Customization of a Mesoscale Numerical Weather Prediction System
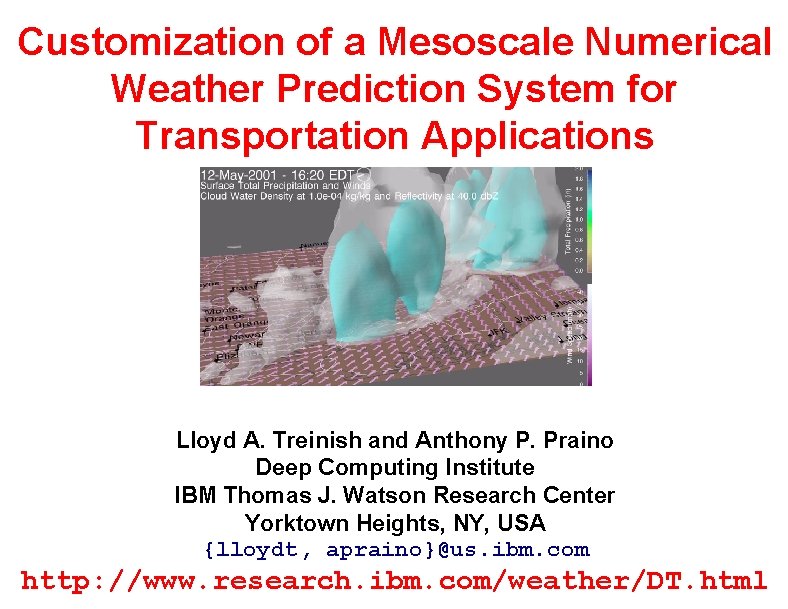
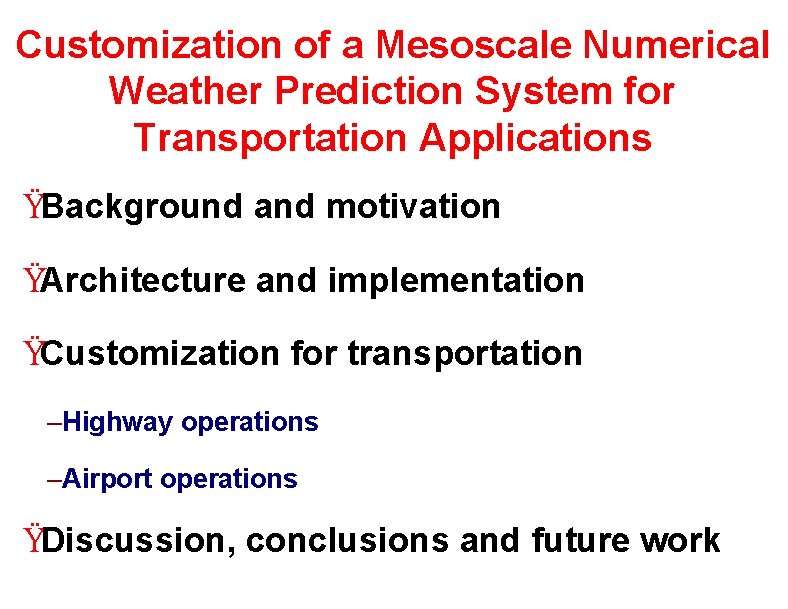
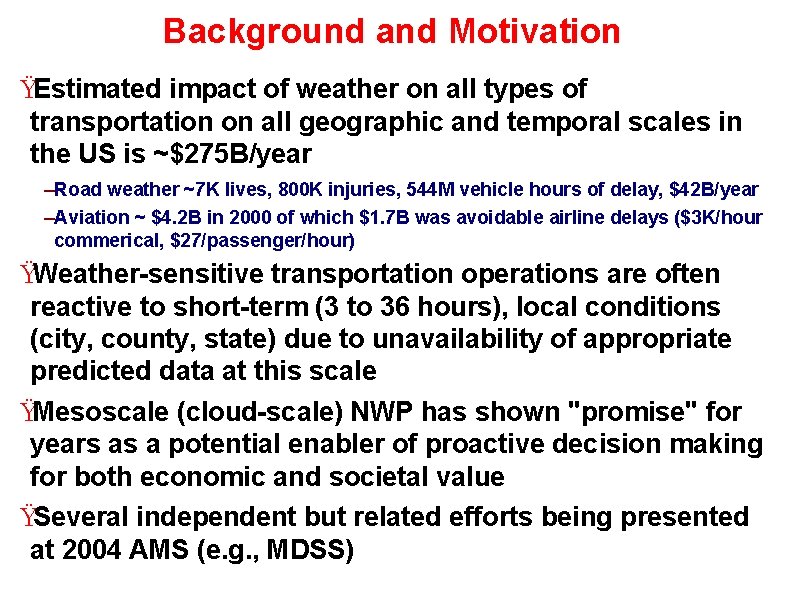
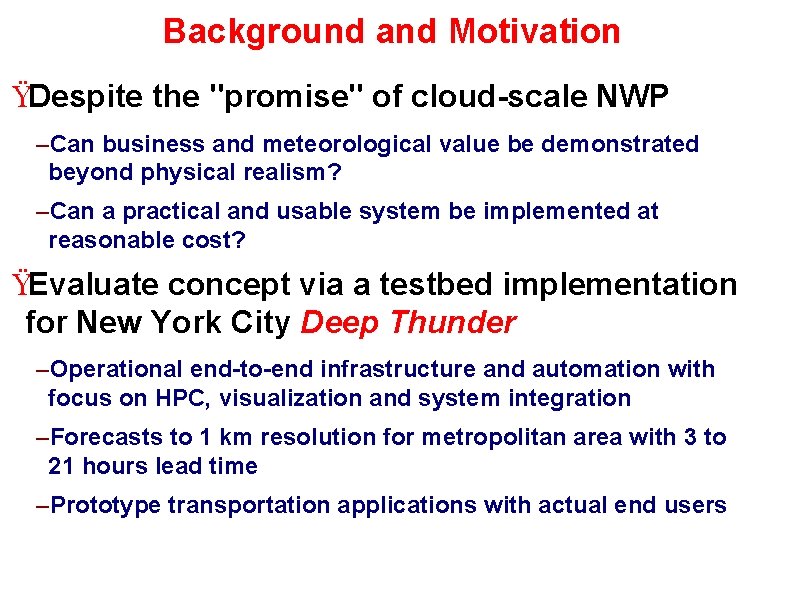
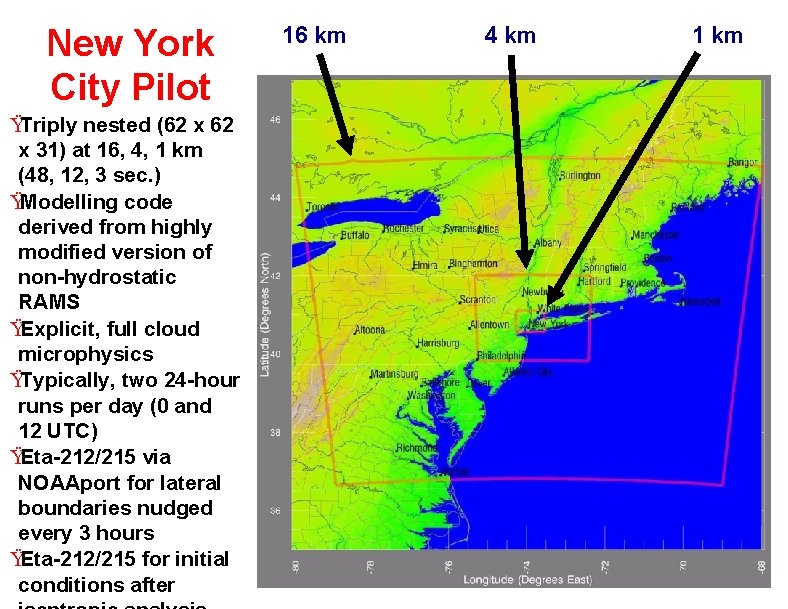
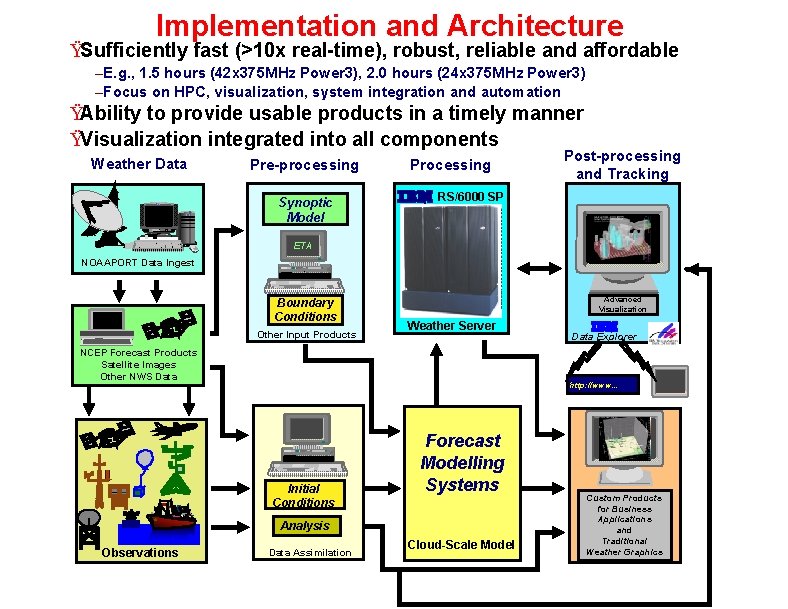
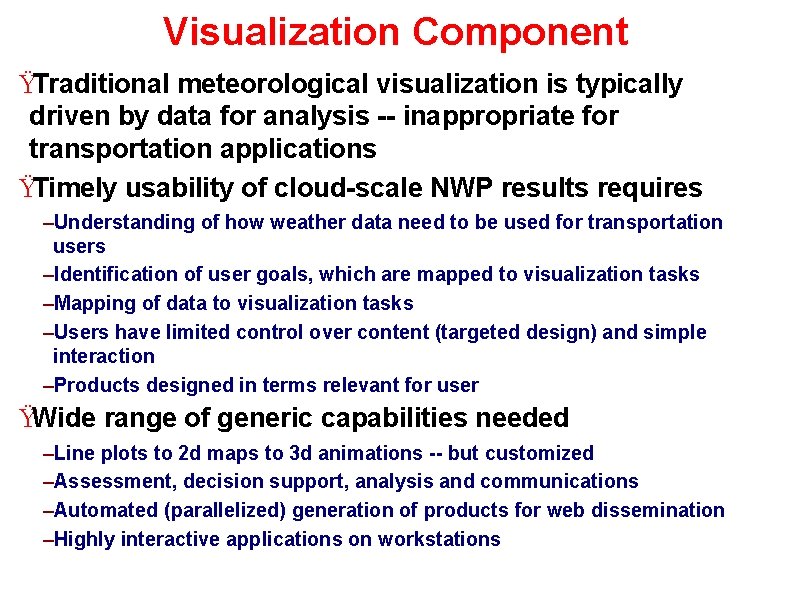
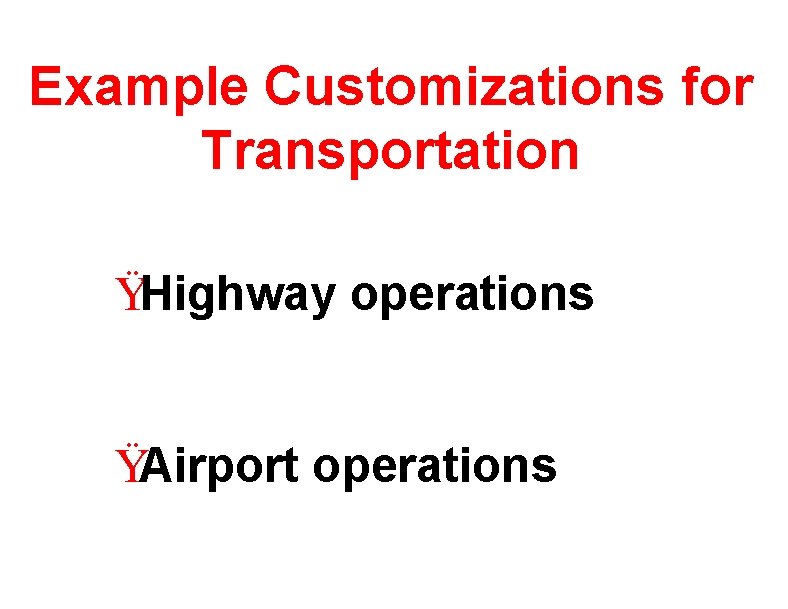
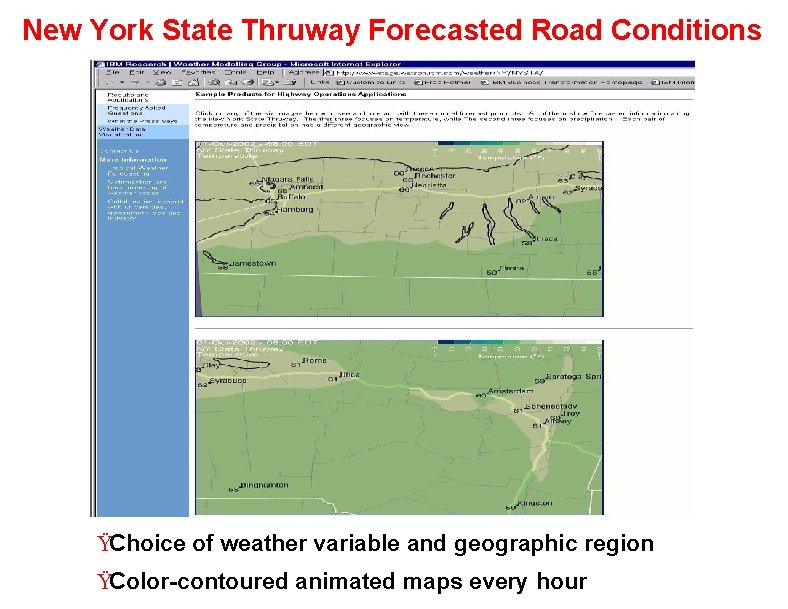
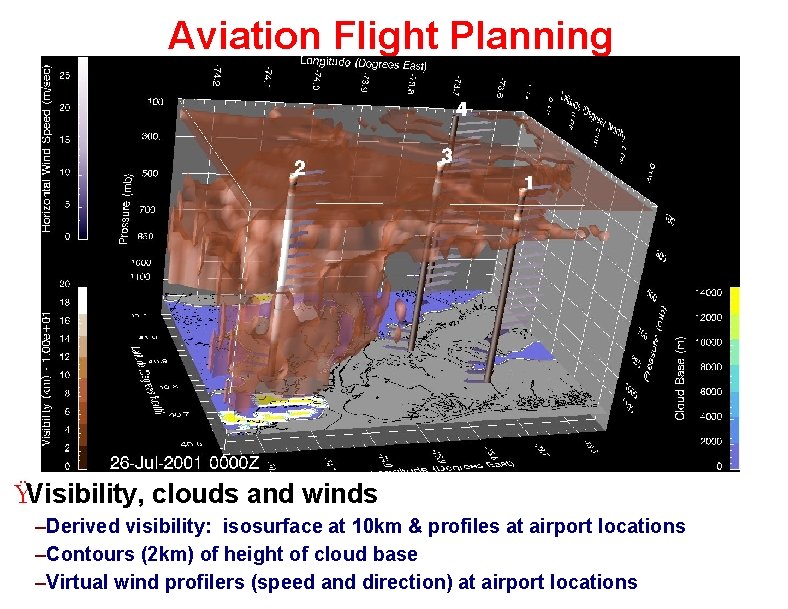
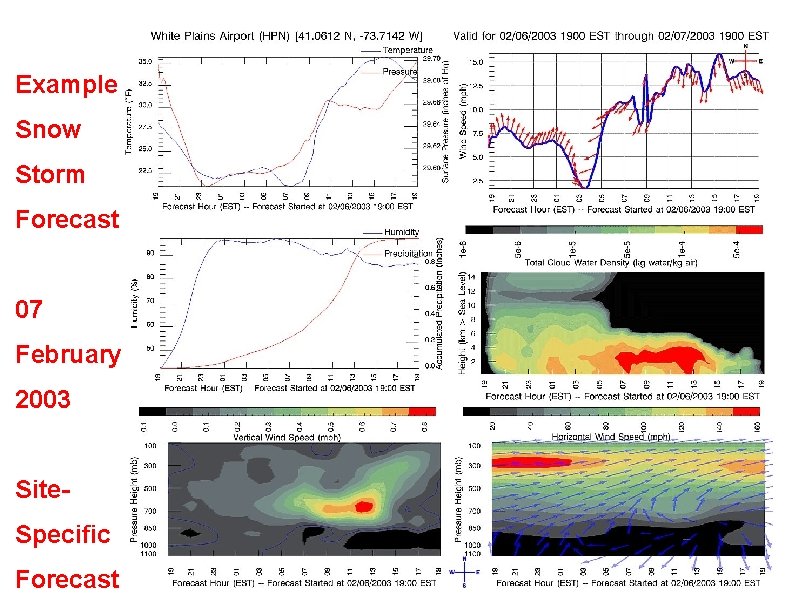
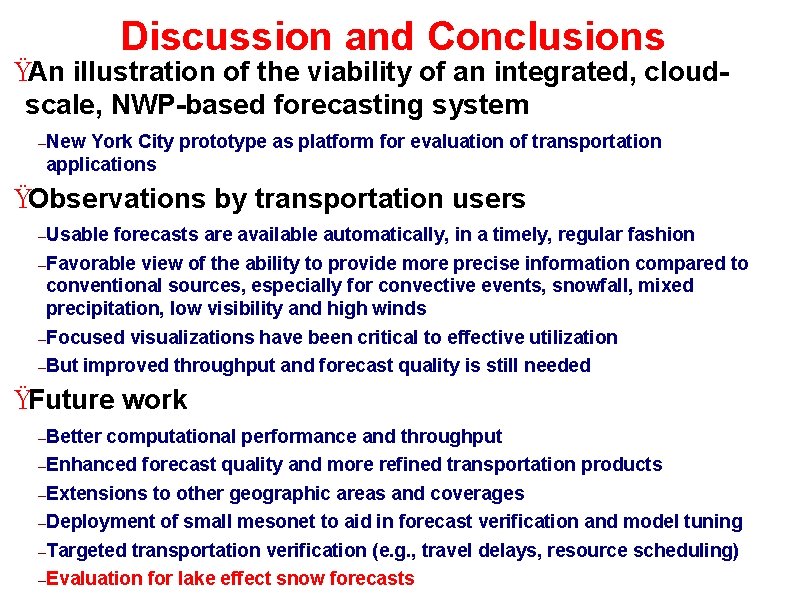
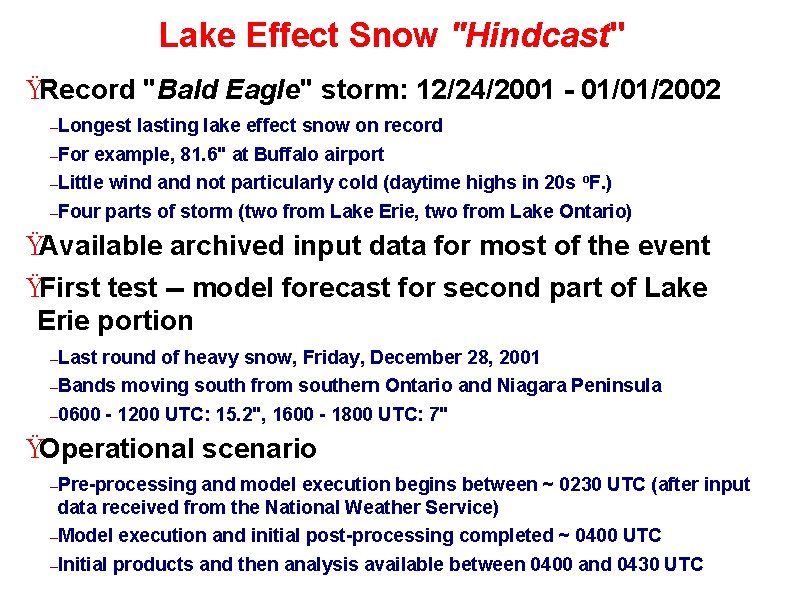
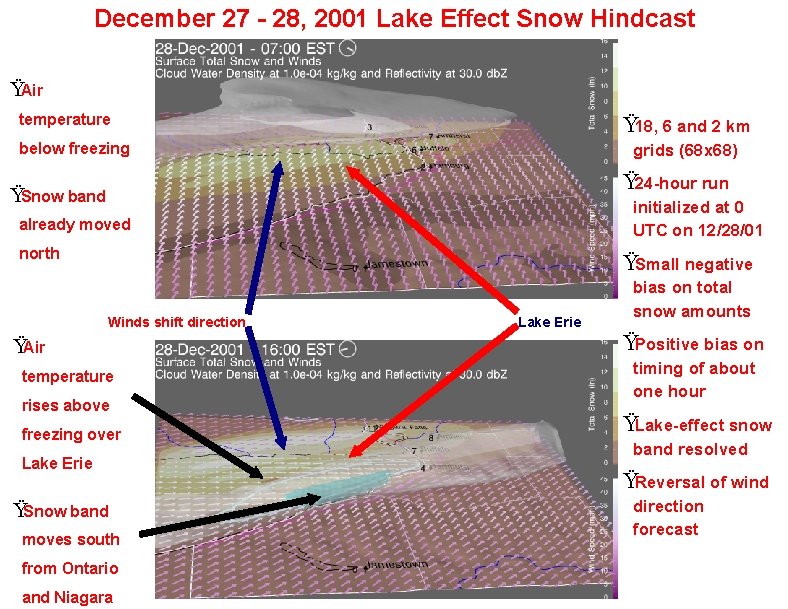
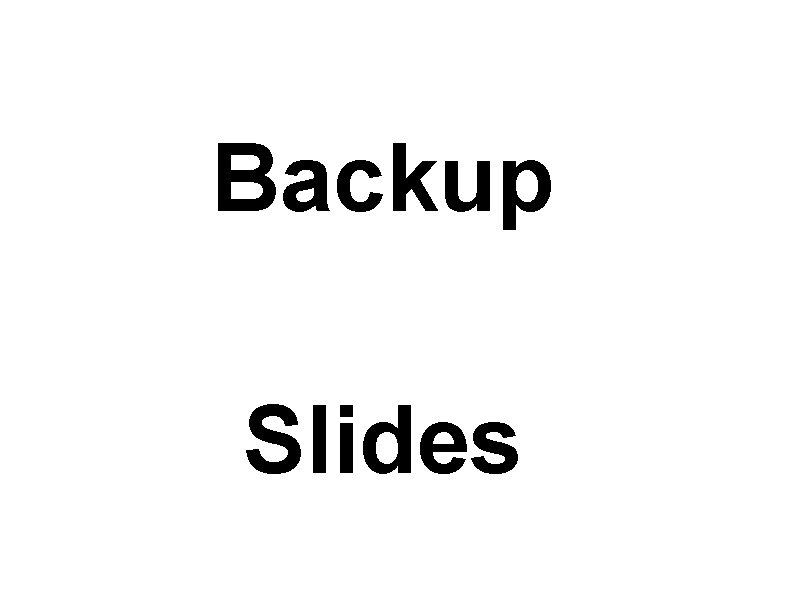
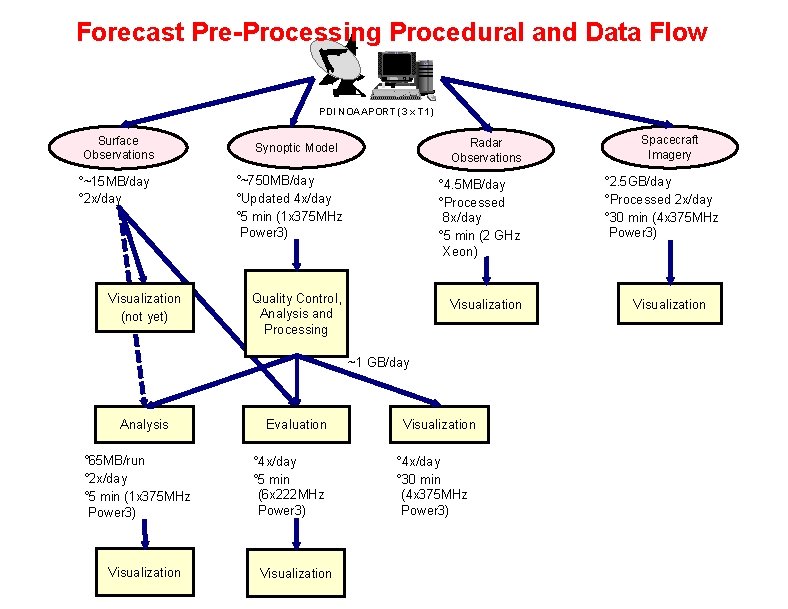
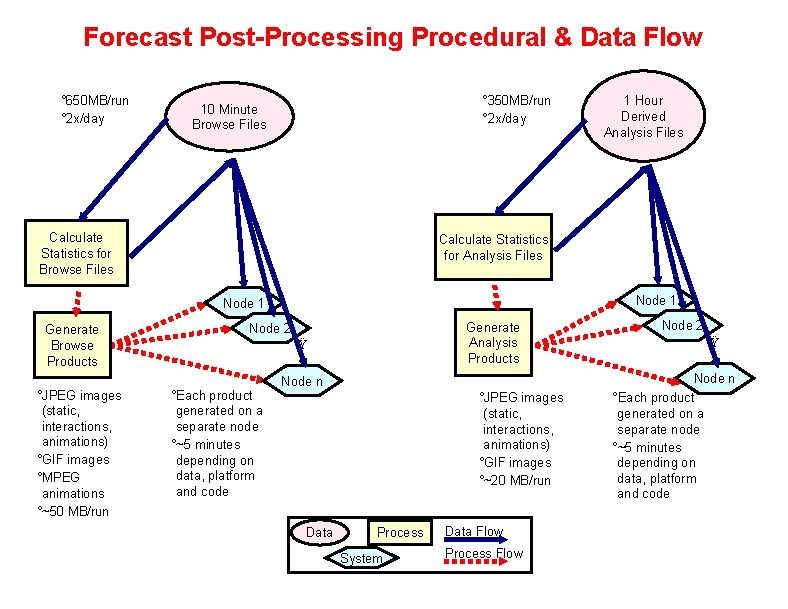
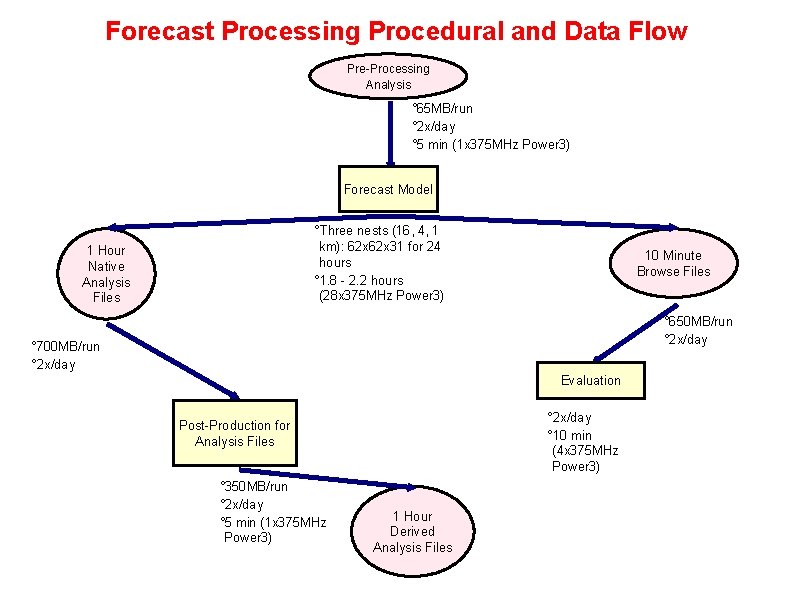
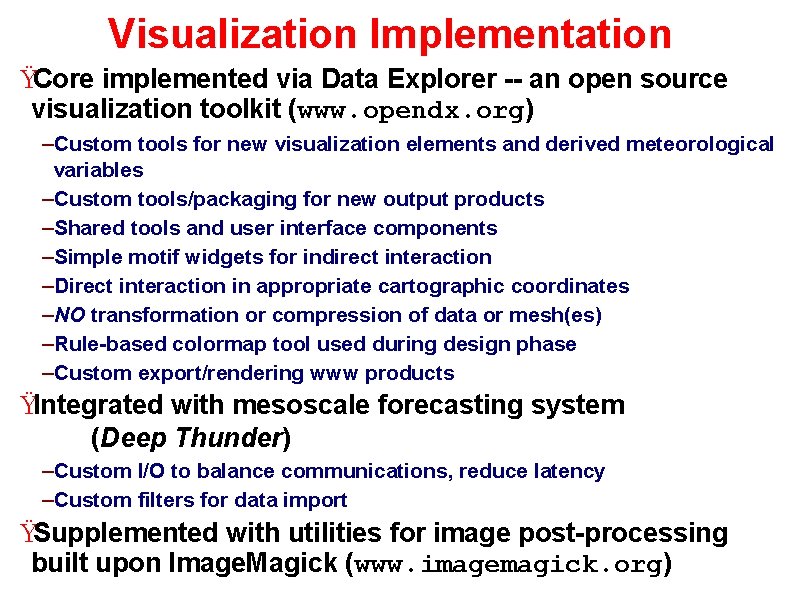
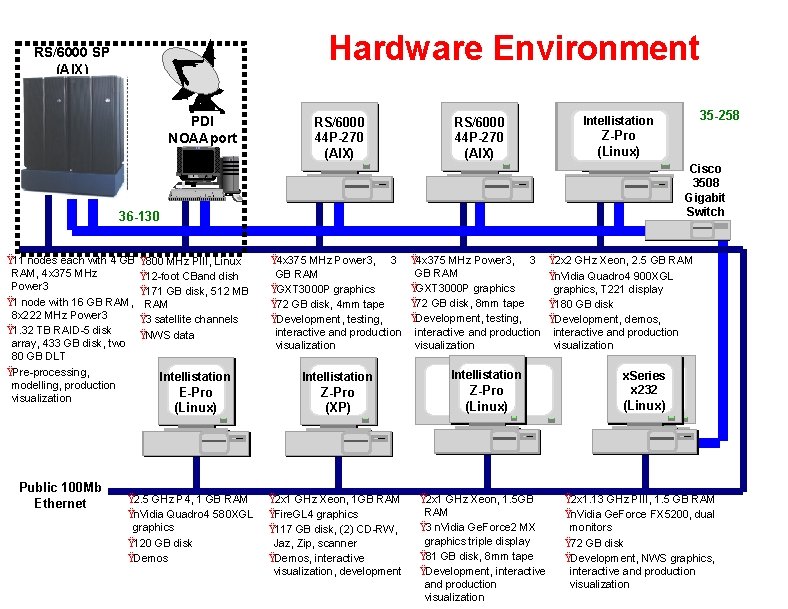
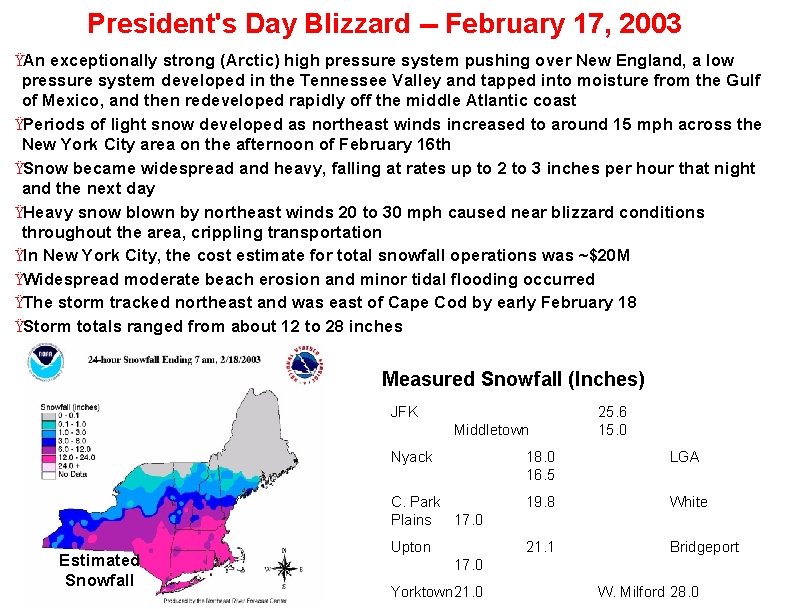
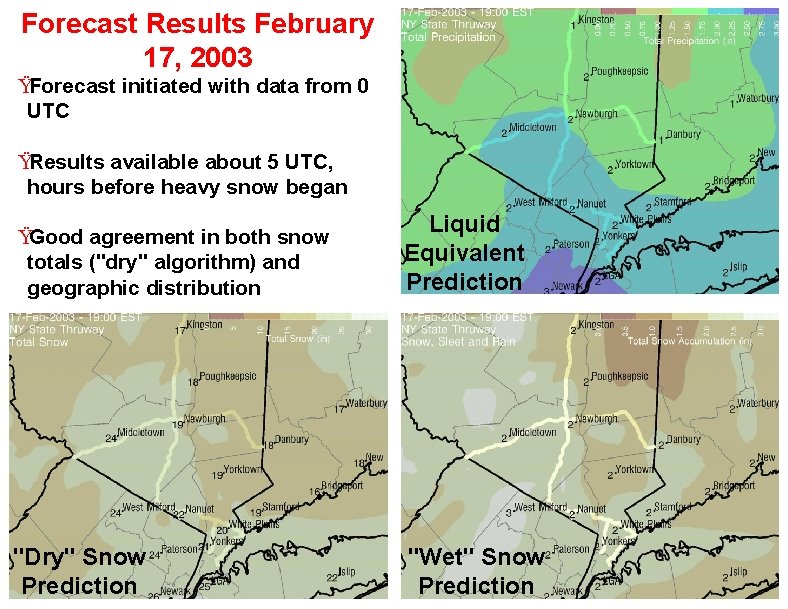
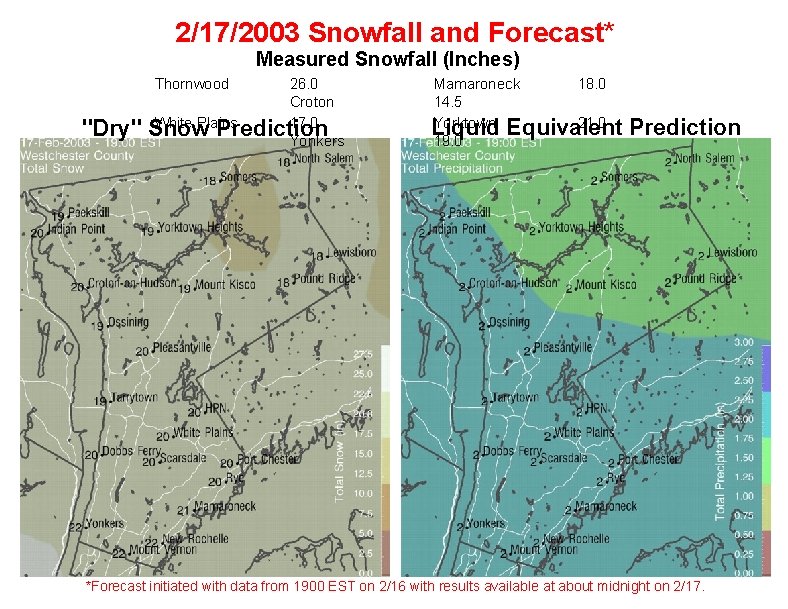
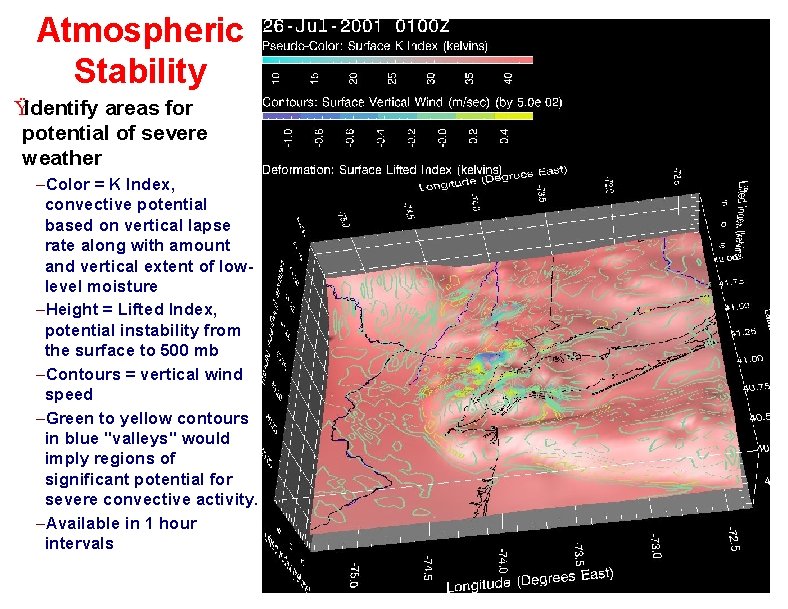
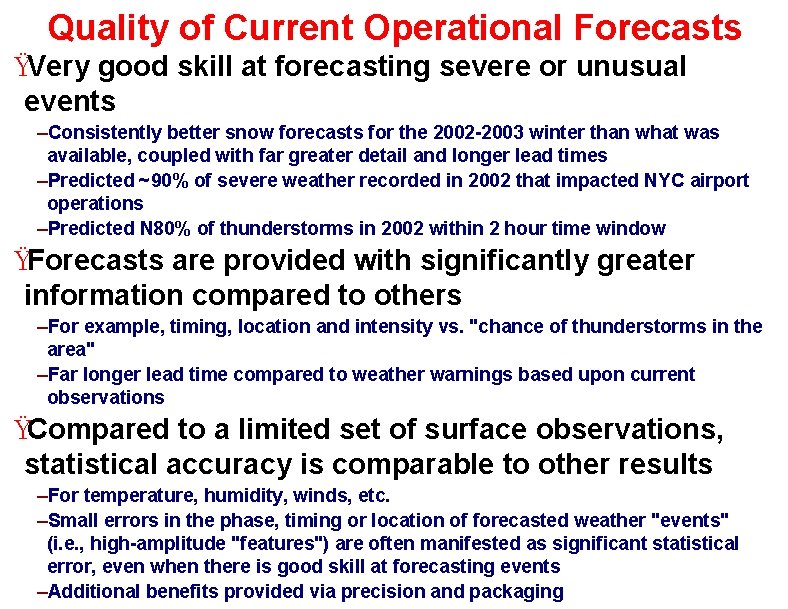
- Slides: 25
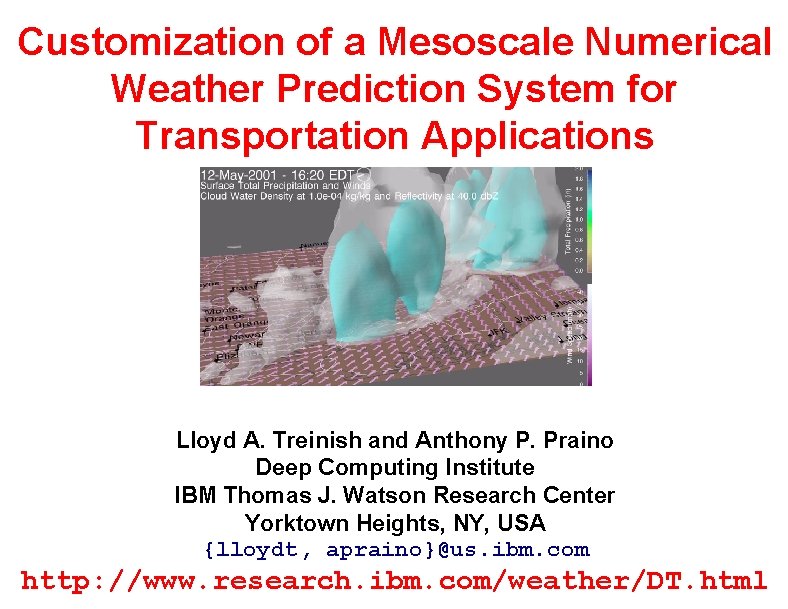
Customization of a Mesoscale Numerical Weather Prediction System for Transportation Applications Lloyd A. Treinish and Anthony P. Praino Deep Computing Institute IBM Thomas J. Watson Research Center Yorktown Heights, NY, USA {lloydt, apraino}@us. ibm. com http: //www. research. ibm. com/weather/DT. html
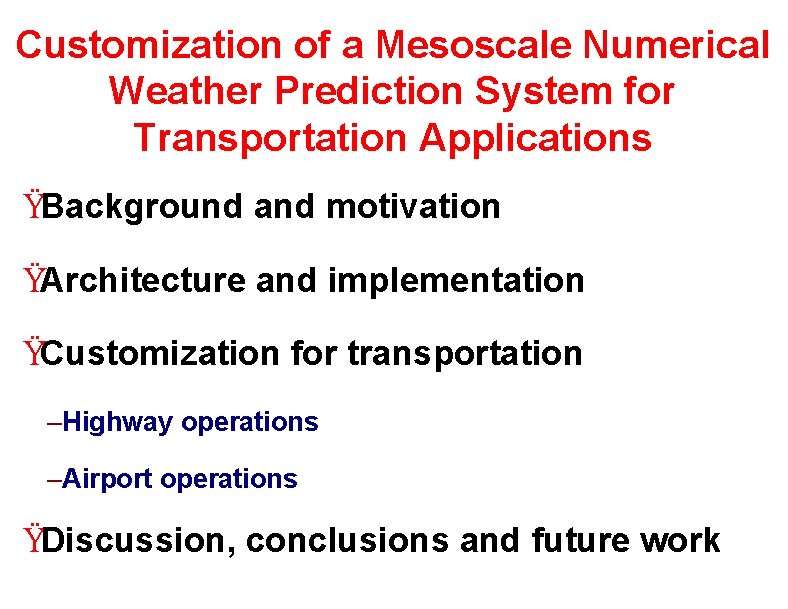
Customization of a Mesoscale Numerical Weather Prediction System for Transportation Applications ŸBackground and motivation ŸArchitecture and implementation ŸCustomization for transportation –Highway operations –Airport operations ŸDiscussion, conclusions and future work
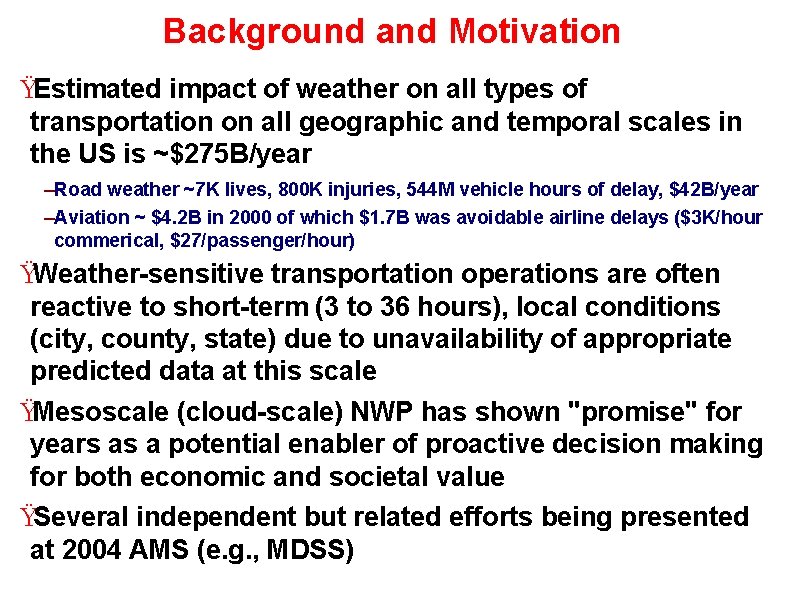
Background and Motivation ŸEstimated impact of weather on all types of transportation on all geographic and temporal scales in the US is ~$275 B/year –Road weather ~7 K lives, 800 K injuries, 544 M vehicle hours of delay, $42 B/year –Aviation ~ $4. 2 B in 2000 of which $1. 