Ch 9 Communities Community Characteristics Many Nutrient Cycles
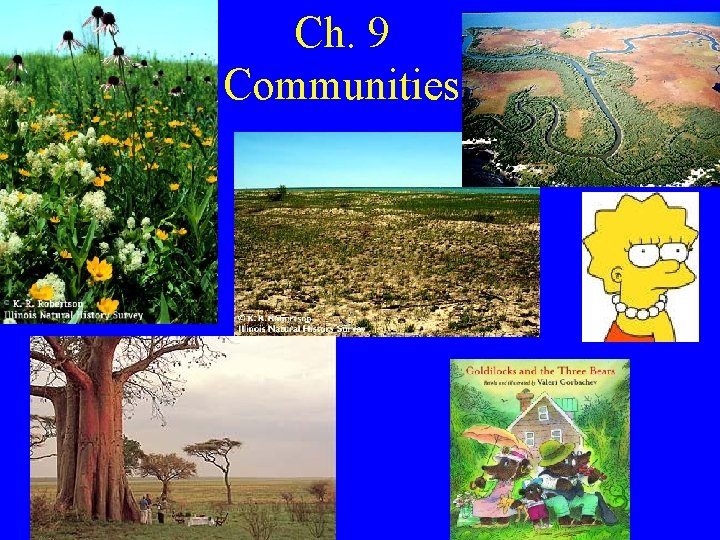
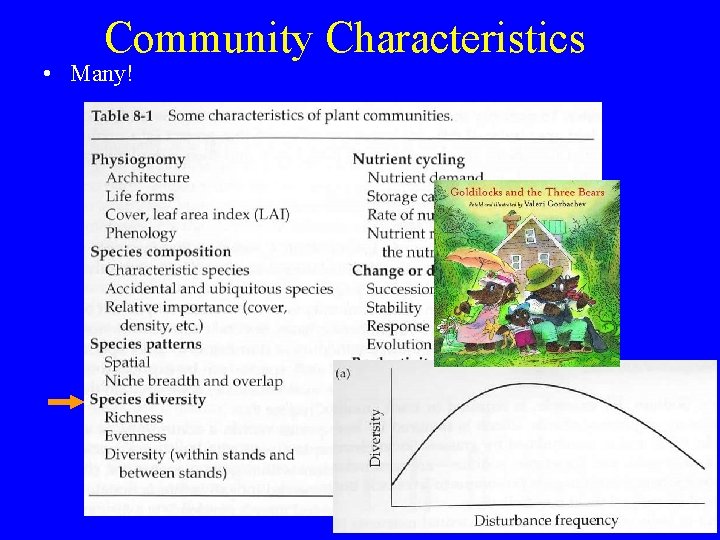
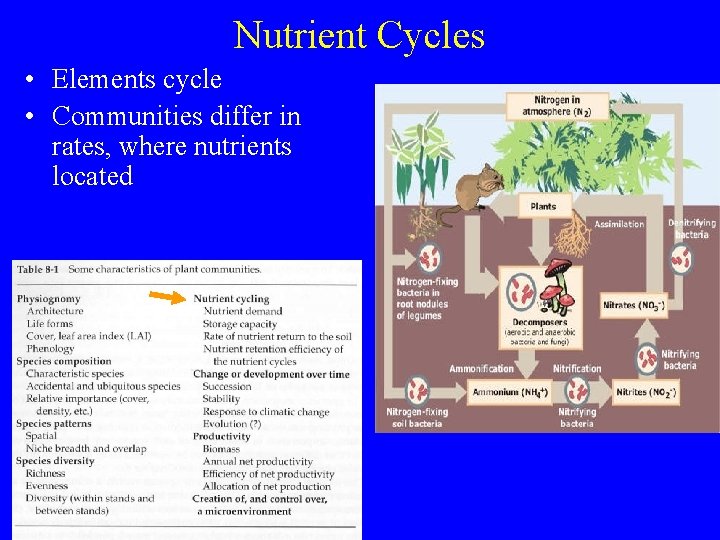
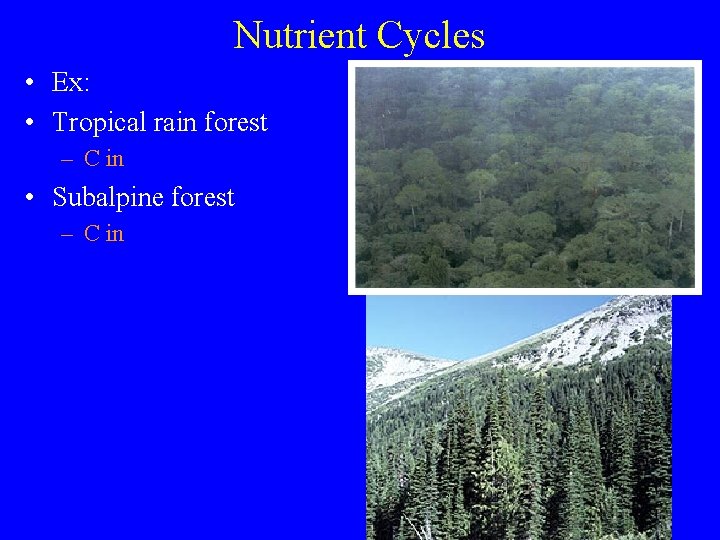
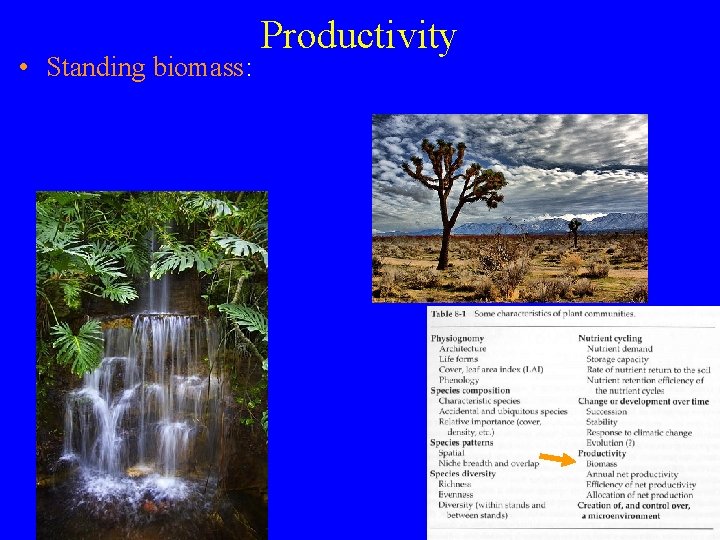
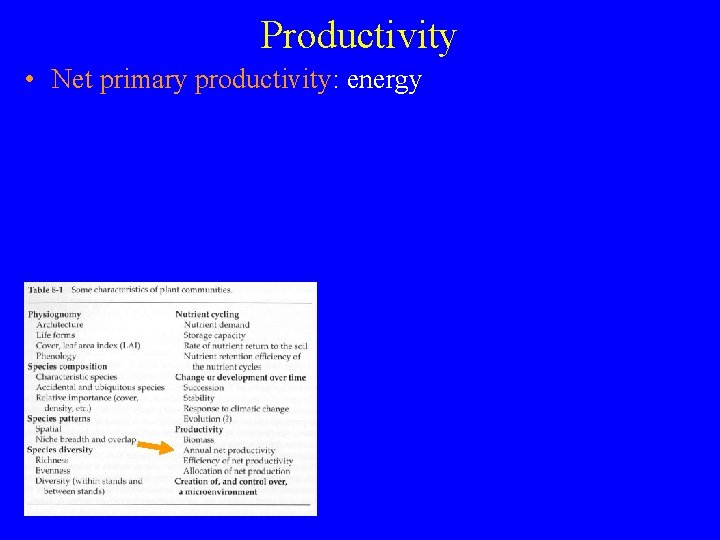
