Gait recognition under nonstandard circumstances Kjetil Holien Disposition
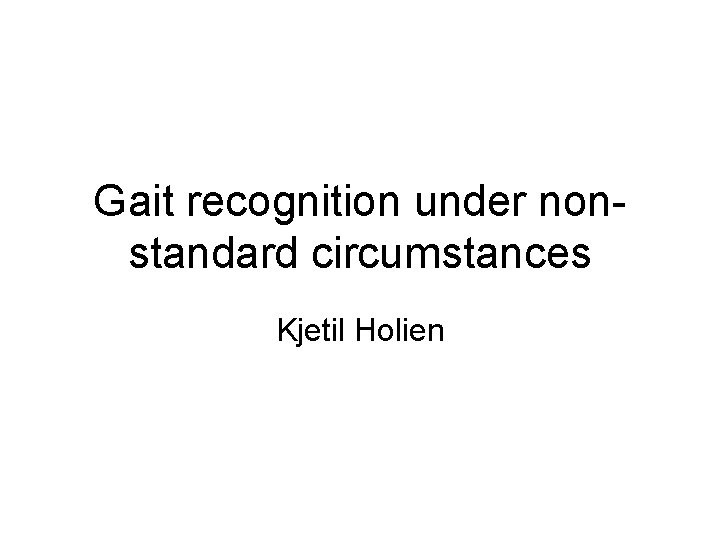
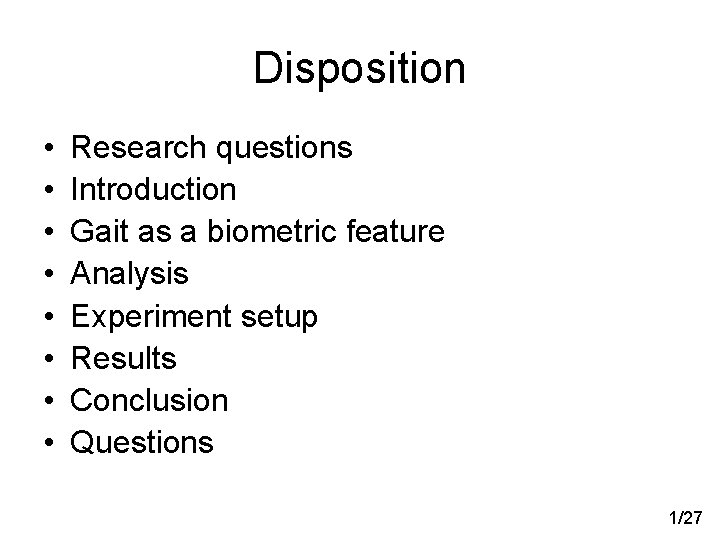
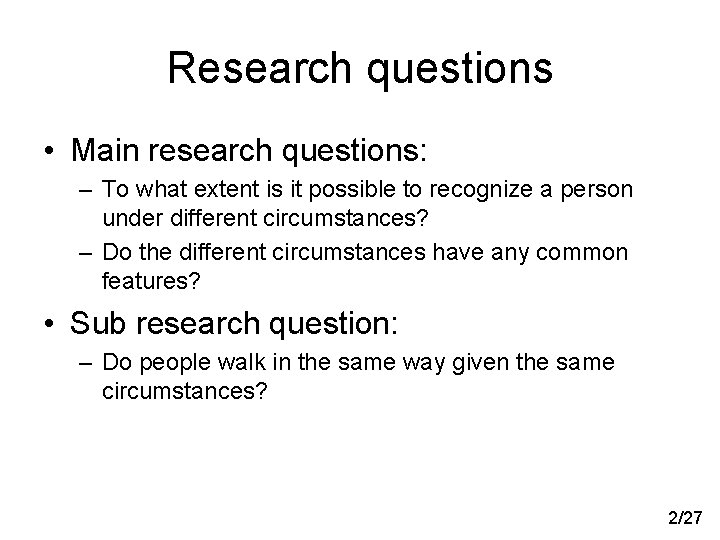
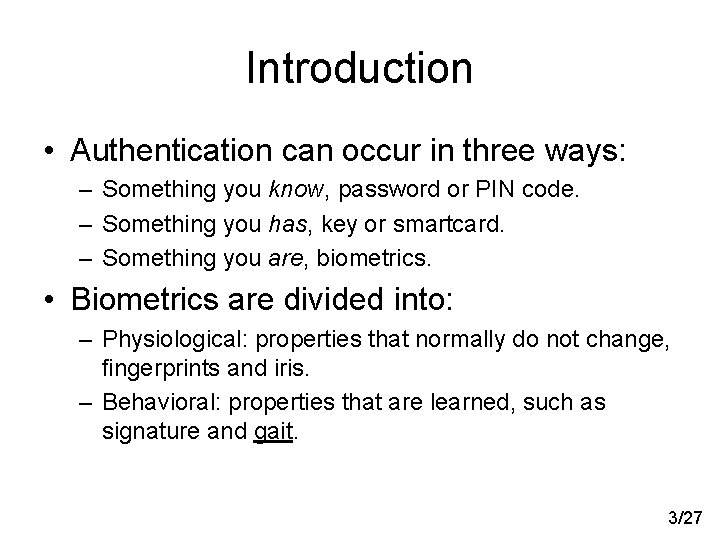
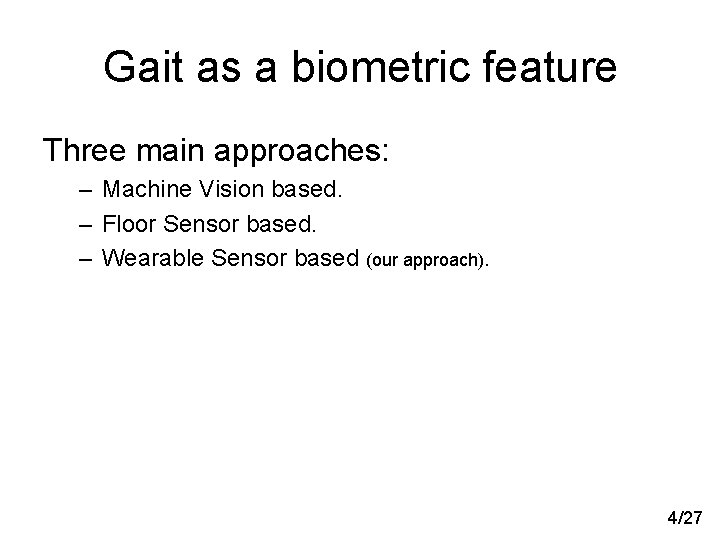
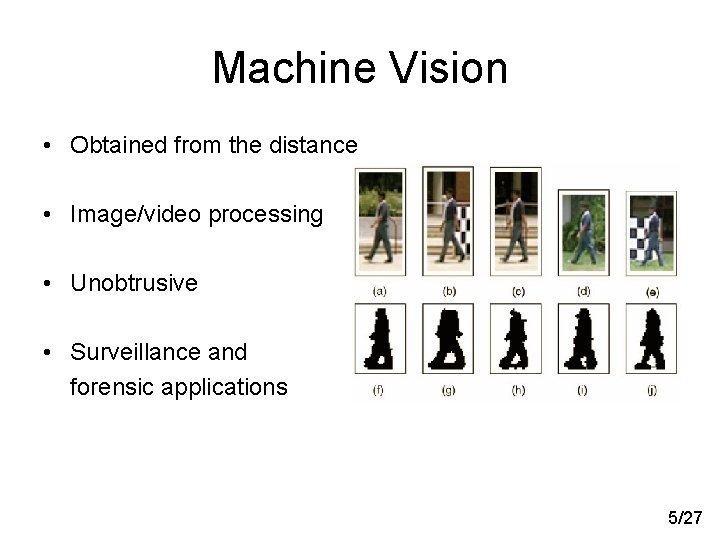
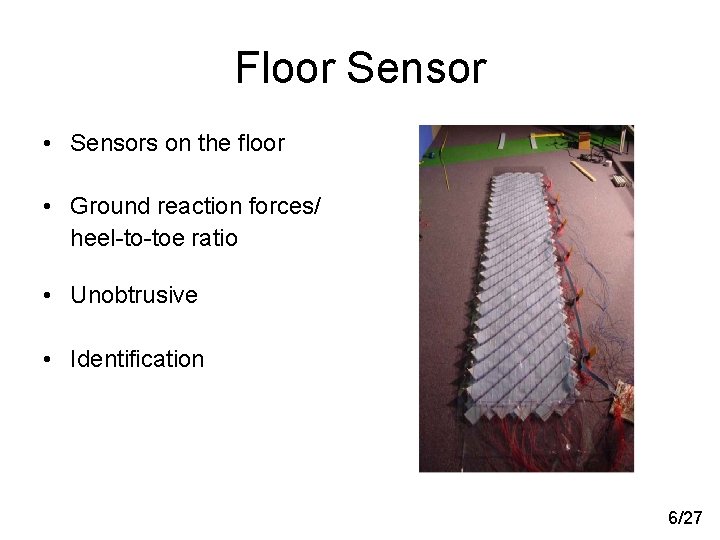
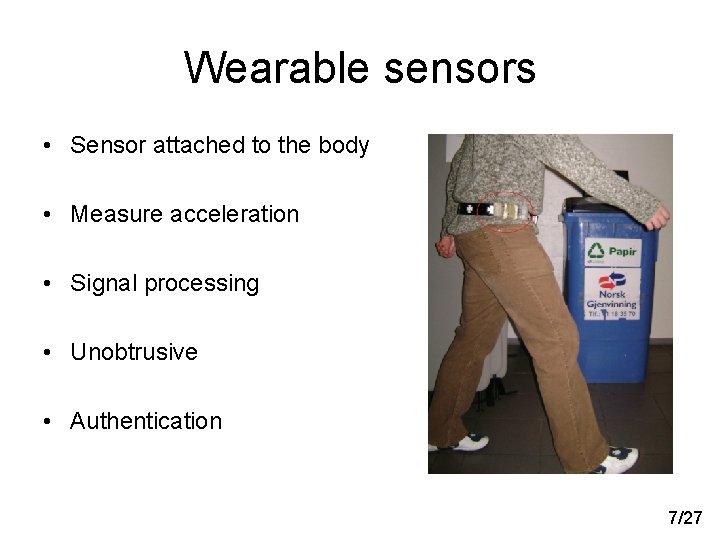
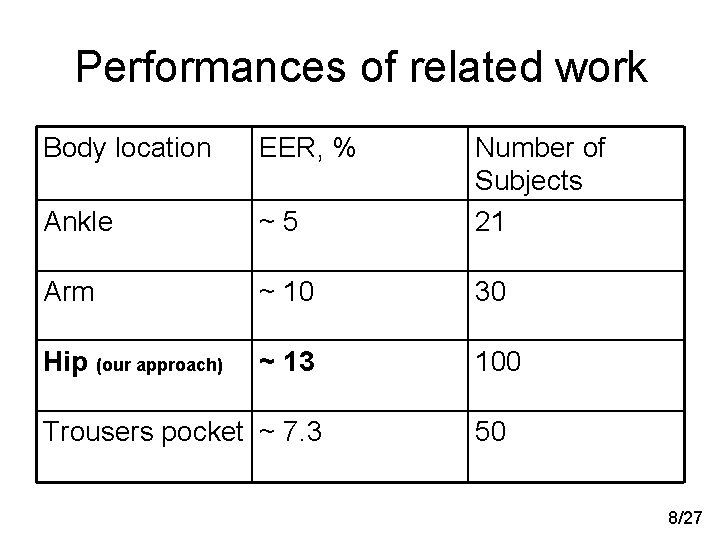
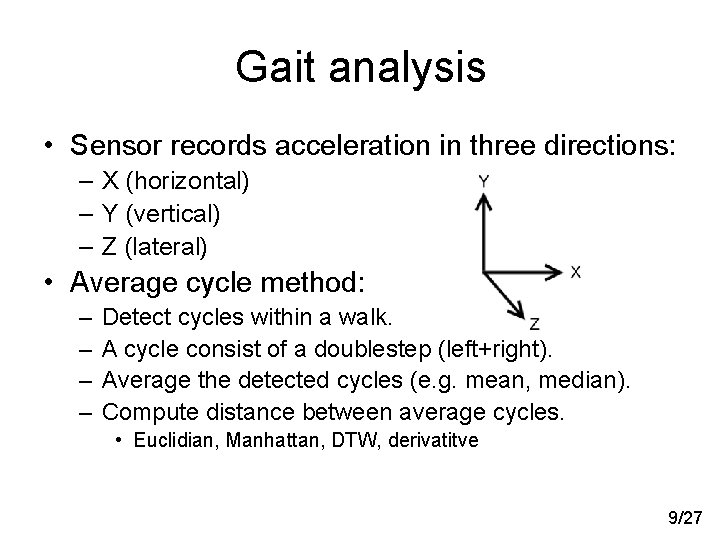
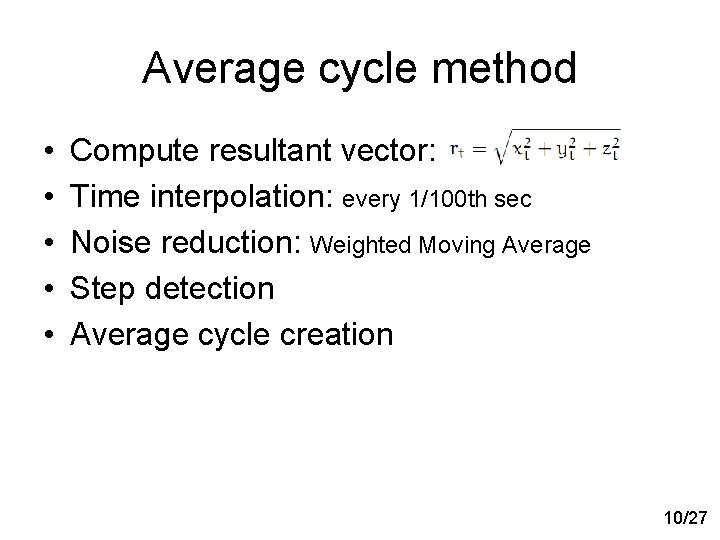
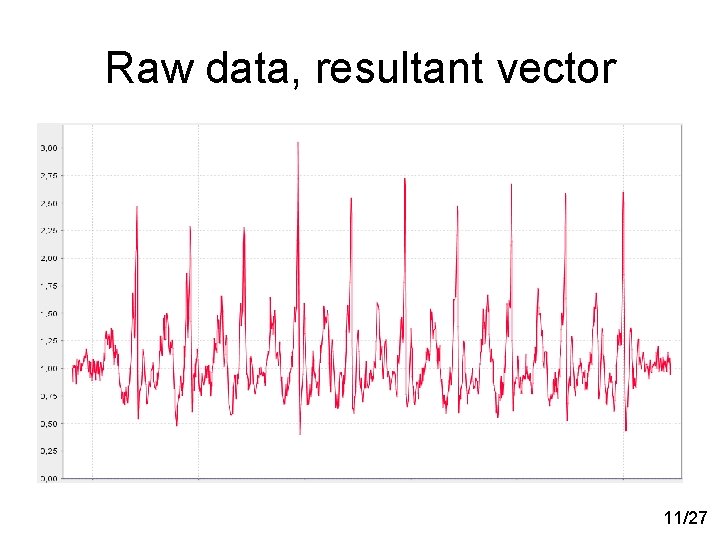
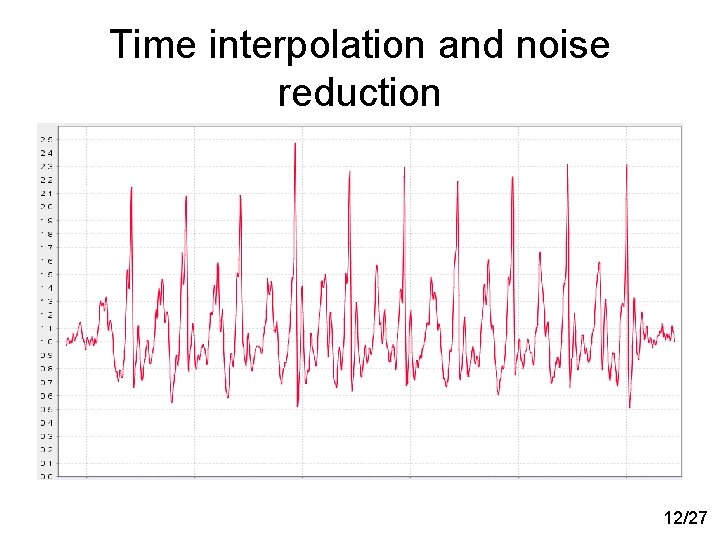
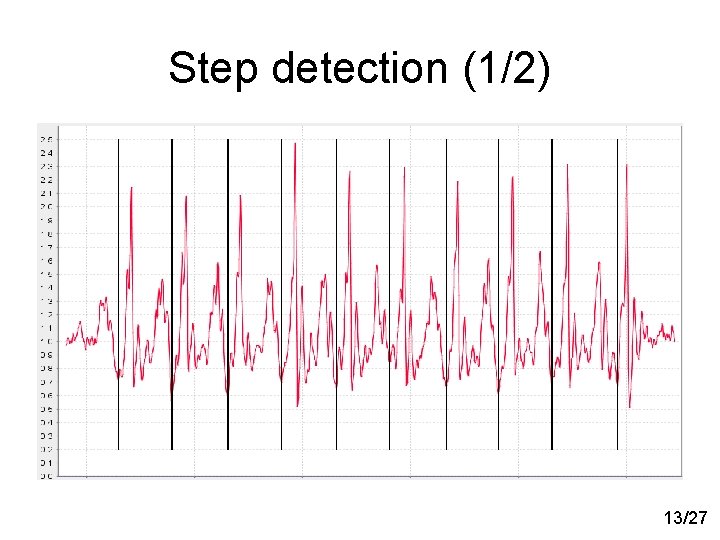
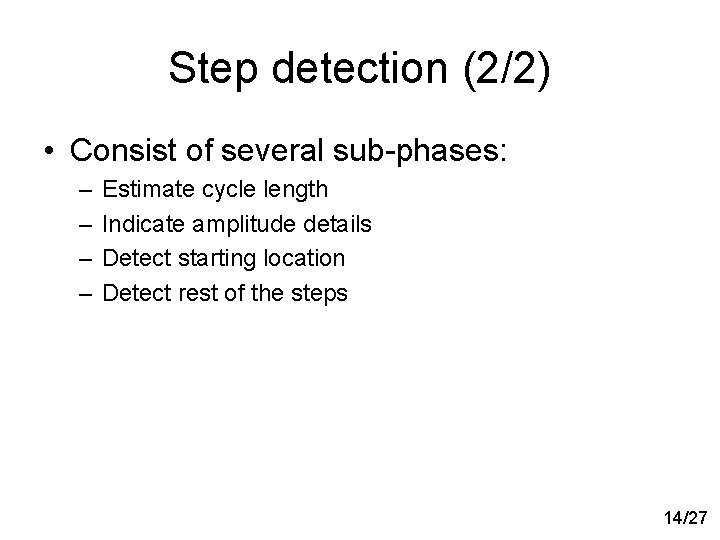
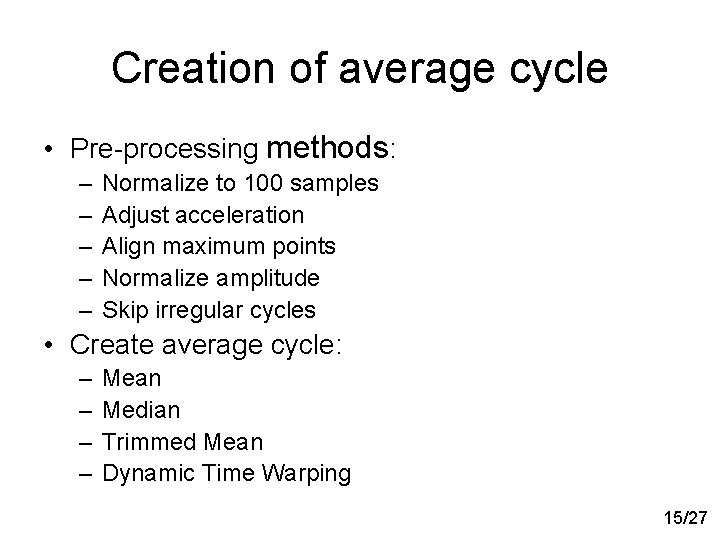
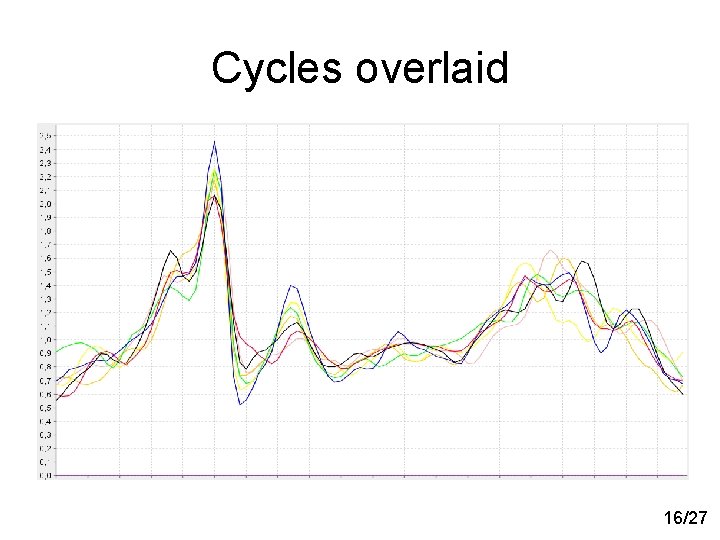
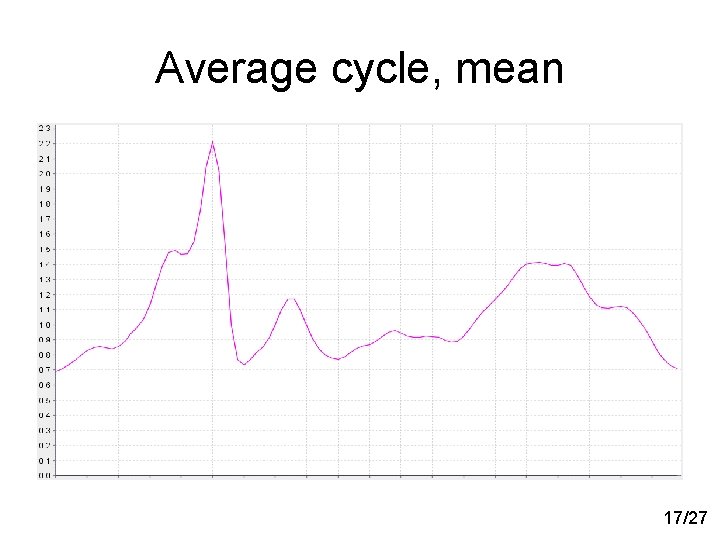
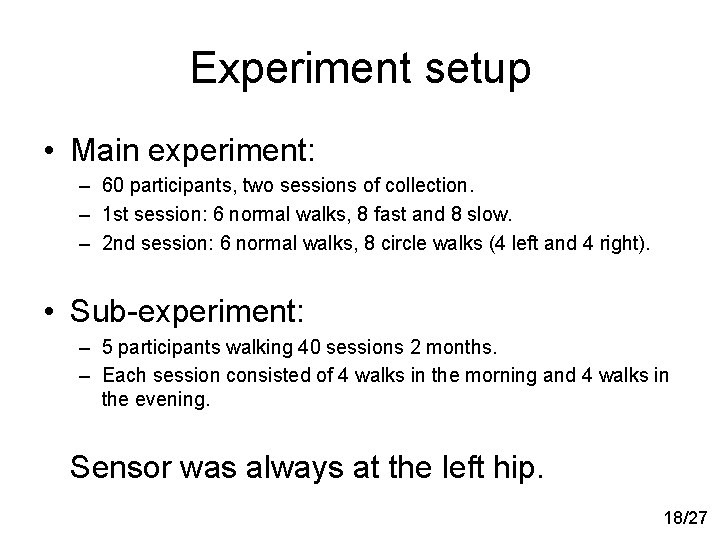
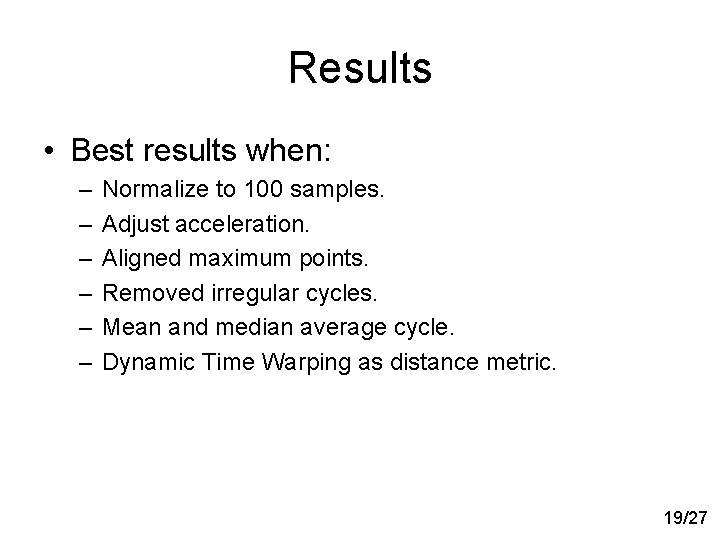
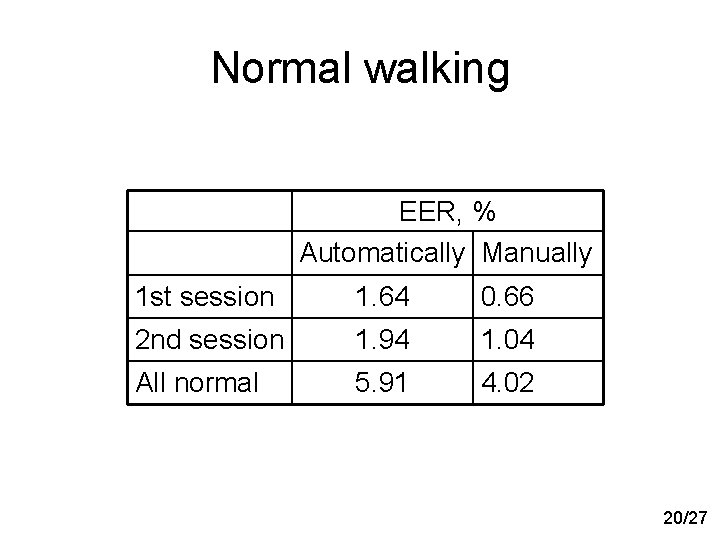
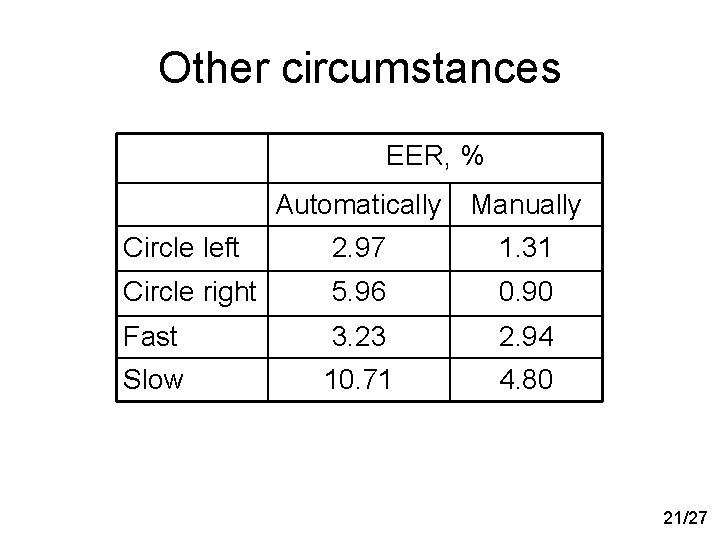
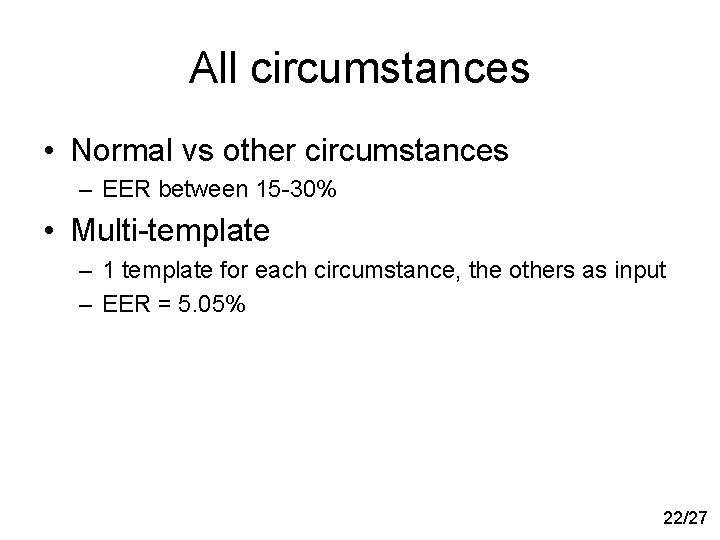