7 B was avoidable airline delays ($3 K/hour commerical, $27/passenger/hour) ŸWeather-sensitive transportation operations are often reactive to short-term (3 to 36 hours), local conditions (city, county, state) due to unavailability of appropriate predicted data at this scale ŸMesoscale (cloud-scale) NWP has shown "promise" for years as a potential enabler of proactive decision making for both economic and societal value ŸSeveral independent but related efforts being presented at 2004 AMS (e. g. , MDSS)
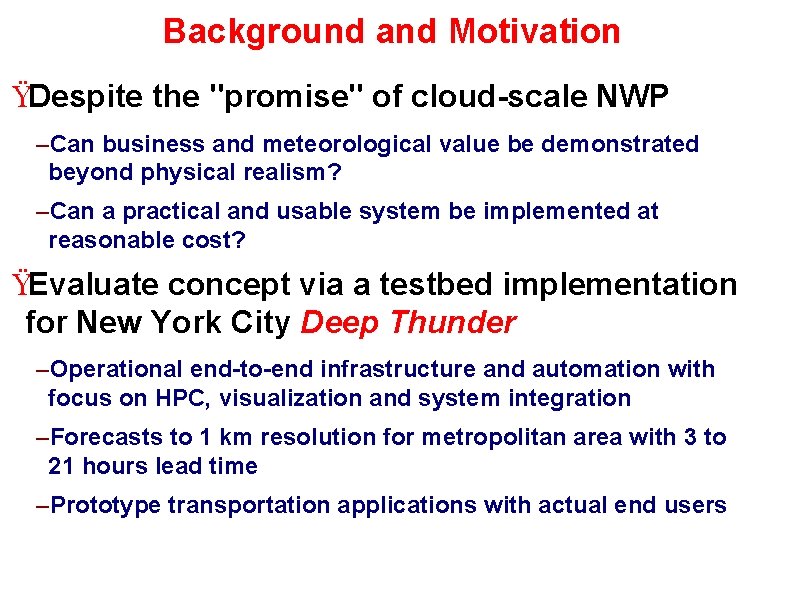
Background and Motivation ŸDespite the "promise" of cloud-scale NWP –Can business and meteorological value be demonstrated beyond physical realism? –Can a practical and usable system be implemented at reasonable cost? ŸEvaluate concept via a testbed implementation for New York City Deep Thunder –Operational end-to-end infrastructure and automation with focus on HPC, visualization and system integration –Forecasts to 1 km resolution for metropolitan area with 3 to 21 hours lead time –Prototype transportation applications with actual end users
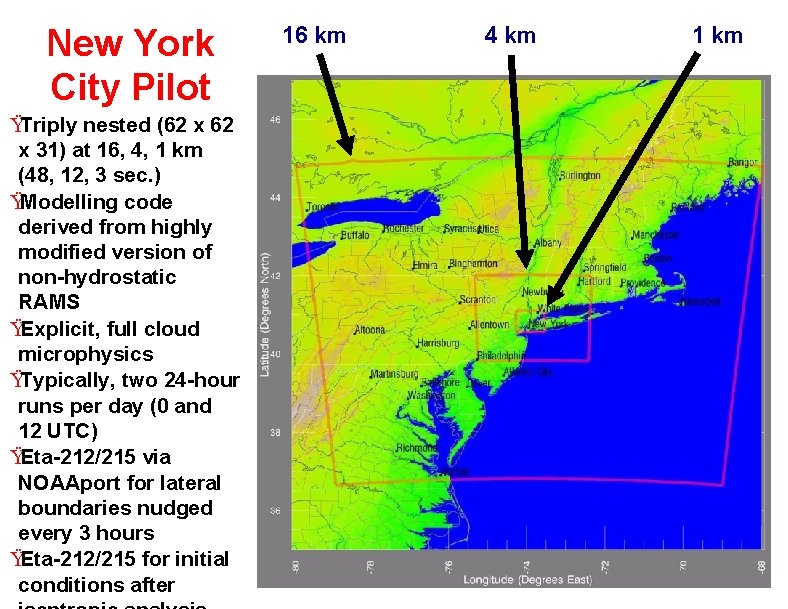
New York City Pilot ŸTriply nested (62 x 31) at 16, 4, 1 km (48, 12, 3 sec. ) ŸModelling code derived from highly modified version of non-hydrostatic RAMS ŸExplicit, full cloud microphysics ŸTypically, two 24 -hour runs per day (0 and 12 UTC) ŸEta-212/215 via NOAAport for lateral boundaries nudged every 3 hours ŸEta-212/215 for initial conditions after 16 km 4 km 1 km
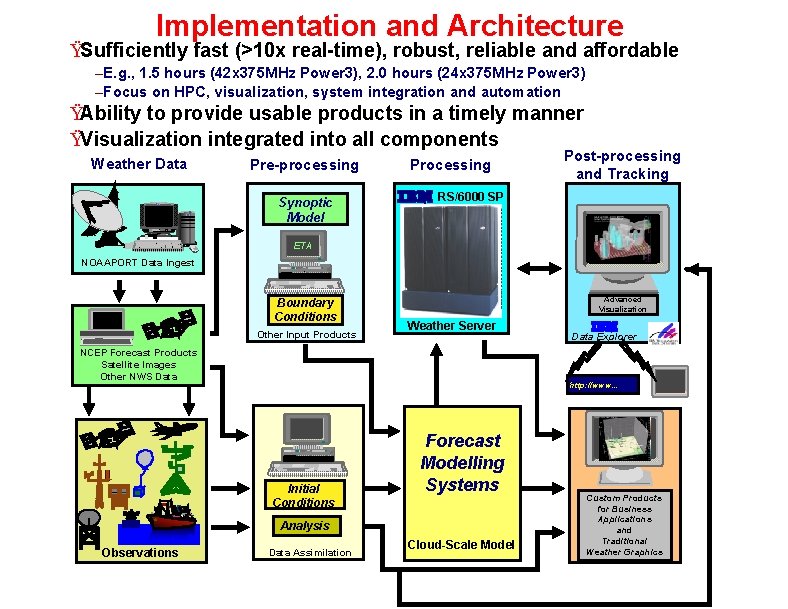
Implementation and Architecture ŸSufficiently fast (>10 x real-time), robust, reliable and affordable –E. g. , 1. 5 hours (42 x 375 MHz Power 3), 2. 0 hours (24 x 375 MHz Power 3) –Focus on HPC, visualization, system integration and automation ŸAbility to provide usable products in a timely manner ŸVisualization integrated into all components Weather Data Pre-processing Synoptic Model Processing Post-processing and Tracking RS/6000 SP ETA NOAAPORT Data Ingest FCST Boundary Conditions Other Input Products Advanced Visualization Weather Server NCEP Forecast Products Satellite Images Other NWS Data http: //www. . . Analysis Initial Conditions Forecast Modelling Systems Analysis Observations Data Explorer Data Assimilation Cloud-Scale Model Custom Products for Business Applications and Traditional Weather Graphics
