Demand Forecasting What is Forecasting n Process of
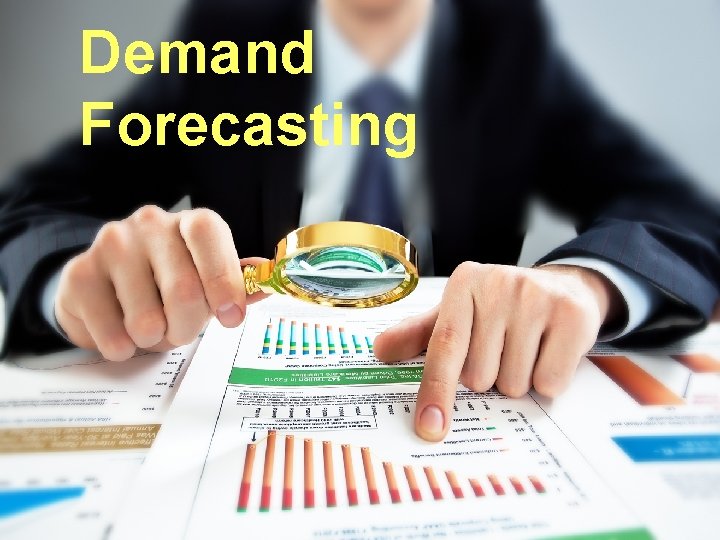
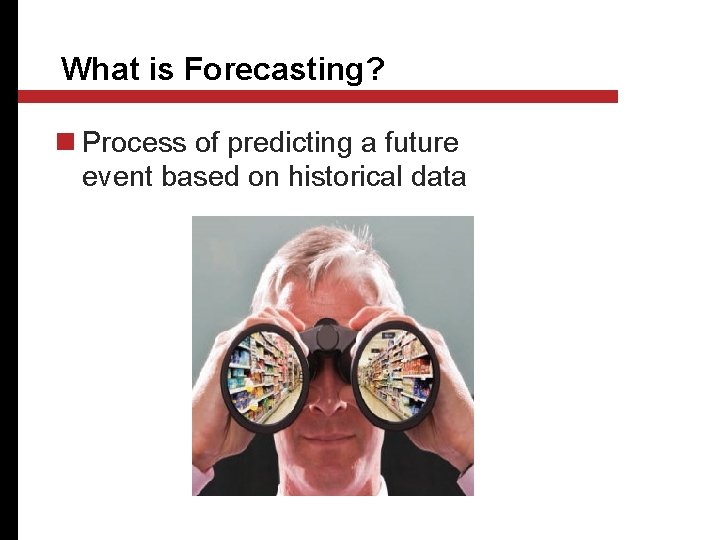
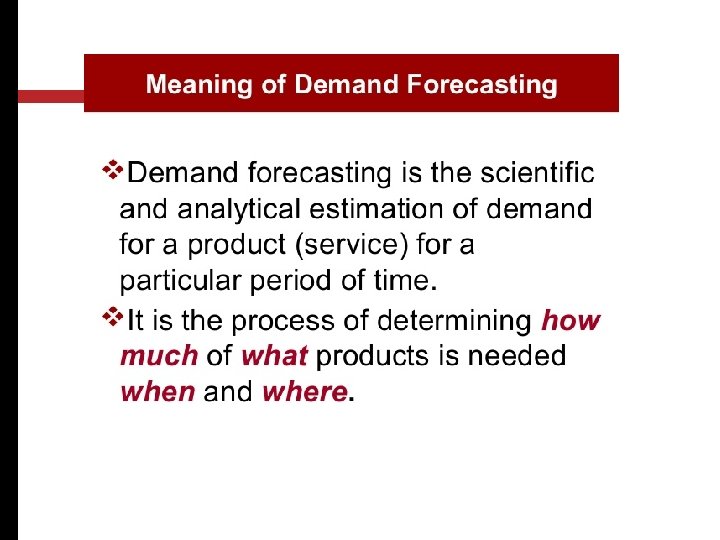
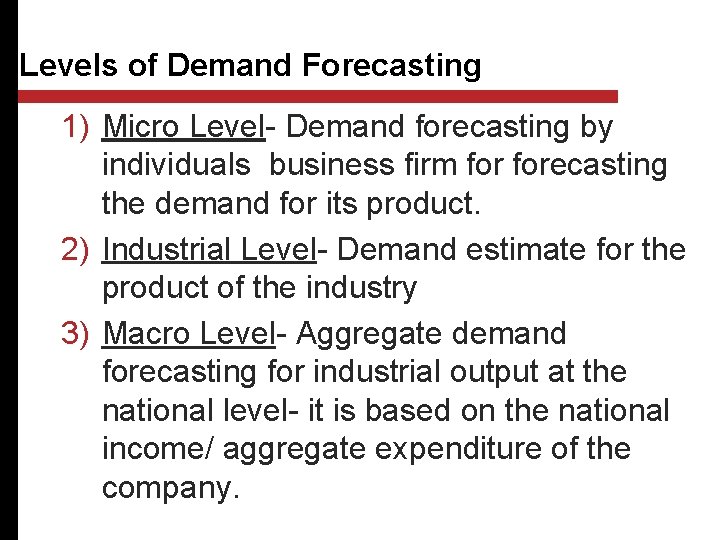
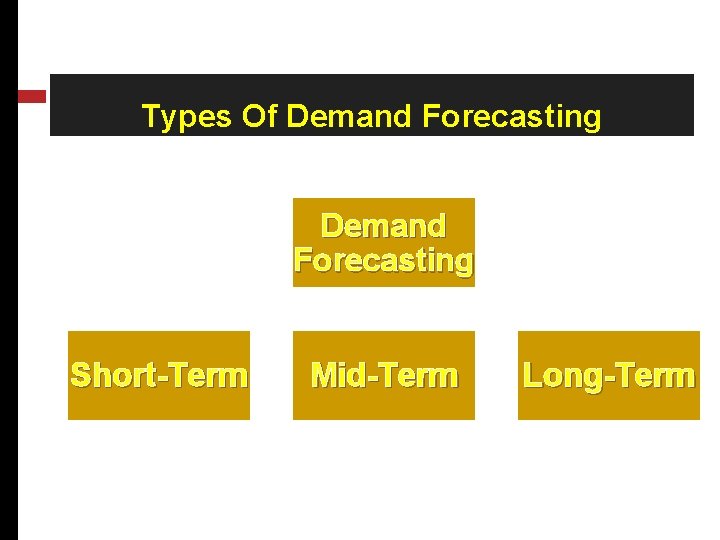
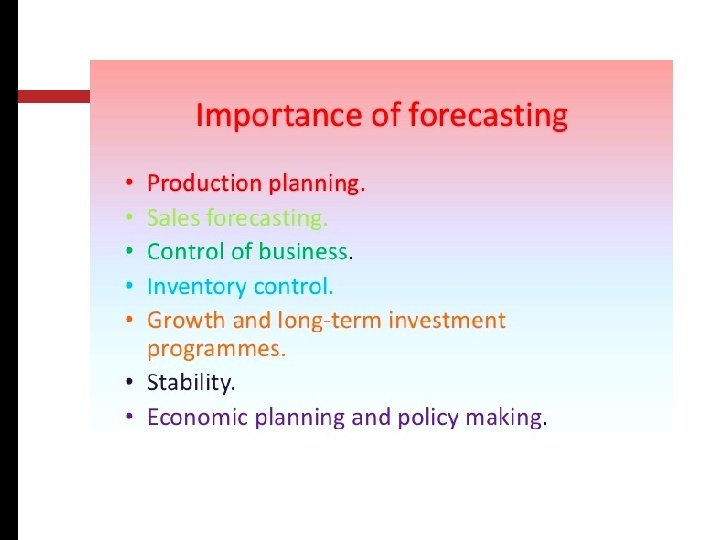
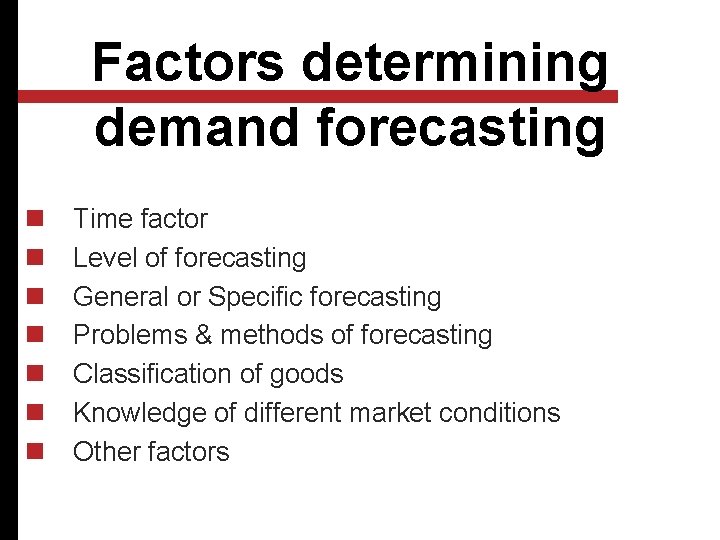
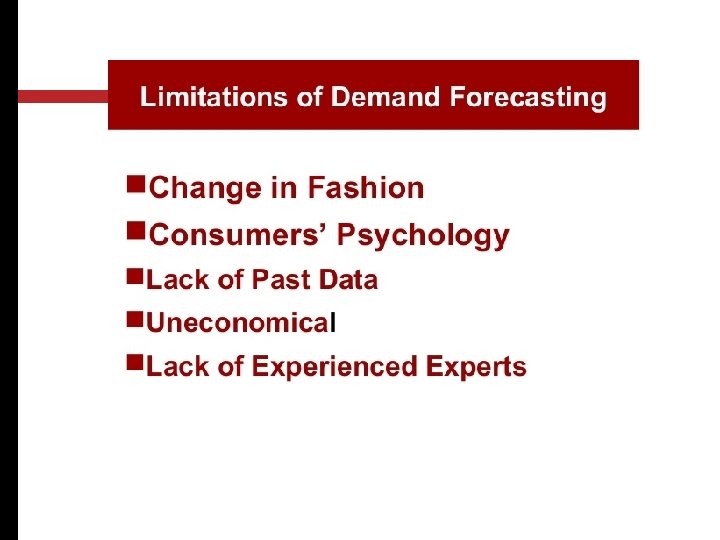
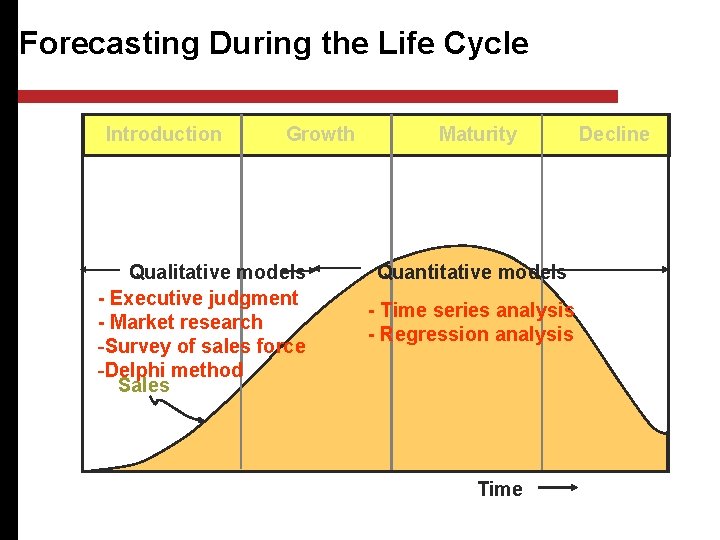
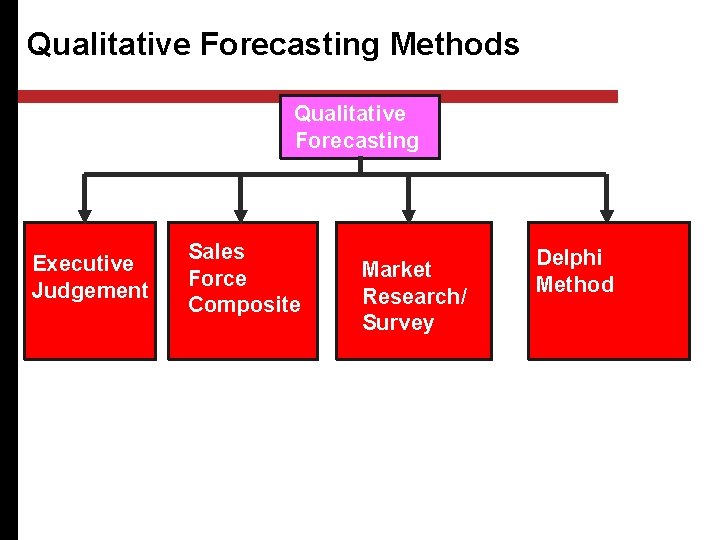
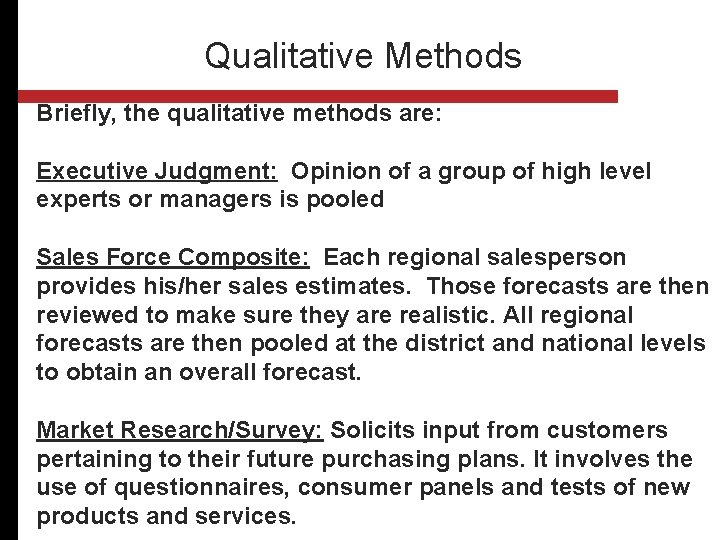
