Linear Hierarchical Models Corinne Iola Giorgia Silani SPM
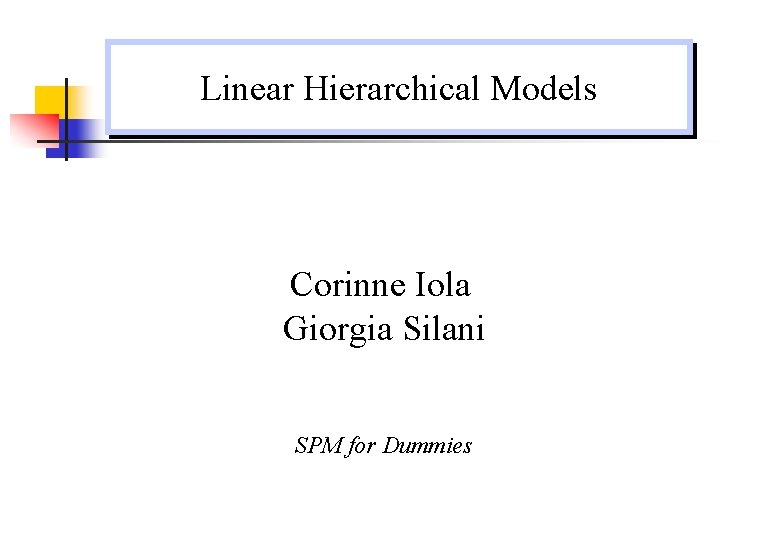
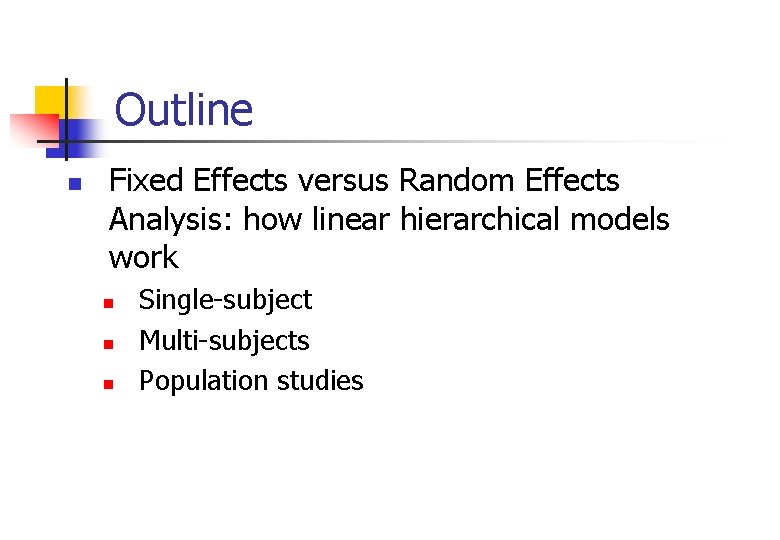
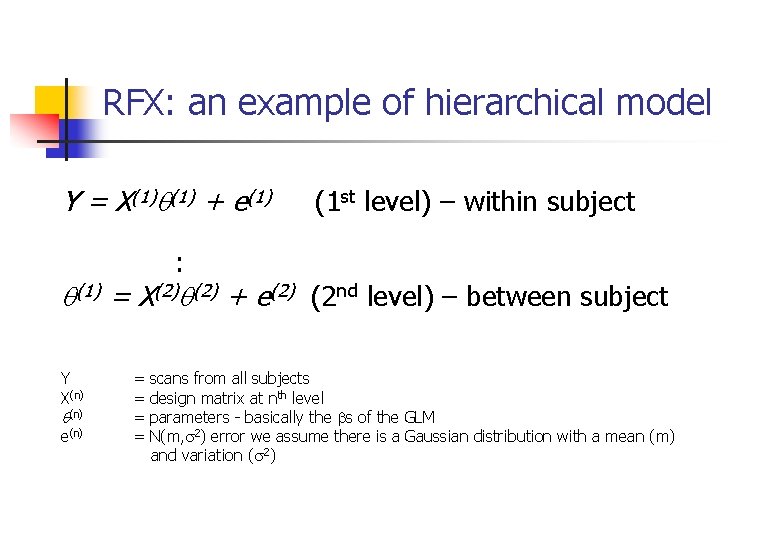
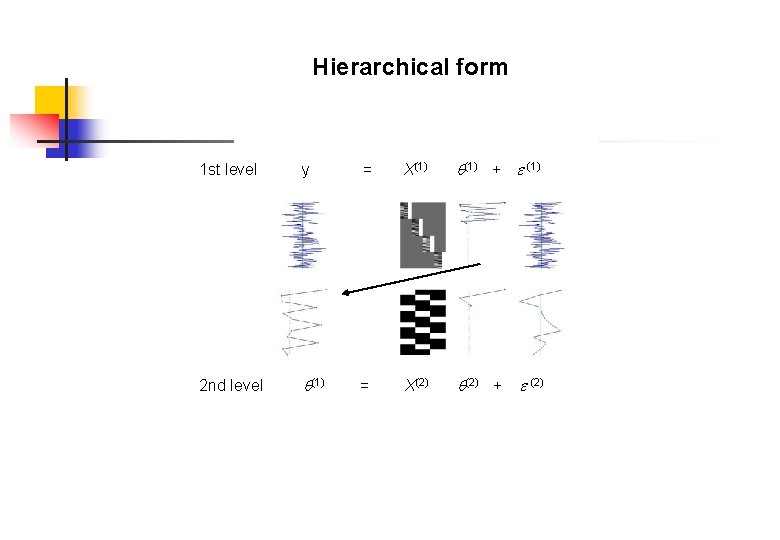
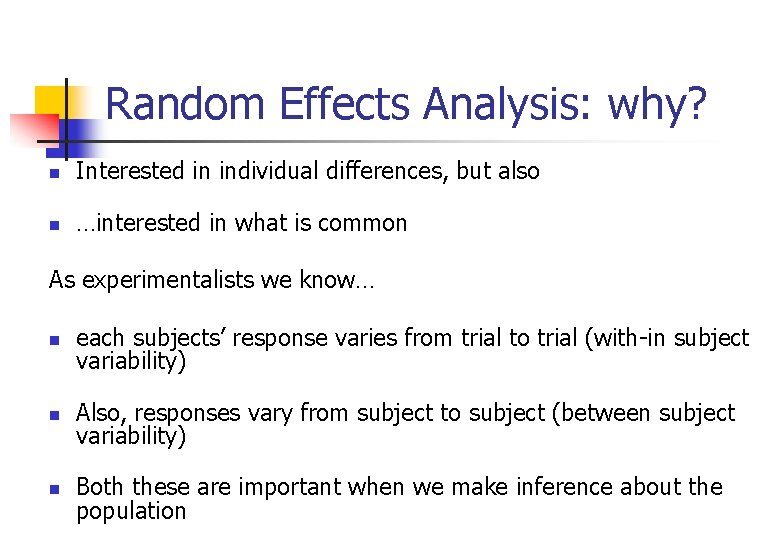
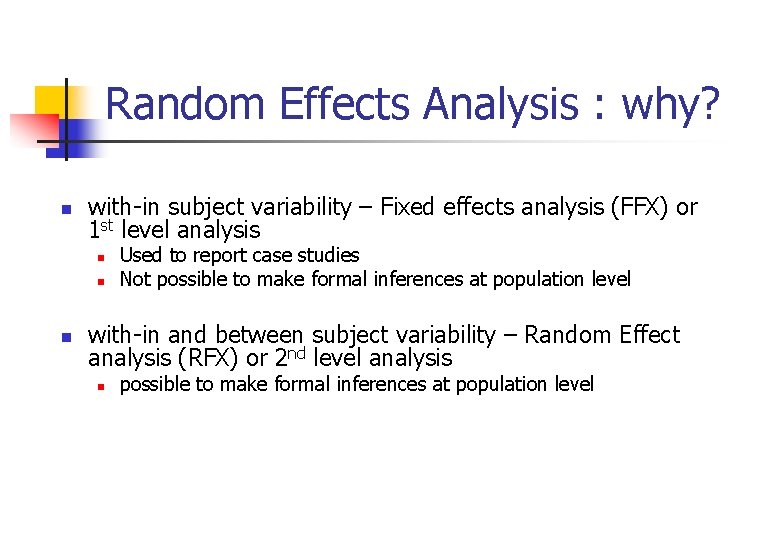
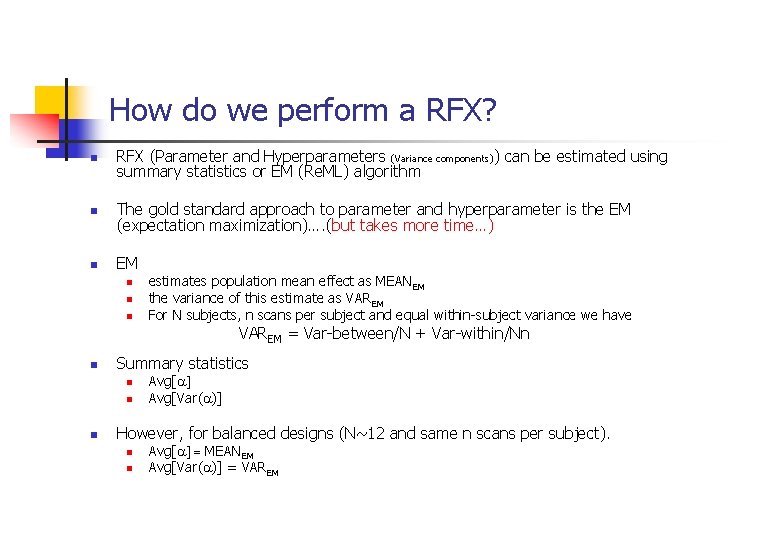
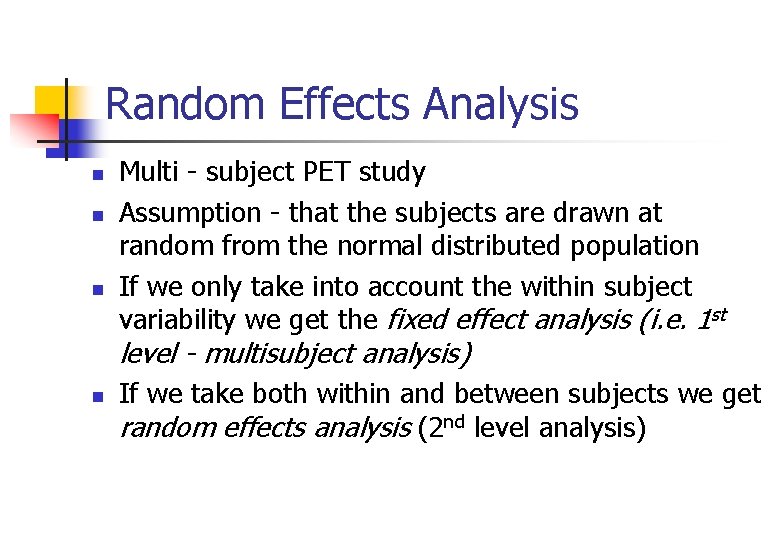
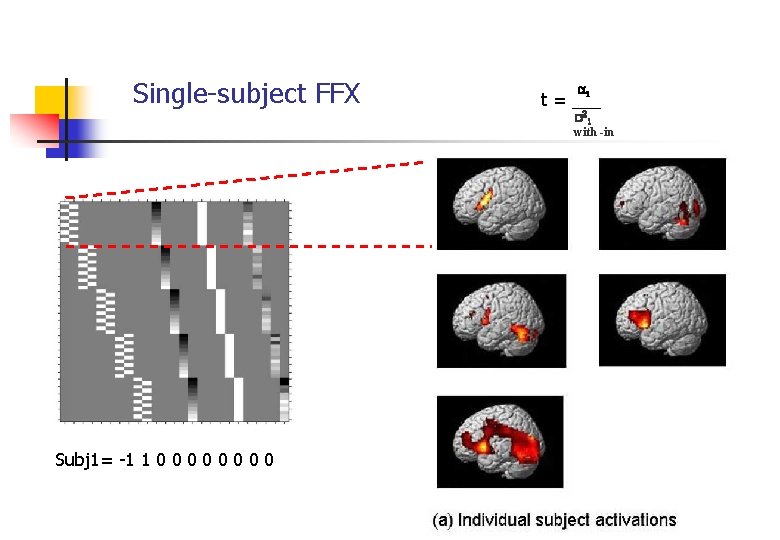
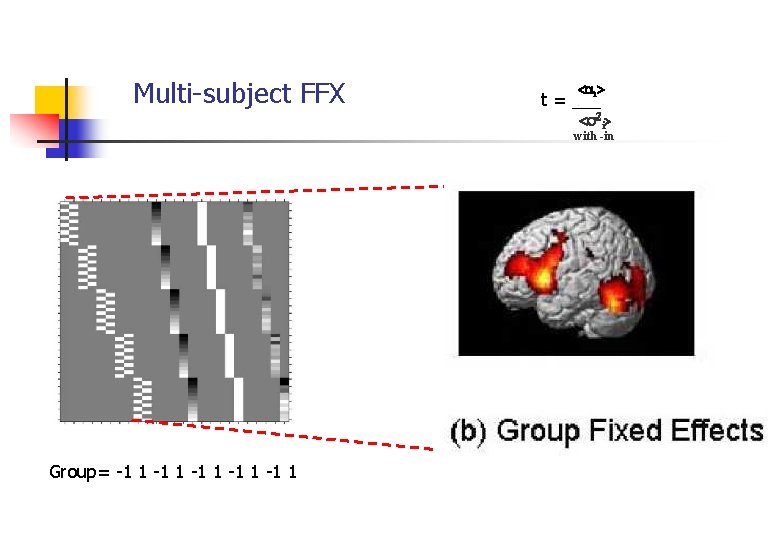
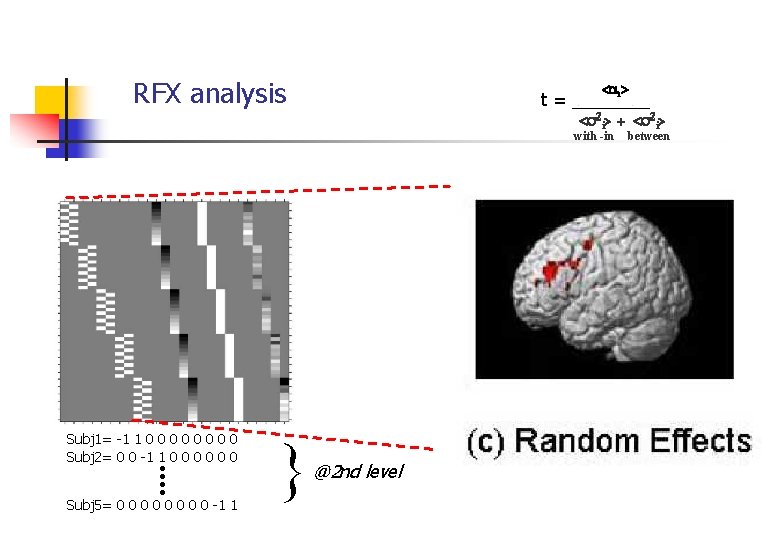
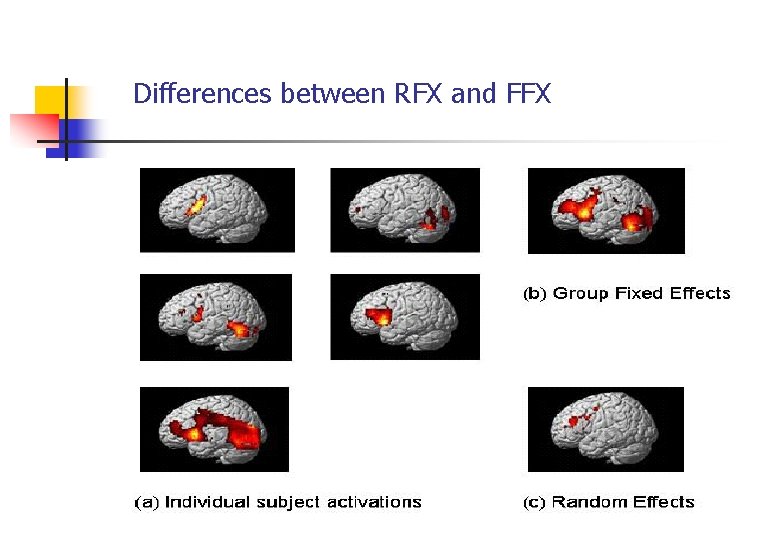