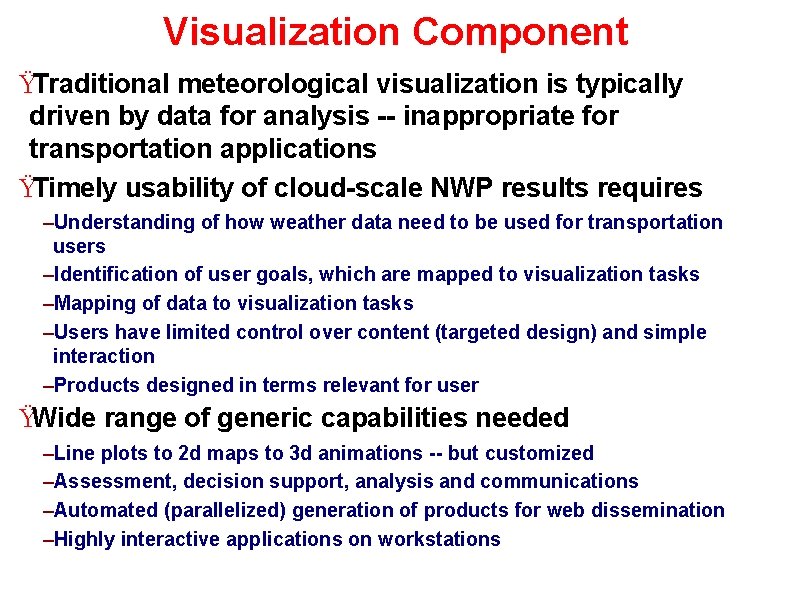
Visualization Component ŸTraditional meteorological visualization is typically driven by data for analysis -- inappropriate for transportation applications ŸTimely usability of cloud-scale NWP results requires –Understanding of how weather data need to be used for transportation users –Identification of user goals, which are mapped to visualization tasks –Mapping of data to visualization tasks –Users have limited control over content (targeted design) and simple interaction –Products designed in terms relevant for user ŸWide range of generic capabilities needed –Line plots to 2 d maps to 3 d animations -- but customized –Assessment, decision support, analysis and communications –Automated (parallelized) generation of products for web dissemination –Highly interactive applications on workstations
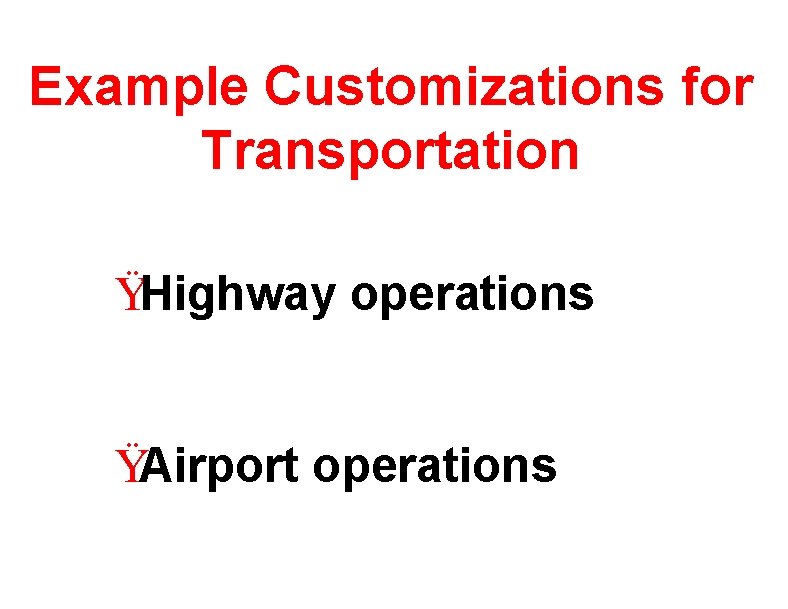
Example Customizations for Transportation ŸHighway operations ŸAirport operations
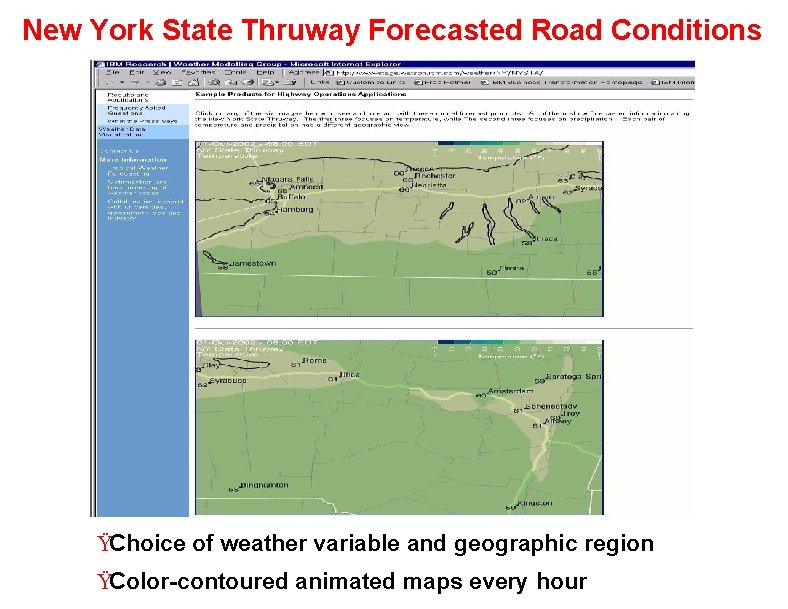
New York State Thruway Forecasted Road Conditions ŸChoice of weather variable and geographic region ŸColor-contoured animated maps every hour
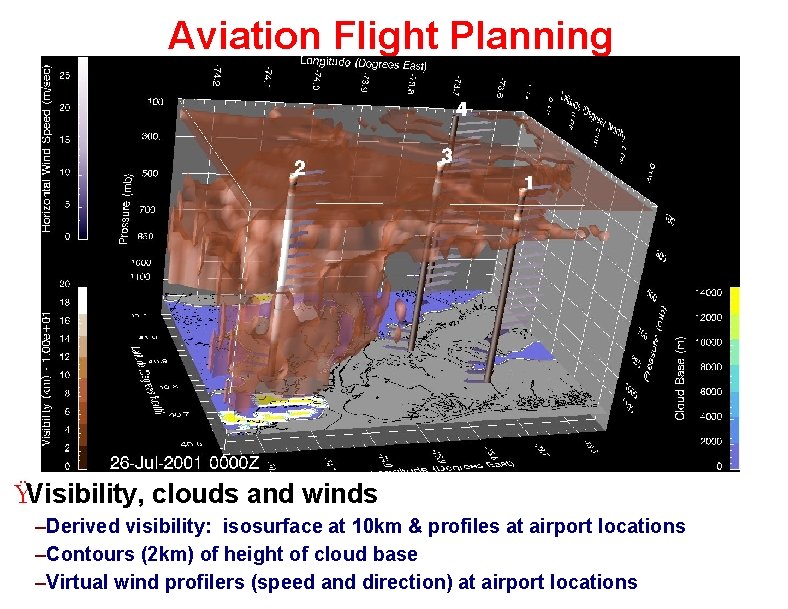
Aviation Flight Planning ŸVisibility, clouds and winds –Derived visibility: isosurface at 10 km & profiles at airport locations –Contours (2 km) of height of cloud base –Virtual wind profilers (speed and direction) at airport locations
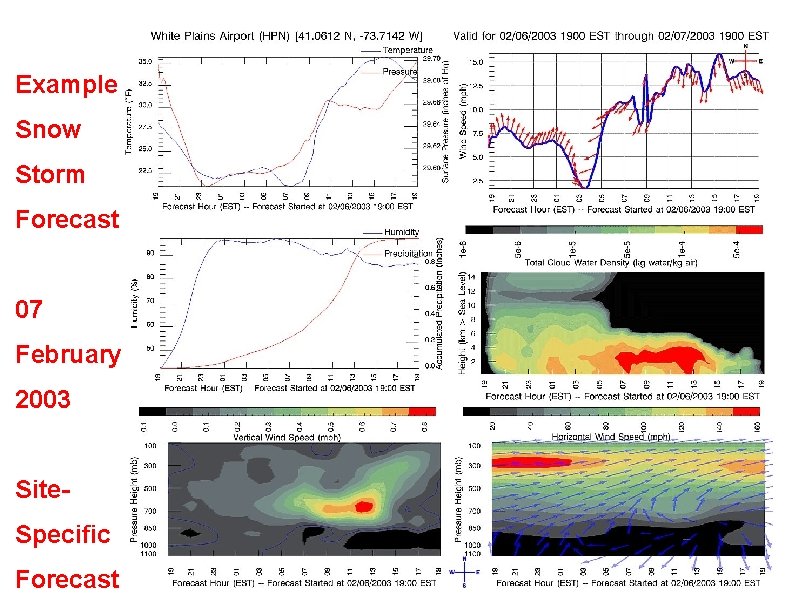
Example Snow Storm Forecast 07 February 2003 Site. Specific Forecast
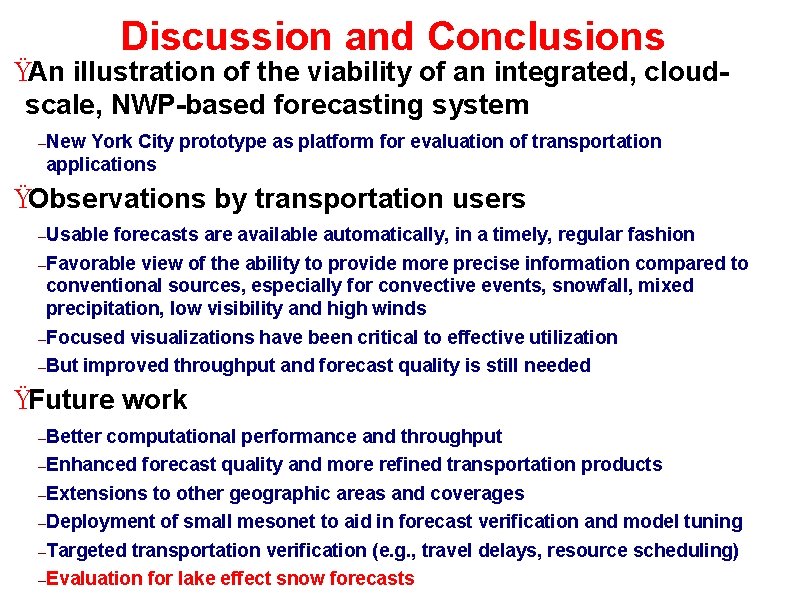
Discussion and Conclusions ŸAn illustration of the viability of an integrated, cloudscale, NWP-based forecasting system –New York City prototype as platform for evaluation of transportation applications ŸObservations by transportation users –Usable forecasts are available automatically, in a timely, regular fashion –Favorable view of the ability to