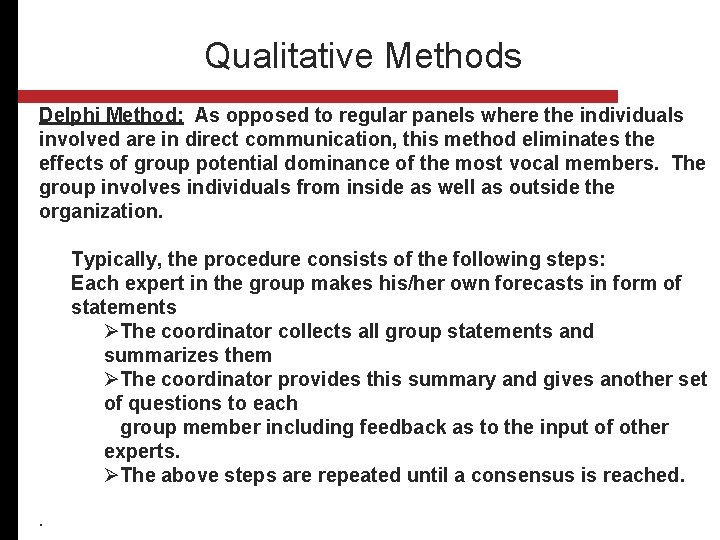
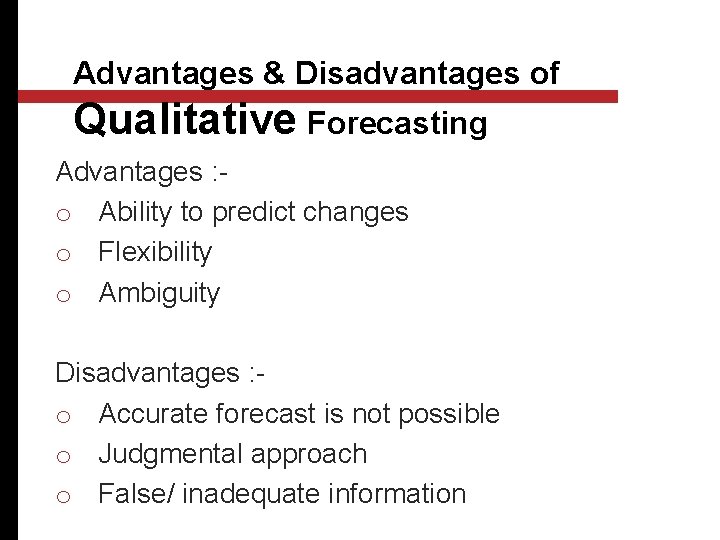
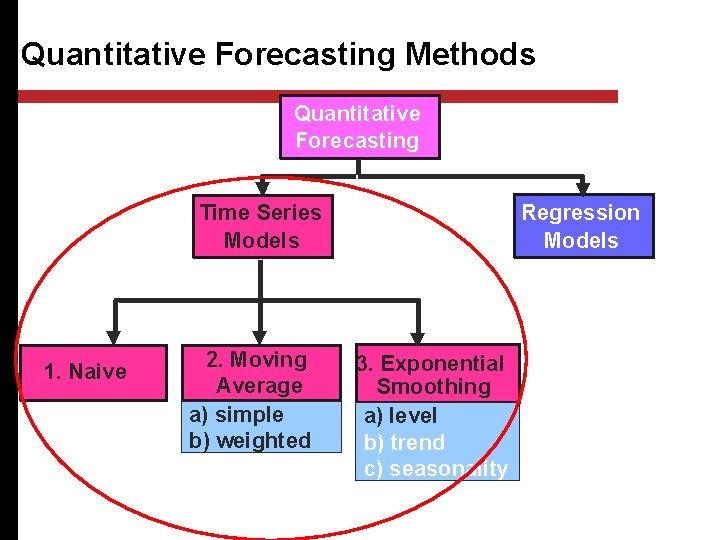
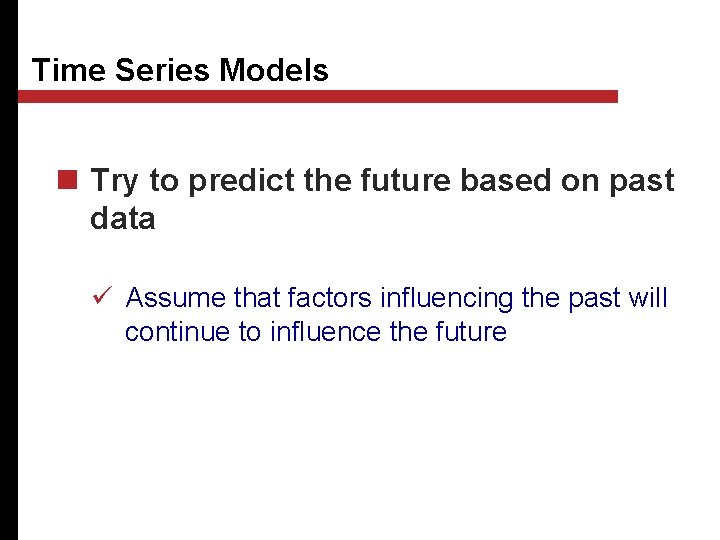
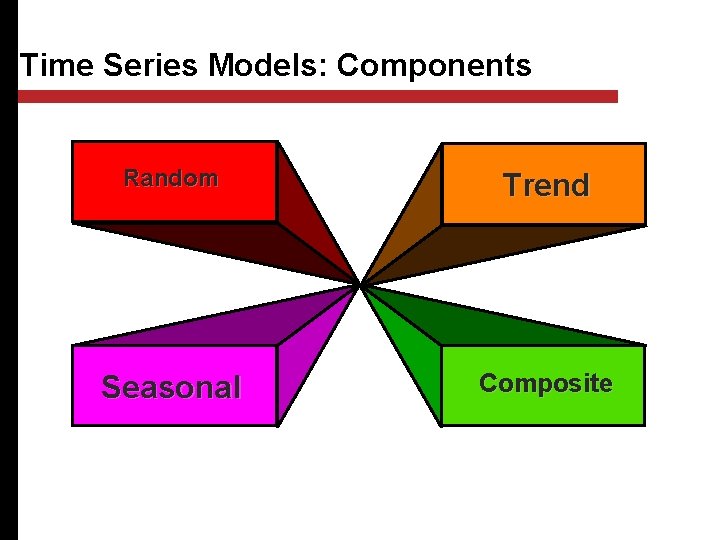
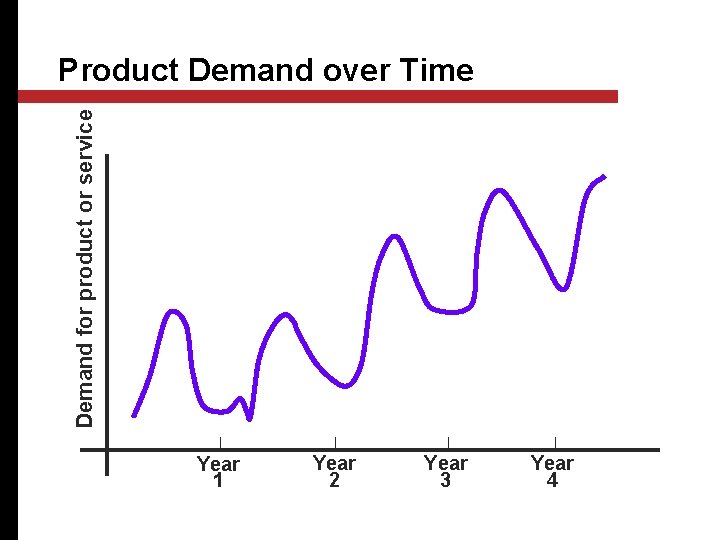
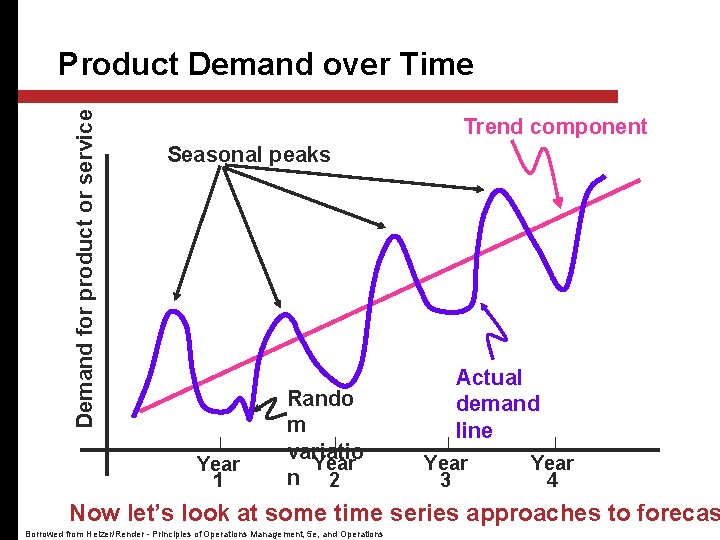
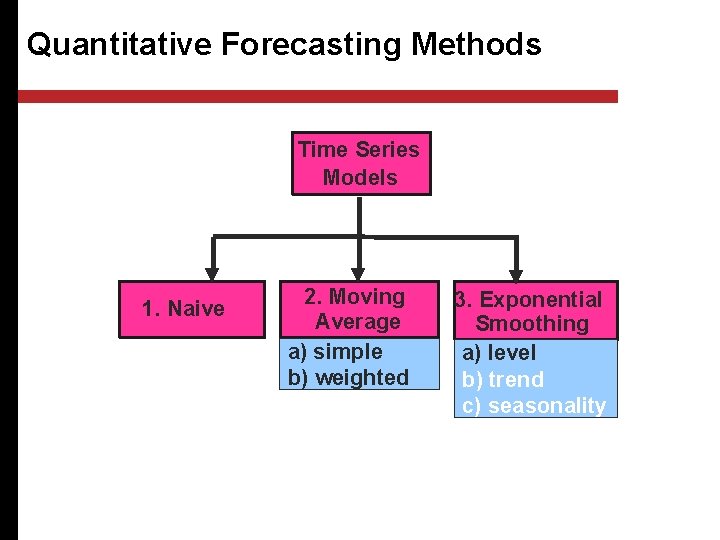
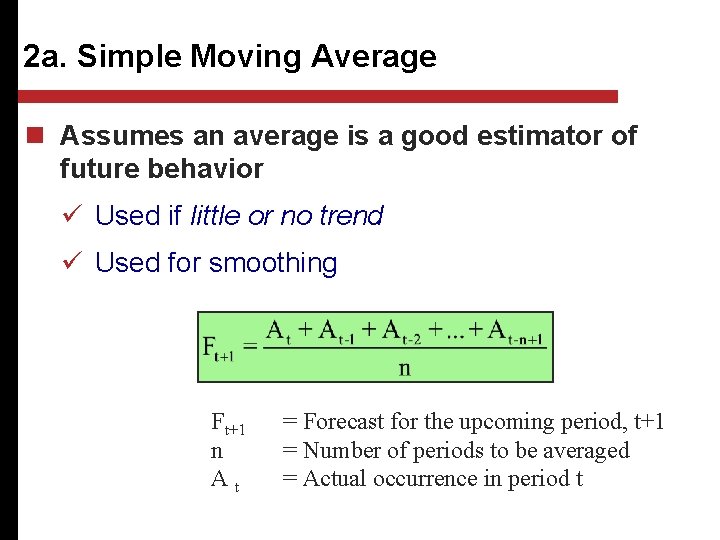
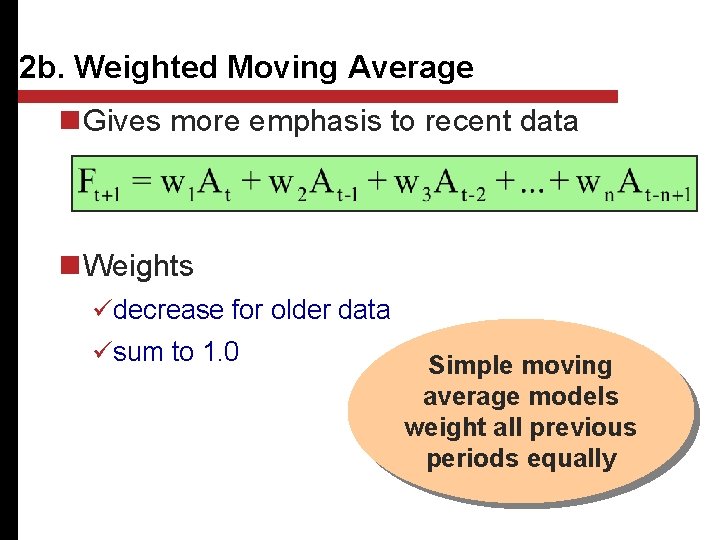
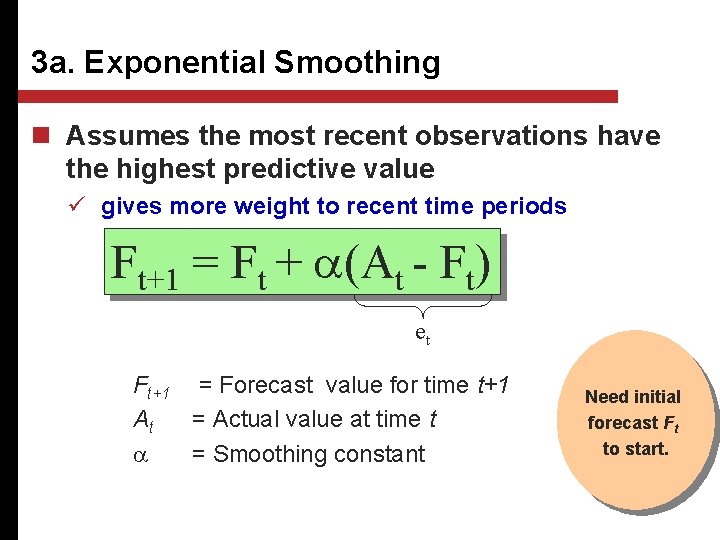
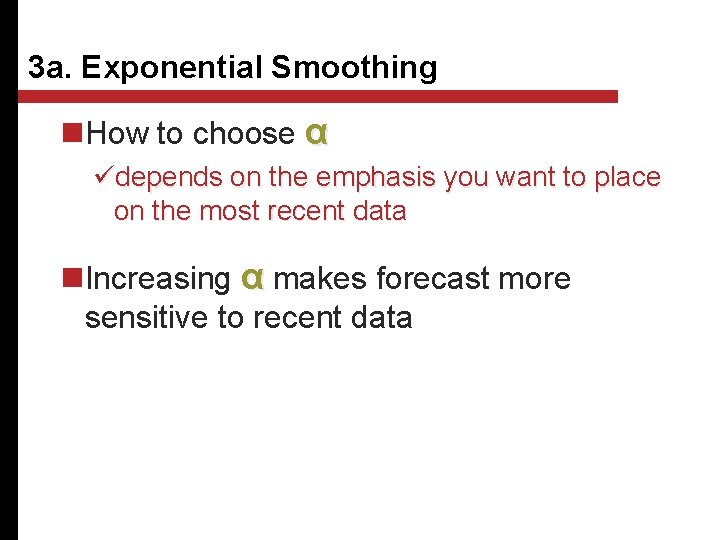
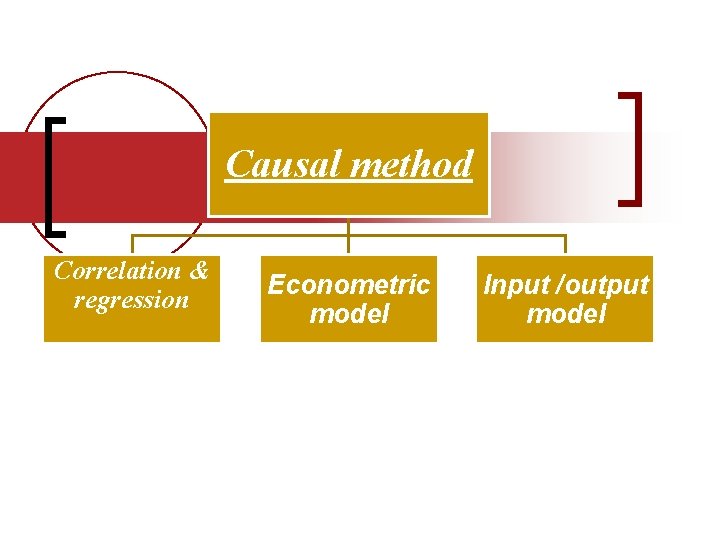
