Descriptive Diagnostic What happened Why did it happen
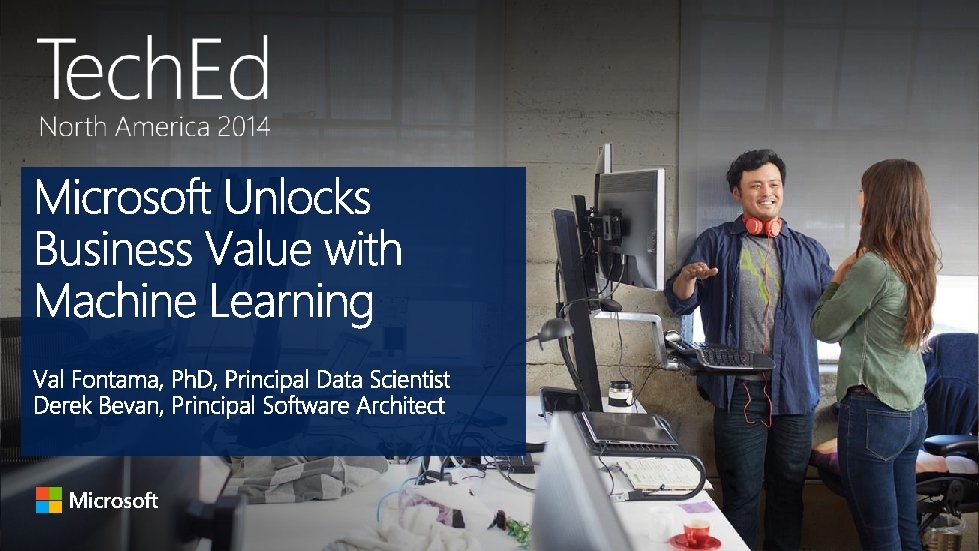
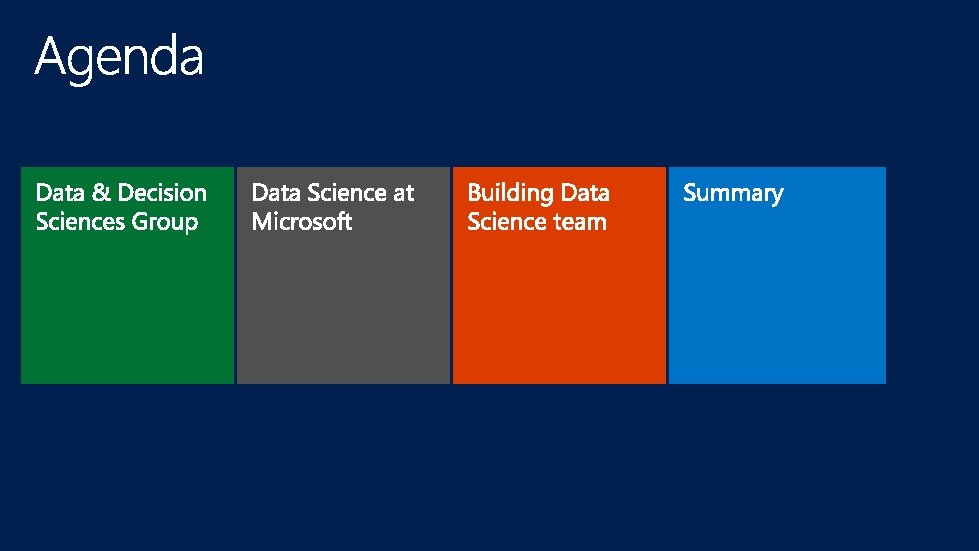
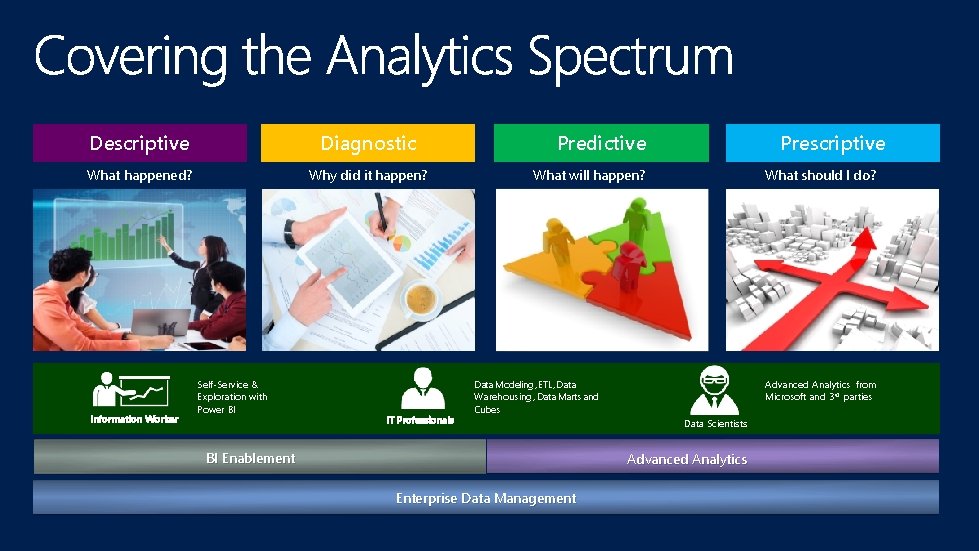
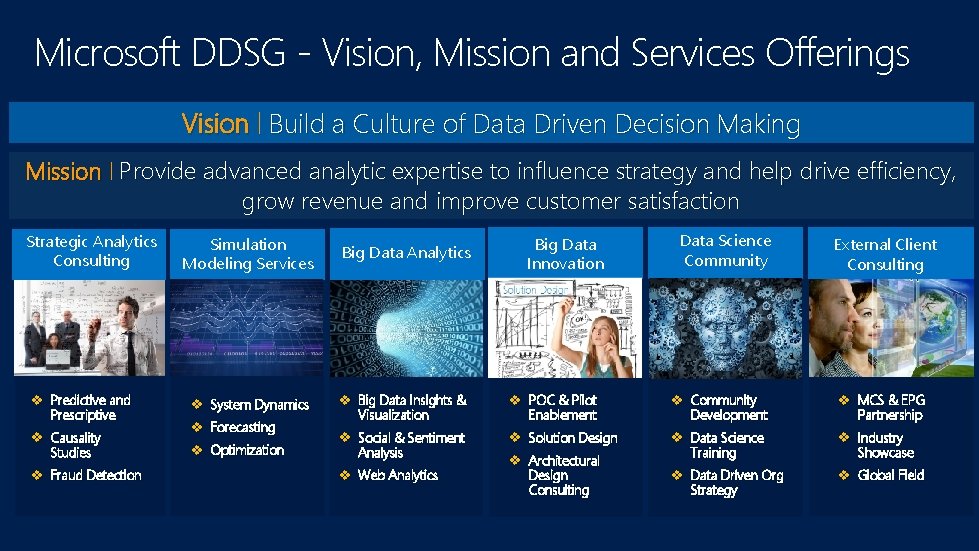
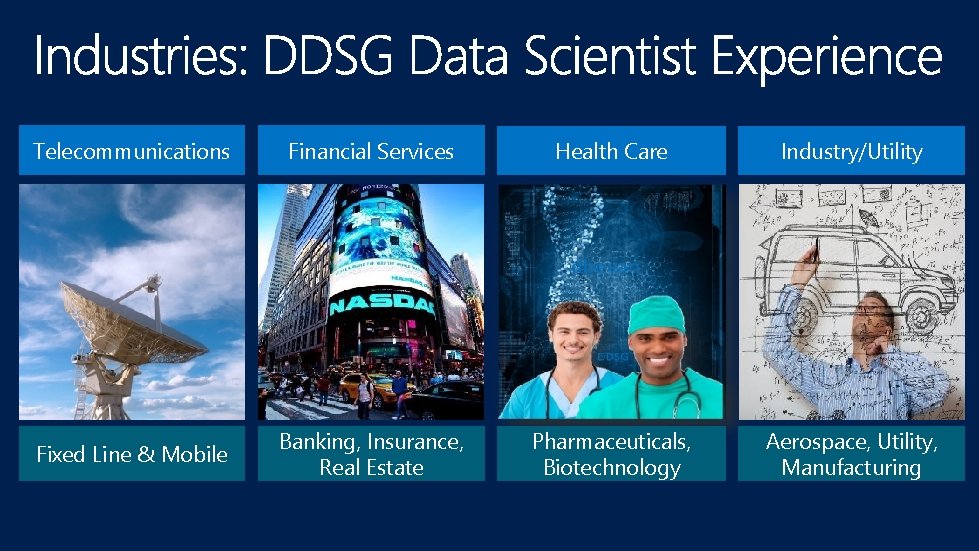
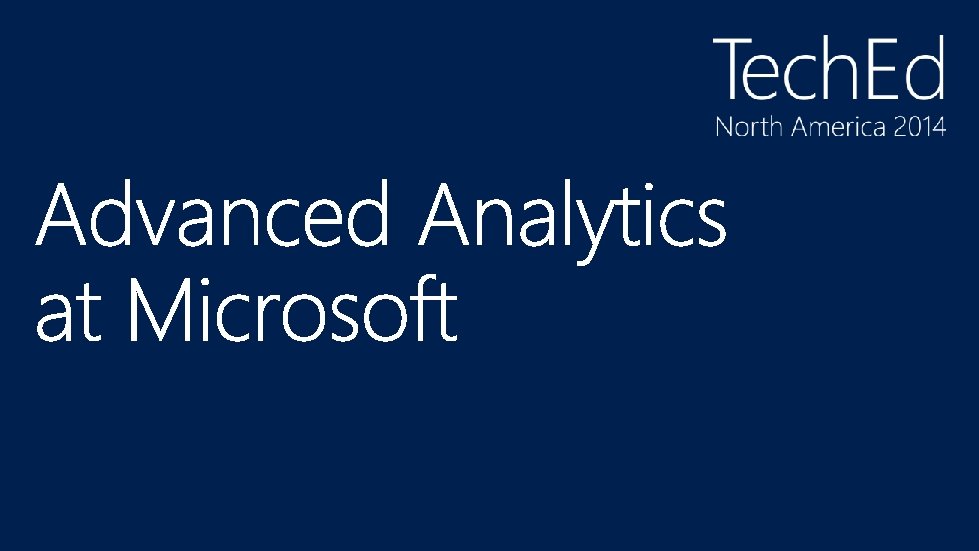
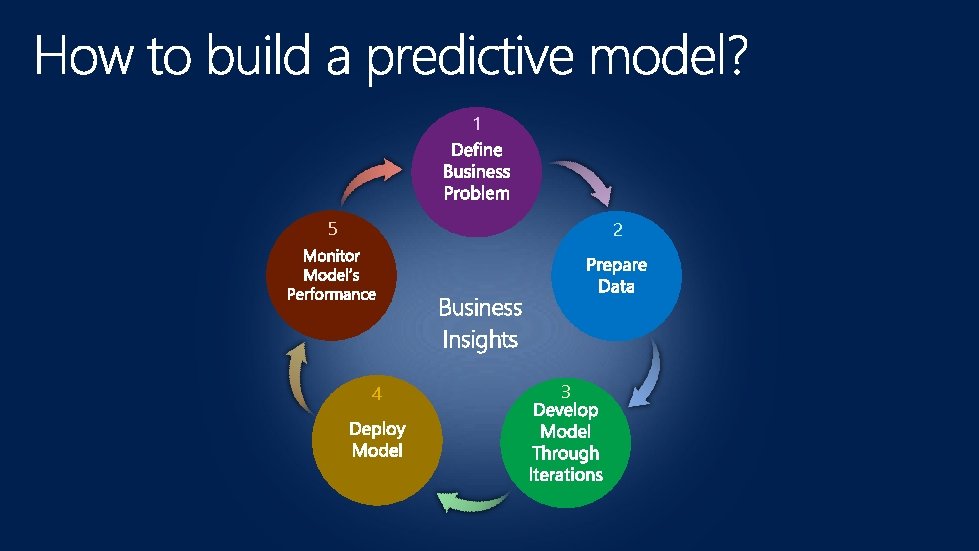
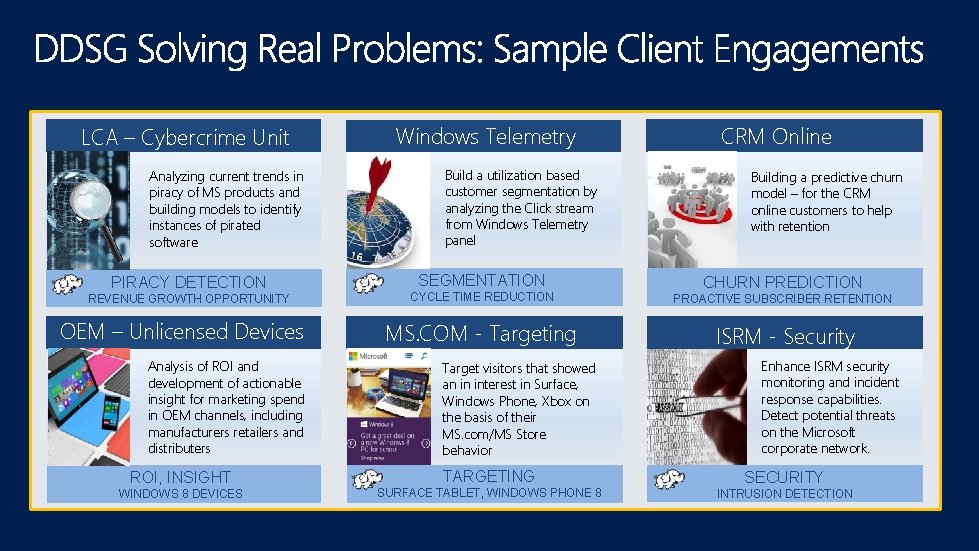
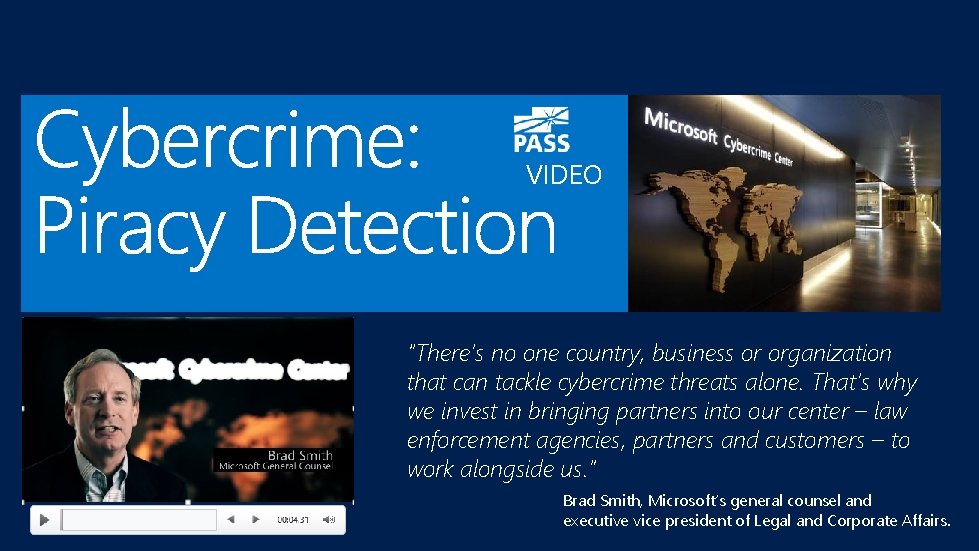
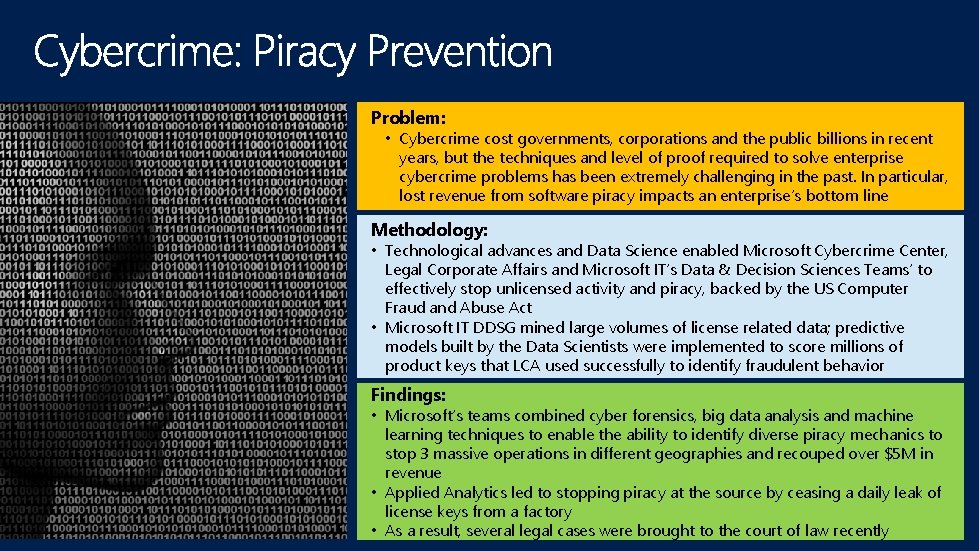
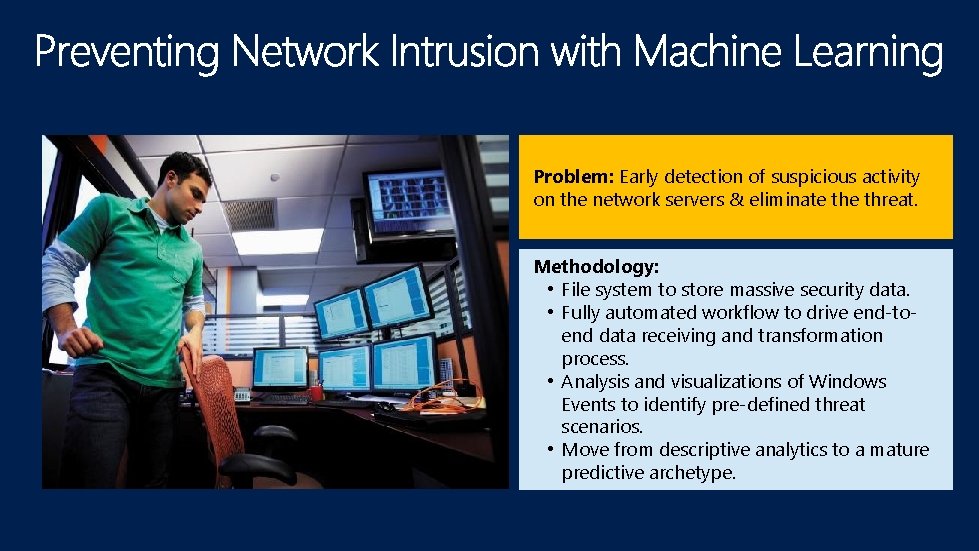
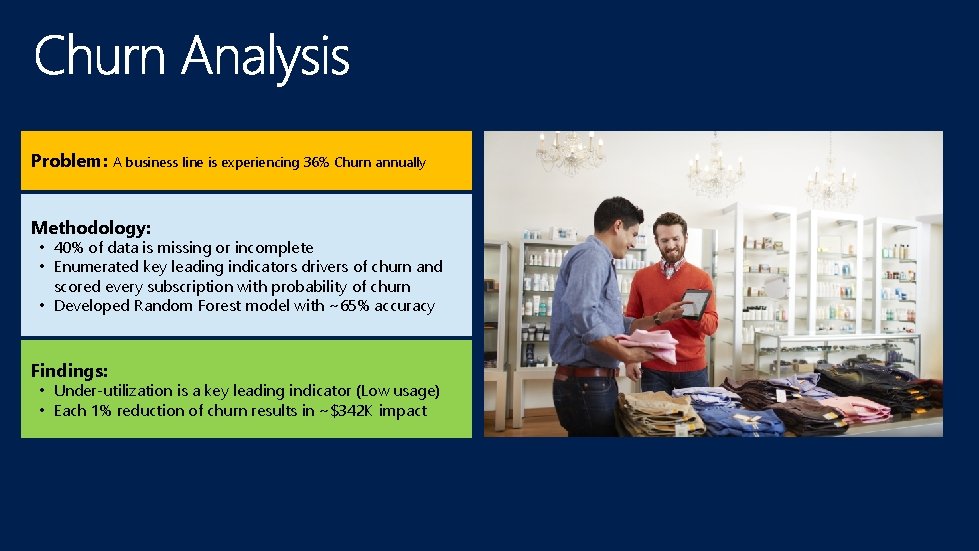
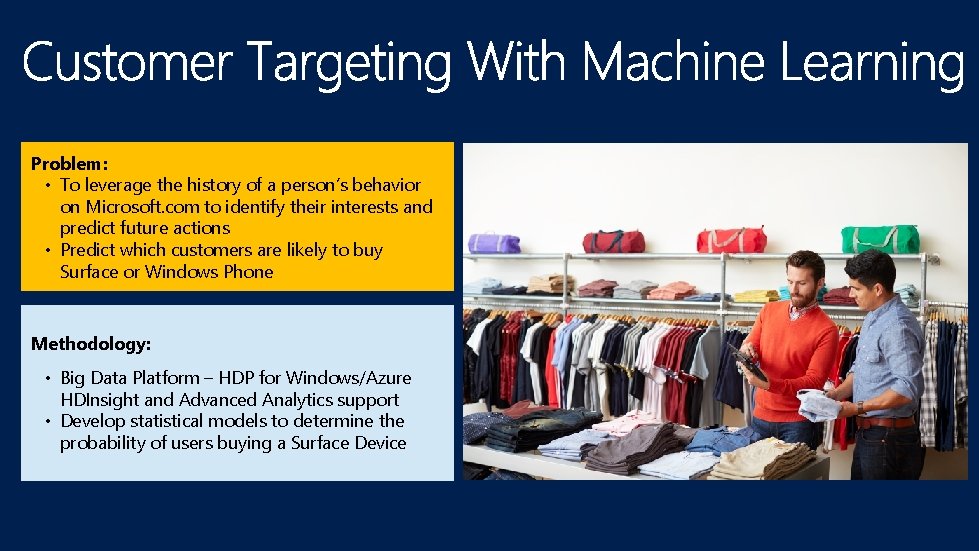
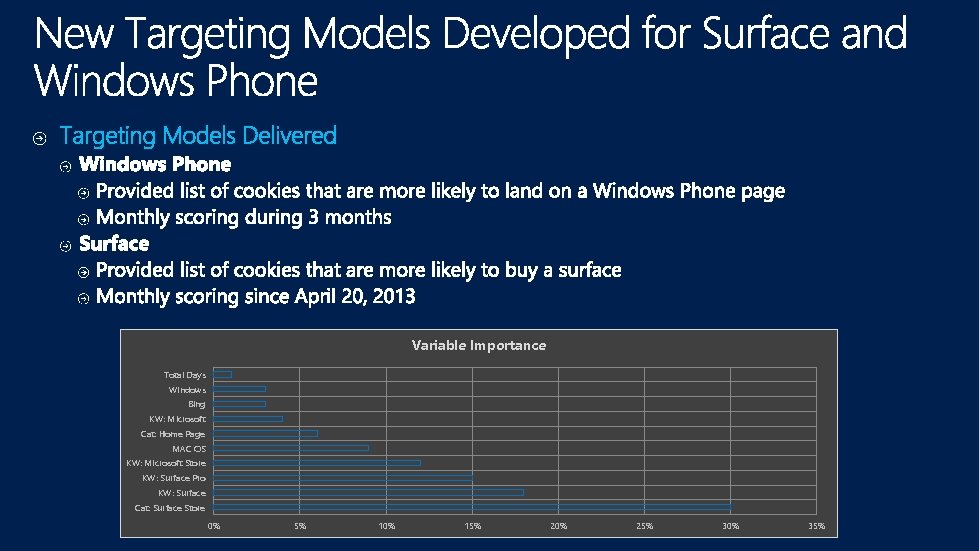
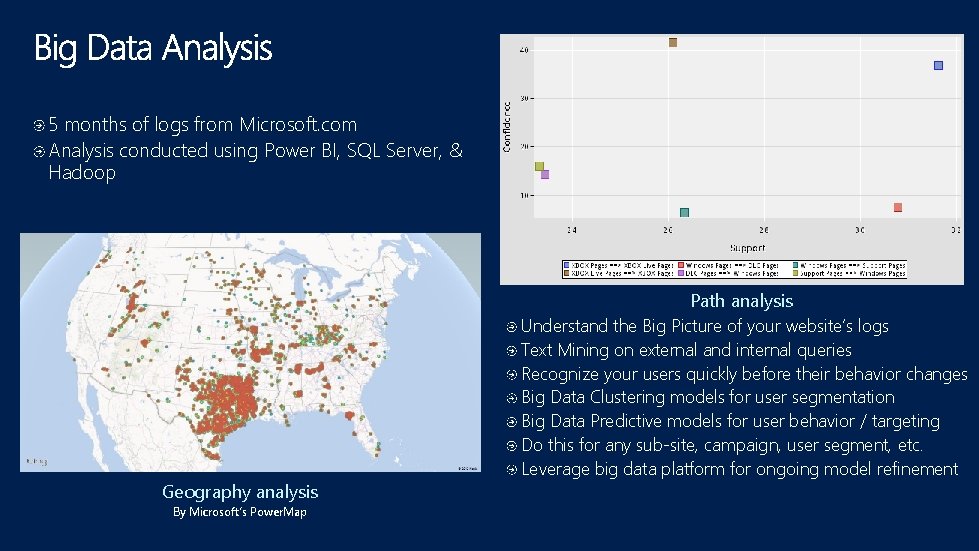
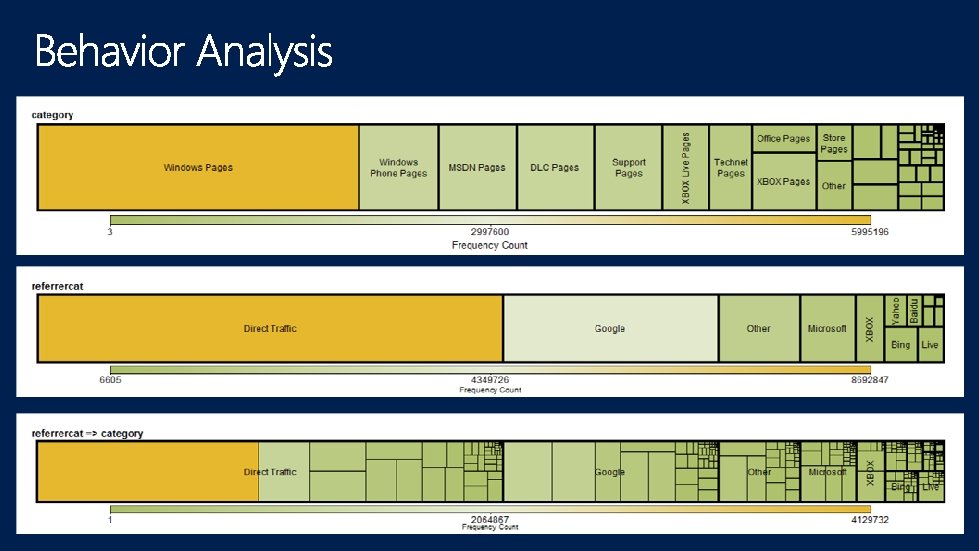
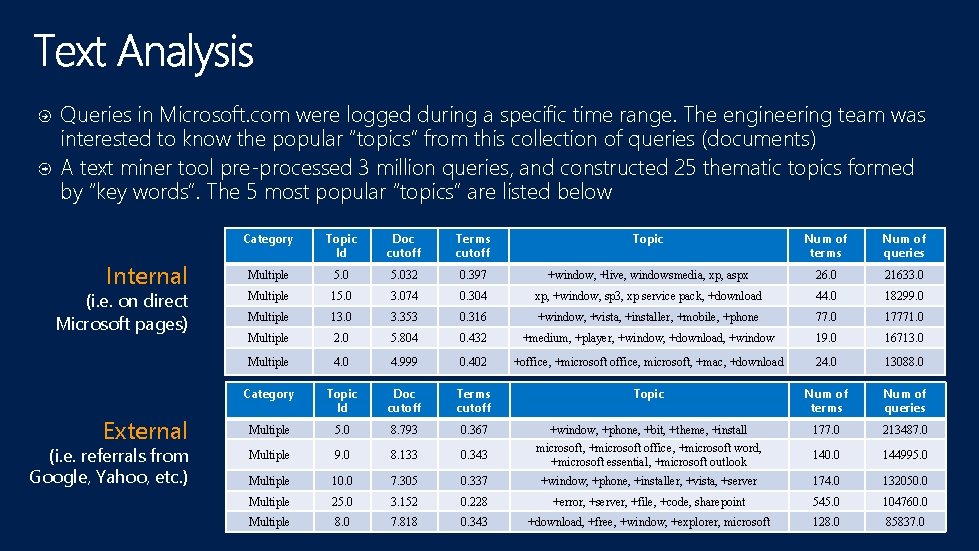
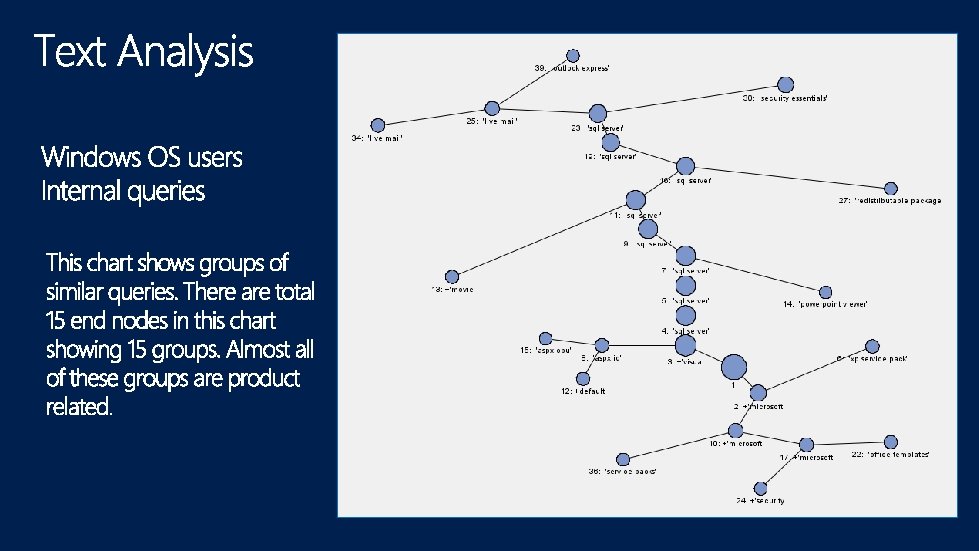
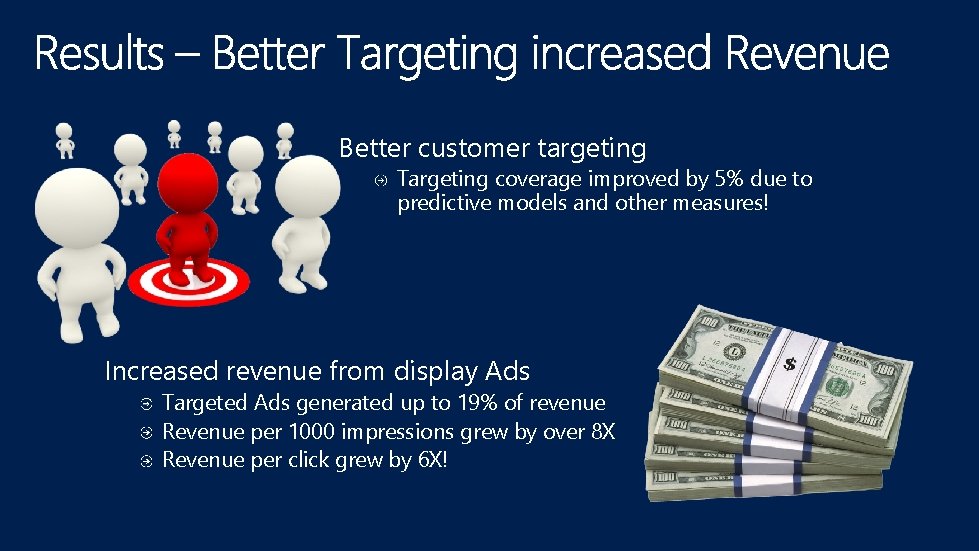
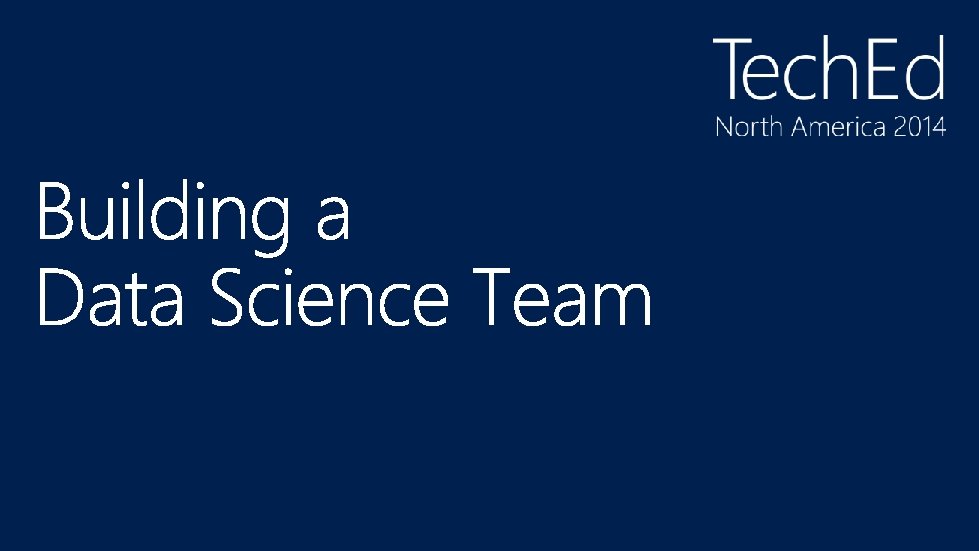
