Machine translation markers in postedited machine translation output
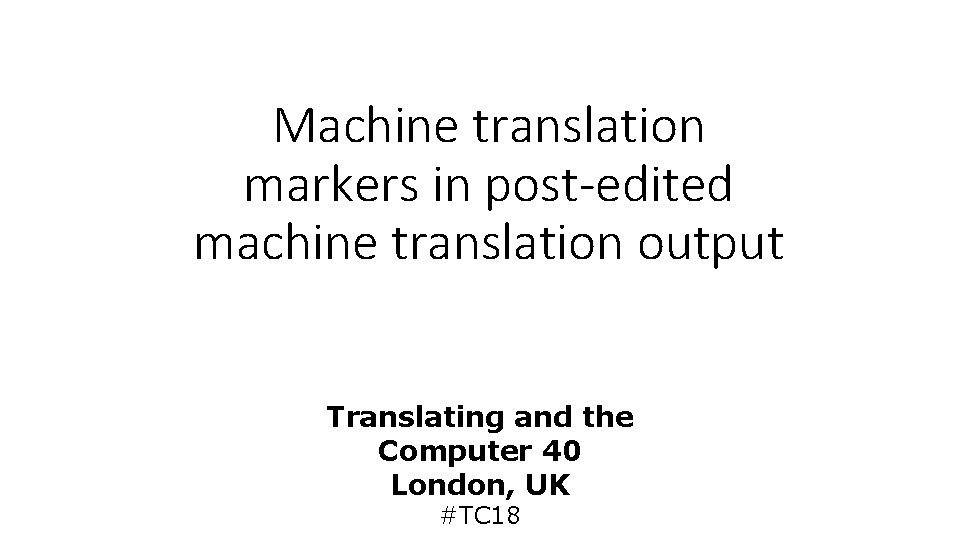
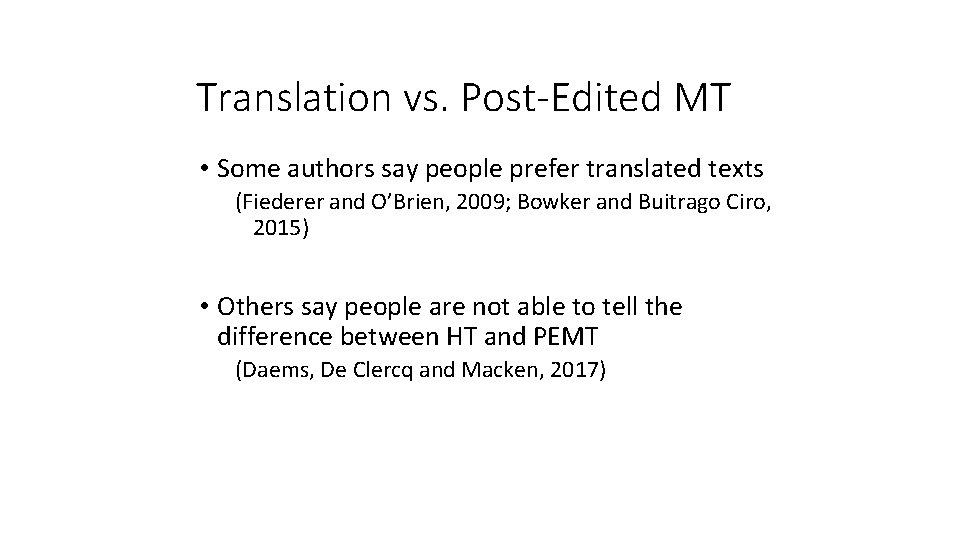
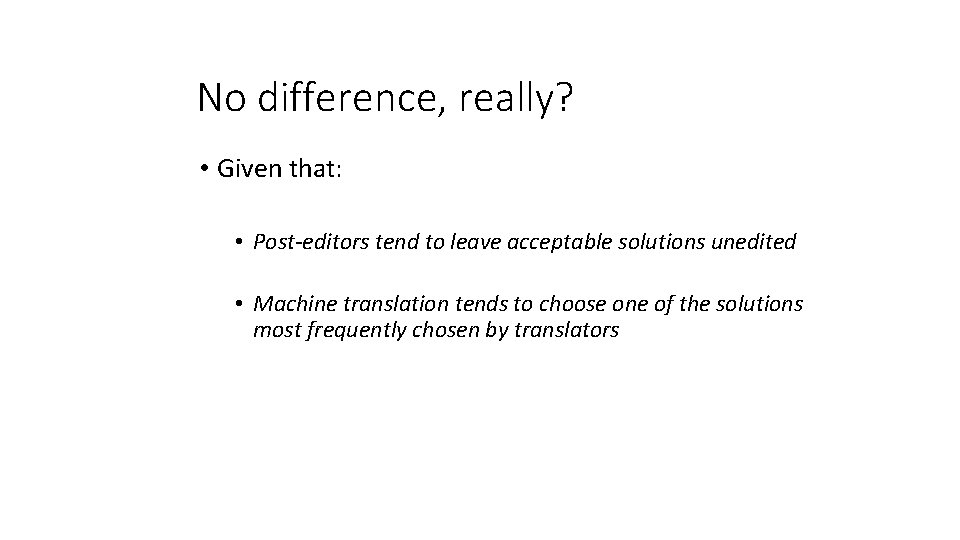
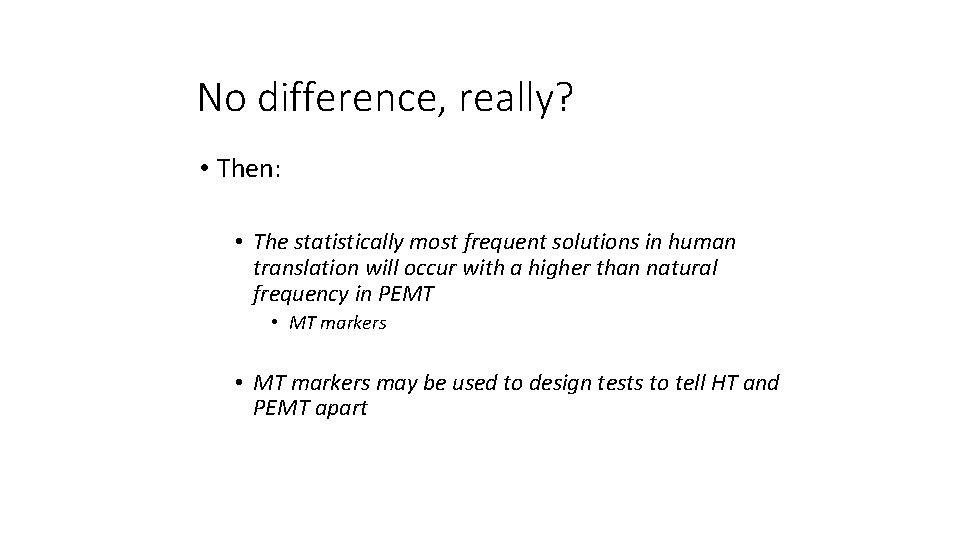
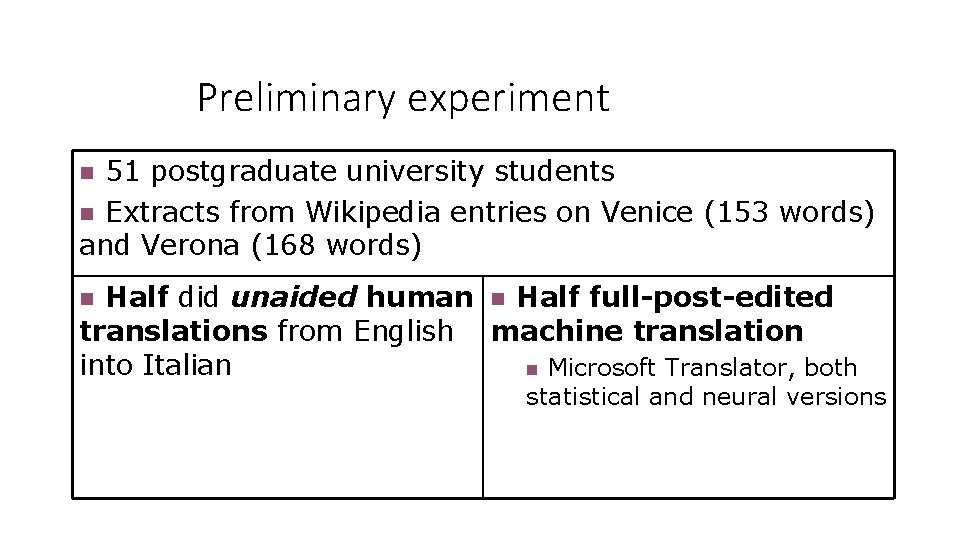
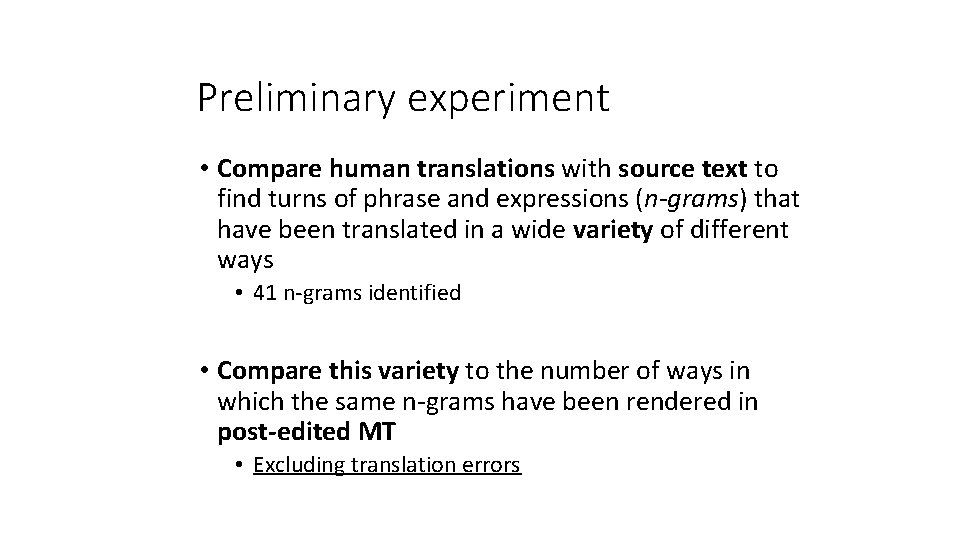
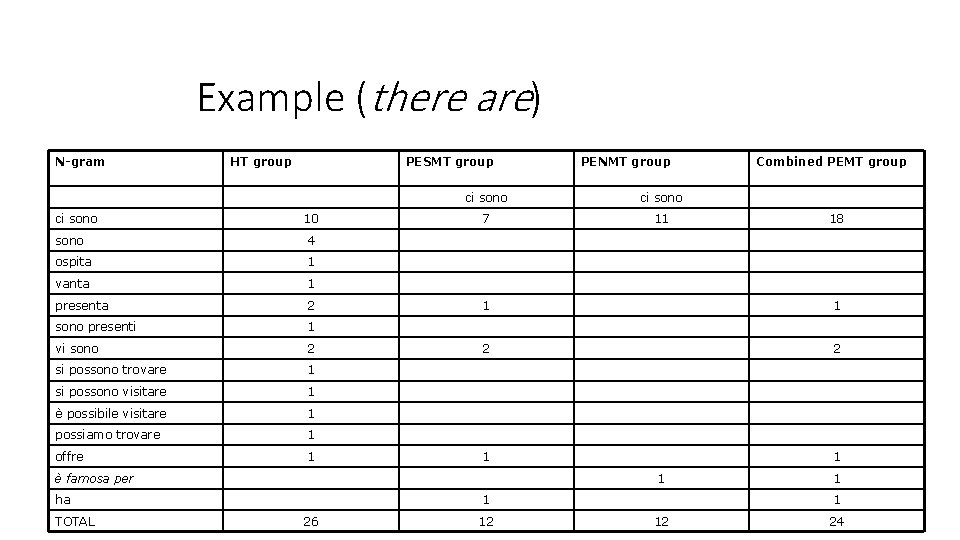
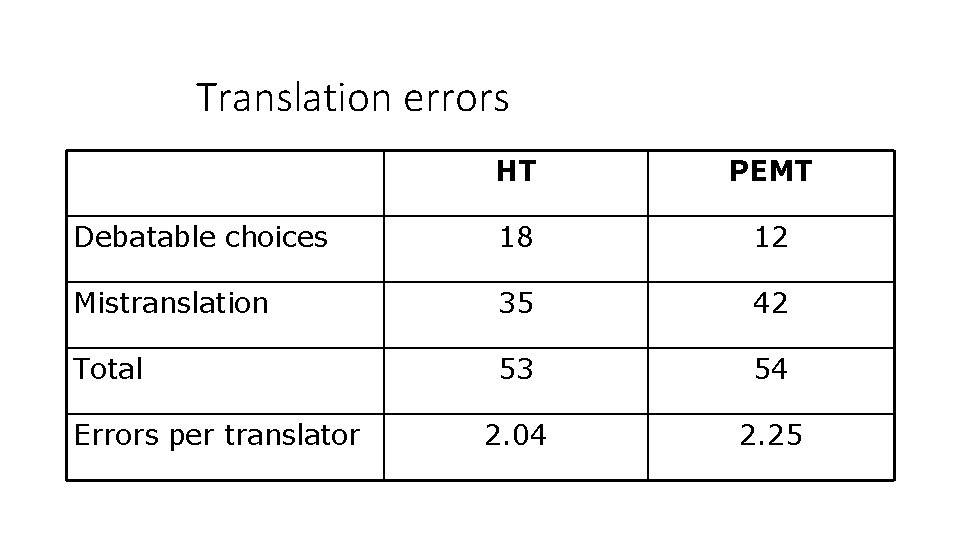
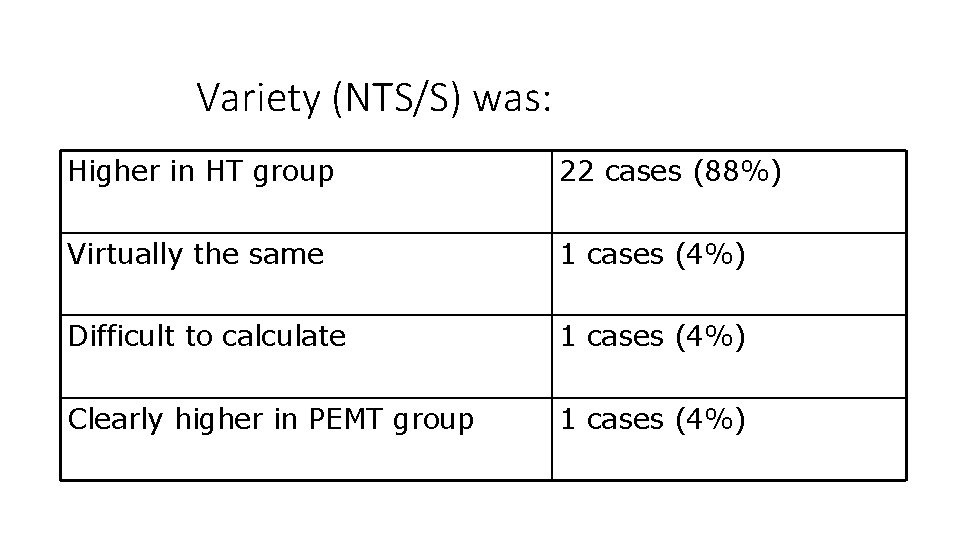
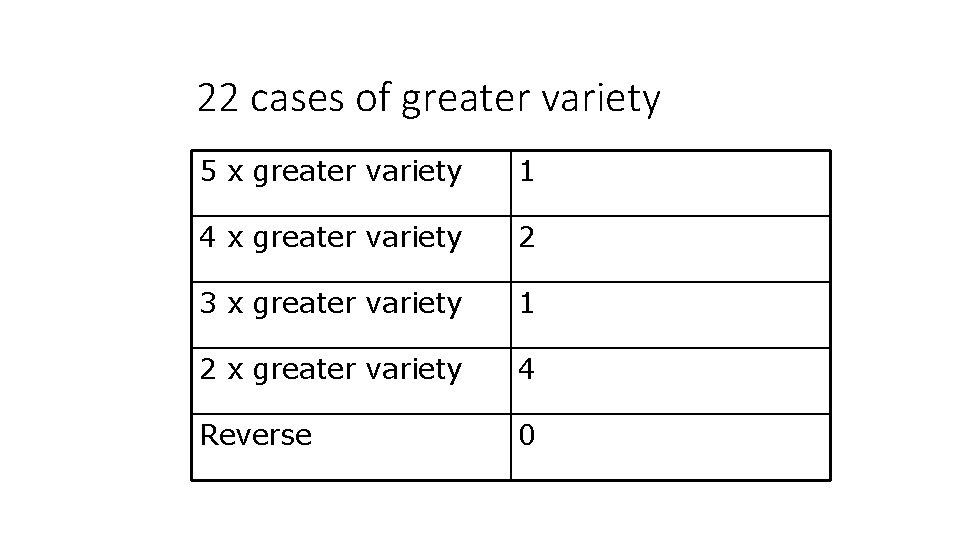
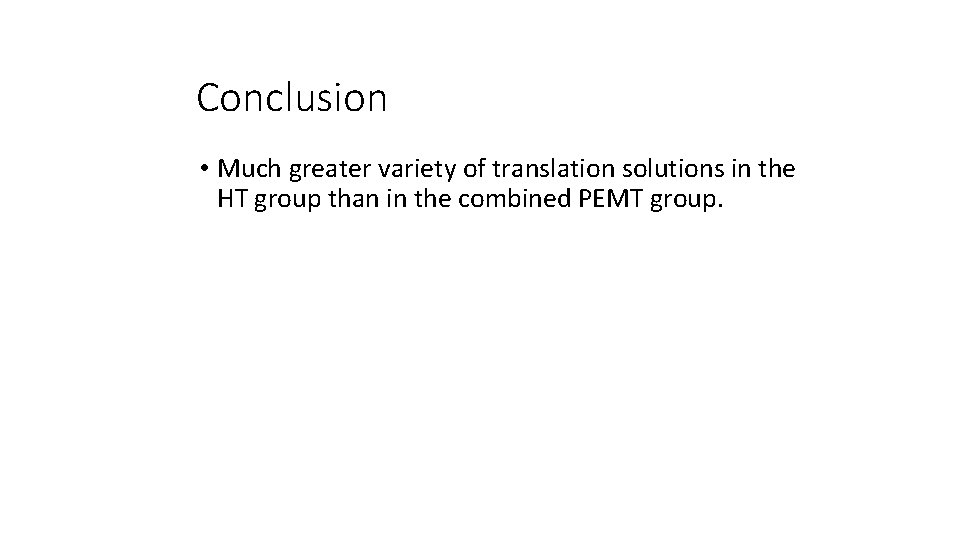
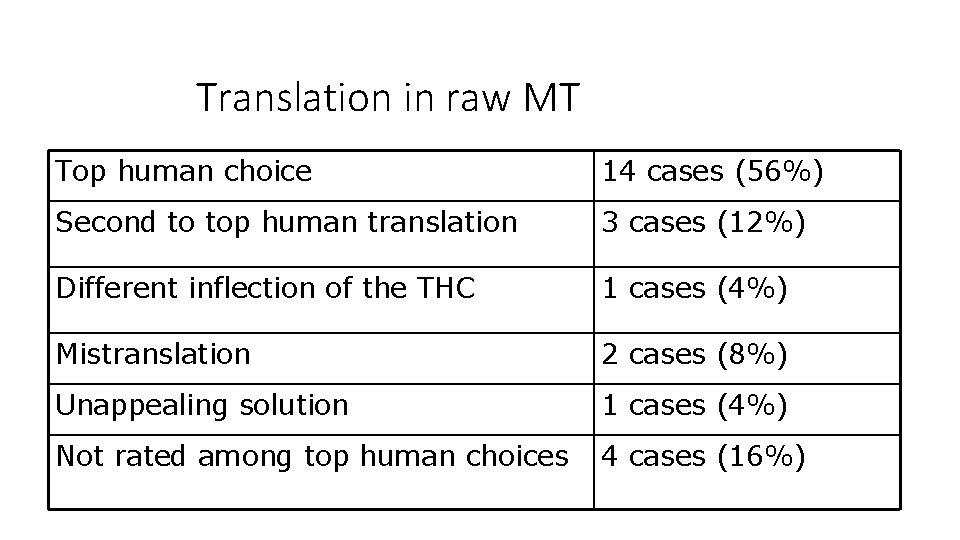
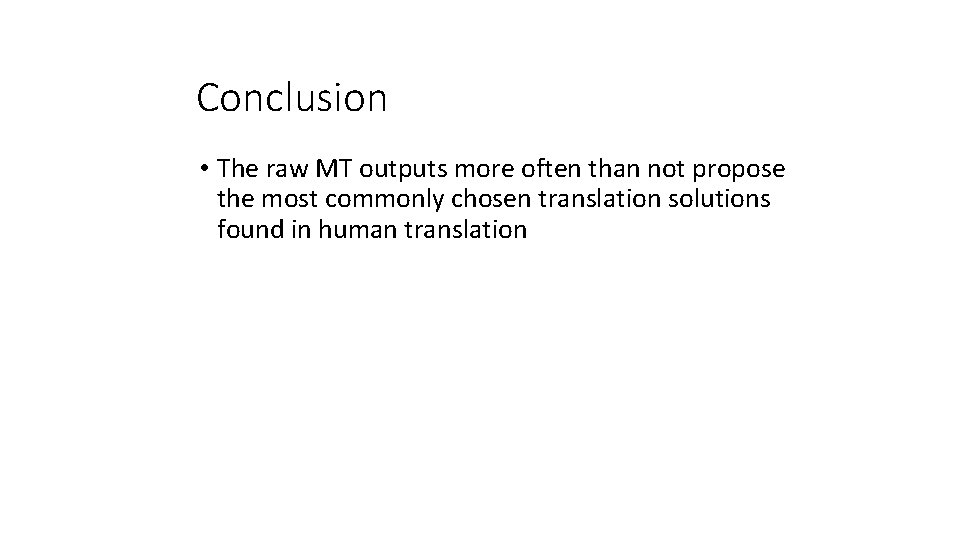
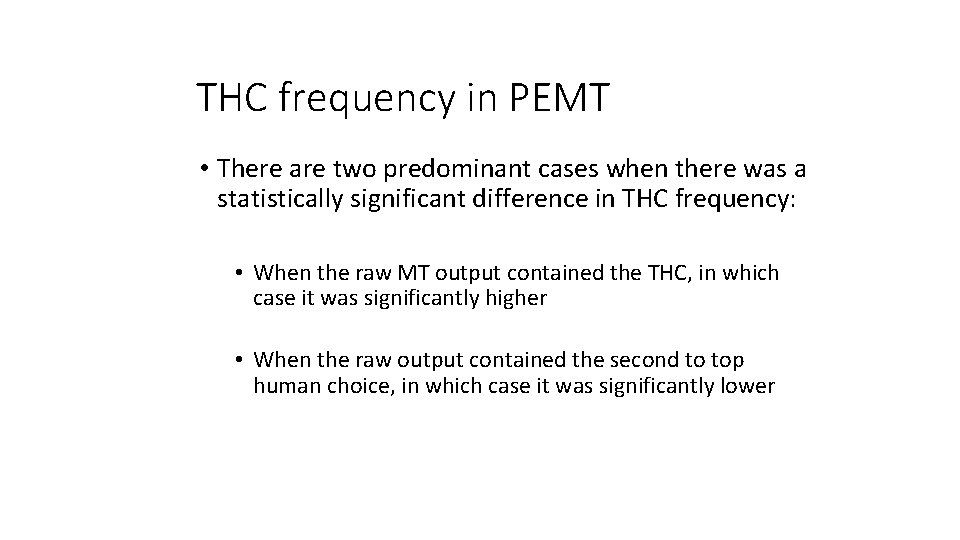