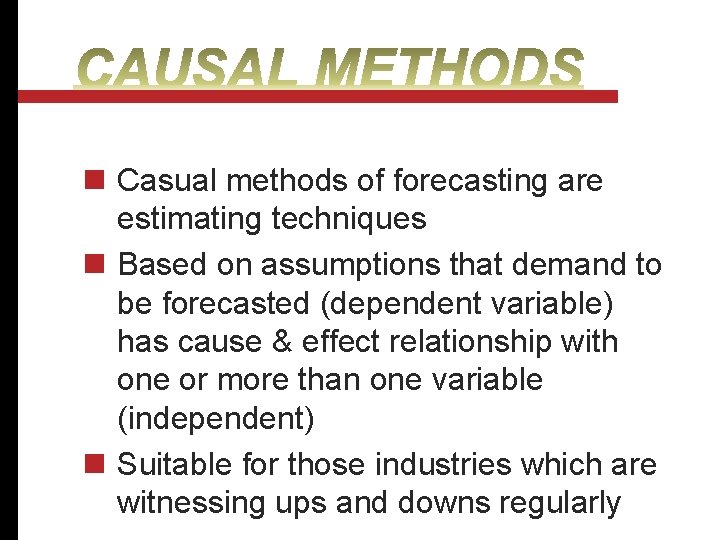
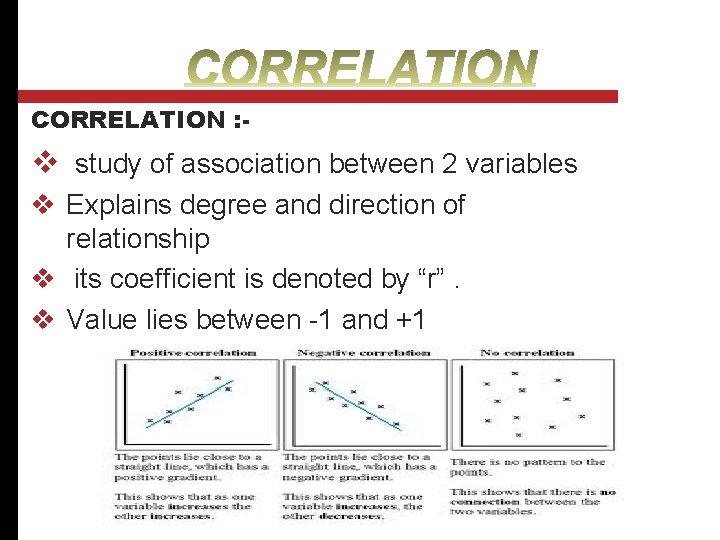
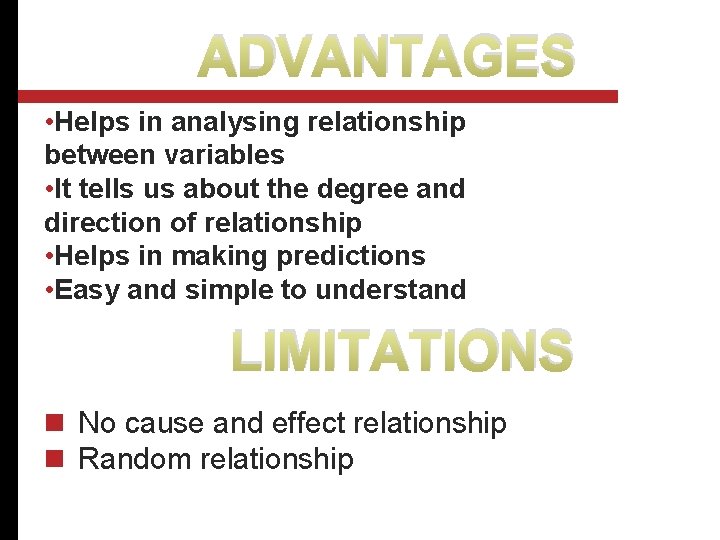
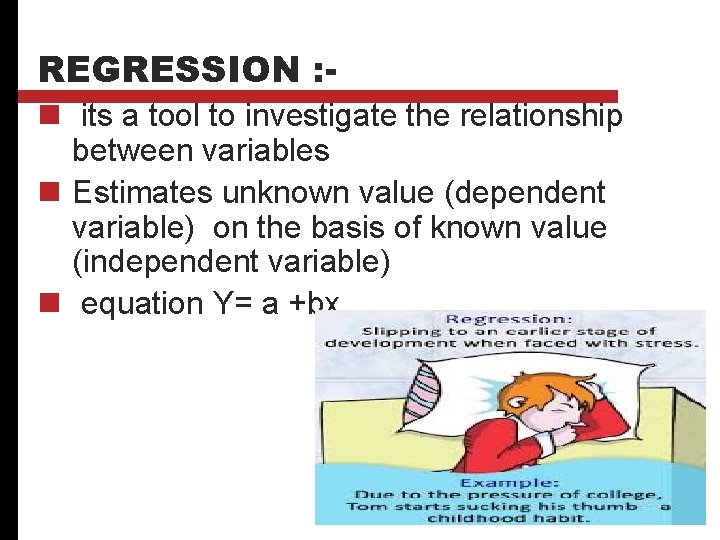
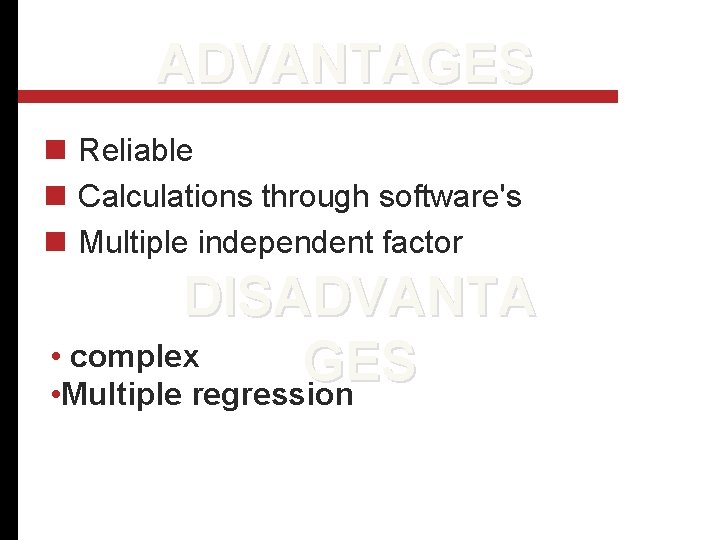
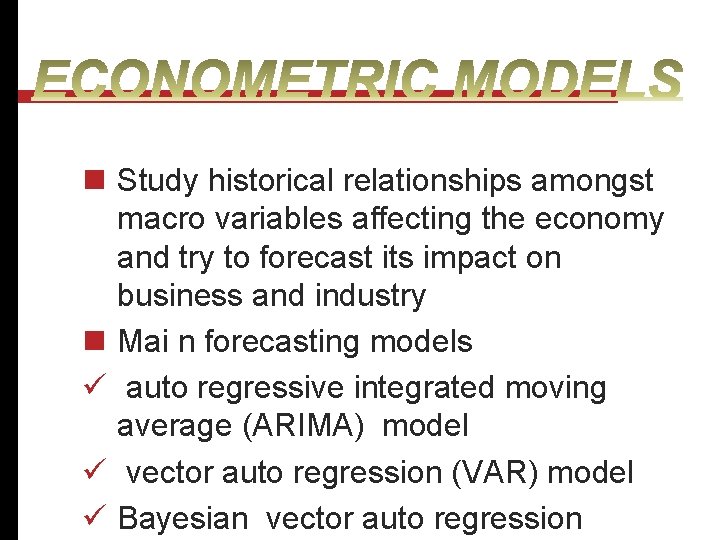
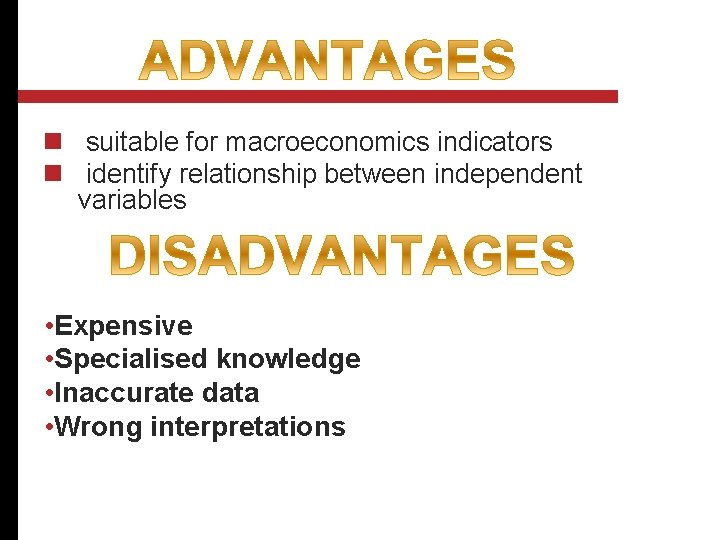
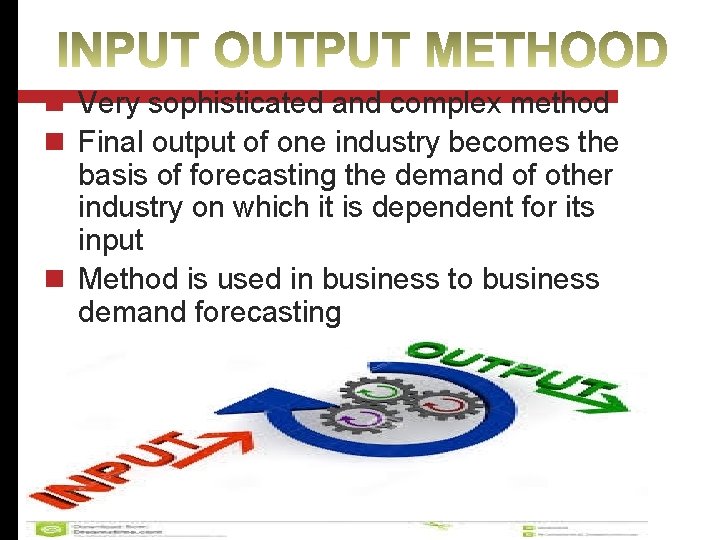
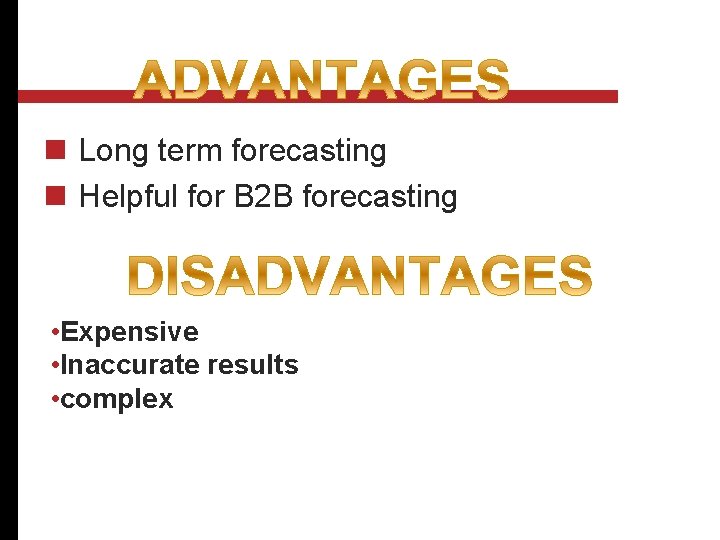
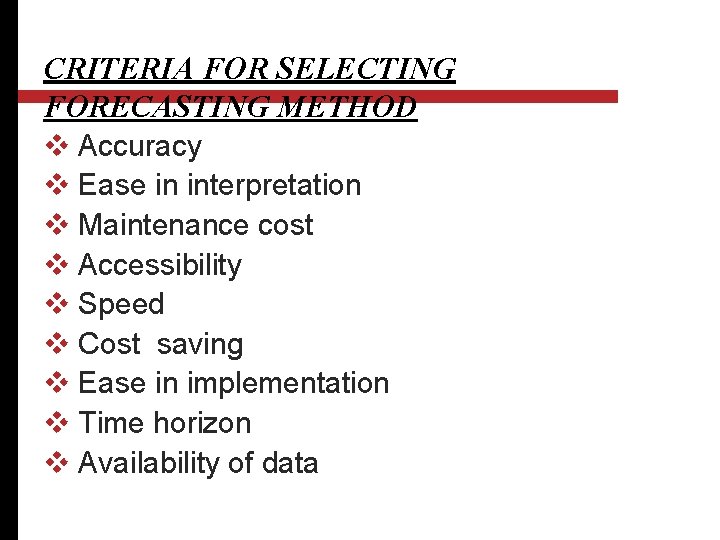
- Slides: 34
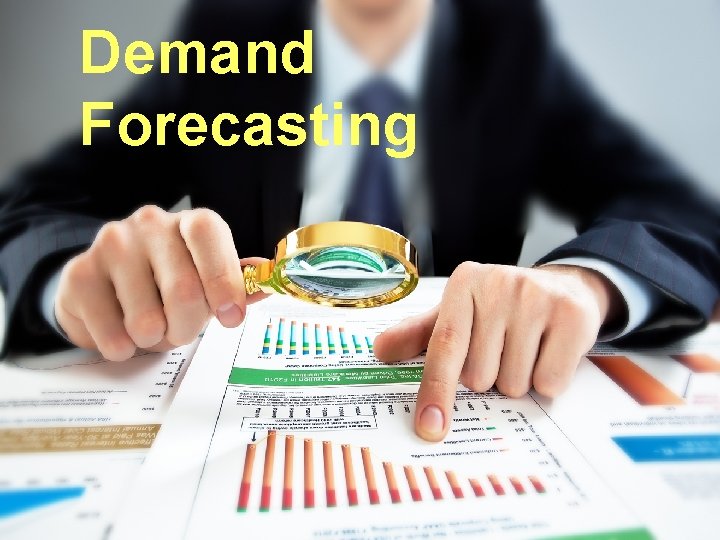
Demand Forecasting
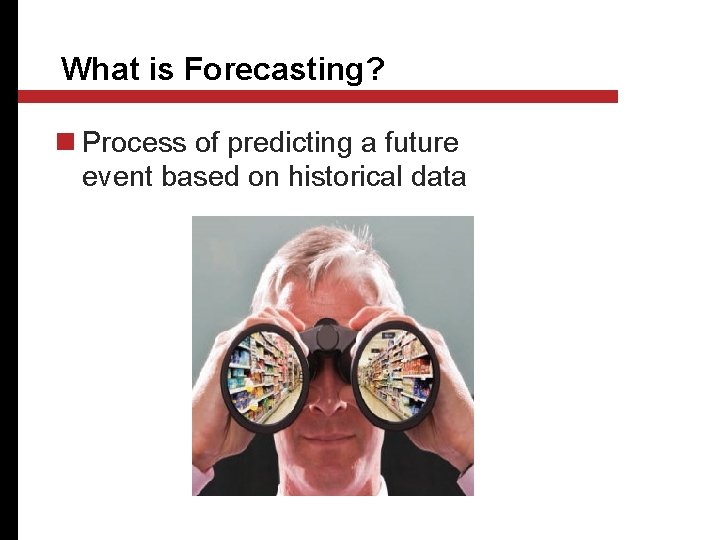
