CSE 5810 Sensor Networks to Monitor Elderly Yusuf
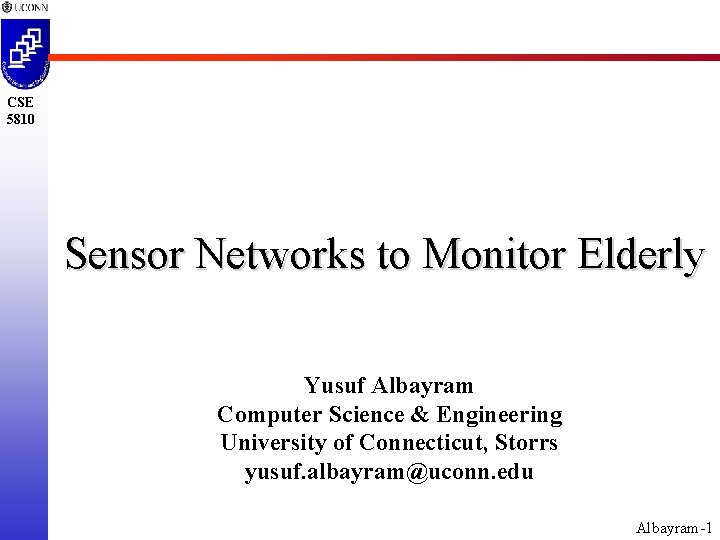
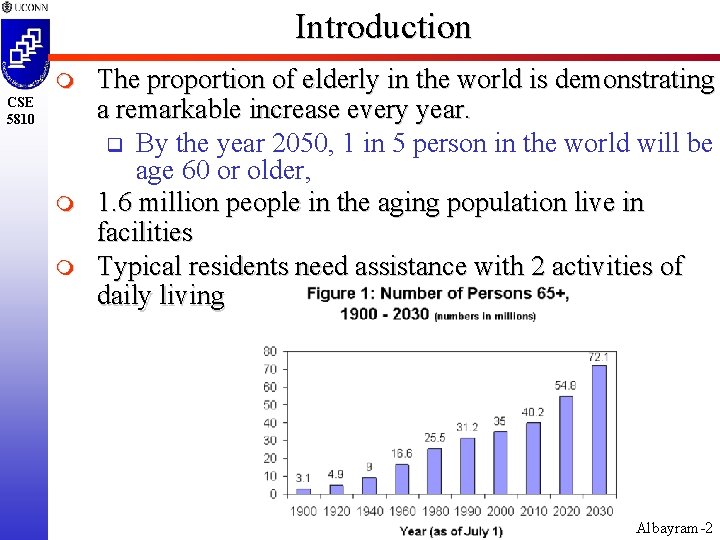
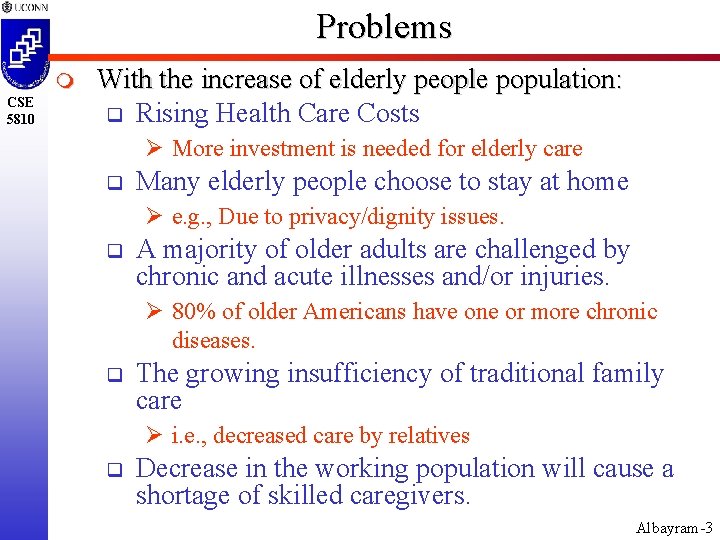
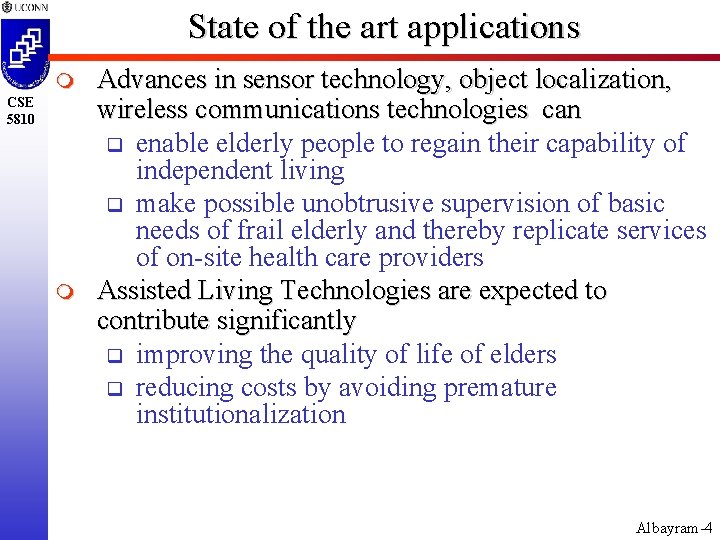
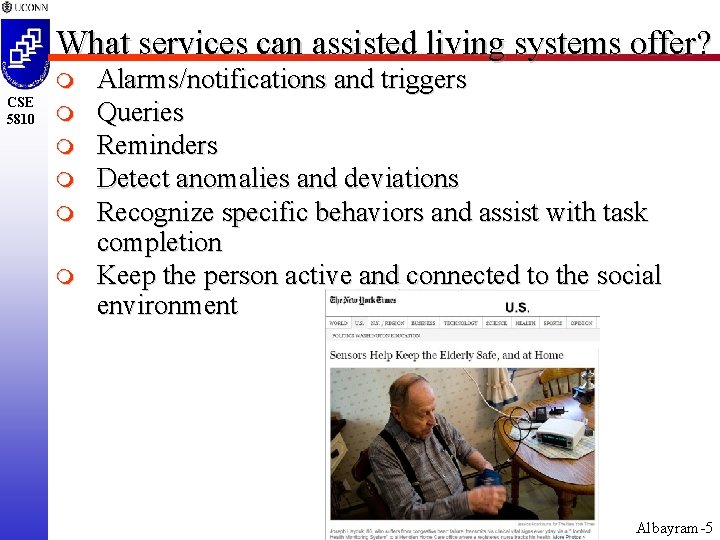
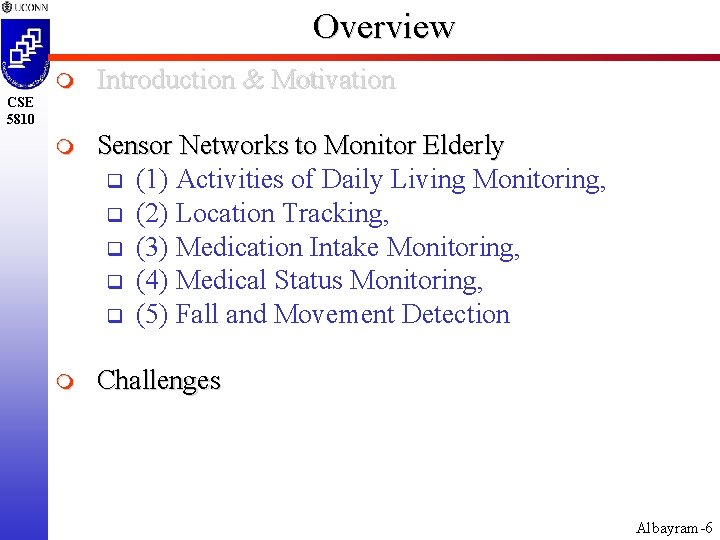
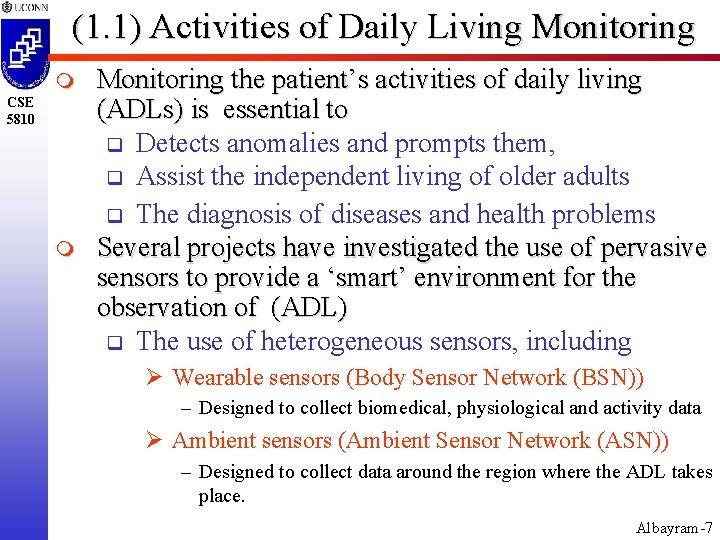
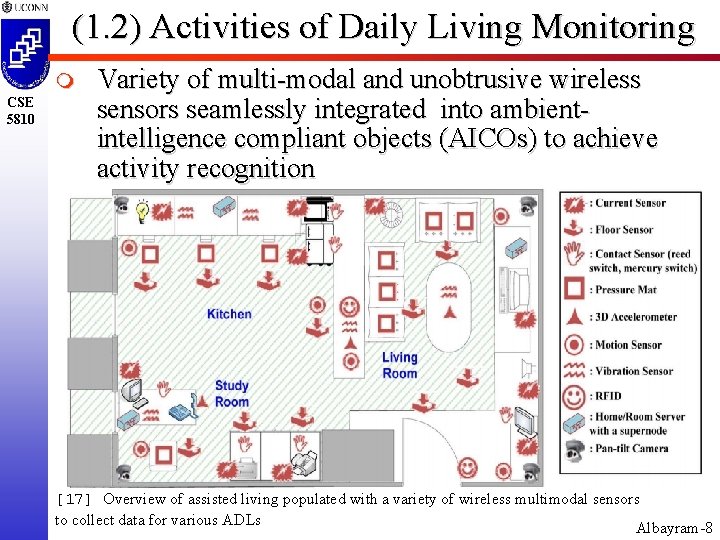
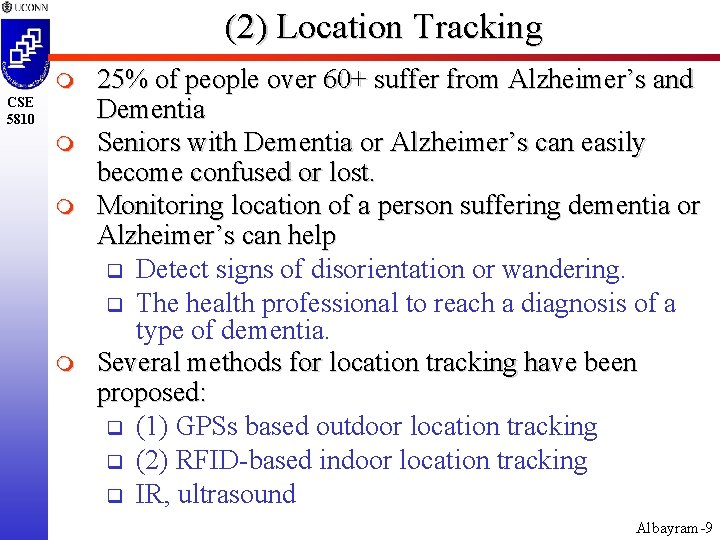
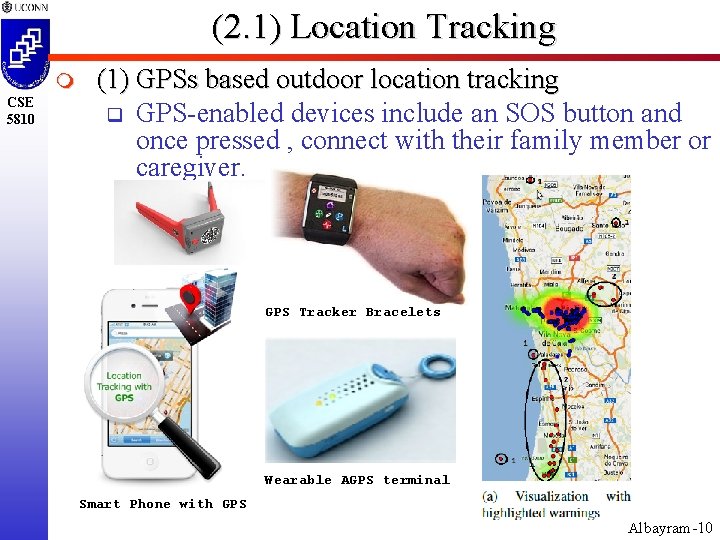
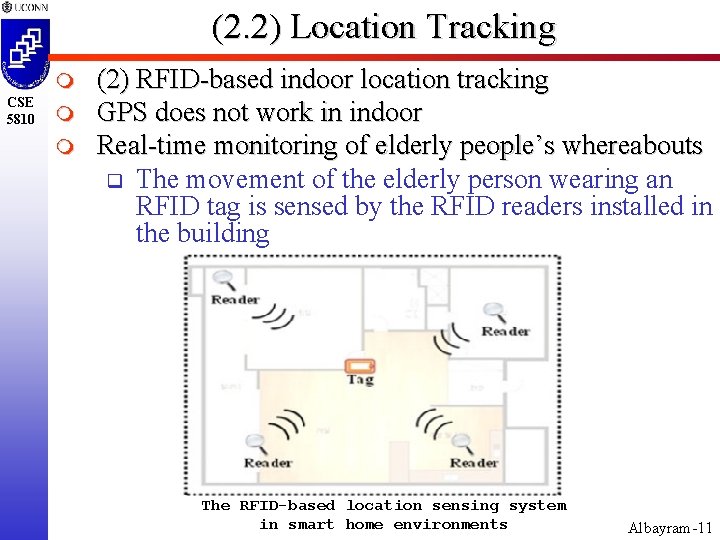
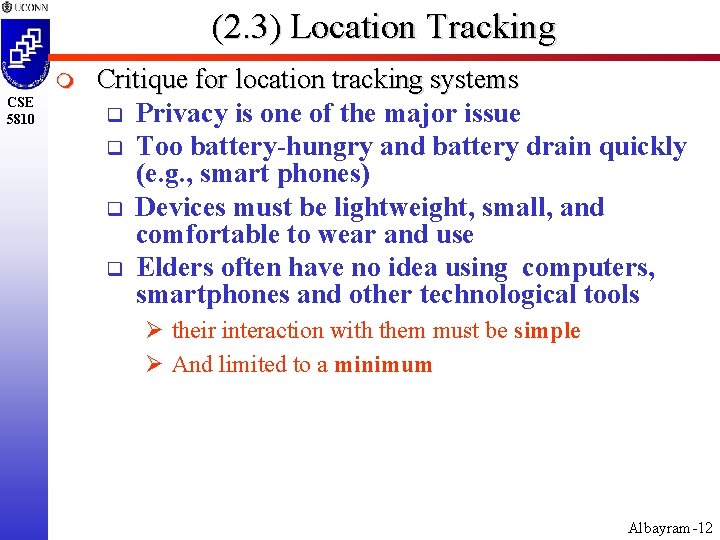
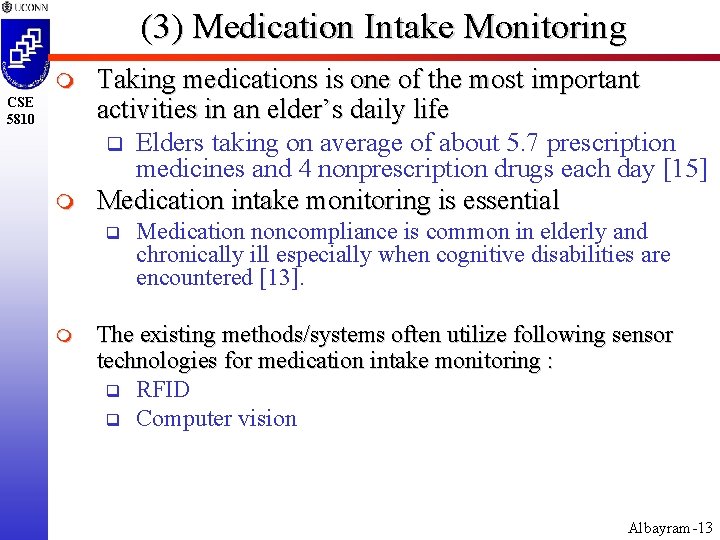
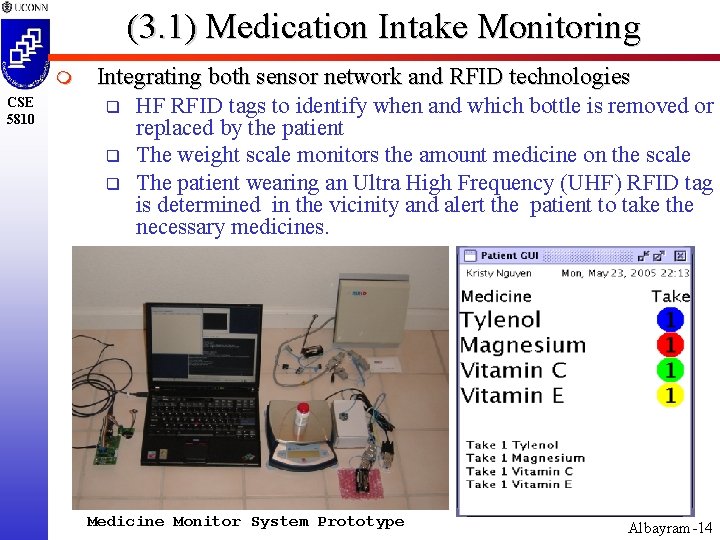
![(3. 2) Medication Intake Monitoring m CSE 5810 Incorporating RFID and video analysis [10] (3. 2) Medication Intake Monitoring m CSE 5810 Incorporating RFID and video analysis [10]](https://slidetodoc.com/presentation_image_h/c6bd3d6d3d9a97aeeb408f0389155153/image-15.jpg)
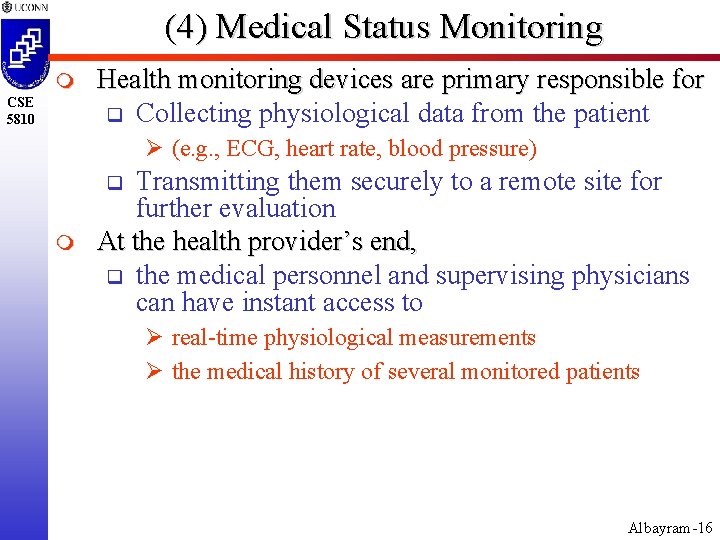
![(4. 1) Medical Status Monitoring CSE 5810 The health monitoring network structure [16] Albayram-17 (4. 1) Medical Status Monitoring CSE 5810 The health monitoring network structure [16] Albayram-17](https://slidetodoc.com/presentation_image_h/c6bd3d6d3d9a97aeeb408f0389155153/image-17.jpg)
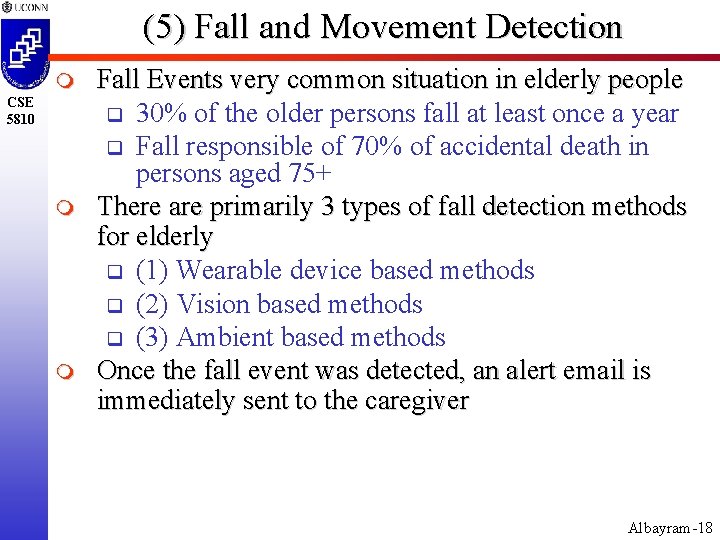
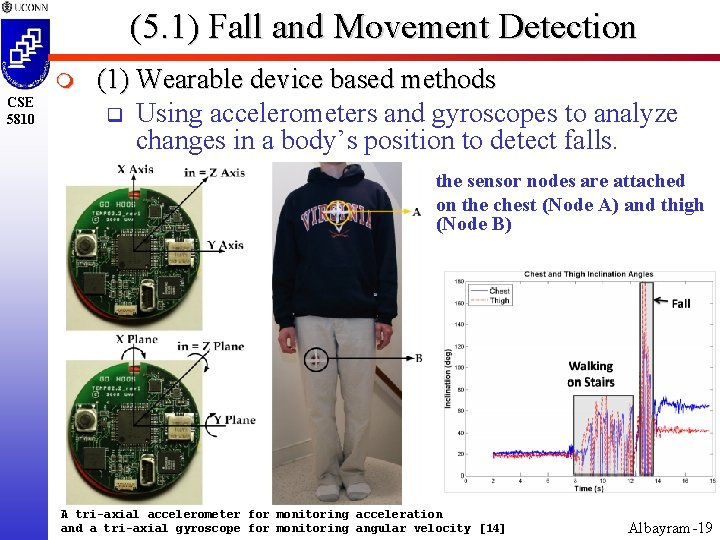
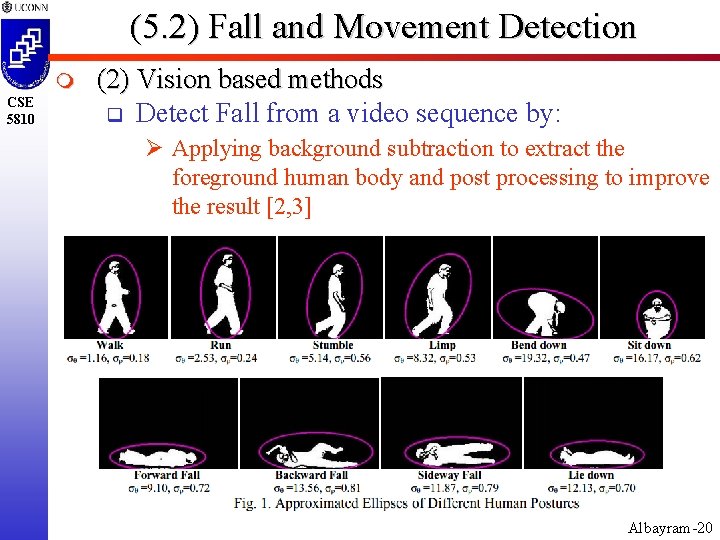
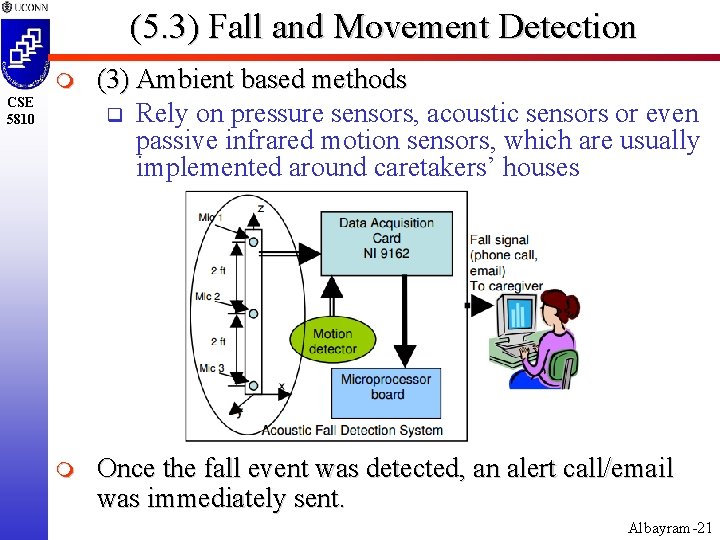
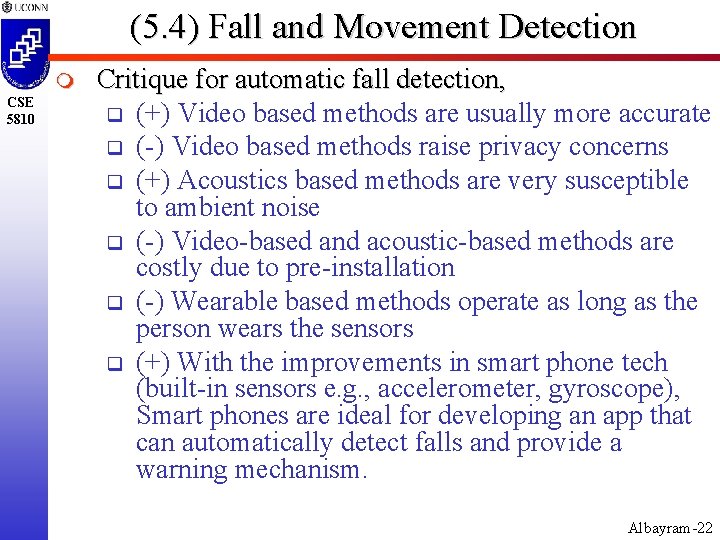
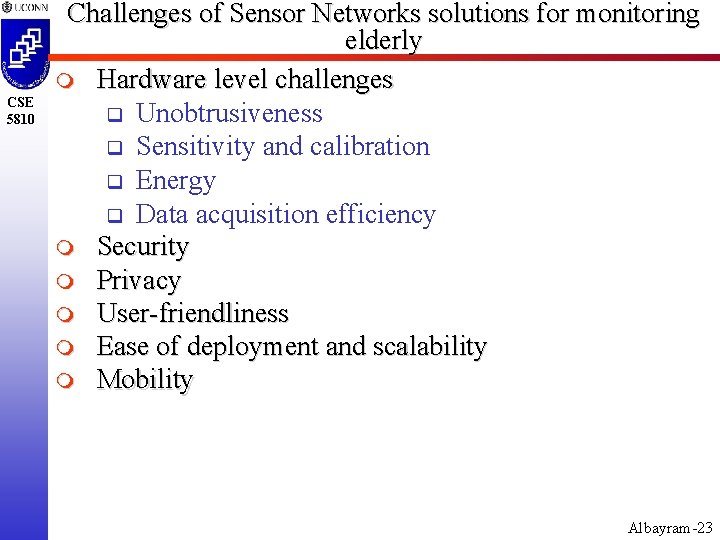
![References CSE 5810 [1] Wang, J. , et al. "An enhanced fall detection system References CSE 5810 [1] Wang, J. , et al. "An enhanced fall detection system](https://slidetodoc.com/presentation_image_h/c6bd3d6d3d9a97aeeb408f0389155153/image-24.jpg)
![References-2 CSE 5810 [11] Pang, Zhibo, Qiang Chen, and Lirong Zheng. "A pervasive and References-2 CSE 5810 [11] Pang, Zhibo, Qiang Chen, and Lirong Zheng. "A pervasive and](https://slidetodoc.com/presentation_image_h/c6bd3d6d3d9a97aeeb408f0389155153/image-25.jpg)
- Slides: 25
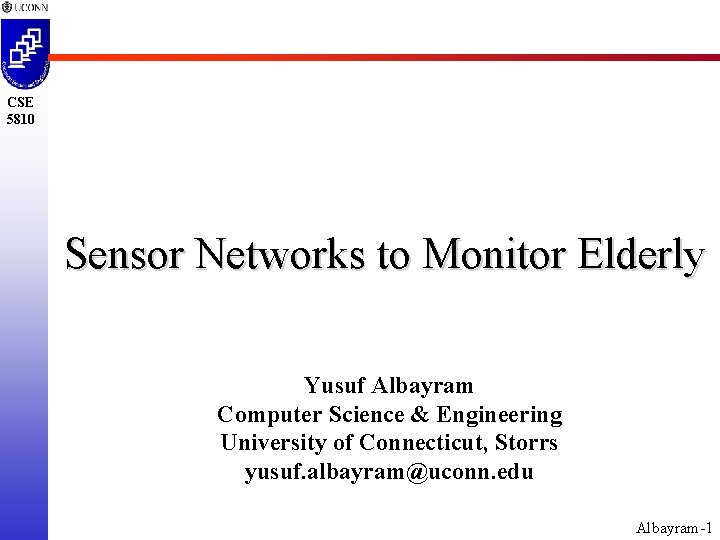
CSE 5810 Sensor Networks to Monitor Elderly Yusuf Albayram Computer Science & Engineering University of Connecticut, Storrs yusuf. albayram@uconn. edu Albayram-1