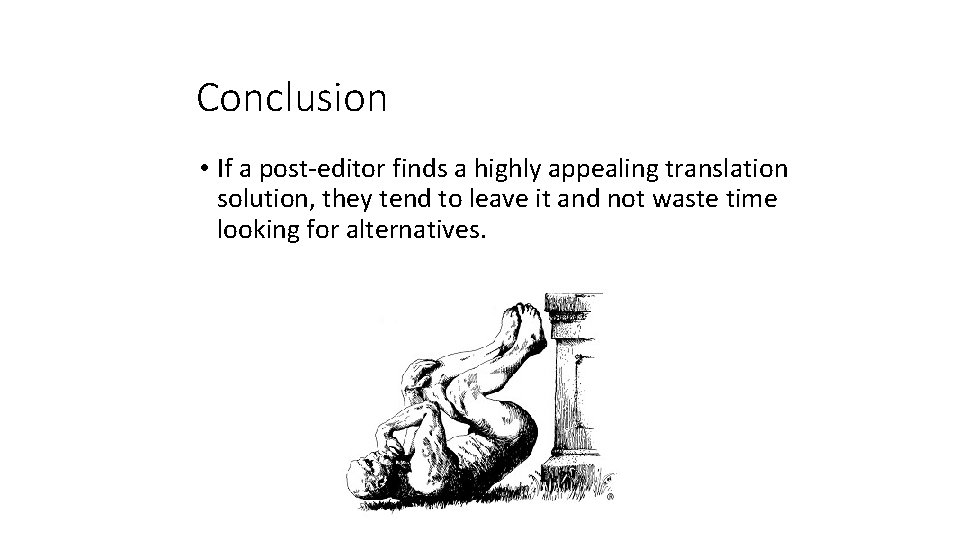
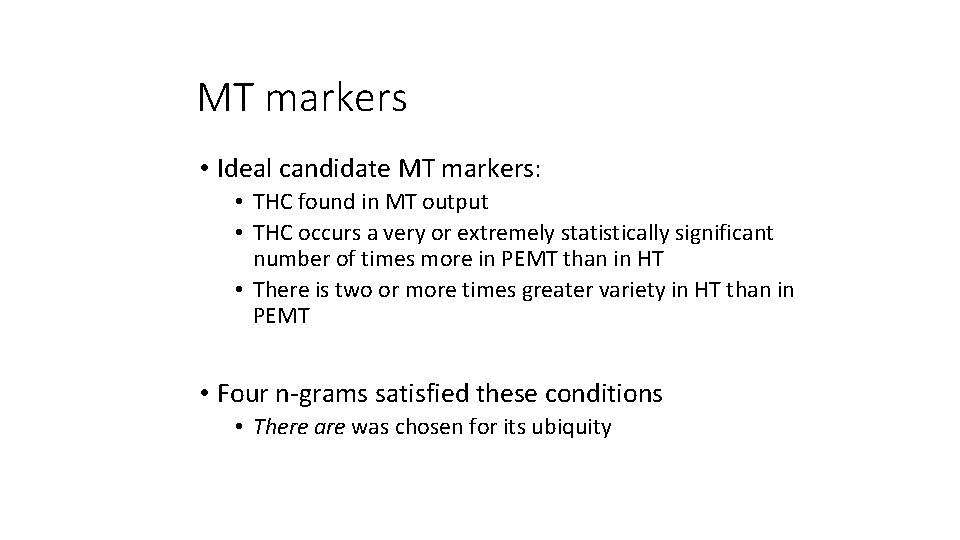
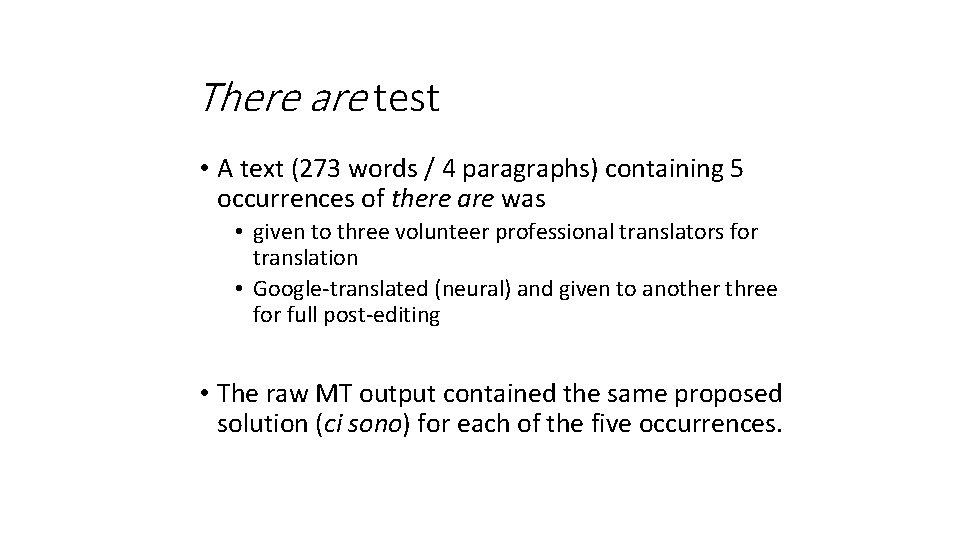
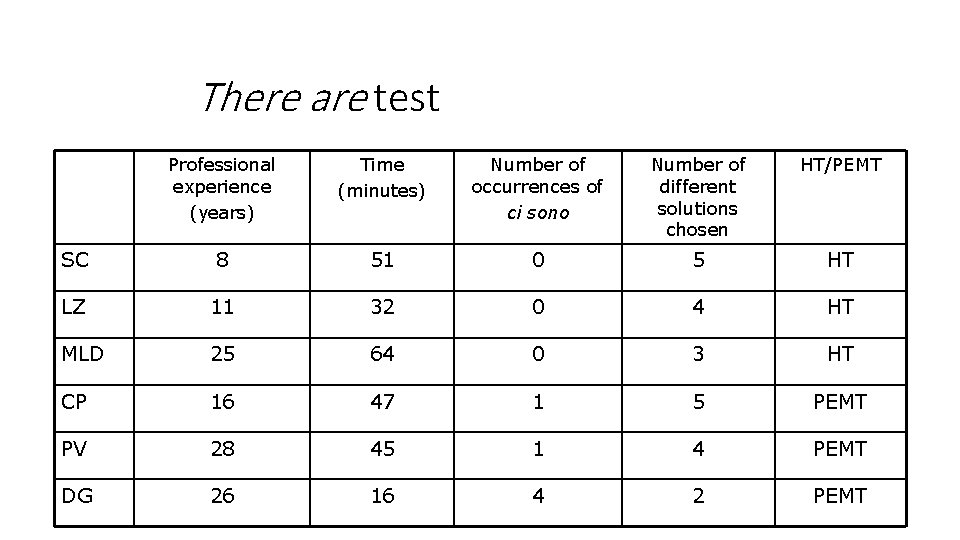
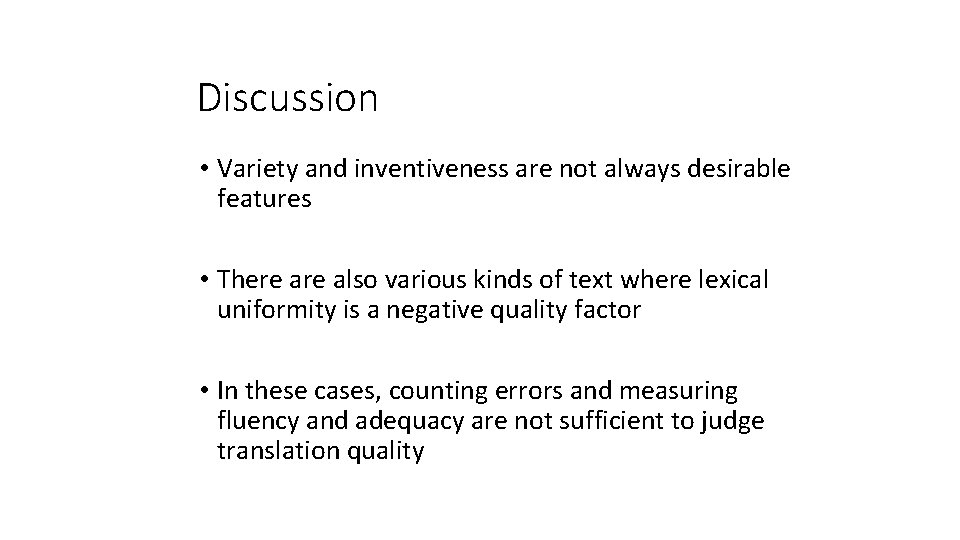
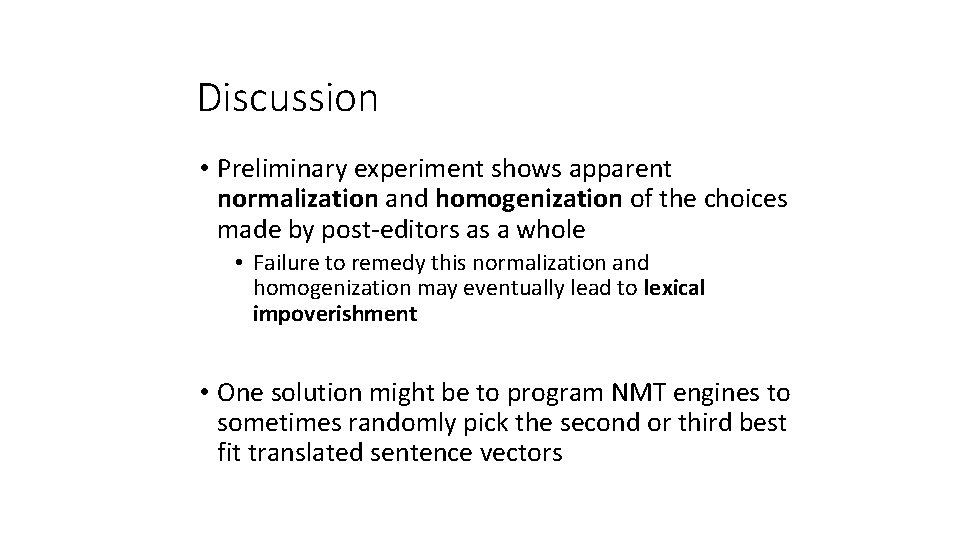
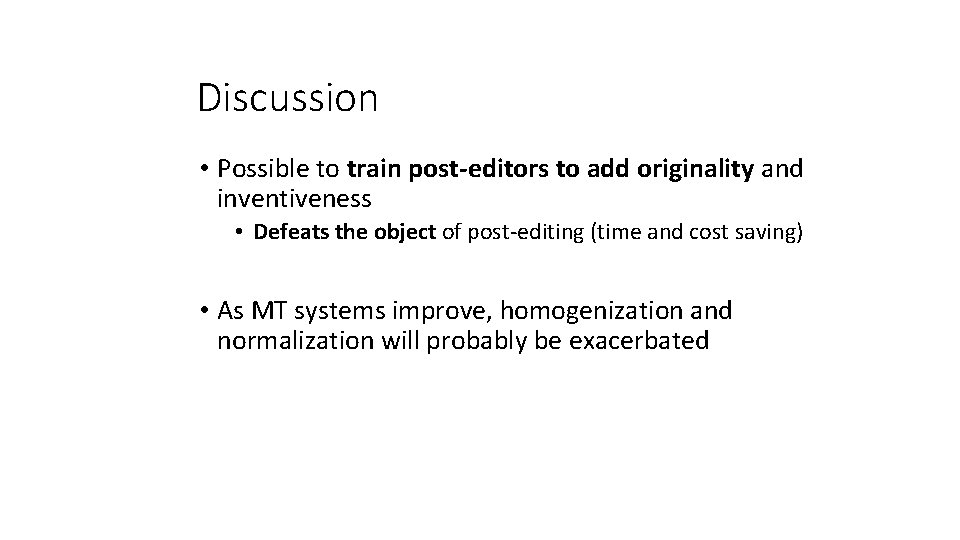
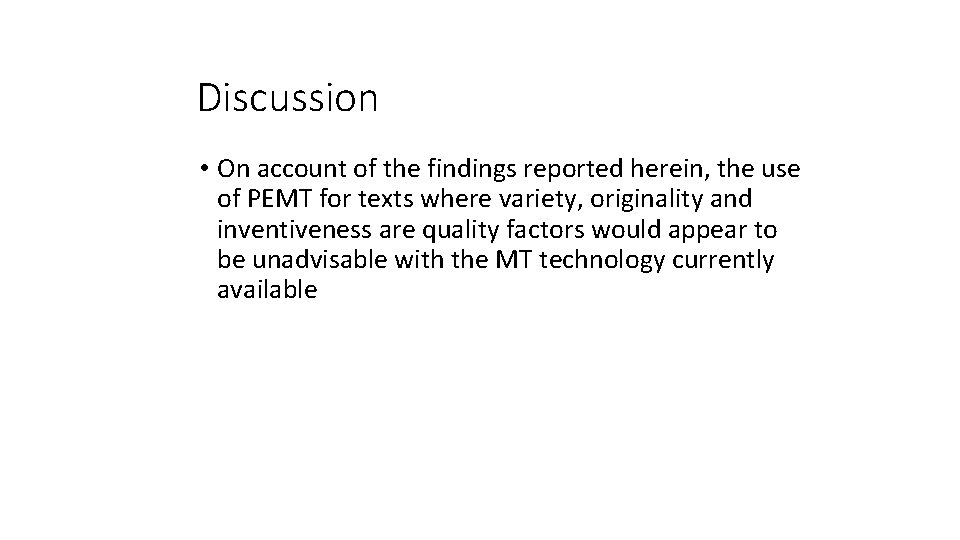
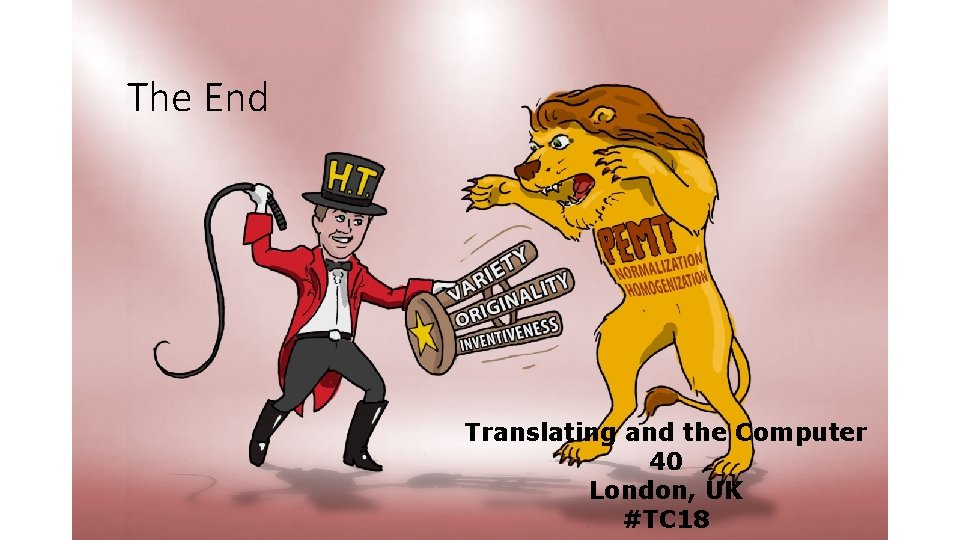
- Slides: 23
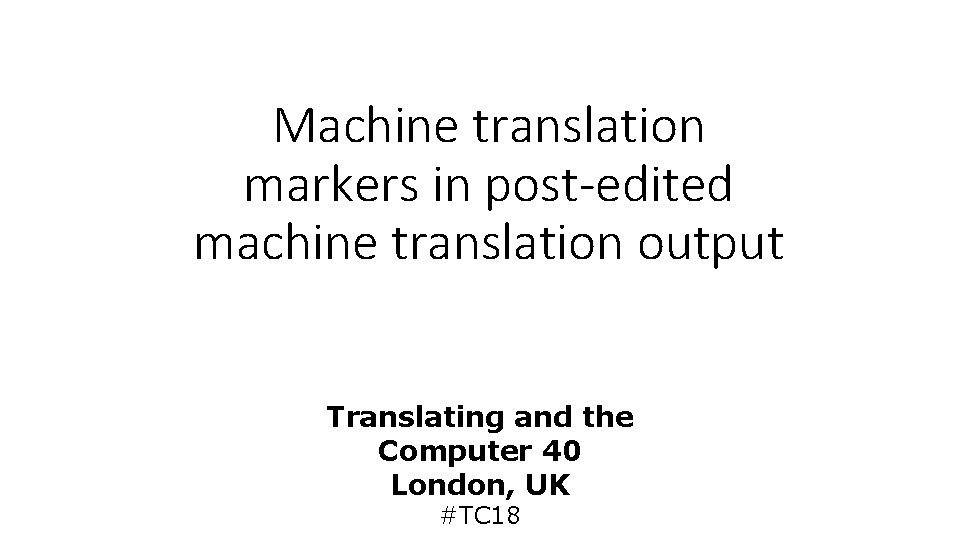
Machine translation markers in post-edited machine translation output Translating and the Computer 40 London, UK #TC 18
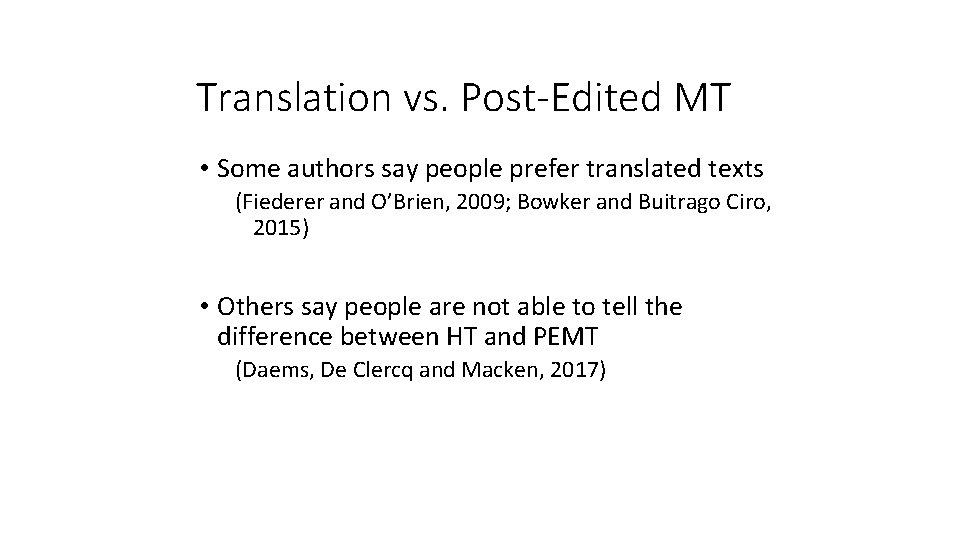
