Applying Queueing Theory and Simulation to the Modeling
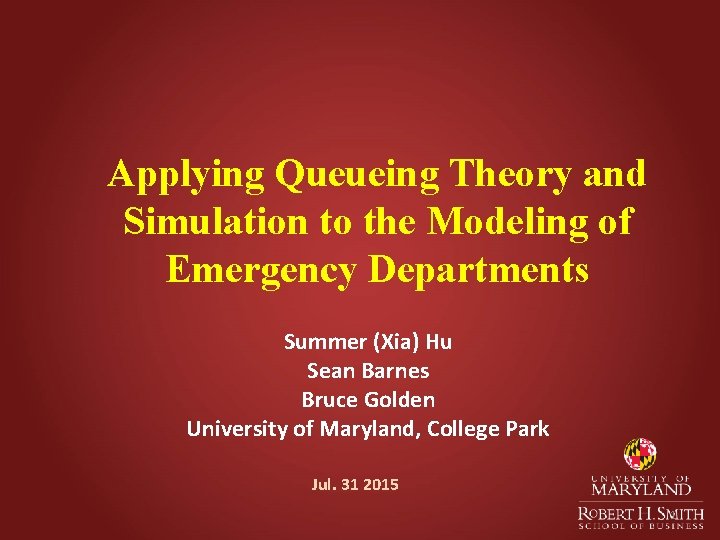
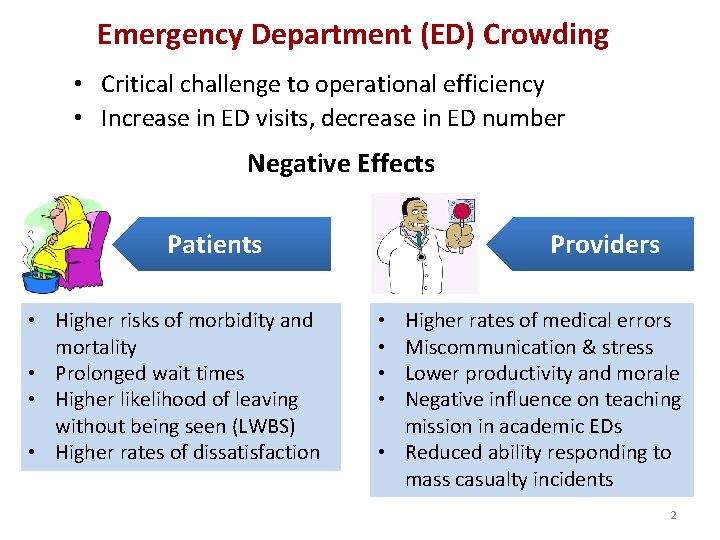
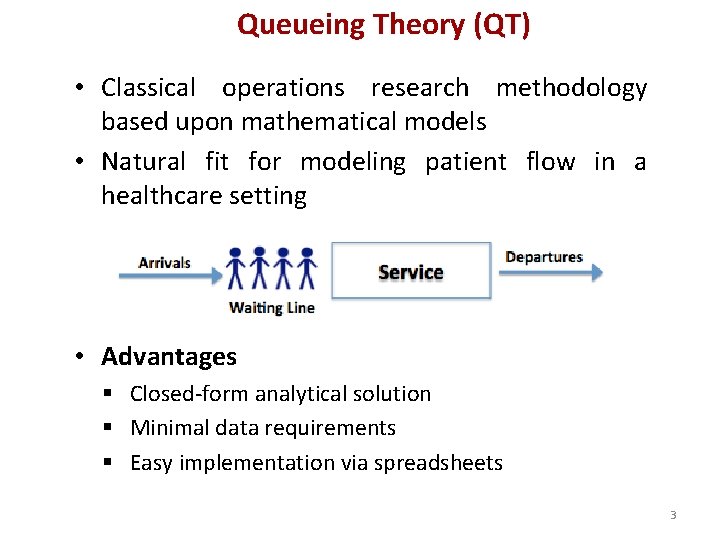
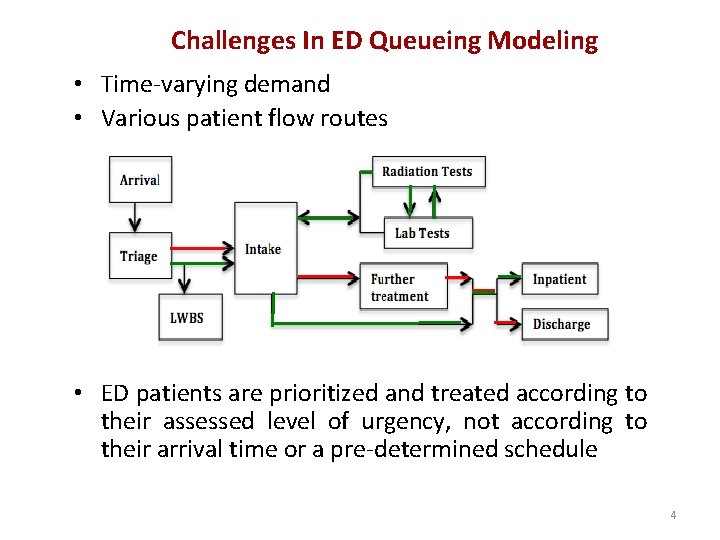
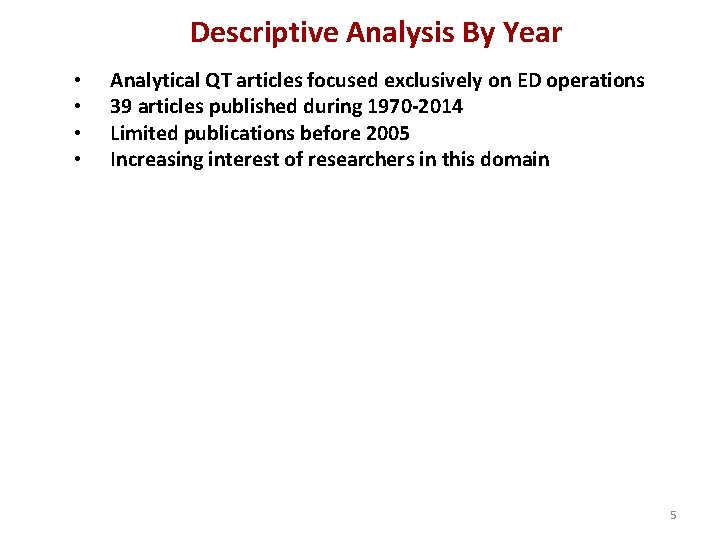
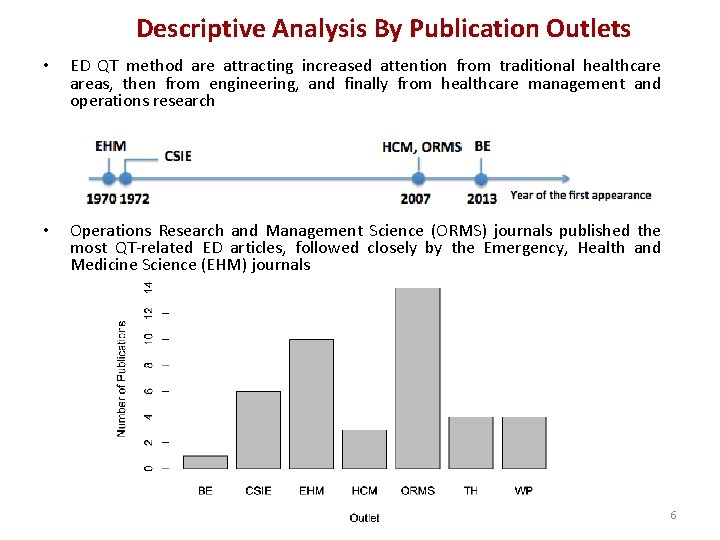