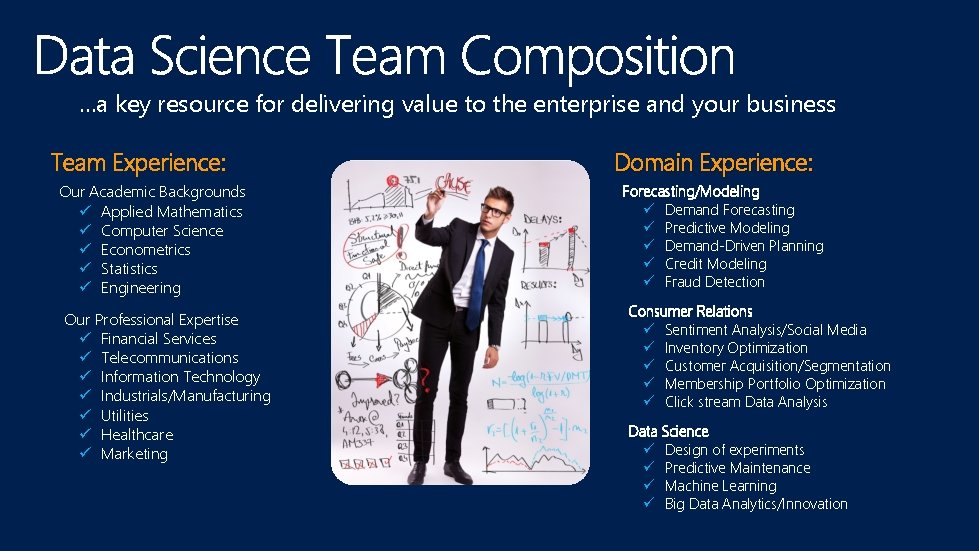
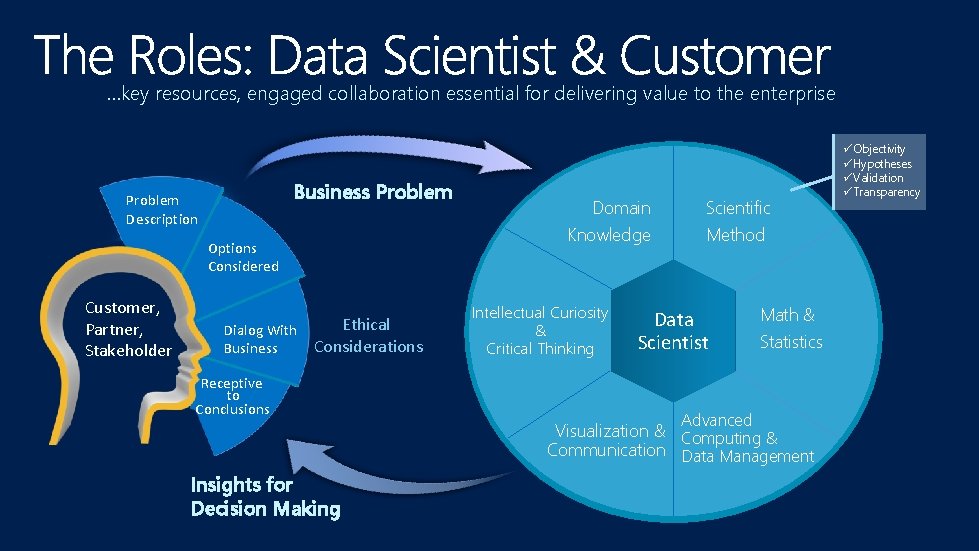
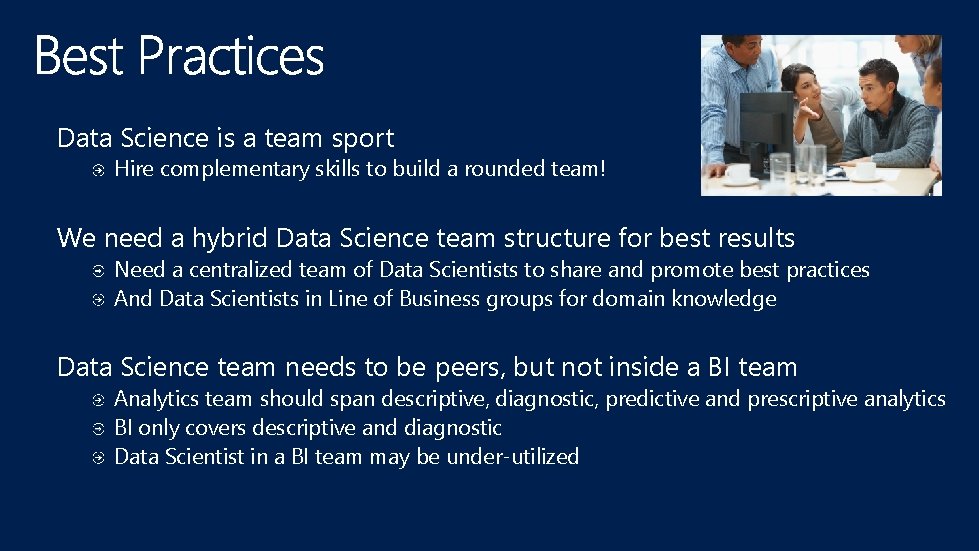
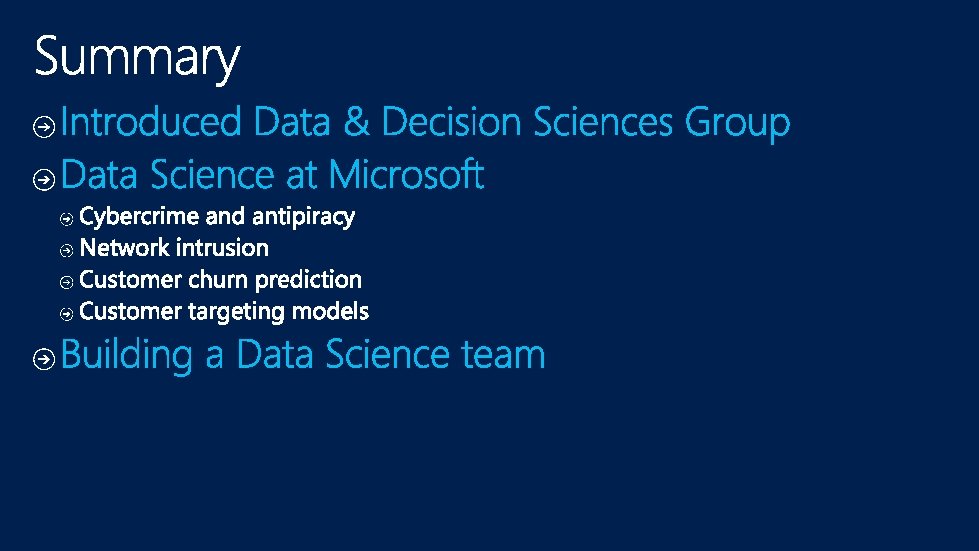
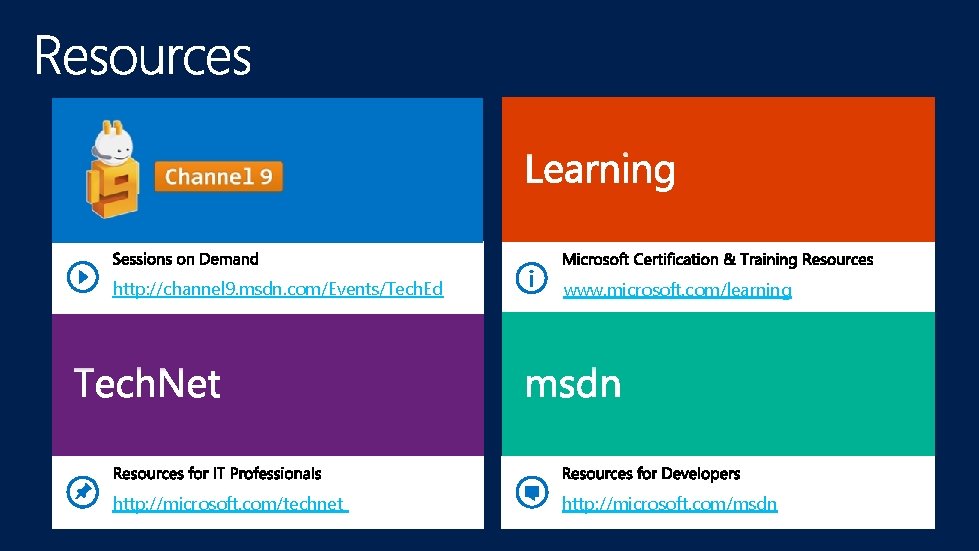
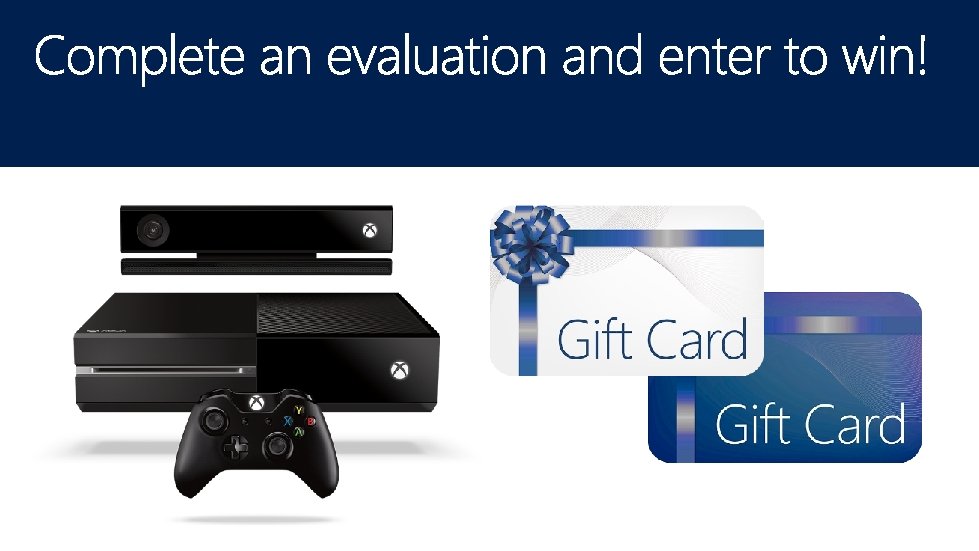
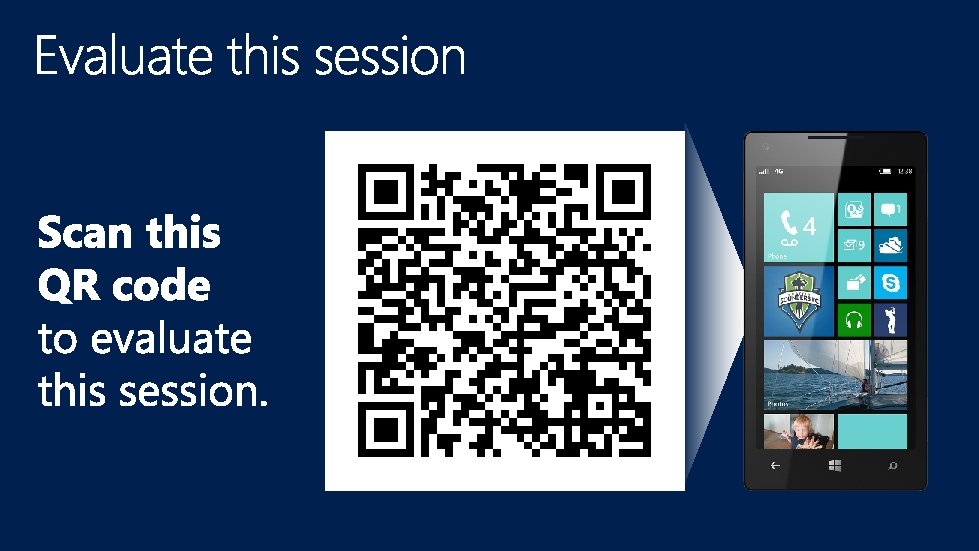
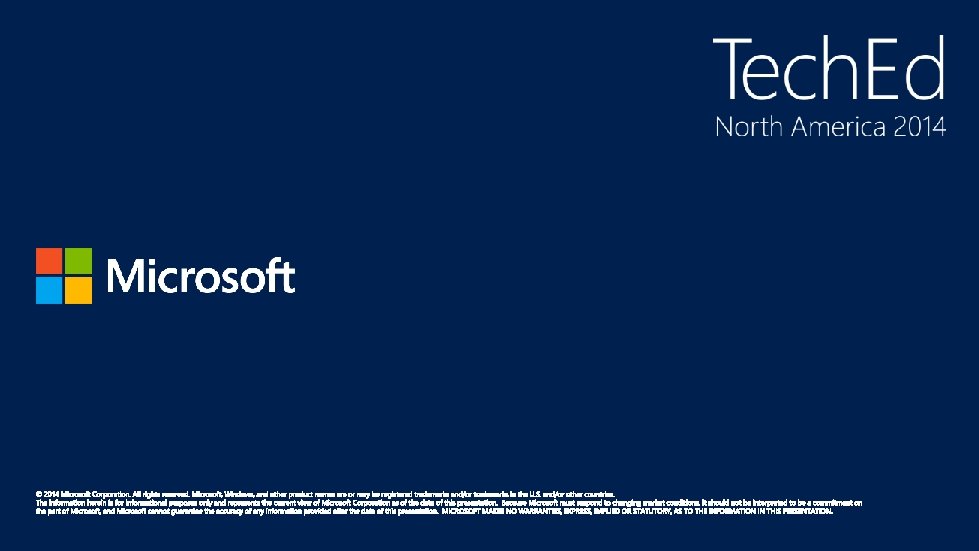
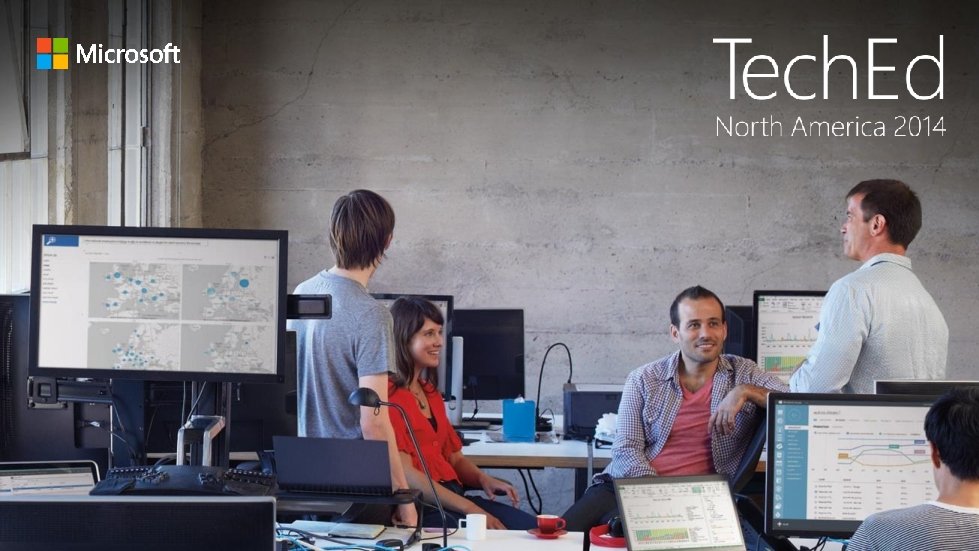
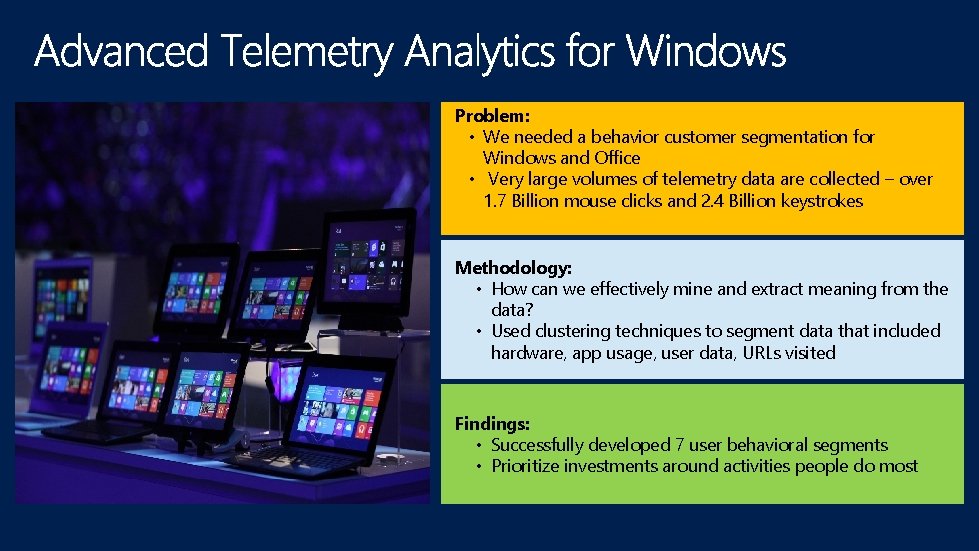
- Slides: 30
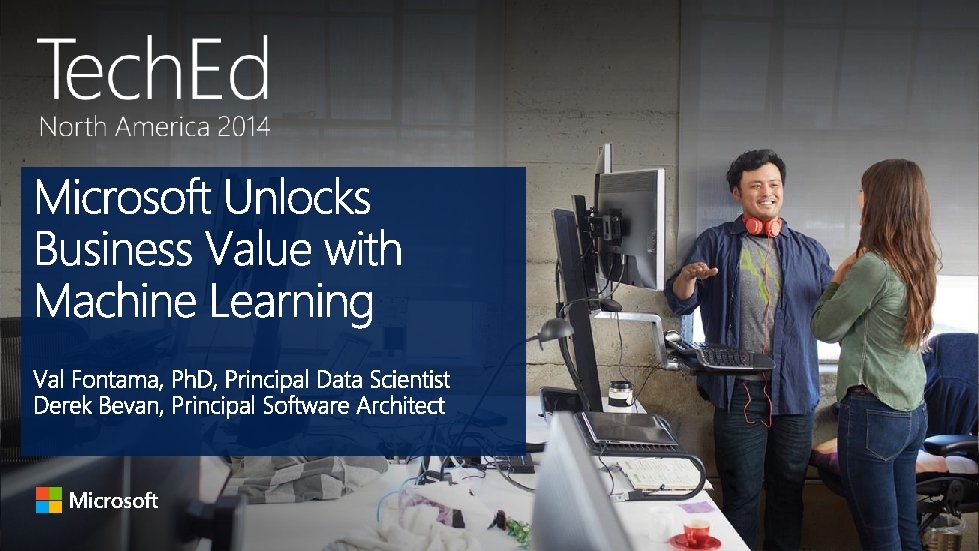
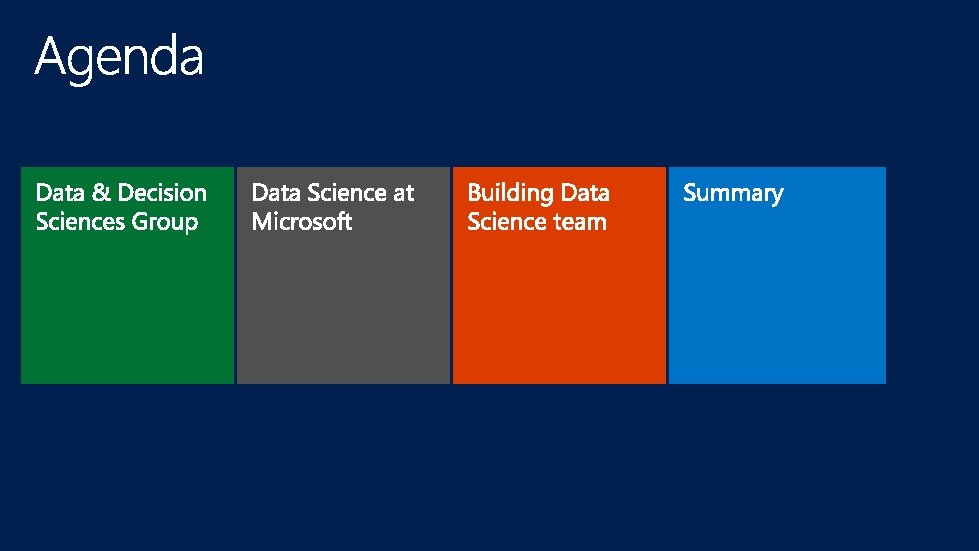
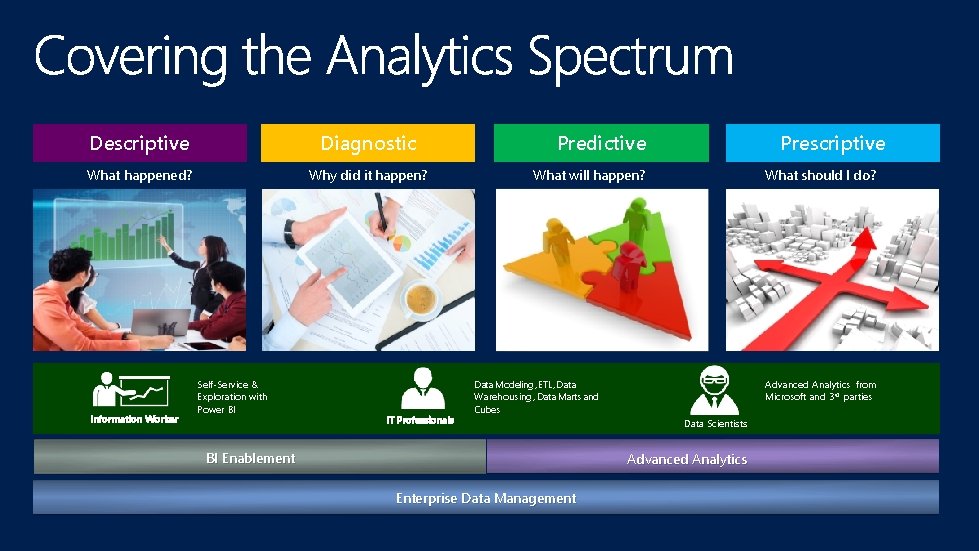
Descriptive Diagnostic What happened? Why did it happen? Information Worker Self-Service & Exploration with Power BI IT Professionals Prescriptive Predictive What will happen? Data Modeling, ETL, Data Warehousing, Data Marts and Cubes BI Enablement What should I do? Advanced Analytics from Microsoft and 3 rd parties Data Scientists Advanced Analytics Enterprise Data Management