![Common features • Cycle length: – – Normal: [95. . 125], average of 109 Common features • Cycle length: – – Normal: [95. . 125], average of 109](https://slidetodoc.com/presentation_image/4ce56e25f0204bccd0f1d209d41bb379/image-24.jpg)
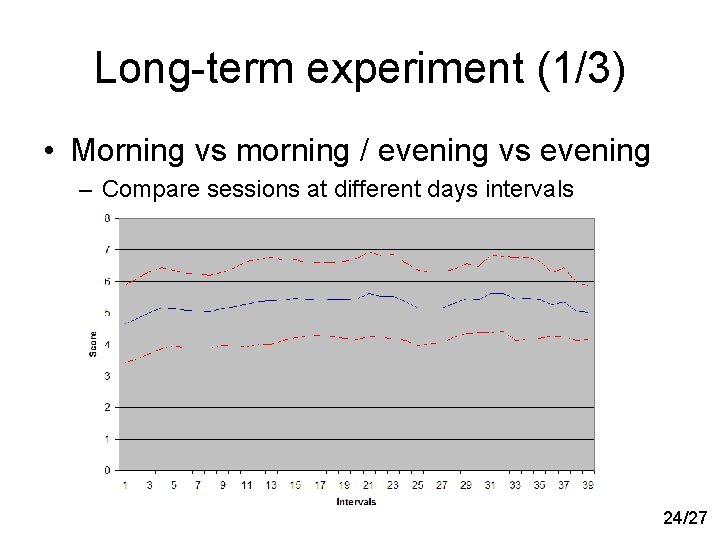
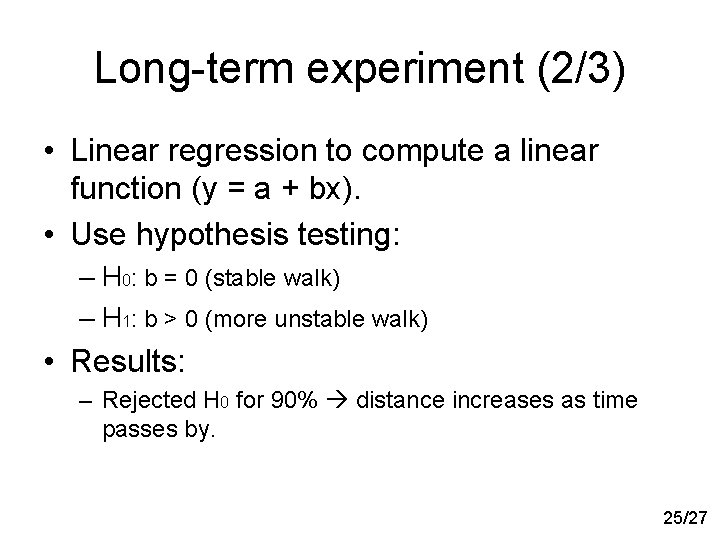
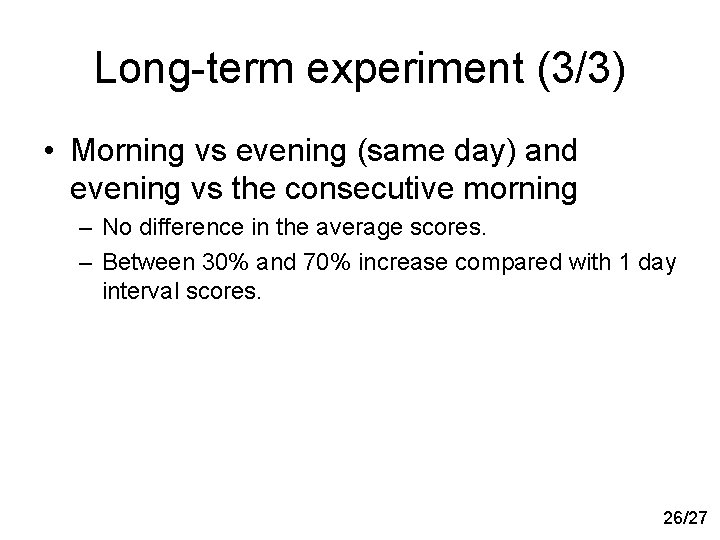
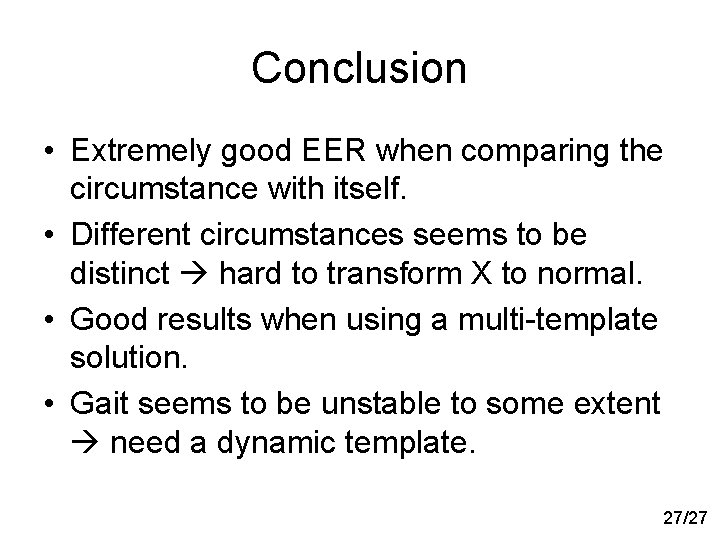
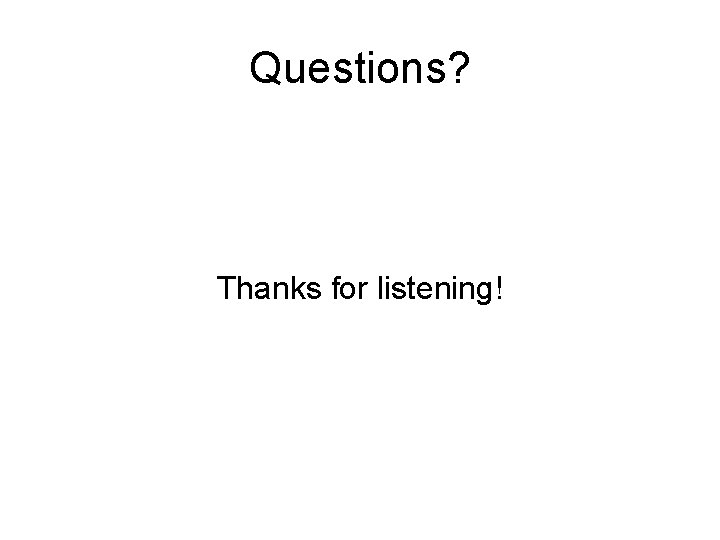
- Slides: 29
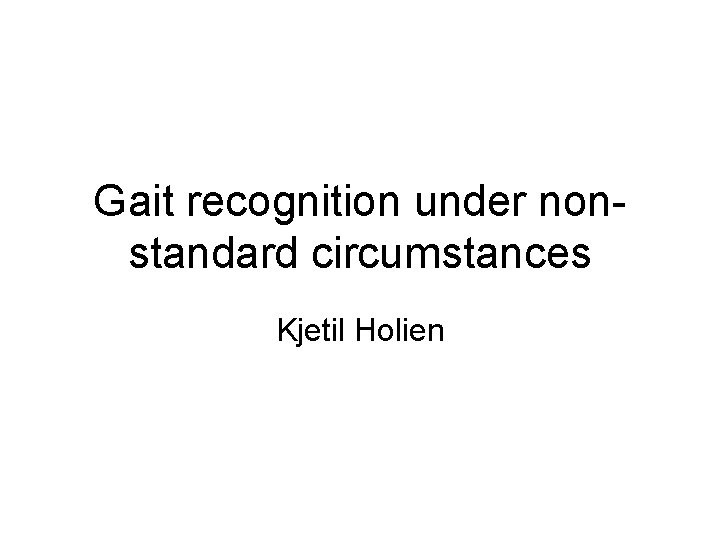
Gait recognition under nonstandard circumstances Kjetil Holien
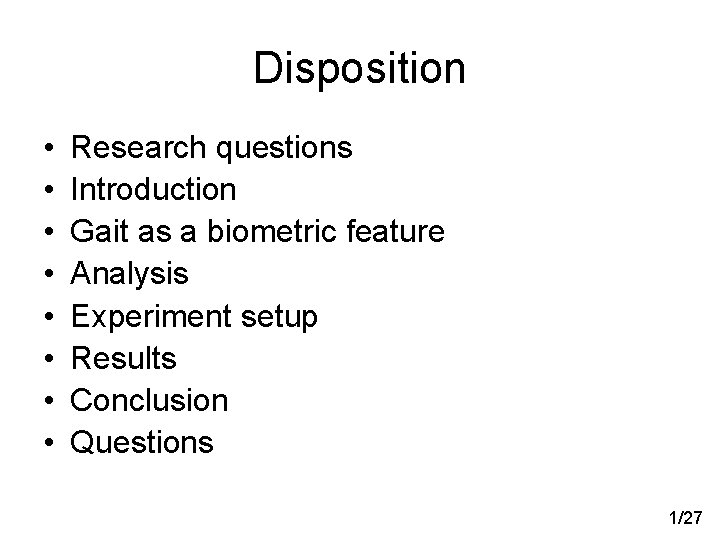
Disposition • • Research questions Introduction Gait as a biometric feature Analysis Experiment setup Results Conclusion Questions 1/27
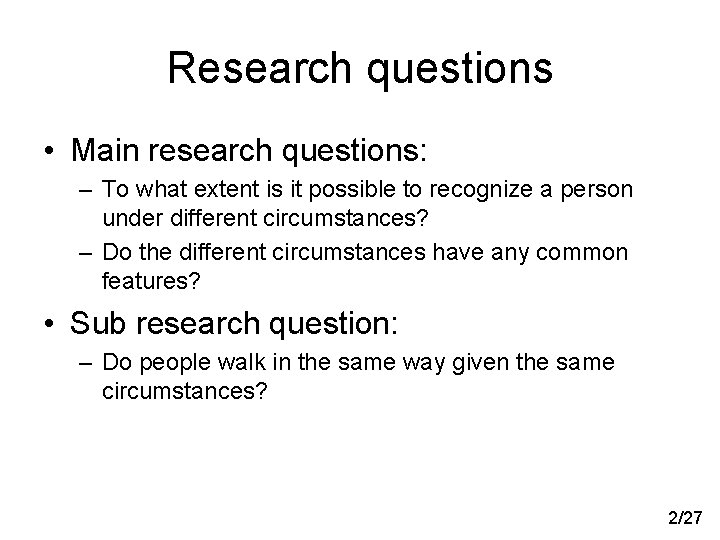
Research questions • Main research questions: – To what extent is it possible to recognize a person under different circumstances? – Do the different circumstances have any common features? • Sub research question: – Do people walk in the same way given the same circumstances? 2/27
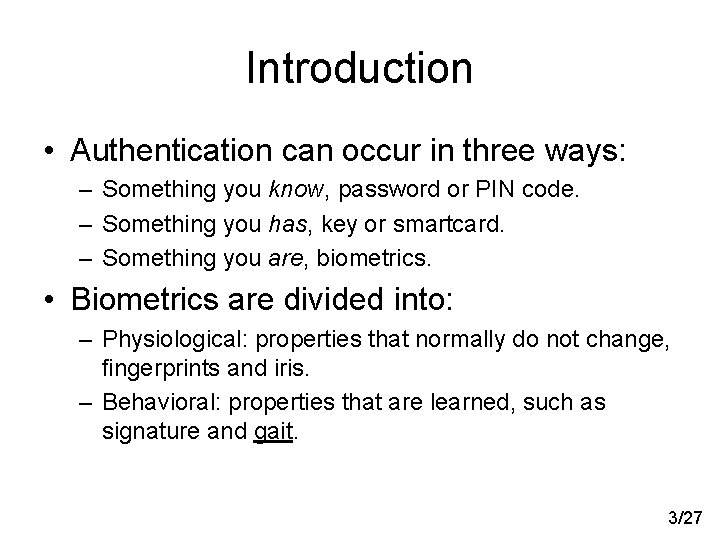