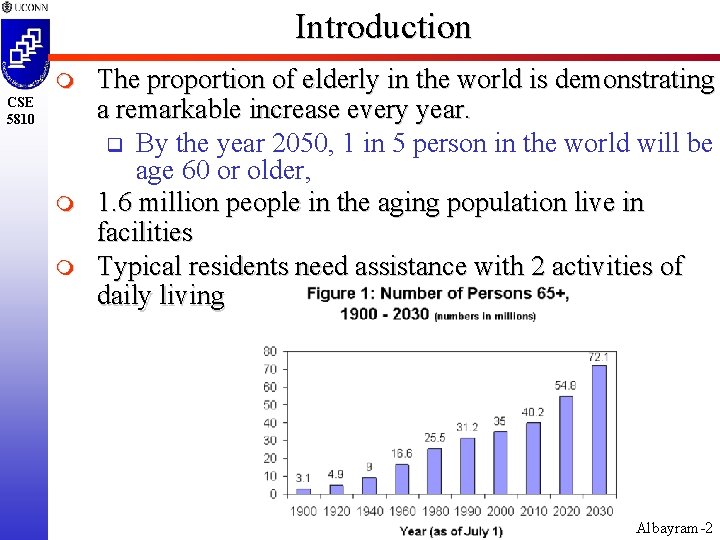
Introduction m CSE 5810 m m The proportion of elderly in the world is demonstrating a remarkable increase every year. q By the year 2050, 1 in 5 person in the world will be age 60 or older, 1. 6 million people in the aging population live in facilities Typical residents need assistance with 2 activities of daily living Albayram-2
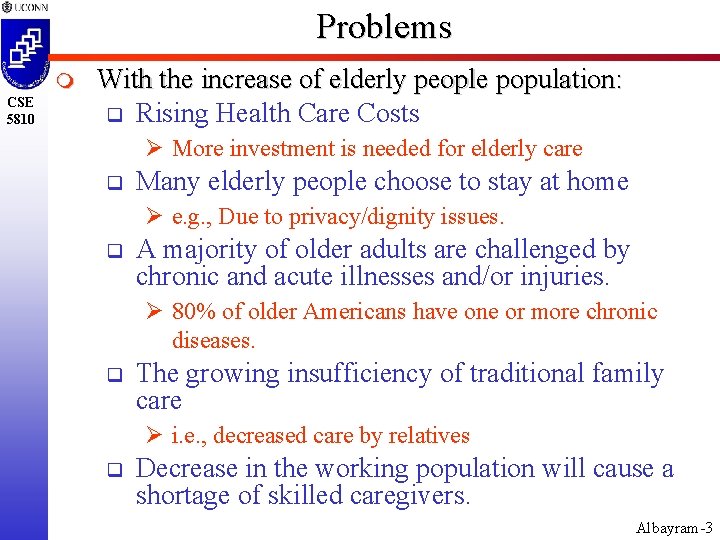
Problems m CSE 5810 With the increase of elderly people population: q Rising Health Care Costs Ø More investment is needed for elderly care q Many elderly people choose to stay at home Ø e. g. , Due to privacy/dignity issues. q A majority of older adults are challenged by chronic and acute illnesses and/or injuries. Ø 80% of older Americans have one or more chronic diseases. q The growing insufficiency of traditional family care Ø i. e. , decreased care by relatives q Decrease in the working population will cause a shortage of skilled caregivers. Albayram-3
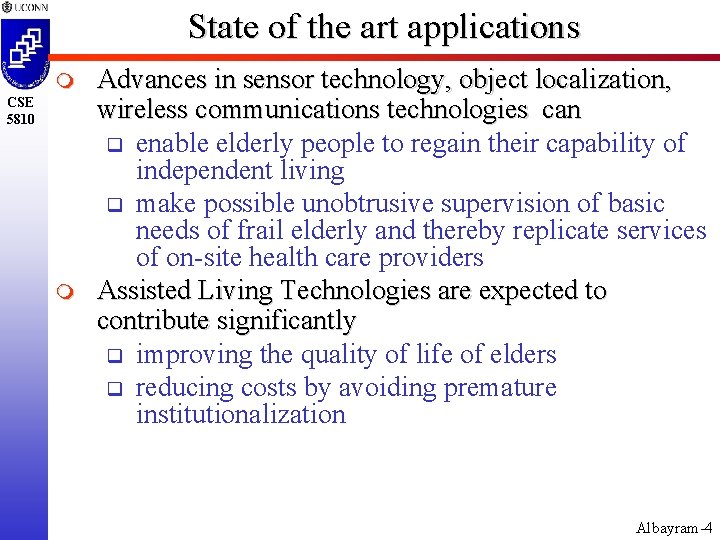
State of the art applications m CSE 5810 m Advances in sensor technology, object localization, wireless communications technologies can q enable elderly people to regain their capability of independent living q make possible unobtrusive supervision of basic needs of frail elderly and thereby replicate services of on-site health care providers Assisted Living Technologies are expected to contribute significantly q improving the quality of life of elders q reducing costs by avoiding premature institutionalization Albayram-4
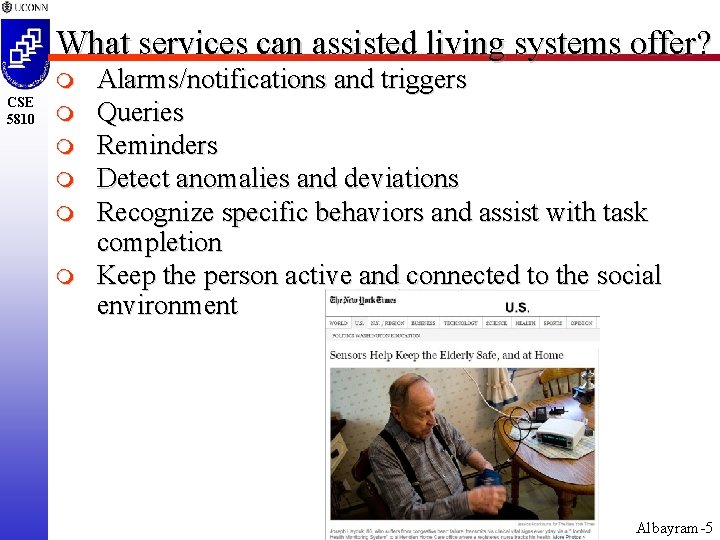
What services can assisted living systems offer? m CSE 5810 m m m Alarms/notifications and triggers Queries Reminders Detect anomalies and deviations Recognize specific behaviors and assist with task completion Keep the person active and connected to the social environment Albayram-5
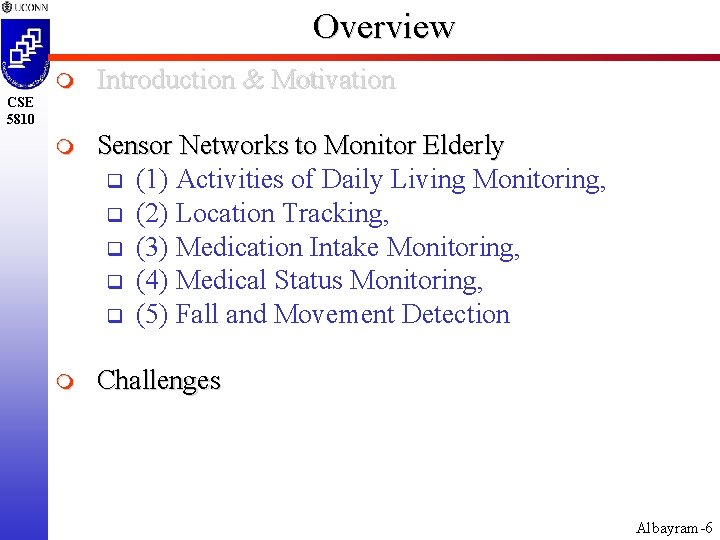
Overview m Introduction & Motivation m Sensor Networks to Monitor Elderly q (1) Activities of Daily Living Monitoring, q (2) Location Tracking, q (3) Medication Intake Monitoring, q (4) Medical Status Monitoring, q (5) Fall and Movement Detection m Challenges CSE 5810 Albayram-6
