Fraud Fighting Actuaries Mathematical Models for Insurance Fraud
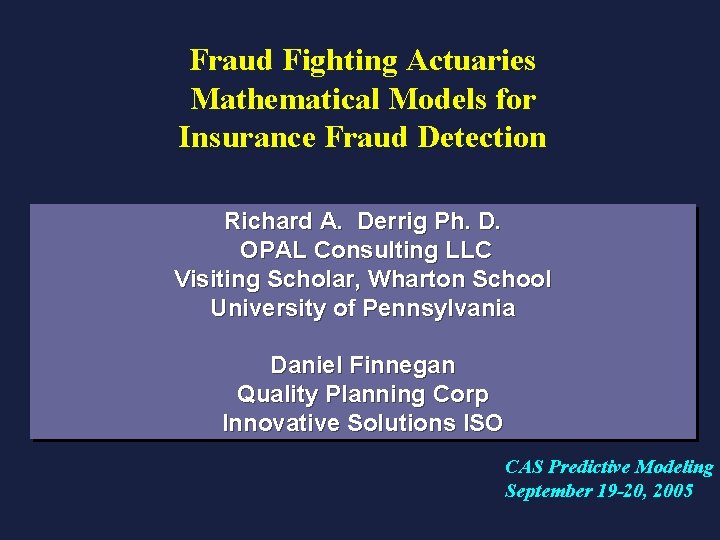
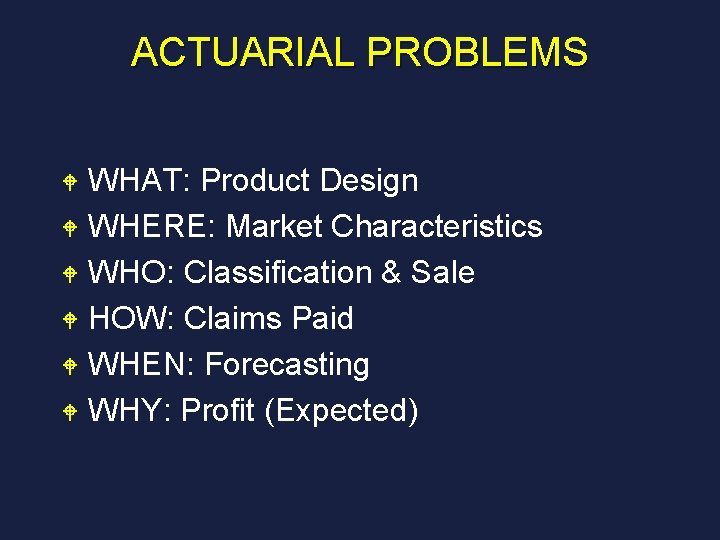
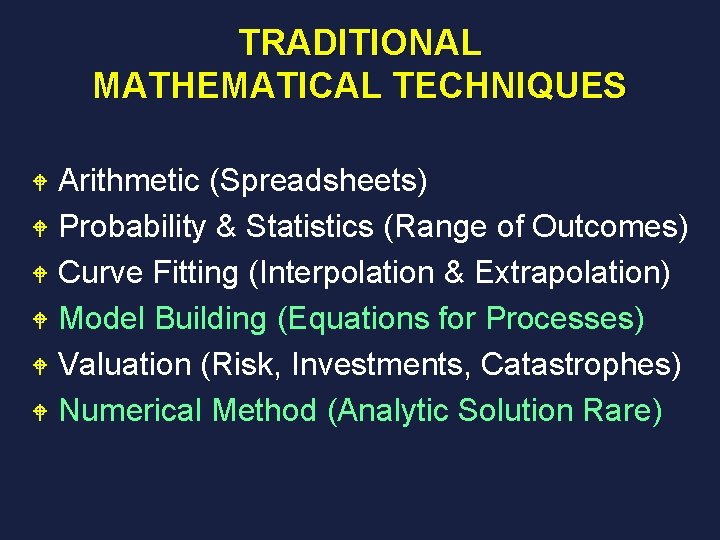
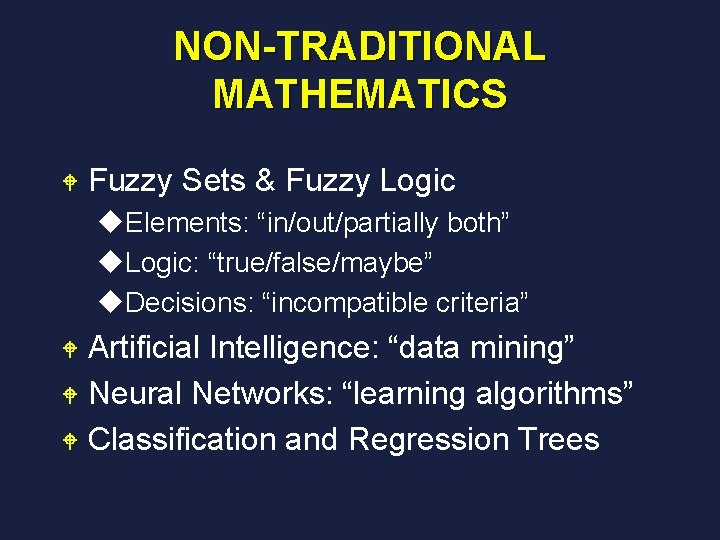
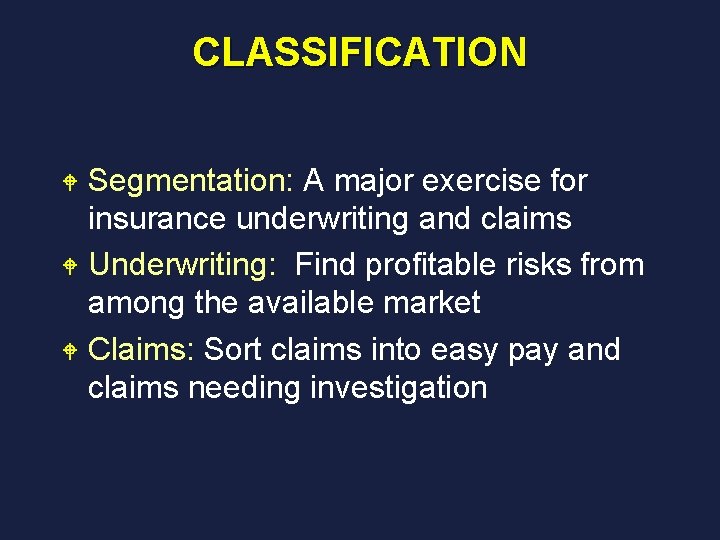
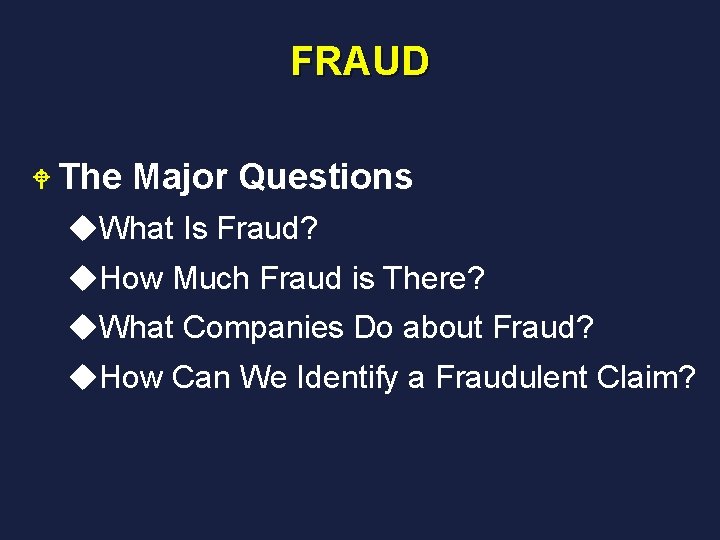
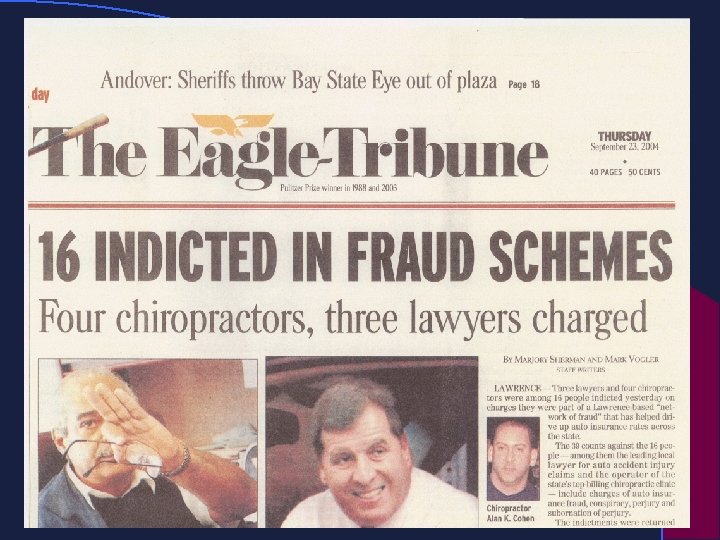
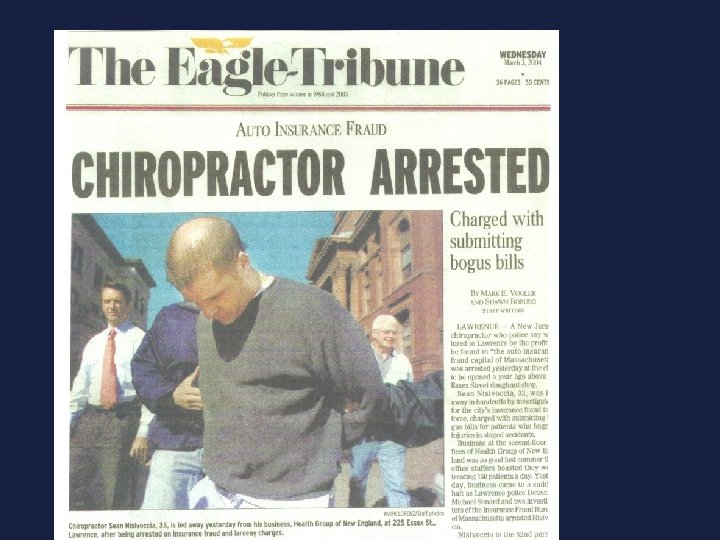
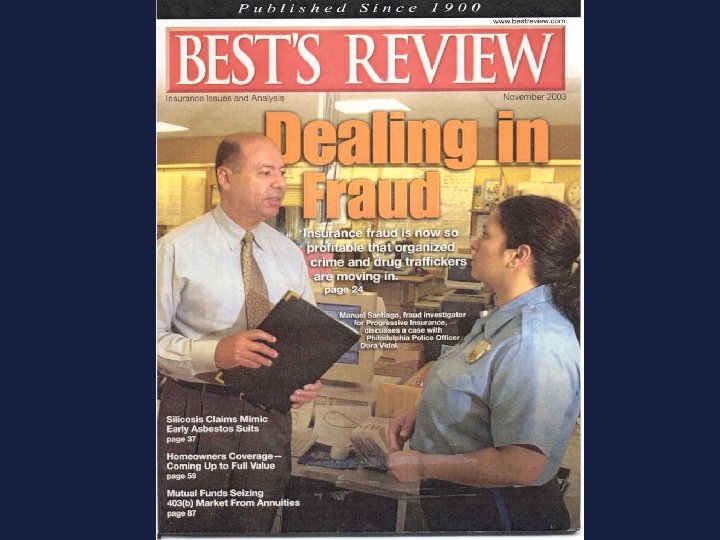
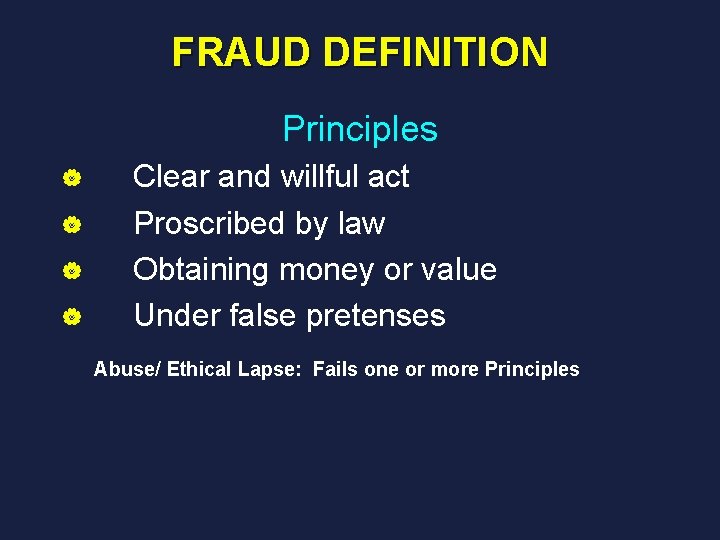
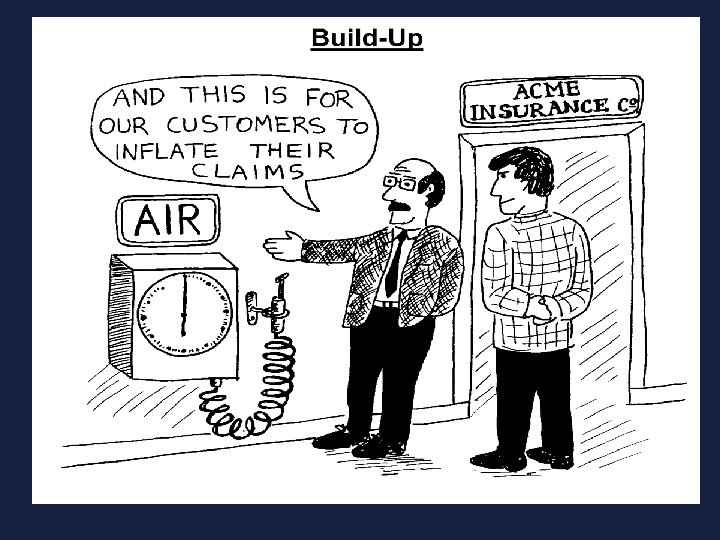
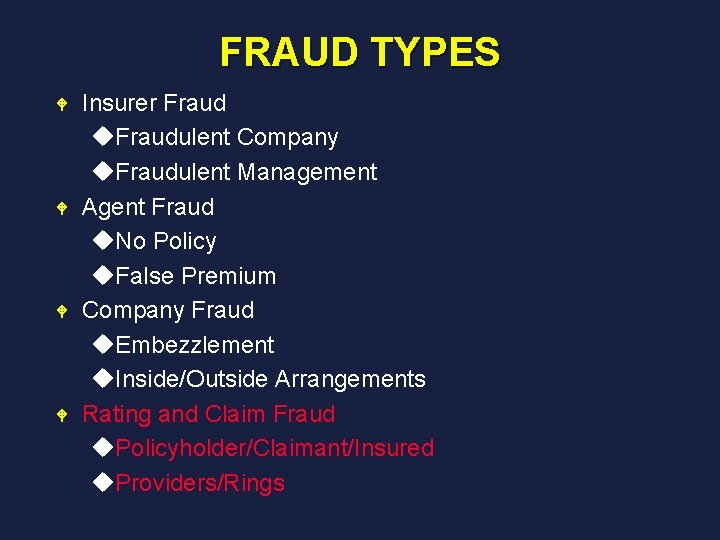
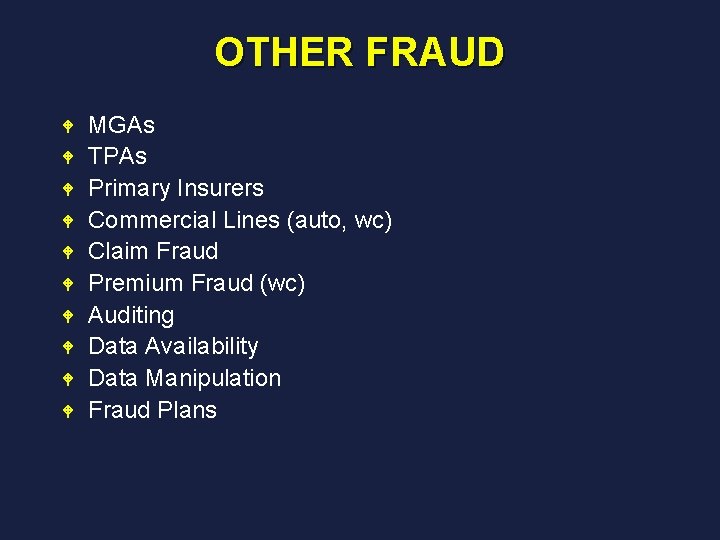
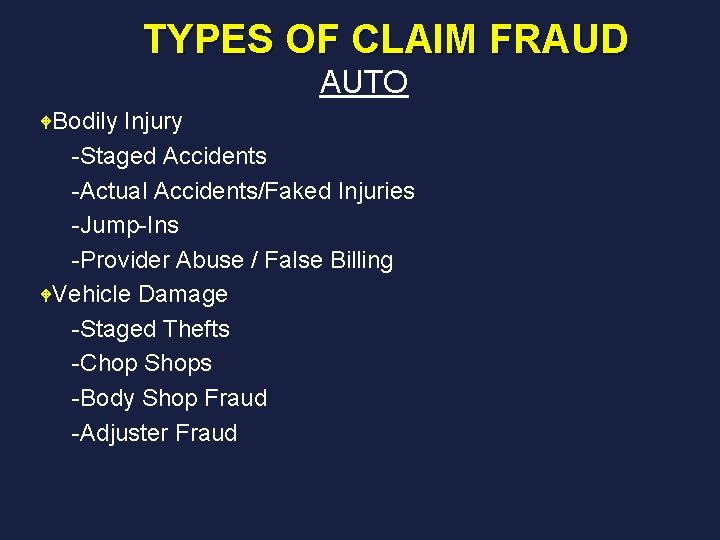
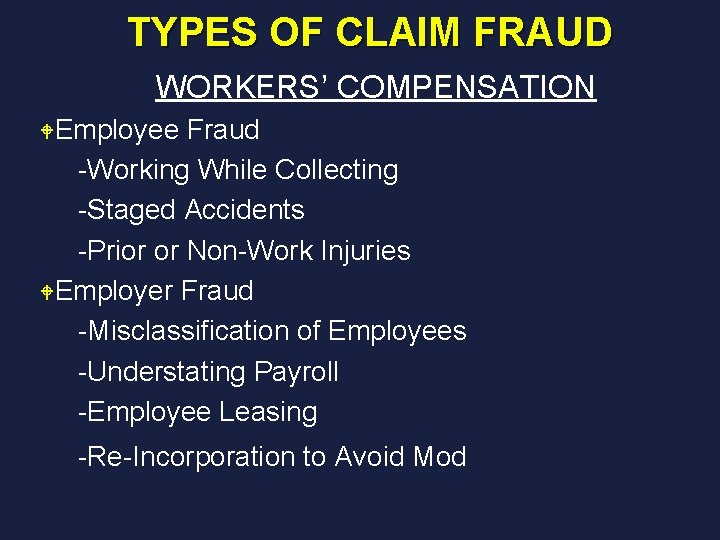
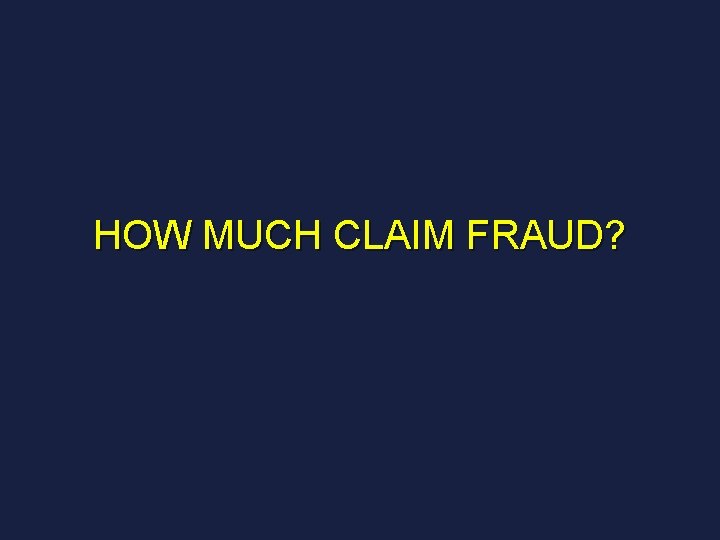
