FROM FUZZY TO INTUITIONISTIC FUZZY Vassia Atanassova Institute
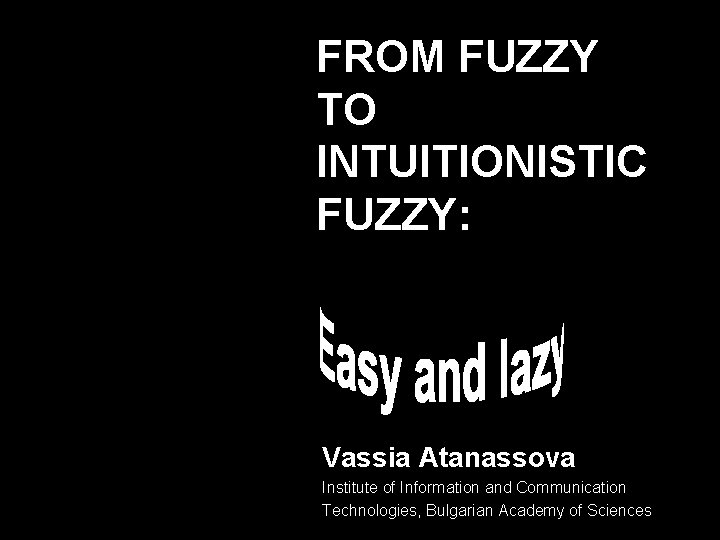
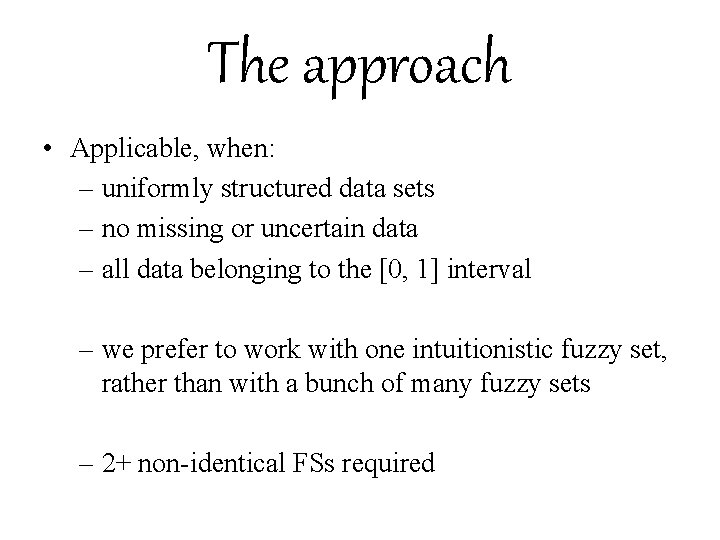
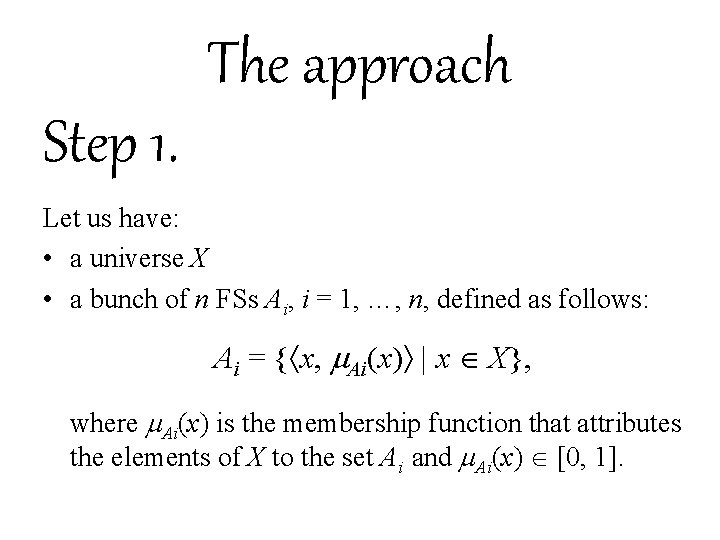
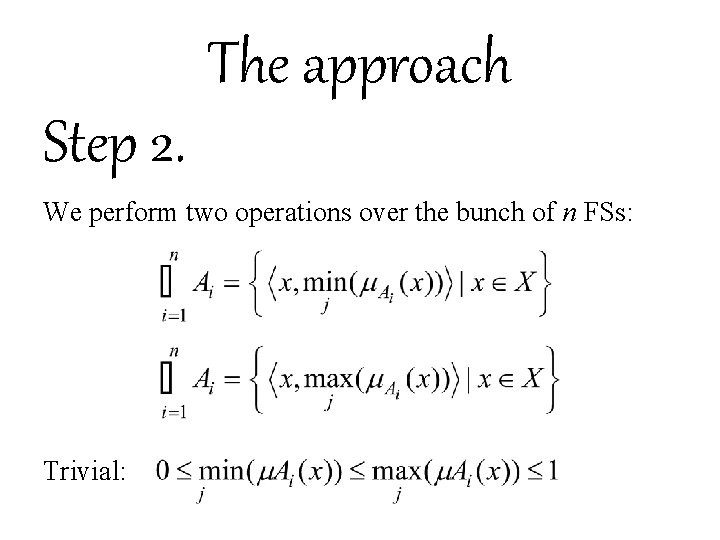
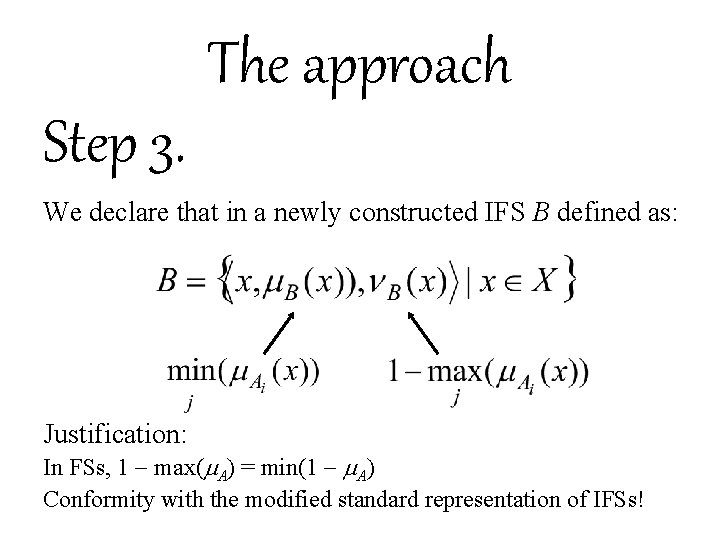
![Numerical example • Example: Average monthly temperatures normed to the [0, 1]-interval (0 = Numerical example • Example: Average monthly temperatures normed to the [0, 1]-interval (0 =](https://slidetodoc.com/presentation_image/7e38cb40305b1e3c53b56c2aa42a66ef/image-6.jpg)
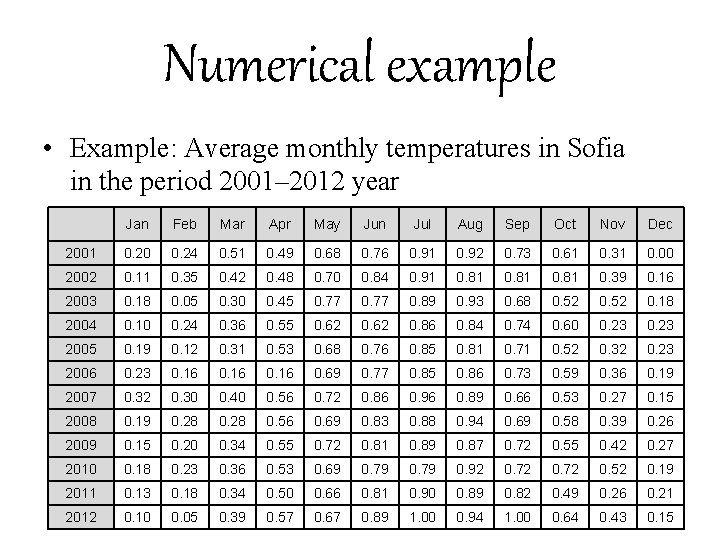
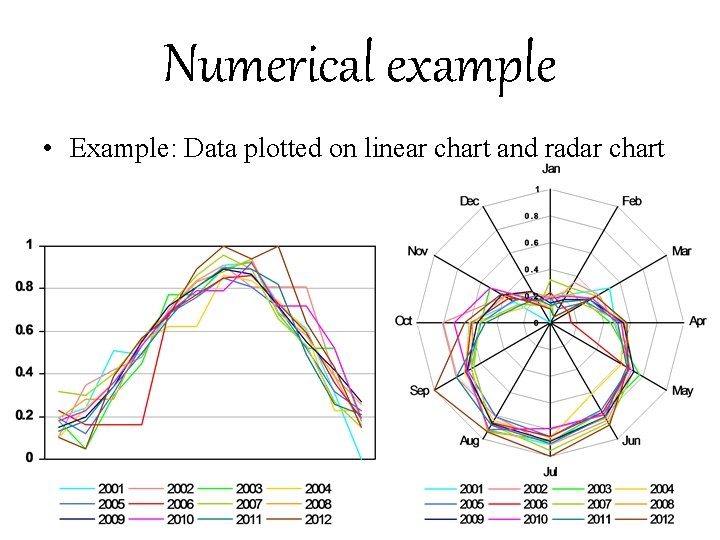
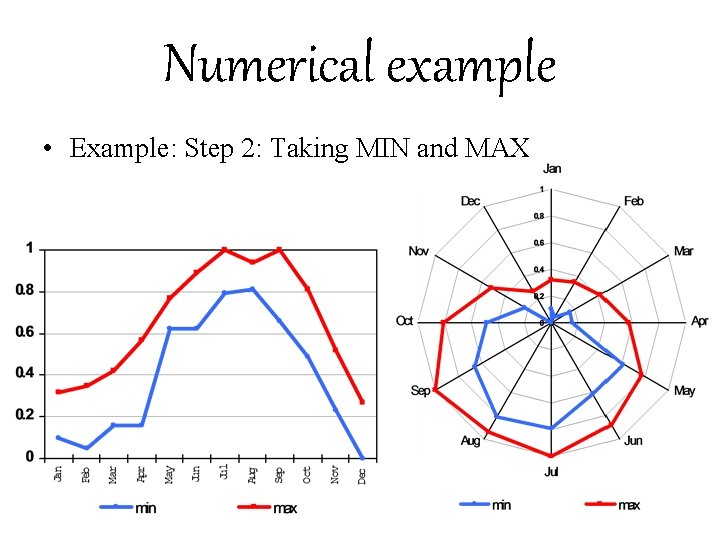
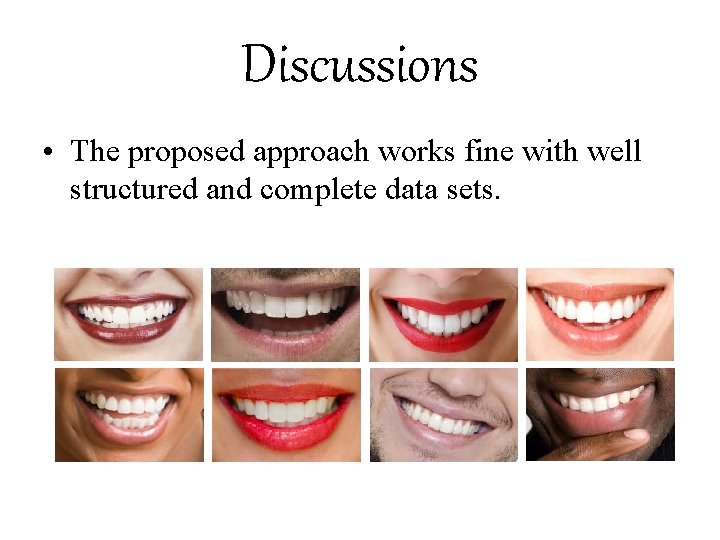
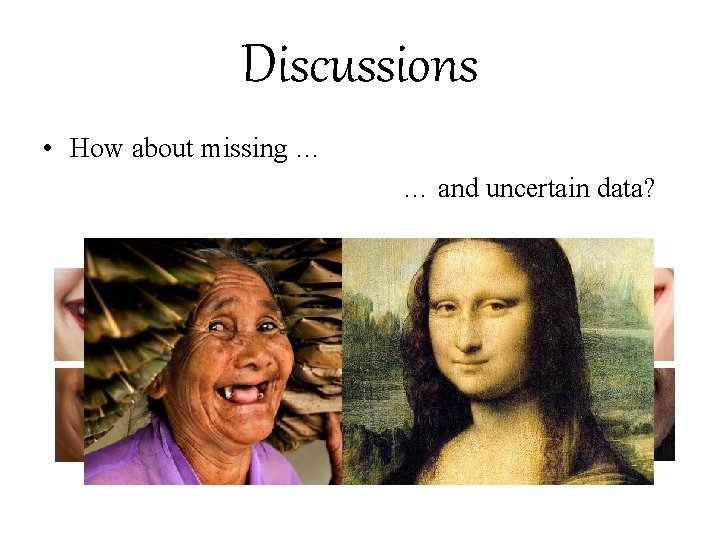
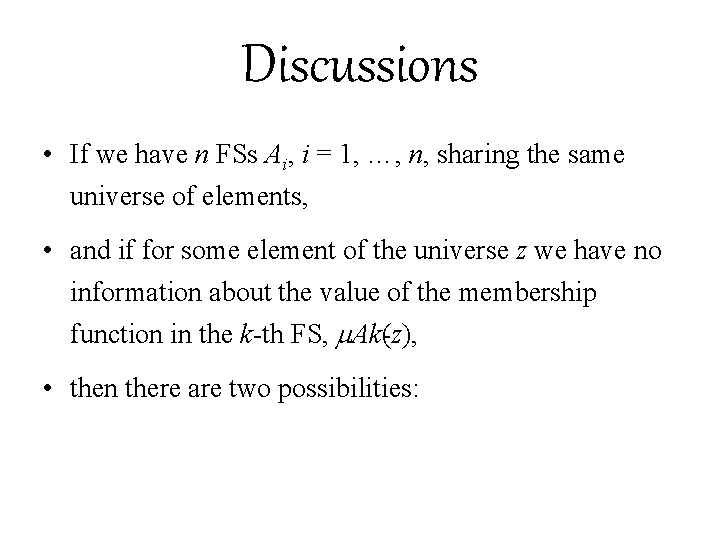
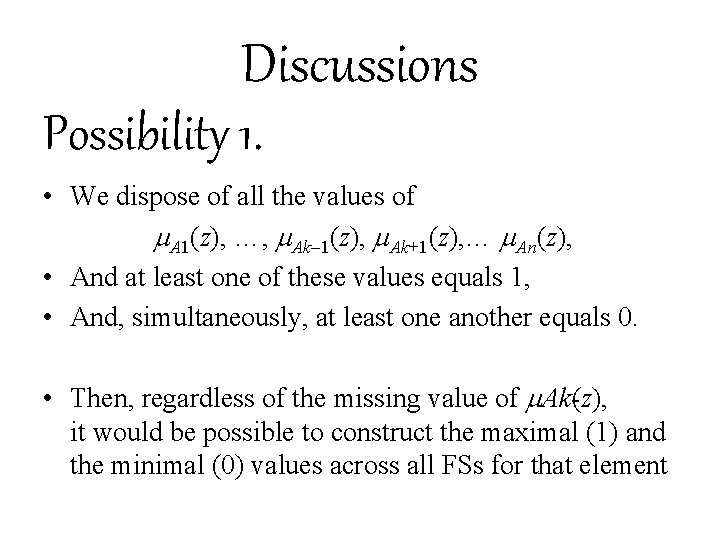
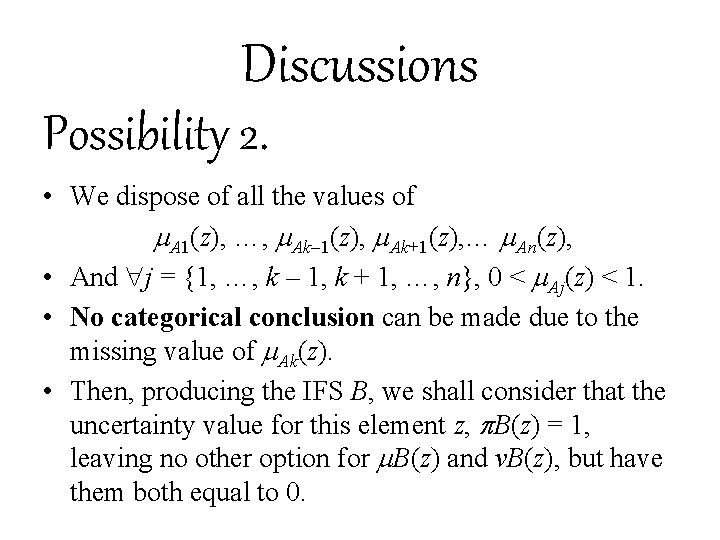