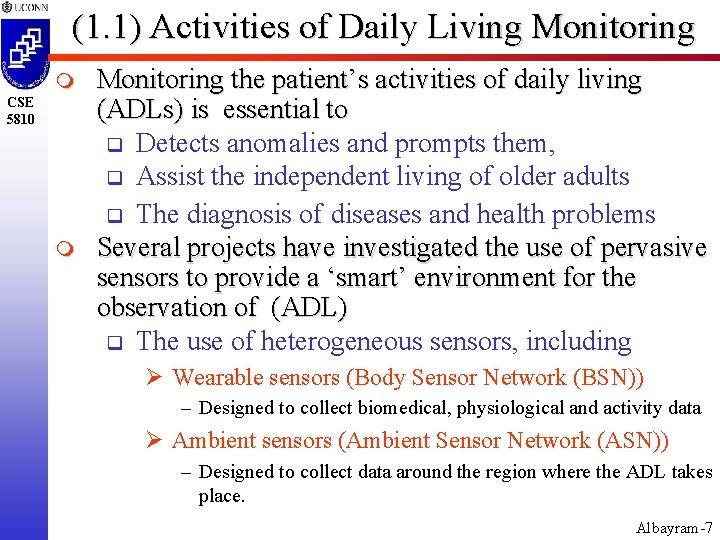
(1. 1) Activities of Daily Living Monitoring m CSE 5810 m Monitoring the patient’s activities of daily living (ADLs) is essential to q Detects anomalies and prompts them, q Assist the independent living of older adults q The diagnosis of diseases and health problems Several projects have investigated the use of pervasive sensors to provide a ‘smart’ environment for the observation of (ADL) q The use of heterogeneous sensors, including Ø Wearable sensors (Body Sensor Network (BSN)) – Designed to collect biomedical, physiological and activity data Ø Ambient sensors (Ambient Sensor Network (ASN)) – Designed to collect data around the region where the ADL takes place. Albayram-7
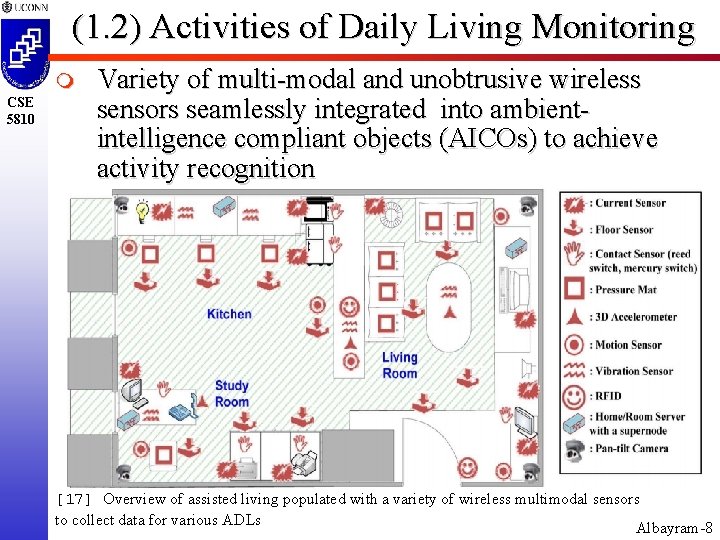
(1. 2) Activities of Daily Living Monitoring m CSE 5810 Variety of multi-modal and unobtrusive wireless sensors seamlessly integrated into ambientintelligence compliant objects (AICOs) to achieve activity recognition [17] Overview of assisted living populated with a variety of wireless multimodal sensors to collect data for various ADLs Albayram-8
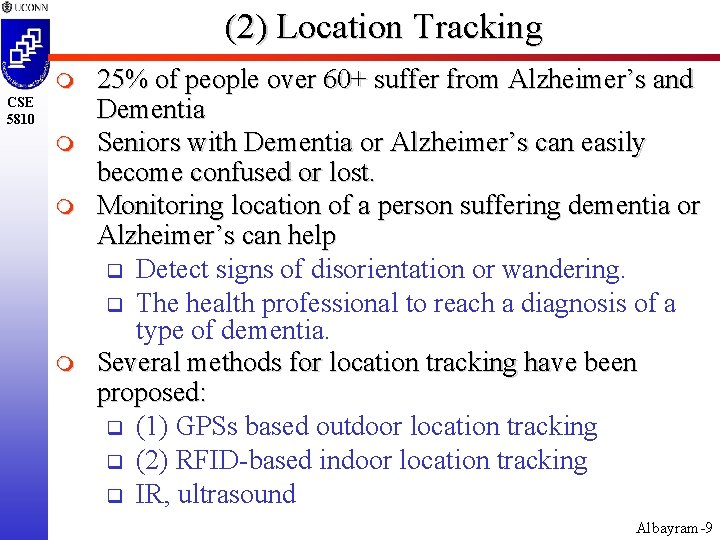
(2) Location Tracking m CSE 5810 m m m 25% of people over 60+ suffer from Alzheimer’s and Dementia Seniors with Dementia or Alzheimer’s can easily become confused or lost. Monitoring location of a person suffering dementia or Alzheimer’s can help q Detect signs of disorientation or wandering. q The health professional to reach a diagnosis of a type of dementia. Several methods for location tracking have been proposed: q (1) GPSs based outdoor location tracking q (2) RFID-based indoor location tracking q IR, ultrasound Albayram-9
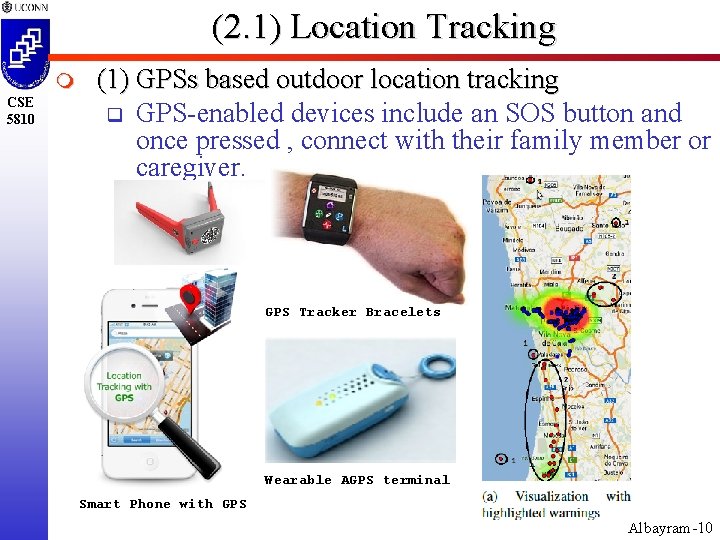
(2. 1) Location Tracking m CSE 5810 (1) GPSs based outdoor location tracking q GPS-enabled devices include an SOS button and once pressed , connect with their family member or caregiver. GPS Tracker Bracelets Wearable AGPS terminal Smart Phone with GPS Albayram-10
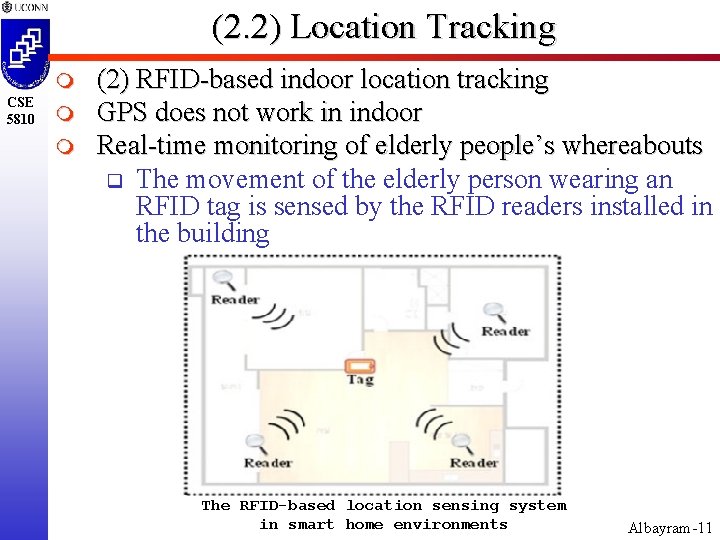