Translation vs. Post-Edited MT • Some authors say people prefer translated texts (Fiederer and O’Brien, 2009; Bowker and Buitrago Ciro, 2015) • Others say people are not able to tell the difference between HT and PEMT (Daems, De Clercq and Macken, 2017)
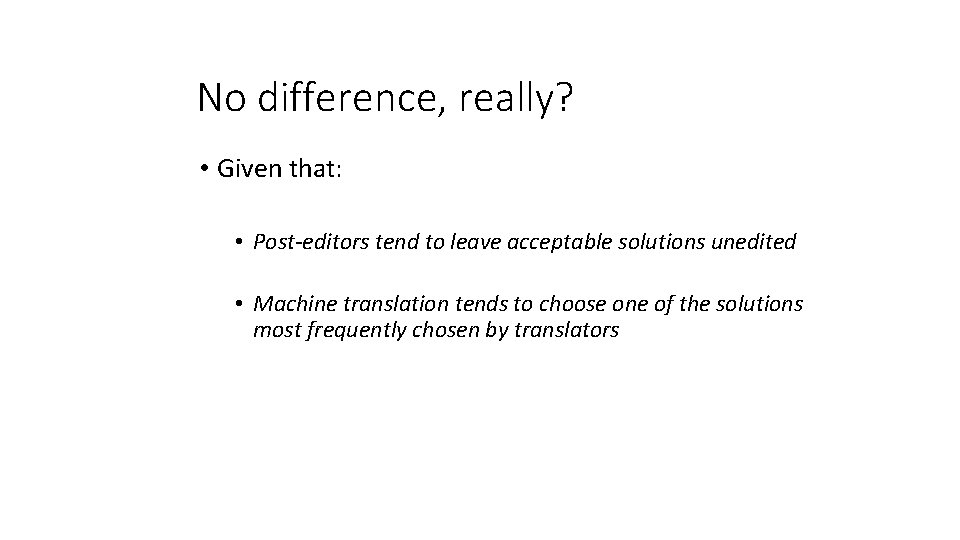
No difference, really? • Given that: • Post-editors tend to leave acceptable solutions unedited • Machine translation tends to choose one of the solutions most frequently chosen by translators
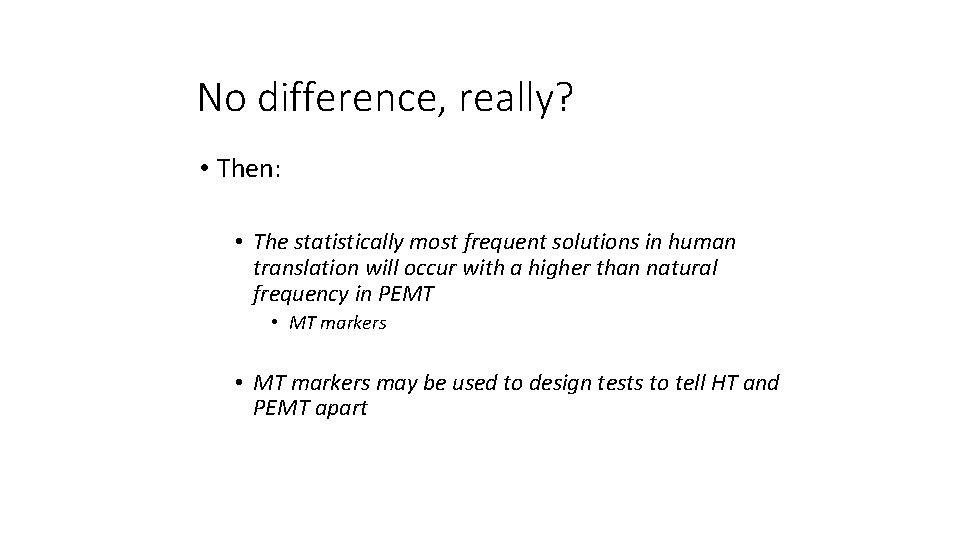
No difference, really? • Then: • The statistically most frequent solutions in human translation will occur with a