Kakhramon Yusupov June 15 th 2017 1 30
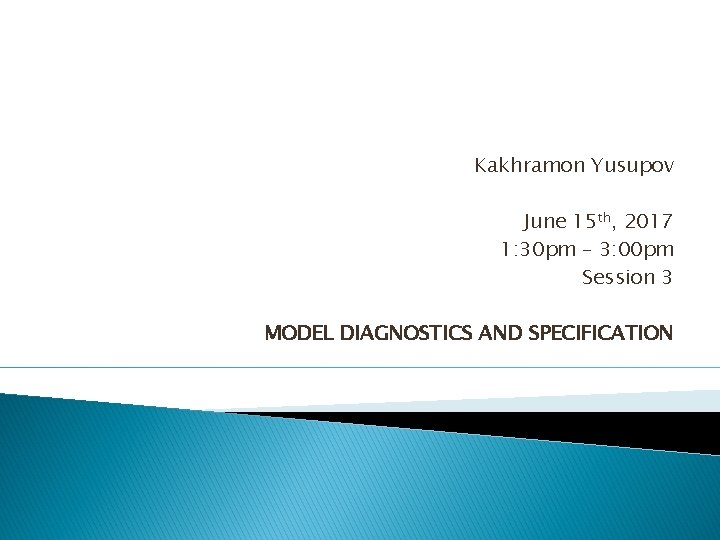
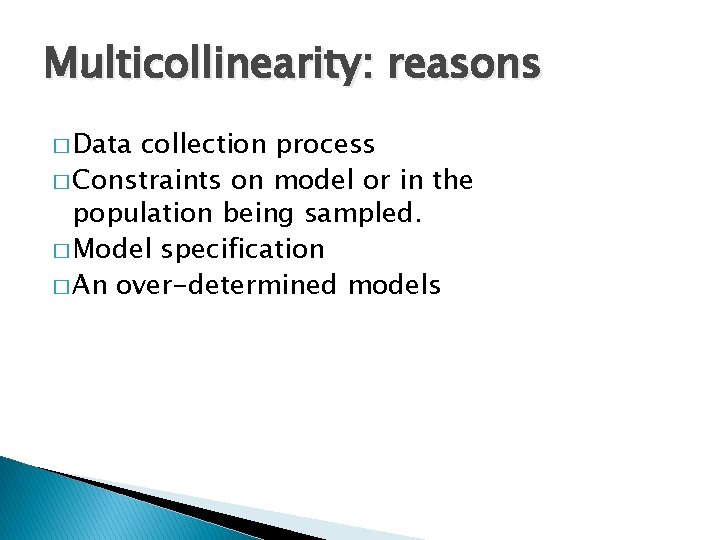
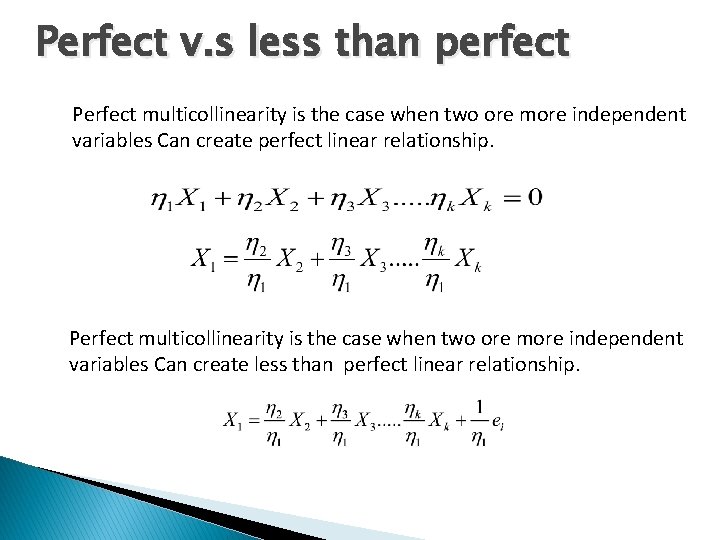
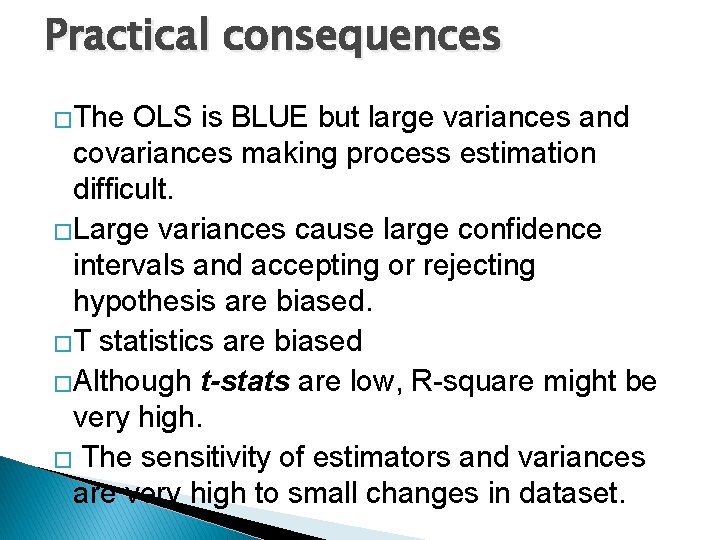
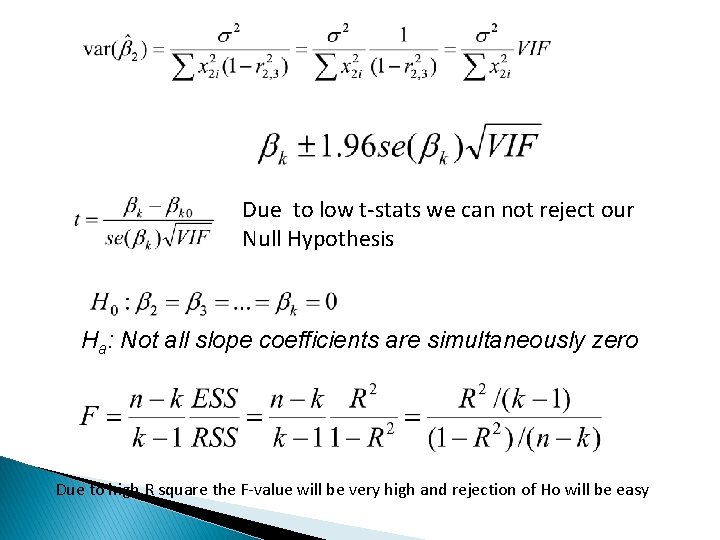
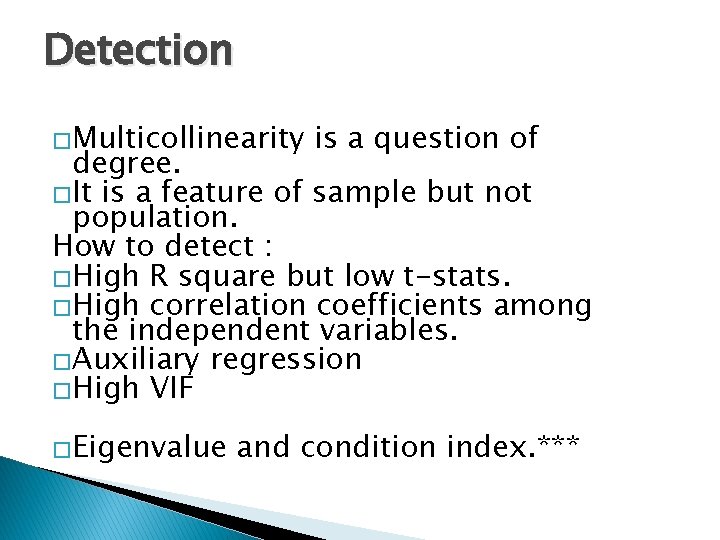
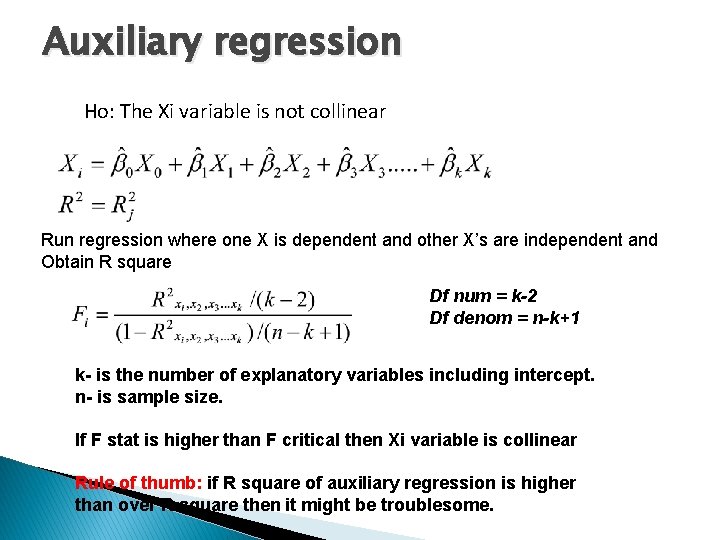
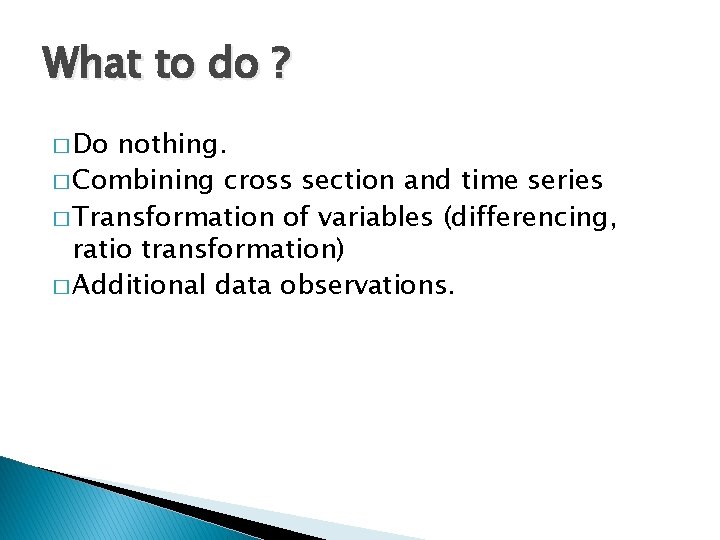
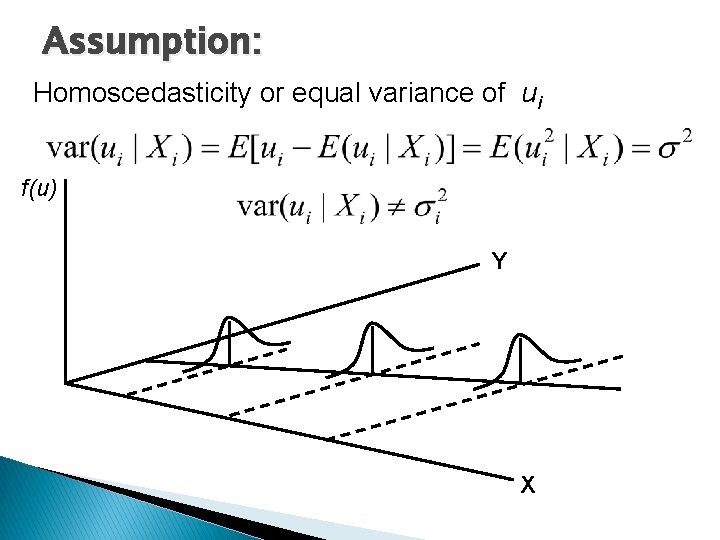
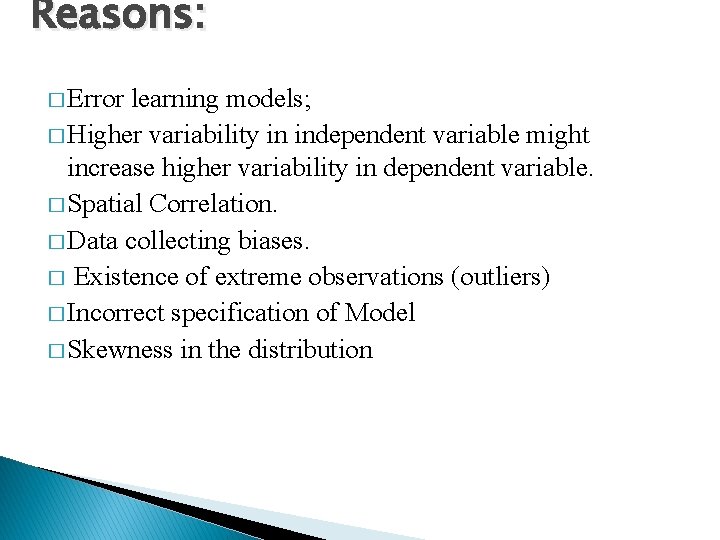
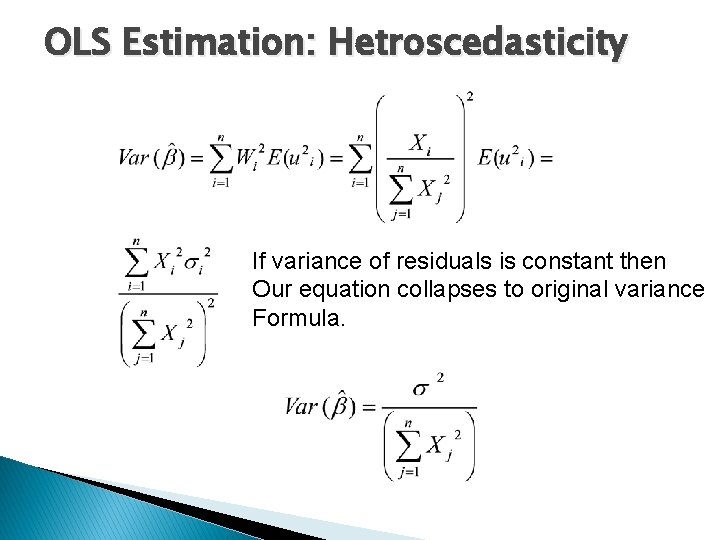