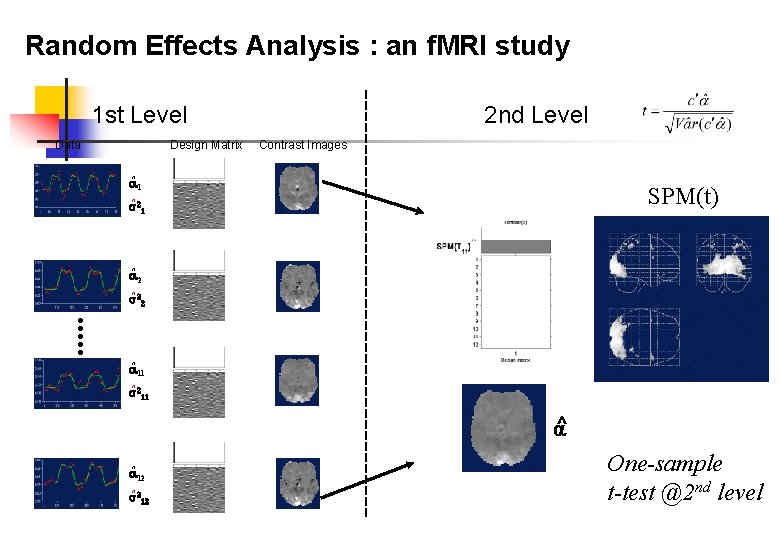
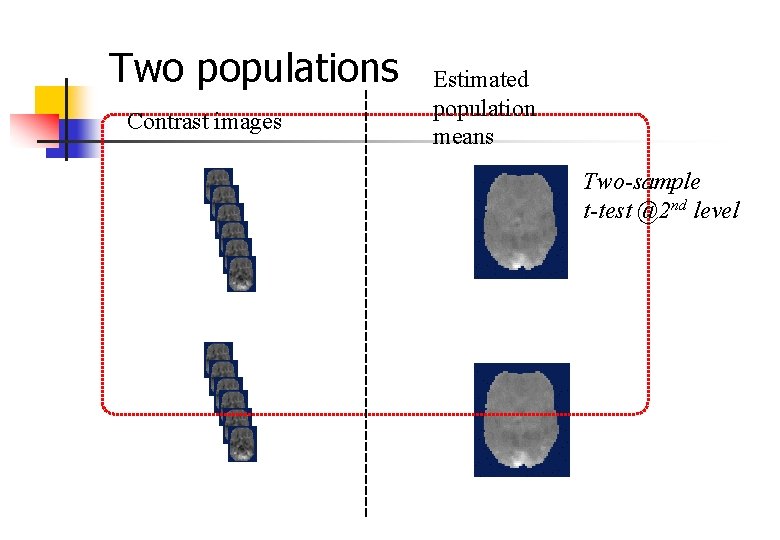
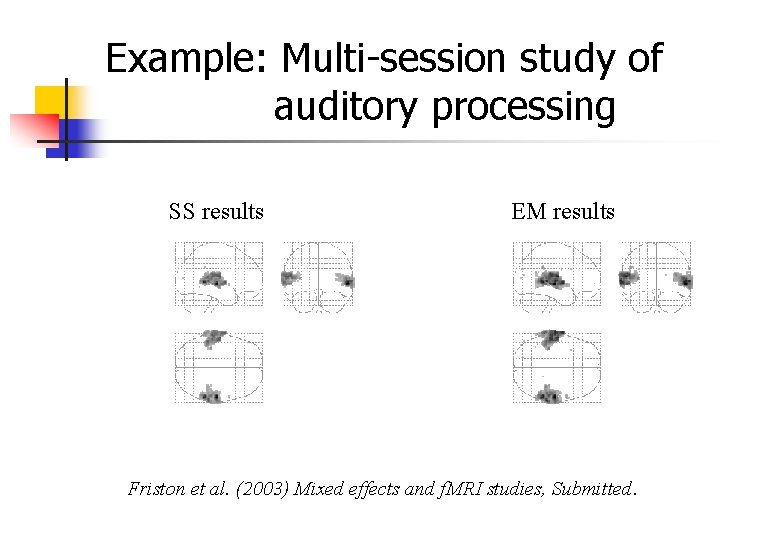
- Slides: 15
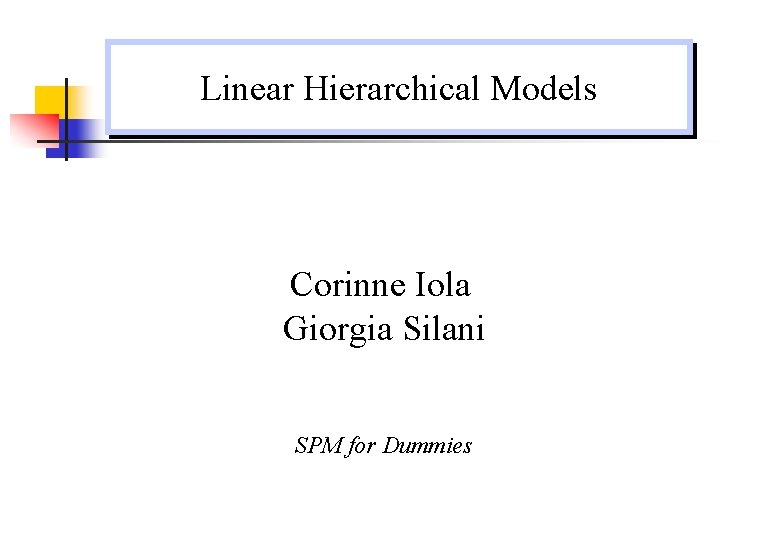
Linear Hierarchical Models Corinne Iola Giorgia Silani SPM for Dummies
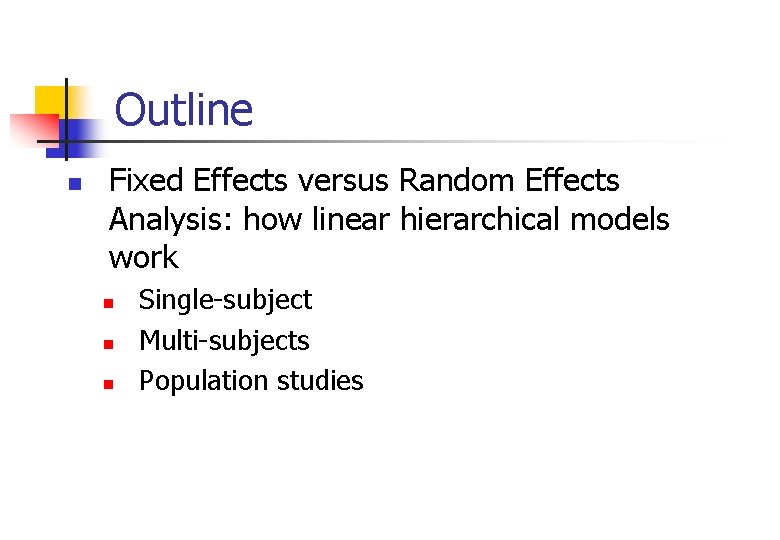
Outline n Fixed Effects versus Random Effects Analysis: how linear hierarchical models work n n n Single-subject Multi-subjects Population studies
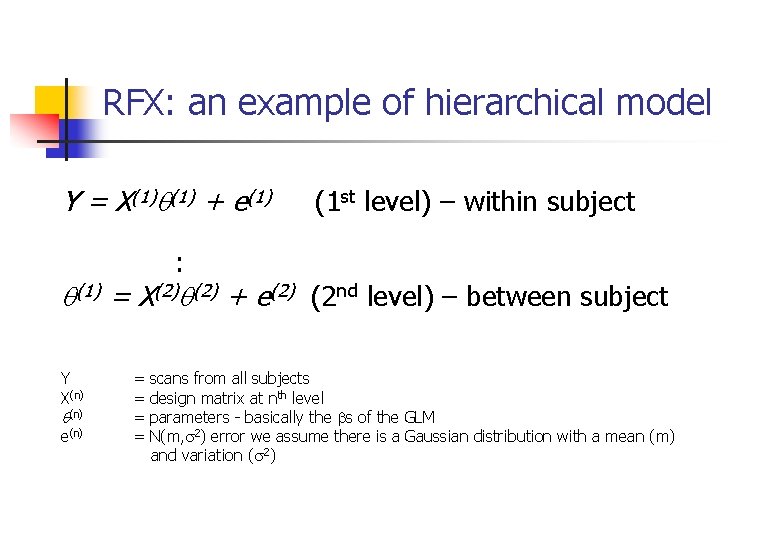