Introduction • Authentication can occur in three ways: – Something you know, password or PIN code. – Something you has, key or smartcard. – Something you are, biometrics. • Biometrics are divided into: – Physiological: properties that normally do not change, fingerprints and iris. – Behavioral: properties that are learned, such as signature and gait. 3/27
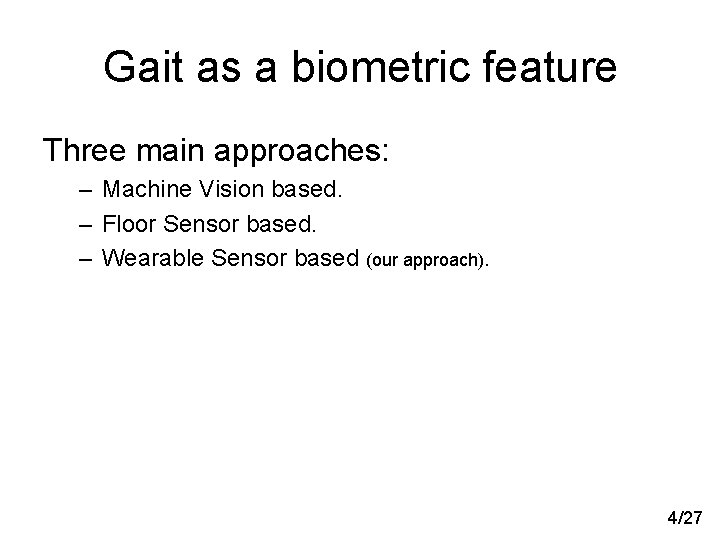
Gait as a biometric feature Three main approaches: – Machine Vision based. – Floor Sensor based. – Wearable Sensor based (our approach). 4/27
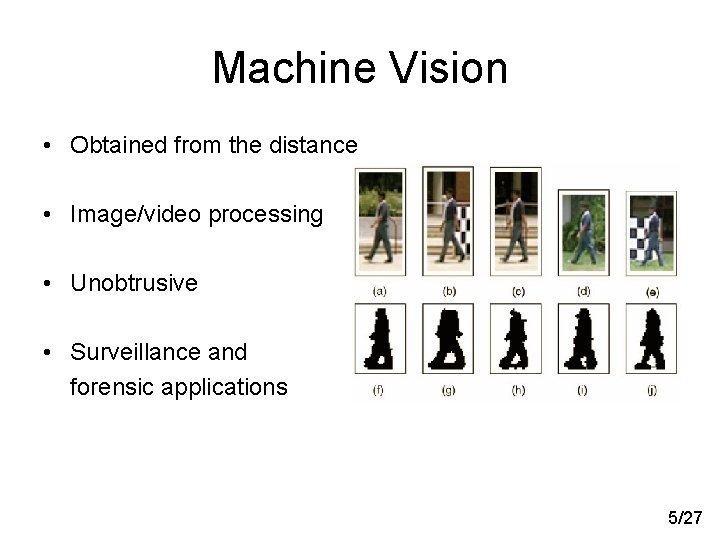
Machine Vision • Obtained from the distance • Image/video processing • Unobtrusive • Surveillance and forensic applications 5/27
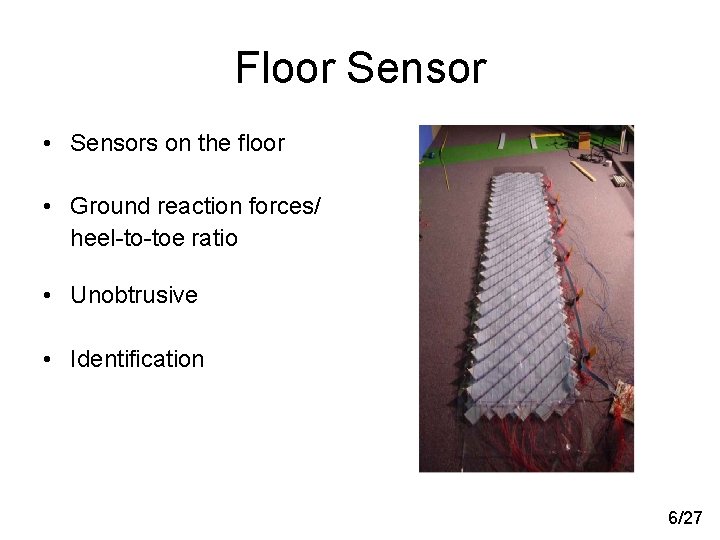
Floor Sensor • Sensors on the floor • Ground reaction forces/ heel-to-toe ratio • Unobtrusive • Identification 6/27
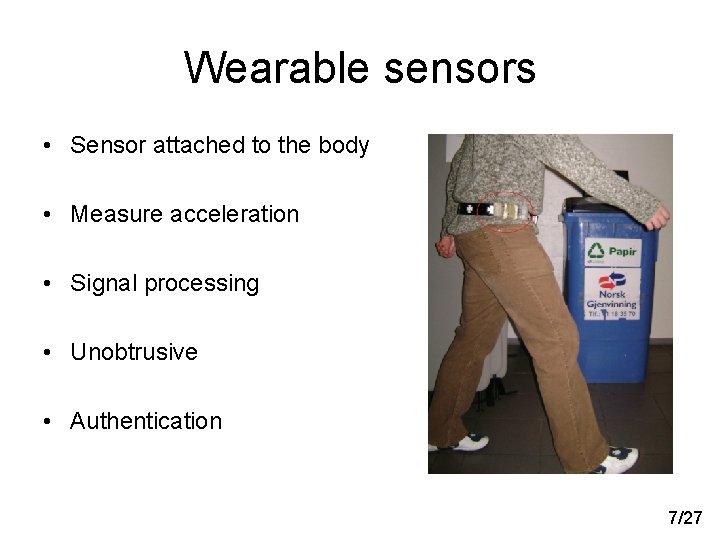
Wearable sensors • Sensor attached to the body • Measure acceleration • Signal processing • Unobtrusive • Authentication 7/27