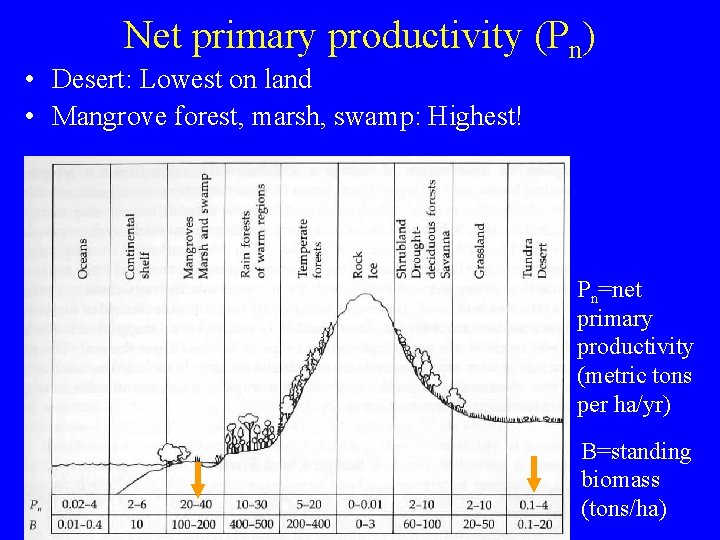
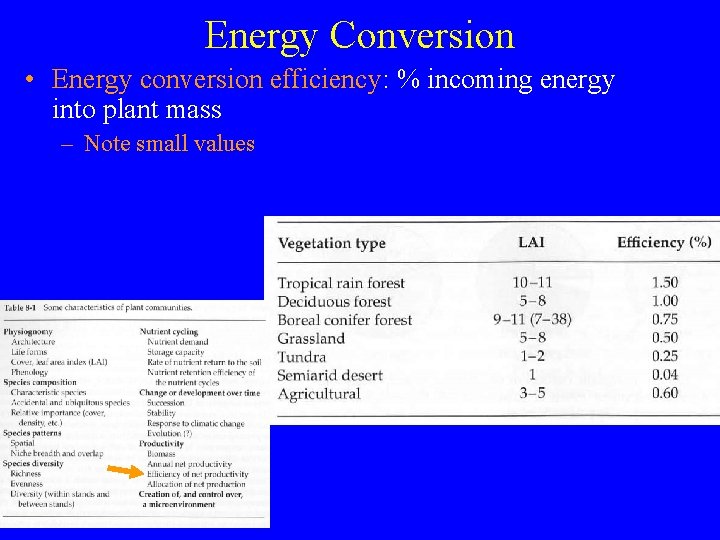
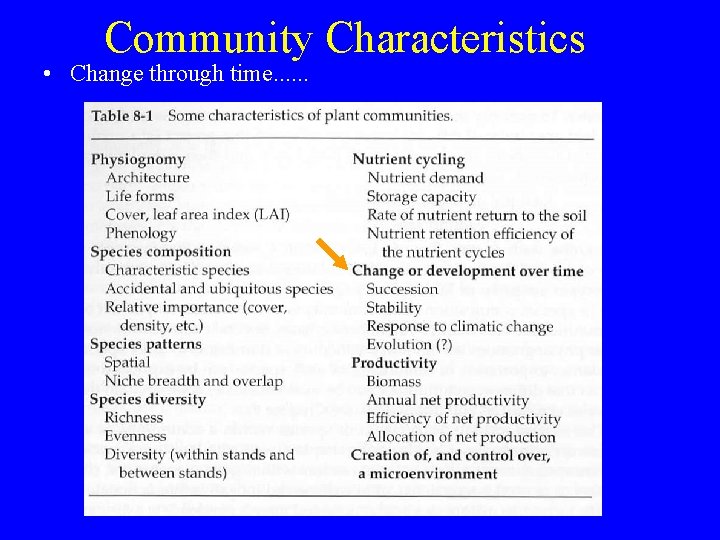
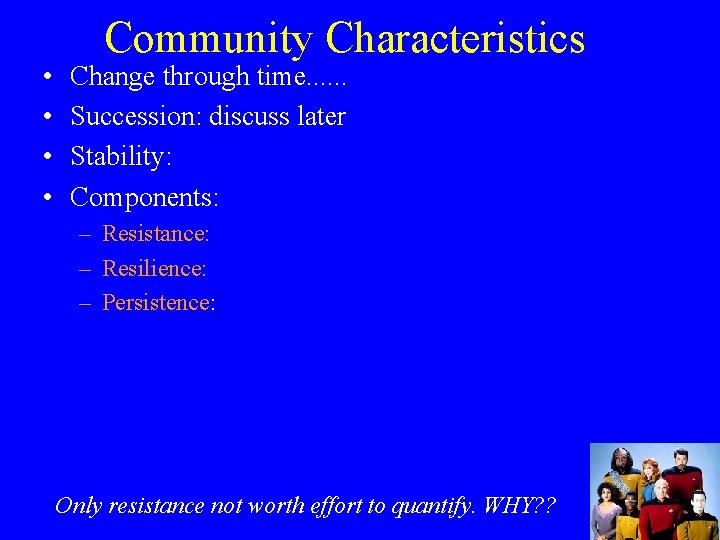
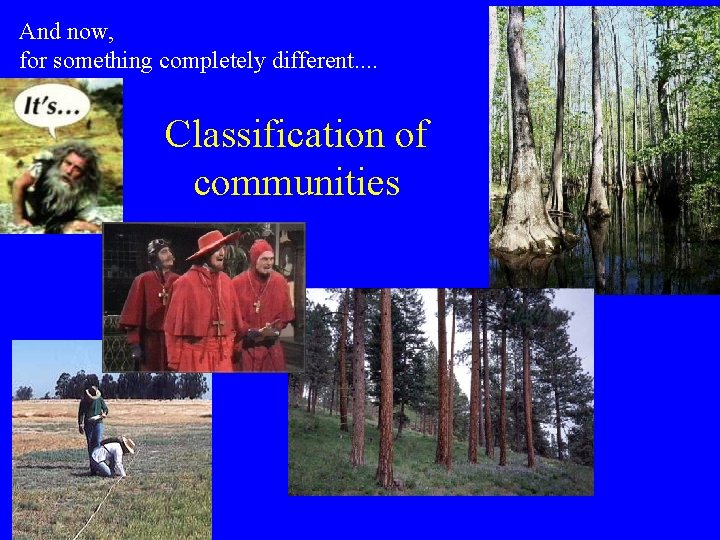
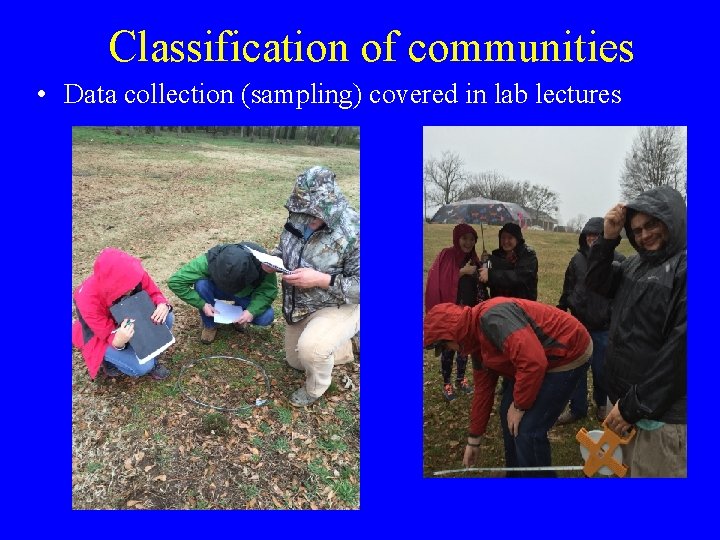
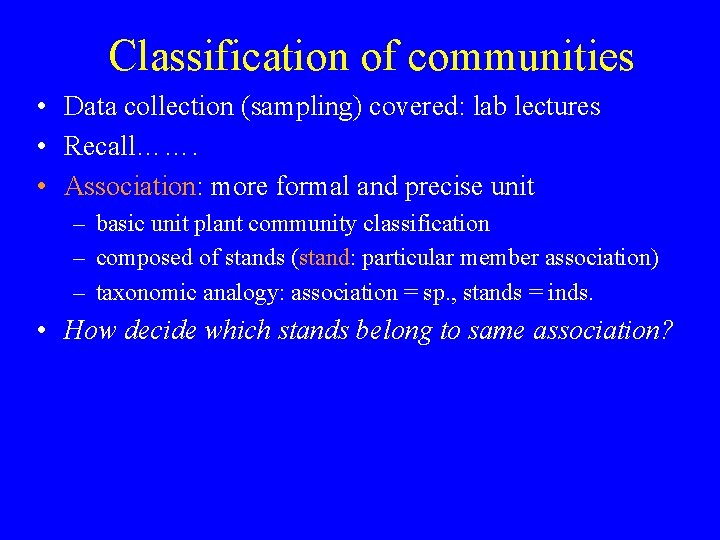