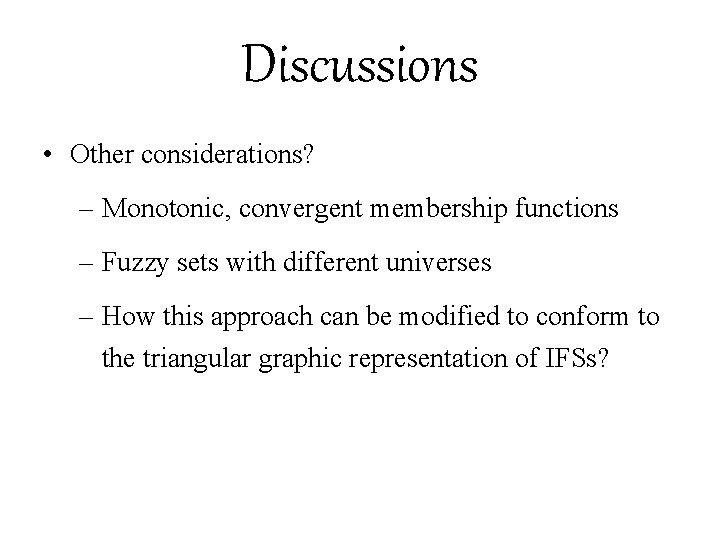
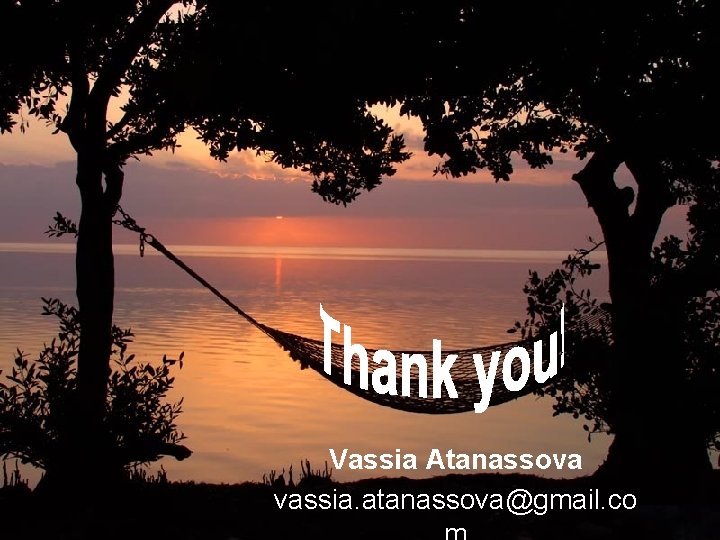
- Slides: 16
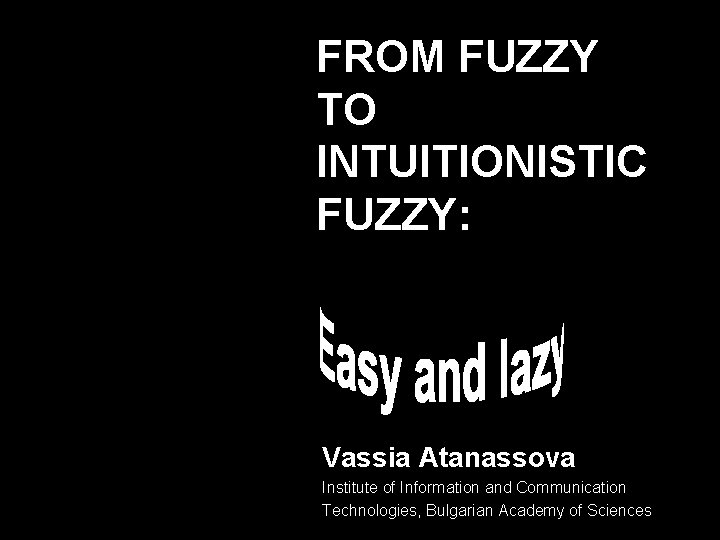
FROM FUZZY TO INTUITIONISTIC FUZZY: Vassia Atanassova Institute of Information and Communication Technologies, Bulgarian Academy of Sciences
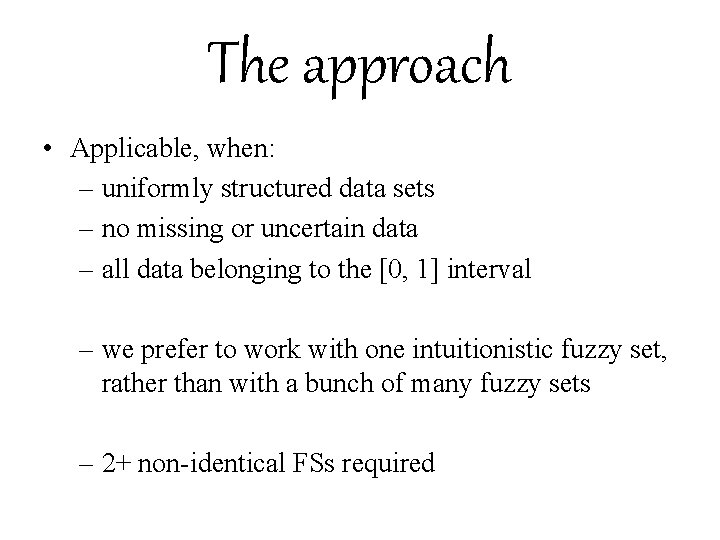
The approach • Applicable, when: – uniformly structured data sets – no missing or uncertain data – all data