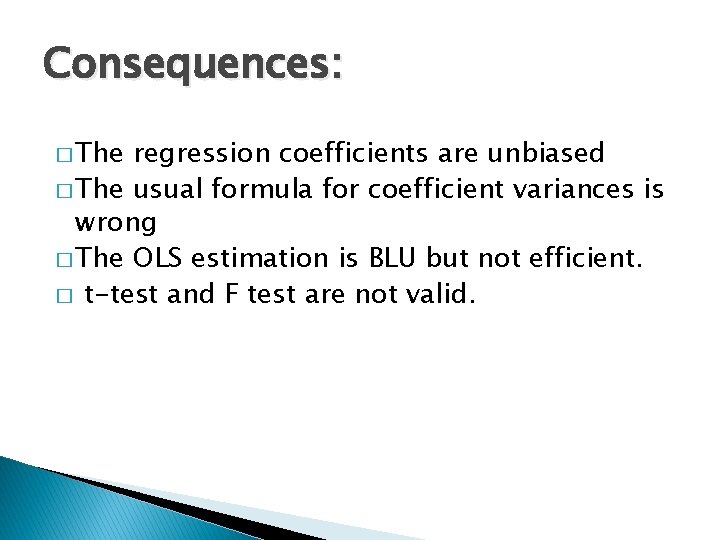
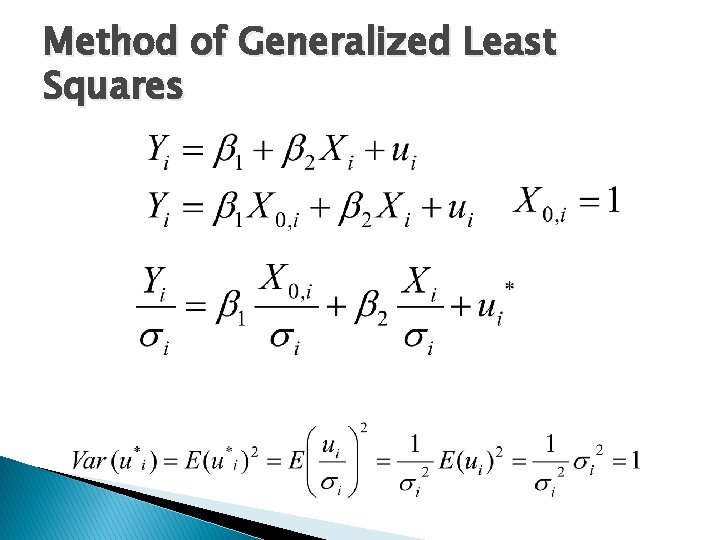
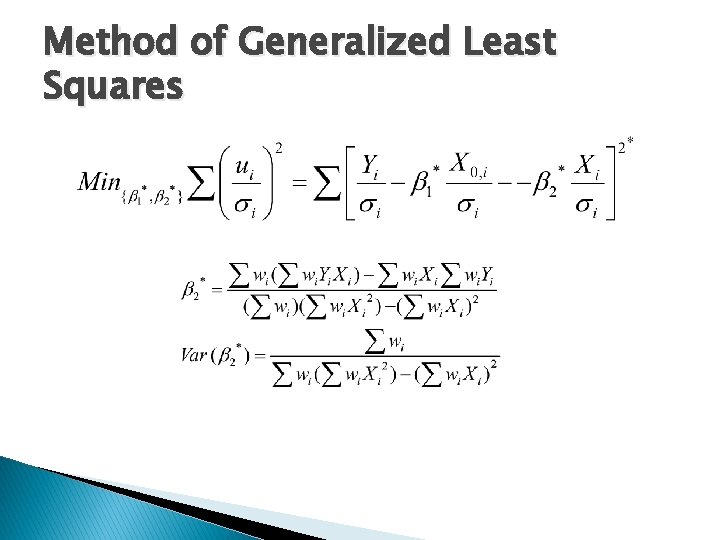
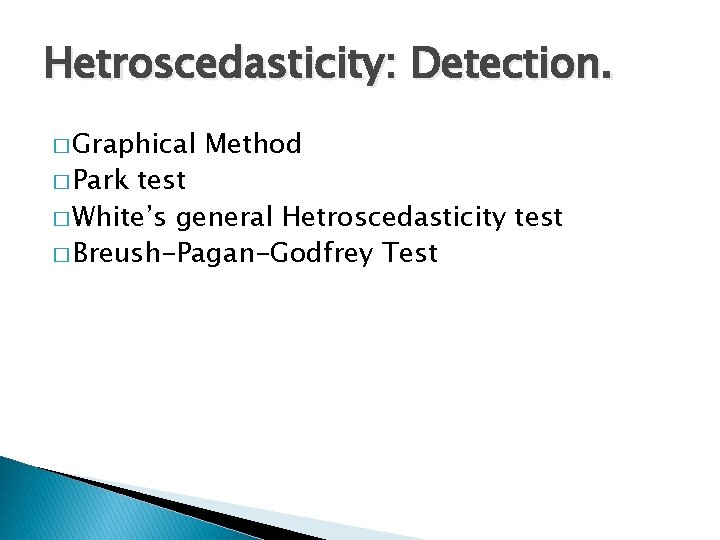
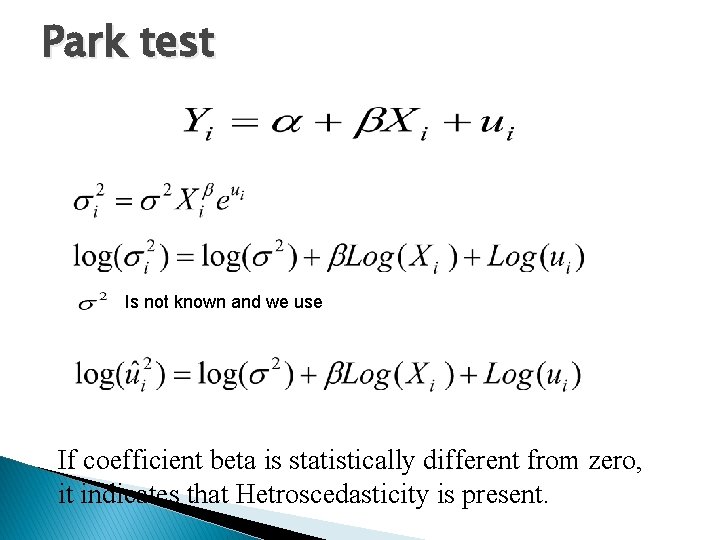
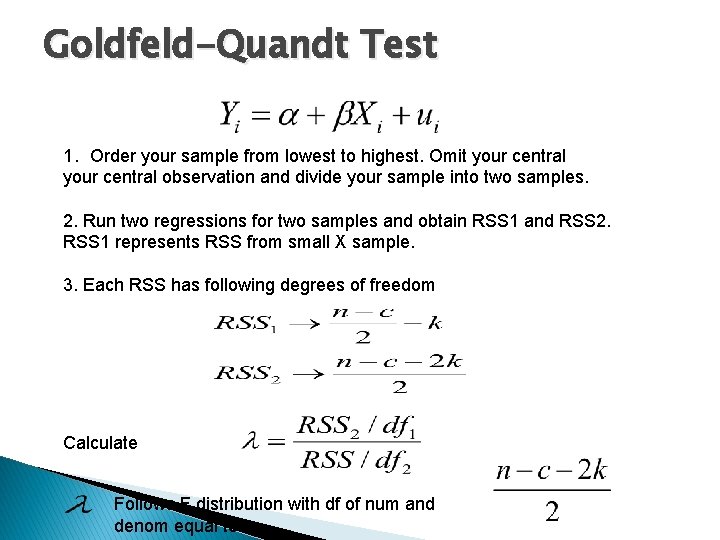
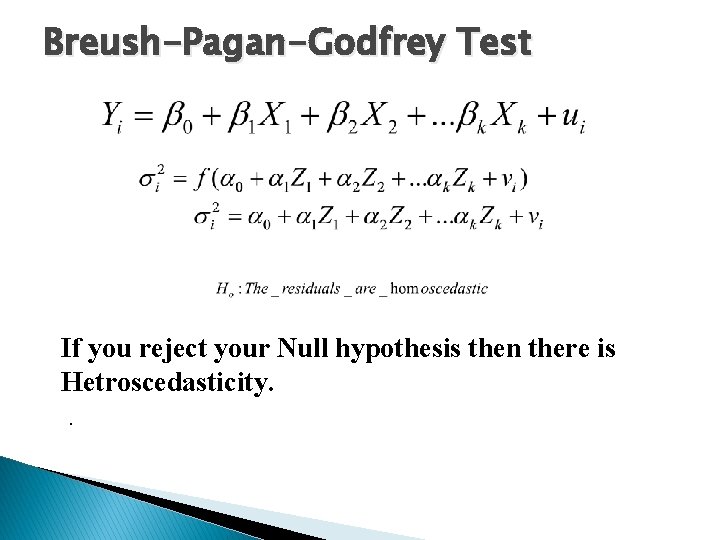
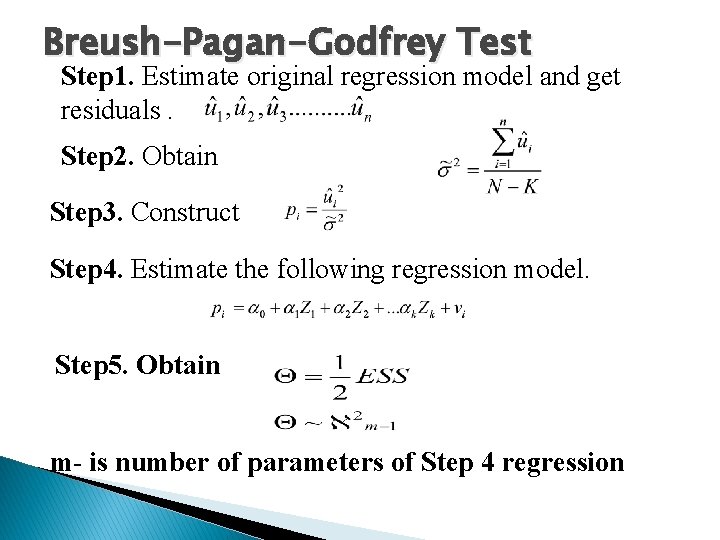
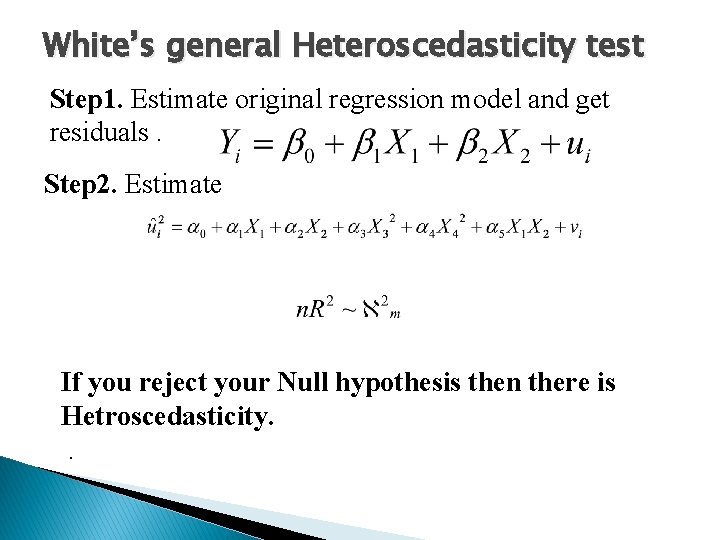
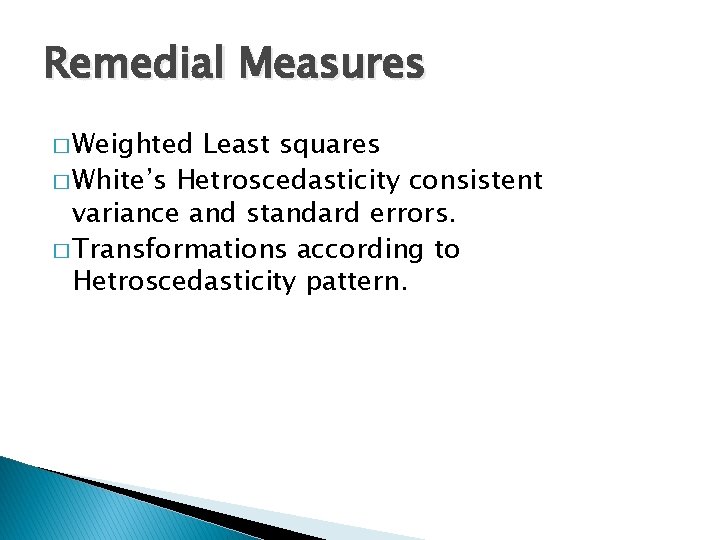
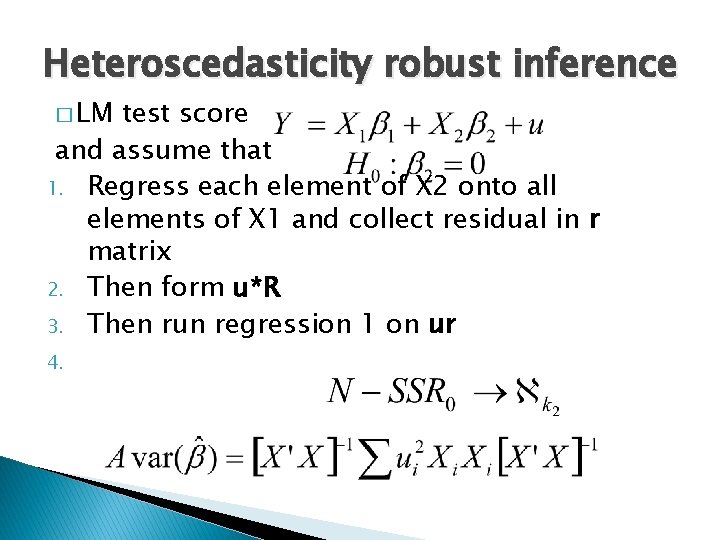
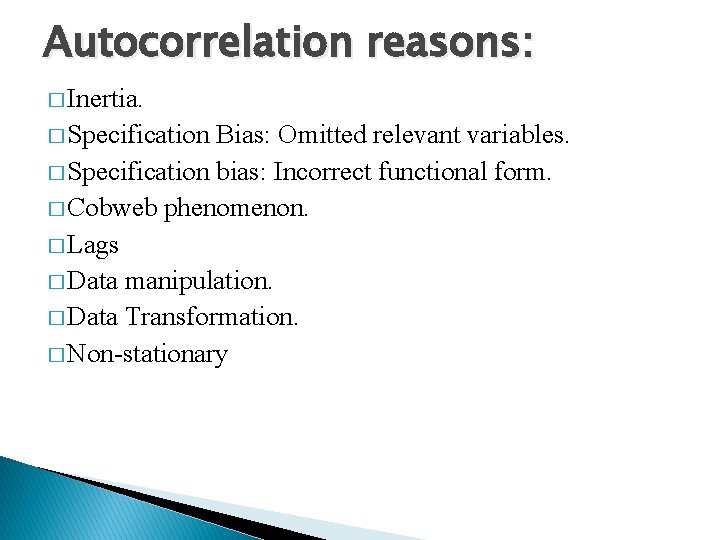
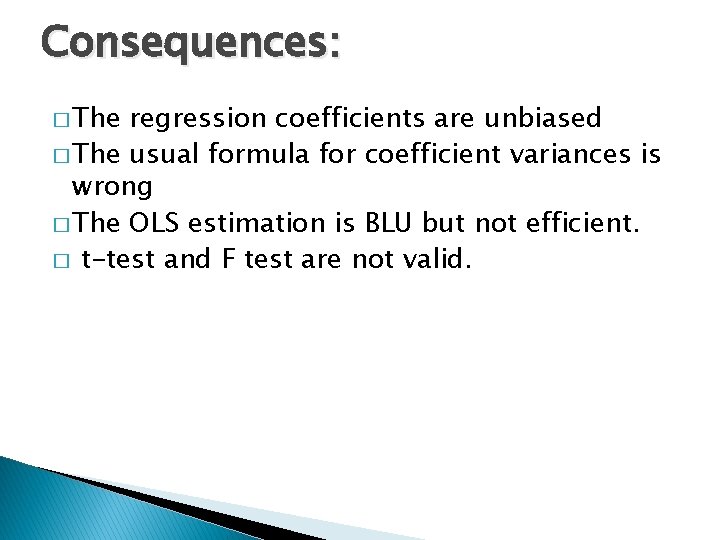