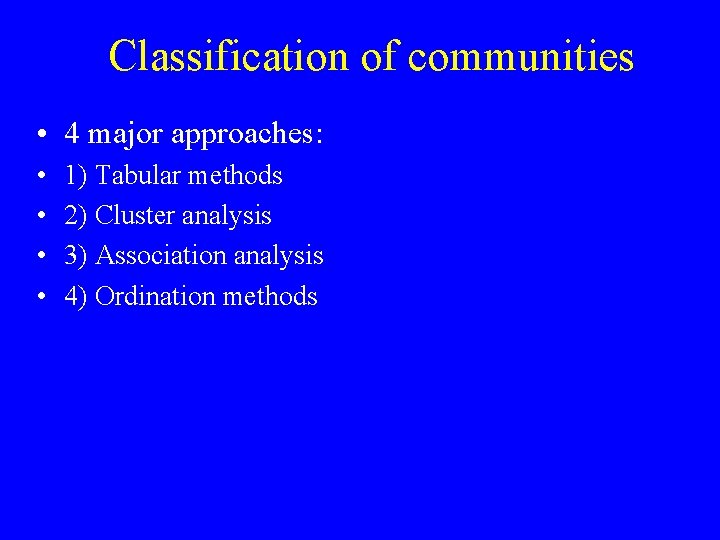
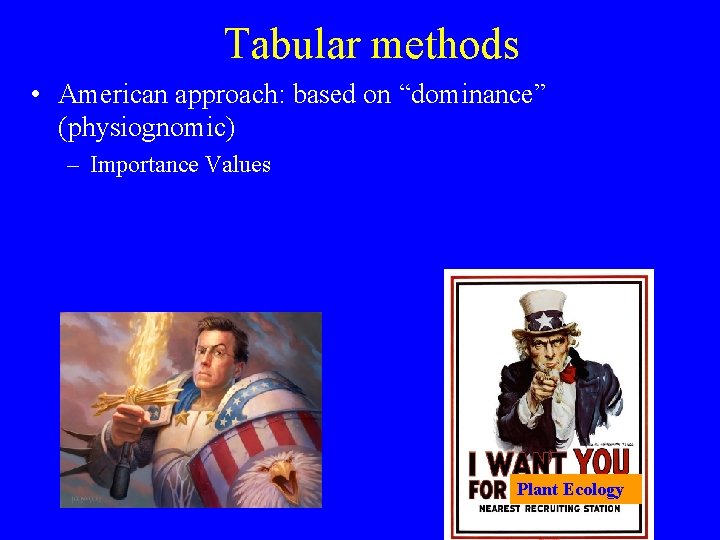
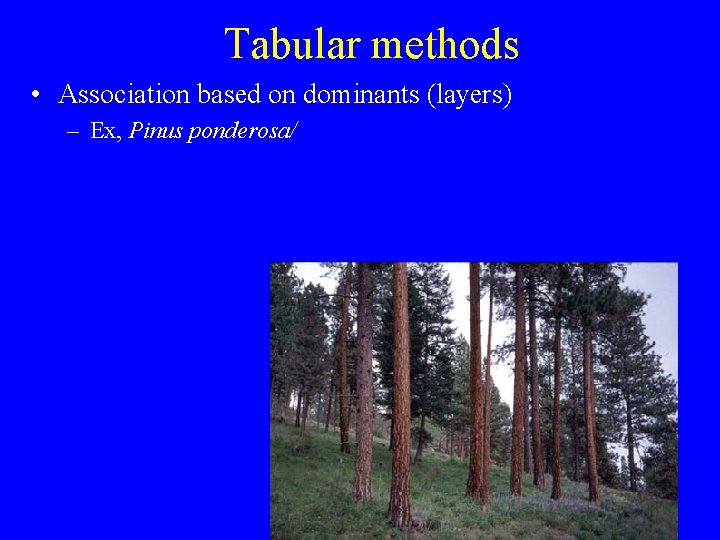
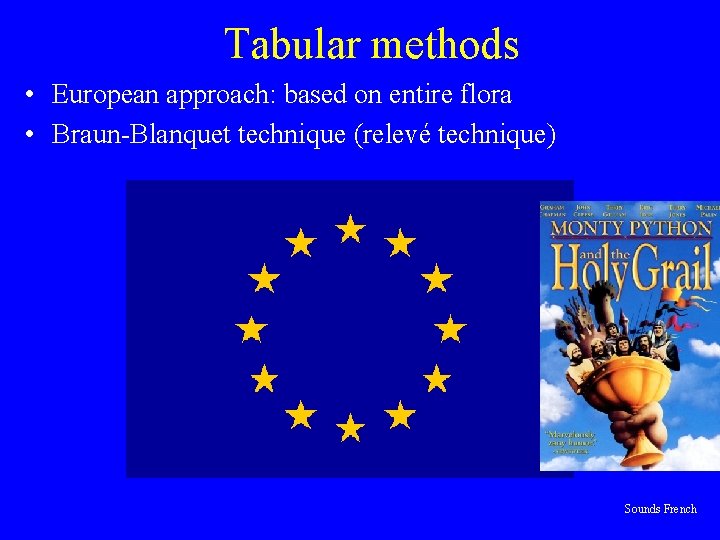
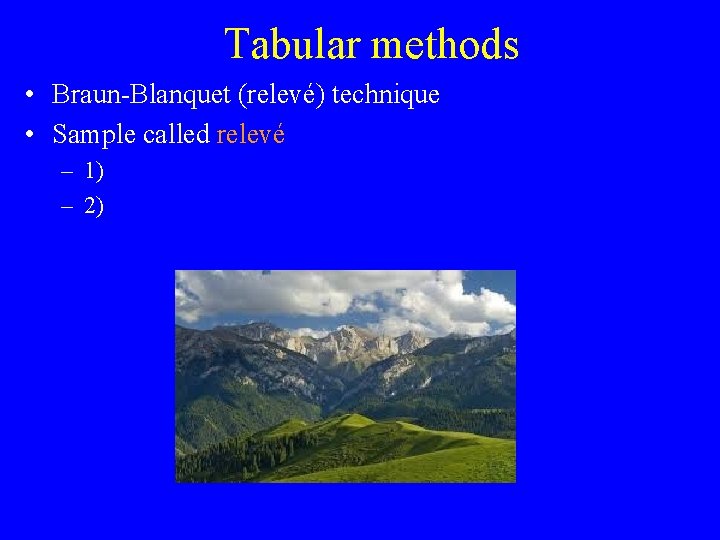
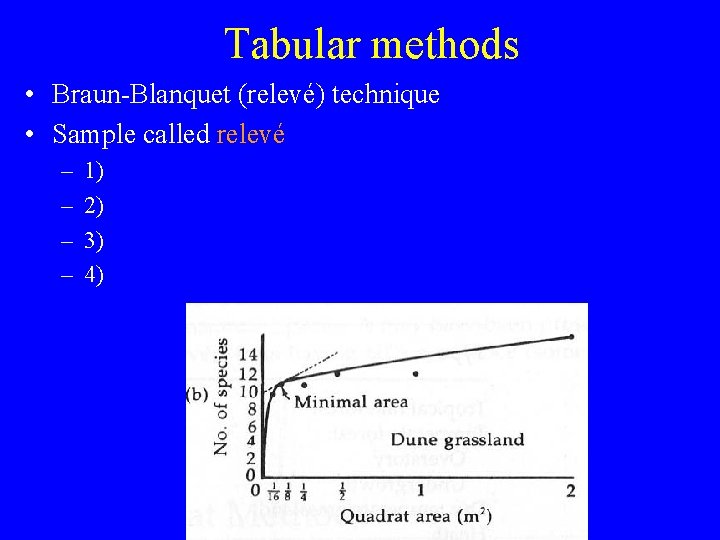
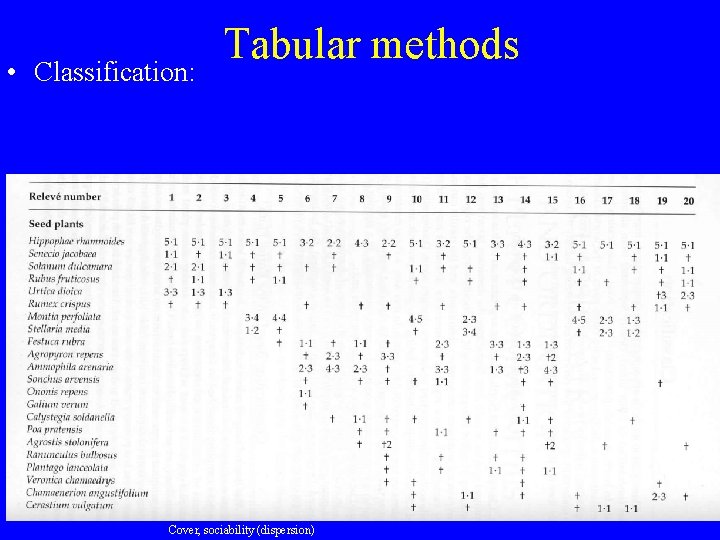