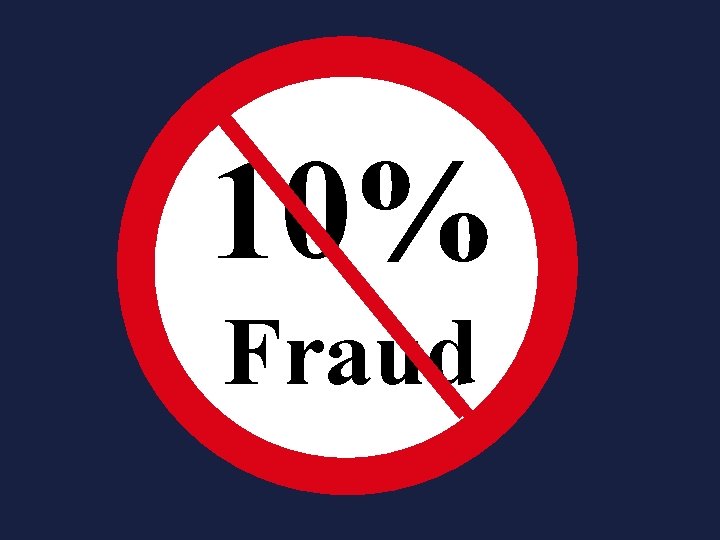
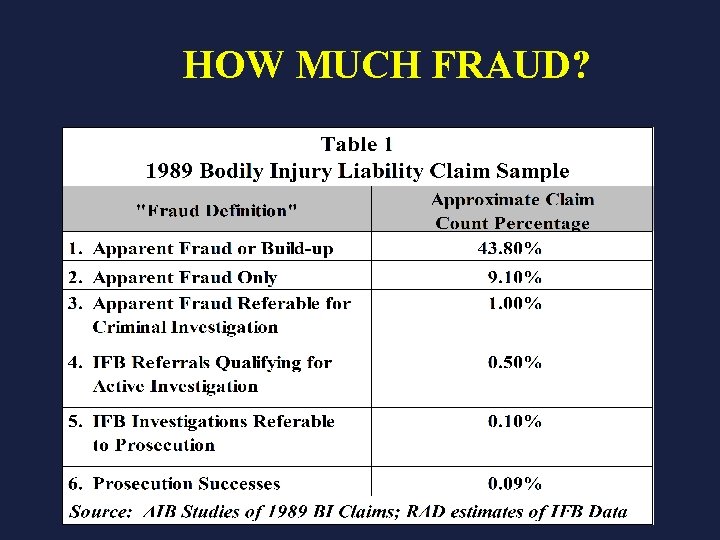
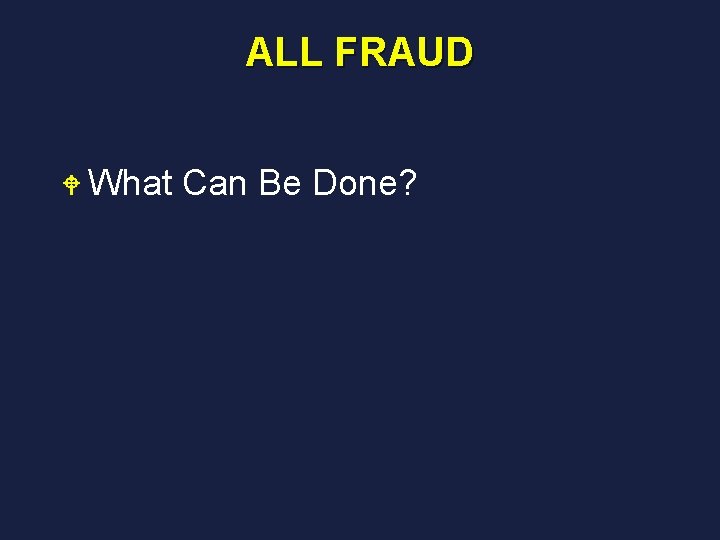
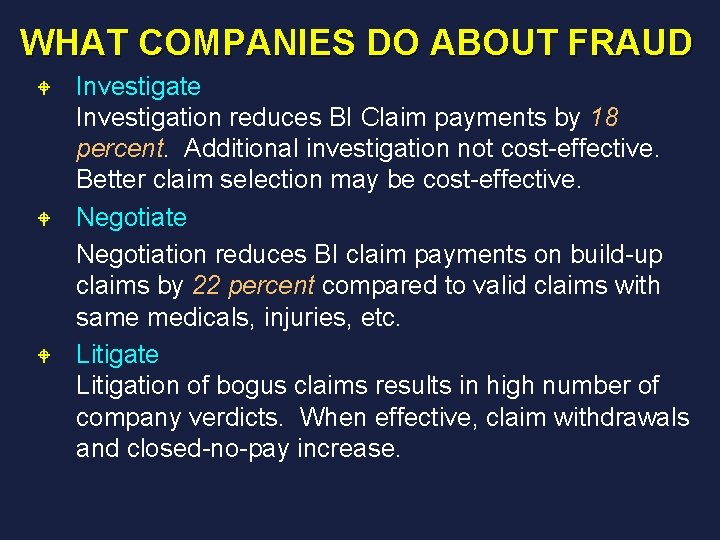
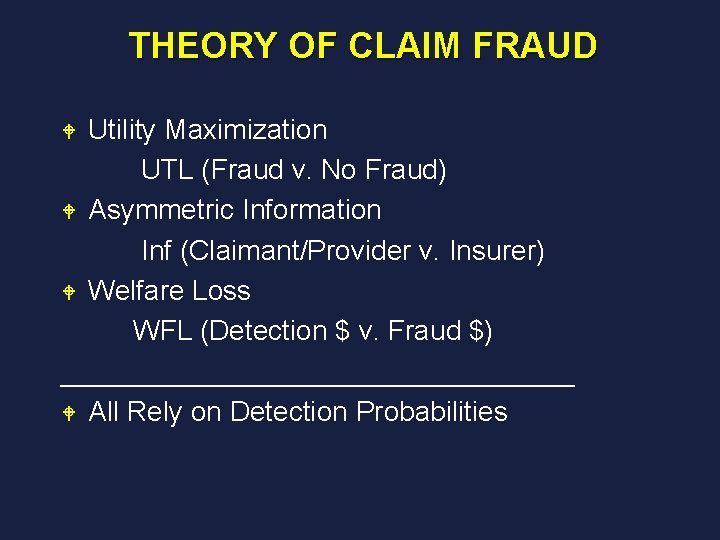
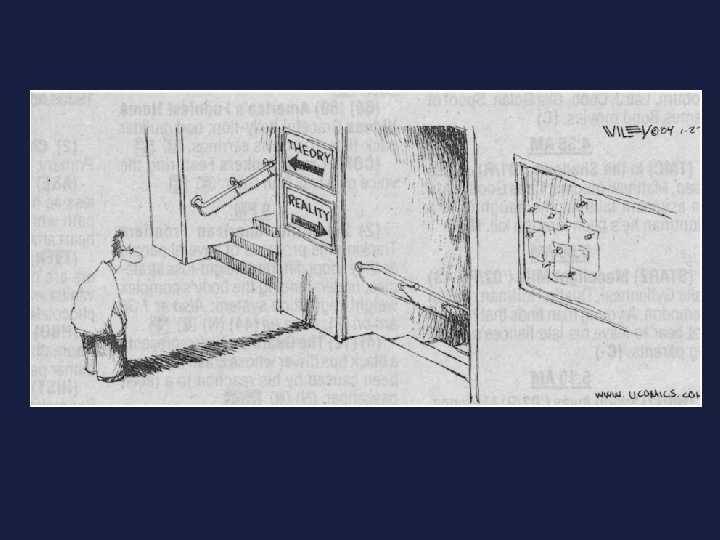
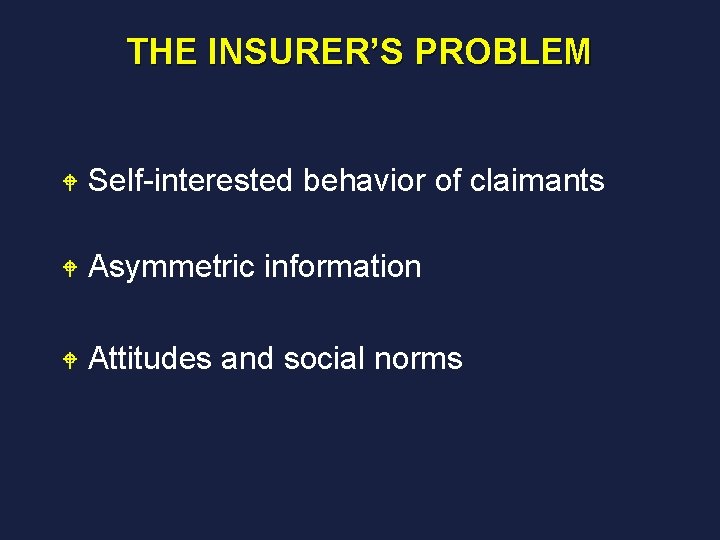
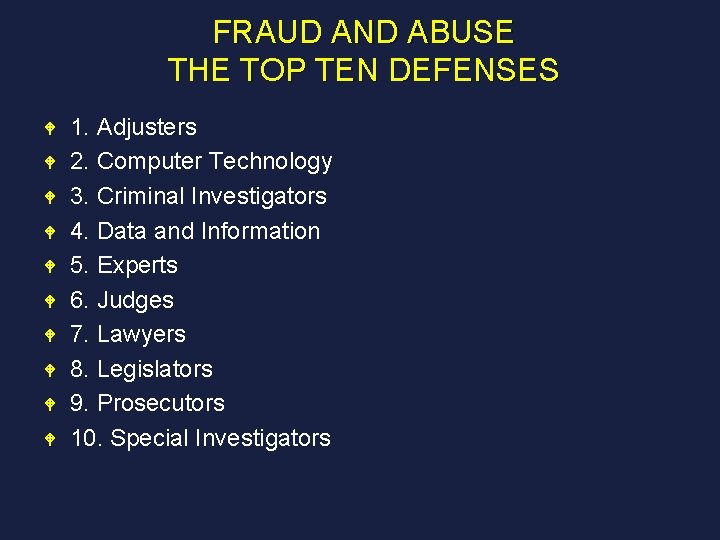
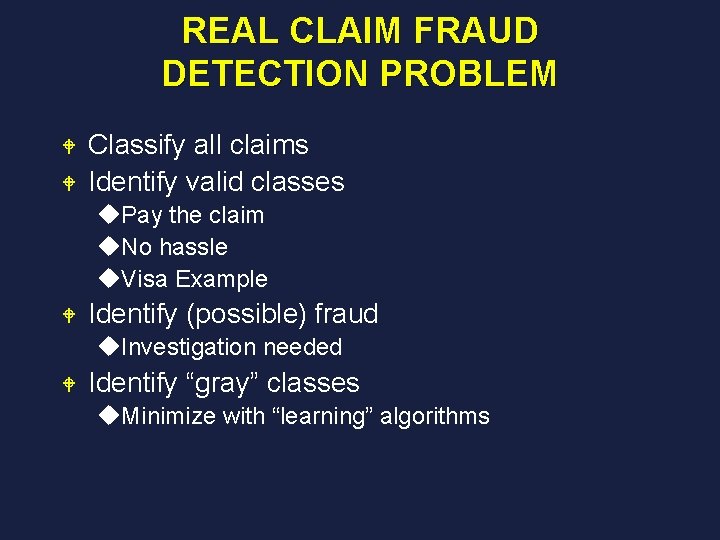
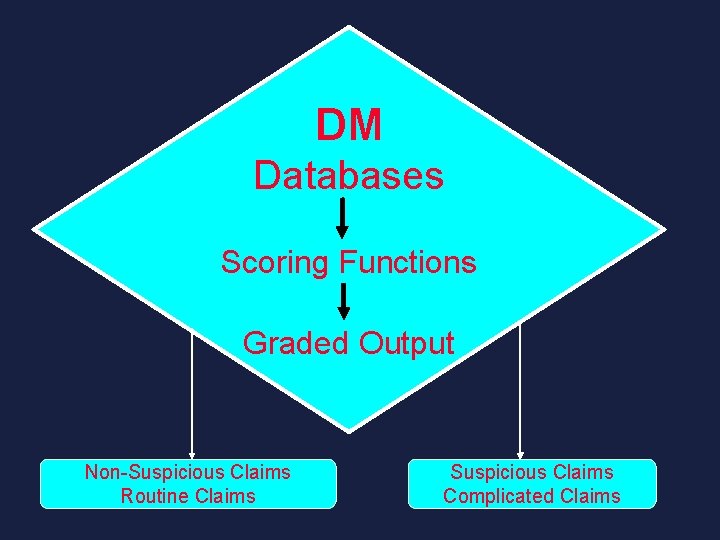
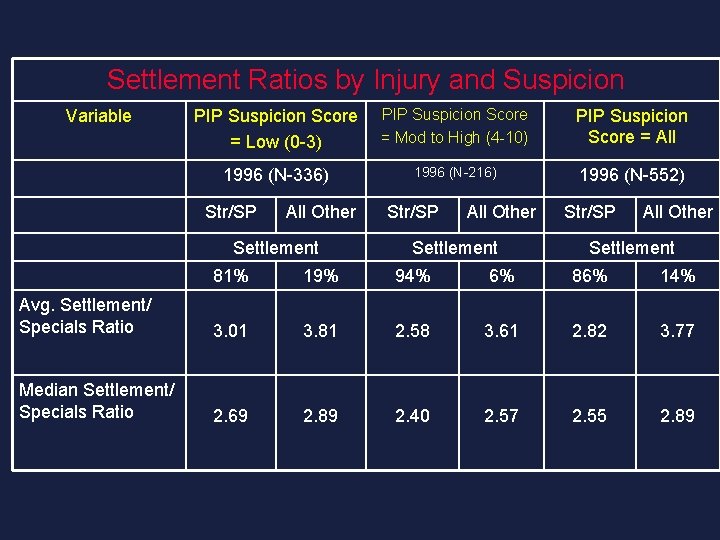
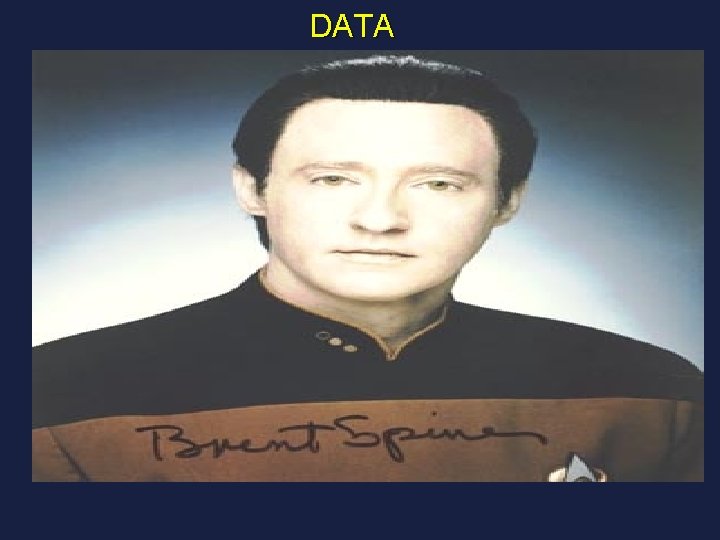
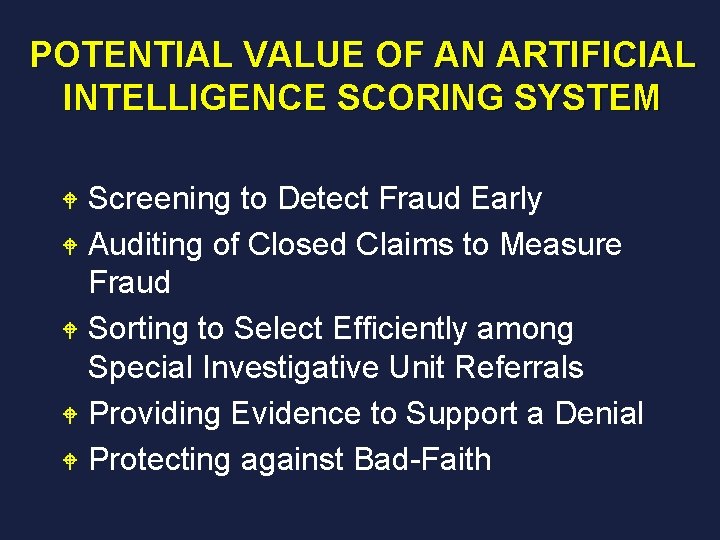
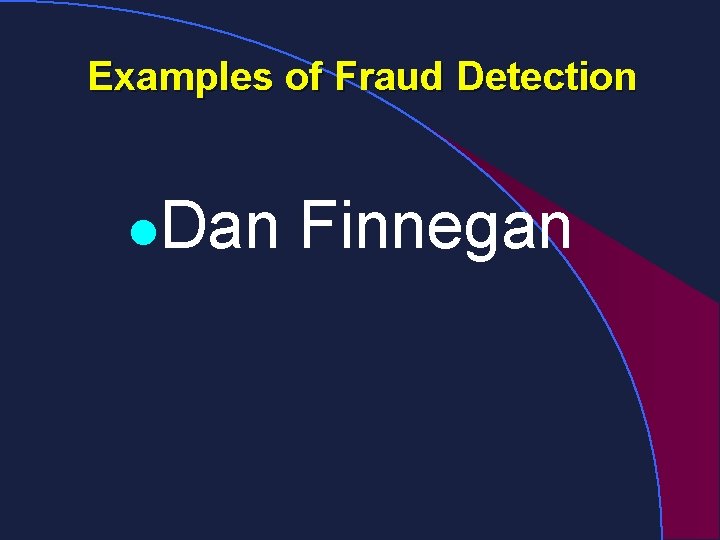
- Slides: 30
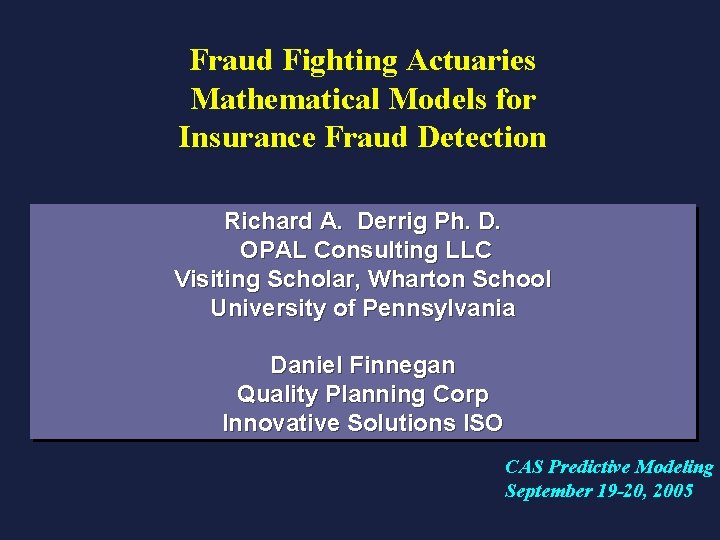
Fraud Fighting Actuaries Mathematical Models for Insurance Fraud Detection Richard A. Derrig Ph. D. OPAL Consulting LLC Visiting