What is Forecasting? n Process of predicting a future event based on historical data
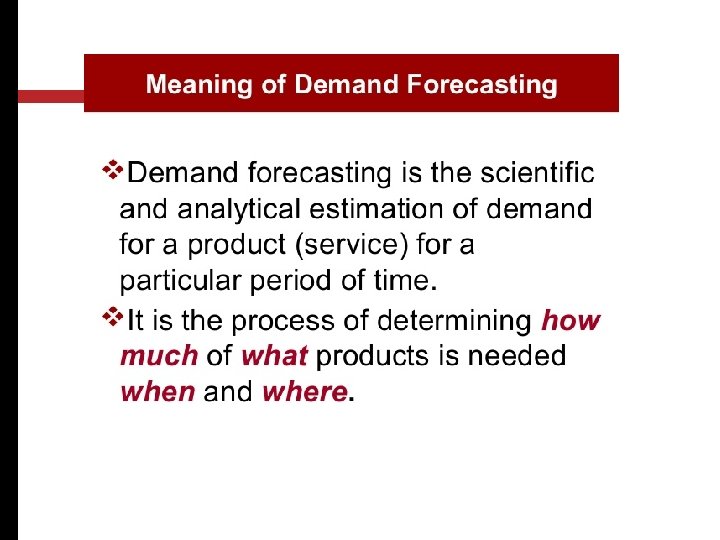
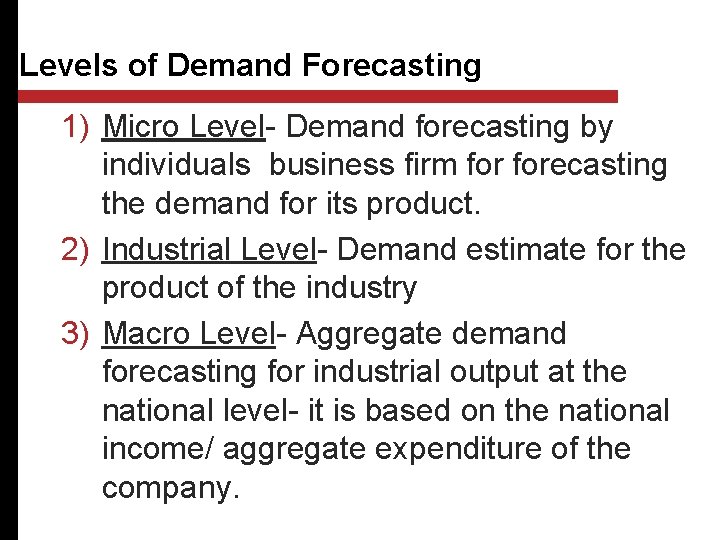
Levels of Demand Forecasting 1) Micro Level- Demand forecasting by individuals business firm forecasting the demand for its product. 2) Industrial Level- Demand estimate for the product of the industry 3) Macro Level- Aggregate demand forecasting for industrial output at the national level- it is based on the national income/ aggregate expenditure of the company.