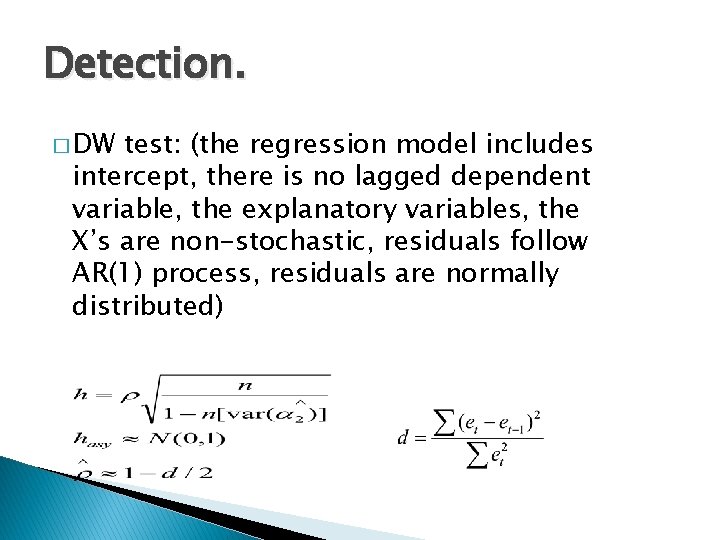
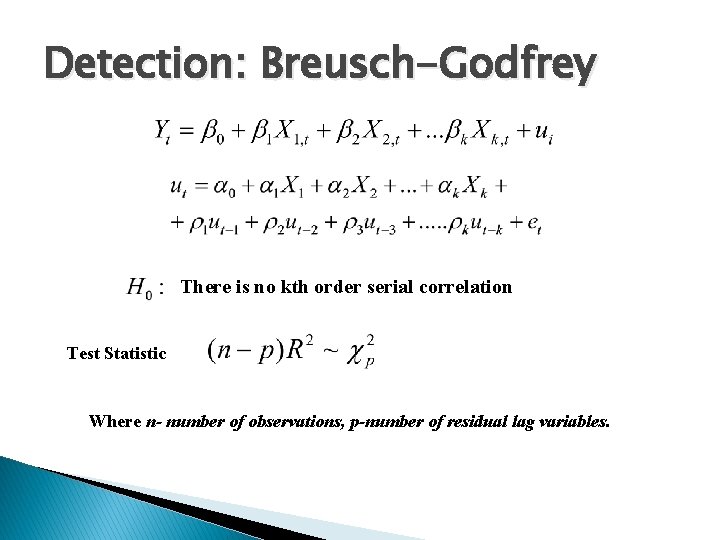
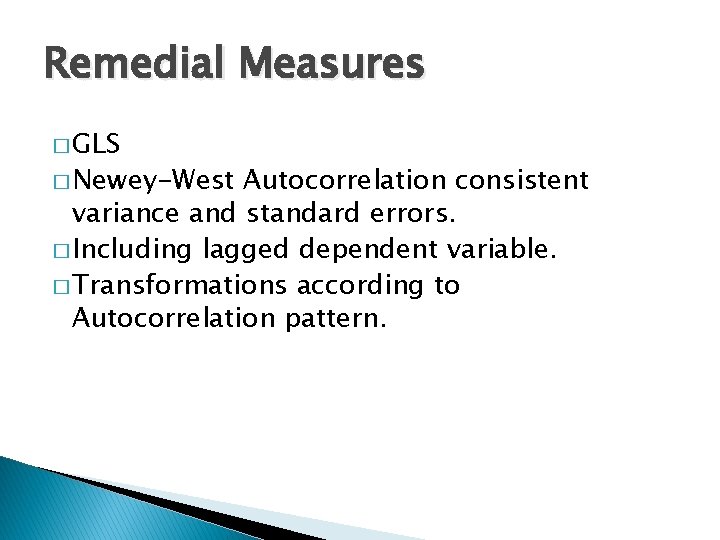
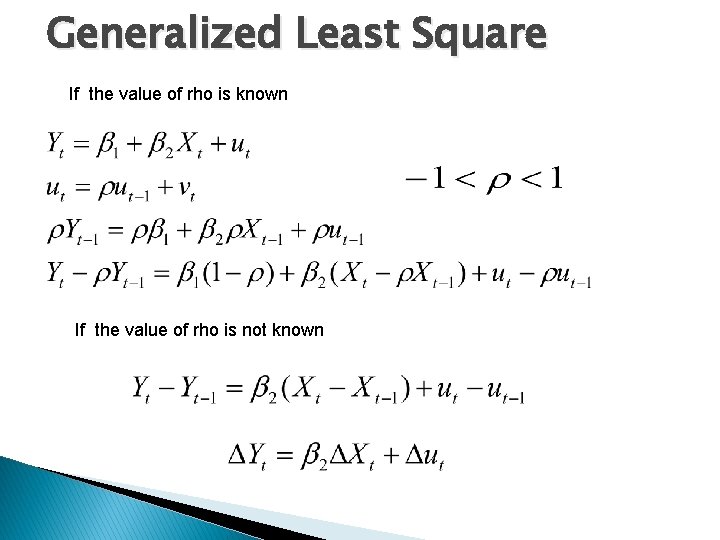
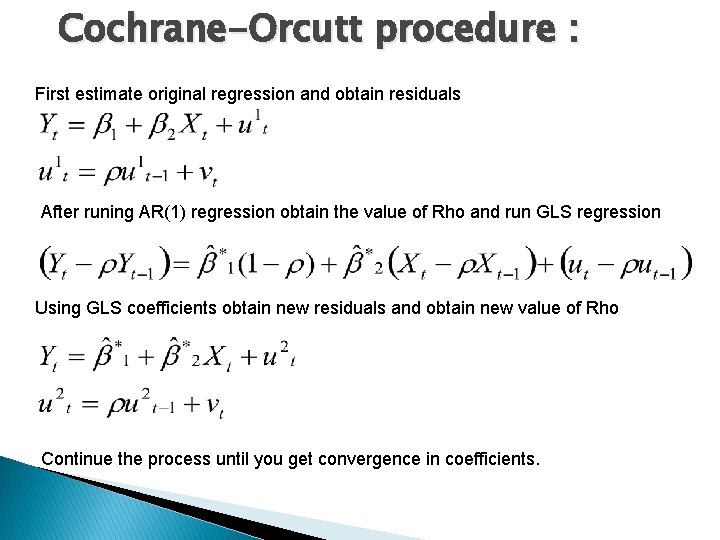
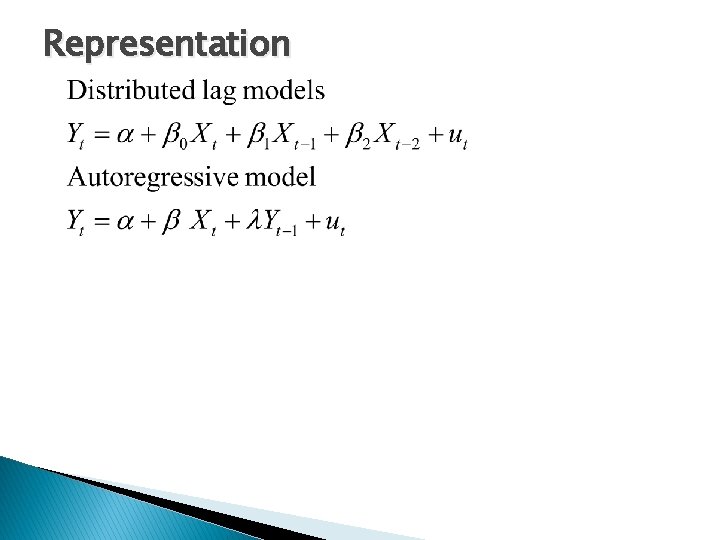
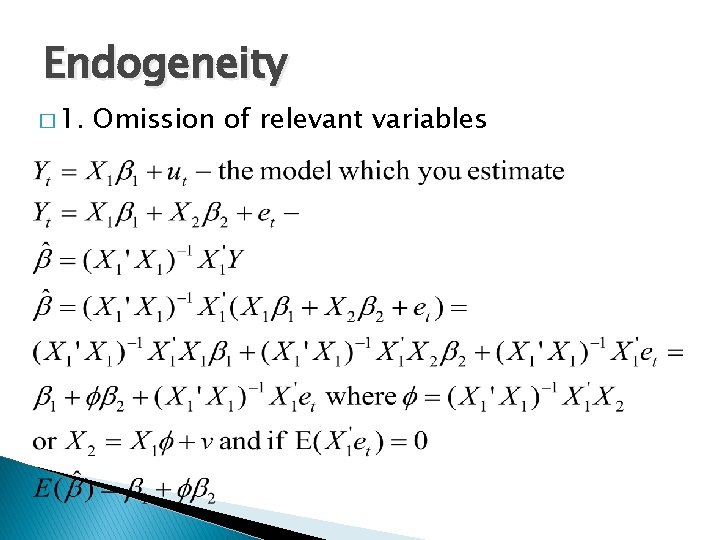
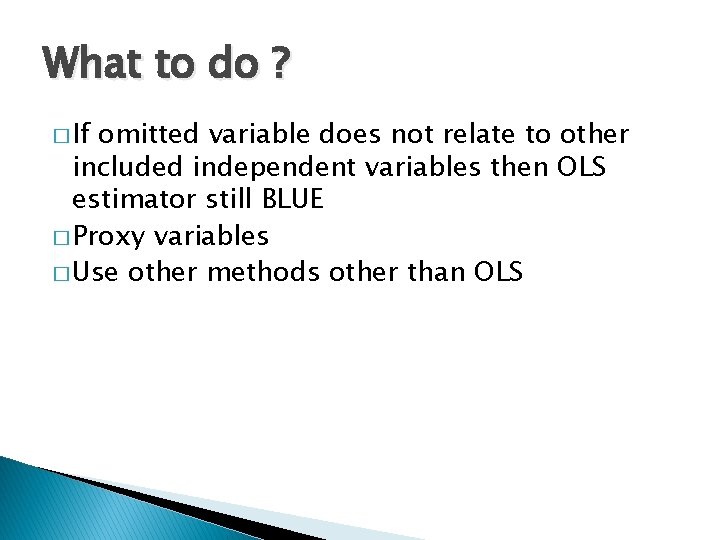
- Slides: 32
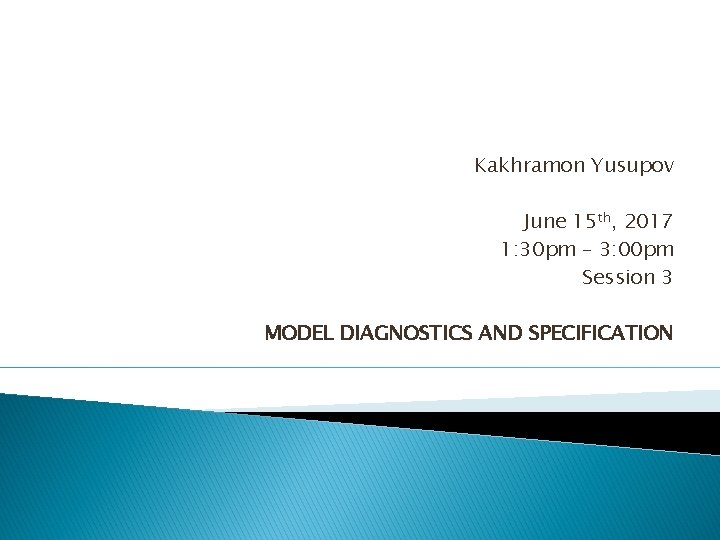
Kakhramon Yusupov June 15 th, 2017 1: 30 pm – 3: 00 pm Session 3 MODEL DIAGNOSTICS AND SPECIFICATION