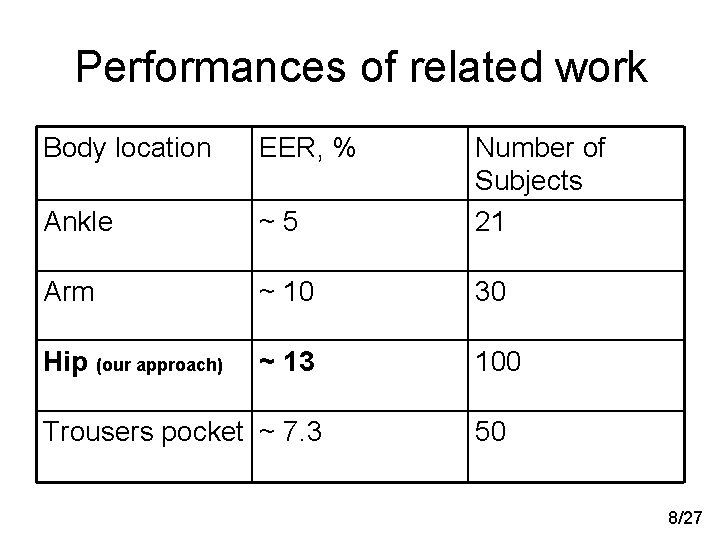
Performances of related work Body location EER, % Ankle ~5 Number of Subjects 21 Arm ~ 10 30 Hip (our approach) ~ 13 100 Trousers pocket ~ 7. 3 50 8/27
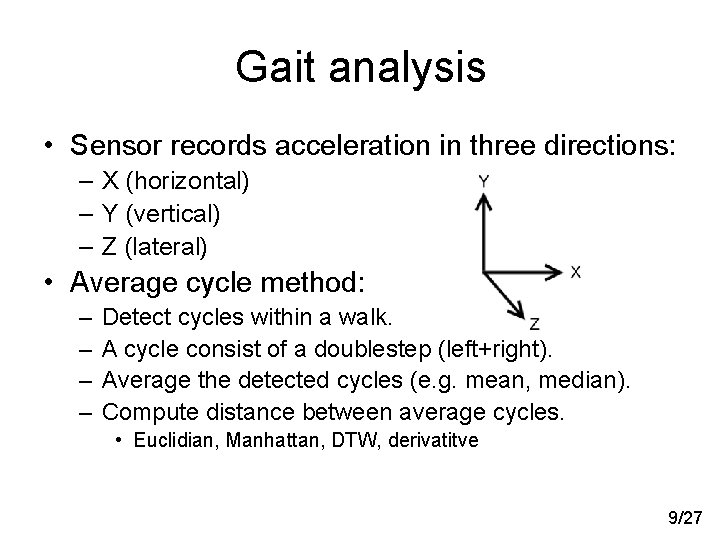
Gait analysis • Sensor records acceleration in three directions: – X (horizontal) – Y (vertical) – Z (lateral) • Average cycle method: – – Detect cycles within a walk. A cycle consist of a doublestep (left+right). Average the detected cycles (e. g. mean, median). Compute distance between average cycles. • Euclidian, Manhattan, DTW, derivatitve 9/27
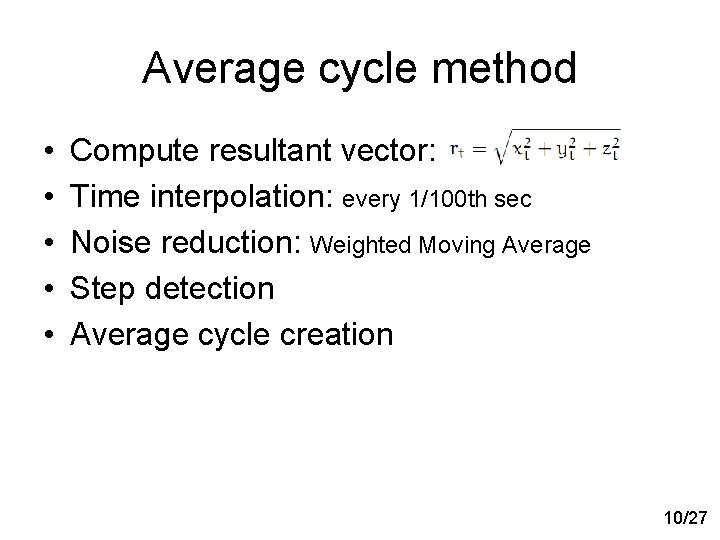
Average cycle method • • • Compute resultant vector: Time interpolation: every 1/100 th sec Noise reduction: Weighted Moving Average Step detection Average cycle creation 10/27
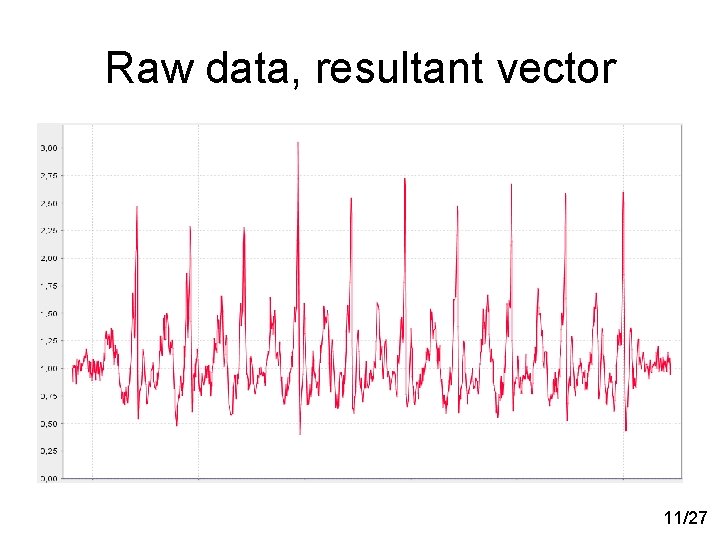
Raw data, resultant vector 11/27
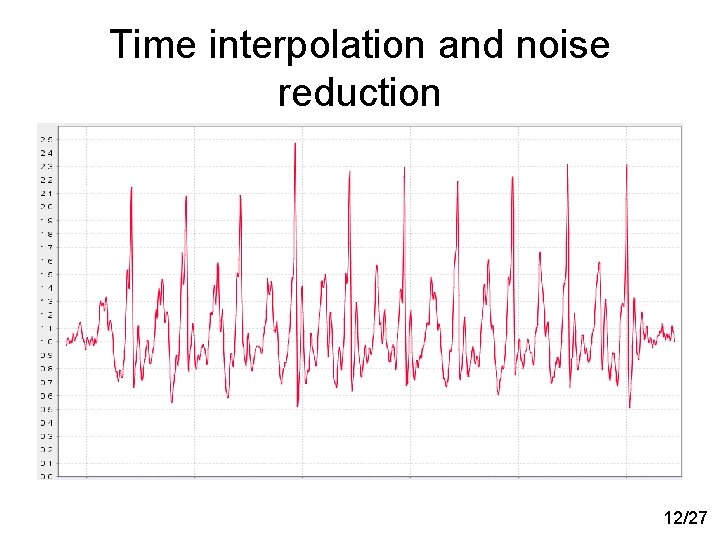
Time interpolation and noise reduction 12/27
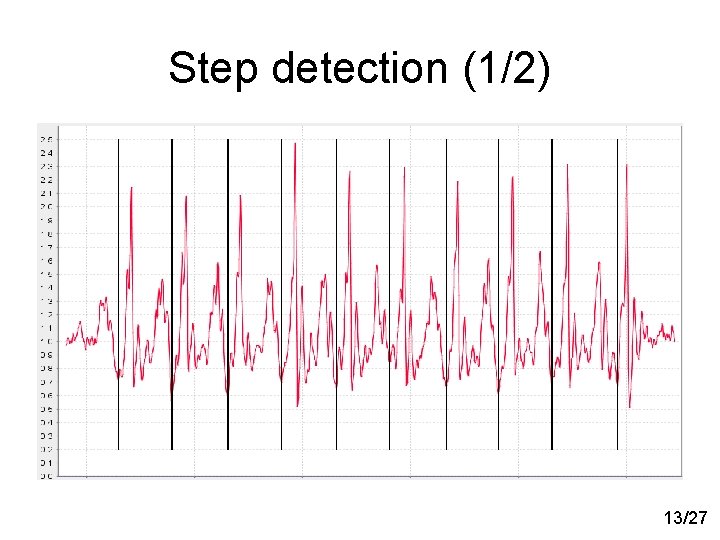
Step detection (1/2) 13/27
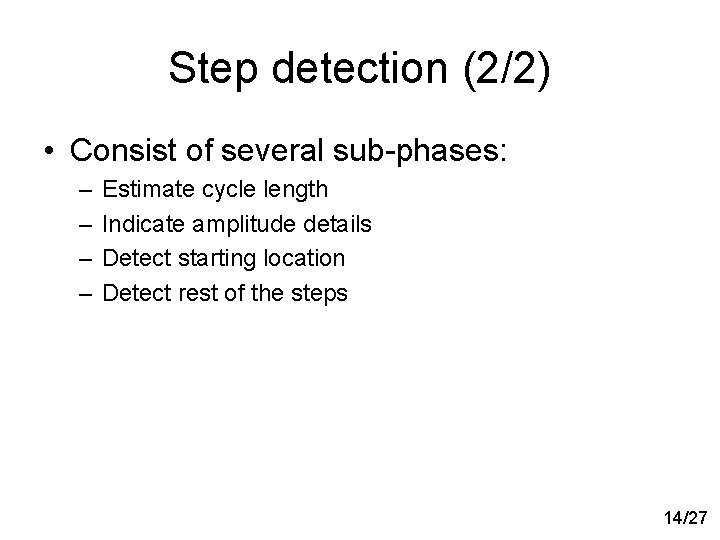