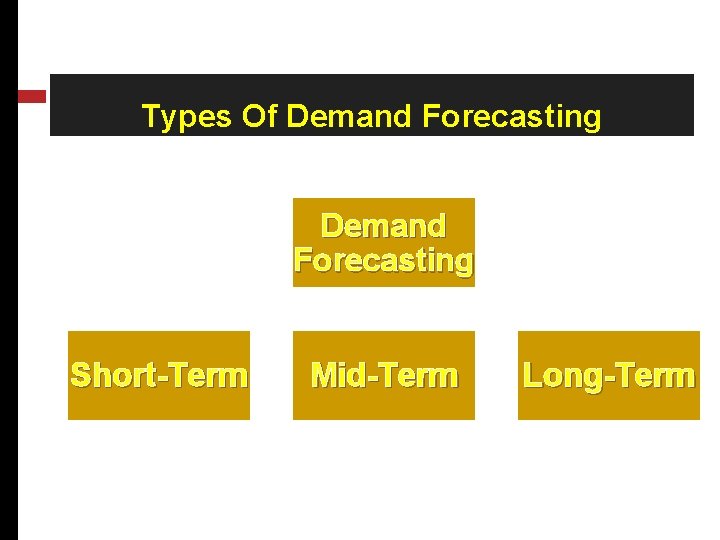
Types Of Demand Forecasting Short-Term Mid-Term Long-Term
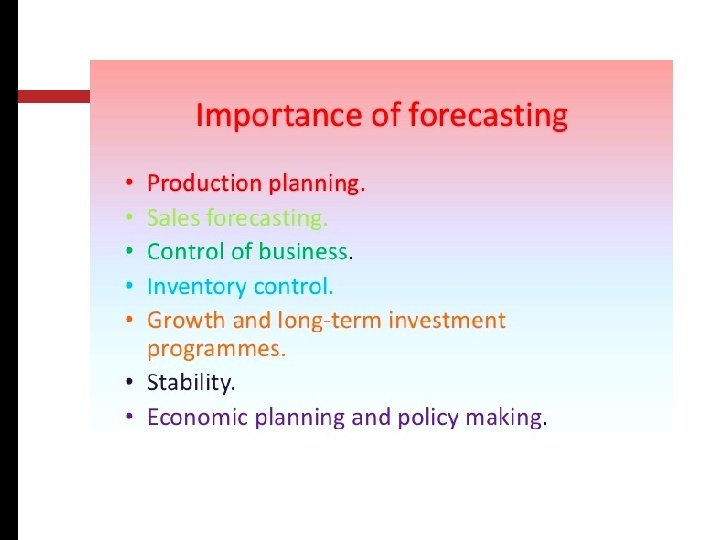
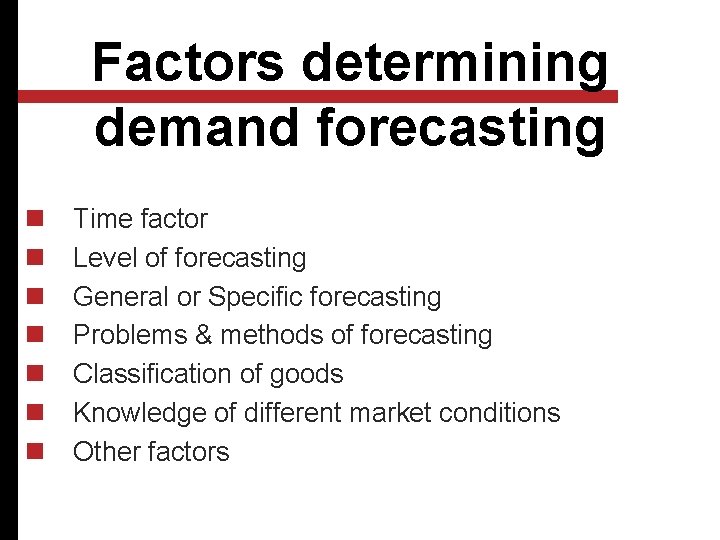
Factors determining demand forecasting n n n n Time factor Level of forecasting General or Specific forecasting Problems & methods of forecasting Classification of goods Knowledge of different market conditions Other factors
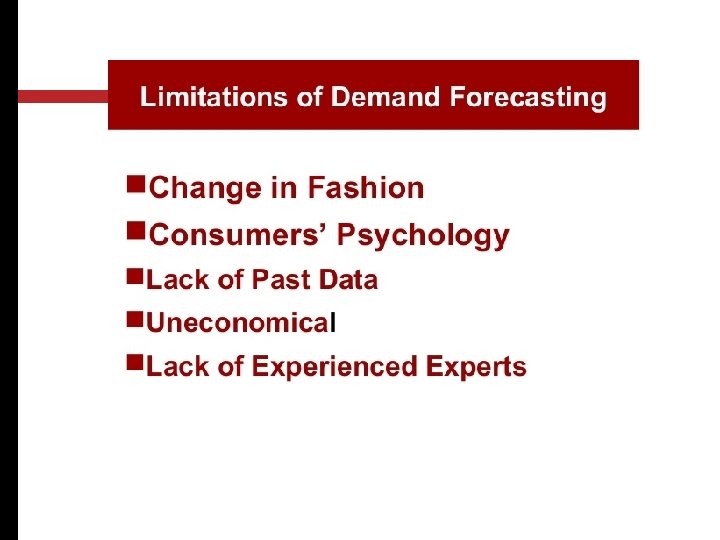
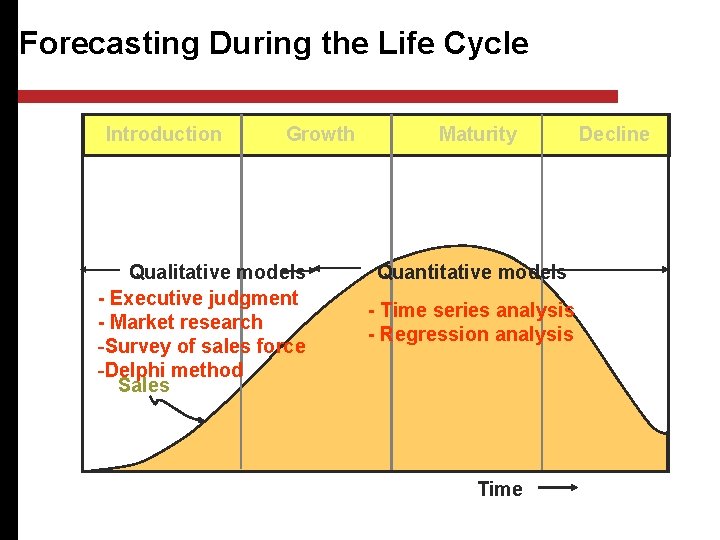
Forecasting During the Life Cycle Introduction Growth Qualitative models - Executive judgment - Market research -Survey of sales force -Delphi method Sales Maturity Quantitative models - Time series analysis - Regression analysis Time Decline
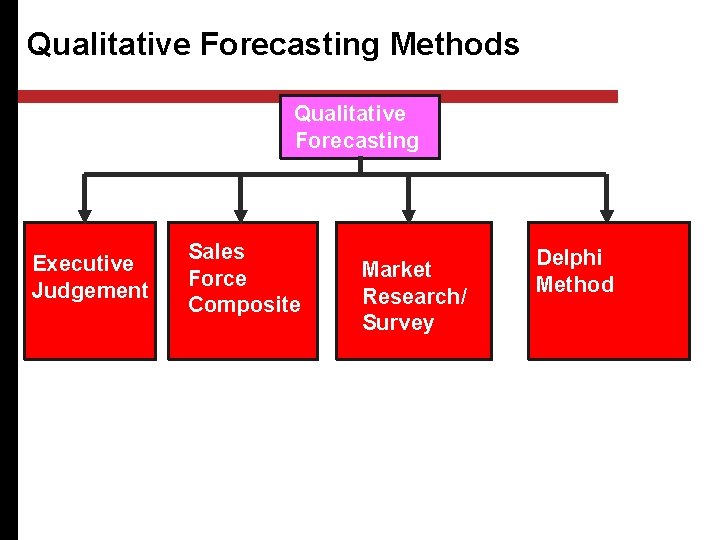
Qualitative Forecasting Methods Qualitative Forecasting Executive Judgement Sales Force Composite Market Research/ Survey Smoothing Models Delphi Method
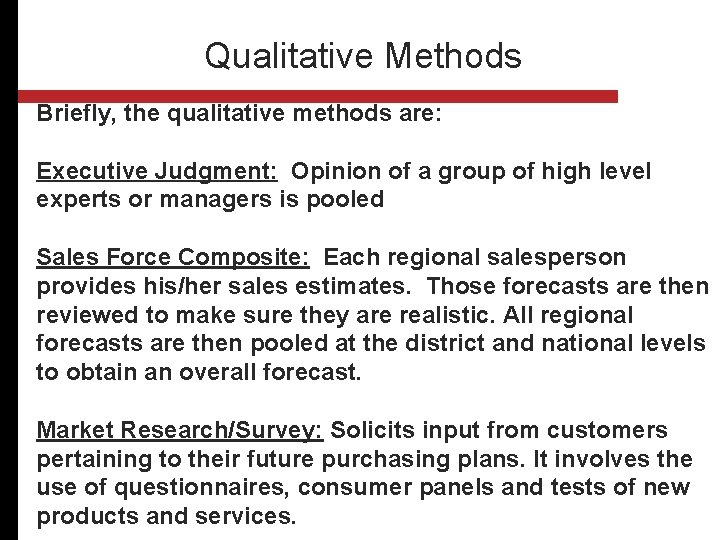
Qualitative Methods Briefly, the qualitative methods are: Executive Judgment: Opinion of a group of high level experts or managers is pooled Sales Force Composite: Each regional salesperson provides his/her sales estimates. Those forecasts are then reviewed to make sure they are realistic. All regional forecasts are then pooled at the district and national levels to obtain an overall forecast. Market Research/Survey: Solicits input from customers pertaining to their future purchasing plans. It involves the use of questionnaires, consumer panels and tests of new products and services.