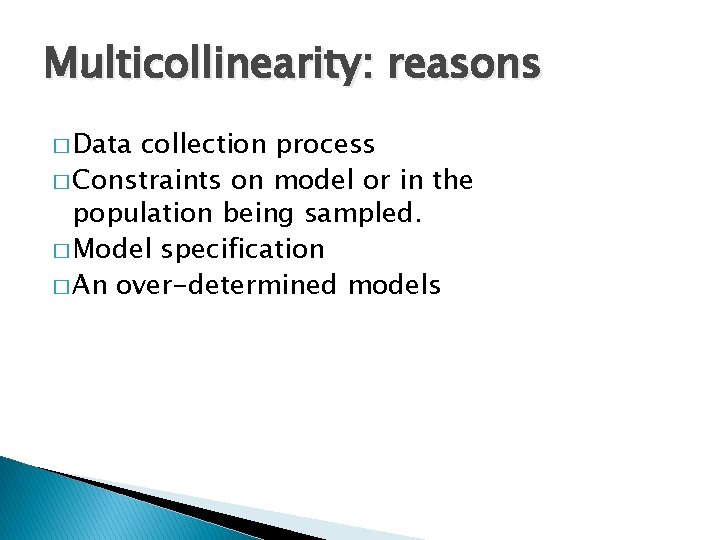
Multicollinearity: reasons � Data collection process � Constraints on model or in the population being sampled. � Model specification � An over-determined models
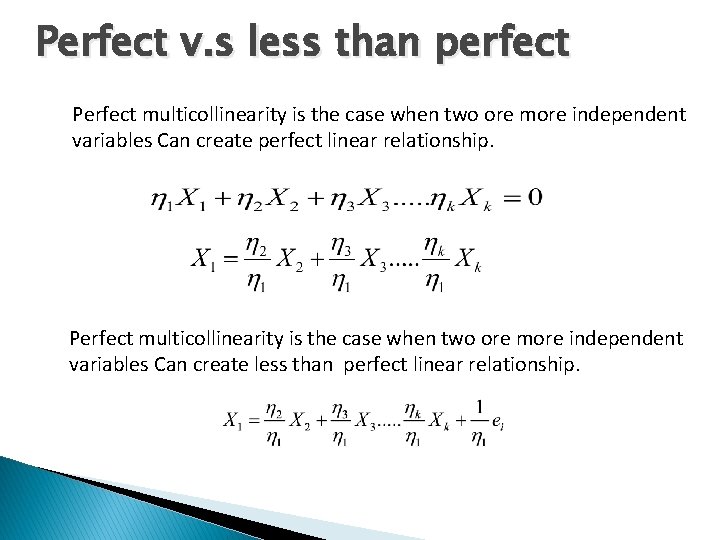
Perfect v. s less than perfect Perfect multicollinearity is the case when two ore more independent variables Can create perfect linear relationship. Perfect multicollinearity is the case when two ore more independent variables Can create less than perfect linear relationship.