Step detection (2/2) • Consist of several sub-phases: – – Estimate cycle length Indicate amplitude details Detect starting location Detect rest of the steps 14/27
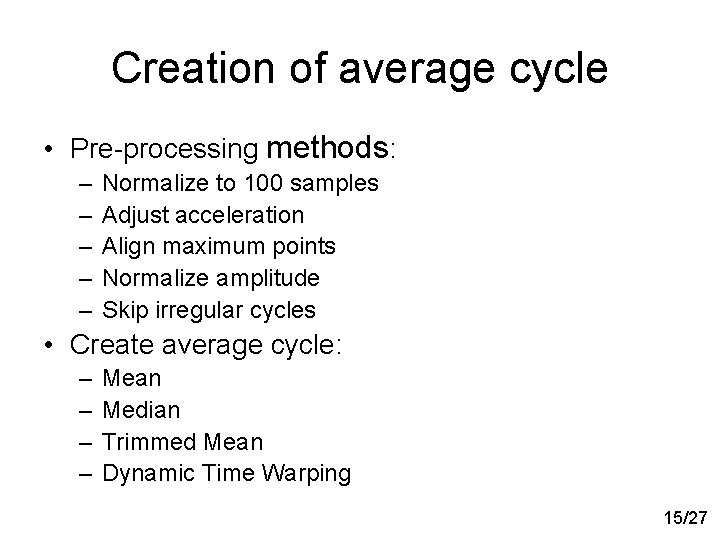
Creation of average cycle • Pre-processing methods: – – – Normalize to 100 samples Adjust acceleration Align maximum points Normalize amplitude Skip irregular cycles • Create average cycle: – – Mean Median Trimmed Mean Dynamic Time Warping 15/27
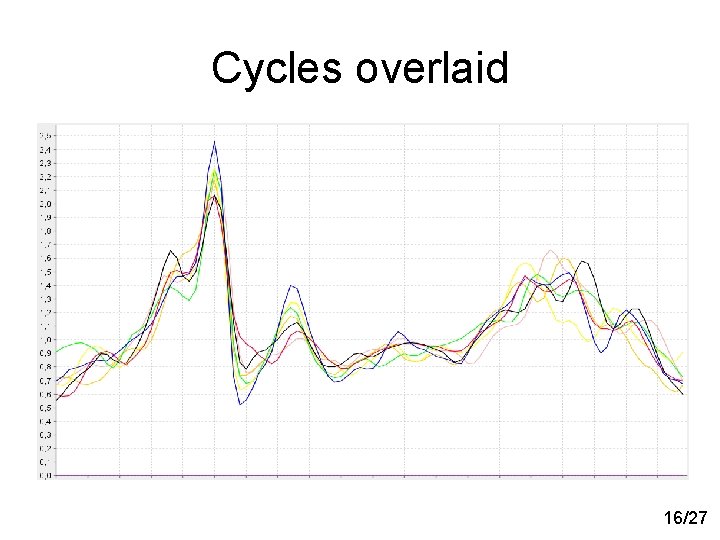
Cycles overlaid 16/27
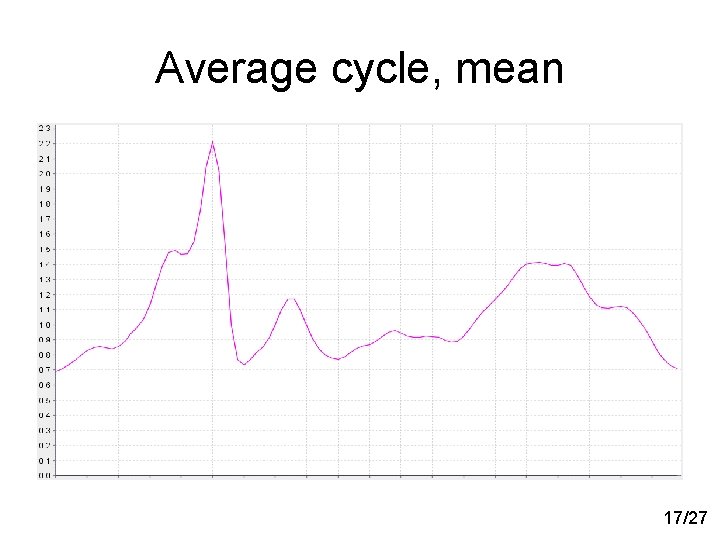
Average cycle, mean 17/27
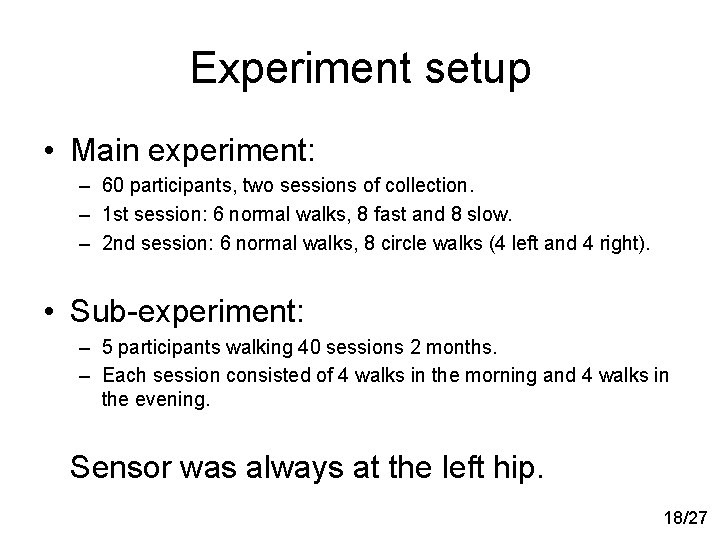
Experiment setup • Main experiment: – 60 participants, two sessions of collection. – 1 st session: 6 normal walks, 8 fast and 8 slow. – 2 nd session: 6 normal walks, 8 circle walks (4 left and 4 right). • Sub-experiment: – 5 participants walking 40 sessions 2 months. – Each session consisted of 4 walks in the morning and 4 walks in the evening. Sensor was always at the left hip. 18/27
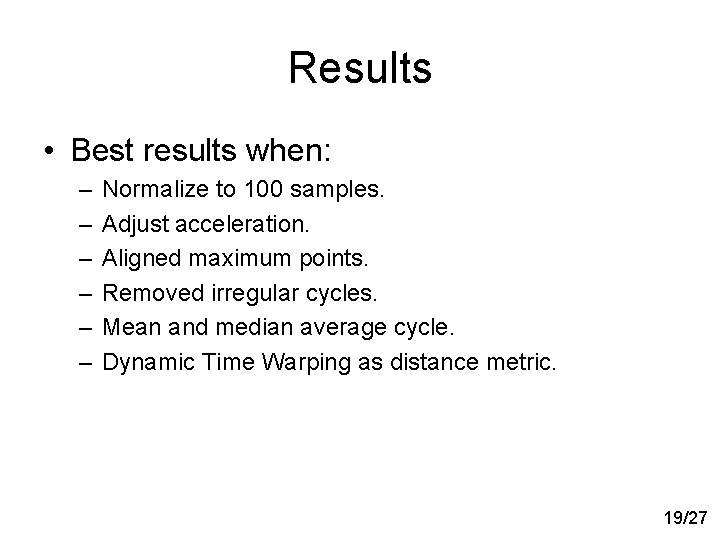