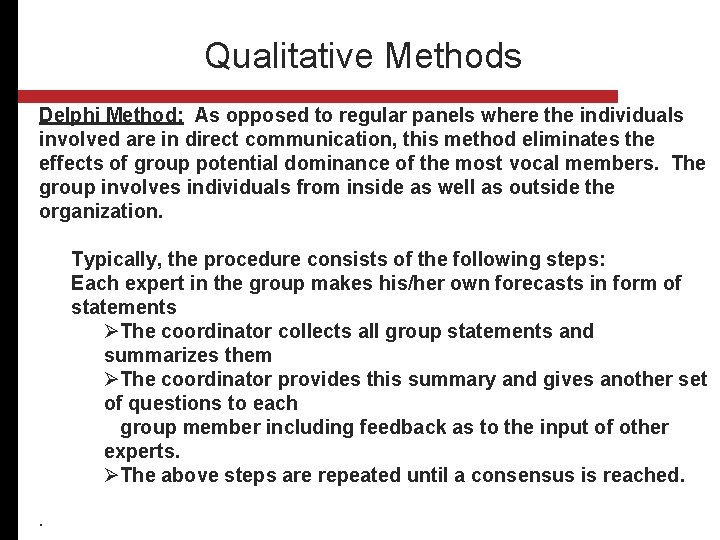
Qualitative Methods Delphi Method: As opposed to regular panels where the individuals involved are in direct communication, this method eliminates the effects of group potential dominance of the most vocal members. The group involves individuals from inside as well as outside the organization. Typically, the procedure consists of the following steps: Each expert in the group makes his/her own forecasts in form of statements The coordinator collects all group statements and summarizes them The coordinator provides this summary and gives another set of questions to each group member including feedback as to the input of other experts. The above steps are repeated until a consensus is reached. .
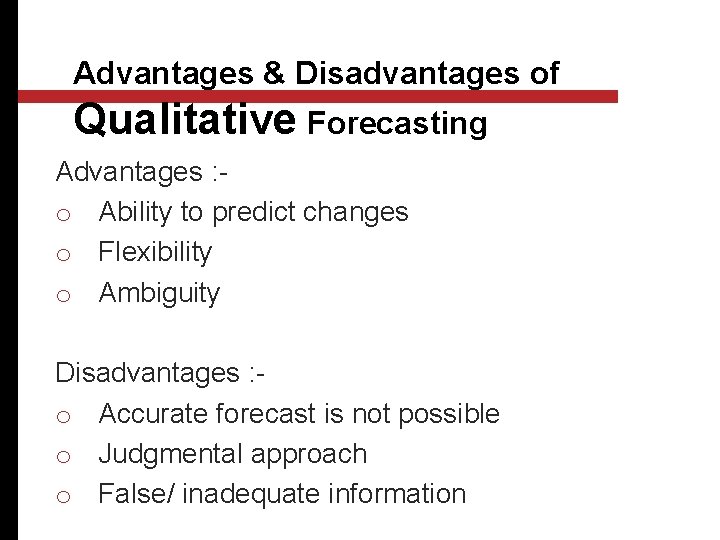
Advantages & Disadvantages of Qualitative Forecasting Advantages : o Ability to predict changes o Flexibility o Ambiguity Disadvantages : o Accurate forecast is not possible o Judgmental approach o False/ inadequate information
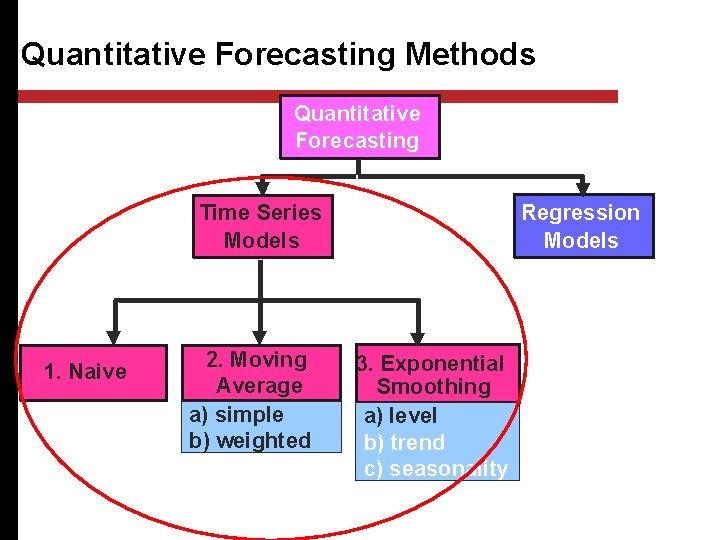
Quantitative Forecasting Methods Quantitative Forecasting Regression Models Time Series Models 1. Naive 2. Moving Average a) simple b) weighted 3. Exponential Smoothing a) level b) trend c) seasonality
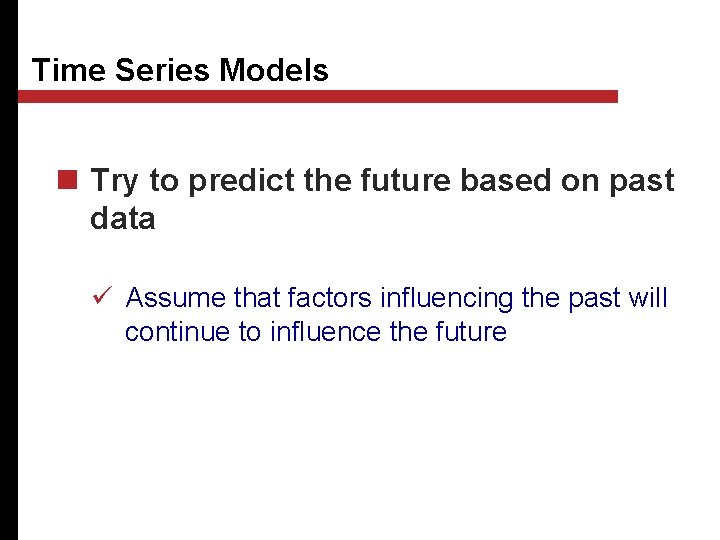
Time Series Models n Try to predict the future based on past data ü Assume that factors influencing the past will continue to influence the future
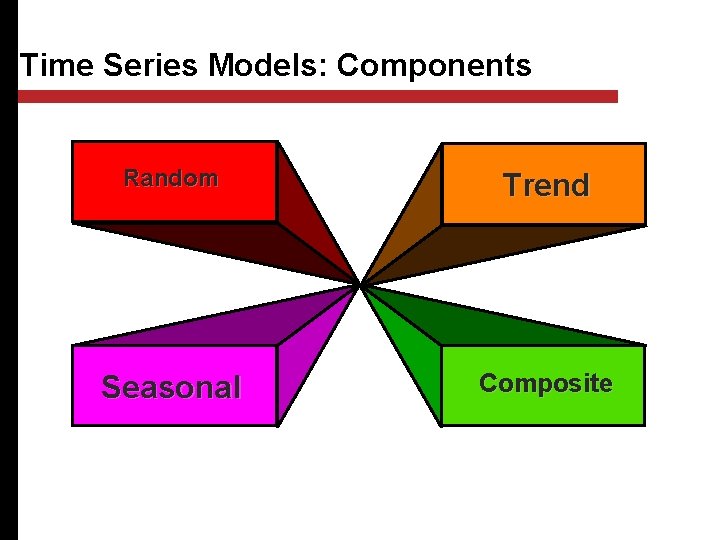
Time Series Models: Components Random Trend Seasonal Composite
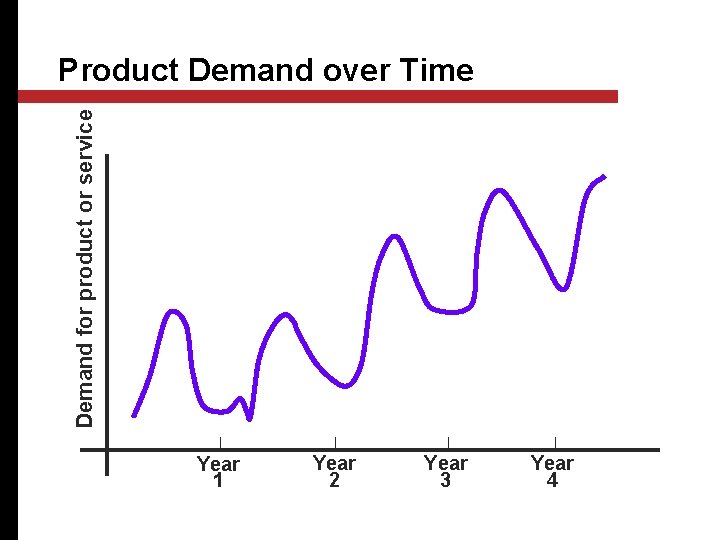
Demand for product or service Product Demand over Time Year 1 Year 2 Year 3 Year 4
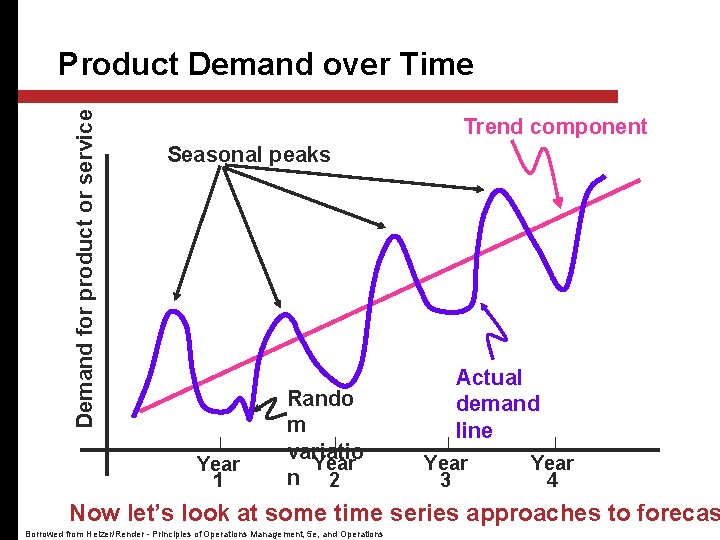
Demand for product or service Product Demand over Time Trend component Seasonal peaks Year 1 Rando m variatio Year n 2 Actual demand line Year 3 Year 4 Now let’s look at some time series approaches to forecas Borrowed from Heizer/Render - Principles of Operations Management, 5 e, and Operations
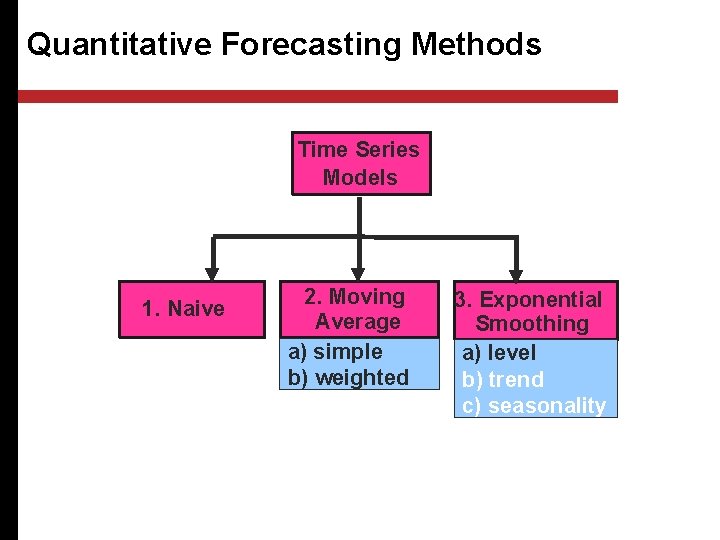
Quantitative Forecasting Methods Quantitative Time Series Models 1. Naive 2. Moving Average a) simple b) weighted 3. Exponential Smoothing a) level b) trend c) seasonality
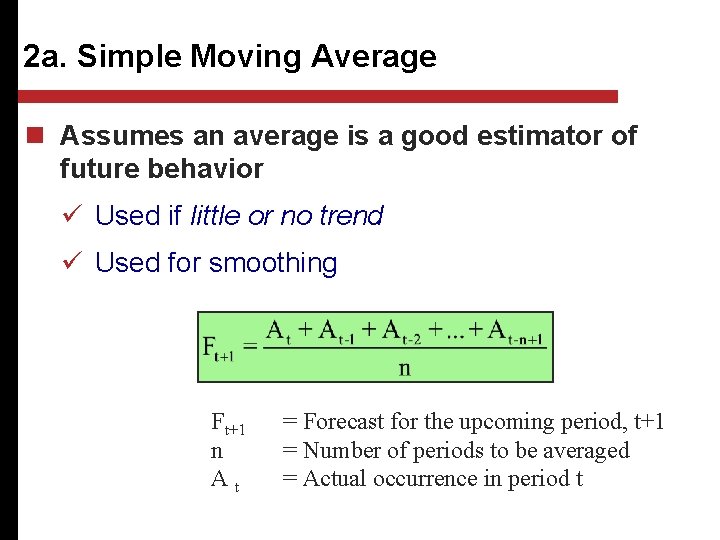