Scholar, Wharton School University of Pennsylvania Daniel Finnegan Quality Planning Corp Innovative Solutions ISO CAS Predictive Modeling September 19 -20, 2005
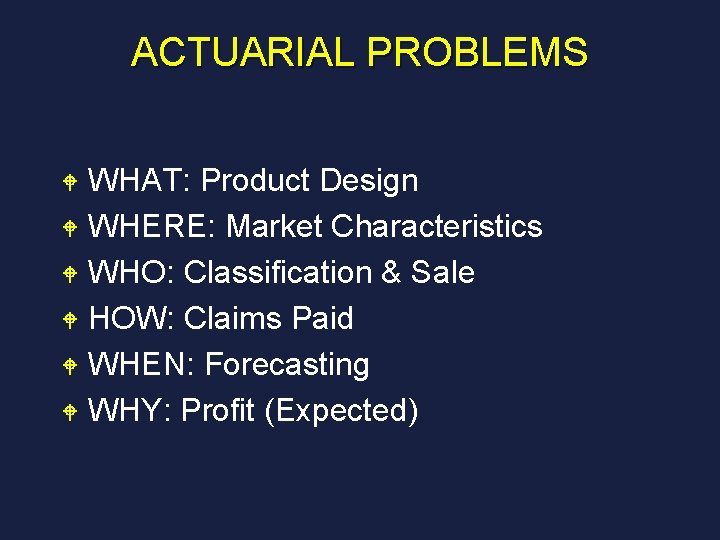
ACTUARIAL PROBLEMS WHAT: Product Design W WHERE: Market Characteristics W WHO: Classification & Sale W HOW: Claims Paid W WHEN: Forecasting W WHY: Profit (Expected) W
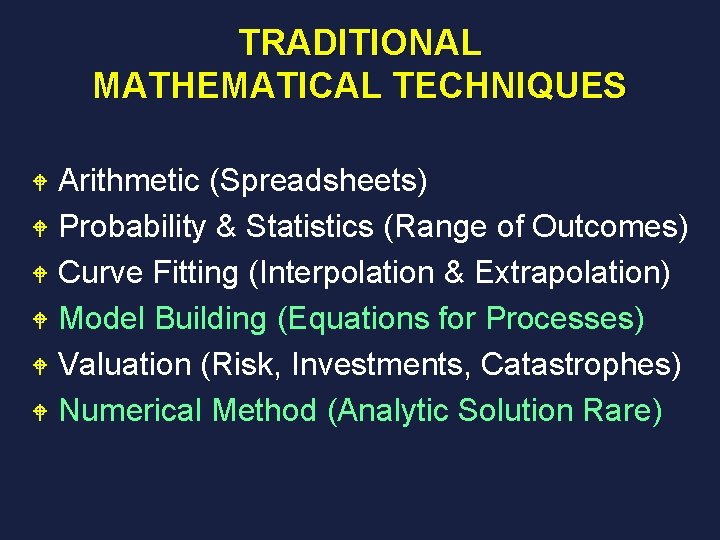
TRADITIONAL MATHEMATICAL TECHNIQUES Arithmetic (Spreadsheets) W Probability & Statistics (Range of Outcomes) W Curve Fitting (Interpolation & Extrapolation) W Model Building (Equations for Processes) W Valuation (Risk, Investments, Catastrophes) W Numerical Method (Analytic Solution Rare) W
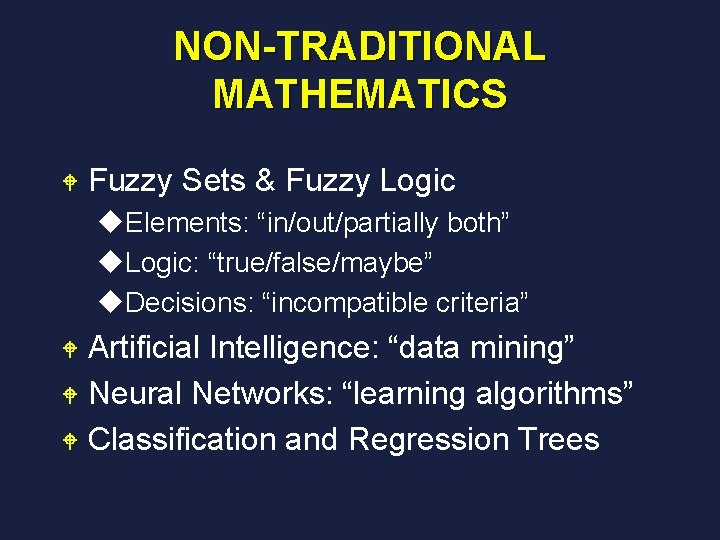