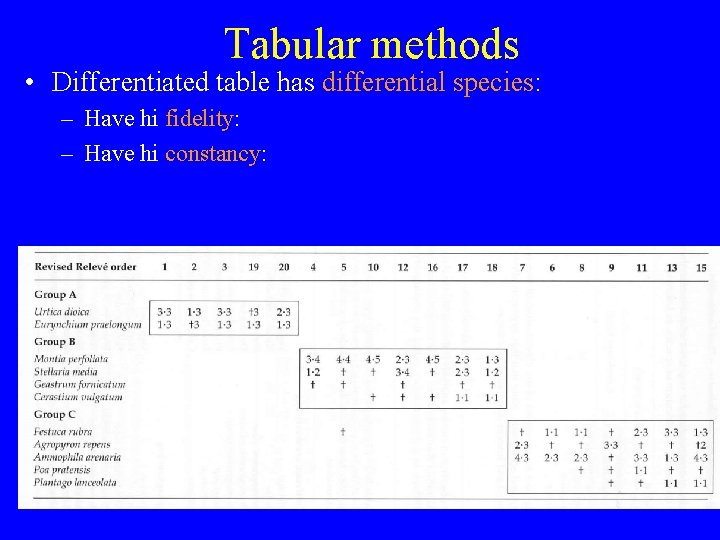
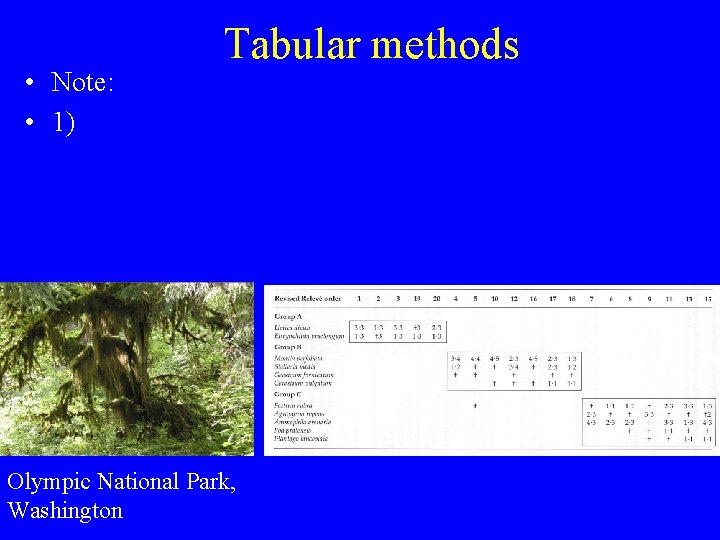
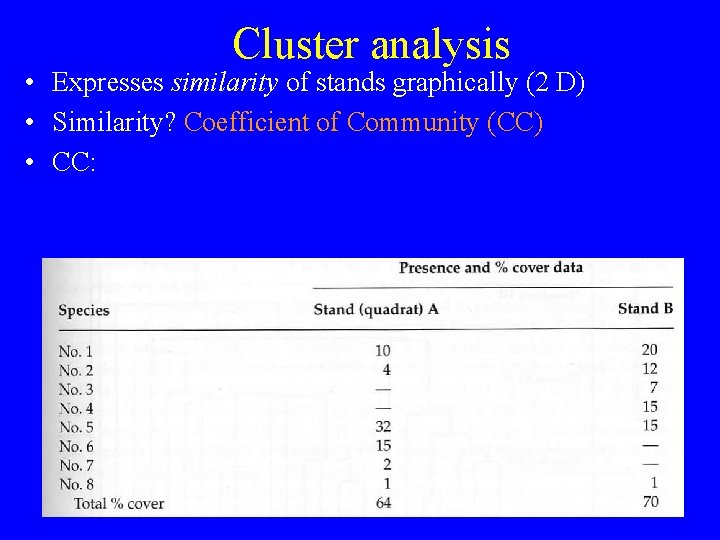
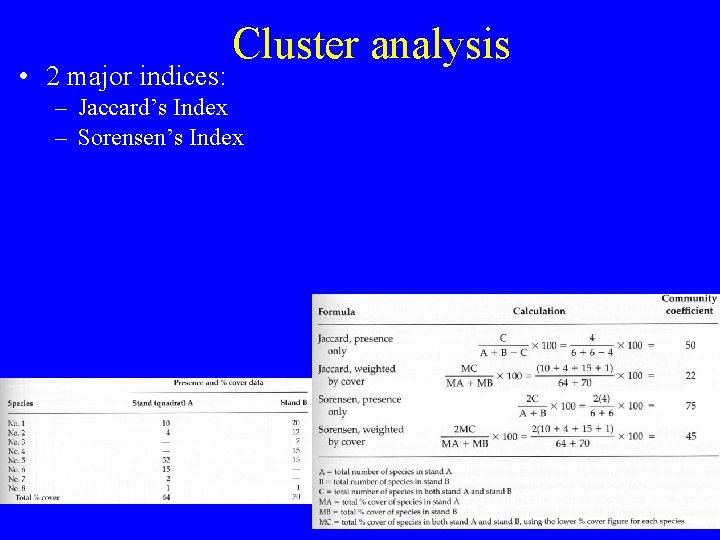
- Slides: 24
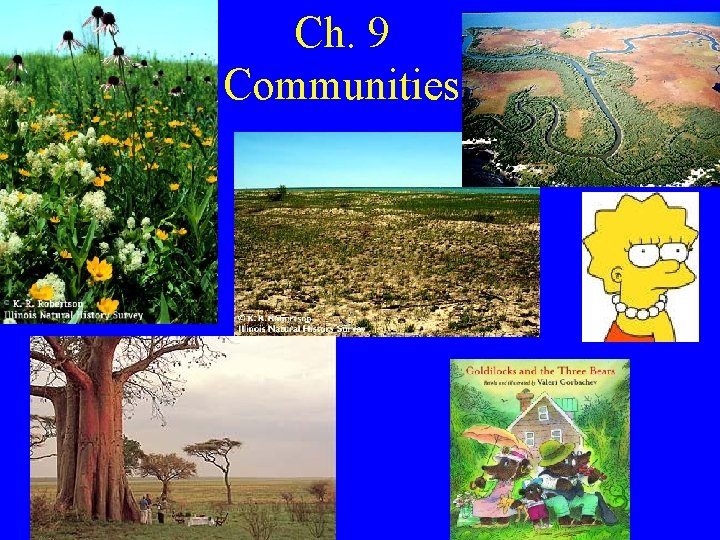
Ch. 9 Communities
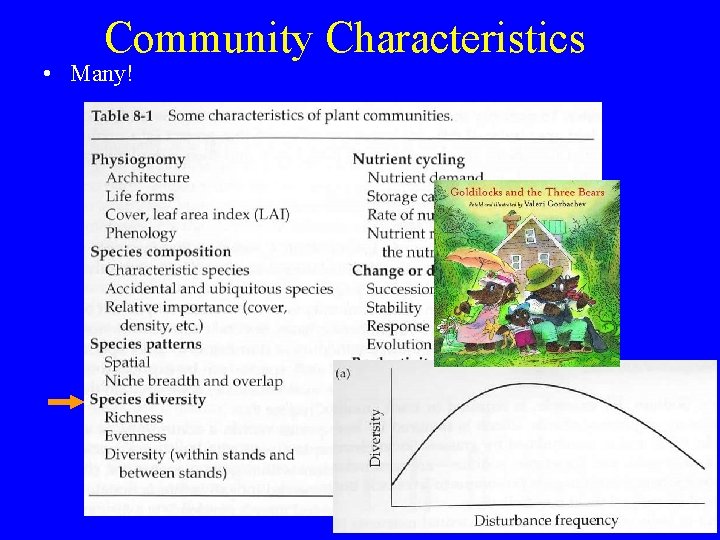
Community Characteristics • Many!