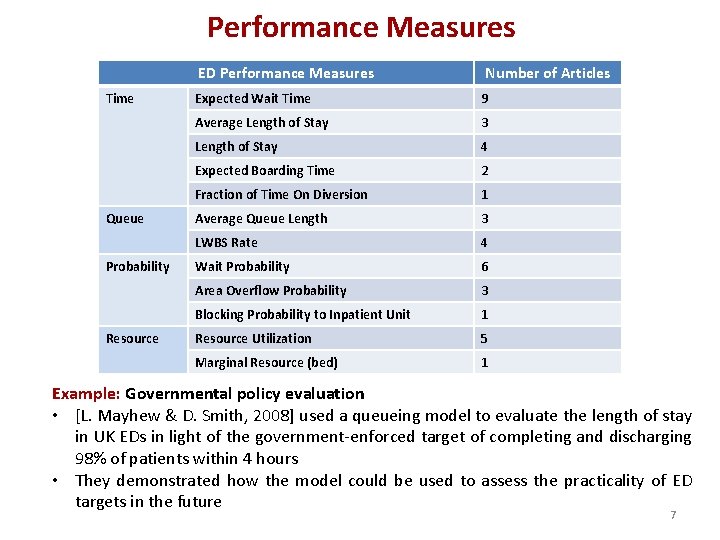
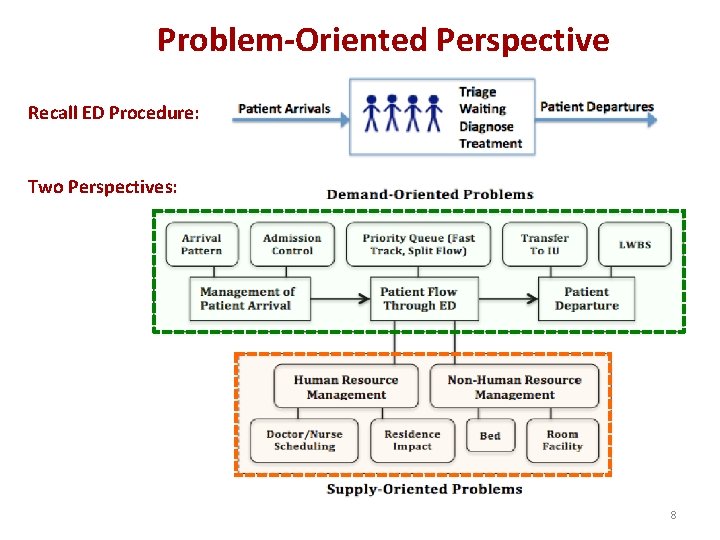
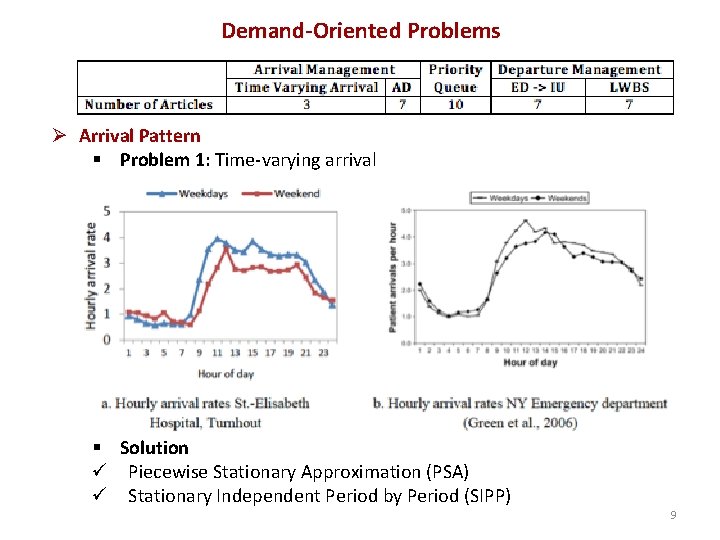
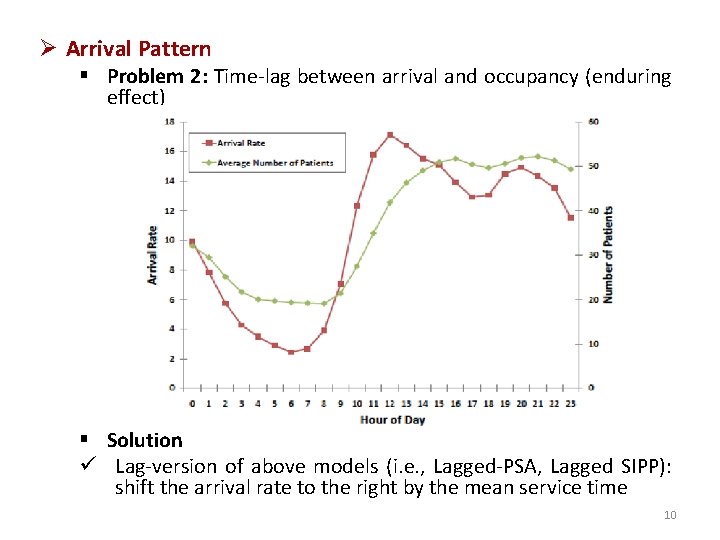
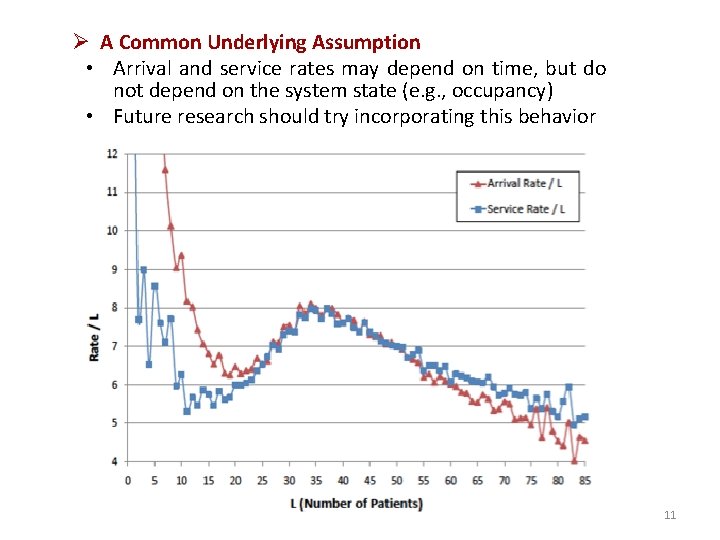
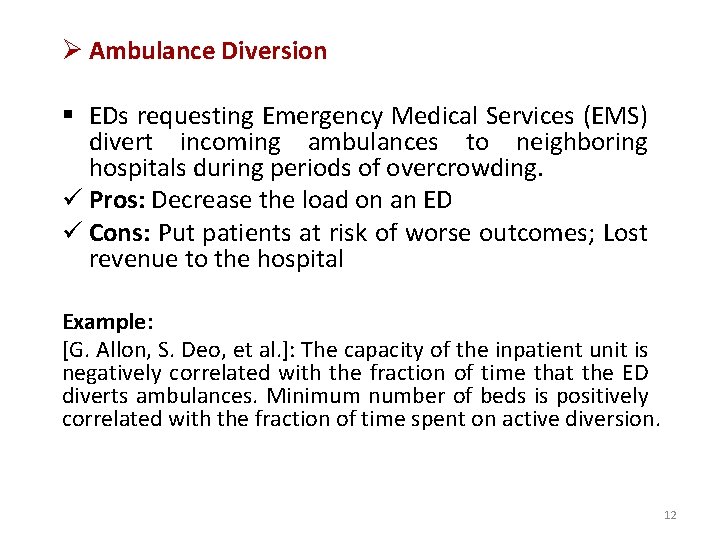
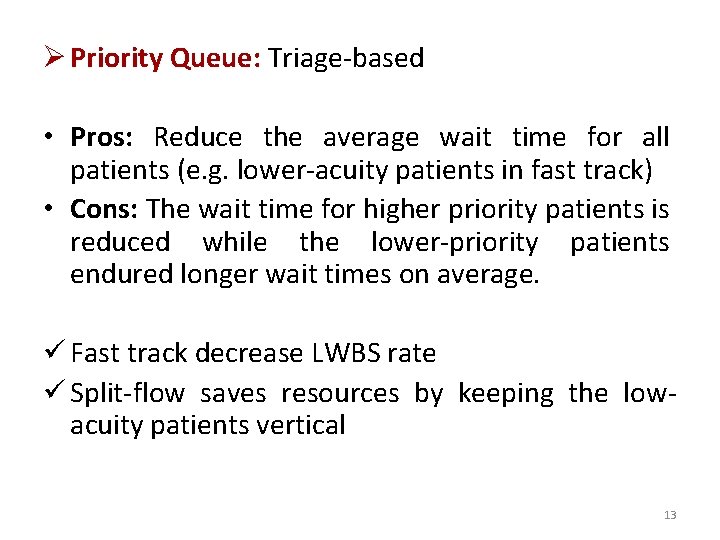