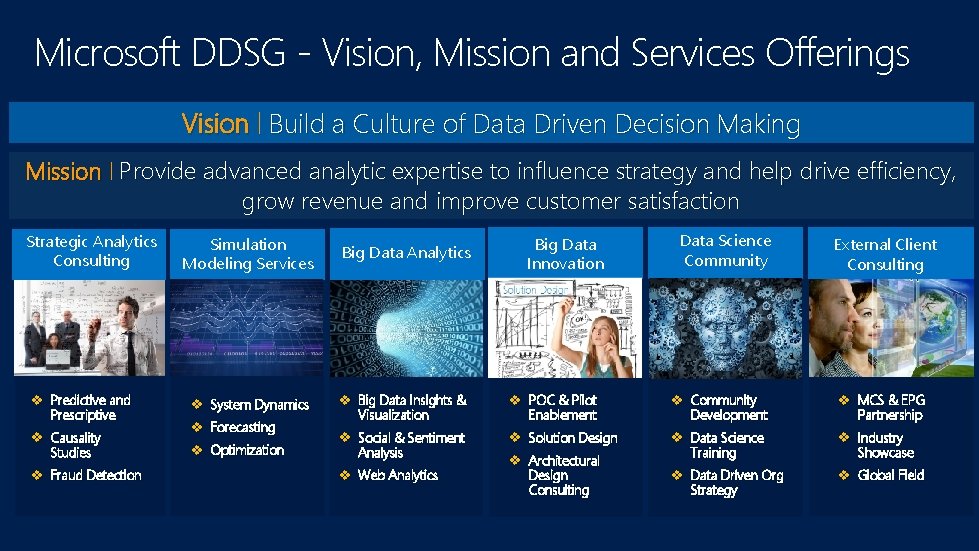
Microsoft DDSG - Vision, Mission and Services Offerings Vision | Build a Culture of Data Driven Decision Making Mission | Provide advanced analytic expertise to influence strategy and help drive efficiency, grow revenue and improve customer satisfaction Strategic Analytics Consulting Data Science Community Simulation Modeling Services Big Data Analytics v Predictive and Prescriptive v System Dynamics v Big Data Insights & Visualization v POC & Pilot Enablement v Community Development v MCS & EPG Partnership v Causality Studies v Optimization v Social & Sentiment Analysis v Solution Design v Data Science Training v Industry Showcase v Data Driven Org Strategy v Global Field v Fraud Detection v Forecasting v Web Analytics Big Data Innovation v Architectural Design Consulting External Client Consulting
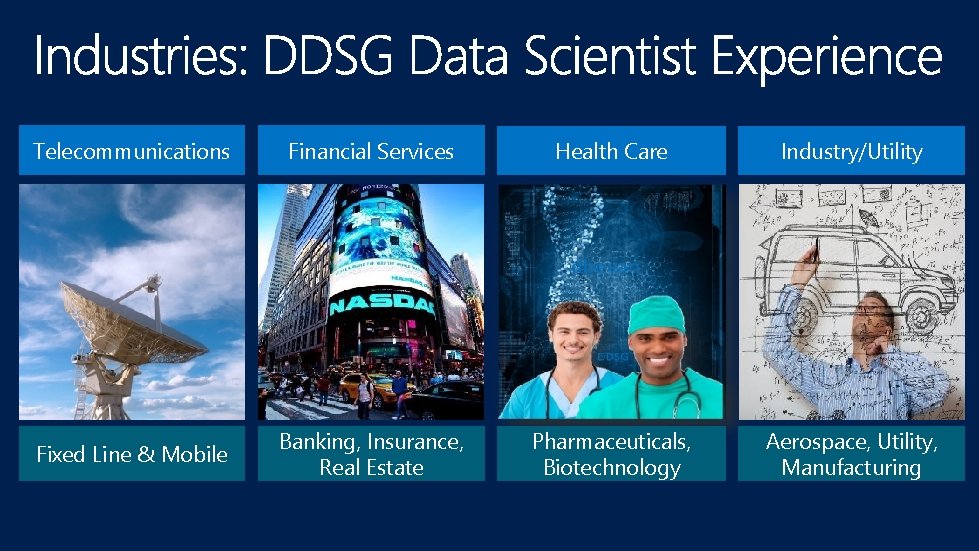