Results • Best results when: – – – Normalize to 100 samples. Adjust acceleration. Aligned maximum points. Removed irregular cycles. Mean and median average cycle. Dynamic Time Warping as distance metric. 19/27
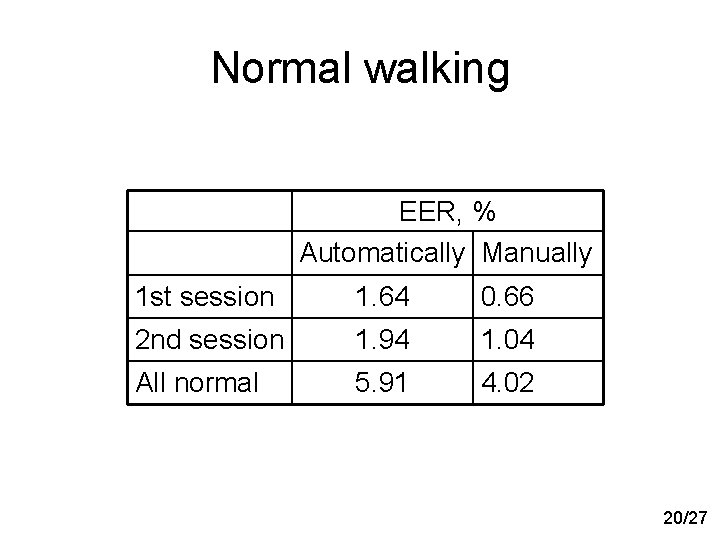
Normal walking EER, % Automatically Manually 1 st session 1. 64 0. 66 2 nd session 1. 94 1. 04 All normal 5. 91 4. 02 20/27
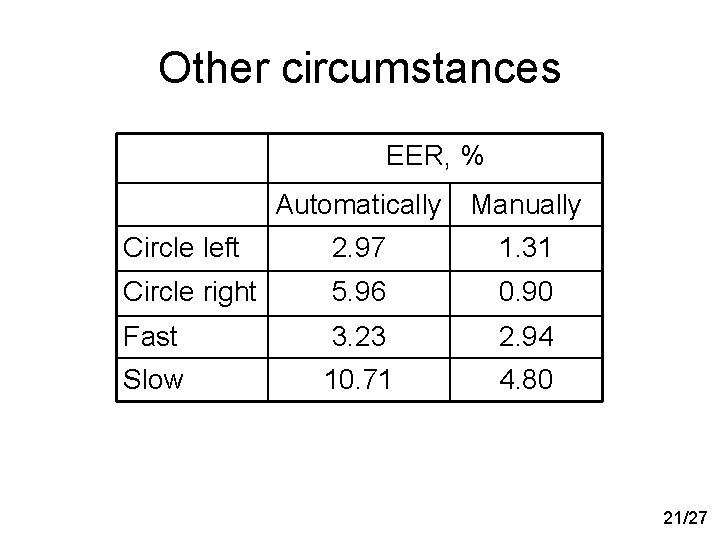
Other circumstances EER, % Automatically Manually Circle left 2. 97 1. 31 Circle right 5. 96 0. 90 Fast 3. 23 2. 94 Slow 10. 71 4. 80 21/27
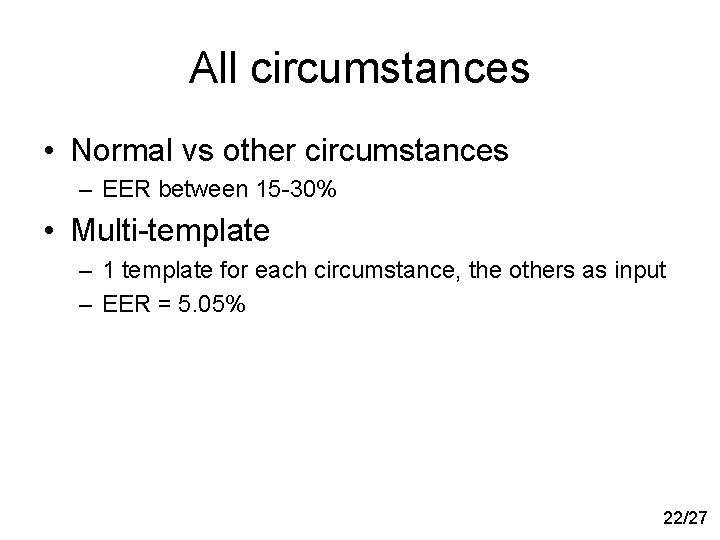
All circumstances • Normal vs other circumstances – EER between 15 -30% • Multi-template – 1 template for each circumstance, the others as input – EER = 5. 05% 22/27
![Common features Cycle length Normal 95 125 average of 109 Common features • Cycle length: – – Normal: [95. . 125], average of 109](https://slidetodoc.com/presentation_image/4ce56e25f0204bccd0f1d209d41bb379/image-24.jpg)