RFX: an example of hierarchical model Y = X(1) + e(1) (1 st level) – within subject : (1) = X(2) + e(2) (2 nd level) – between subject Y X(n) e(n) = = scans from all subjects design matrix at nth level parameters - basically the s of the GLM N(m, 2) error we assume there is a Gaussian distribution with a mean (m) and variation ( 2)
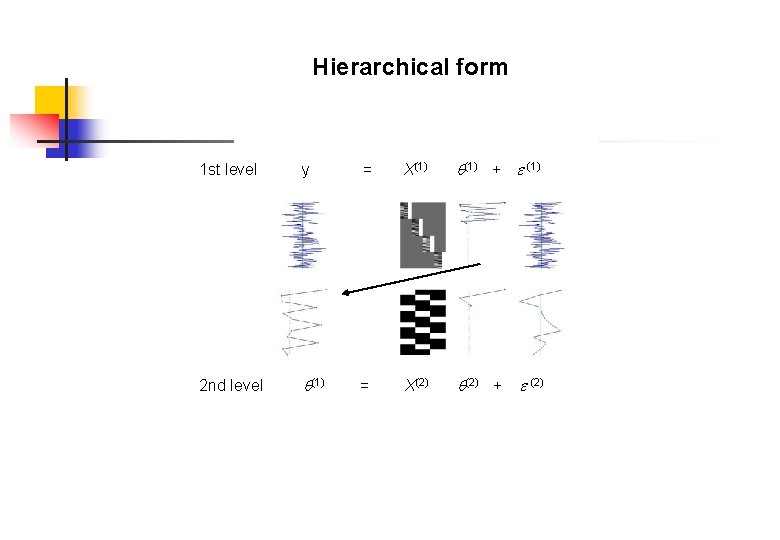
Hierarchical form 1 st level y = X(1) + (1) 2 nd level (1) = X(2) + (2)
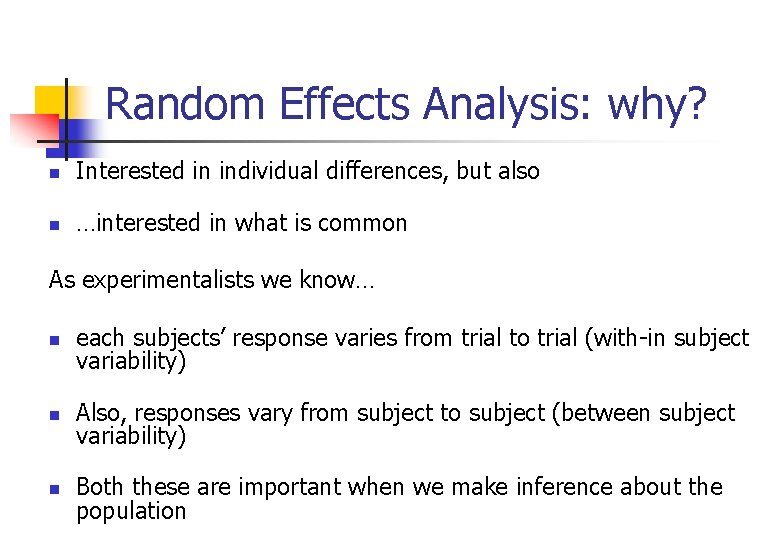
Random Effects Analysis: why? n Interested in individual differences, but also n …interested in what is common As experimentalists