Telecommunications Financial Services Health Care Industry/Utility Fixed Line & Mobile Banking, Insurance, Real Estate Pharmaceuticals, Biotechnology Aerospace, Utility, Manufacturing
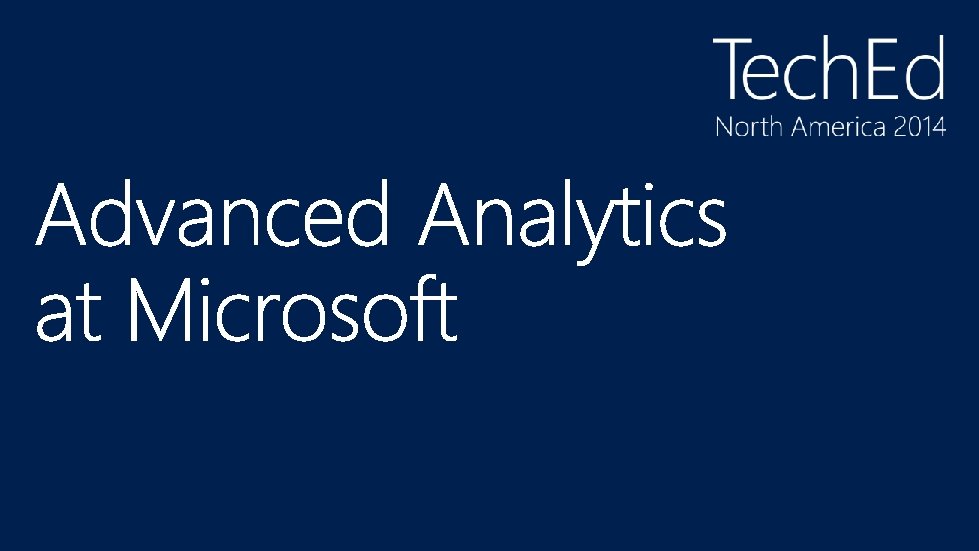
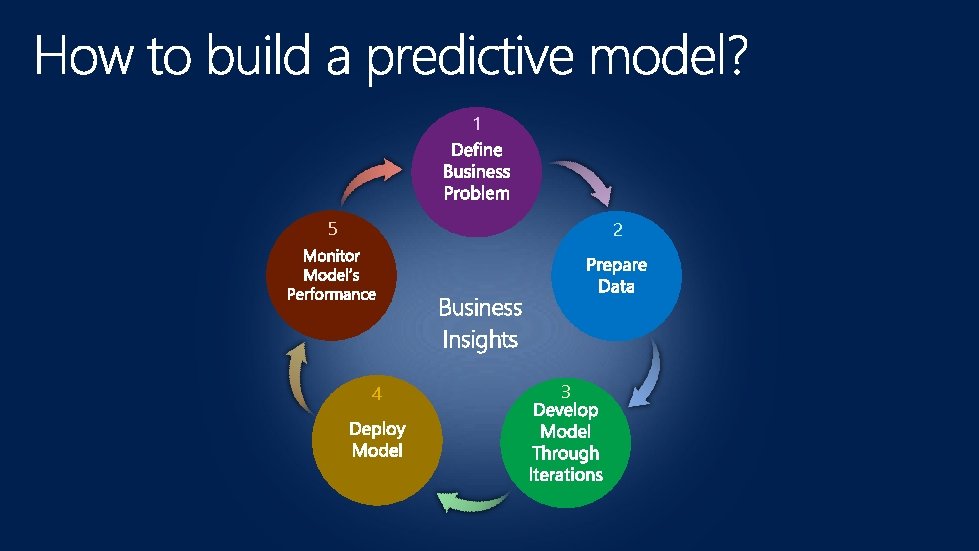
1 5 2 Business Insights 4 3
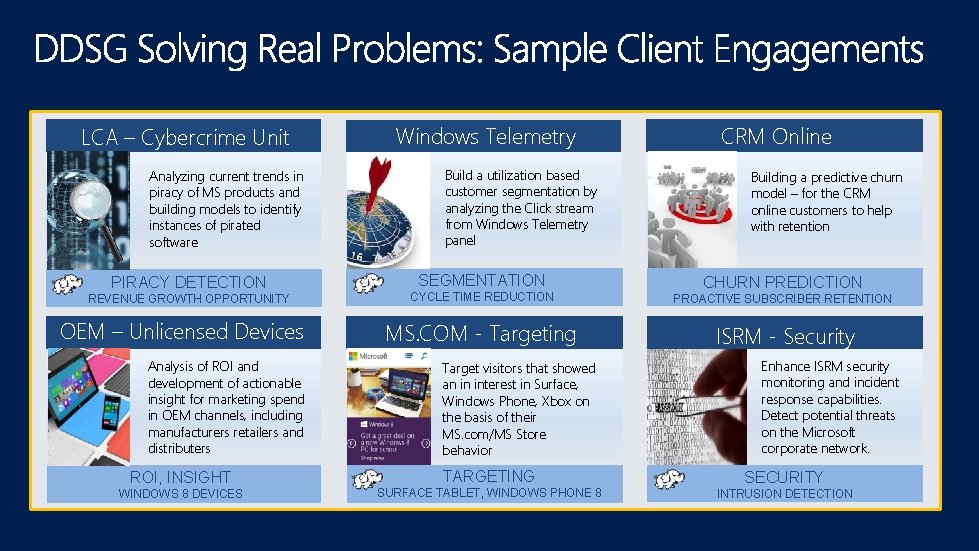
LCA – Cybercrime Unit Analyzing current trends in piracy of MS products and building models to identify instances of pirated software PIRACY DETECTION REVENUE GROWTH OPPORTUNITY OEM – Unlicensed Devices Analysis of ROI and development of actionable insight for marketing spend in OEM channels, including manufacturers retailers and distributers ROI, INSIGHT WINDOWS 8 DEVICES Windows Industry Stats Telemetry Build a utilization based customer segmentation by analyzing the Click stream from Windows Telemetry panel SEGMENTATION CRM Online