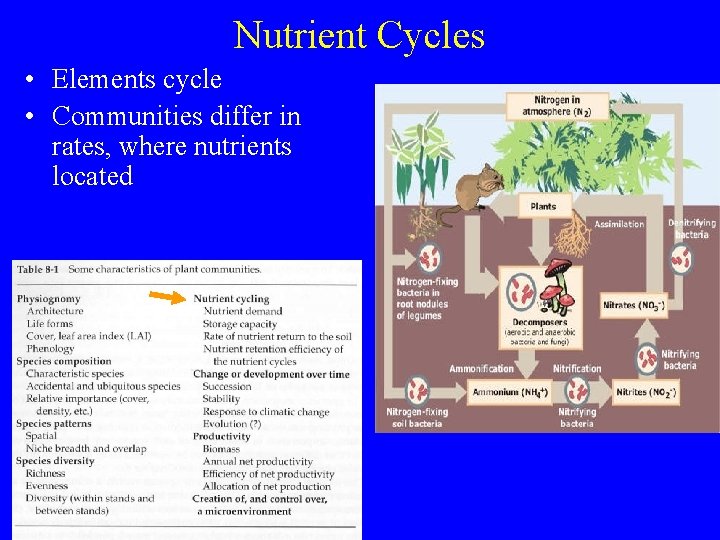
Nutrient Cycles • Elements cycle • Communities differ in rates, where nutrients located
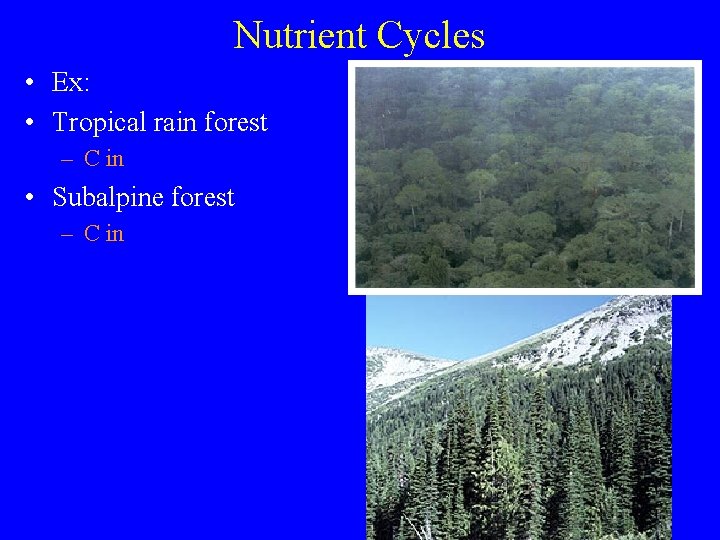
Nutrient Cycles • Ex: • Tropical rain forest – C in • Subalpine forest – C in
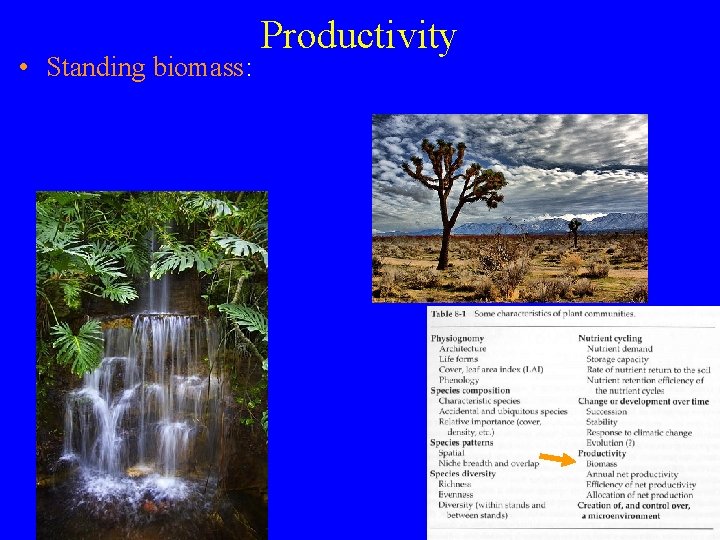
• Standing biomass: Productivity
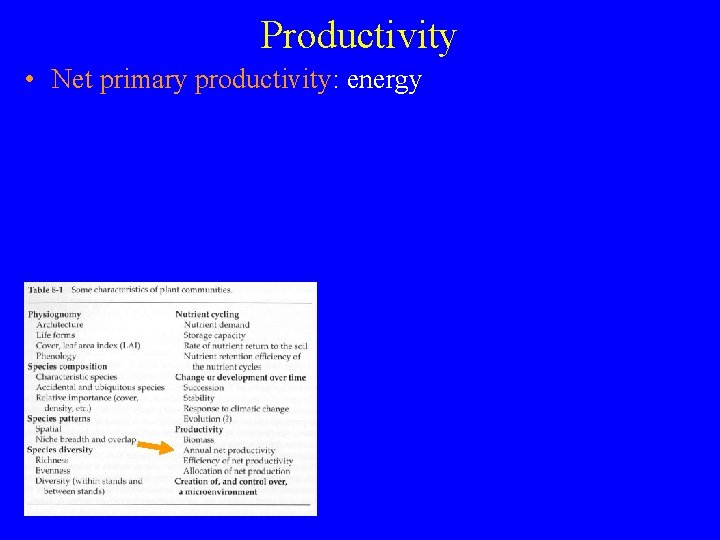
Productivity • Net primary productivity: energy
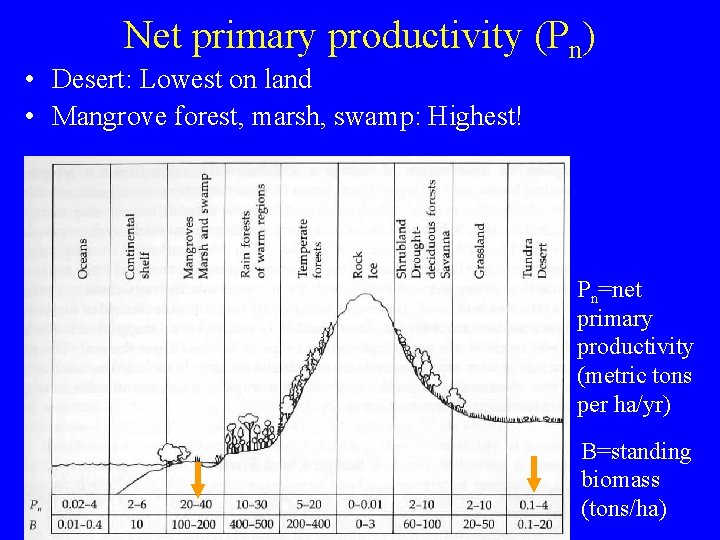
Net primary productivity (Pn) • Desert: Lowest on land • Mangrove forest, marsh, swamp: Highest! Pn=net primary productivity (metric tons per ha/yr) B=standing biomass (tons/ha)
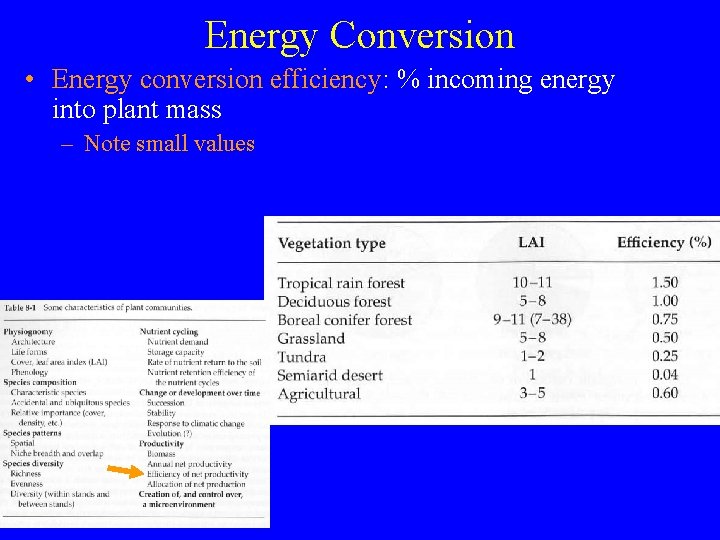
Energy Conversion • Energy conversion efficiency: % incoming energy into plant mass – Note small values
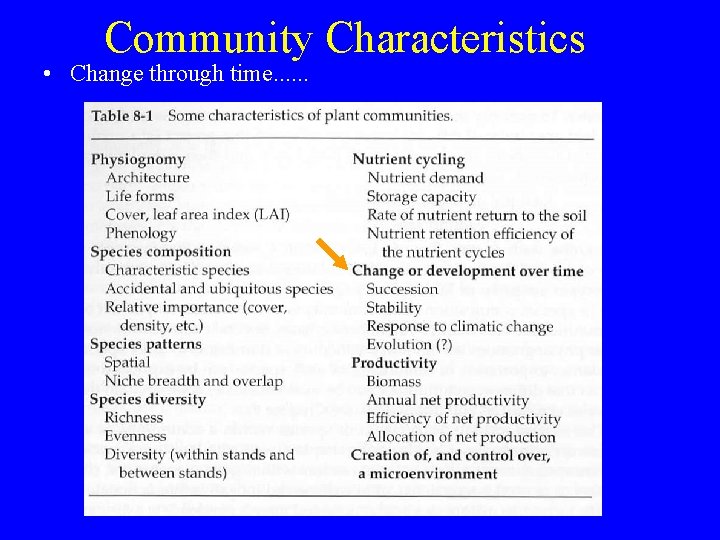
Community Characteristics • Change through time. . .