NON-TRADITIONAL MATHEMATICS W Fuzzy Sets & Fuzzy Logic u. Elements: “in/out/partially both” u. Logic: “true/false/maybe” u. Decisions: “incompatible criteria” Artificial Intelligence: “data mining” W Neural Networks: “learning algorithms” W Classification and Regression Trees W
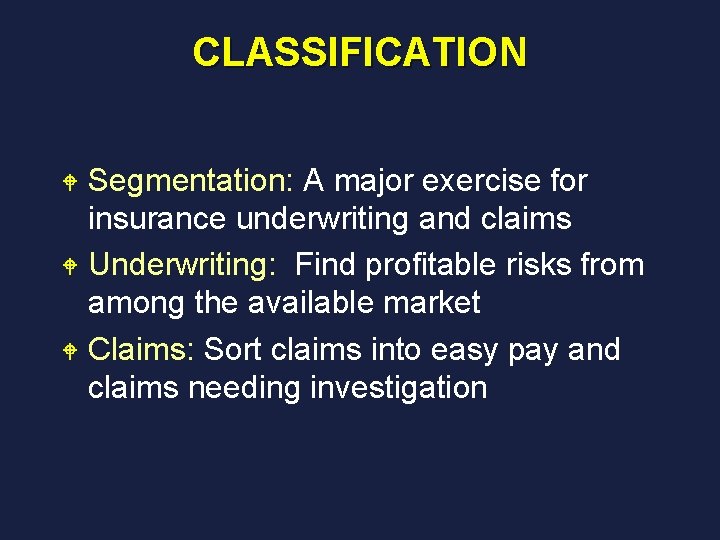
CLASSIFICATION Segmentation: A major exercise for insurance underwriting and claims W Underwriting: Find profitable risks from among the available market W Claims: Sort claims into easy pay and claims needing investigation W
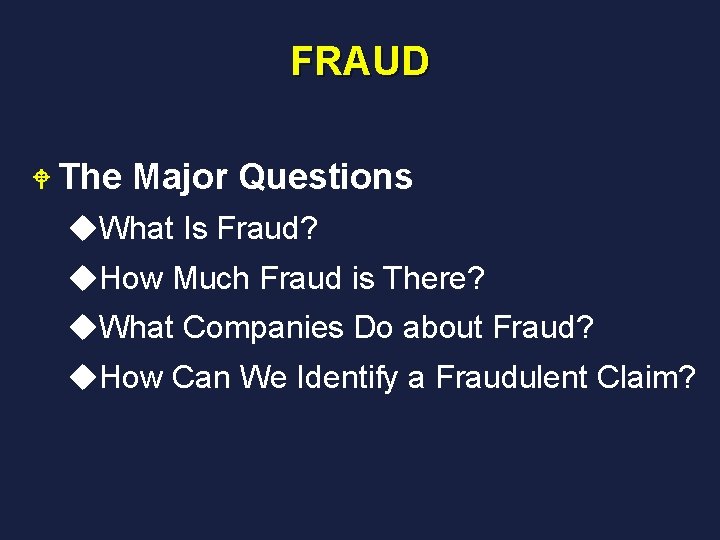
FRAUD W The Major Questions u. What Is Fraud? u. How Much Fraud is There? u. What Companies Do about Fraud? u. How Can We Identify a Fraudulent Claim?