higher than natural frequency in PEMT • MT markers may be used to design tests to tell HT and PEMT apart
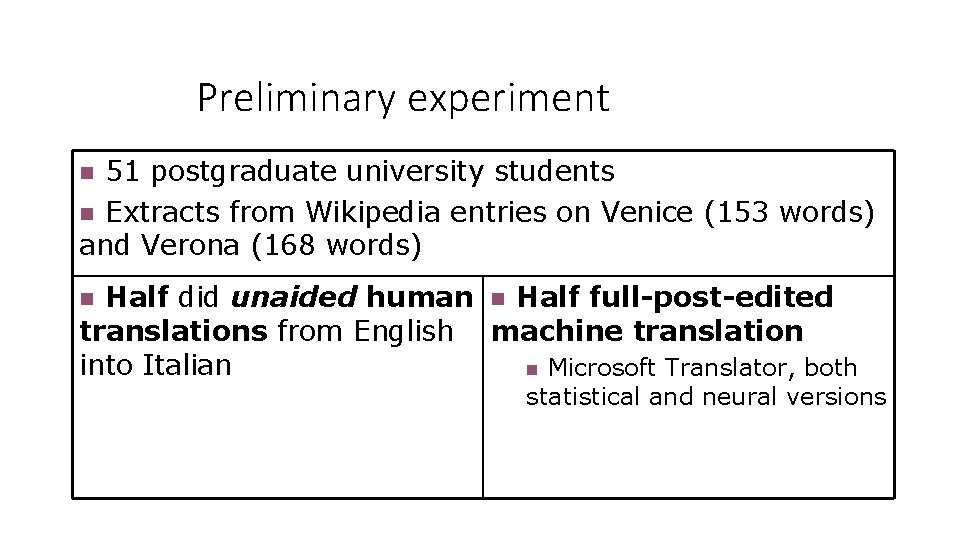
Preliminary experiment 51 postgraduate university students n Extracts from Wikipedia entries on Venice (153 words) and Verona (168 words) n Half did unaided human n Half full-post-edited translations from English machine translation into Italian n Microsoft Translator, both n statistical and neural versions
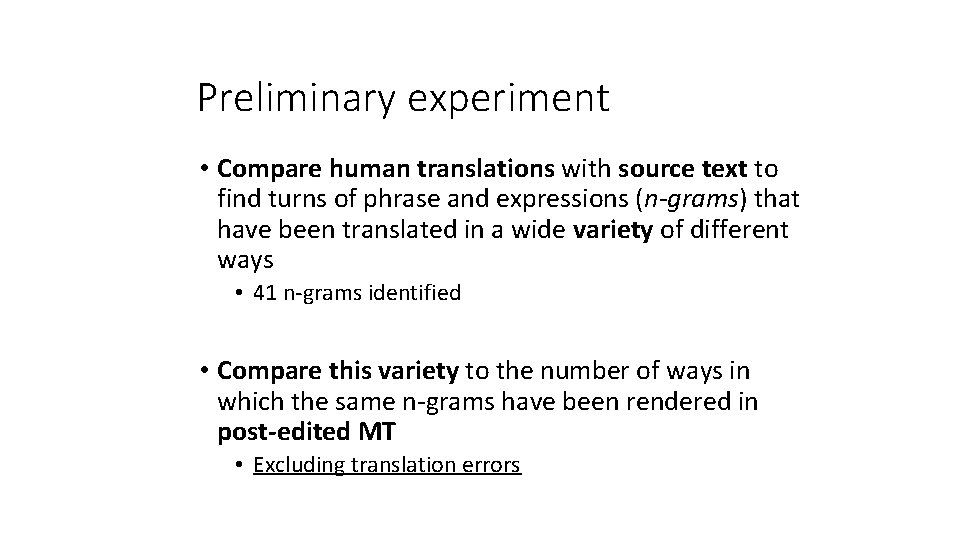
Preliminary experiment • Compare human translations with source text to find turns of phrase and expressions (n-grams) that have been