we know… n each subjects’ response varies from trial to trial (with-in subject variability) n Also, responses vary from subject to subject (between subject variability) n Both these are important when we make inference about the population
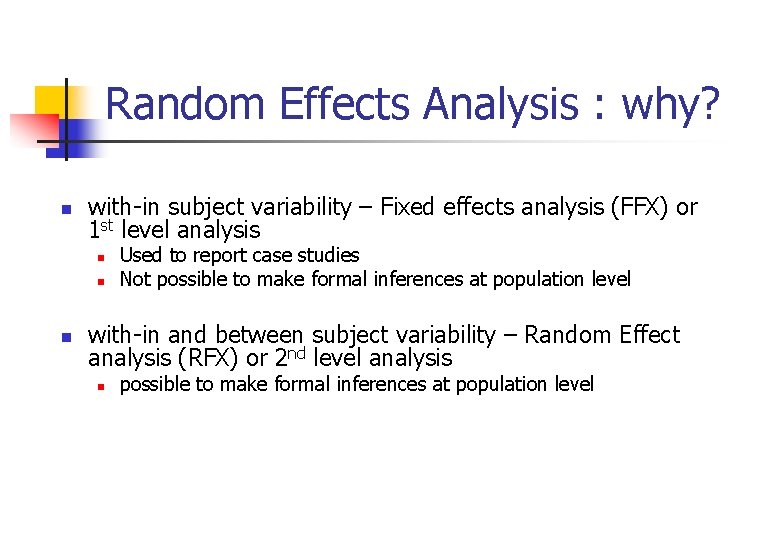
Random Effects Analysis : why? n with-in subject variability – Fixed effects analysis (FFX) or 1 st level analysis n n n Used to report case studies Not possible to make formal inferences at population level with-in and between subject variability – Random