belonging to the [0, 1] interval – we prefer to work with one intuitionistic fuzzy set, rather than with a bunch of many fuzzy sets – 2+ non-identical FSs required
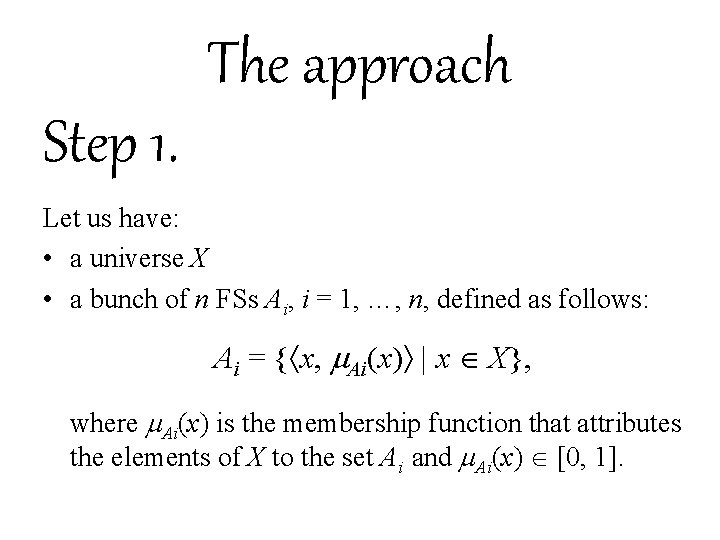
The approach Step 1. Let us have: • a universe X • a bunch of n FSs Ai, i = 1, …, n, defined as follows: Ai = { x, Ai(x) | x X}, where Ai(x) is the membership function that attributes the elements of X to the set Ai and Ai(x) [0, 1].