translated in a wide variety of different ways • 41 n-grams identified • Compare this variety to the number of ways in which the same n-grams have been rendered in post-edited MT • Excluding translation errors
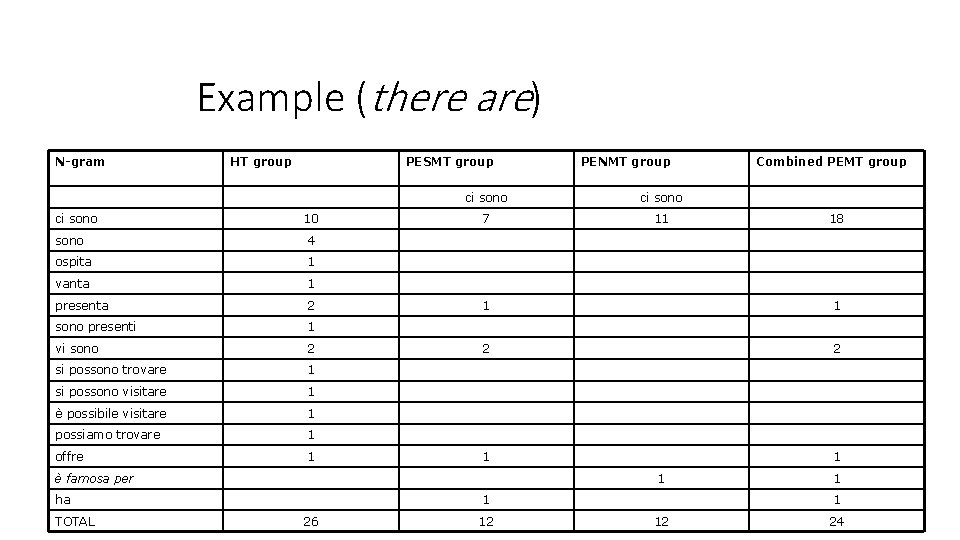
Example (there are) N-gram ci sono HT group PESMT group 10 sono 4 ospita 1 vanta 1 presenta 2 sono presenti 1 vi sono 2 si possono trovare 1 si possono visitare 1 è possibile visitare 1 possiamo trovare 1 offre 1 ci sono 7 11 2 2 1 1 1 26 18 1 1 ha Combined PEMT group 1 è famosa per TOTAL PENMT group 12 1 1 12 24
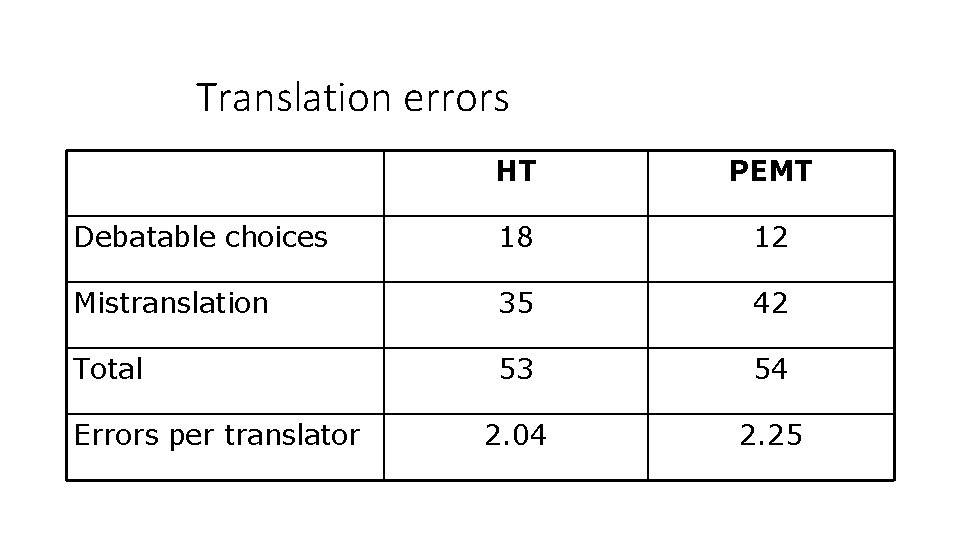