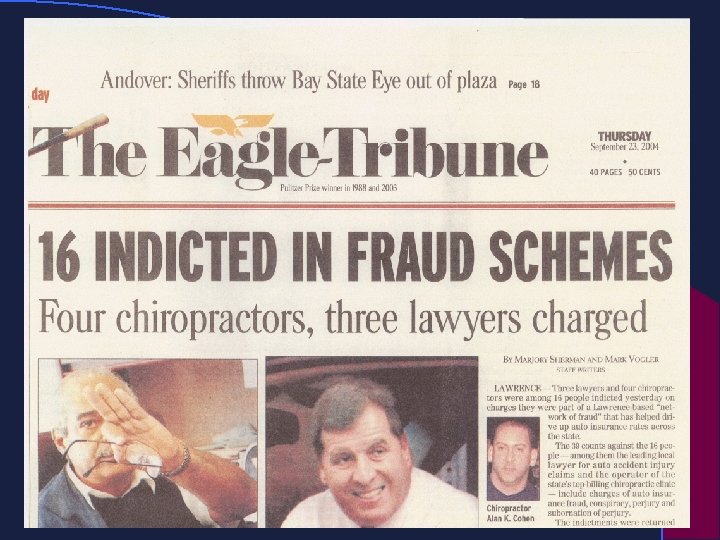
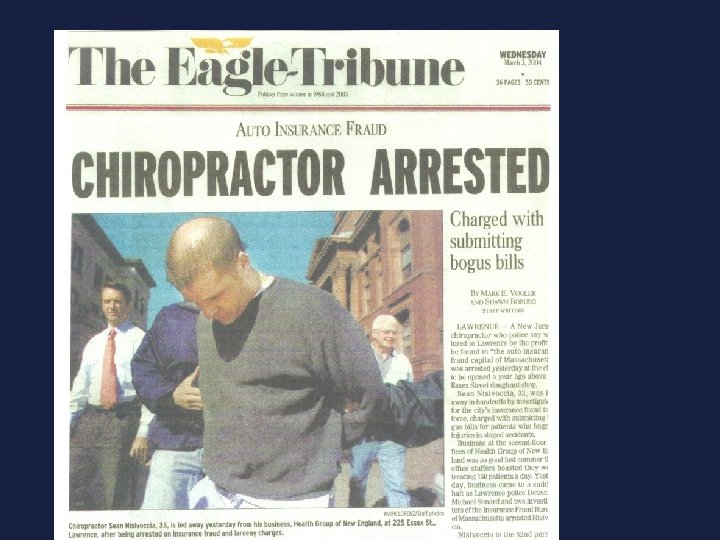
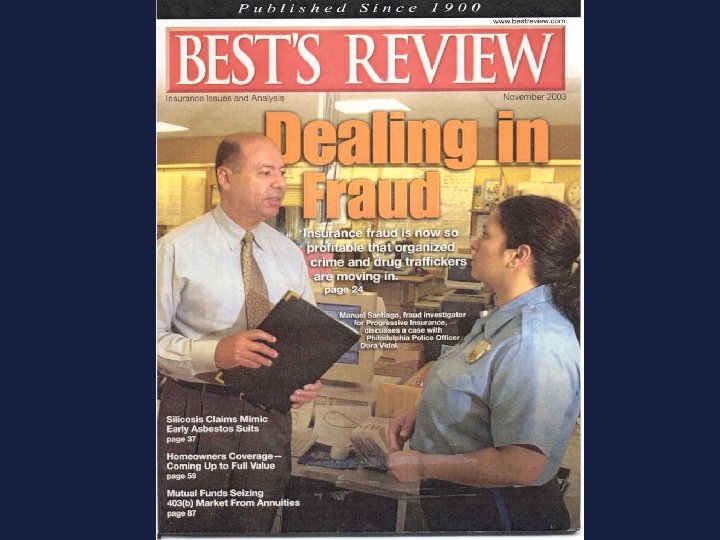
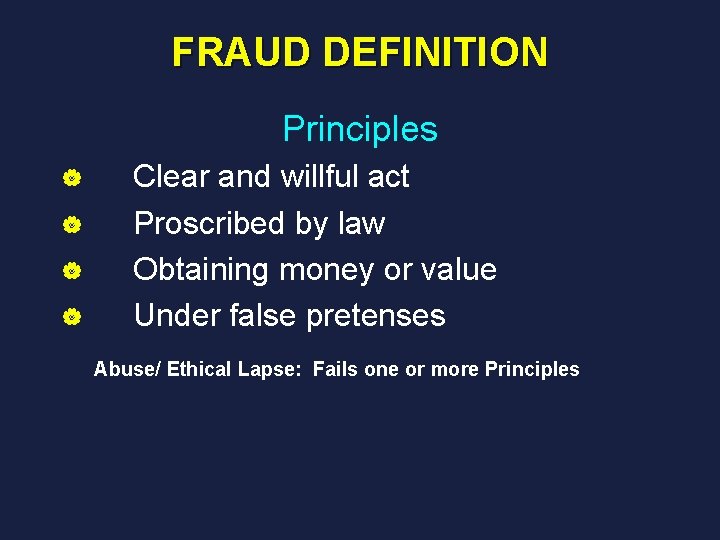
FRAUD DEFINITION Principles | | Clear and willful act Proscribed by law Obtaining money or value Under false pretenses Abuse/ Ethical Lapse: Fails one or more Principles
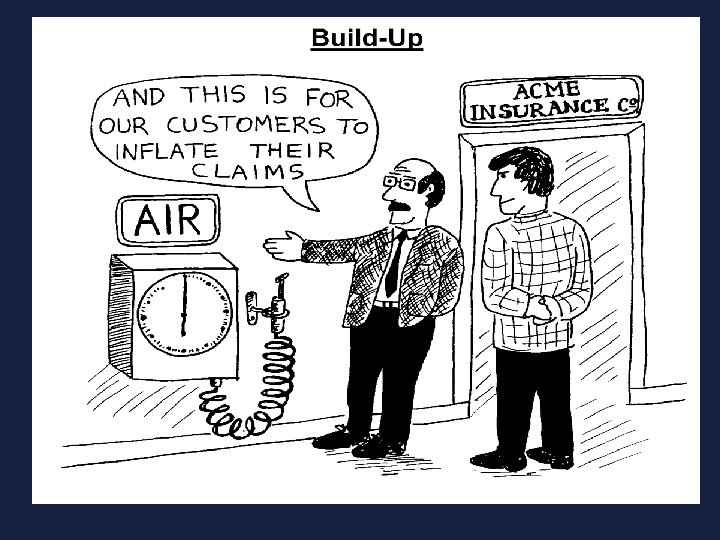
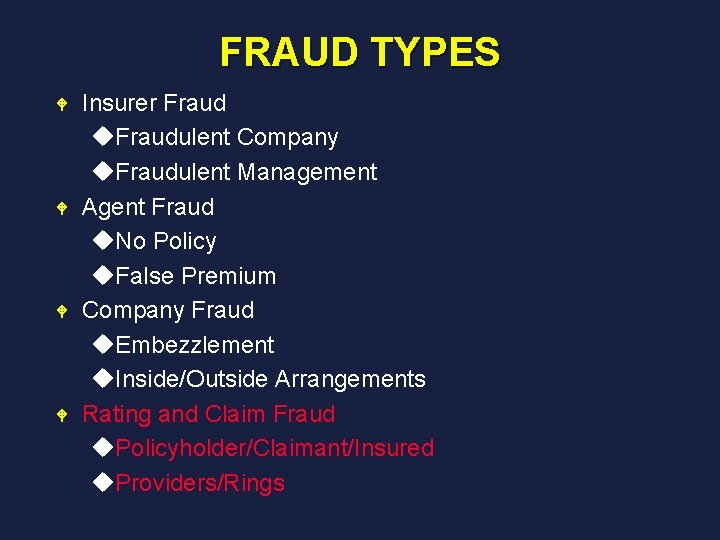
FRAUD TYPES W W Insurer Fraud u. Fraudulent Company u. Fraudulent Management Agent Fraud u. No Policy u. False Premium Company Fraud u. Embezzlement u. Inside/Outside Arrangements Rating and Claim Fraud u. Policyholder/Claimant/Insured u. Providers/Rings
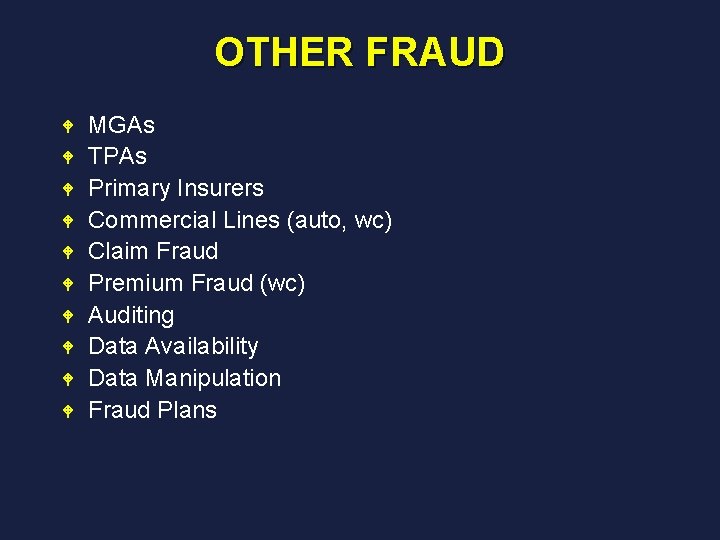