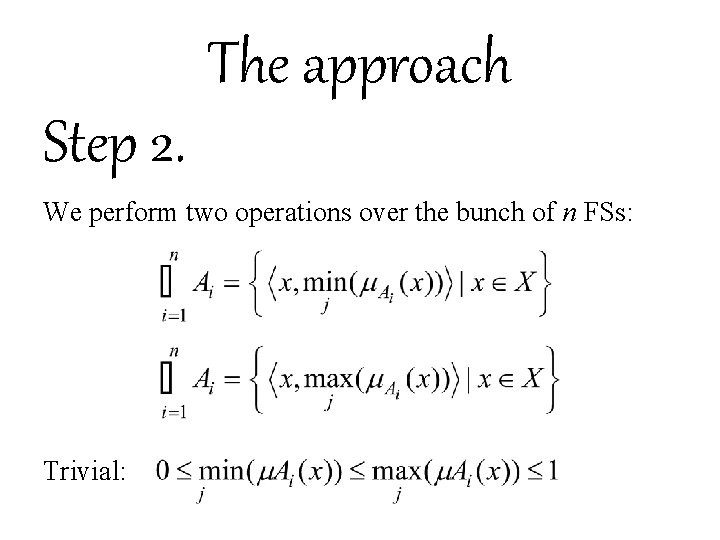
The approach Step 2. We perform two operations over the bunch of n FSs: Trivial:
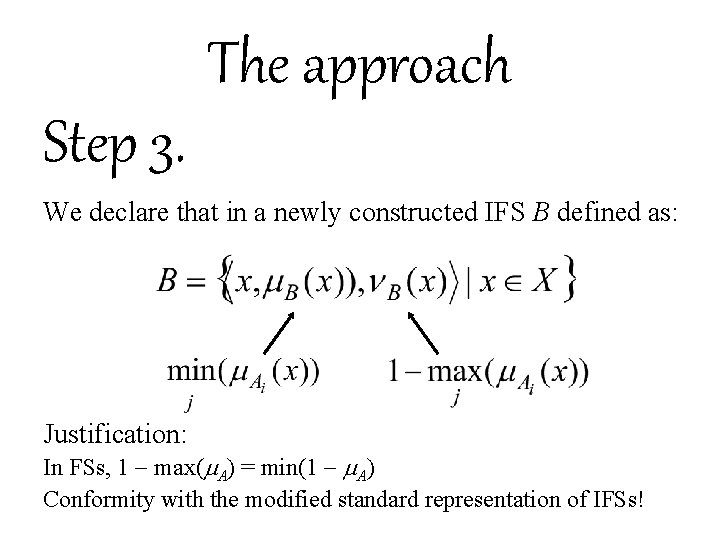
The approach Step 3. We declare that in a newly constructed IFS B defined as: Justification: In FSs, 1 max( A) = min(1 A) Conformity with the modified standard representation of IFSs!