Effect analysis (RFX) or 2 nd level analysis n possible to make formal inferences at population level
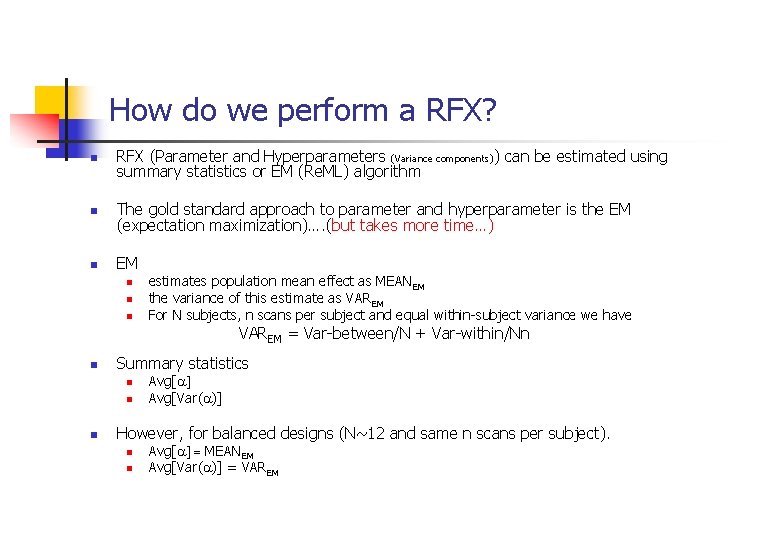
How do we perform a RFX? n RFX (Parameter and Hyperparameters (Variance components)) can be estimated using summary statistics or EM (Re. ML) algorithm n The gold standard approach to parameter and hyperparameter is the EM (expectation maximization)…. (but takes more time…) n EM n n n estimates population mean effect as MEANEM the variance of this estimate as VAREM For N subjects, n scans per subject