Building a predictive churn model – for the CRM online customers to help with retention CHURN PREDICTION CYCLE TIME REDUCTION PROACTIVE SUBSCRIBER RETENTION MS. COM - Targeting ISRM - Security Target visitors that showed an in interest in Surface, Windows Phone, Xbox on the basis of their MS. com/MS Store behavior Enhance ISRM security monitoring and incident response capabilities. Detect potential threats on the Microsoft corporate network. TARGETING SECURITY SURFACE TABLET, WINDOWS PHONE 8 INTRUSION DETECTION
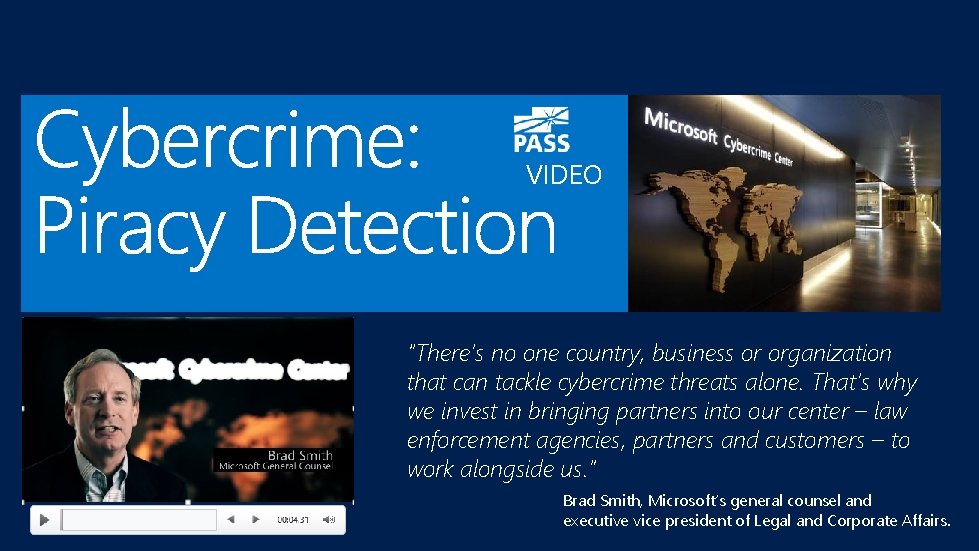
VIDEO “There’s no one country, business or organization that can tackle cybercrime threats alone. That’s why we invest in bringing partners into our center – law enforcement agencies, partners and customers – to work alongside us. ” Brad Smith, Microsoft’s general counsel and executive vice president of Legal and Corporate Affairs.