provide more precise information compared to conventional sources, especially for convective events, snowfall, mixed precipitation, low visibility and high winds –Focused –But visualizations have been critical to effective utilization improved throughput and forecast quality is still needed ŸFuture work –Better computational performance and throughput –Enhanced forecast quality and more refined transportation products –Extensions to other geographic areas and coverages –Deployment –Targeted of small mesonet to aid in forecast verification and model tuning transportation verification (e. g. , travel delays, resource scheduling) –Evaluation for lake effect snow forecasts
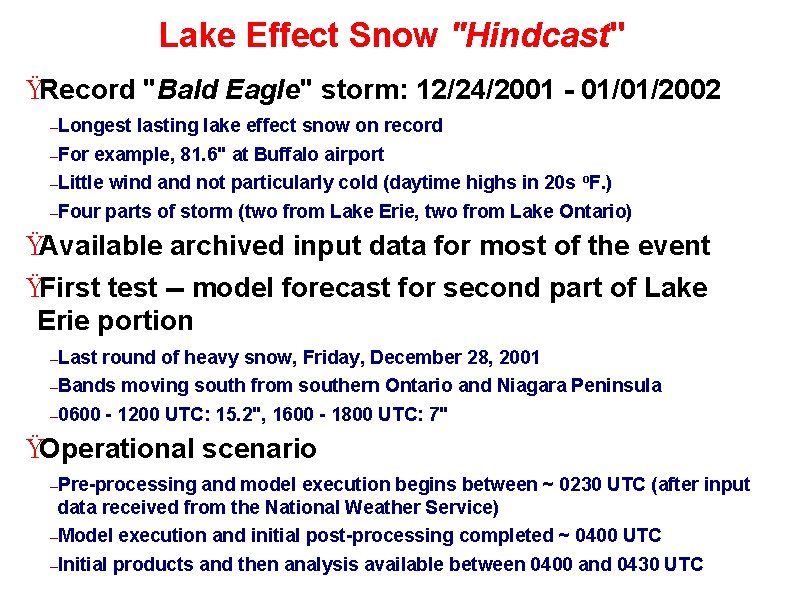
Lake Effect Snow "Hindcast" ŸRecord "Bald Eagle" storm: 12/24/2001 - 01/01/2002 –Longest lasting lake effect snow on record –For example, 81. 6" at Buffalo airport –Little wind and not particularly cold (daytime highs in 20 s o. F. ) –Four parts of storm (two from Lake Erie, two from Lake Ontario) ŸAvailable archived input data for most of the event ŸFirst test -- model forecast for second part of Lake Erie portion –Last round of heavy snow, Friday, December 28, 2001 –Bands – 0600 moving south from southern Ontario and Niagara Peninsula - 1200 UTC: 15. 2", 1600 - 1800 UTC: 7" ŸOperational scenario –Pre-processing and model execution begins between ~ 0230 UTC (after input data received from the National Weather Service) –Model –Initial execution and initial post-processing completed ~ 0400 UTC products and then analysis available between 0400 and 0430 UTC
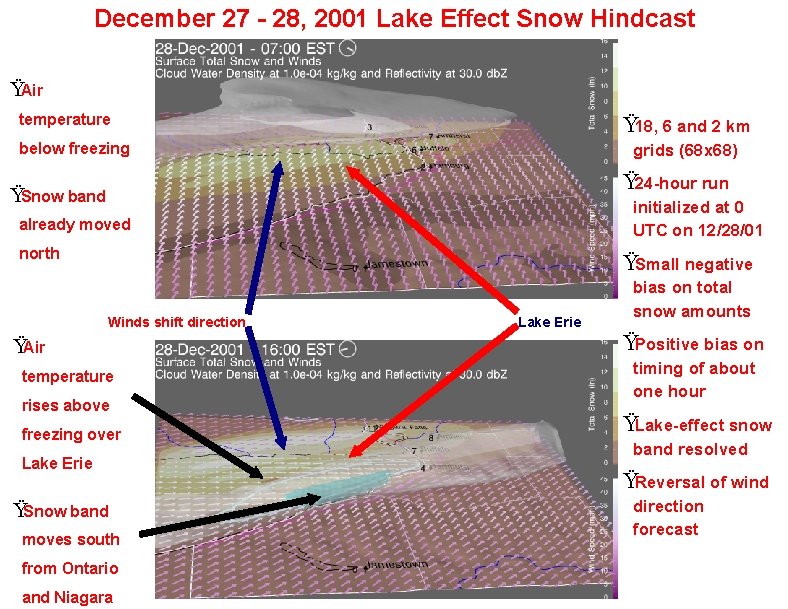
December 27 - 28, 2001 Lake Effect Snow Hindcast ŸAir temperature Ÿ 18, 6 and 2 km below freezing grids (68 x 68) Ÿ 24 -hour run ŸSnow band initialized at 0 UTC on 12/28/01 already moved north ŸSmall negative Winds shift direction ŸAir temperature rises above freezing over Lake Erie ŸSnow band moves south from Ontario and Niagara Lake Erie bias on total snow amounts ŸPositive bias on timing of about one hour ŸLake-effect snow band resolved ŸReversal of wind direction forecast
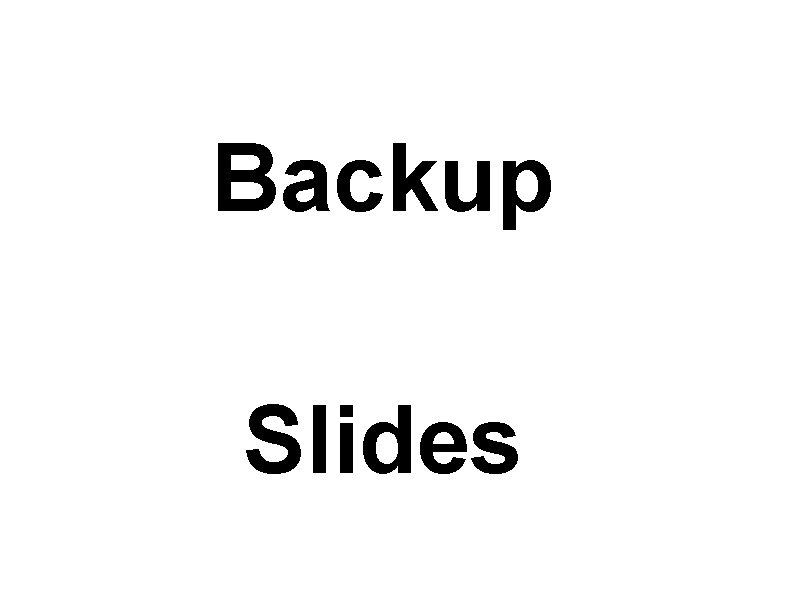