(2. 2) Location Tracking m CSE 5810 m m (2) RFID-based indoor location tracking GPS does not work in indoor Real-time monitoring of elderly people’s whereabouts q The movement of the elderly person wearing an RFID tag is sensed by the RFID readers installed in the building The RFID-based location sensing system in smart home environments Albayram-11
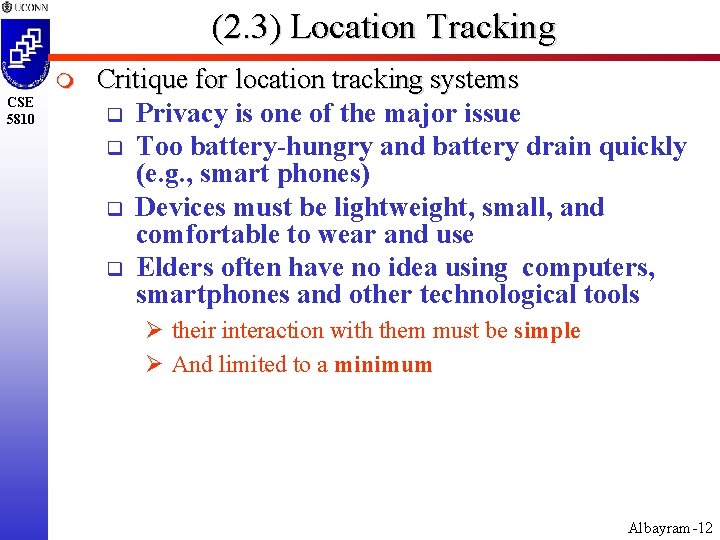
(2. 3) Location Tracking m CSE 5810 Critique for location tracking systems q Privacy is one of the major issue q Too battery-hungry and battery drain quickly (e. g. , smart phones) q Devices must be lightweight, small, and comfortable to wear and use q Elders often have no idea using computers, smartphones and other technological tools Ø their interaction with them must be simple Ø And limited to a minimum Albayram-12
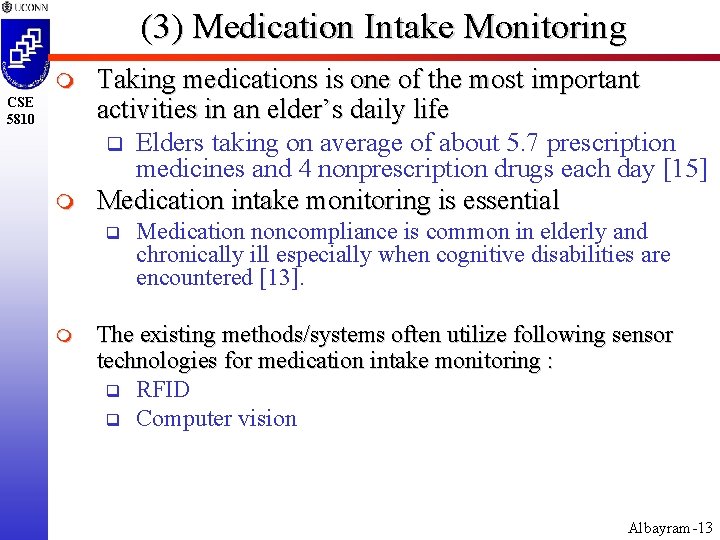
(3) Medication Intake Monitoring m CSE 5810 m Taking medications is one of the most important activities in an elder’s daily life q Elders taking on average of about 5. 7 prescription medicines and 4 nonprescription drugs each day [15] Medication intake monitoring is essential q m Medication noncompliance is common in elderly and chronically ill especially when cognitive disabilities are encountered [13]. The existing methods/systems often utilize following sensor technologies for medication intake monitoring : q RFID q Computer vision Albayram-13
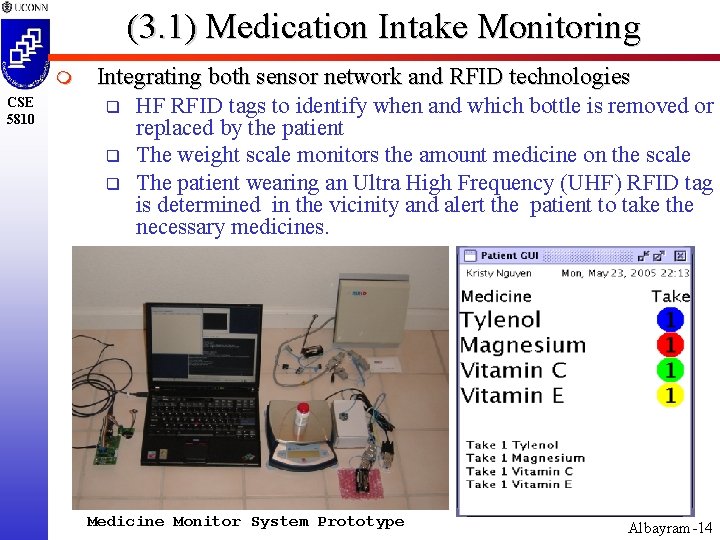
(3. 1) Medication Intake Monitoring m CSE 5810 Integrating both sensor network and RFID technologies q HF RFID tags to identify when and which bottle is removed or replaced by the patient q The weight scale monitors the amount medicine on the scale q The patient wearing an Ultra High Frequency (UHF) RFID tag is determined in the vicinity and alert the patient to take the necessary medicines. Medicine Monitor System Prototype Albayram-14
![3 2 Medication Intake Monitoring m CSE 5810 Incorporating RFID and video analysis 10 (3. 2) Medication Intake Monitoring m CSE 5810 Incorporating RFID and video analysis [10]](https://slidetodoc.com/presentation_image_h/c6bd3d6d3d9a97aeeb408f0389155153/image-15.jpg)