OTHER FRAUD W W W W W MGAs TPAs Primary Insurers Commercial Lines (auto, wc) Claim Fraud Premium Fraud (wc) Auditing Data Availability Data Manipulation Fraud Plans
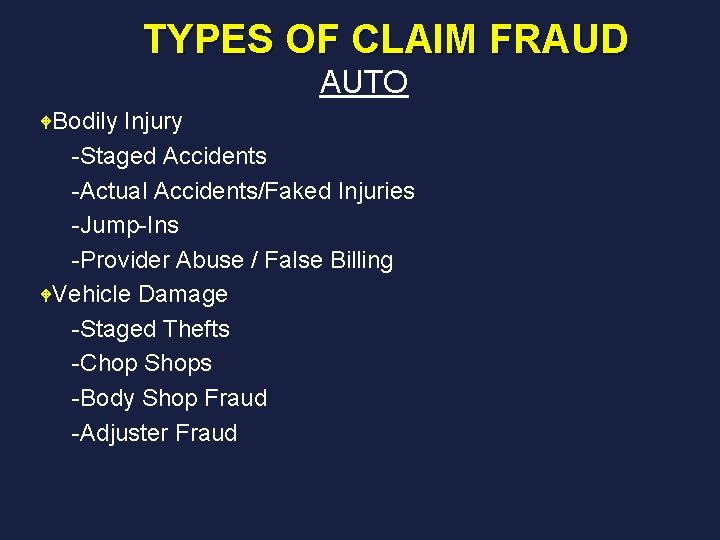
TYPES OF CLAIM FRAUD AUTO WBodily Injury -Staged Accidents -Actual Accidents/Faked Injuries -Jump-Ins -Provider Abuse / False Billing WVehicle Damage -Staged Thefts -Chop Shops -Body Shop Fraud -Adjuster Fraud
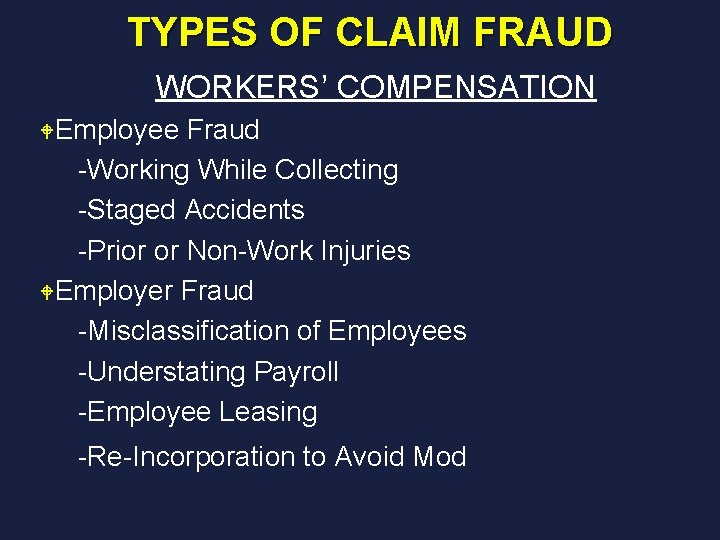
TYPES OF CLAIM FRAUD WORKERS’ COMPENSATION WEmployee Fraud -Working While Collecting -Staged Accidents -Prior or Non-Work Injuries WEmployer Fraud -Misclassification of Employees -Understating Payroll -Employee Leasing -Re-Incorporation to Avoid Mod
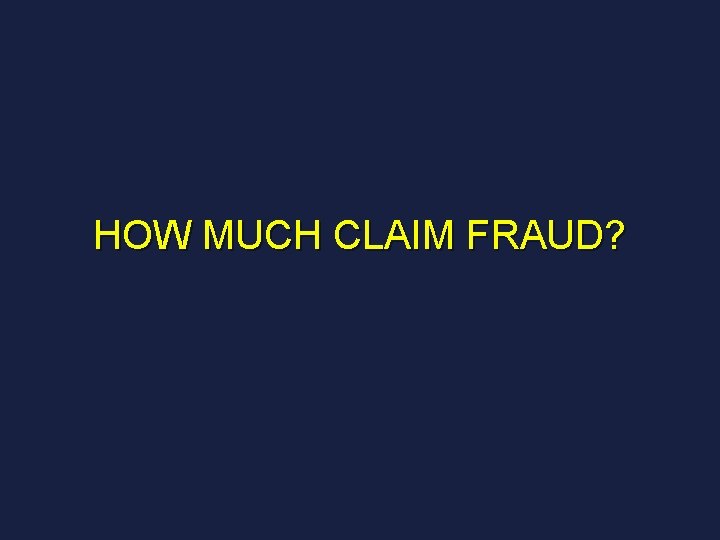
HOW MUCH CLAIM FRAUD?