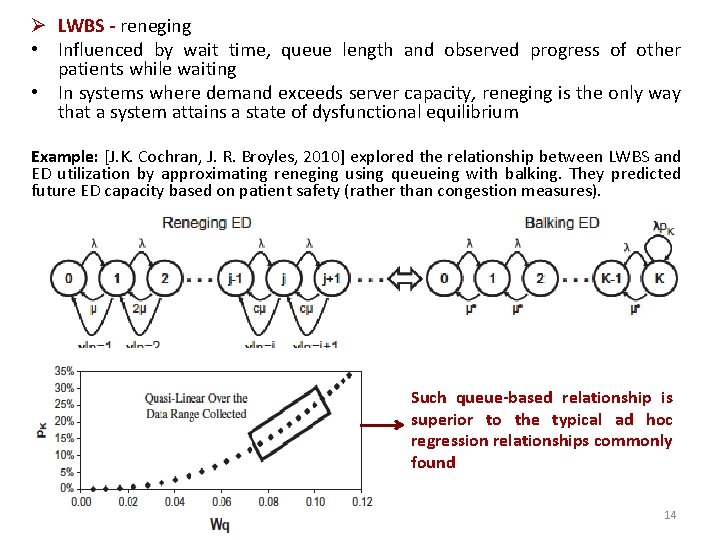
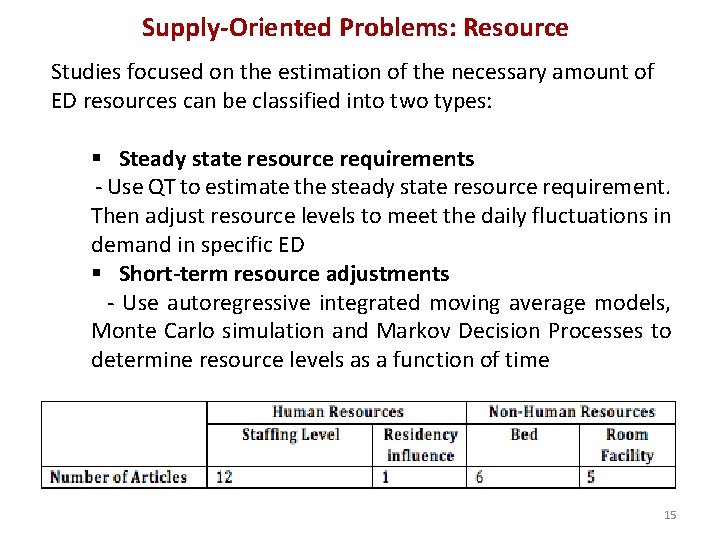
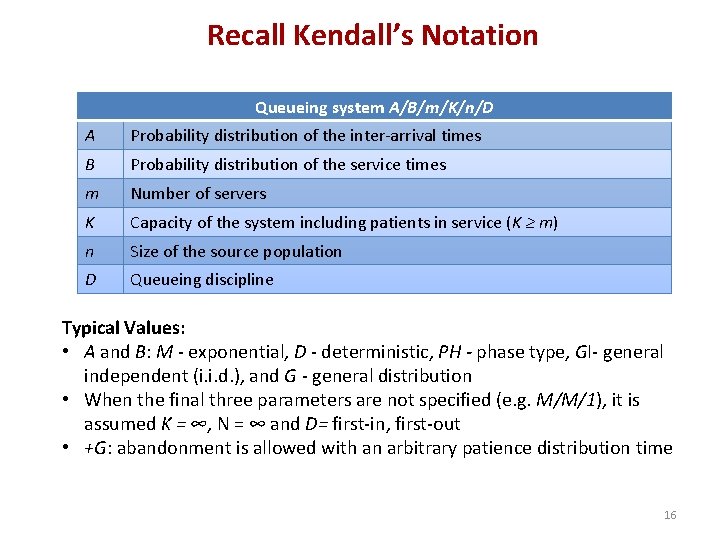
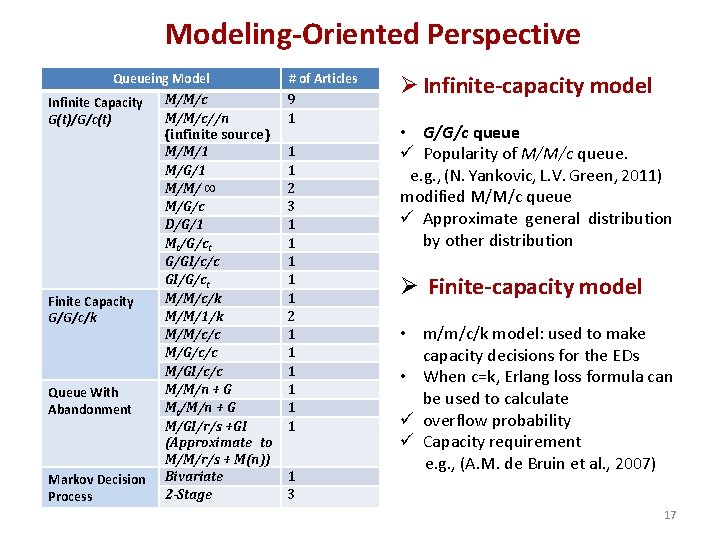
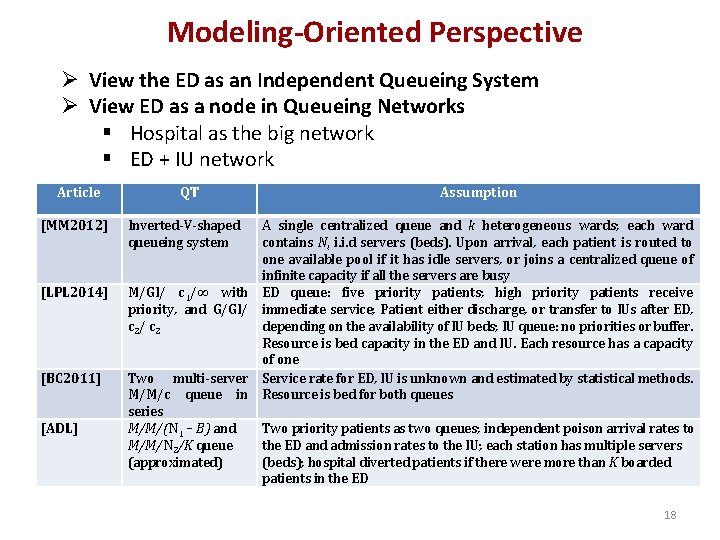
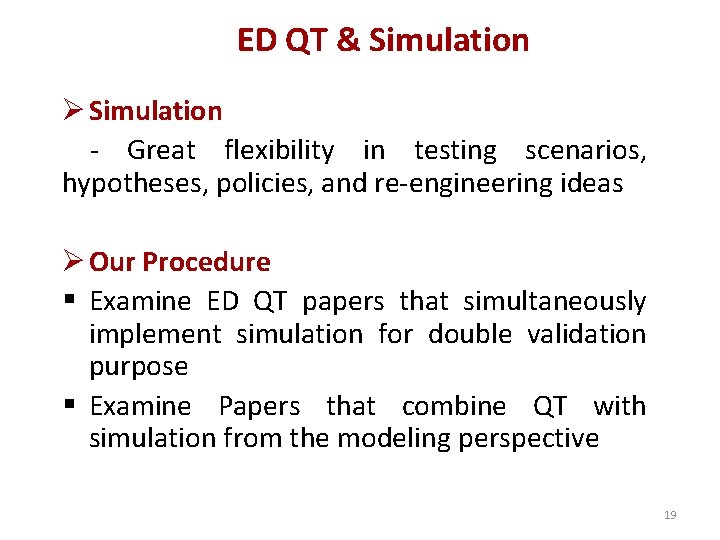
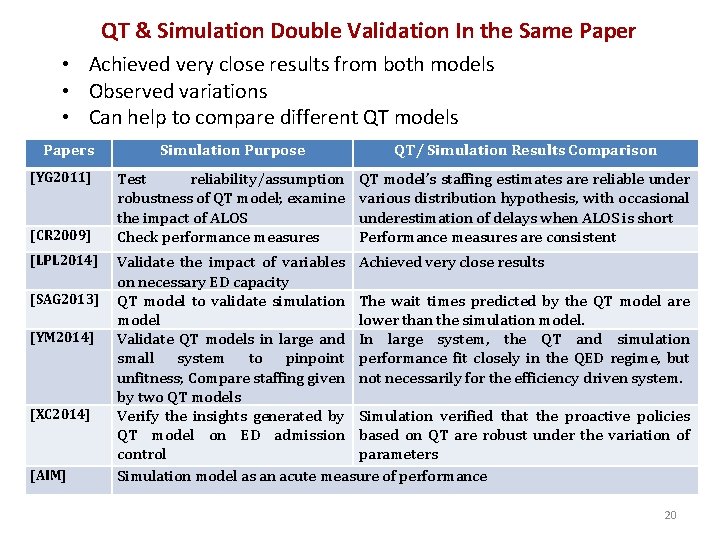