![Numerical example Example Average monthly temperatures normed to the 0 1interval 0 Numerical example • Example: Average monthly temperatures normed to the [0, 1]-interval (0 =](https://slidetodoc.com/presentation_image/7e38cb40305b1e3c53b56c2aa42a66ef/image-6.jpg)
Numerical example • Example: Average monthly temperatures normed to the [0, 1]-interval (0 = “coldest”, 1 = “hottest month” Jan Feb Mar Apr May Jun Jul Aug Sep Oct Nov Dec 2001 0. 9 2. 0 10. 0 9. 6 15. 1 17. 6 22. 0 22. 4 16. 7 13. 2 4. 1 -5. 2 2002 -2. 0 5. 2 7. 5 9. 1 15. 8 19. 9 22. 0 19. 2 6. 5 -0. 3 2003 0. 3 -3. 7 3. 9 8. 4 17. 9 21. 5 22. 7 15. 3 10. 4 0. 3 2004 -2. 3 2. 1 5. 6 11. 3 13. 4 20. 7 20. 1 17. 1 12. 9 1. 6 1. 8 2005 0. 6 -1. 5 4. 0 10. 6 15. 2 17. 7 20. 3 19. 1 16. 1 10. 3 4. 4 1. 6 2006 1. 8 -0. 4 15. 4 17. 9 20. 3 20. 7 16. 6 12. 5 5. 5 0. 4 2007 4. 5 3. 9 6. 9 11. 6 16. 5 20. 5 23. 7 21. 5 14. 6 10. 8 2. 8 -0. 7 2008 0. 4 3. 2 11. 6 15. 6 19. 7 21. 1 23. 1 15. 6 12. 1 6. 4 2. 5 2009 -0. 8 0. 7 5. 1 11. 3 16. 3 19. 1 21. 4 20. 8 16. 4 11. 2 7. 5 2. 8 2010 0. 2 1. 8 5. 7 10. 7 15. 6 18. 6 22. 5 16. 5 10. 5 0. 6 2011 -1. 3 0. 2 5. 1 9. 9 14. 7 19. 0 21. 8 21. 4 19. 5 9. 4 2. 7 1. 2 2012 -2. 2 -3. 6 6. 6 11. 9 14. 8 21. 5 24. 7 22. 9 24. 8 14. 0 7. 7 -0. 8
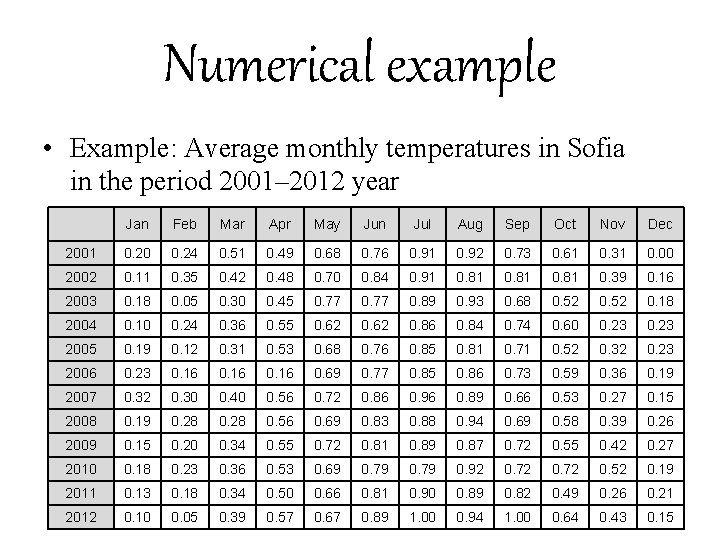
Numerical example • Example: Average monthly temperatures in Sofia in the period 2001– 2012 year Jan Feb Mar Apr May Jun Jul Aug Sep Oct Nov Dec 2001 0. 20 0. 24 0. 51 0. 49 0. 68 0. 76 0. 91 0. 92 0. 73 0. 61 0. 31 0. 00 2002 0. 