(3. 2) Medication Intake Monitoring m CSE 5810 Incorporating RFID and video analysis [10] q RFID tags applied on medicine bottles located in a medicine cabinet and RFID readers detect if any of these bottles are taken away q A video camera monitoring the activity of taking medicine by integrating face and mouth detection Monitoring the activity of taking medications using computer vision-based method RFID system includes antenna and RFID reader Albayram-15
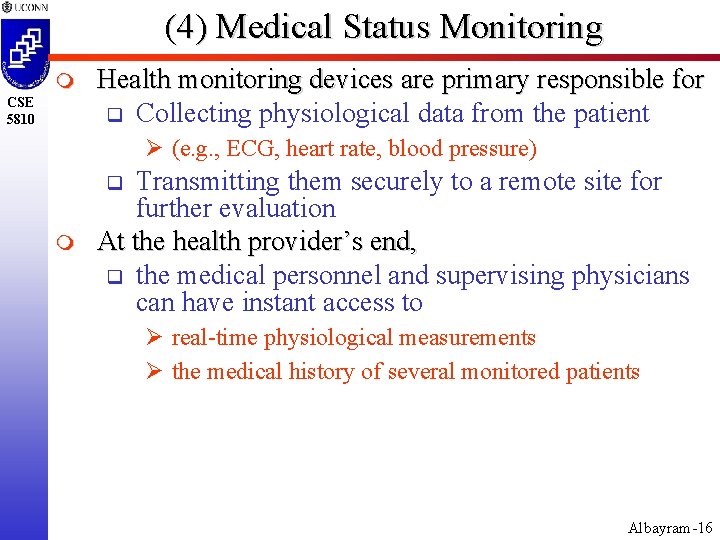
(4) Medical Status Monitoring m CSE 5810 Health monitoring devices are primary responsible for q Collecting physiological data from the patient Ø (e. g. , ECG, heart rate, blood pressure) Transmitting them securely to a remote site for further evaluation At the health provider’s end, q the medical personnel and supervising physicians can have instant access to q m Ø real-time physiological measurements Ø the medical history of several monitored patients Albayram-16
![4 1 Medical Status Monitoring CSE 5810 The health monitoring network structure 16 Albayram17 (4. 1) Medical Status Monitoring CSE 5810 The health monitoring network structure [16] Albayram-17](https://slidetodoc.com/presentation_image_h/c6bd3d6d3d9a97aeeb408f0389155153/image-17.jpg)
(4. 1) Medical Status Monitoring CSE 5810 The health monitoring network structure [16] Albayram-17
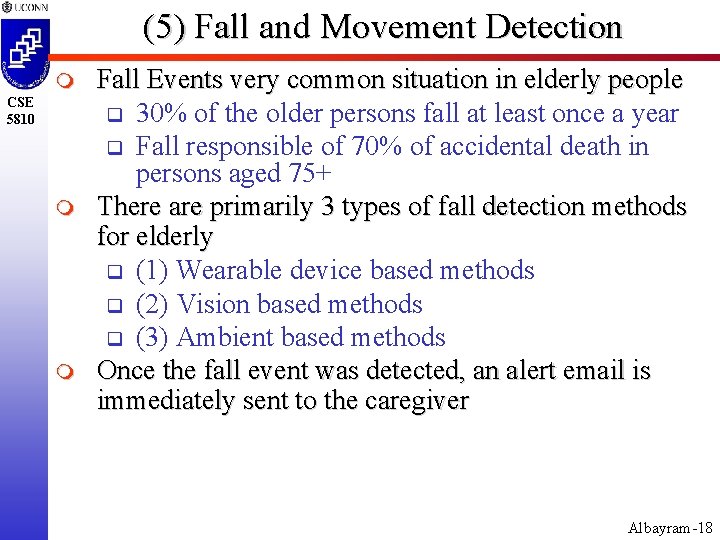
(5) Fall and Movement Detection m CSE 5810 m m Fall Events very common situation in elderly people q 30% of the older persons fall at least once a year q Fall responsible of 70% of accidental death in persons aged 75+ There are primarily 3 types of fall detection methods for elderly q (1) Wearable device based methods q (2) Vision based methods q (3) Ambient based methods Once the fall event was detected, an alert email is immediately sent to the caregiver Albayram-18
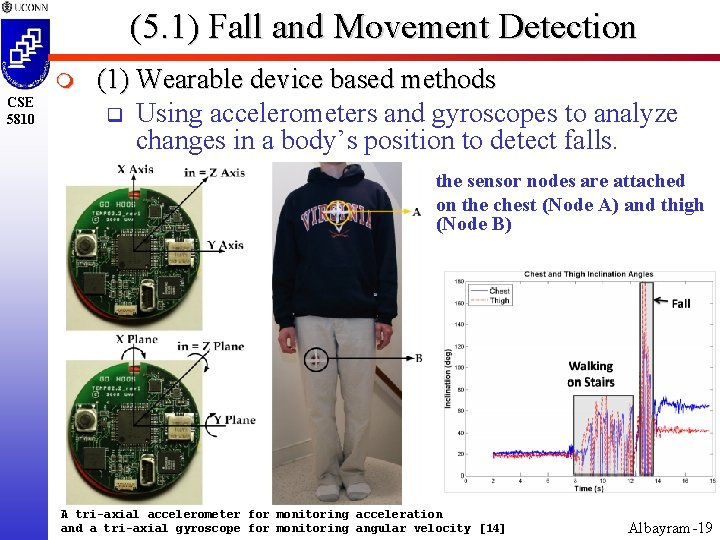
(5. 1) Fall and Movement Detection m CSE 5810 (1) Wearable device based methods q Using accelerometers and gyroscopes to analyze changes in a body’s position to detect falls. the sensor nodes are attached on the chest (Node A) and thigh (Node B) A tri-axial accelerometer for monitoring acceleration and a tri-axial gyroscope for monitoring angular velocity [14] Albayram-19