![QT & Simulation Double Validation In the Same Paper Example: [AIM] Simulation Purpose: Test QT & Simulation Double Validation In the Same Paper Example: [AIM] Simulation Purpose: Test](https://slidetodoc.com/presentation_image/401ce25fef3333a8203073b94e8dcc3c/image-21.jpg)
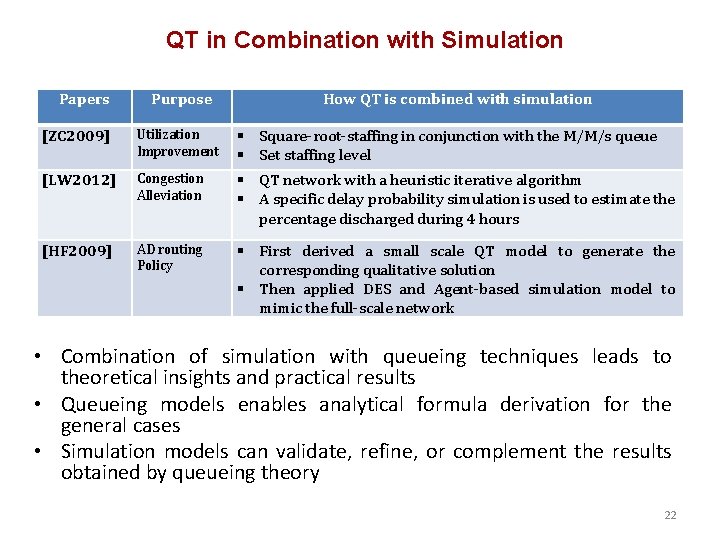