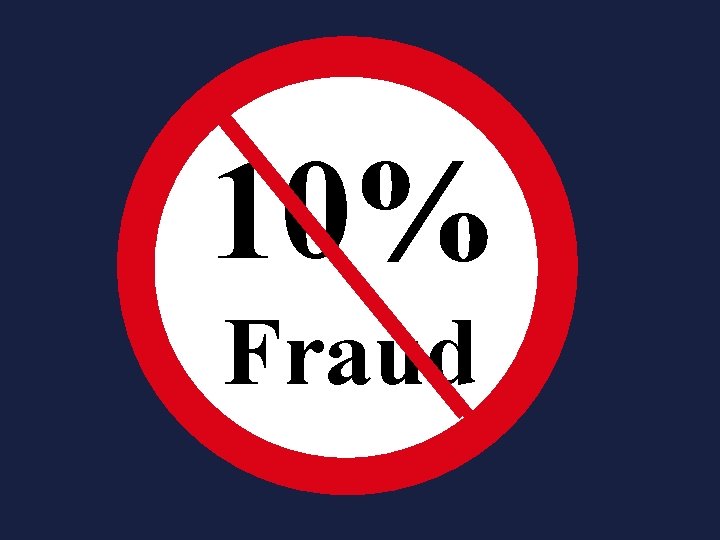
10% Fraud
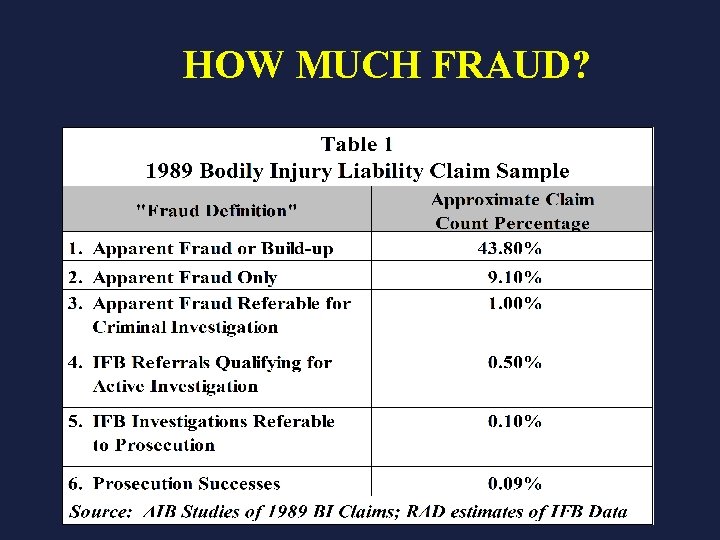
HOW MUCH FRAUD?
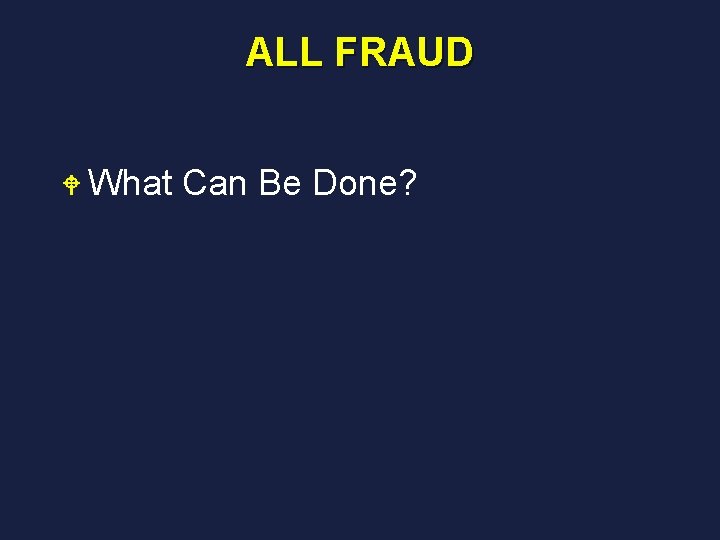
ALL FRAUD W What Can Be Done?
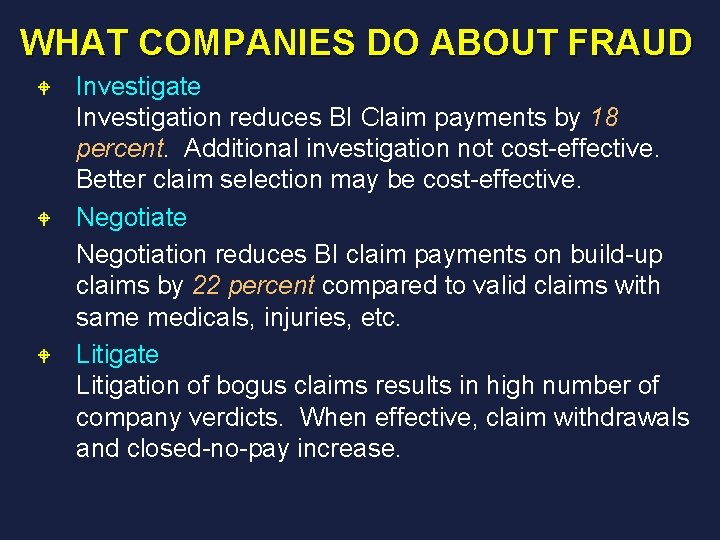
WHAT COMPANIES DO ABOUT FRAUD W W W Investigate Investigation reduces BI Claim payments by 18 percent. Additional investigation not cost-effective. Better claim selection may be cost-effective. Negotiate Negotiation reduces BI claim payments on build-up claims by 22 percent compared to valid claims with same medicals, injuries, etc. Litigate Litigation of bogus claims results in high number of company verdicts. When effective, claim withdrawals and closed-no-pay increase.
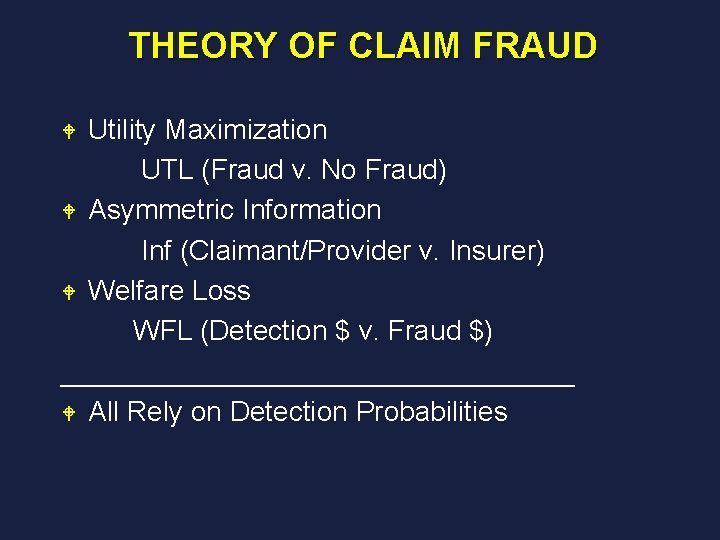
THEORY OF CLAIM FRAUD Utility Maximization UTL (Fraud v. No Fraud) W Asymmetric Information Inf (Claimant/Provider v. Insurer) W Welfare Loss WFL (Detection $ v. Fraud $) _________________ W All Rely on Detection Probabilities W
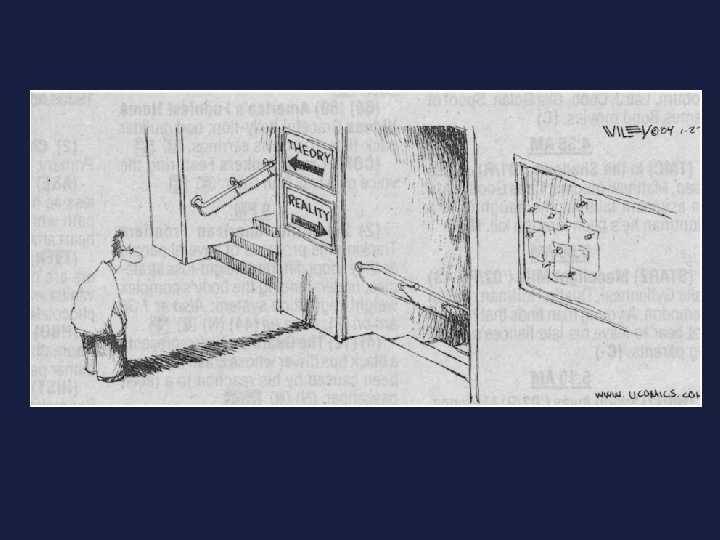
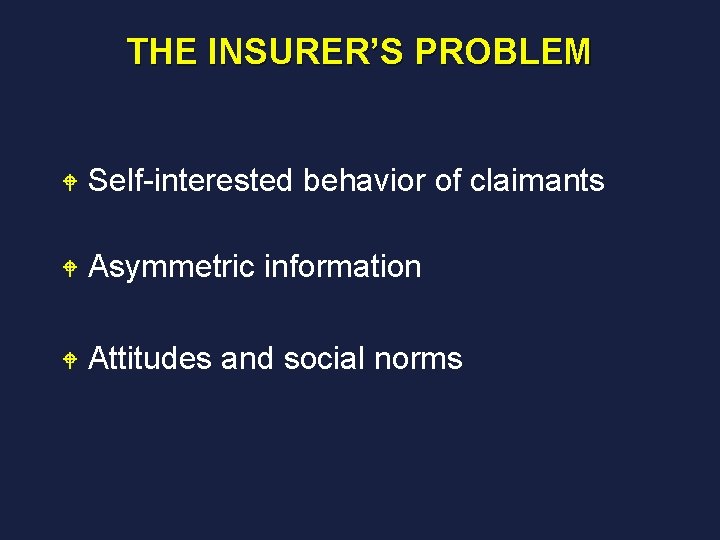
THE INSURER’S PROBLEM W Self-interested behavior of claimants W Asymmetric information W Attitudes and social norms
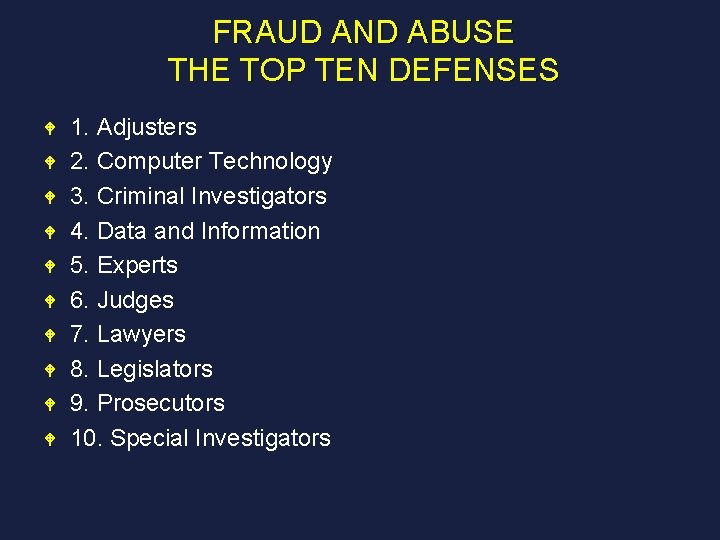
FRAUD AND ABUSE THE TOP TEN DEFENSES W W W W W 1. Adjusters 2. Computer Technology 3. Criminal Investigators 4. Data and Information 5. Experts 6. Judges 7. Lawyers 8. Legislators 9. Prosecutors 10. Special Investigators
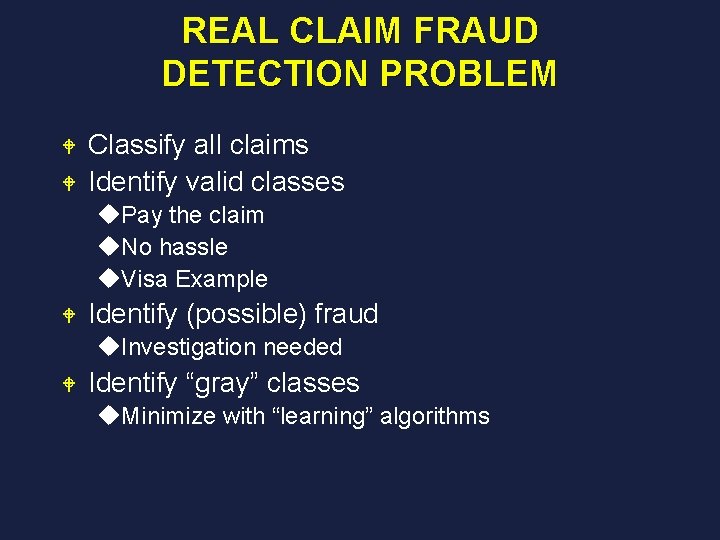
REAL CLAIM FRAUD DETECTION PROBLEM W W Classify all claims Identify valid classes u. Pay the claim u. No hassle u. Visa Example W Identify (possible) fraud u. Investigation needed W Identify “gray” classes u. Minimize with “learning” algorithms
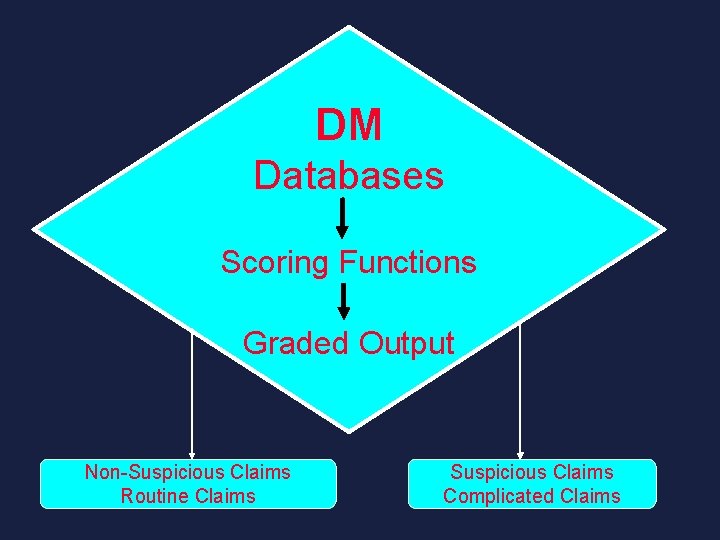
DM Databases Scoring Functions Graded Output Non-Suspicious Claims Routine Claims Suspicious Claims Complicated Claims