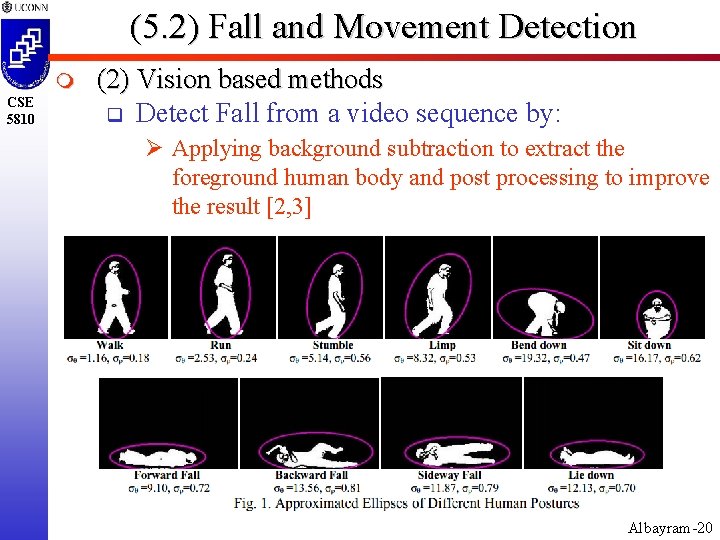
(5. 2) Fall and Movement Detection m CSE 5810 (2) Vision based methods q Detect Fall from a video sequence by: Ø Applying background subtraction to extract the foreground human body and post processing to improve the result [2, 3] Albayram-20
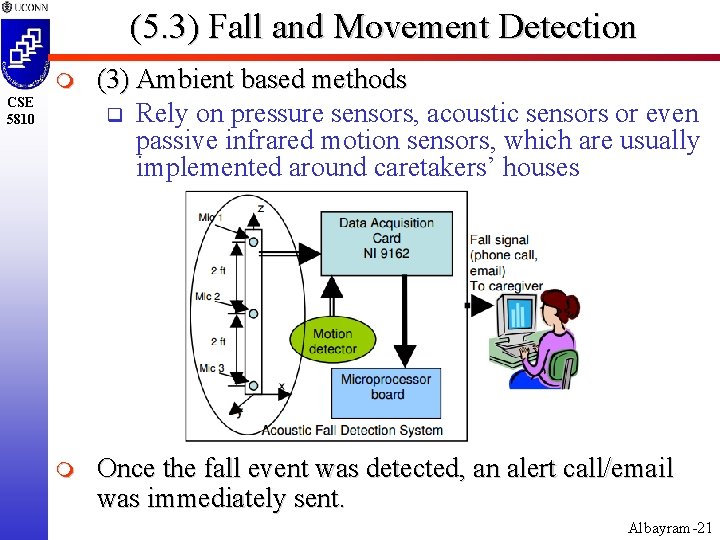
(5. 3) Fall and Movement Detection m (3) Ambient based methods q Rely on pressure sensors, acoustic sensors or even passive infrared motion sensors, which are usually implemented around caretakers’ houses m Once the fall event was detected, an alert call/email was immediately sent. CSE 5810 Albayram-21
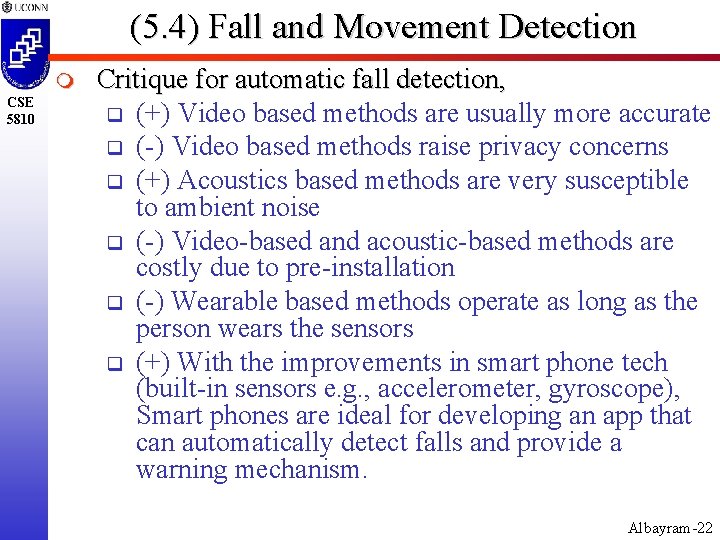
(5. 4) Fall and Movement Detection m CSE 5810 Critique for automatic fall detection, q (+) Video based methods are usually more accurate q (-) Video based methods raise privacy concerns q (+) Acoustics based methods are very susceptible to ambient noise q (-) Video-based and acoustic-based methods are costly due to pre-installation q (-) Wearable based methods operate as long as the person wears the sensors q (+) With the improvements in smart phone tech (built-in sensors e. g. , accelerometer, gyroscope), Smart phones are ideal for developing an app that can automatically detect falls and provide a warning mechanism. Albayram-22
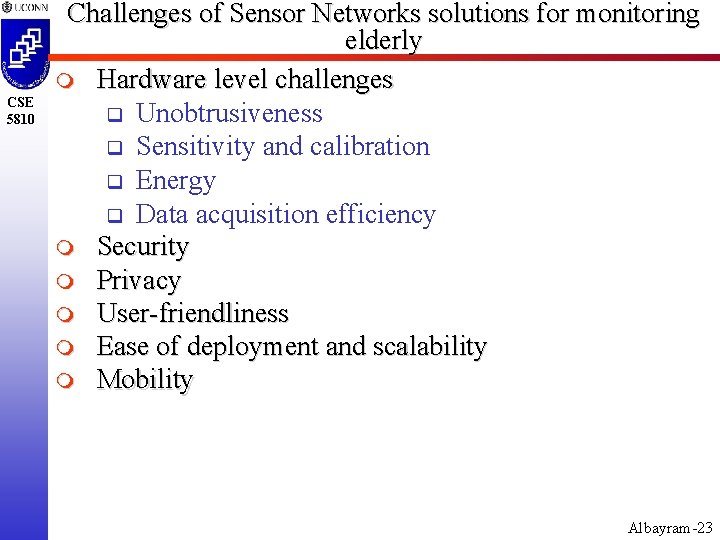
CSE 5810 Challenges of Sensor Networks solutions for monitoring elderly m Hardware level challenges q Unobtrusiveness q Sensitivity and calibration q Energy q Data acquisition efficiency m Security m Privacy m User-friendliness m Ease of deployment and scalability m Mobility Albayram-23
![References CSE 5810 1 Wang J et al An enhanced fall detection system References CSE 5810 [1] Wang, J. , et al. "An enhanced fall detection system](https://slidetodoc.com/presentation_image_h/c6bd3d6d3d9a97aeeb408f0389155153/image-24.jpg)