Translation errors HT PEMT Debatable choices 18 12 Mistranslation 35 42 Total 53 54 2. 04 2. 25 Errors per translator
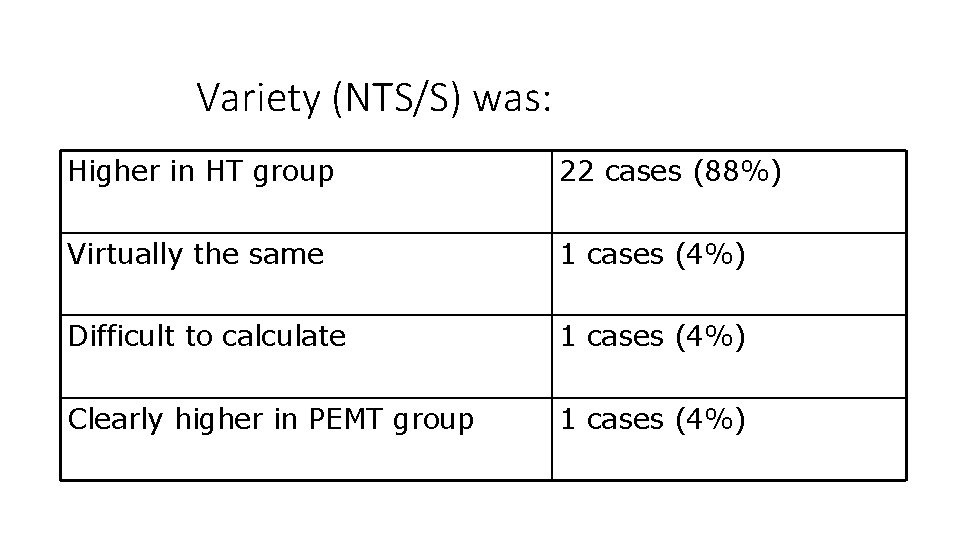
Variety (NTS/S) was: Higher in HT group 22 cases (88%) Virtually the same 1 cases (4%) Difficult to calculate 1 cases (4%) Clearly higher in PEMT group 1 cases (4%)
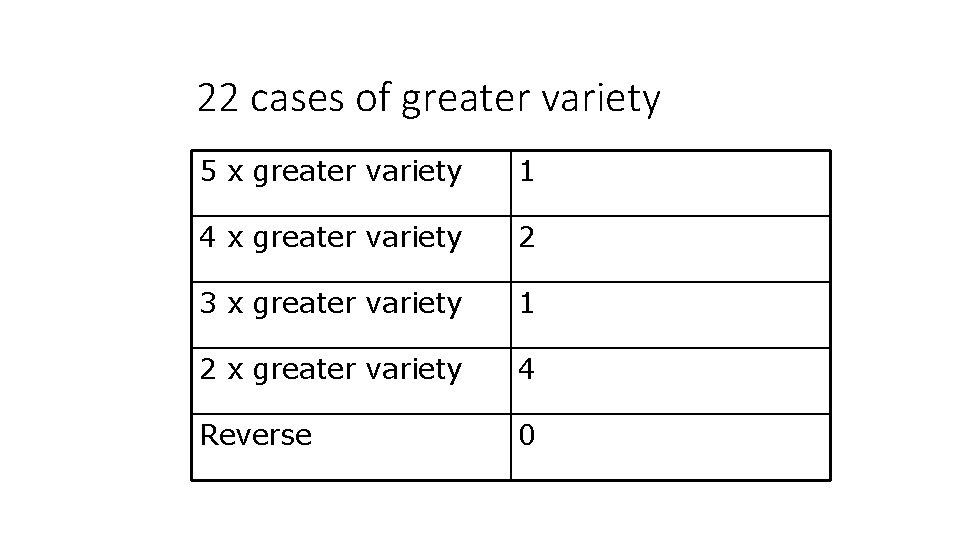
22 cases of greater variety 5 x greater variety 1 4 x greater variety 2 3 x greater variety 1 2 x greater variety 4 Reverse 0
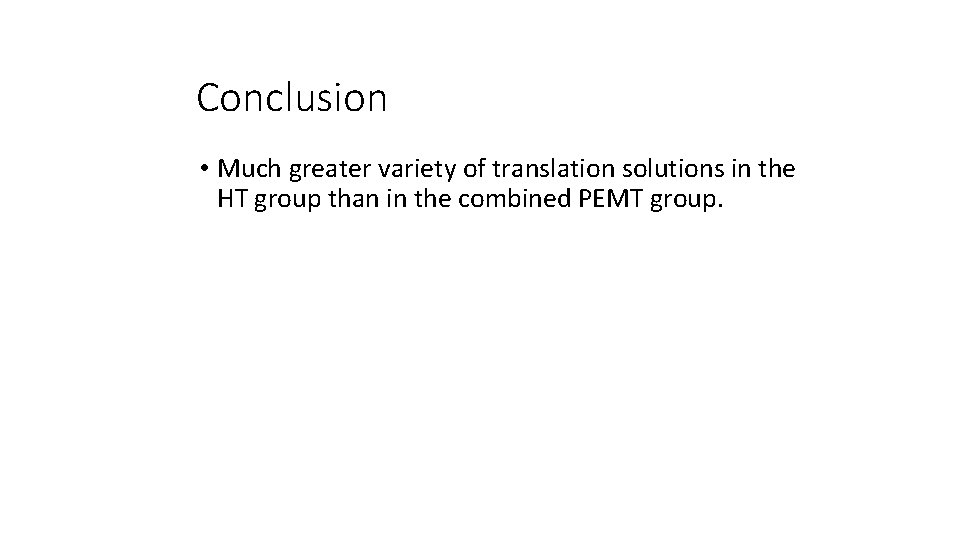
Conclusion • Much greater variety of translation solutions in the HT group than in the combined PEMT group.