and equal within-subject variance we have VAREM = Var-between/N + Var-within/Nn n Summary statistics n n n Avg[a] Avg[Var(a)] However, for balanced designs (N~12 and same n scans per subject). n n Avg[a] = MEANEM Avg[Var(a)] = VAREM
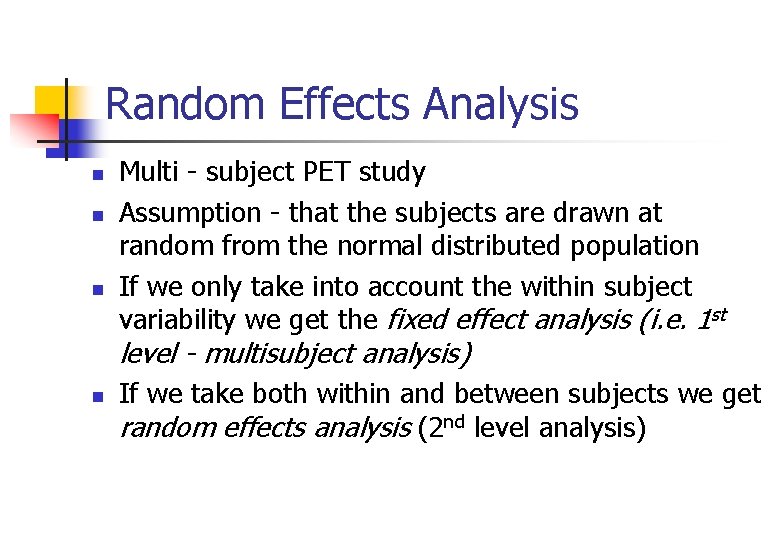
Random Effects Analysis n n n Multi - subject PET study Assumption - that the subjects are drawn at random from the normal distributed population If we only take into account the within subject variability we get the fixed effect analysis (i. e. 1 st level - multisubject