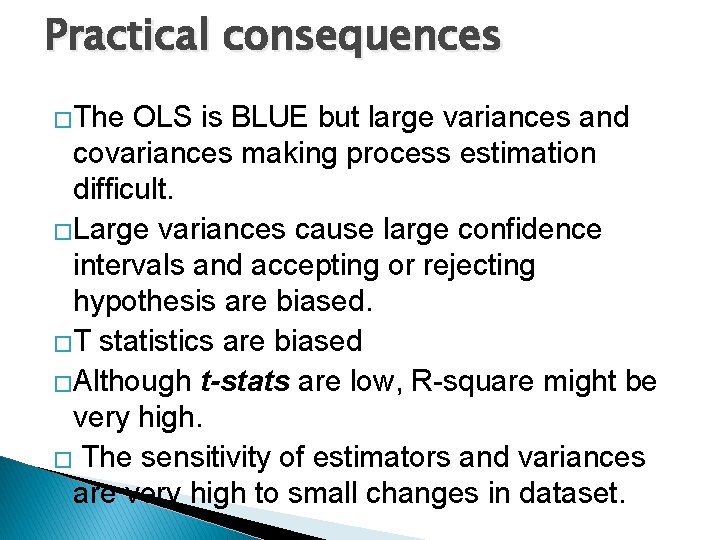
Practical consequences �The OLS is BLUE but large variances and covariances making process estimation difficult. �Large variances cause large confidence intervals and accepting or rejecting hypothesis are biased. �T statistics are biased �Although t-stats are low, R-square might be very high. � The sensitivity of estimators and variances are very high to small changes in dataset.
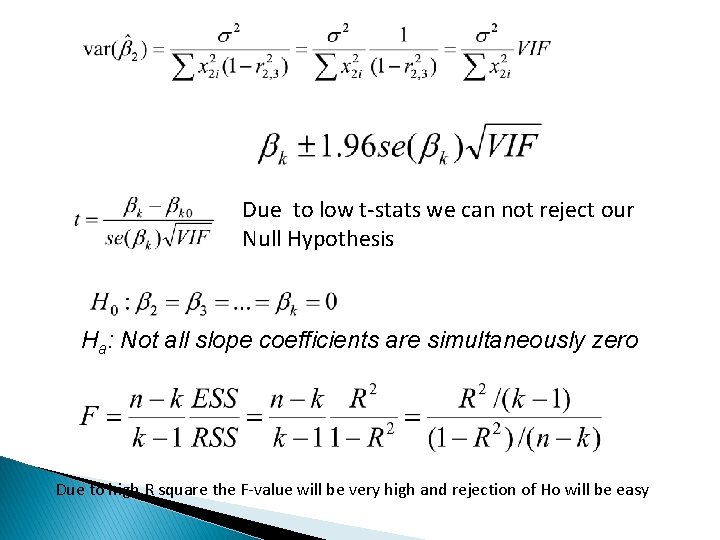
Due to low t-stats we can not reject our Null Hypothesis Ha: Not all slope coefficients are simultaneously zero Due to high R square the F-value will be very high and rejection of Ho will be easy
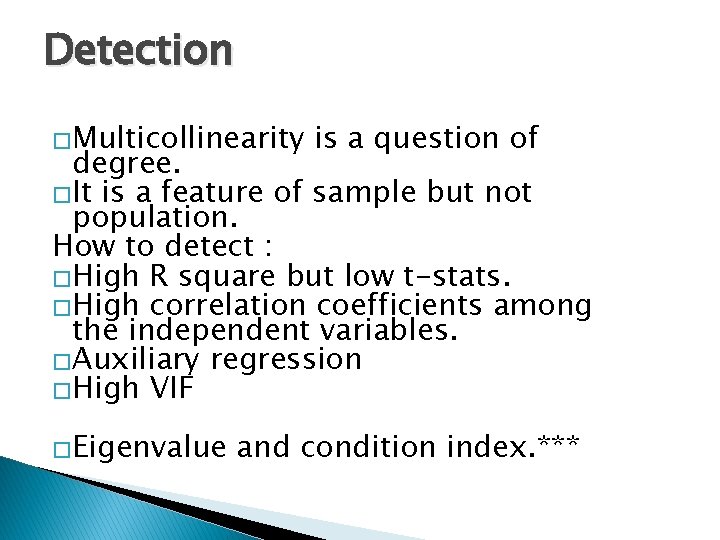
Detection �Multicollinearity is a question of degree. �It is a feature of sample but not population. How to detect : �High R square but low t-stats. �High correlation coefficients among the independent variables. �Auxiliary regression �High VIF �Eigenvalue and condition index. ***
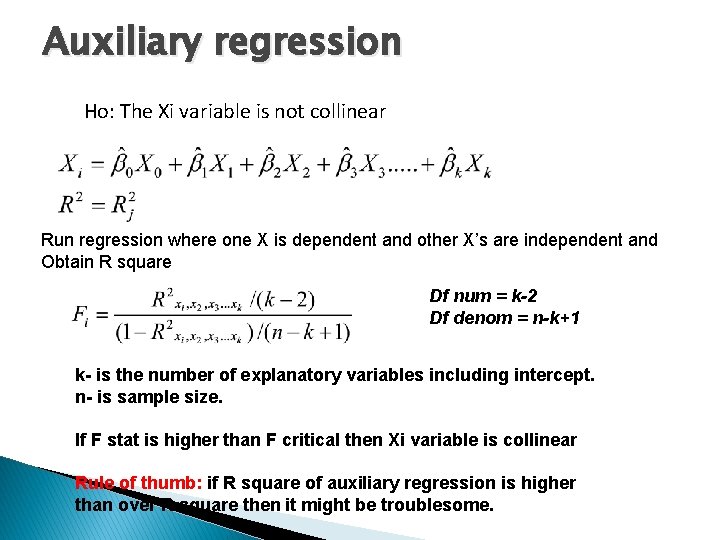
Auxiliary regression Ho: The Xi variable is not collinear Run regression where one X is dependent and other X’s are independent and Obtain R square Df num = k-2 Df denom = n-k+1 k- is the number of explanatory variables including intercept. n- is sample size. If F stat is higher than F critical then Xi variable is collinear Rule of thumb: if R square of auxiliary regression is higher than over R square then it might be troublesome.
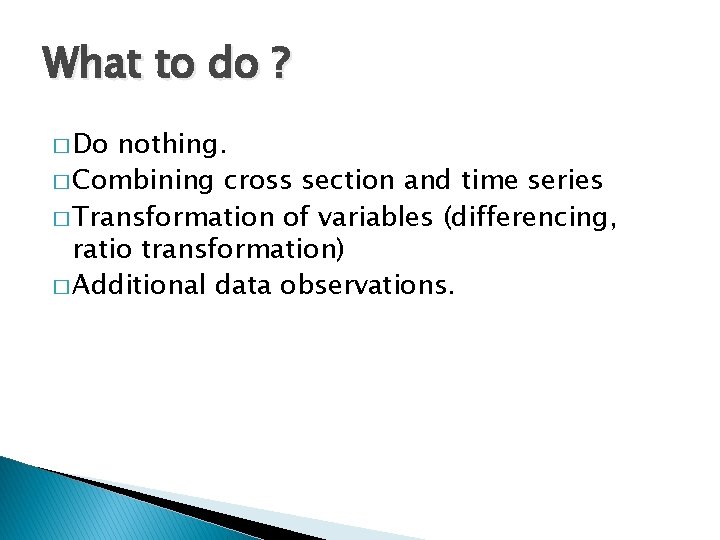
What to do ? � Do nothing. � Combining cross section and time series � Transformation of variables (differencing, ratio transformation) � Additional data observations.