Backup Slides
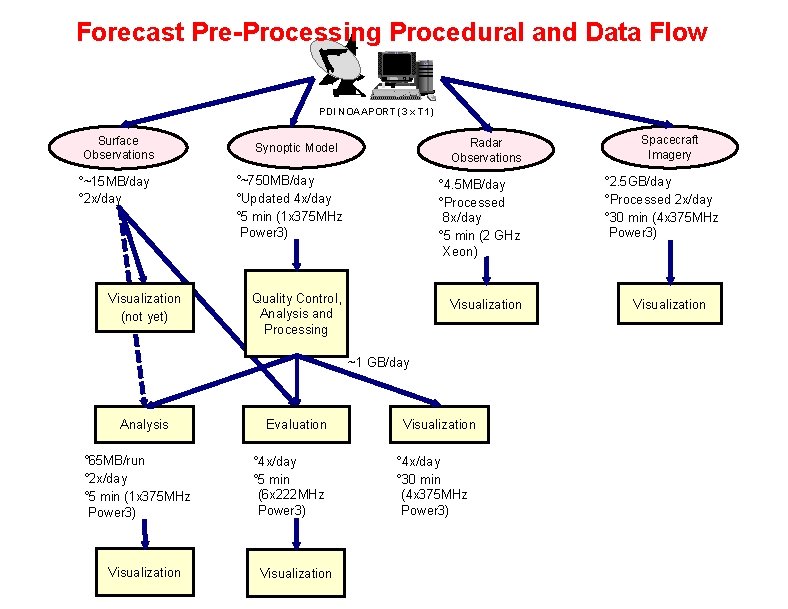
Forecast Pre-Processing Procedural and Data Flow PDI NOAAPORT (3 x T 1) Surface Observations °~15 MB/day ° 2 x/day Visualization (not yet) Radar Observations Synoptic Model °~750 MB/day °Updated 4 x/day ° 5 min (1 x 375 MHz Power 3) ° 4. 5 MB/day °Processed 8 x/day ° 5 min (2 GHz Xeon) Quality Control, Analysis and Processing Visualization ~1 GB/day Analysis ° 65 MB/run ° 2 x/day ° 5 min (1 x 375 MHz Power 3) Visualization Evaluation ° 4 x/day ° 5 min (6 x 222 MHz Power 3) Visualization ° 4 x/day ° 30 min (4 x 375 MHz Power 3) Spacecraft Imagery ° 2. 5 GB/day °Processed 2 x/day ° 30 min (4 x 375 MHz Power 3) Visualization
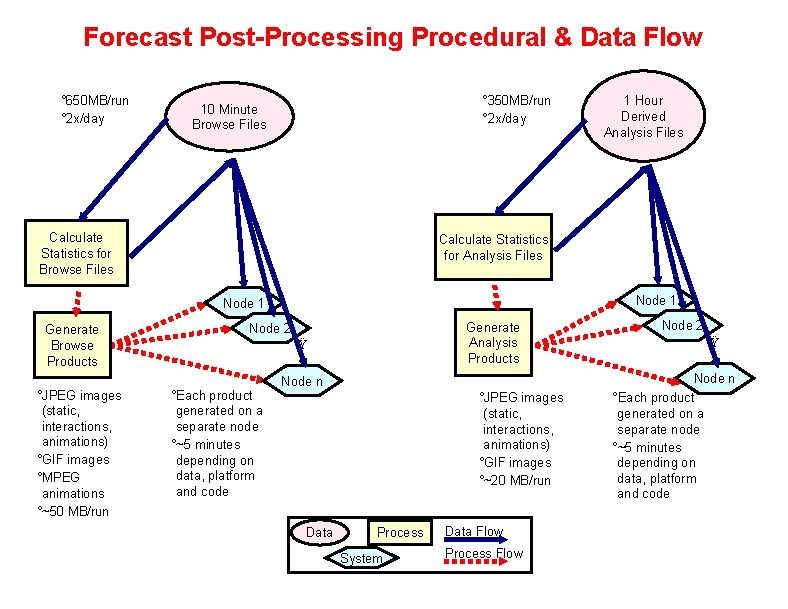
Forecast Post-Processing Procedural & Data Flow ° 650 MB/run ° 2 x/day ° 350 MB/run ° 2 x/day 10 Minute Browse Files Calculate Statistics for Analysis Files Node 1 Generate Browse Products °JPEG images (static, interactions, animations) °GIF images °MPEG animations °~50 MB/run 1 Hour Derived Analysis Files Generate Analysis Products Node 2 Ÿ °Each product generated on a separate node °~5 minutes depending on data, platform and code Node n °JPEG images (static, interactions, animations) °GIF images °~20 MB/run Data Process System Data Flow Process Flow Node 2 Ÿ Node n °Each product generated on a separate node °~5 minutes depending on data, platform and code
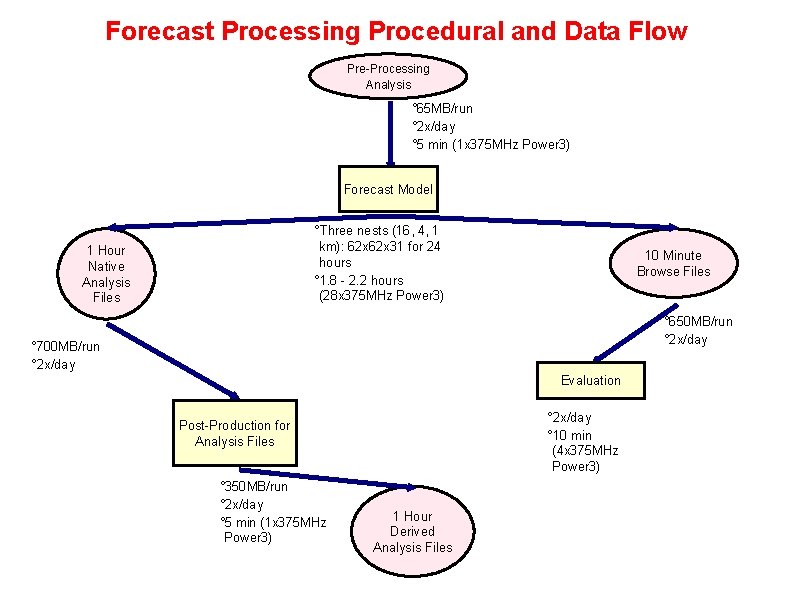
Forecast Processing Procedural and Data Flow Pre-Processing Analysis ° 65 MB/run ° 2 x/day ° 5 min (1 x 375 MHz Power 3) Forecast Model °Three nests (16, 4, 1 km): 62 x 31 for 24 hours ° 1. 8 - 2. 2 hours (28 x 375 MHz Power 3) 1 Hour Native Analysis Files 10 Minute Browse Files ° 650 MB/run ° 2 x/day ° 700 MB/run ° 2 x/day Evaluation ° 2 x/day ° 10 min (4 x 375 MHz Power 3) Post-Production for Analysis Files ° 350 MB/run ° 2 x/day ° 5 min (1 x 375 MHz Power 3) 1 Hour Derived Analysis Files
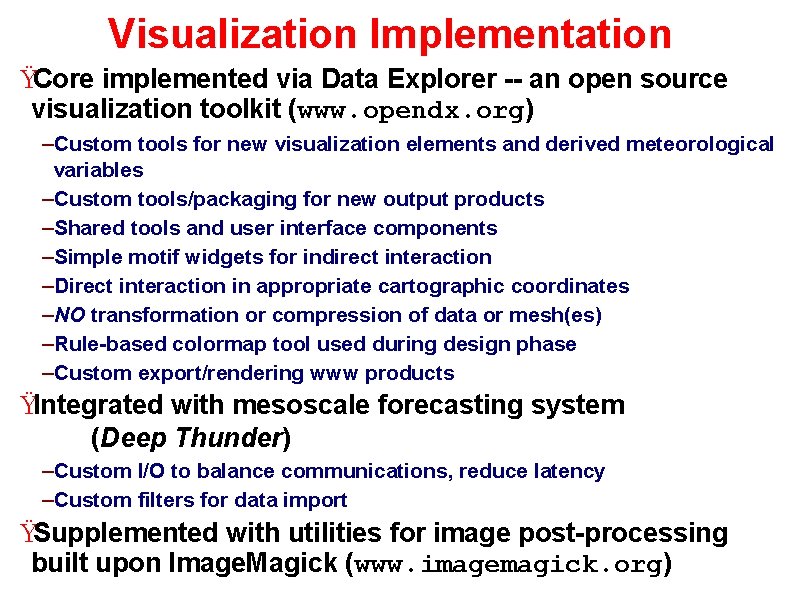