References CSE 5810 [1] Wang, J. , et al. "An enhanced fall detection system for elderly person monitoring using consumer home networks. " Consumer Electronics, IEEE Transactions on 60. 1 (2014): 23 -29. [2] Yu, Miao, et al. "A posture recognition-based fall detection system for monitoring an elderly person in a smart home environment. " Information Technology in Biomedicine, IEEE Transactions on 16. 6 (2012): 1274 -1286. [3] Foroughi, Homa, Baharak Shakeri Aski, and Hamidreza Pourreza. "Intelligent video surveillance for monitoring fall detection of elderly in home environments. " Computer and Information Technology, 2008. ICCIT 2008. 11 th International Conference on. IEEE, 2008. [4] Yavuz, Gokhan, et al. "A smartphone based fall detector with online location support. " International Workshop on Sensing for App Phones; Zurich, Switzerland. 2010. [5] Popescu, Mihail, et al. "An acoustic fall detector system that uses sound height information to reduce the false alarm rate. " Engineering in Medicine and Biology Society, 2008. EMBS 2008. 30 th Annual International Conference of the IEEE, 2008. [6] Huang, Kung-Ta, et al. "An intelligent RFID system for improving elderly daily life independent in indoor environment. " Smart homes and health telematics. Springer Berlin Heidelberg, 2008. 1 -8. [7] Ferreira, João. "Behavioral Analytics for Medical Decision Support: Supporting dementia diagnosis through outlier detection. " (2012). [8] Wong, AK-S. , et al. "An AGPS-based elderly tracking system. " Ubiquitous and Future Networks, 2009. ICUFN 2009. First International Conference on. IEEE, 2009. [9] Kim, Soo-Cheol, Young-Sik Jeong, and Sang-Oh Park. "RFID-based indoor location tracking to ensure the safety of the elderly in smart home environments. " Personal and ubiquitous computing 17. 8 (2013): 1699 -1707. [10] Hasanuzzaman, Faiz M. , et al. "Monitoring activity of taking medicine by incorporating RFID and video analysis. " Network Modeling Analysis in Health Informatics and Bioinformatics 2. 2 (2013): 61 -70. Albayram-24
![References2 CSE 5810 11 Pang Zhibo Qiang Chen and Lirong Zheng A pervasive and References-2 CSE 5810 [11] Pang, Zhibo, Qiang Chen, and Lirong Zheng. "A pervasive and](https://slidetodoc.com/presentation_image_h/c6bd3d6d3d9a97aeeb408f0389155153/image-25.jpg)