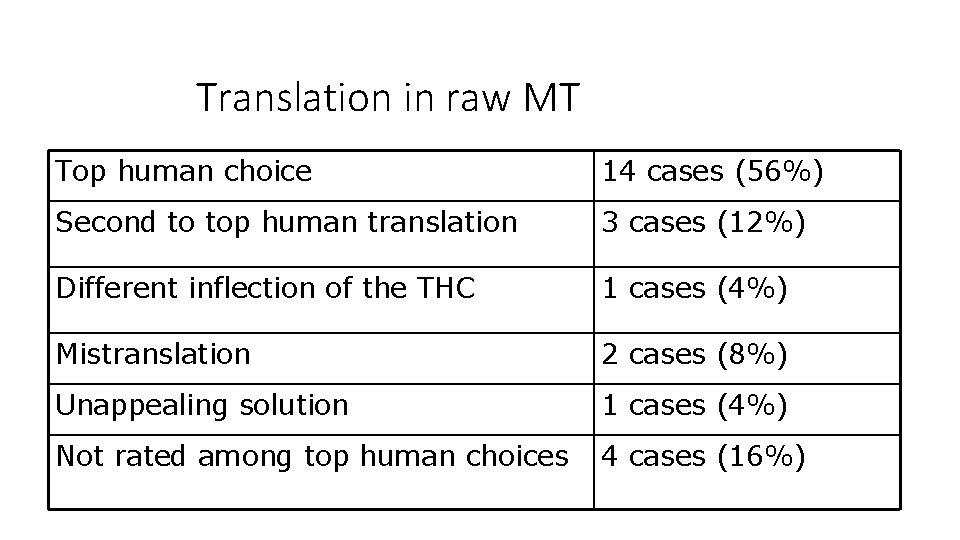
Translation in raw MT Top human choice 14 cases (56%) Second to top human translation 3 cases (12%) Different inflection of the THC 1 cases (4%) Mistranslation 2 cases (8%) Unappealing solution 1 cases (4%) Not rated among top human choices 4 cases (16%)
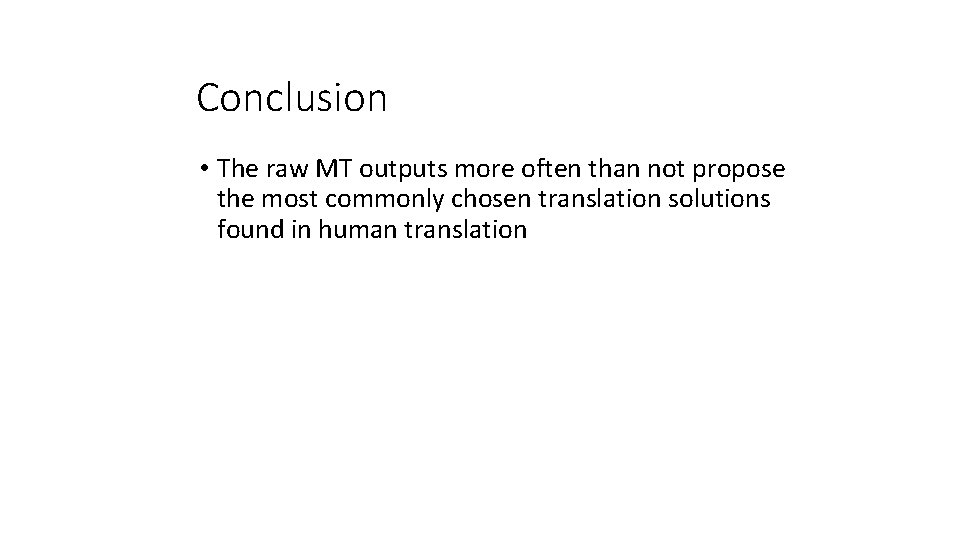
Conclusion • The raw MT outputs more often than not propose the most commonly chosen translation solutions found in human translation
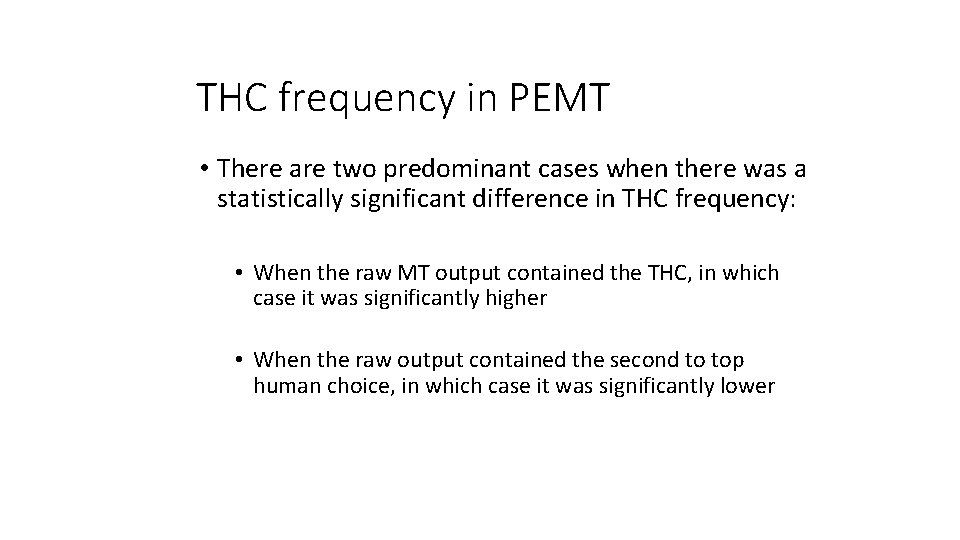
THC frequency in PEMT • There are two predominant cases when there was a statistically significant difference in THC frequency: • When the raw MT output contained the THC, in which case it was significantly higher • When the raw output contained the second to top human choice, in which case it was significantly lower
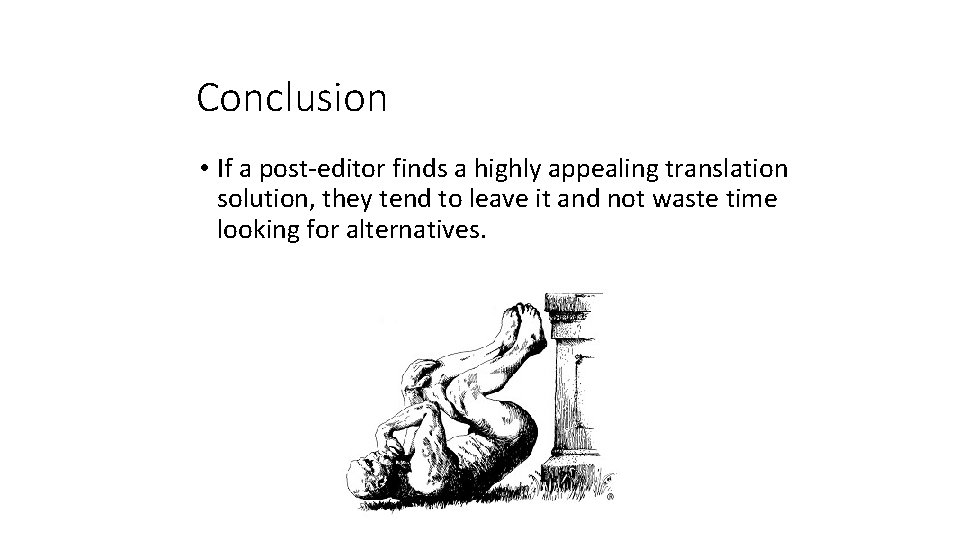
Conclusion • If a post-editor finds a highly appealing translation solution, they tend to leave it and not waste time looking for alternatives.
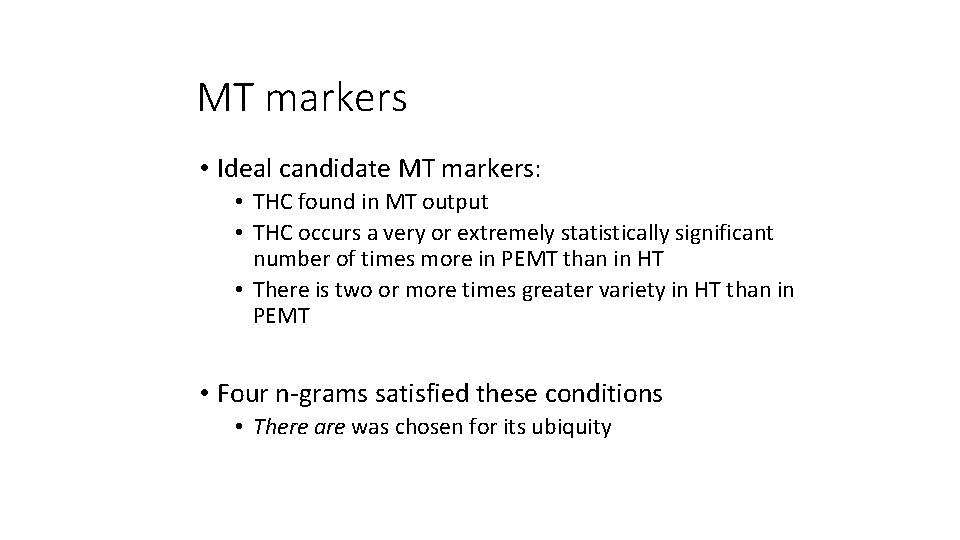
MT markers • Ideal candidate MT markers: • THC found in MT output • THC occurs a very or extremely statistically significant number of times more in PEMT than in HT • There is two or more times greater variety in HT than in PEMT • Four n-grams satisfied these conditions • There are was chosen for its ubiquity
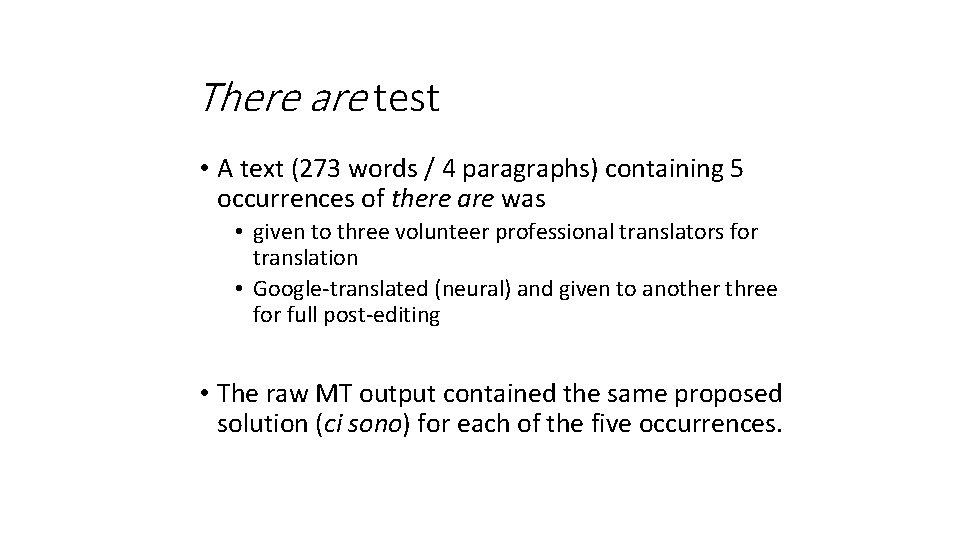
There are test • A text (273 words / 4 paragraphs) containing 5 occurrences of there are was • given to three volunteer professional translators for translation • Google-translated (neural) and given to another three for full post-editing • The raw MT output contained the same proposed solution (ci sono) for each of the five occurrences.