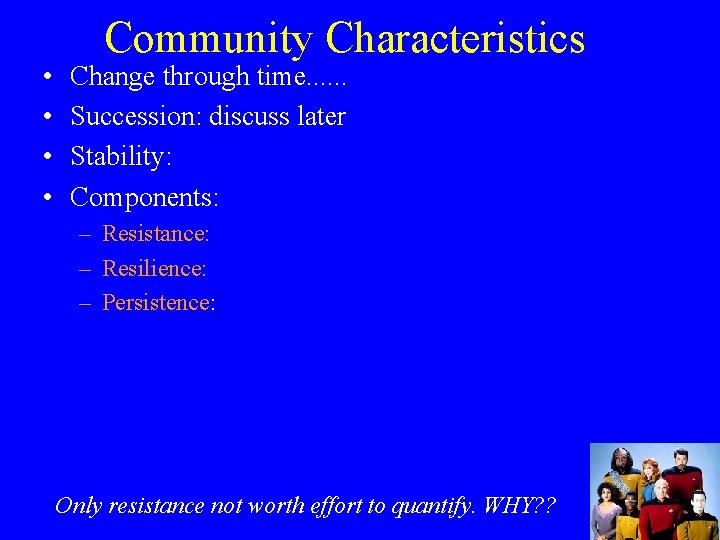
• • Community Characteristics Change through time. . . Succession: discuss later Stability: Components: – Resistance: – Resilience: – Persistence: Only resistance not worth effort to quantify. WHY? ?
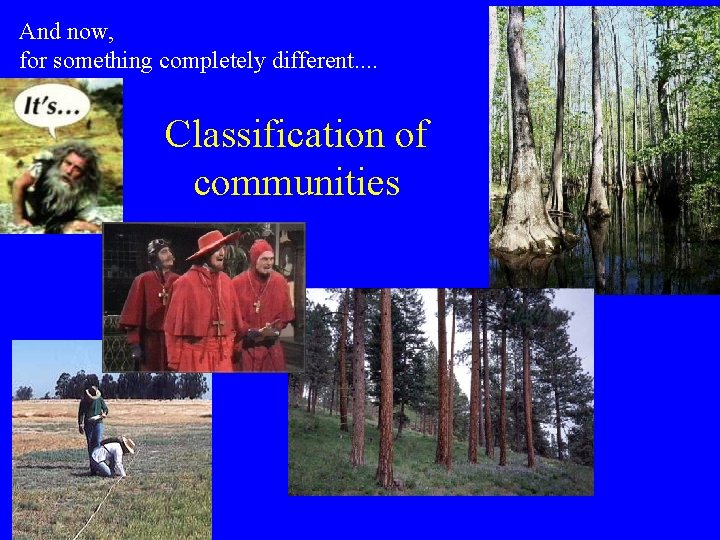
And now, for something completely different. . Classification of communities
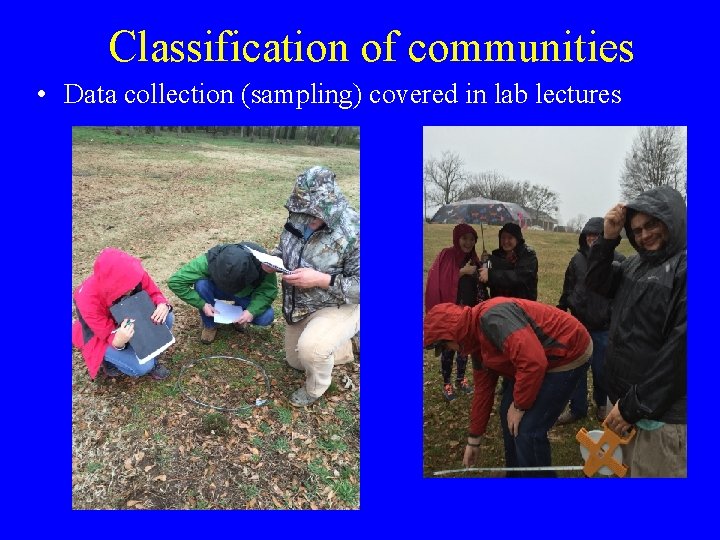
Classification of communities • Data collection (sampling) covered in lab lectures
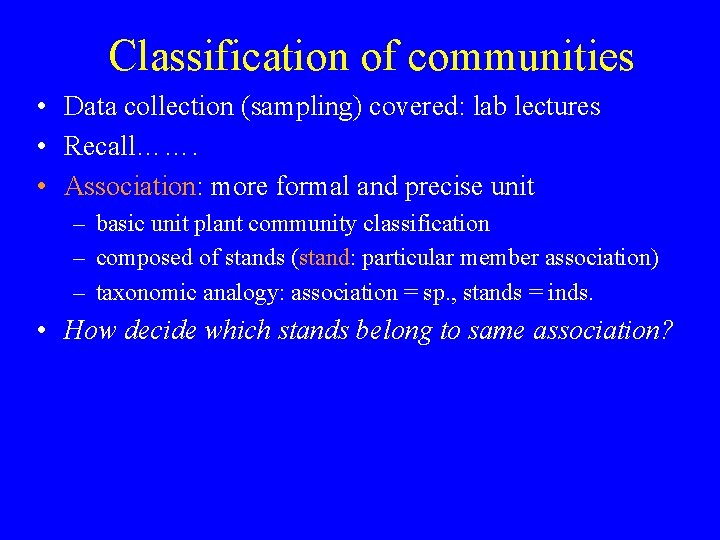
Classification of communities • Data collection (sampling) covered: lab lectures • Recall……. • Association: more formal and precise unit – basic unit plant community classification – composed of stands (stand: particular member association) – taxonomic analogy: association = sp. , stands = inds. • How decide which stands belong to same association?