analysis) n If we take both within and between subjects we get random effects analysis (2 nd level analysis)
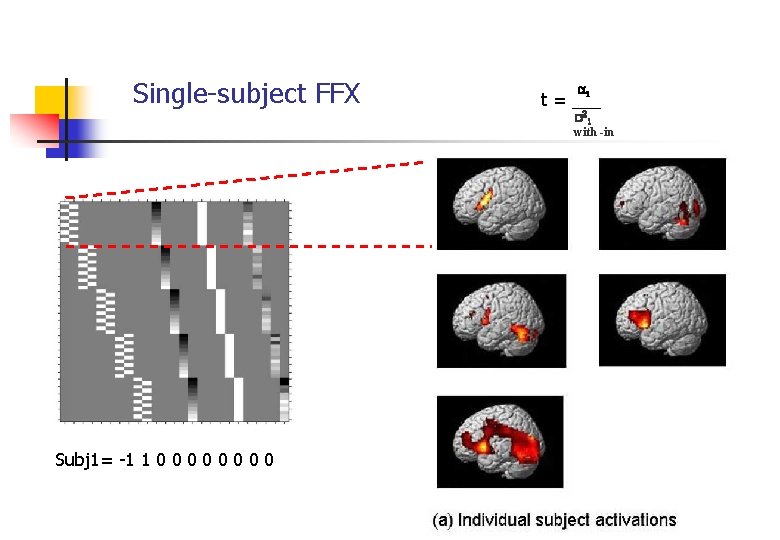
Single-subject FFX 1 t = ___ ^ 1 with -in Subj 1= -1 1 0 0 0 0
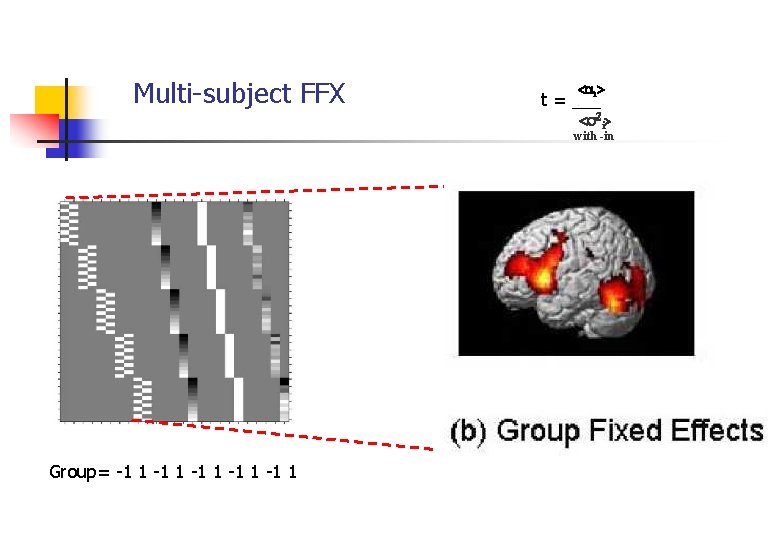
Multi-subject FFX < > t = ___i ^ < i> with -in Group= -1 1 -1 1
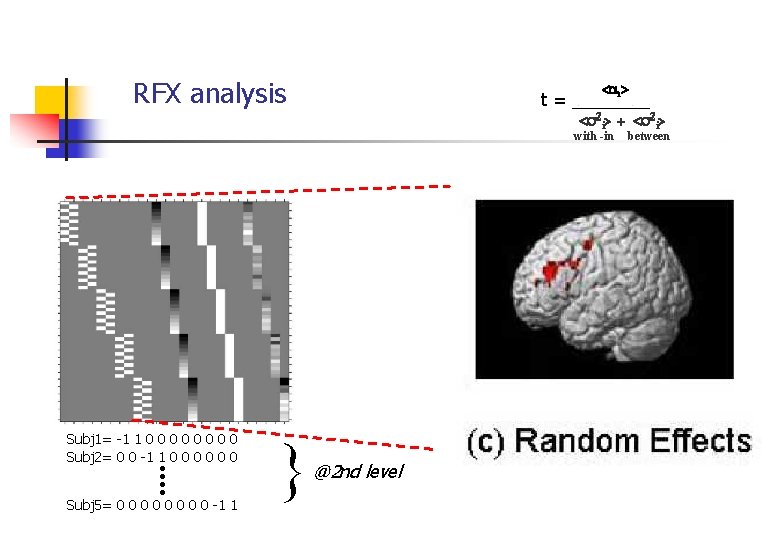
RFX analysis < > i t = ____ ^ < i> + with -in Subj 1= -1 1 0 0 0 0 Subj 2= 0 0 -1 1 0 0 0 Subj 5= 0 0 0 0 -1 1 } @2 nd level ^ < i> between
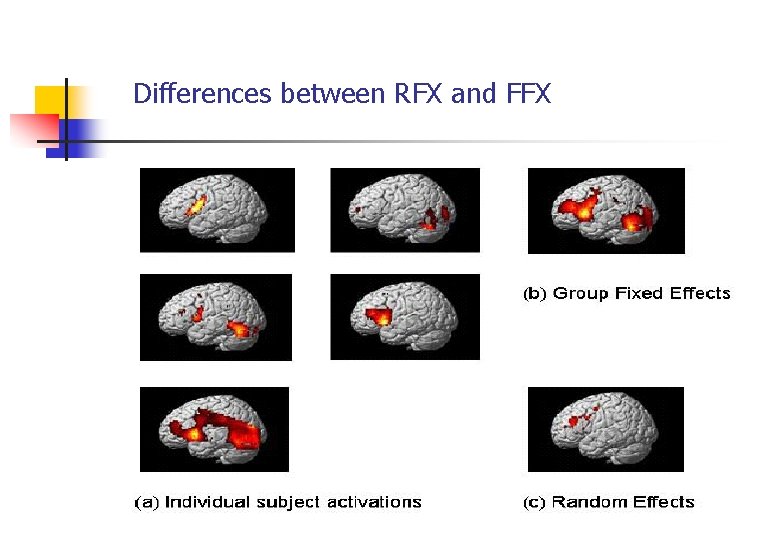