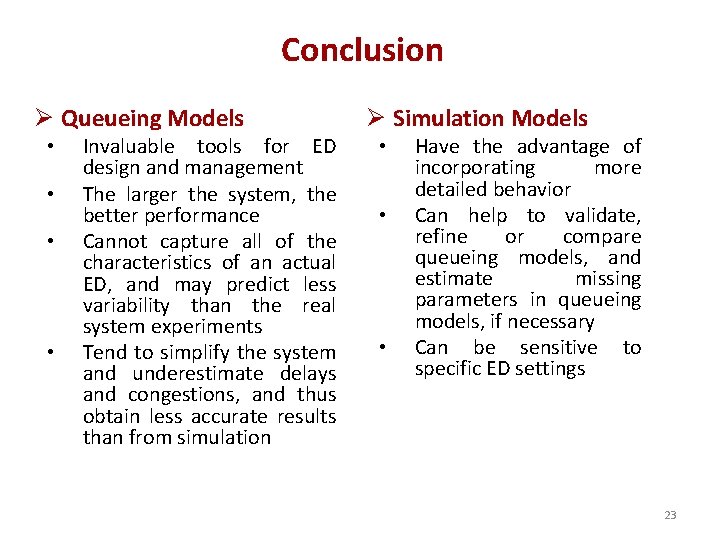
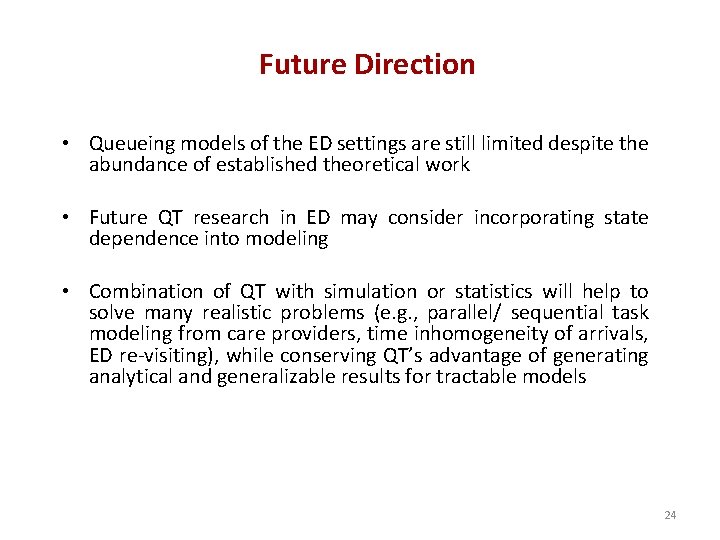
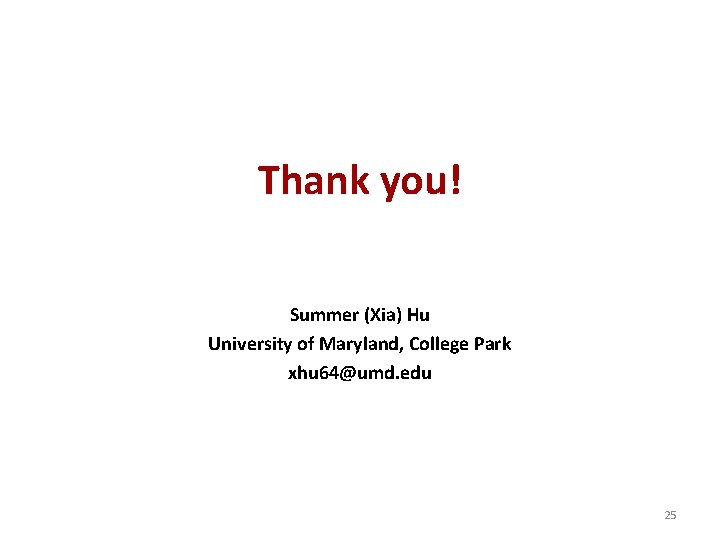
- Slides: 25
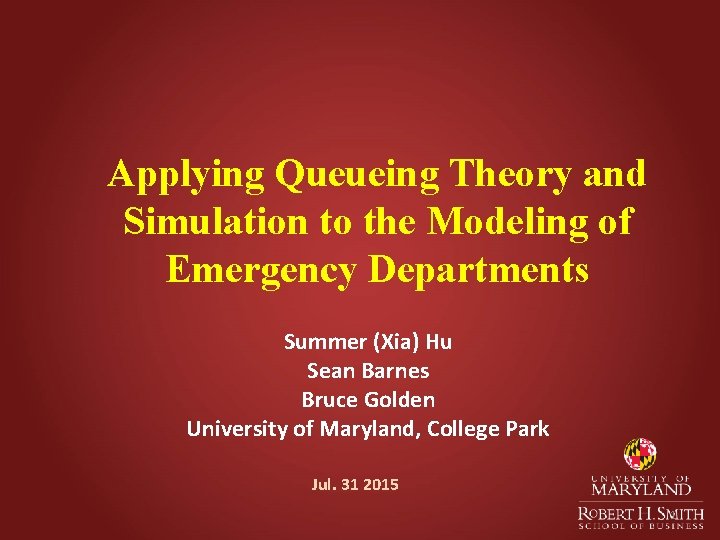
Applying Queueing Theory and Simulation to the Modeling of Emergency Departments Summer (Xia) Hu Sean Barnes Bruce Golden University of Maryland, College Park Jul. 31 2015 1