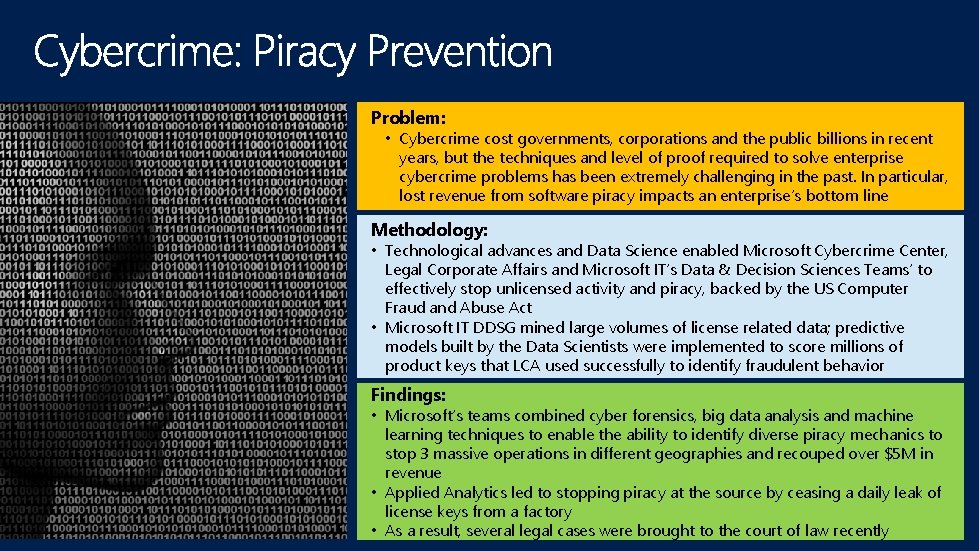
Problem: • Cybercrime cost governments, corporations and the public billions in recent years, but the techniques and level of proof required to solve enterprise cybercrime problems has been extremely challenging in the past. In particular, lost revenue from software piracy impacts an enterprise’s bottom line Methodology: • Technological advances and Data Science enabled Microsoft Cybercrime Center, Legal Corporate Affairs and Microsoft IT’s Data & Decision Sciences Teams’ to effectively stop unlicensed activity and piracy, backed by the US Computer Fraud and Abuse Act • Microsoft IT DDSG mined large volumes of license related data; predictive models built by the Data Scientists were implemented to score millions of product keys that LCA used successfully to identify fraudulent behavior Findings: • Microsoft’s teams combined cyber forensics, big data analysis and machine learning techniques to enable the ability to identify diverse piracy mechanics to stop 3 massive operations in different geographies and recouped over $5 M in revenue • Applied Analytics led to stopping piracy at the source by ceasing a daily leak of license keys from a factory • As a result, several legal cases were brought to the court of law recently
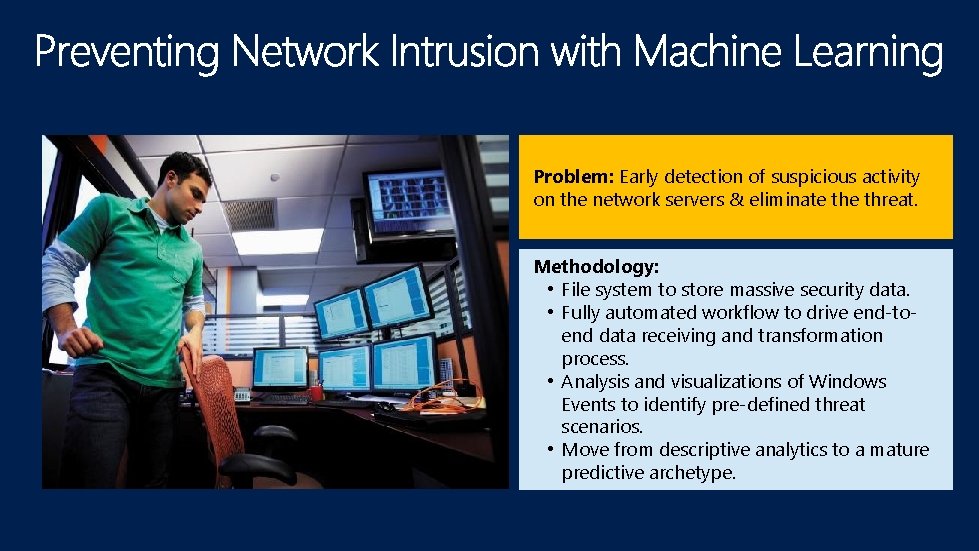
Problem: Early detection of suspicious activity on the network servers & eliminate threat. Methodology: • File system to store massive security data. • Fully automated workflow to drive end-toend data receiving and transformation process. • Analysis and visualizations of Windows Events to identify pre-defined threat scenarios. • Move from descriptive analytics to a mature predictive archetype.
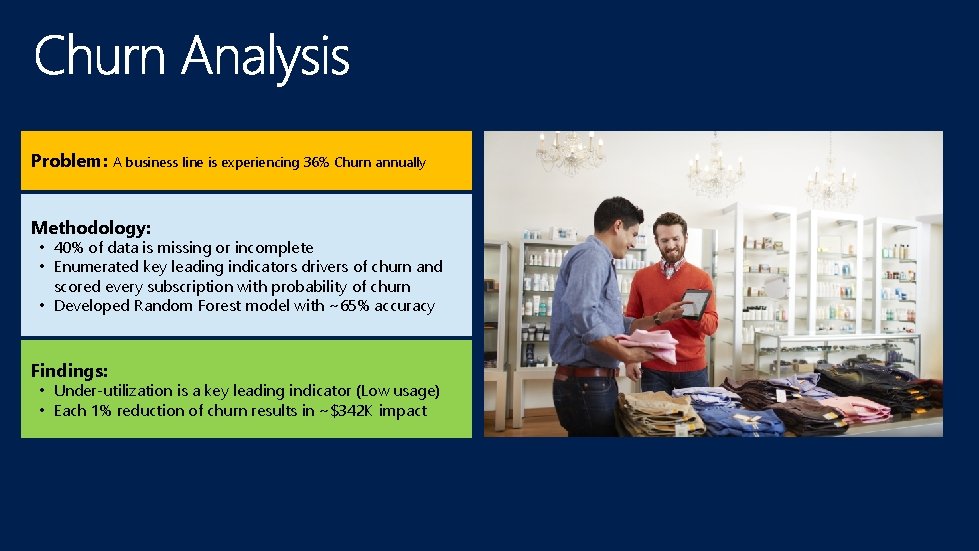
Problem: A business line is experiencing 36% Churn annually Methodology: • 40% of data is missing or incomplete • Enumerated key leading indicators drivers of churn and scored every subscription with probability of churn • Developed Random Forest model with ~65% accuracy Findings: • Under-utilization is a key leading indicator (Low usage) • Each 1% reduction of churn results in ~$342 K impact
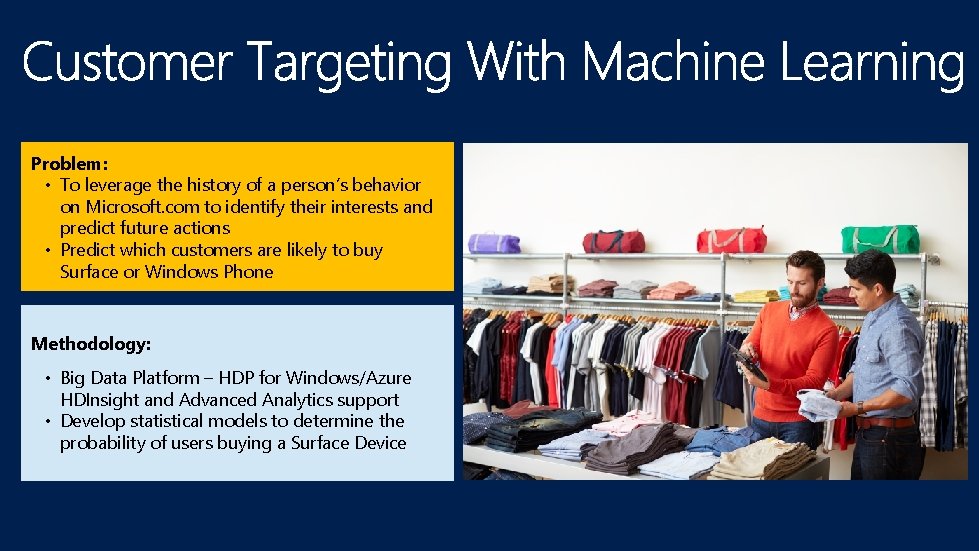
Problem: • To leverage the history of a person’s behavior on Microsoft. com to identify their interests and predict future actions • Predict which customers are likely to buy Surface or Windows Phone Methodology: • Big Data Platform – HDP for Windows/Azure HDInsight and Advanced Analytics support • Develop statistical models to determine the probability of users buying a Surface Device
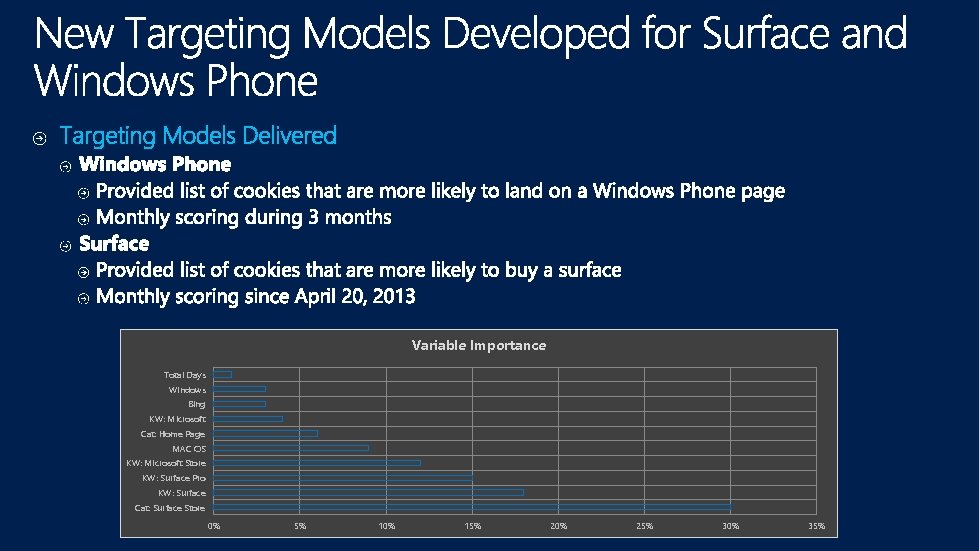
Variable Importance Total Days Windows Bing KW: Microsoft Cat: Home Page MAC OS KW: Microsoft Store KW: Surface Pro KW: Surface Cat: Surface Store 0% 5% 10% 15% 20% 25% 30% 35%
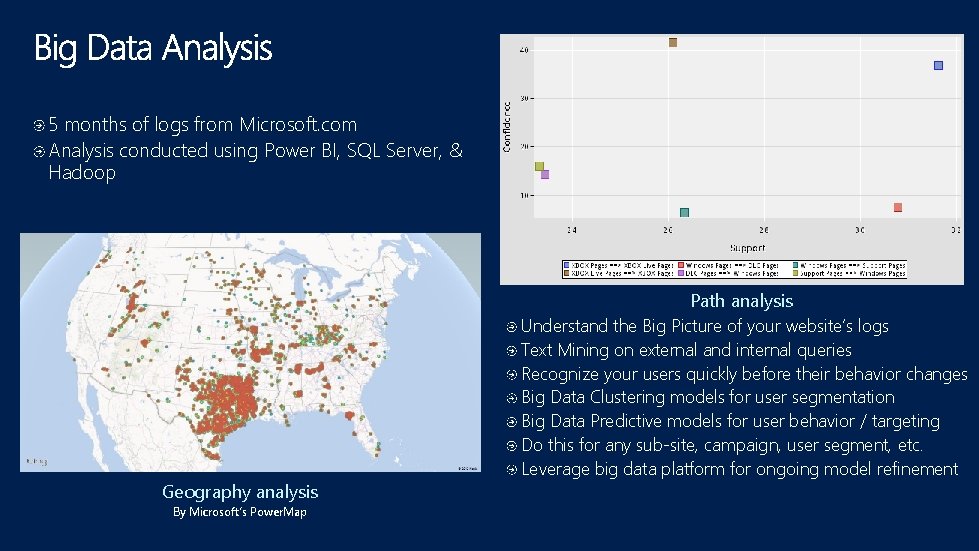