11 0. 35 0. 42 0. 48 0. 70 0. 84 0. 91 0. 81 0. 39 0. 16 2003 0. 18 0. 05 0. 30 0. 45 0. 77 0. 89 0. 93 0. 68 0. 52 0. 18 2004 0. 10 0. 24 0. 36 0. 55 0. 62 0. 86 0. 84 0. 74 0. 60 0. 23 2005 0. 19 0. 12 0. 31 0. 53 0. 68 0. 76 0. 85 0. 81 0. 71 0. 52 0. 32 0. 23 2006 0. 23 0. 16 0. 69 0. 77 0. 85 0. 86 0. 73 0. 59 0. 36 0. 19 2007 0. 32 0. 30 0. 40 0. 56 0. 72 0. 86 0. 96 0. 89 0. 66 0. 53 0. 27 0. 15 2008 0. 19 0. 28 0. 56 0. 69 0. 83 0. 88 0. 94 0. 69 0. 58 0. 39 0. 26 2009 0. 15 0. 20 0. 34 0. 55 0. 72 0. 81 0. 89 0. 87 0. 72 0. 55 0. 42 0. 27 2010 0. 18 0. 23 0. 36 0. 53 0. 69 0. 79 0. 92 0. 72 0. 52 0. 19 2011 0. 13 0. 18 0. 34 0. 50 0. 66 0. 81 0. 90 0. 89 0. 82 0. 49 0. 26 0. 21 2012 0. 10 0. 05 0. 39 0. 57 0. 67 0. 89 1. 00 0. 94 1. 00 0. 64 0. 43 0. 15
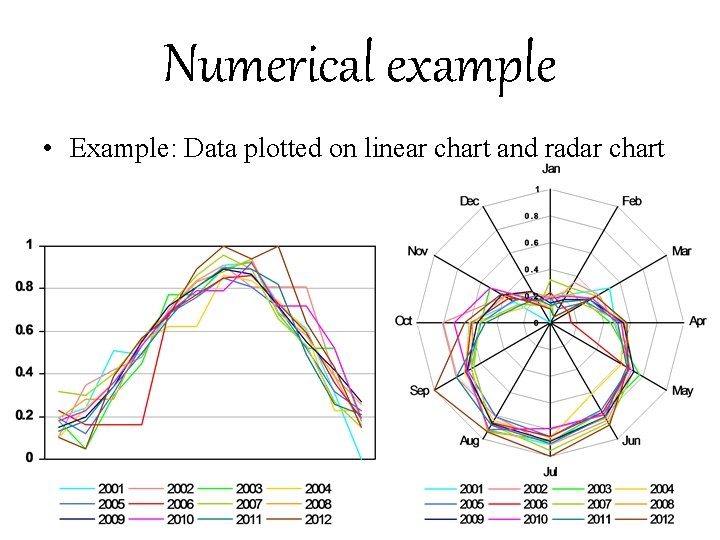
Numerical example • Example: Data plotted on linear chart and radar chart
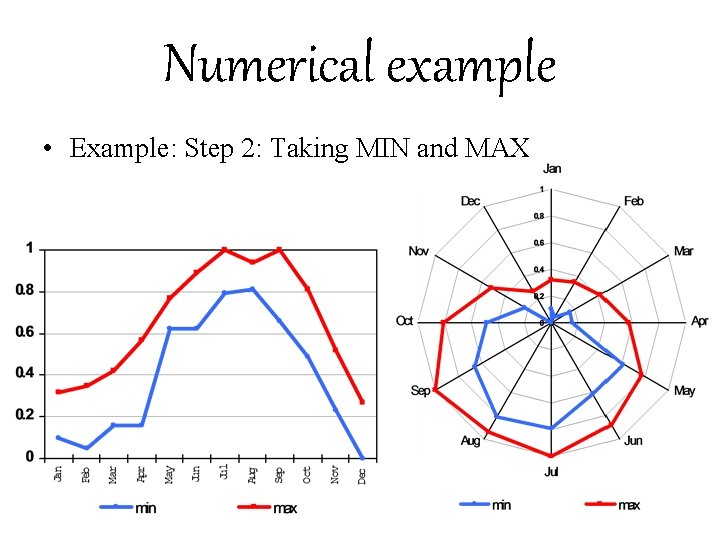
Numerical example • Example: Step 2: Taking MIN and MAX
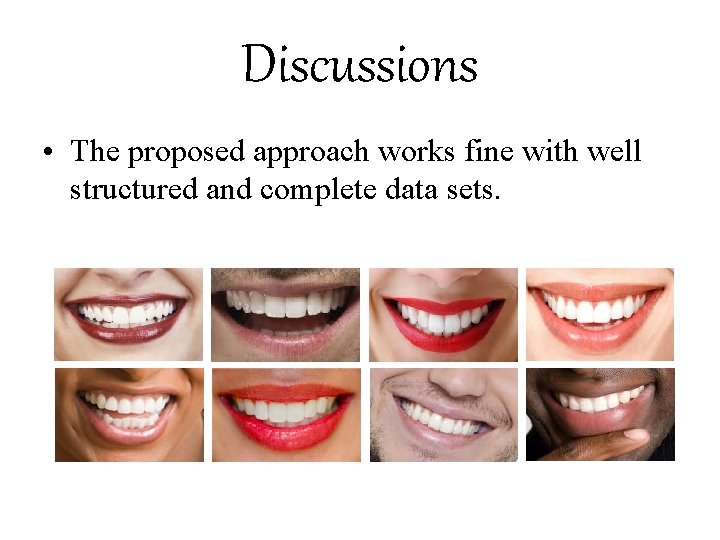
Discussions • The proposed approach works fine with well structured and complete data sets.
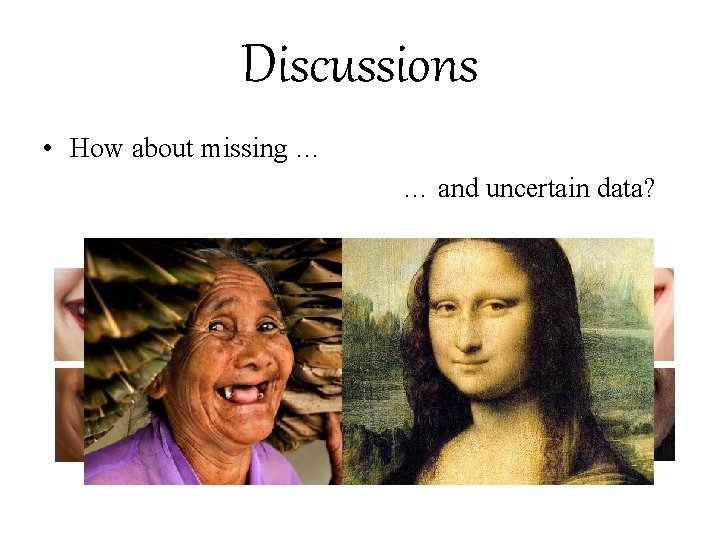
Discussions • How about missing … … and uncertain data?
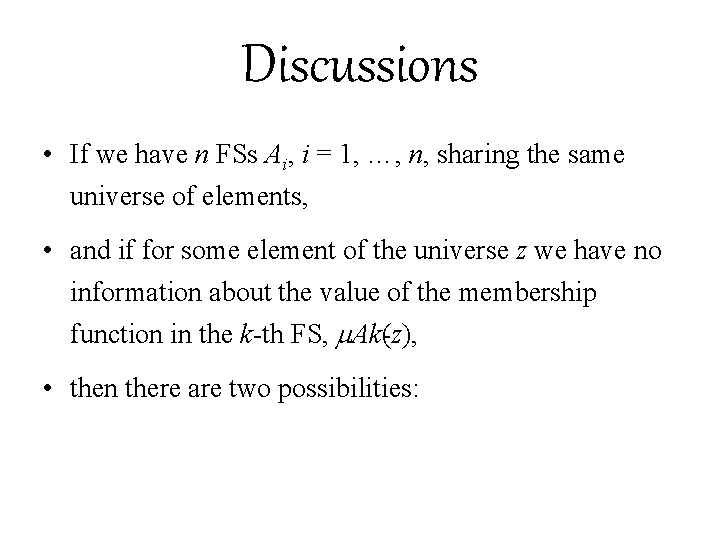
Discussions • If we have n FSs Ai, i = 1, …, n, sharing the same universe of elements, • and if for some element of the universe z we have no information about the value of the membership function in the k-th FS, Ak (z), • then there are two possibilities:
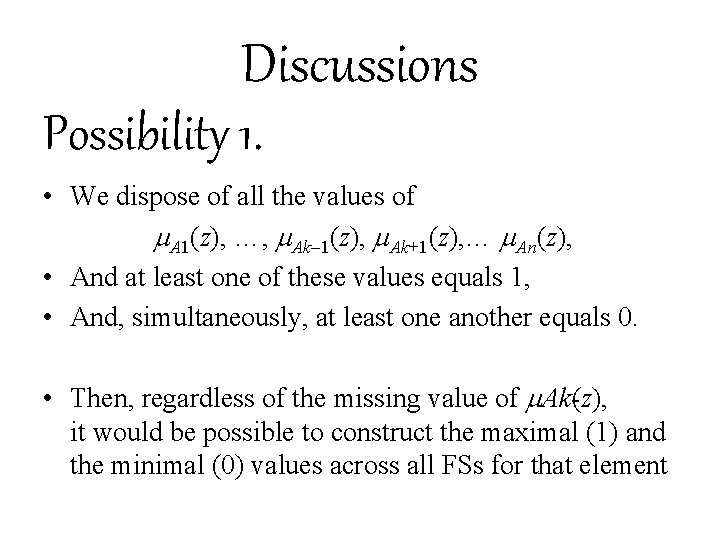
Discussions Possibility 1. • We dispose of all the values of A 1(z), …, Ak– 1(z), Ak+1(z), … An(z), • And at least one of these values equals 1, • And, simultaneously, at least one another equals 0. • Then, regardless of the missing value of Ak (z), it would be possible to construct the maximal (1) and the minimal (0) values across all FSs for that element
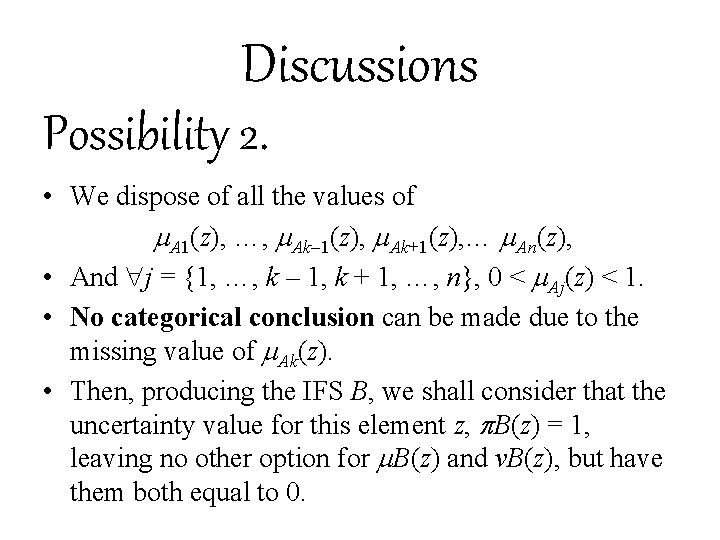
Discussions Possibility 2. • We dispose of all the values of A 1(z), …, Ak– 1(z), Ak+1(z), … An(z), • And j = {1, …, k – 1, k + 1, …, n}, 0 < Aj(z) < 1. • No categorical conclusion can be made due to the missing value of Ak(z). • Then, producing the IFS B, we shall consider that the uncertainty value for this element z, B(z) = 1, leaving no other option for B(z) and νB(z), but have them both equal to 0.
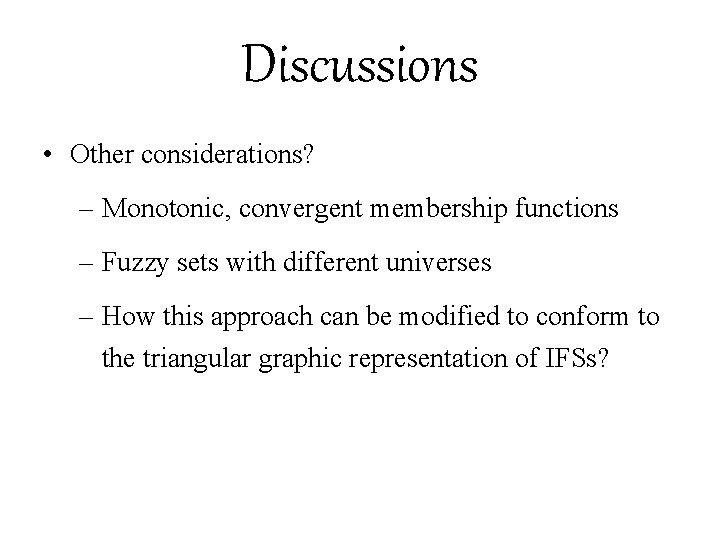
Discussions • Other considerations? – Monotonic, convergent membership functions – Fuzzy sets with different universes – How this approach can be modified to conform to the triangular graphic representation of IFSs?
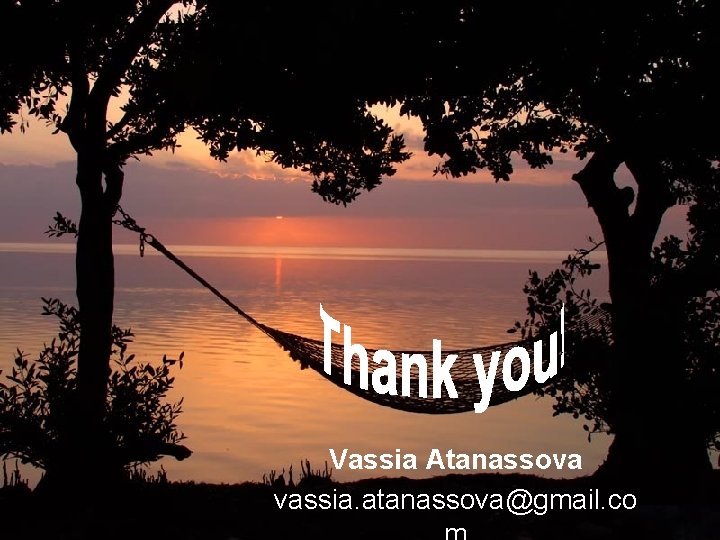
Vassia Atanassova vassia. atanassova@gmail. co
Vassia atanassova
Fuzzy logic wiki
Fuzzy sets and fuzzy logic theory and applications
Fuzzy fidelity
Pengertian logika fuzzy
Fuzzy logic tutorial
Fuzzy art
Fuzzy logic examples from real world
Ahp層級分析法
Fuzzy l
Fuzzification
Generalized modus ponens
Decomposition theorem of fuzzy sets
Fuzzy search mysql
Fuzzy expert system
Aka hashing
Fuzzy logic lecture