Visualization Implementation ŸCore implemented via Data Explorer -- an open source visualization toolkit (www. opendx. org) –Custom tools for new visualization elements and derived meteorological variables –Custom tools/packaging for new output products –Shared tools and user interface components –Simple motif widgets for indirect interaction –Direct interaction in appropriate cartographic coordinates –NO transformation or compression of data or mesh(es) –Rule-based colormap tool used during design phase –Custom export/rendering www products ŸIntegrated with mesoscale forecasting system (Deep Thunder) –Custom I/O to balance communications, reduce latency –Custom filters for data import ŸSupplemented with utilities for image post-processing built upon Image. Magick (www. imagemagick. org)
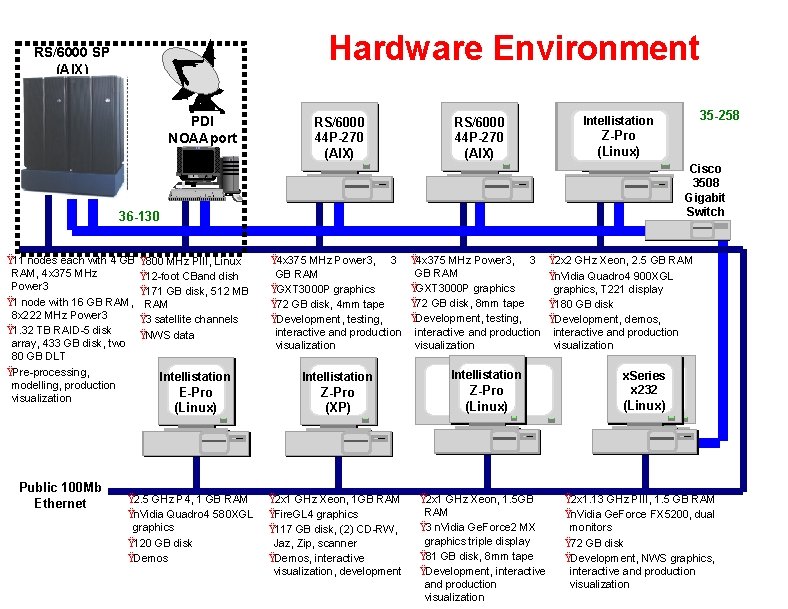
Hardware Environment RS/6000 SP (AIX) PDI NOAAport RS/6000 44 P-270 (AIX) Z-Pro (Linux) Cisco 3508 Gigabit Switch 36 -130 Ÿ 11 nodes each with 4 GB RAM, 4 x 375 MHz Power 3 Ÿ 1 node with 16 GB RAM, 8 x 222 MHz Power 3 Ÿ 1. 32 TB RAID-5 disk array, 433 GB disk, two 80 GB DLT ŸPre-processing, modelling, production visualization Public 100 Mb Ethernet 35 -258 Intellistation Ÿ 800 MHz PIII, Linux Ÿ 12 -foot CBand dish Ÿ 171 GB disk, 512 MB RAM Ÿ 3 satellite channels ŸNWS data Ÿ 4 x 375 MHz Power 3, 3 GB RAM ŸGXT 3000 P graphics Ÿ 72 GB disk, 4 mm tape ŸDevelopment, testing, interactive and production visualization Intellistation E-Pro (Linux) Z-Pro (XP) Ÿ 2. 5 GHz P 4, 1 GB RAM Ÿn. Vidia Quadro 4 580 XGL graphics Ÿ 120 GB disk ŸDemos Ÿ 2 x 1 GHz Xeon, 1 GB RAM ŸFire. GL 4 graphics Ÿ 117 GB disk, (2) CD-RW, Jaz, Zip, scanner ŸDemos, interactive visualization, development Ÿ 4 x 375 MHz Power 3, 3 GB RAM ŸGXT 3000 P graphics Ÿ 72 GB disk, 8 mm tape ŸDevelopment, testing, interactive and production visualization Intellistation Z-Pro (Linux) Ÿ 2 x 1 GHz Xeon, 1. 5 GB RAM Ÿ 3 n. Vidia Ge. Force 2 MX graphics triple display Ÿ 81 GB disk, 8 mm tape ŸDevelopment, interactive and production visualization Ÿ 2 x 2 GHz Xeon, 2. 5 GB RAM Ÿn. Vidia Quadro 4 900 XGL graphics, T 221 display Ÿ 180 GB disk ŸDevelopment, demos, interactive and production visualization x. Series x 232 (Linux) Ÿ 2 x 1. 13 GHz PIII, 1. 5 GB RAM Ÿn. Vidia Ge. Force FX 5200, dual monitors Ÿ 72 GB disk ŸDevelopment, NWS graphics, interactive and production visualization