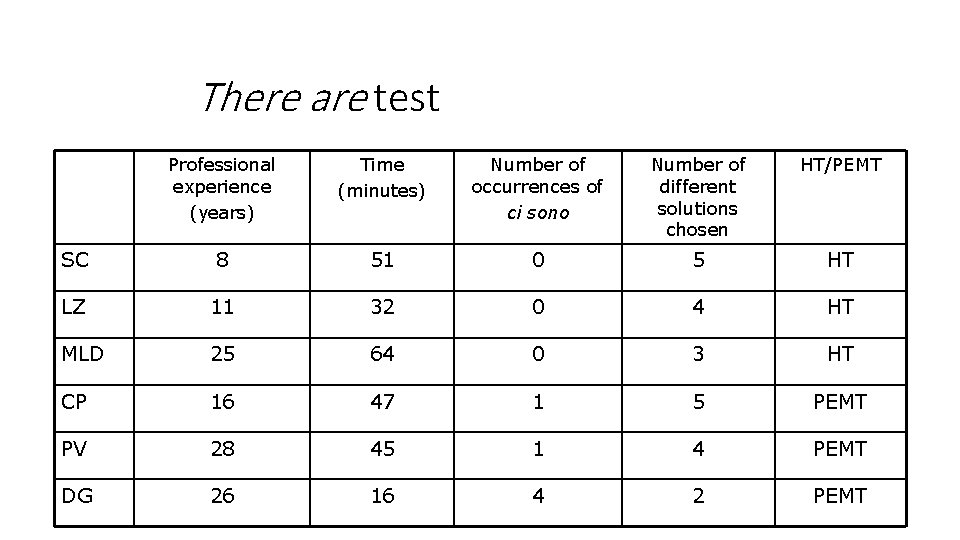
There are test Professional experience (years) Time (minutes) Number of occurrences of ci sono Number of different solutions chosen HT/PEMT SC 8 51 0 5 HT LZ 11 32 0 4 HT MLD 25 64 0 3 HT CP 16 47 1 5 PEMT PV 28 45 1 4 PEMT DG 26 16 4 2 PEMT
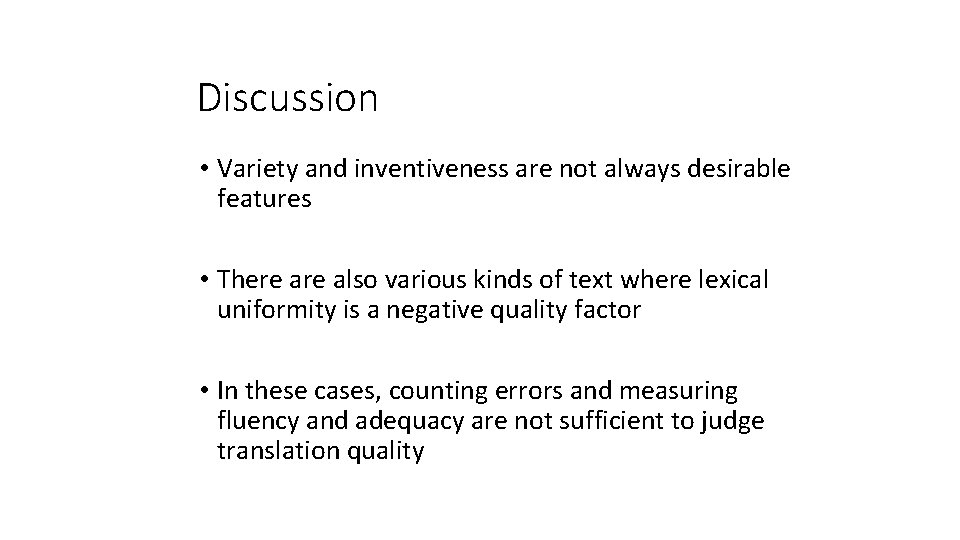
Discussion • Variety and inventiveness are not always desirable features • There also various kinds of text where lexical uniformity is a negative quality factor • In these cases, counting errors and measuring fluency and adequacy are not sufficient to judge translation quality
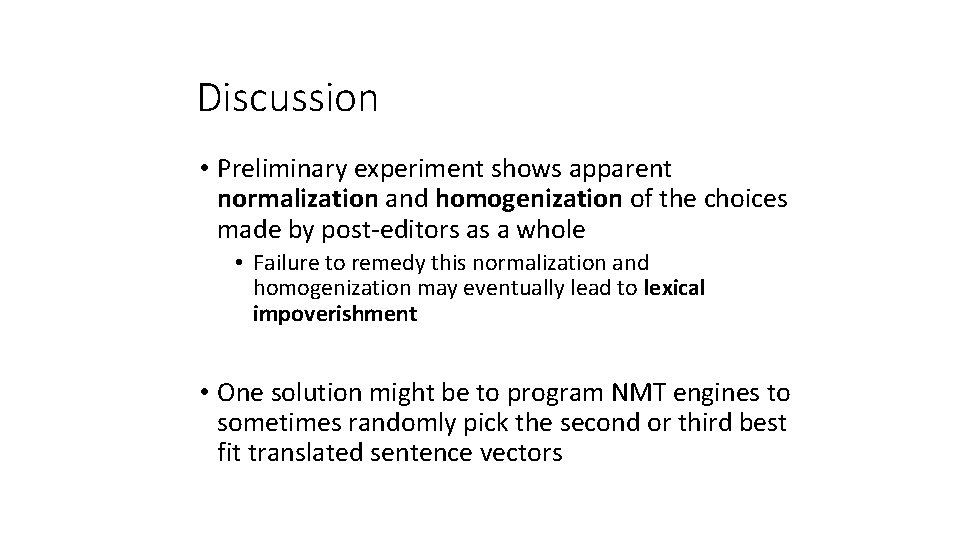
Discussion • Preliminary experiment shows apparent normalization and homogenization of the choices made by post-editors as a whole • Failure to remedy this normalization and homogenization may eventually lead to lexical impoverishment • One solution might be to program NMT engines to sometimes randomly pick the second or third best fit translated sentence vectors
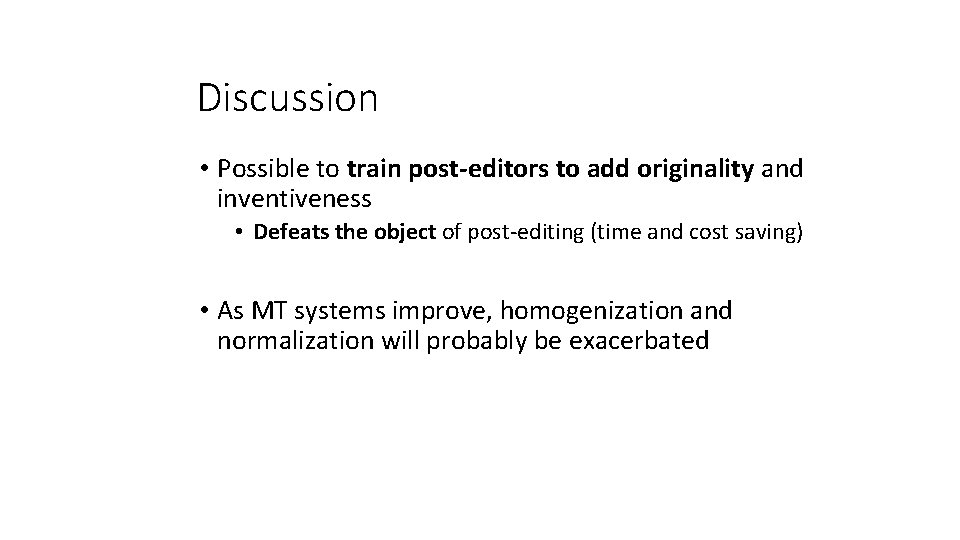
Discussion • Possible to train post-editors to add originality and inventiveness • Defeats the object of post-editing (time and cost saving) • As MT systems improve, homogenization and normalization will probably be exacerbated
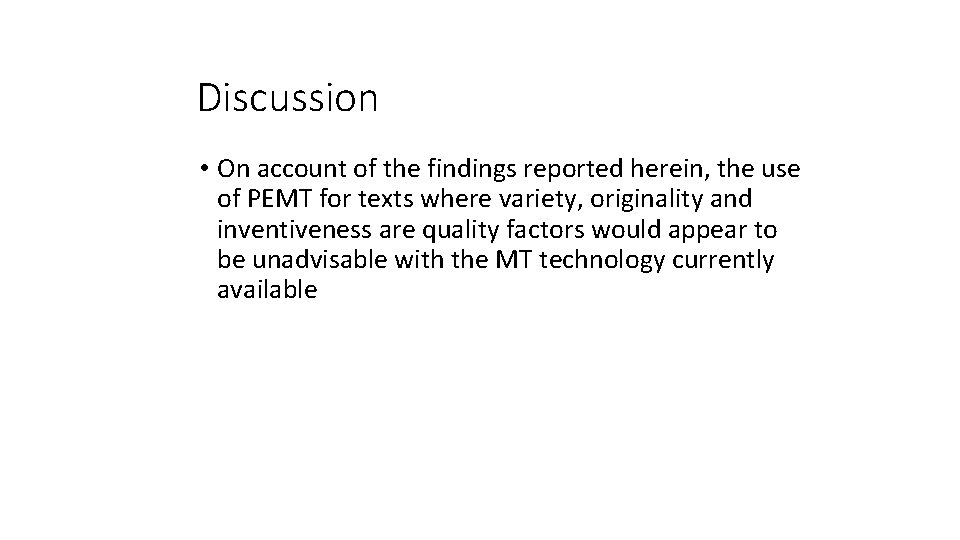
Discussion • On account of the findings reported herein, the use of PEMT for texts where variety, originality and inventiveness are quality factors would appear to be unadvisable with the MT technology currently available
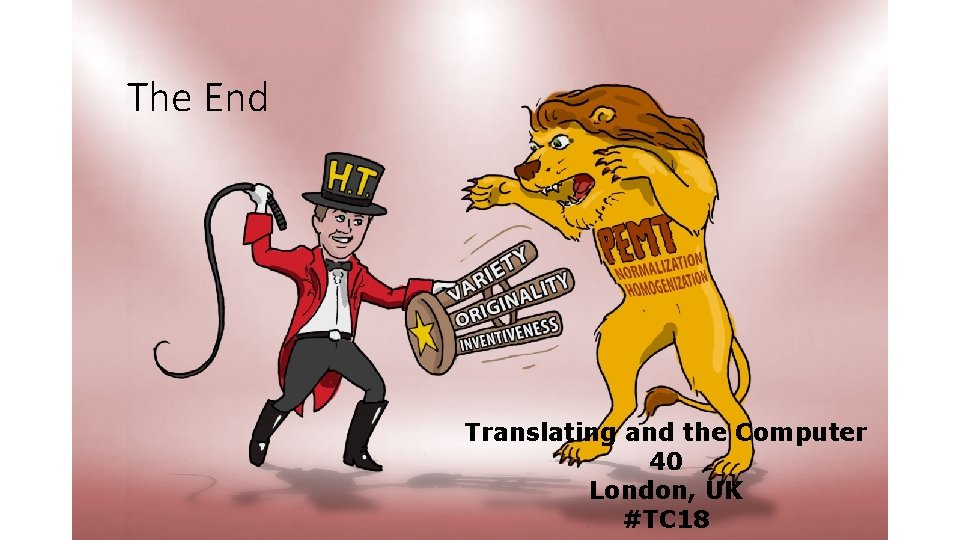
The End Translating and the Computer 40 London, UK #TC 18
Written and spoken discourse examples
Fudicial markers
Dependent claus
Rflp vs rapd
Penina wants to insert markers
Achrolic
Genetic markers
Stem cell markers slideshare
Novice audience
Past continuous
Stansw meet the markers
Nlp semantic analysis
Stance markers
What is a subordinate sentence
Fair division
Rf4 inheritance
Tense markers
Koilocyt
Discourse markers list
Visual marker in biotechnology
Voice markers they say i say
A whale bone that originally contained 200 grams
Non manual markers wh question
Pku diet