References-2 CSE 5810 [11] Pang, Zhibo, Qiang Chen, and Lirong Zheng. "A pervasive and preventive healthcare solution for medication noncompliance and daily monitoring. " Applied Sciences in Biomedical and Communication Technologies, 2009. ISABEL 2009. 2 nd International Symposium on. IEEE, 2009. [12] Ho, Loc, et al. "A prototype on RFID and sensor networks for elder healthcare: progress report. " Proceedings of the 2005 ACM SIGCOMM workshop on Experimental approaches to wireless network design and analysis. ACM, 2005. [13] Alemdar, Hande, and Cem Ersoy. "Wireless sensor networks for healthcare: A survey. " Computer Networks 54. 15 (2010): 2688 -2710. [14] Li, Qiang, et al. "Accurate, fast fall detection using gyroscopes and accelerometer-derived posture information. " Wearable and Implantable Body Sensor Networks, 2009. BSN 2009. Sixth International Workshop on. IEEE, 2009. [15 ] Johnston C (2001) Falls in the Elderly, UCSF Division of Geriatrics Primary Care Lecture Series. http: //s 3. amazonaws. com/engrademyfiles/4063195431780411/sf_falls. ppt [16] Pantelopoulos, Alexandros, and Nikolaos G. Bourbakis. "Prognosis—a wearable healthmonitoring system for people at risk: Methodology and modeling. " Information Technology in Biomedicine, IEEE Transactions on 14. 3 (2010): 613 -621. [17] Lu, Ching-Hu, and Li-Chen Fu. "Robust location-aware activity recognition using wireless sensor network in an attentive home. " Automation Science and Engineering, IEEE Transactions on 6. 4 (2009): 598 -609. Albayram-25
Hodan milano
Army skl tm
Bluetooth based smart sensor networks
Bluetooth based smart sensor networks
Habitat monitoring sensor
Single node architecture in wireless sensor networks
Wireless sensor networks for habitat monitoring
Basestore iptv
Virtual circuit network uses
Chapter 10:4 confusion and disorientation in the elderly
Rotterdam elderly pain observation scale
Elderly mobility scale
Low sodium levels in the elderly
Clay county transit for elderly
"aspirin" elderly
Milwaukee elderly neglect
Characht
Bp goal for elderly
Nphce logo
Psychosocial needs of the elderly
Objectives of elderly care
Common psychosocial care problems of the elderly
Chapter 10:5 meeting the needs of the elderly
Non verbal communication with the elderly
Colon cancer kras wild type
Dependency ratio definition geography