Common features • Cycle length: – – Normal: [95. . 125], average of 109 samples Fast: [80. . 110], average of 96 samples Slow: [110. . 180], average of 137 samples Circle same as normal • Amplitudes related to cycle length 23/27
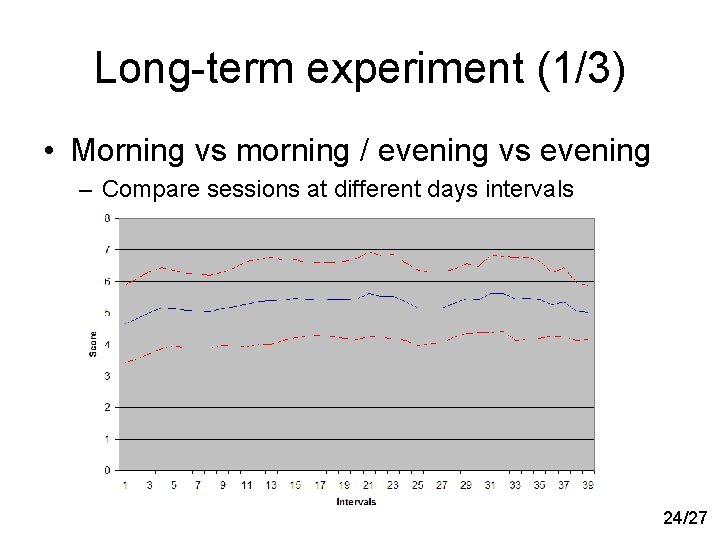
Long-term experiment (1/3) • Morning vs morning / evening vs evening – Compare sessions at different days intervals 24/27
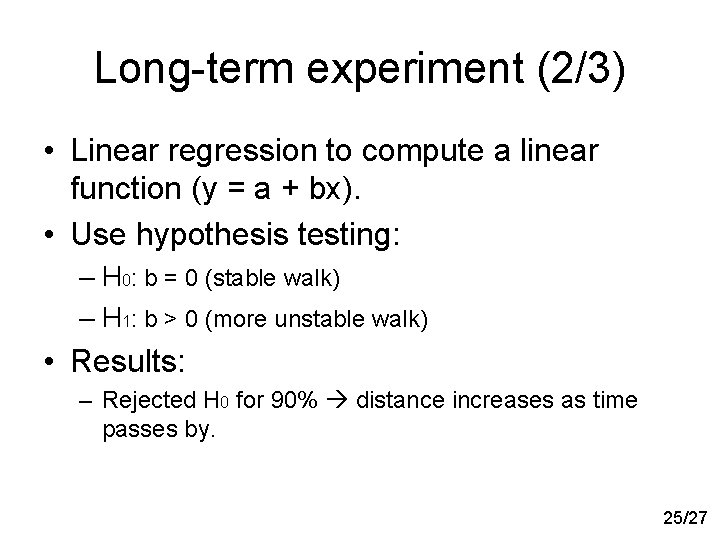
Long-term experiment (2/3) • Linear regression to compute a linear function (y = a + bx). • Use hypothesis testing: – H 0: b = 0 (stable walk) – H 1: b > 0 (more unstable walk) • Results: – Rejected H 0 for 90% distance increases as time passes by. 25/27
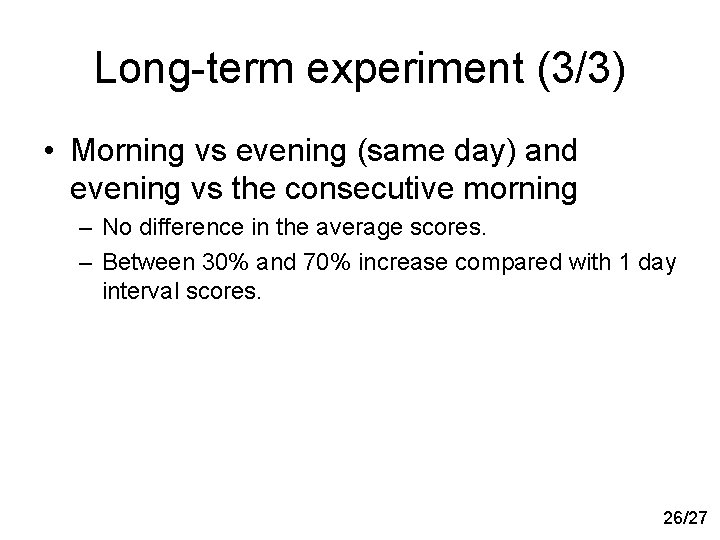
Long-term experiment (3/3) • Morning vs evening (same day) and evening vs the consecutive morning – No difference in the average scores. – Between 30% and 70% increase compared with 1 day interval scores. 26/27
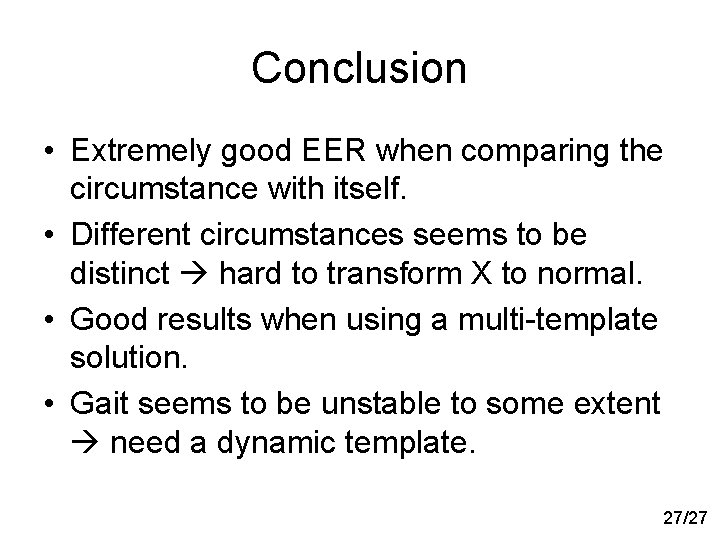
Conclusion • Extremely good EER when comparing the circumstance with itself. • Different circumstances seems to be distinct hard to transform X to normal. • Good results when using a multi-template solution. • Gait seems to be unstable to some extent need a dynamic template. 27/27
