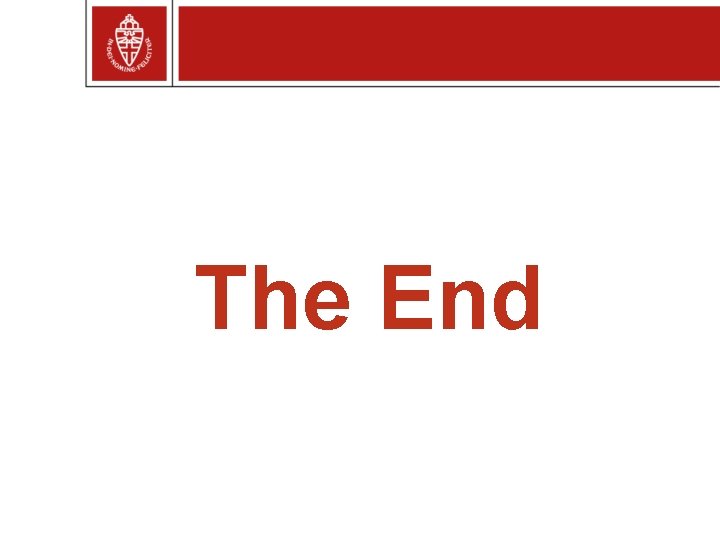
The End
Eva van rikxoort
Outsiders vocabulary chapter 7-9
I/o management in operating system
Write a poem about your dragon mimicking anglo-saxon style
The surface quality or "feel" of an object.
Eva van den broek
Human vs non human bones
Cna chapter 8 human needs and human development
Chapter 8 human needs and human development
Human development index definition ap human geography
Human and non human nouns
Trappe van vergelyking opgewonde
Wet van behoud van impuls
Het stokske van johan van oldenbarnevelt
Wet van behoud van gedoe