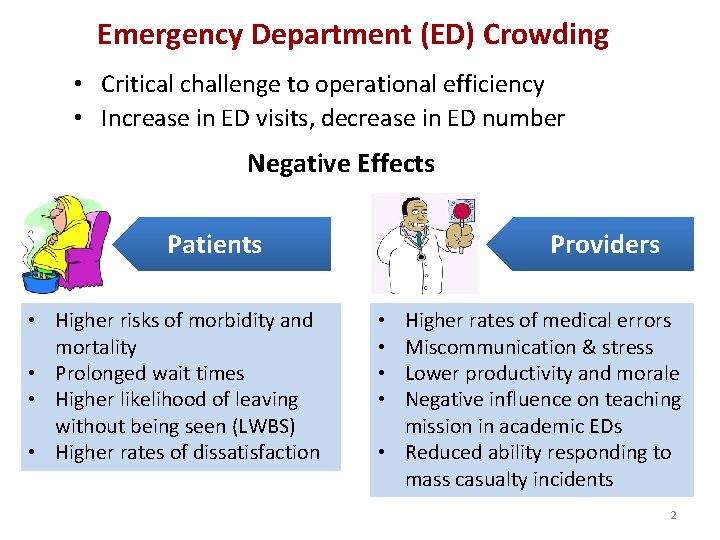
Emergency Department (ED) Crowding • Critical challenge to operational efficiency • Increase in ED visits, decrease in ED number Negative Effects Patients • Higher risks of morbidity and mortality • Prolonged wait times • Higher likelihood