5 months of logs from Microsoft. com Analysis conducted using Power BI, SQL Server, & Hadoop Geography analysis By Microsoft’s Power. Map Path analysis Understand the Big Picture of your website’s logs Text Mining on external and internal queries Recognize your users quickly before their behavior changes Big Data Clustering models for user segmentation Big Data Predictive models for user behavior / targeting Do this for any sub-site, campaign, user segment, etc. Leverage big data platform for ongoing model refinement
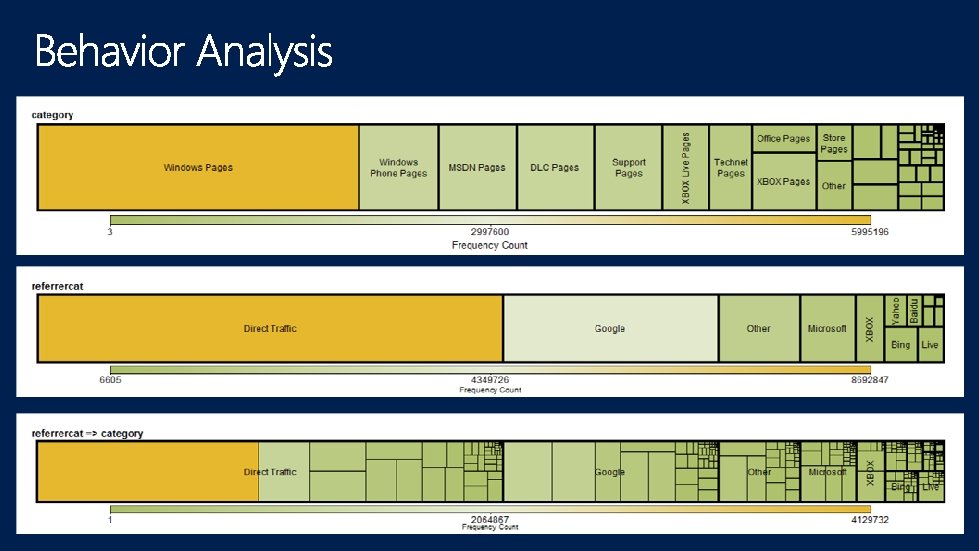
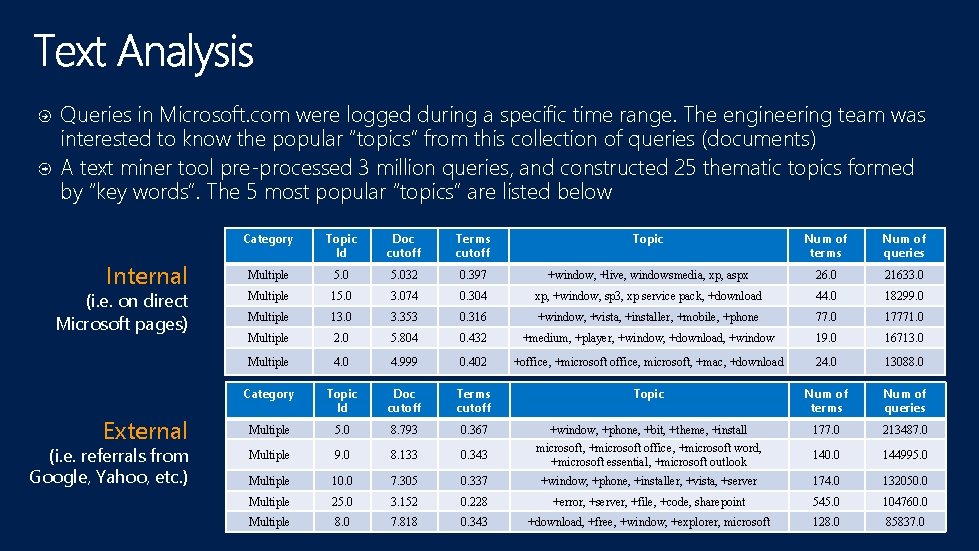
Queries in Microsoft. com were logged during a specific time range. The engineering team was interested to know the popular “topics” from this collection of queries (documents) A text miner tool pre-processed 3 million queries, and constructed 25 thematic topics formed by “key words”. The 5 most popular “topics” are listed below Internal (i. e. on direct Microsoft pages) External (i. e. referrals from Google, Yahoo, etc. ) Category Topic Id Doc cutoff Terms cutoff Topic Num of terms Num of queries Multiple 5. 032 0. 397 +window, +live, windowsmedia, xp, aspx 26. 0 21633. 0 Multiple 15. 0 3. 074 0. 304 xp, +window, sp 3, xp service pack, +download 44. 0 18299. 0 Multiple 13. 0 3. 353 0. 316 +window, +vista, +installer, +mobile, +phone 77. 0 17771. 0 Multiple 2. 0 5. 804 0. 432 +medium, +player, +window, +download, +window 19. 0 16713. 0 Multiple 4. 0 4. 999 0. 402 +office, +microsoft office, microsoft, +mac, +download 24. 0 13088. 0 Category Topic Id Doc cutoff Terms cutoff Topic Num of terms Num of queries Multiple 5. 0 8. 793 0. 367 +window, +phone, +bit, +theme, +install 177. 0 213487. 0 Multiple 9. 0 8. 133 0. 343 microsoft, +microsoft office, +microsoft word, +microsoft essential, +microsoft outlook 140. 0 144995. 0 Multiple 10. 0 7. 305 0. 337 +window, +phone, +installer, +vista, +server 174. 0 132050. 0 Multiple 25. 0 3. 152 0. 228 +error, +server, +file, +code, sharepoint 545. 0 104760. 0 Multiple 8. 0 7. 818 0. 343 +download, +free, +window, +explorer, microsoft 128. 0 85837. 0
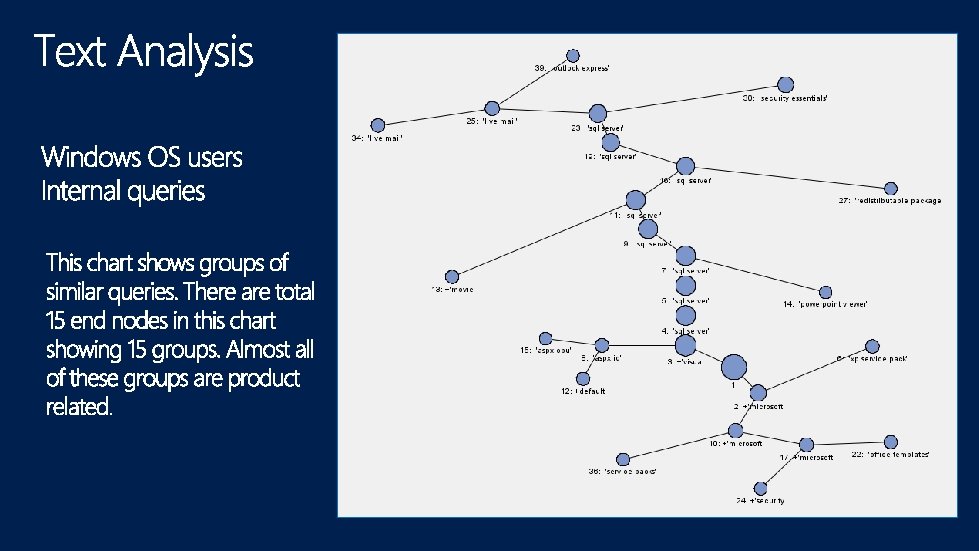
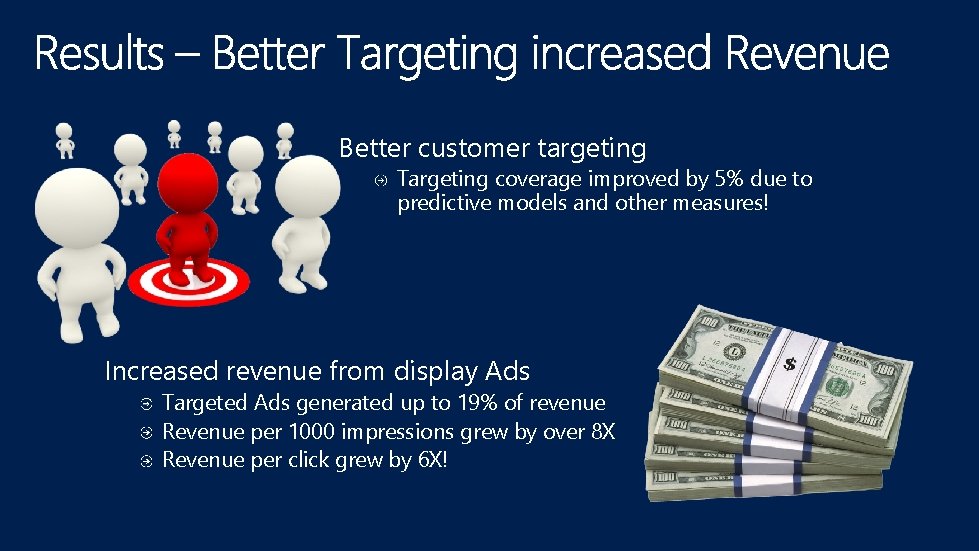
Better customer targeting Targeting coverage improved by 5% due to predictive models and other measures! Increased revenue from display Ads Targeted Ads generated up to 19% of revenue Revenue per 1000 impressions grew by over 8 X Revenue per click grew by 6 X!
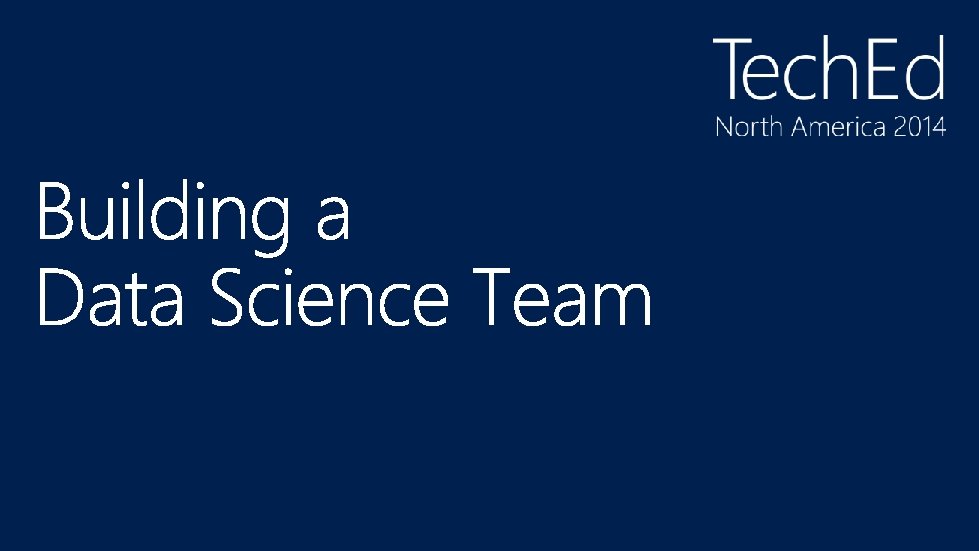
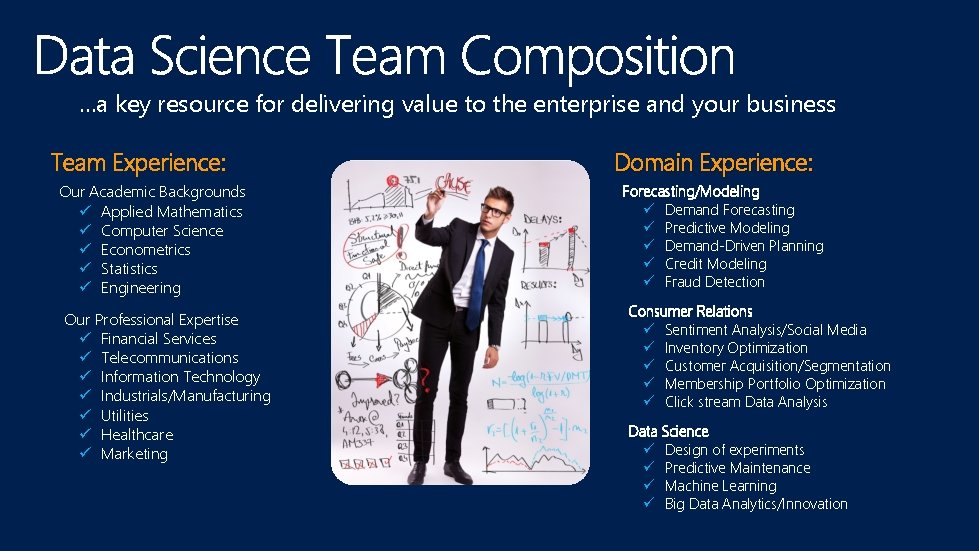
…a key resource for delivering value to the enterprise and your business Team Experience: Our Academic Backgrounds ü Applied Mathematics ü Computer Science ü Econometrics ü Statistics ü Engineering Our Professional Expertise ü Financial Services ü Telecommunications ü Information Technology ü Industrials/Manufacturing ü Utilities ü Healthcare ü Marketing Domain Experience: Forecasting/Modeling ü Demand Forecasting ü Predictive Modeling ü Demand-Driven Planning ü Credit Modeling ü Fraud Detection Consumer Relations ü Sentiment Analysis/Social Media ü Inventory Optimization ü Customer Acquisition/Segmentation ü Membership Portfolio Optimization ü Click stream Data Analysis Data Science ü Design of experiments ü Predictive Maintenance ü Machine Learning ü Big Data Analytics/Innovation