2 a. Simple Moving Average n Assumes an average is a good estimator of future behavior ü Used if little or no trend ü Used for smoothing Ft+1 n At = Forecast for the upcoming period, t+1 = Number of periods to be averaged = Actual occurrence in period t
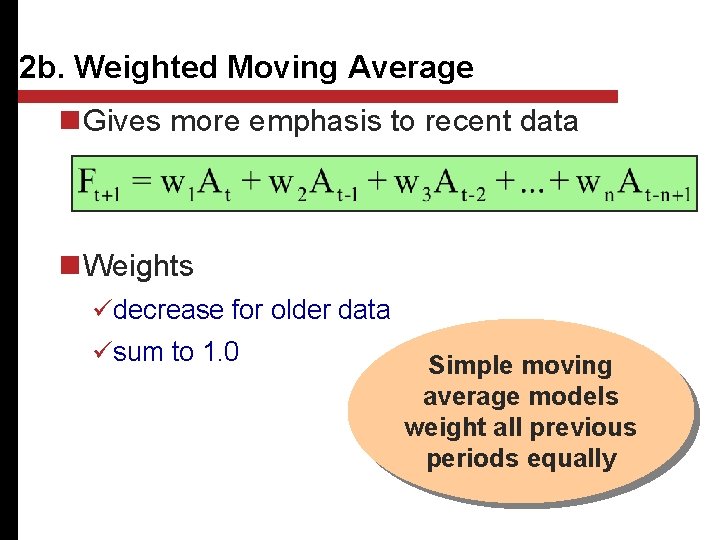
2 b. Weighted Moving Average n Gives more emphasis to recent data n Weights üdecrease for older data üsum to 1. 0 Simple moving average models weight all previous periods equally
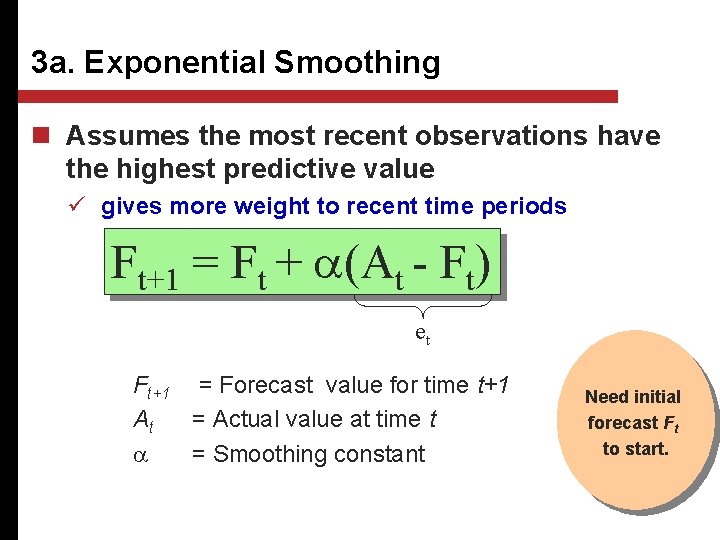
3 a. Exponential Smoothing n Assumes the most recent observations have the highest predictive value ü gives more weight to recent time periods Ft+1 = Ft + (At - Ft) et Ft+1 = Forecast value for time t+1 At = Actual value at time t = Smoothing constant Need initial forecast Ft to start.
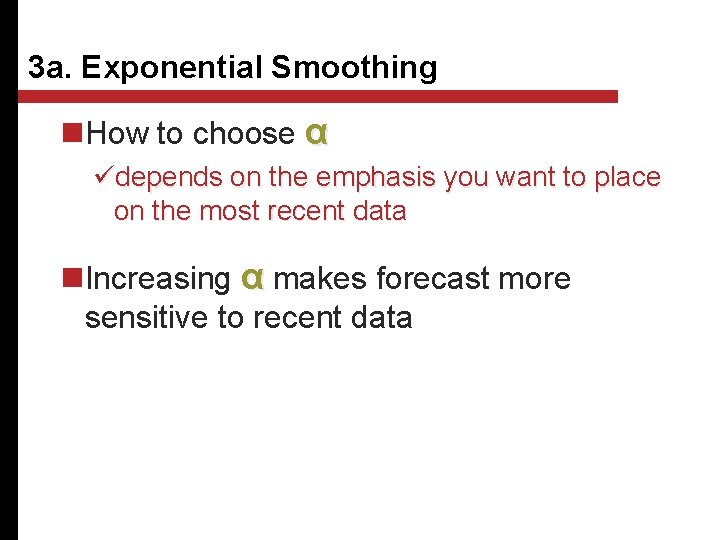
3 a. Exponential Smoothing n. How to choose α üdepends on the emphasis you want to place on the most recent data n. Increasing α makes forecast more sensitive to recent data
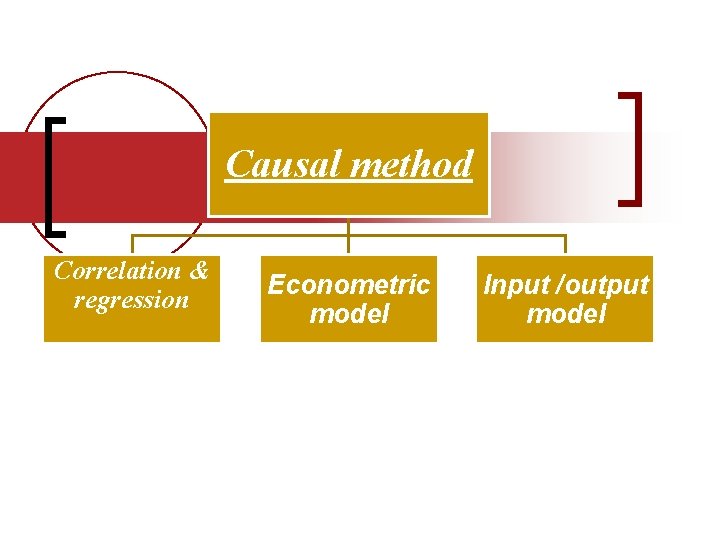
Causal method Correlation & regression Econometric model Input /output model
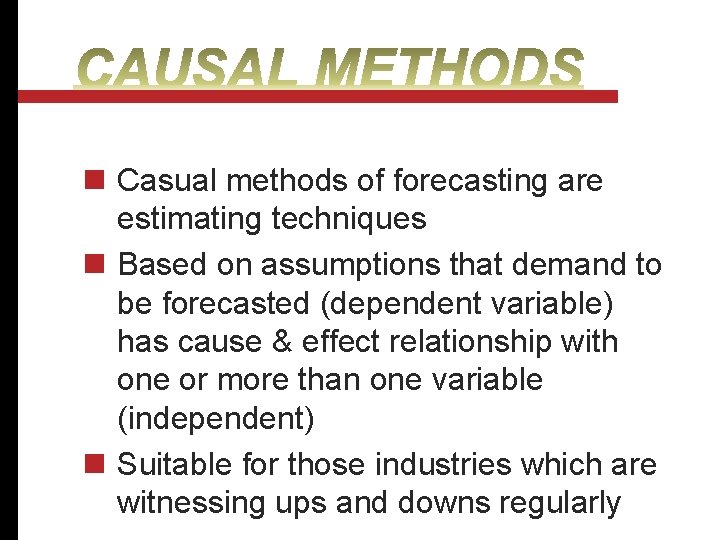
n Casual methods of forecasting are estimating techniques n Based on assumptions that demand to be forecasted (dependent variable) has cause & effect relationship with one or more than one variable (independent) n Suitable for those industries which are witnessing ups and downs regularly
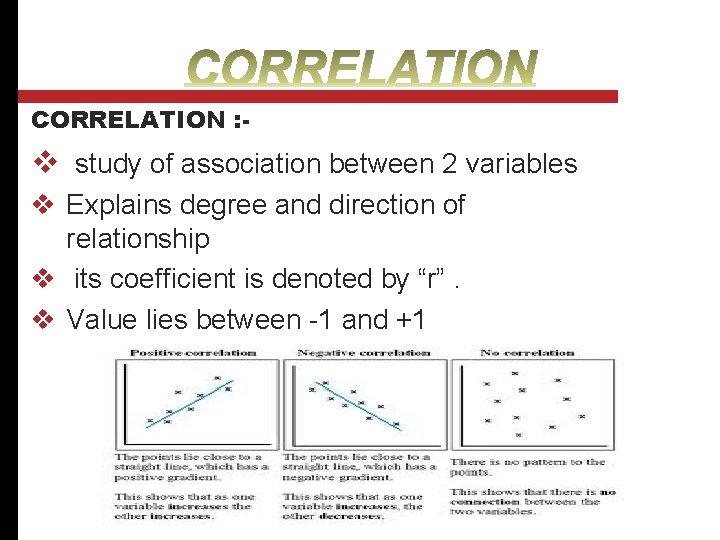
CORRELATION : - v study of association between 2 variables v Explains degree and direction of relationship v its coefficient is denoted by “r”. v Value lies between -1 and +1
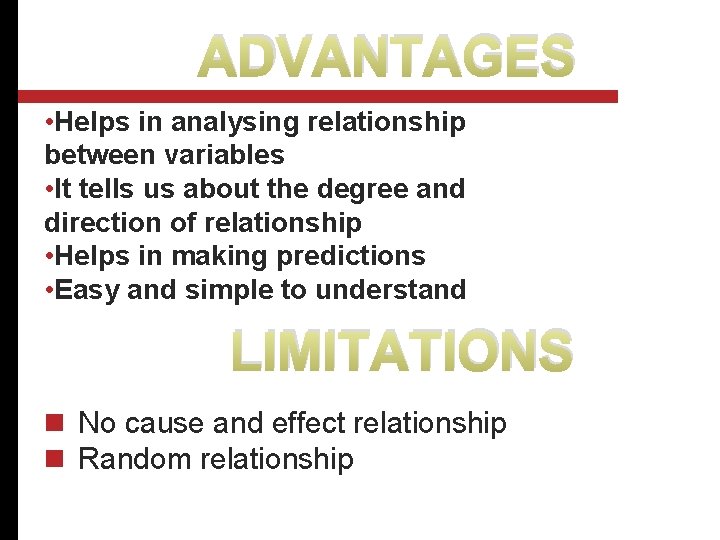
ADVANTAGES • Helps in analysing relationship between variables • It tells us about the degree and direction of relationship • Helps in making predictions • Easy and simple to understand LIMITATIONS n No cause and effect relationship n Random relationship
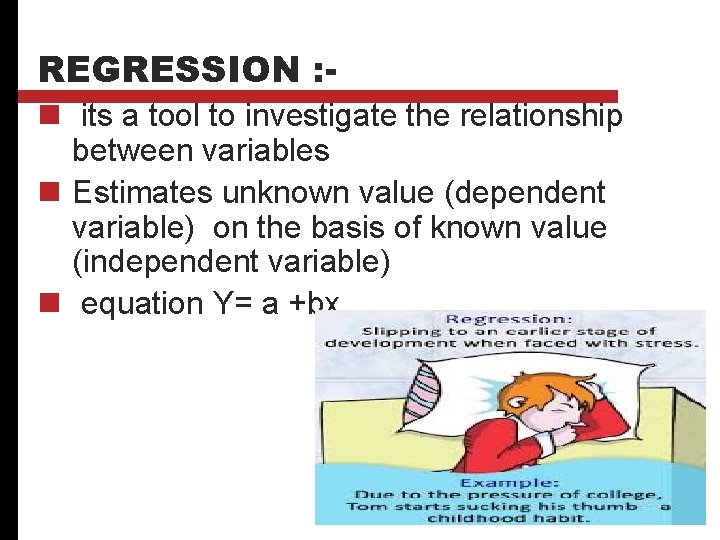
REGRESSION : n its a tool to investigate the relationship between variables n Estimates unknown value (dependent variable) on the basis of known value (independent variable) n equation Y= a +bx
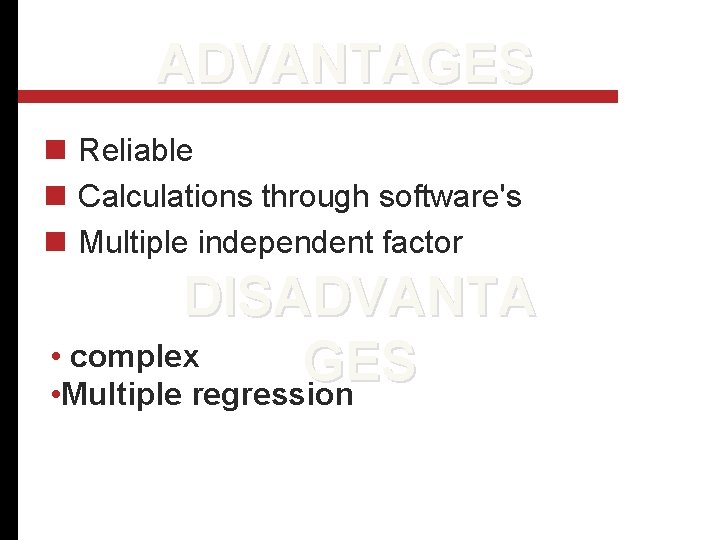
ADVANTAGES n Reliable n Calculations through software's n Multiple independent factor DISADVANTA • complex GES • Multiple regression
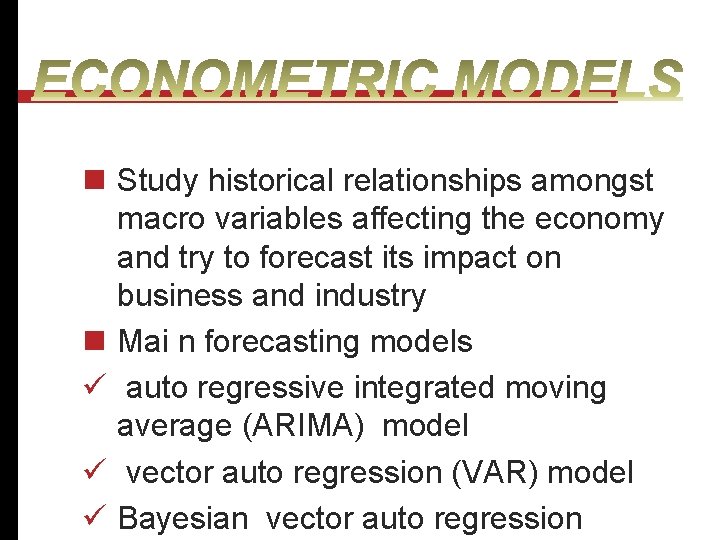