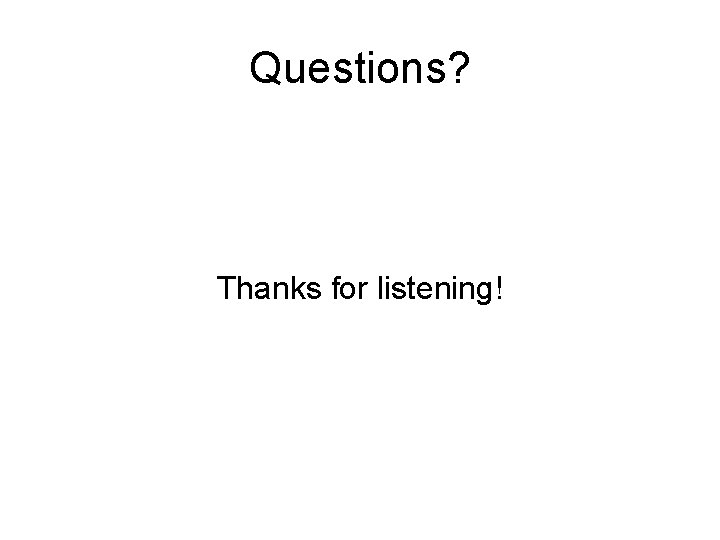
Questions? Thanks for listening!
How to find cell potential under nonstandard conditions
Non standard english
Kjetil hestad
Kjetil kausland
Kjetil gulsrud
Kjetil kjerstad
Kjetil mujezinovic larsen
Examples of special circumstances letter
He sends romeo into exile.
Participants processes and circumstances
Trusting god in all circumstances
Totality of the circumstances
Relevant circumstances adalah
Change in circumstances
Carroll doctrine
What three circumstances hurt native americans
Short text about pollution
Seas bonus points
Life is never made unbearable by circumstances reflection
Concatenation of circumstances
Deakin seas
Justifying circumstances
Face bnok
Temporal circumstance
Special circumstances instructional assistance assessment
Disposition model of decision making
What does being audience-centered involve
Romeo and juliet vocab
Mental disposition
Hvad er en disposition