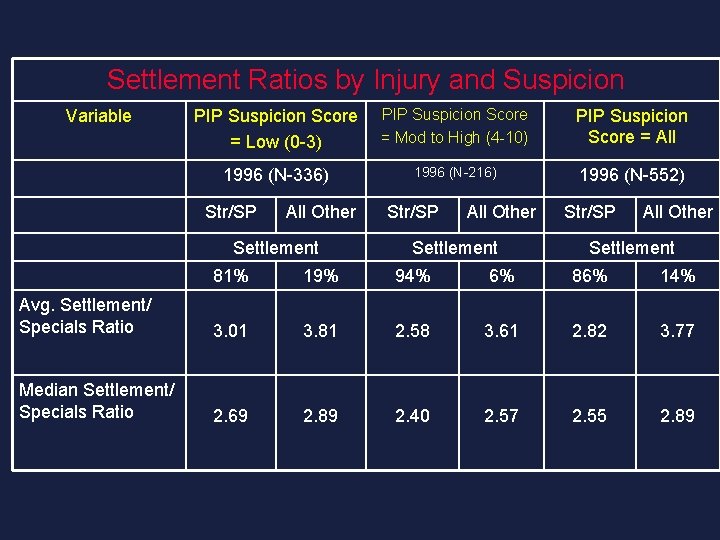
Settlement Ratios by Injury and Suspicion Variable PIP Suspicion Score = Low (0 -3) PIP Suspicion Score = Mod to High (4 -10) PIP Suspicion Score = All 1996 (N-336) 1996 (N-216) 1996 (N-552) Str/SP All Other Settlement 81% 19% 94% 6% 86% 14% Avg. Settlement/ Specials Ratio 3. 01 3. 81 2. 58 3. 61 2. 82 3. 77 Median Settlement/ Specials Ratio 2. 69 2. 89 2. 40 2. 57 2. 55 2. 89
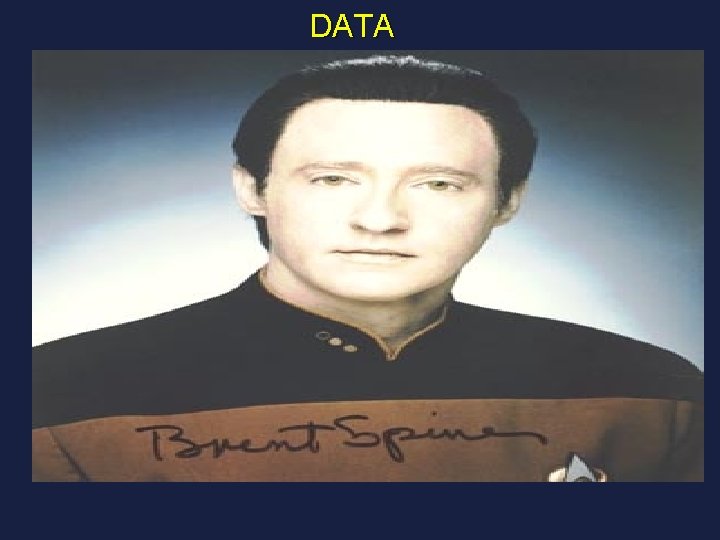
DATA
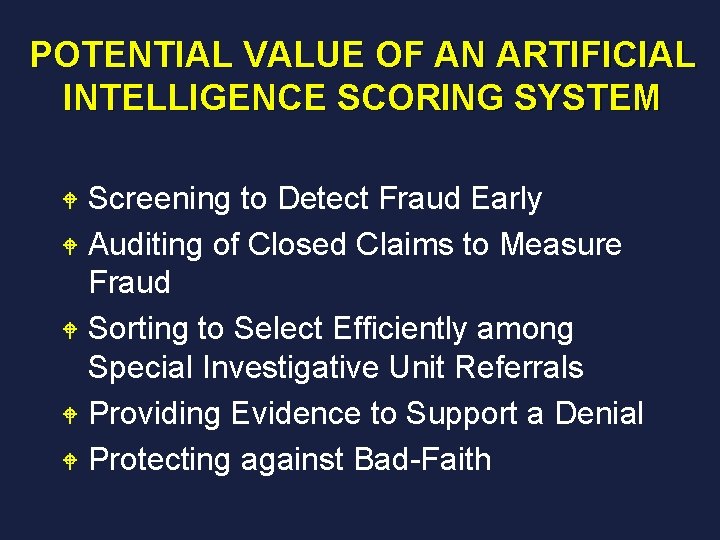
POTENTIAL VALUE OF AN ARTIFICIAL INTELLIGENCE SCORING SYSTEM Screening to Detect Fraud Early W Auditing of Closed Claims to Measure Fraud W Sorting to Select Efficiently among Special Investigative Unit Referrals W Providing Evidence to Support a Denial W Protecting against Bad-Faith W
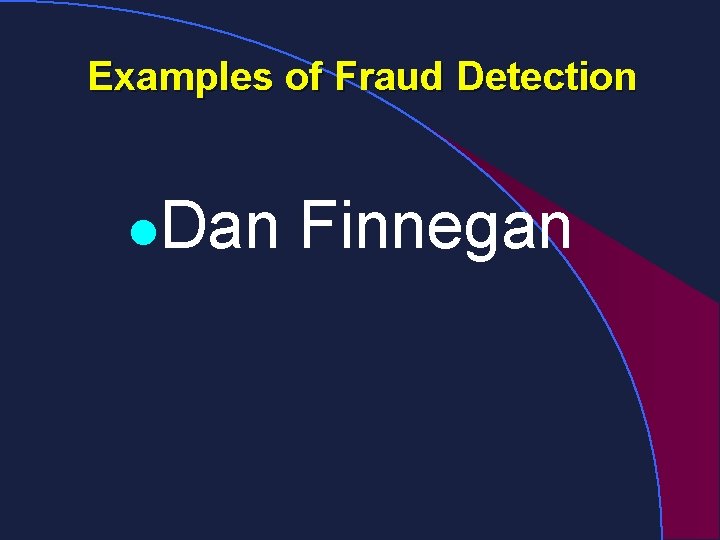
Examples of Fraud Detection l. Dan Finnegan
How do fraud symptoms help in detecting fraud
Actuaries act 2006
University of michigan actuarial science
Conrad siegel actuaries
Akhtar and hasan
International association of black actuaries
Non mathematical economics
Linear functions as mathematical models
Mathematical models for impact prediction
Linear functions as mathematical models
Real world phenomena
Types of mathematical models
Conceptual physical and mathematical models are used to
Functions of fire insurance
Modals and semimodals
Broken stream fire fighting
Vietnam fighting style
Piercing nozzles fire fighting
Bushido fighting style
Shipboard fire fighting organization
The civil war the fighting escalates
Firefighter motto
Why are the von gradwitz and znaeym families fighting
Fighting hunger
Fire fighting techniques
Fighting l
When fighting fires a firefighter must use great caution
Physical appearance of the weird sisters in macbeth
Dove
Liberals vs conservatives apush
Method of fire fighting