Differences between RFX and FFX
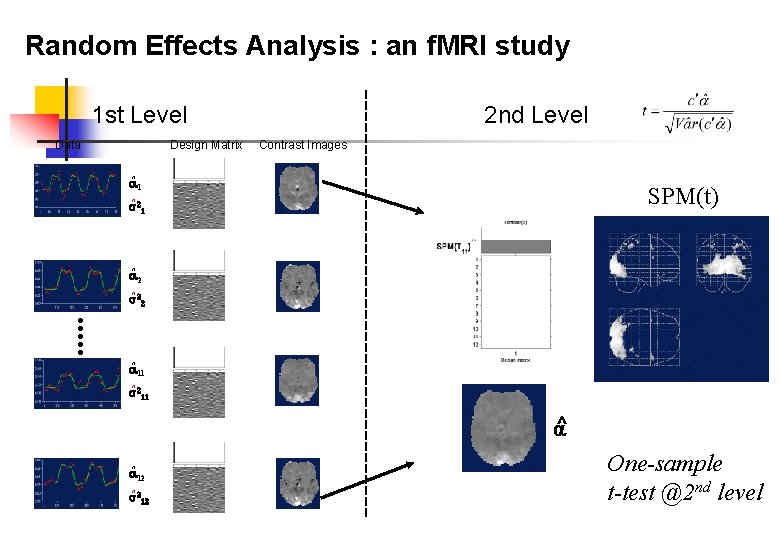
Random Effects Analysis : an f. MRI study 1 st Level Data Design Matrix 2 nd Level Contrast Images ^ 1 SPM(t) ^ 1 ^ 2 ^ ^ 11 ^ 11 ^ ^ 12 ^ 1 One-sample t-test @2 nd level
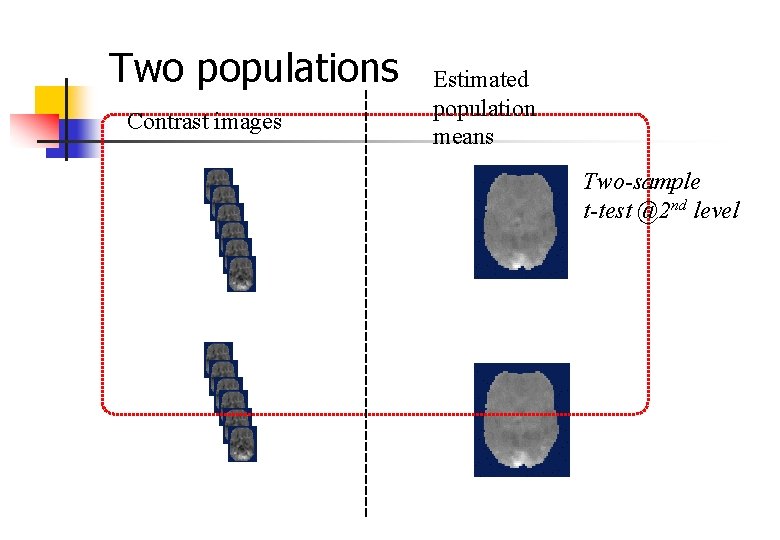
Two populations Contrast images Estimated population means Two-sample t-test @2 nd level
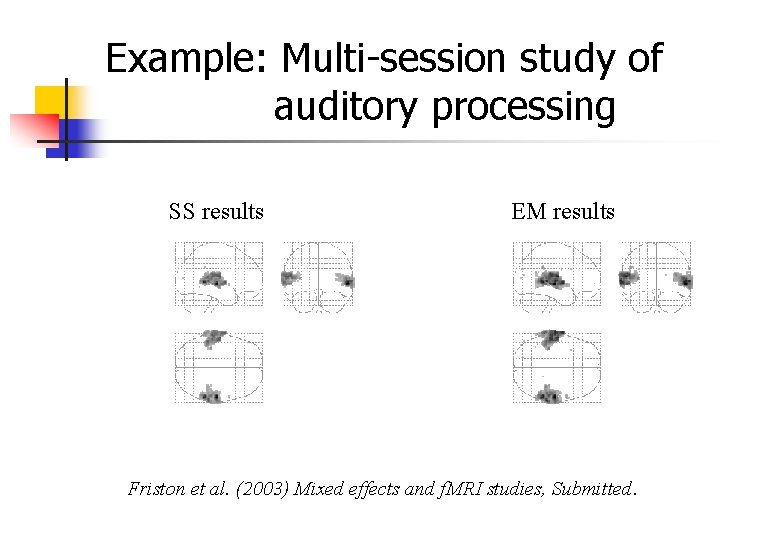
Example: Multi-session study of auditory processing SS results EM results Friston et al. (2003) Mixed effects and f. MRI studies, Submitted.