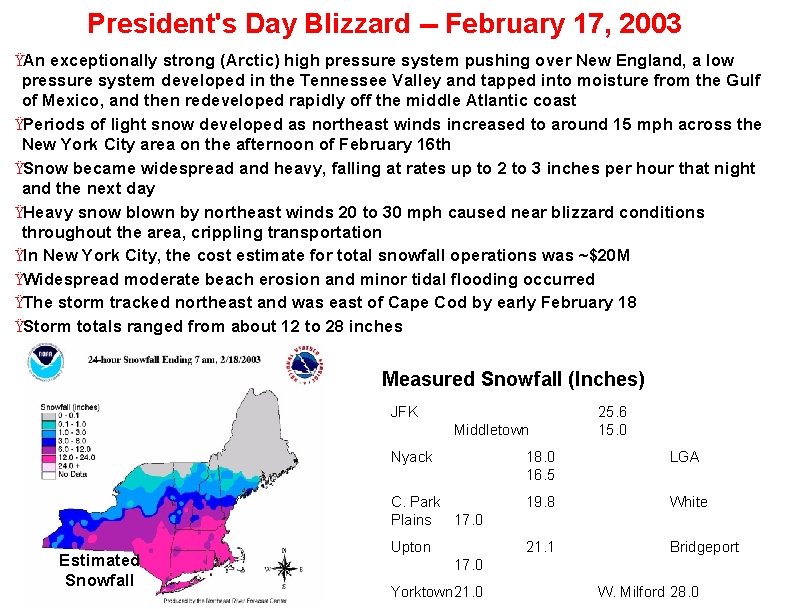
President's Day Blizzard -- February 17, 2003 ŸAn exceptionally strong (Arctic) high pressure system pushing over New England, a low pressure system developed in the Tennessee Valley and tapped into moisture from the Gulf of Mexico, and then redeveloped rapidly off the middle Atlantic coast ŸPeriods of light snow developed as northeast winds increased to around 15 mph across the New York City area on the afternoon of February 16 th ŸSnow became widespread and heavy, falling at rates up to 2 to 3 inches per hour that night and the next day ŸHeavy snow blown by northeast winds 20 to 30 mph caused near blizzard conditions throughout the area, crippling transportation ŸIn New York City, the cost estimate for total snowfall operations was ~$20 M ŸWidespread moderate beach erosion and minor tidal flooding occurred ŸThe storm tracked northeast and was east of Cape Cod by early February 18 ŸStorm totals ranged from about 12 to 28 inches Measured Snowfall (Inches) JFK Middletown Estimated Snowfall 25. 6 15. 0 Nyack 18. 0 16. 5 LGA C. Park Plains 17. 0 19. 8 White Upton 21. 1 Bridgeport 17. 0 Yorktown 21. 0 W. Milford 28. 0
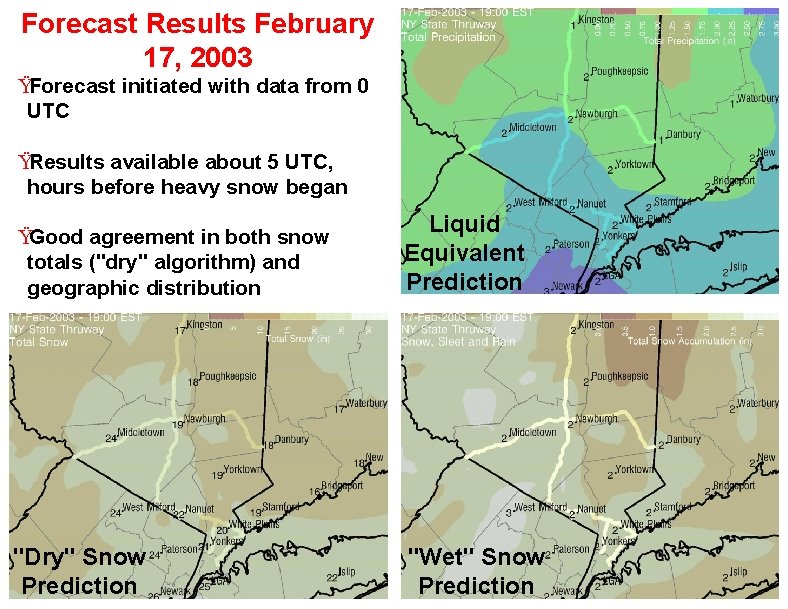
Forecast Results February 17, 2003 ŸForecast initiated with data from 0 UTC ŸResults available about 5 UTC, hours before heavy snow began ŸGood agreement in both snow totals ("dry" algorithm) and geographic distribution Liquid Equivalent Prediction "Dry" Snow Prediction "Wet" Snow Prediction
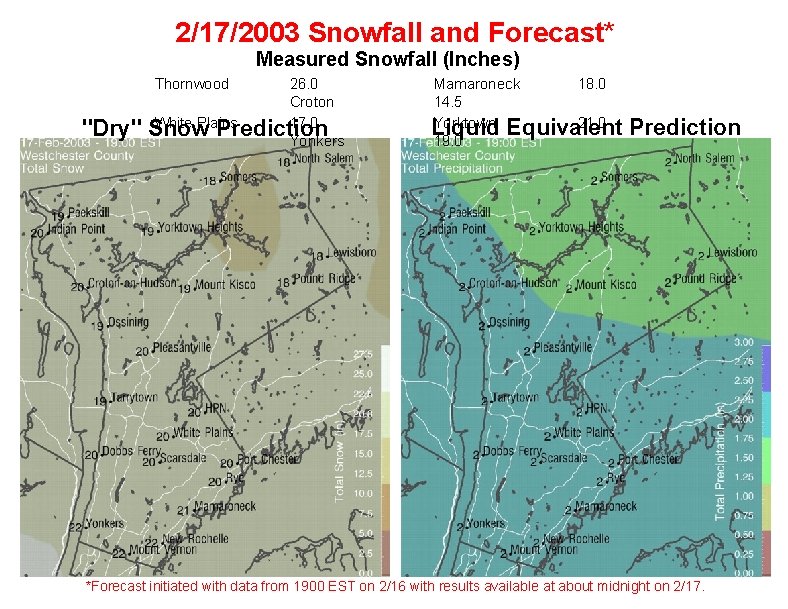
2/17/2003 Snowfall and Forecast* Measured Snowfall (Inches) Thornwood "Dry" 26. 0 Croton White Plains 17. 0 Snow Prediction Yonkers Mamaroneck 18. 0 14. 5 Yorktown Equivalent 21. 0 Liquid 19. 0 Prediction *Forecast initiated with data from 1900 EST on 2/16 with results available at about midnight on 2/17.
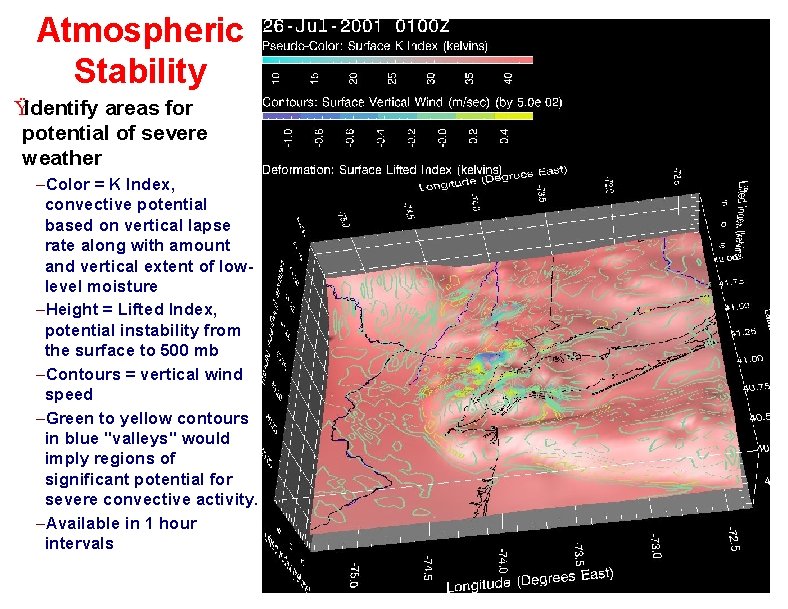