n Study historical relationships amongst macro variables affecting the economy and try to forecast its impact on business and industry n Mai n forecasting models ü auto regressive integrated moving average (ARIMA) model ü vector auto regression (VAR) model ü Bayesian vector auto regression
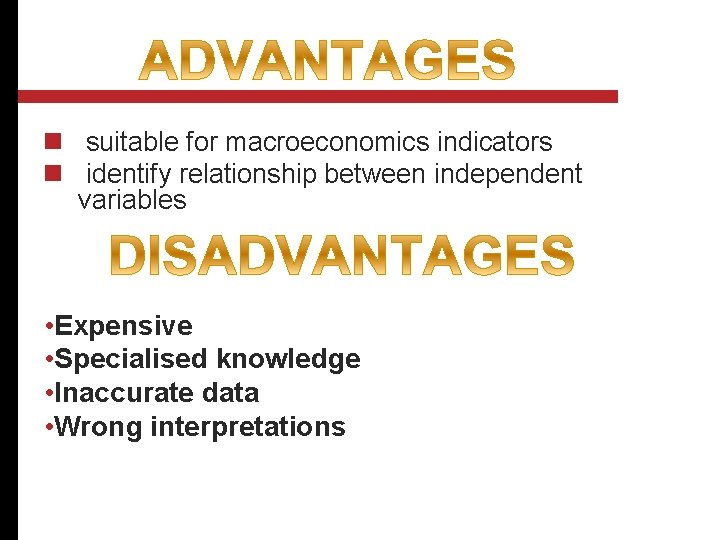
n suitable for macroeconomics indicators n identify relationship between independent variables • Expensive • Specialised knowledge • Inaccurate data • Wrong interpretations
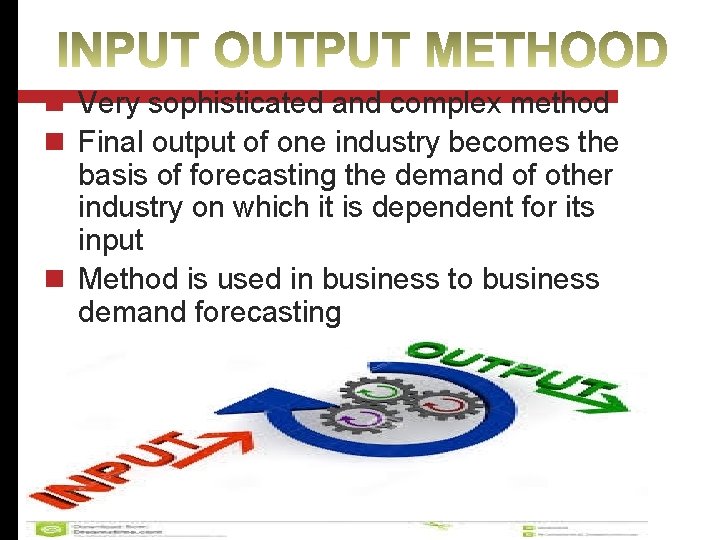
n Very sophisticated and complex method n Final output of one industry becomes the basis of forecasting the demand of other industry on which it is dependent for its input n Method is used in business to business demand forecasting
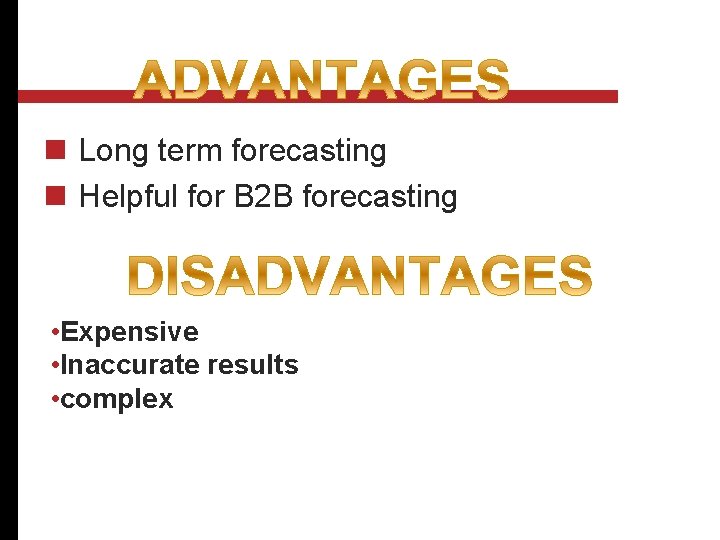
n Long term forecasting n Helpful for B 2 B forecasting • Expensive • Inaccurate results • complex
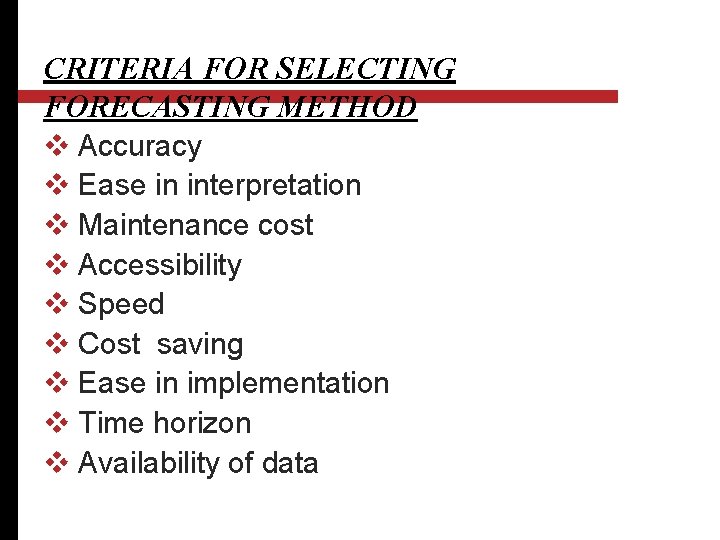
CRITERIA FOR SELECTING FORECASTING METHOD v Accuracy v Ease in interpretation v Maintenance cost v Accessibility v Speed v Cost saving v Ease in implementation v Time horizon v Availability of data
Methods of demand estimation
Hotel demand forecasting
Statistical methods of demand forecasting
Chain ratio method of demand forecasting
Importance of forcasting
Statistical methods of demand forecasting
Demand estimation and forecasting
Demand forecasting and replenishment
Barometric methods are used to forecast
Forecasting and demand measurement
Measuring and forecasting demand
Collecting information and forecasting demand summary
Forecasting demand for autonomous vehicles
Capturing marketing insights examples
Factors affecting hr demand forecasting
Demand forecasting objectives
Micro level demand forecasting
Demand forecasting operations management
Forecasting and demand measurement in marketing
What is demand forecasting and estimation
Collecting information and forecasting demand
Dynamic traffic assignment
Hierarchical production planning
Demand forecasting objectives
Limitations of demand forecasting
Simultaneous equation method in demand forecasting
Gathering information and measuring market demand
Conducting marketing research and forecasting demand
Conducting marketing research and forecasting demand
Demand forecasting
Chain ratio method of demand forecasting
Demand forecasting introduction
Tracking signal
Forecasting and demand measurement in marketing
Air travel demand forecasting