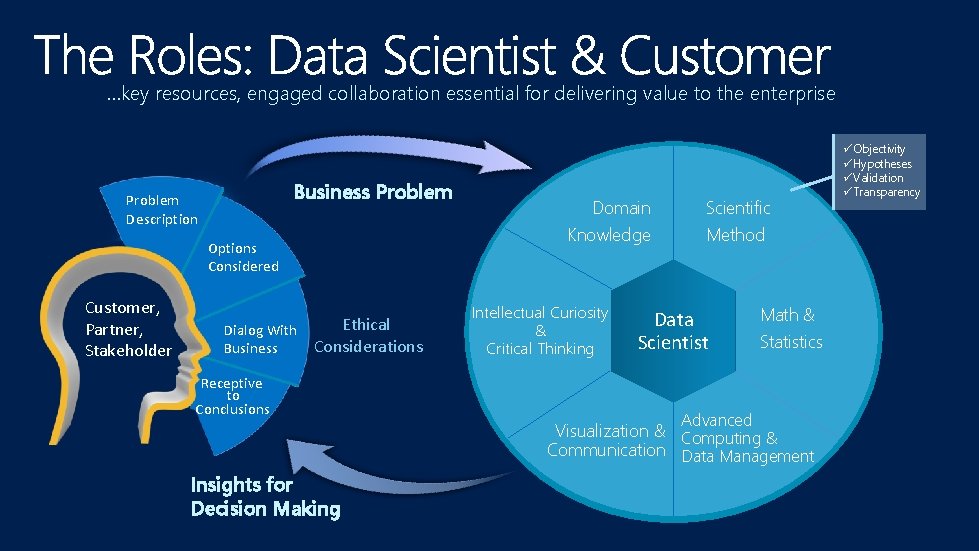
…key resources, engaged collaboration essential for delivering value to the enterprise Business Problem Description Options Considered Customer, Partner, Stakeholder Ethical Dialog With Considerations Business Receptive to Conclusions Insights for Decision Making Domain Knowledge Intellectual Curiosity & Critical Thinking Scientific Method Data Scientist Advanced Math & Statistics Visualization & Computing & Communication Data Management ü Objectivity ü Hypotheses ü Validation ü Transparency
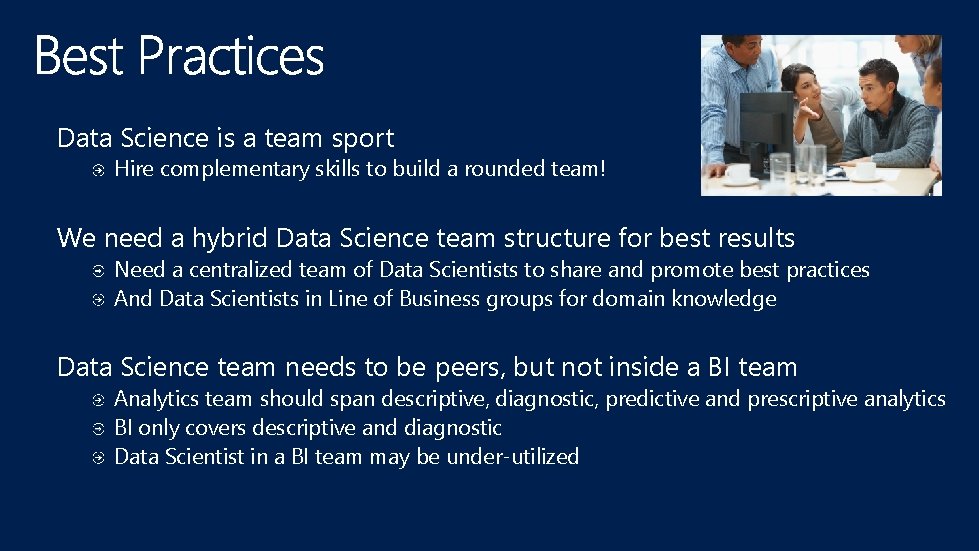
Data Science is a team sport Hire complementary skills to build a rounded team! We need a hybrid Data Science team structure for best results Need a centralized team of Data Scientists to share and promote best practices And Data Scientists in Line of Business groups for domain knowledge Data Science team needs to be peers, but not inside a BI team Analytics team should span descriptive, diagnostic, predictive and prescriptive analytics BI only covers descriptive and diagnostic Data Scientist in a BI team may be under-utilized
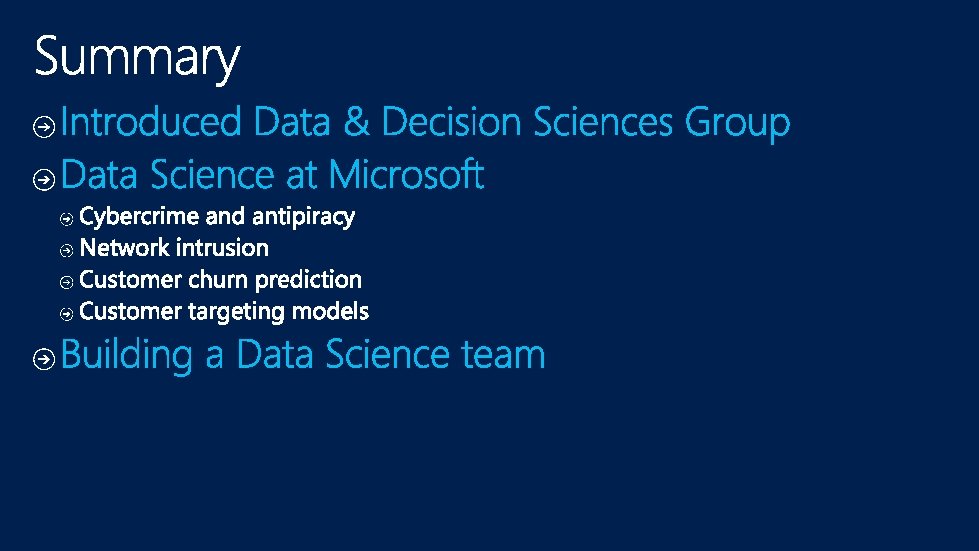
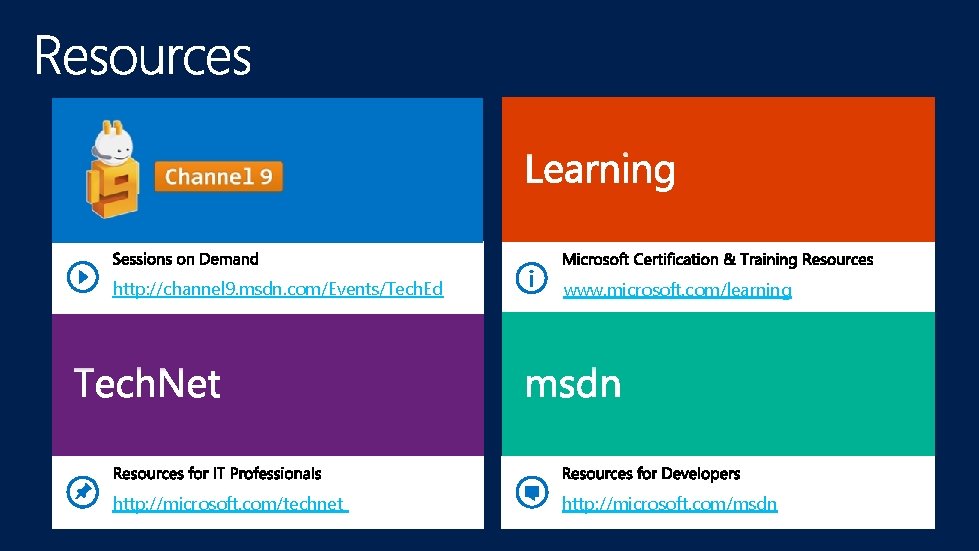
http: //channel 9. msdn. com/Events/Tech. Ed www. microsoft. com/learning http: //microsoft. com/technet http: //microsoft. com/msdn
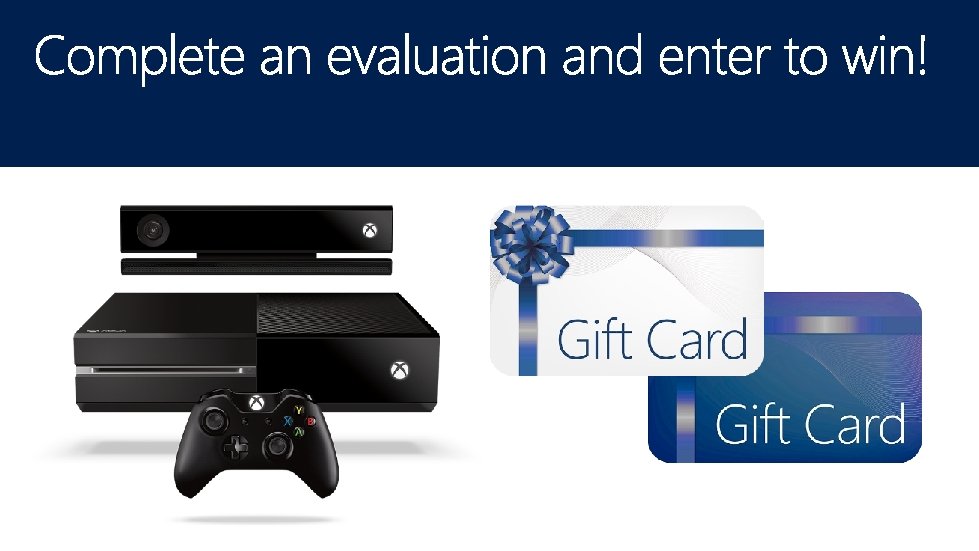
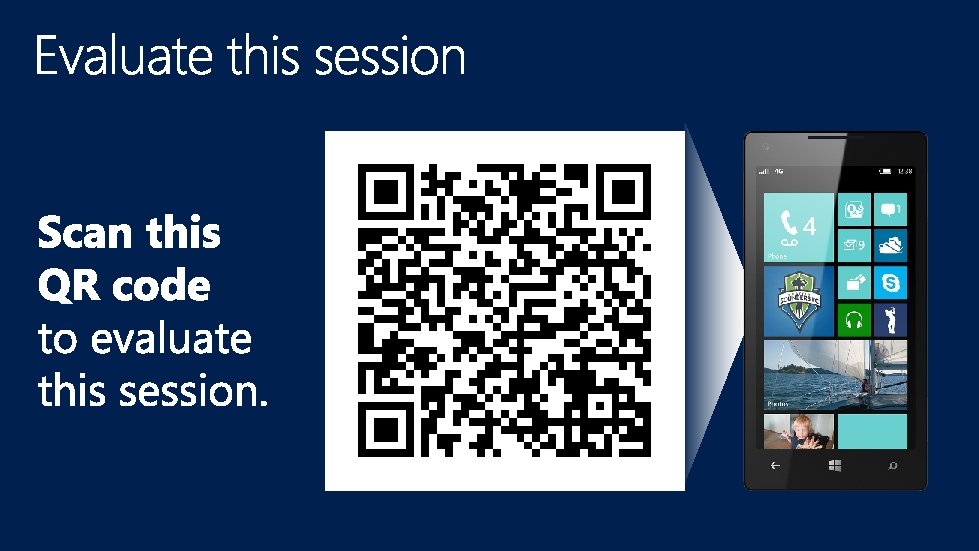
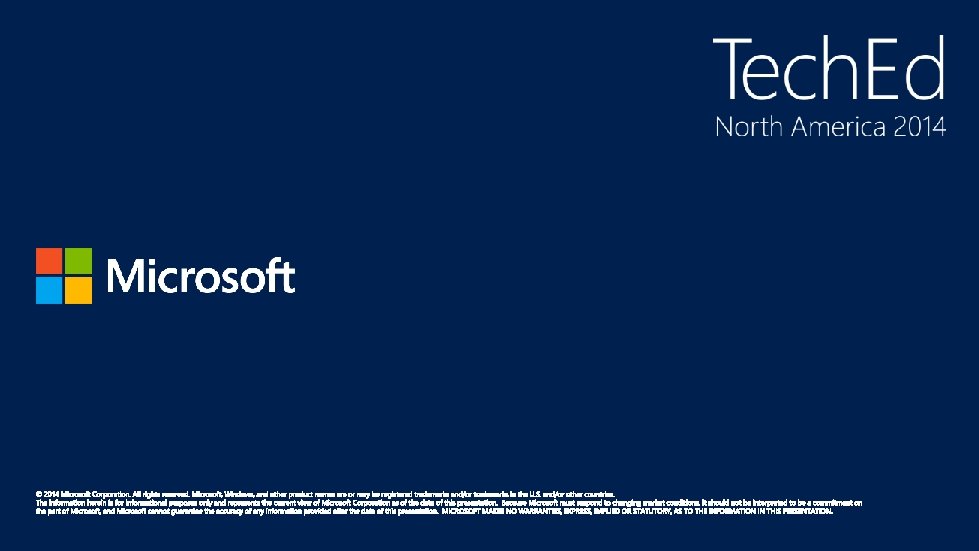
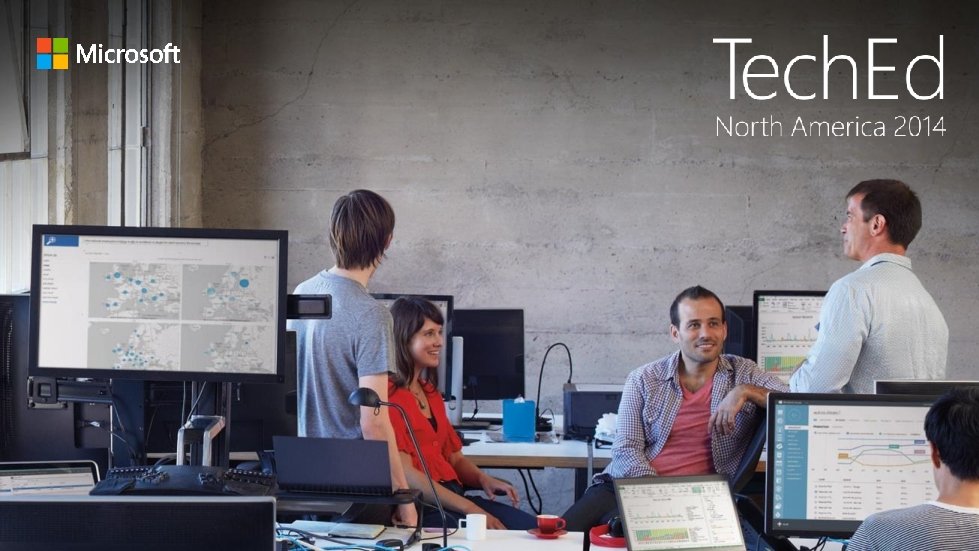
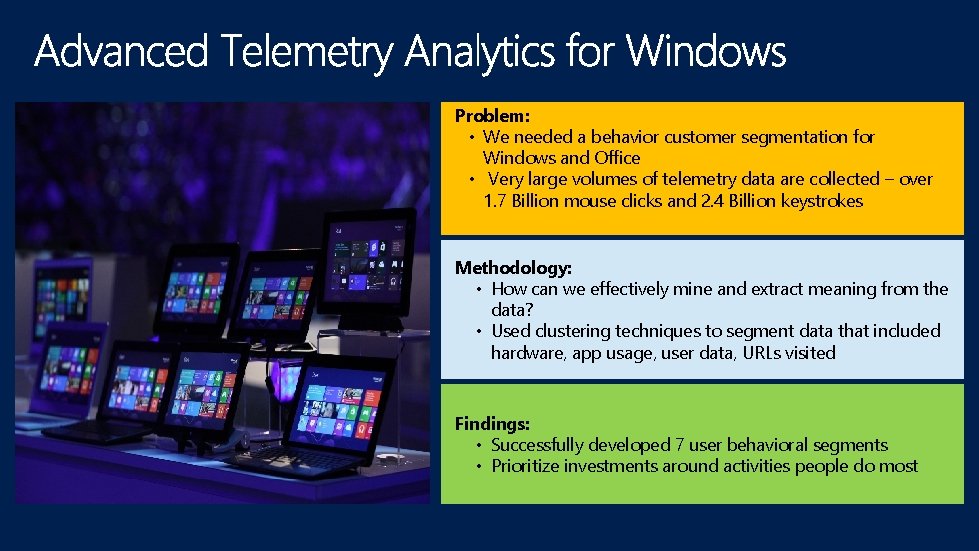
Problem: • We needed a behavior customer segmentation for Windows and Office • Very large volumes of telemetry data are collected – over 1. 7 Billion mouse clicks and 2. 4 Billion keystrokes Methodology: • How can we effectively mine and extract meaning from the data? • Used clustering techniques to segment data that included hardware, app usage, user data, URLs visited Findings: • Successfully developed 7 user behavioral segments • Prioritize investments around activities people do most
In which things
Boscastle flood facts
Trench foot
Why did the homestead strike turn violent?
When did the mayflower compact happen
Why did this happen
1605
What happens during the reign of terror
Why did operation rolling thunder happen
When big bang happened
Why did the battle of lexington and concord happen
Explain why did it happen
Munster plantation
Hey hey bye bye
Where did happen
When did this happen
Where did the 2015 nepal earthquake happen
Where did the battle of vicksburg happen
When did it happen
Where did this happen
When did it happen
Where did the dust bowl happen
Trenton battle date
Why does it happen
Why does this happen
Why does it happen
Modern physics vs classical physics
Why convection cannot happen in solids
Why do things happen the way they do
Why does happen
What happened? why _____ they _____? *