Atmospheric Stability ŸIdentify areas for potential of severe weather –Color = K Index, convective potential based on vertical lapse rate along with amount and vertical extent of lowlevel moisture –Height = Lifted Index, potential instability from the surface to 500 mb –Contours = vertical wind speed –Green to yellow contours in blue "valleys" would imply regions of significant potential for severe convective activity. –Available in 1 hour intervals
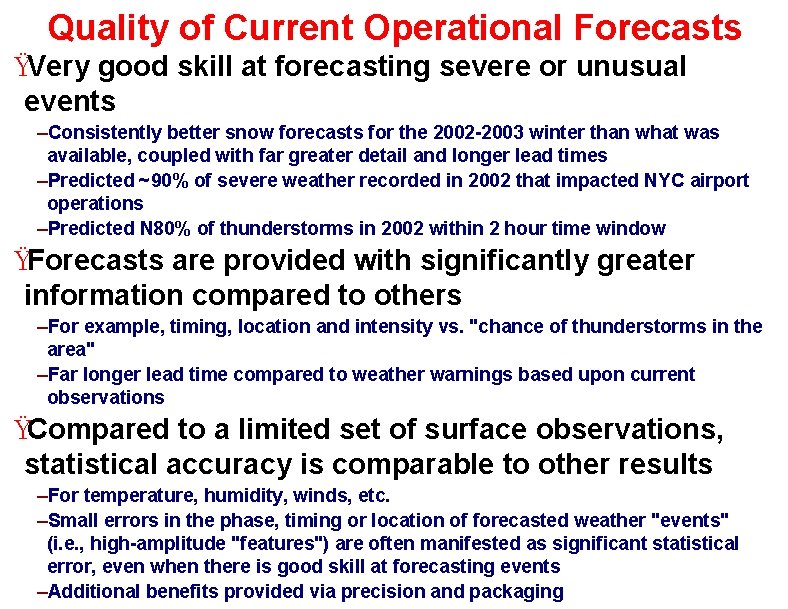
Quality of Current Operational Forecasts ŸVery good skill at forecasting severe or unusual events –Consistently better snow forecasts for the 2002 -2003 winter than what was available, coupled with far greater detail and longer lead times –Predicted ~90% of severe weather recorded in 2002 that impacted NYC airport operations –Predicted N 80% of thunderstorms in 2002 within 2 hour time window ŸForecasts are provided with significantly greater information compared to others –For example, timing, location and intensity vs. "chance of thunderstorms in the area" –Far longer lead time compared to weather warnings based upon current observations ŸCompared to a limited set of surface observations, statistical accuracy is comparable to other results –For temperature, humidity, winds, etc. –Small errors in the phase, timing or location of forecasted weather "events" (i. e. , high-amplitude "features") are often manifested as significant statistical error, even when there is good skill at forecasting events –Additional benefits provided via precision and packaging
Mesoscale definition
Pfms bulk customization
Mass customization and rapid product development
Prophet 21 dynachange
Mass customization adidas
Process choice
Breadcrumb
My workday yale
Customization tool
Ansys project file management
Nearshore wave prediction system
Station model symbol
Winter tongue twister
Her poem clothing
Sunny rainy cloudy stormy
Whether the weather is fine
Heavy weather by weather report
Capital weather gang weather wall
Teeth numbering system
Will may might for predictions
Championship branch prediction
Corner prediction
Why have the gamemakers sent the thunderstorm
Phd secondary structure prediction
Standard error of prediction
Meritsprediction