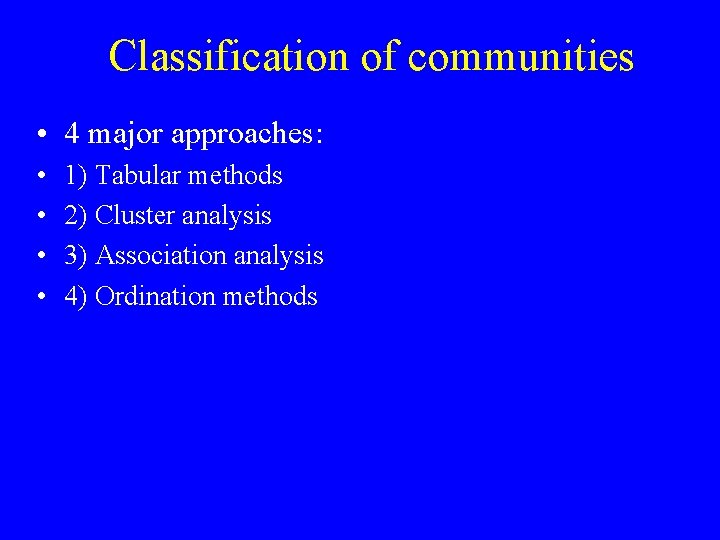
Classification of communities • 4 major approaches: • • 1) Tabular methods 2) Cluster analysis 3) Association analysis 4) Ordination methods
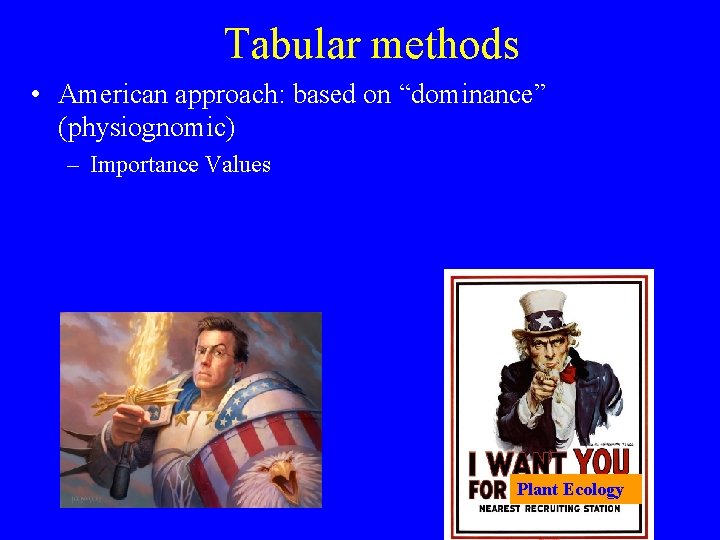
Tabular methods • American approach: based on “dominance” (physiognomic) – Importance Values Plant Ecology
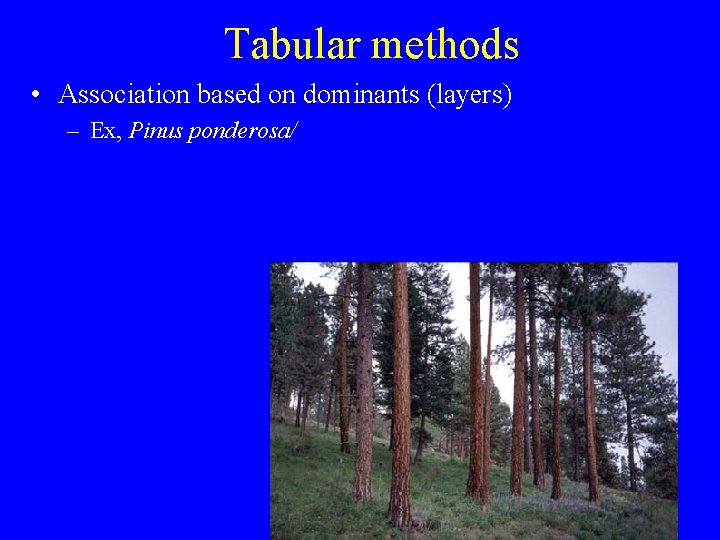
Tabular methods • Association based on dominants (layers) – Ex, Pinus ponderosa/
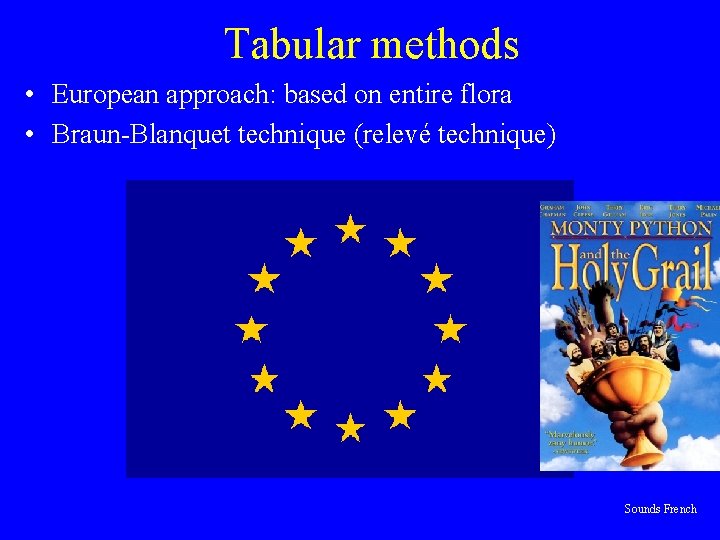
Tabular methods • European approach: based on entire flora • Braun-Blanquet technique (relevé technique) Sounds French
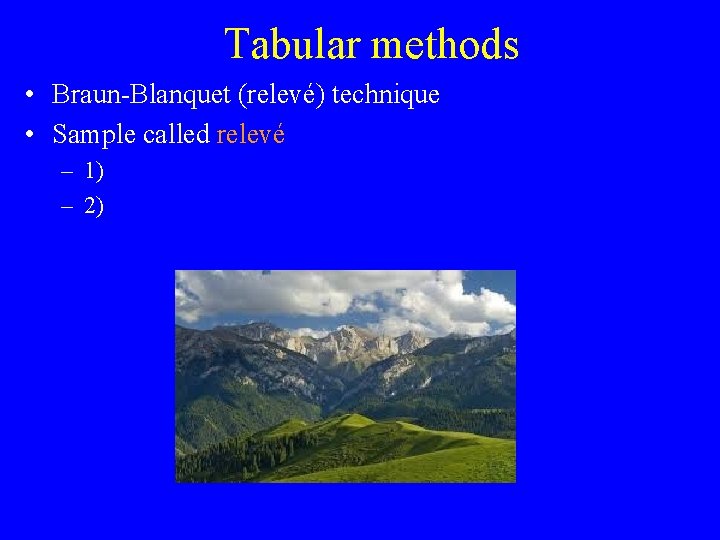
Tabular methods • Braun-Blanquet (relevé) technique • Sample called relevé – 1) – 2)
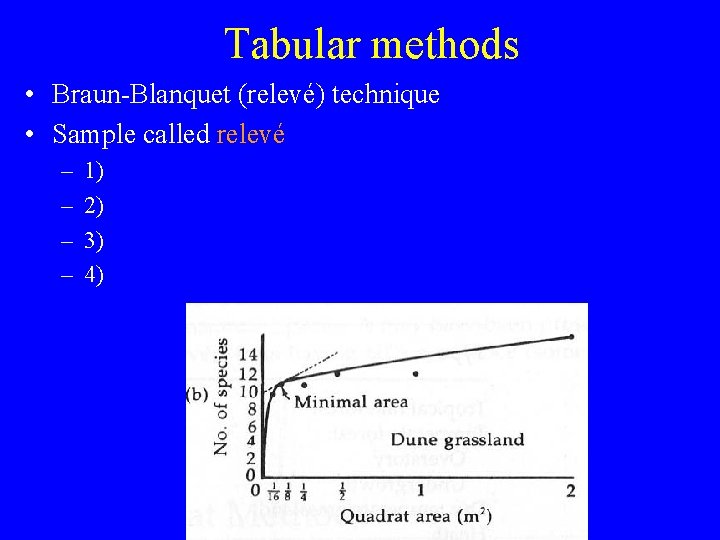
Tabular methods • Braun-Blanquet (relevé) technique • Sample called relevé – – 1) 2) 3) 4)
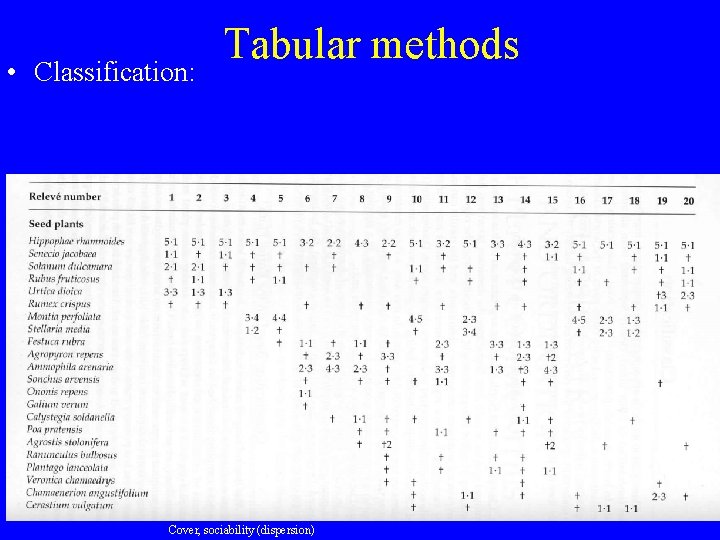
• Classification: Tabular methods Cover, sociability (dispersion)
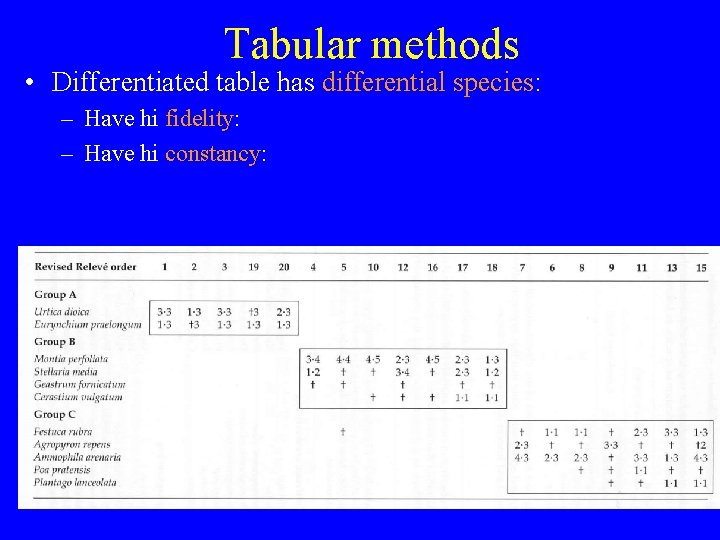
Tabular methods • Differentiated table has differential species: – Have hi fidelity: – Have hi constancy:
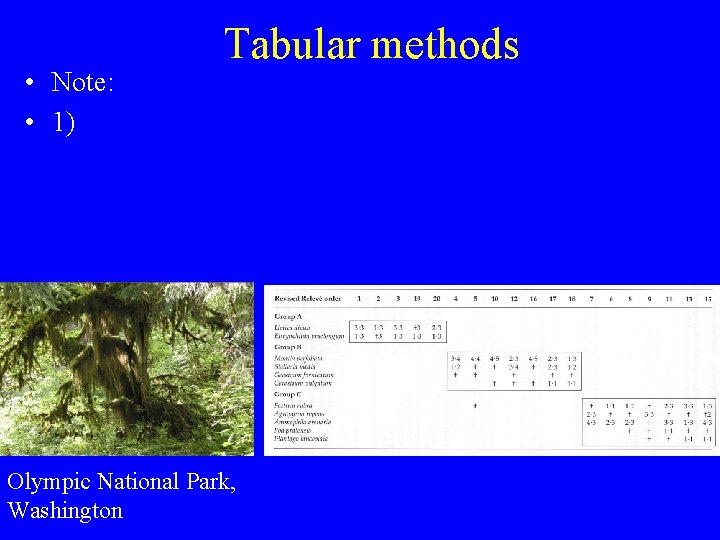
• Note: • 1) Tabular methods Olympic National Park, Washington
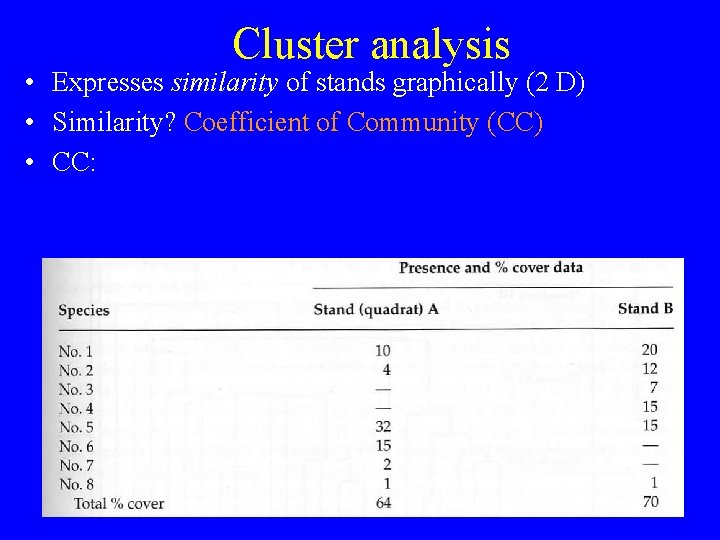
Cluster analysis • Expresses similarity of stands graphically (2 D) • Similarity? Coefficient of Community (CC) • CC:
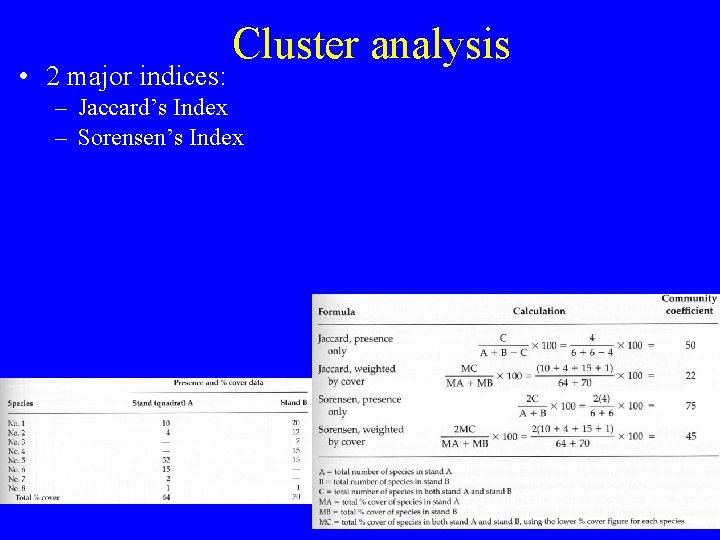
• 2 major indices: Cluster analysis – Jaccard’s Index – Sorensen’s Index
Transport medium examples
Apes nutrient cycles
Nutrients cycle
Nutrient cycles in marine ecosystems
Why is primary succession an orderly process
Termination of brachial artery
Nutrient cycle of a tropical rainforest
What are the six nutrient groups
Differential media
Eggs as emulsifier
Nutrient cycle in the serengeti
Gersmehl model deciduous woodland
Foods used in nutrition activities should be nutrient-dense
Bray nutrient mobility concept
Nutrient chain foldable
Journey 2050 student handout 2 word search
Chlorsis
Nutrient basics
Funtions of food
Journey 2050 student handout 3 crossword puzzle
Which ecosystem is this nutrient cycle from?
Defination of nutrients
Dorsalis pedis artery
Skeletal system
Tamoxifen nutrient depletion