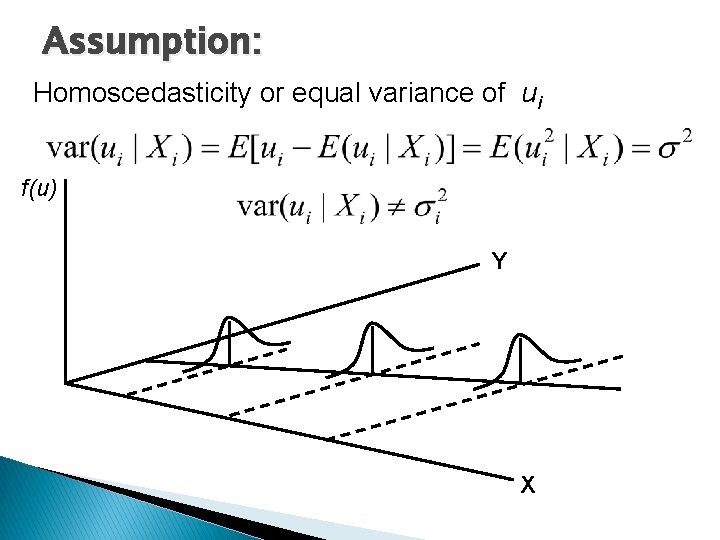
Assumption: Homoscedasticity or equal variance of ui f(u) Y X
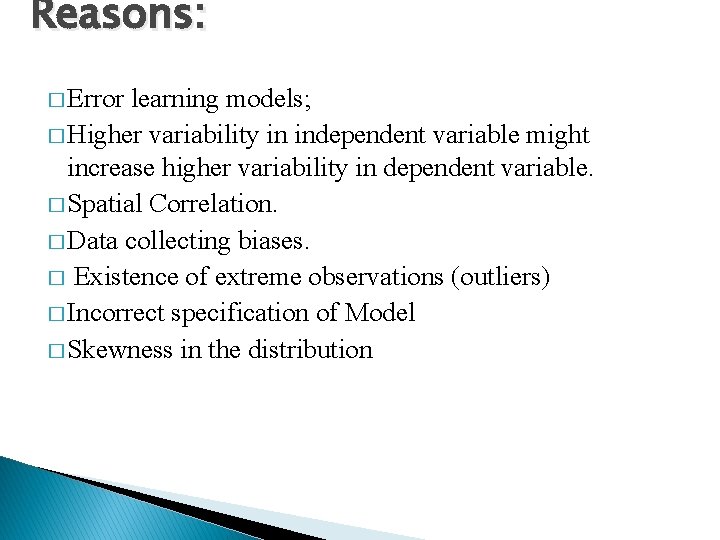
Reasons: � Error learning models; � Higher variability in independent variable might increase higher variability in dependent variable. � Spatial Correlation. � Data collecting biases. � Existence of extreme observations (outliers) � Incorrect specification of Model � Skewness in the distribution
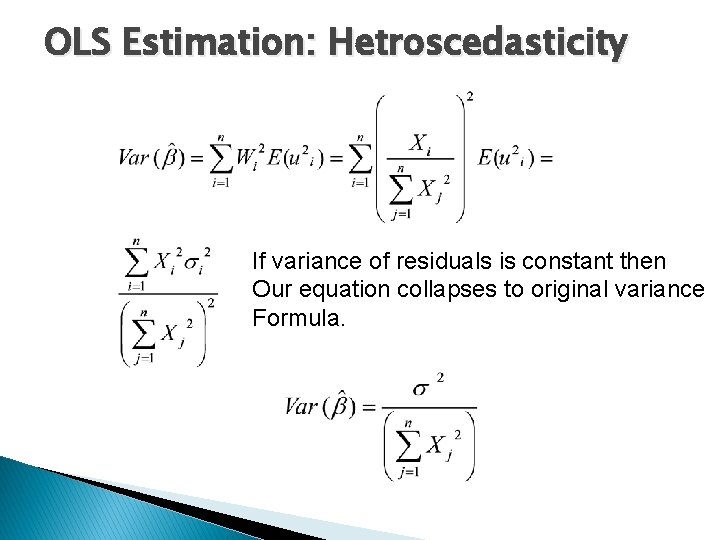
OLS Estimation: Hetroscedasticity If variance of residuals is constant then Our equation collapses to original variance Formula.
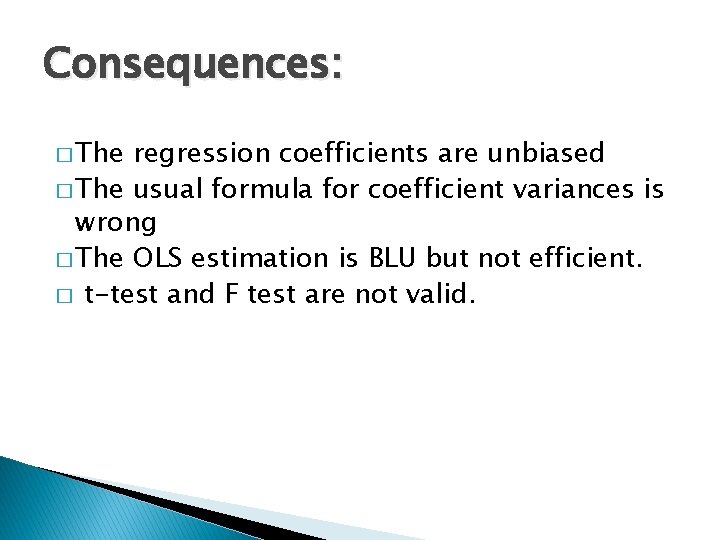
Consequences: � The regression coefficients are unbiased � The usual formula for coefficient variances is wrong � The OLS estimation is BLU but not efficient. � t-test and F test are not valid.
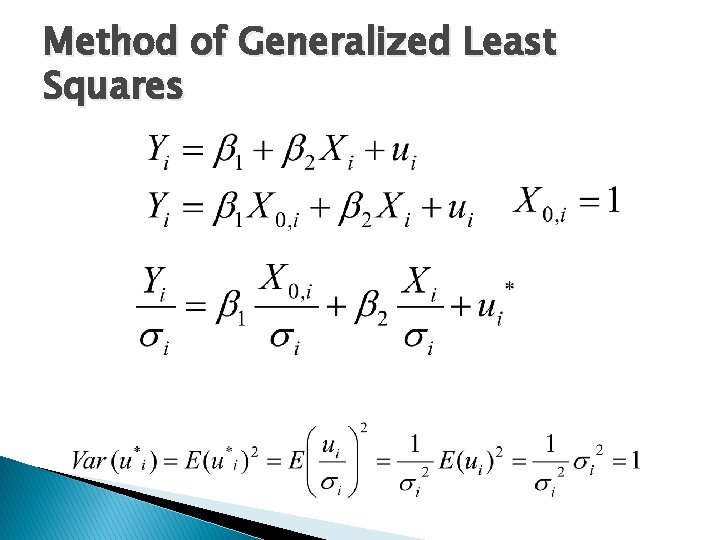
Method of Generalized Least Squares
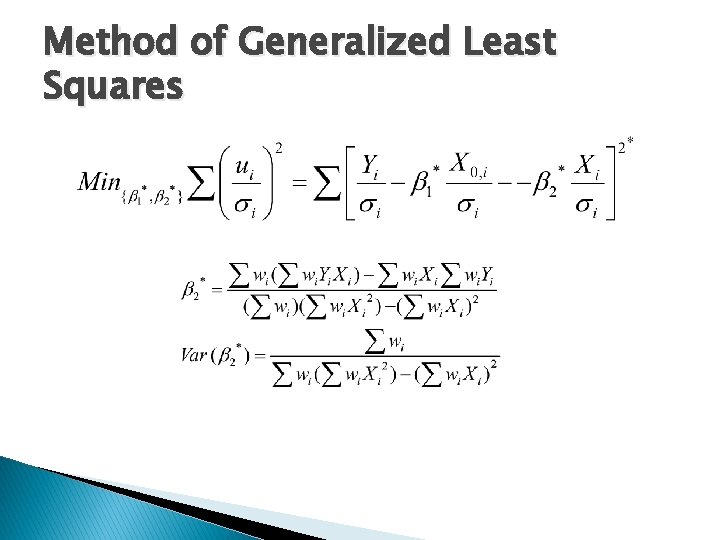
Method of Generalized Least Squares
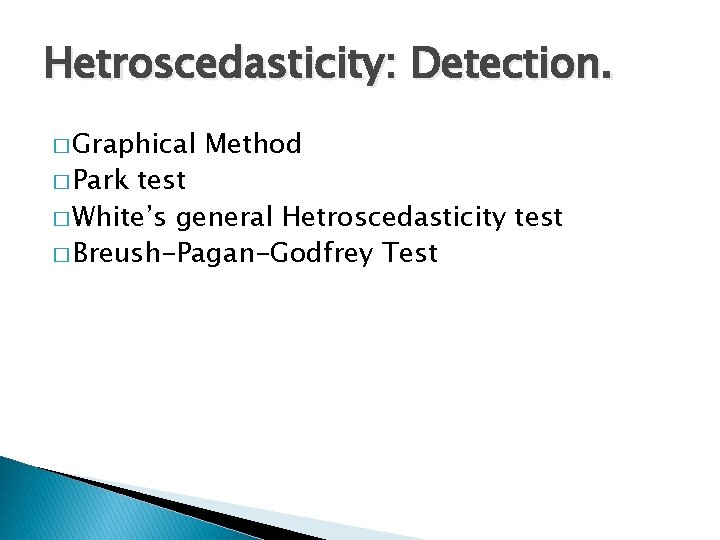
Hetroscedasticity: Detection. � Graphical � Park Method test � White’s general Hetroscedasticity test � Breush-Pagan-Godfrey Test
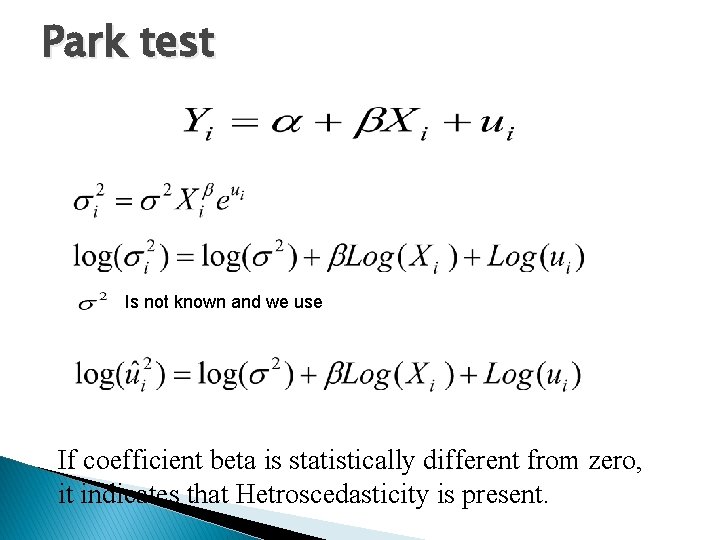
Park test Is not known and we use If coefficient beta is statistically different from zero, it indicates that Hetroscedasticity is present.
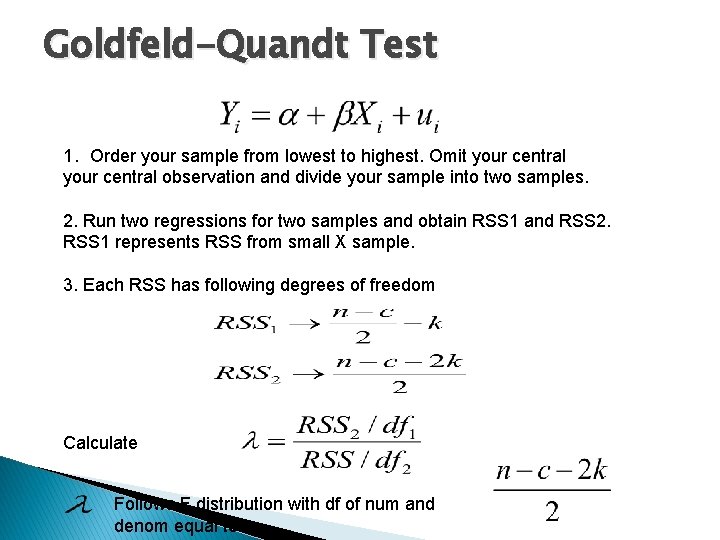
Goldfeld-Quandt Test 1. Order your sample from lowest to highest. Omit your central observation and divide your sample into two samples. 2. Run two regressions for two samples and obtain RSS 1 and RSS 2. RSS 1 represents RSS from small X sample. 3. Each RSS has following degrees of freedom Calculate Follows F distribution with df of num and denom equal to
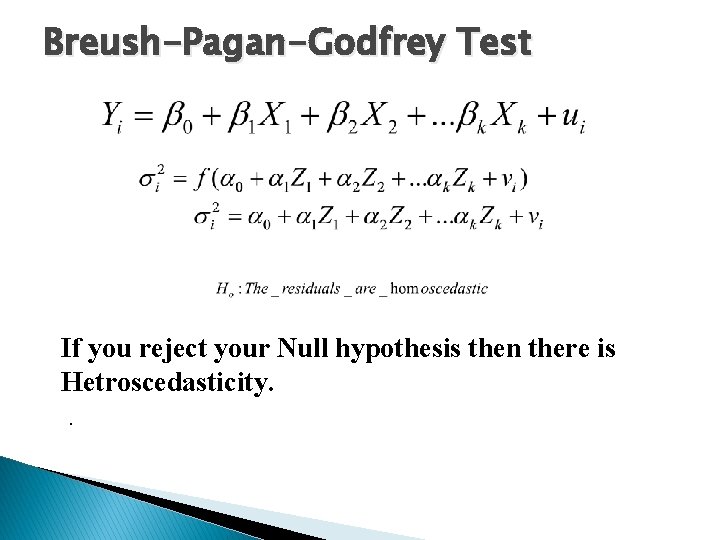
Breush-Pagan-Godfrey Test If you reject your Null hypothesis then there is Hetroscedasticity. .
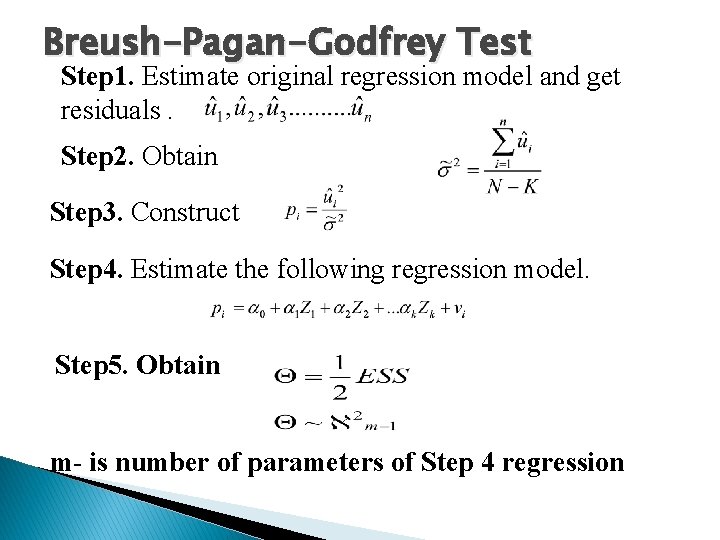
Breush-Pagan-Godfrey Test Step 1. Estimate original regression model and get residuals. Step 2. Obtain Step 3. Construct Step 4. Estimate the following regression model. Step 5. Obtain m- is number of parameters of Step 4 regression
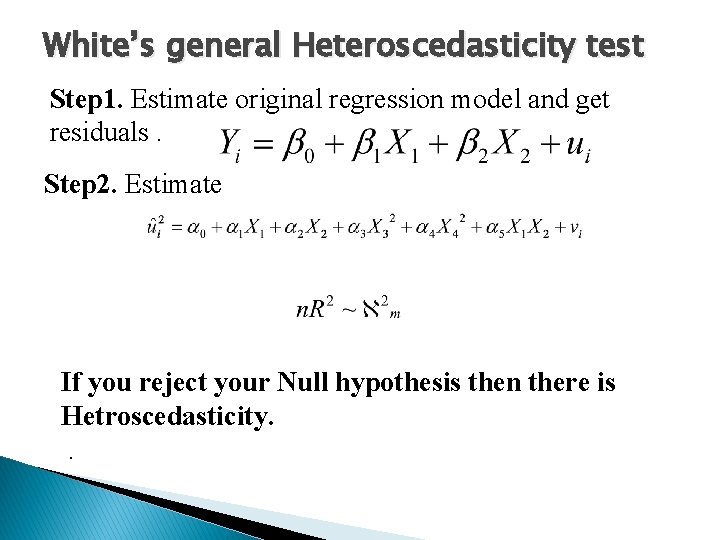
White’s general Heteroscedasticity test Step 1. Estimate original regression model and get residuals. Step 2. Estimate If you reject your Null hypothesis then there is Hetroscedasticity. .
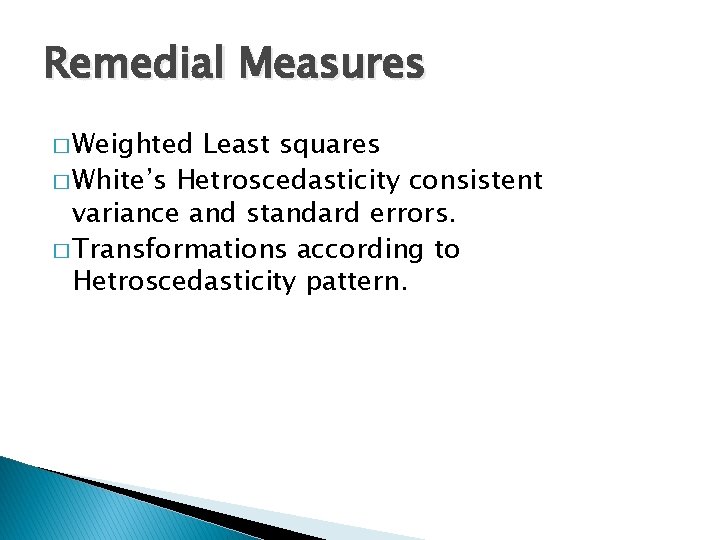
Remedial Measures � Weighted Least squares � White’s Hetroscedasticity consistent variance and standard errors. � Transformations according to Hetroscedasticity pattern.
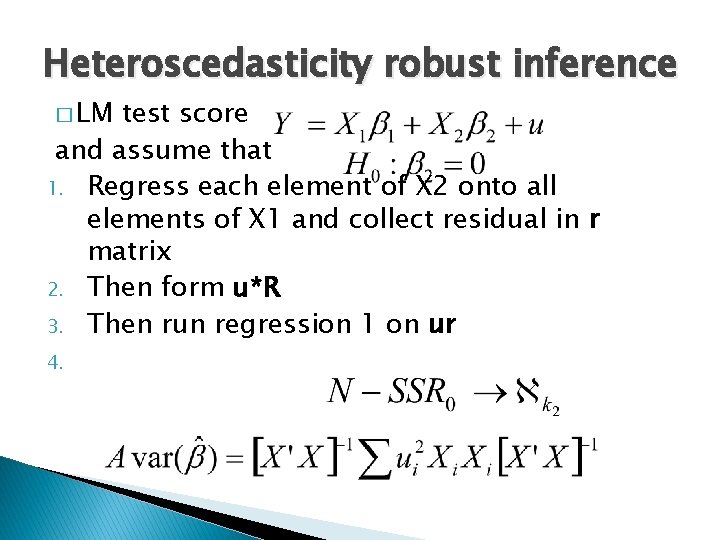
Heteroscedasticity robust inference � LM test score and assume that 1. Regress each element of X 2 onto all elements of X 1 and collect residual in r matrix 2. Then form u*R 3. Then run regression 1 on ur 4.
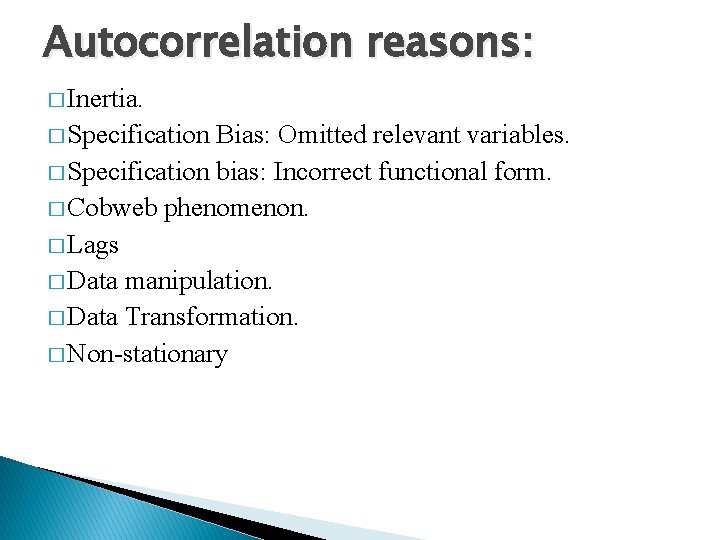
Autocorrelation reasons: � Inertia. � Specification Bias: Omitted relevant variables. � Specification bias: Incorrect functional form. � Cobweb phenomenon. � Lags � Data manipulation. � Data Transformation. � Non-stationary
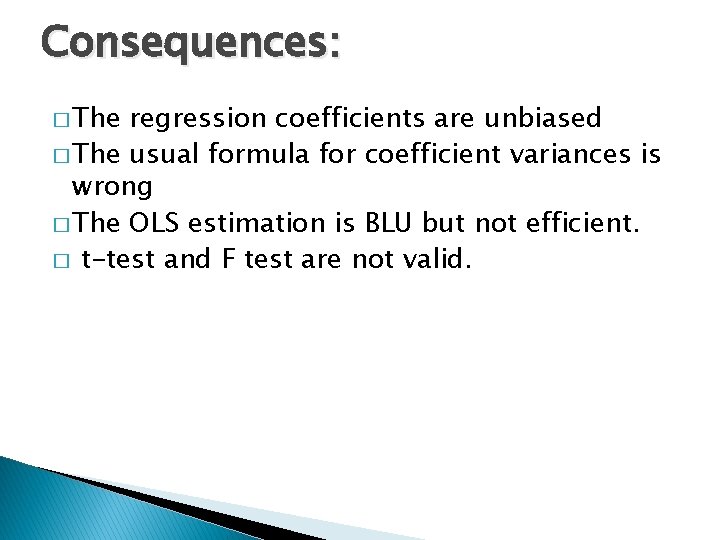
Consequences: � The regression coefficients are unbiased � The usual formula for coefficient variances is wrong � The OLS estimation is BLU but not efficient. � t-test and F test are not valid.
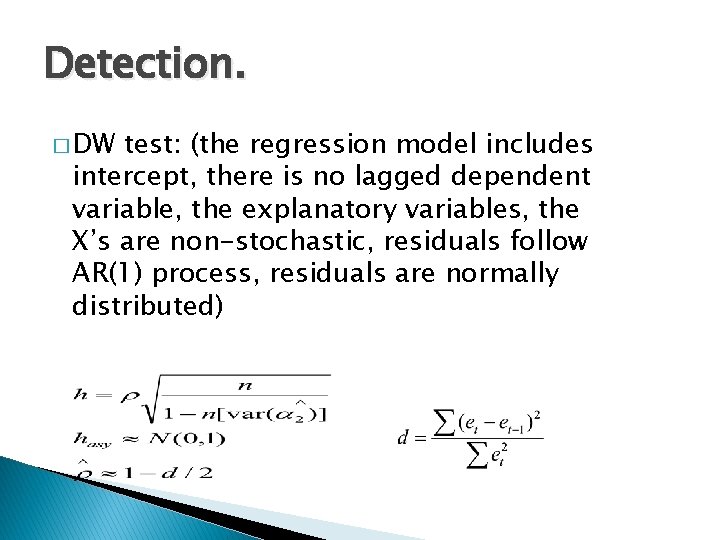
Detection. � DW test: (the regression model includes intercept, there is no lagged dependent variable, the explanatory variables, the X’s are non-stochastic, residuals follow AR(1) process, residuals are normally distributed)
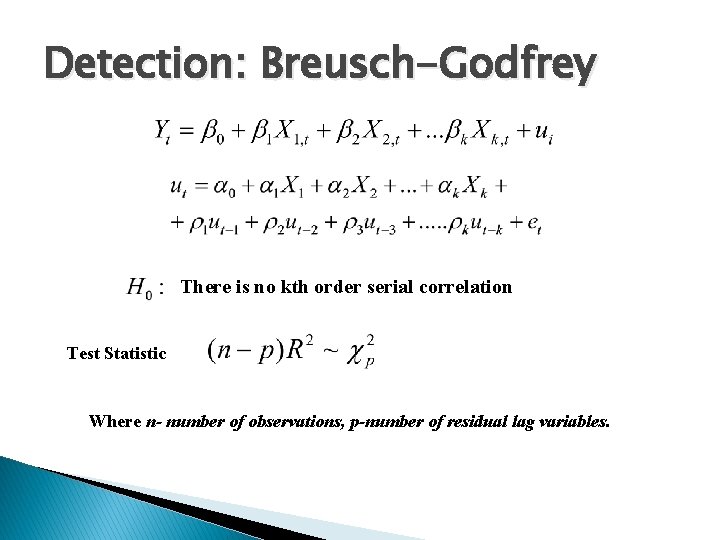
Detection: Breusch-Godfrey There is no kth order serial correlation Test Statistic Where n- number of observations, p-number of residual lag variables.
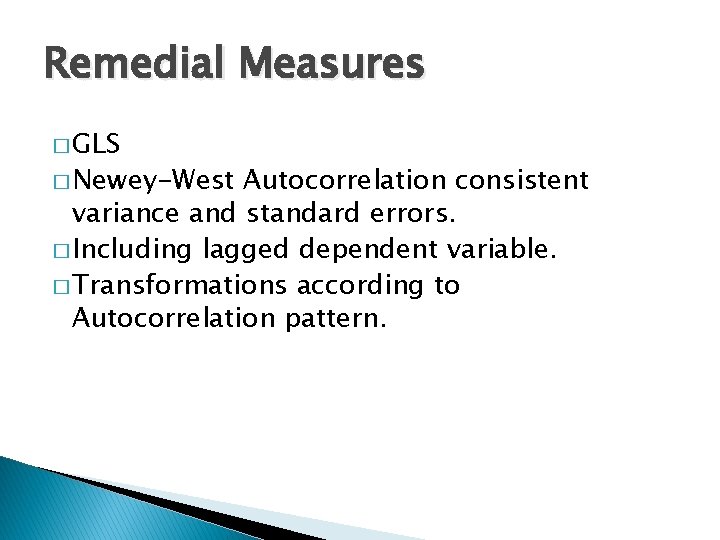
Remedial Measures � GLS � Newey-West Autocorrelation consistent variance and standard errors. � Including lagged dependent variable. � Transformations according to Autocorrelation pattern.
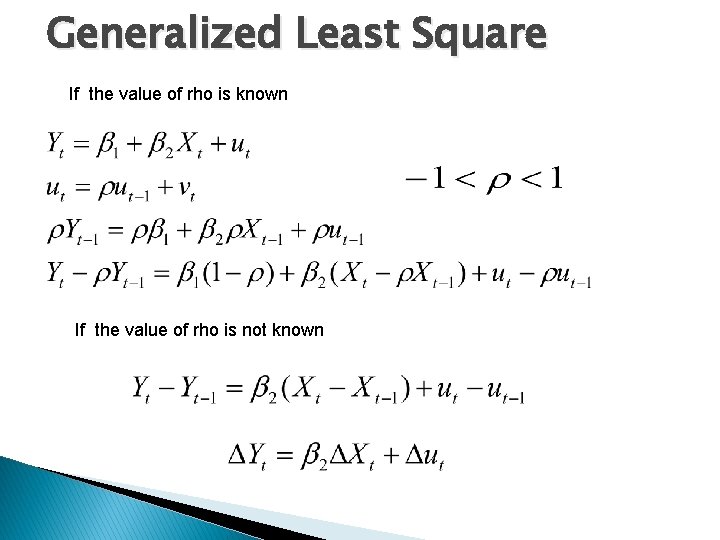
Generalized Least Square If the value of rho is known If the value of rho is not known
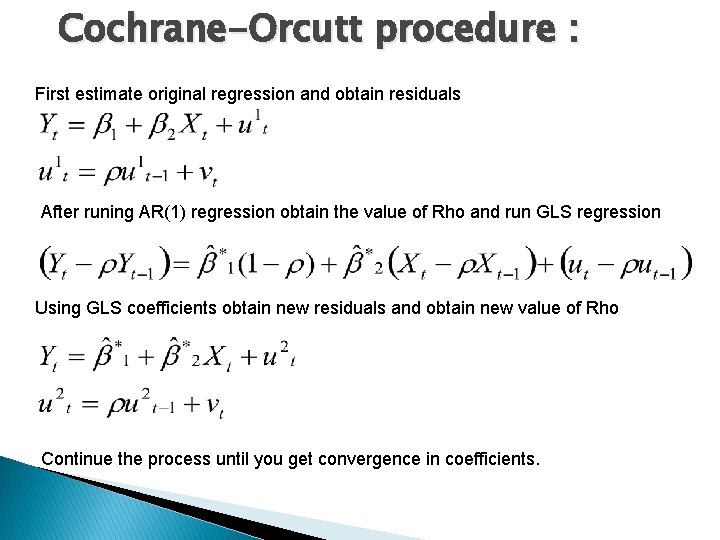
Cochrane-Orcutt procedure : First estimate original regression and obtain residuals After runing AR(1) regression obtain the value of Rho and run GLS regression Using GLS coefficients obtain new residuals and obtain new value of Rho Continue the process until you get convergence in coefficients.
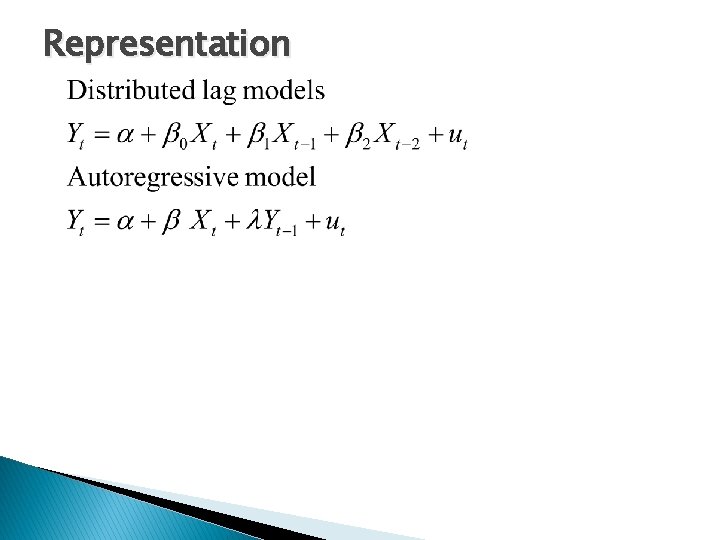
Representation
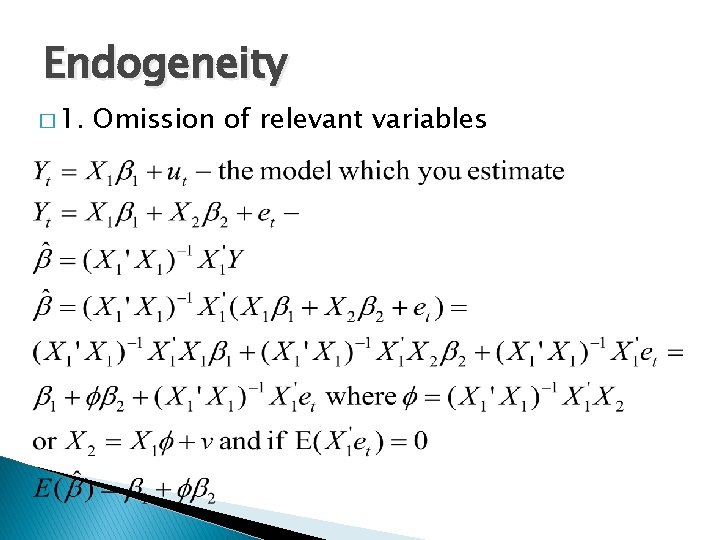
Endogeneity � 1. Omission of relevant variables
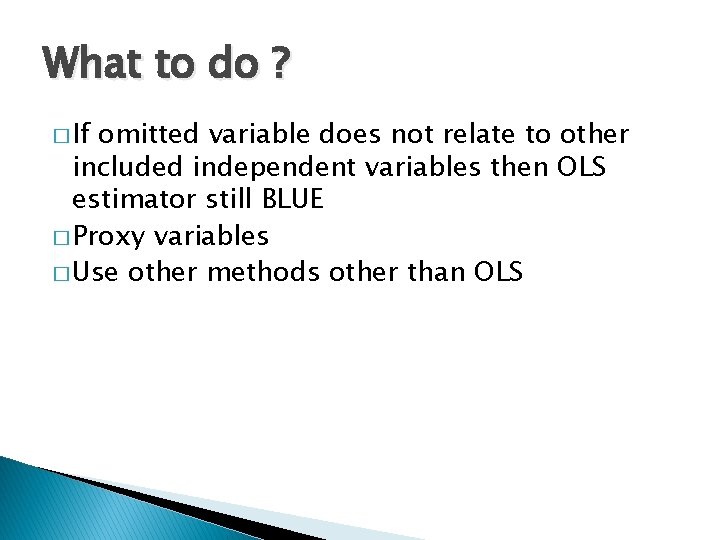
What to do ? � If omitted variable does not relate to other included independent variables then OLS estimator still BLUE � Proxy variables � Use other methods other than OLS
Irina romanov
Flacs checkpoint b spanish exam june 2017 answers
January 2012 chemistry regents answers
Good morning welcome june
French music awards
Dr june james
When your child leaves home poem
Tsc timetable
Mai june july august
June 22 to july 22
June's journey
Summary period: june 2021 poem
June canavan foundation
2019 june calendar
Companies in june
Welcome june blessings
June preschool newsletter
Flax exam
June too soon july stand by
New irpwm june 2020
June holley
June 21 2019 geometry regents answers
Lenore hetrick image
January 2012 chemistry regents answers
Good morning 1 june
June 6 1944
Good morning please
Battle of midway june 1942
Market practice definition
1 june children's day
June 2021 english language paper 1
June 23
June safety tips