of leaving without being seen (LWBS) • Higher rates of dissatisfaction Providers Higher rates of medical errors Miscommunication & stress Lower productivity and morale Negative influence on teaching mission in academic EDs • Reduced ability responding to mass casualty incidents • • 2
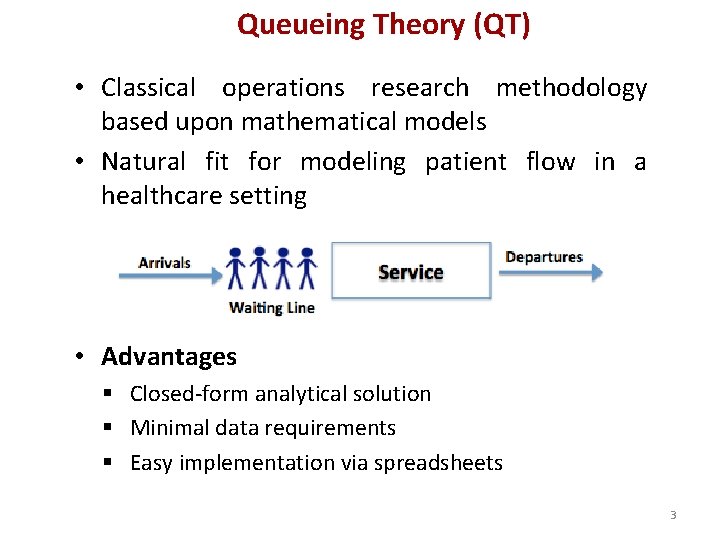
Queueing Theory (QT) • Classical operations research methodology based upon mathematical models • Natural fit for modeling patient flow in a healthcare setting • Advantages § Closed-form analytical solution § Minimal data requirements § Easy implementation via spreadsheets 3
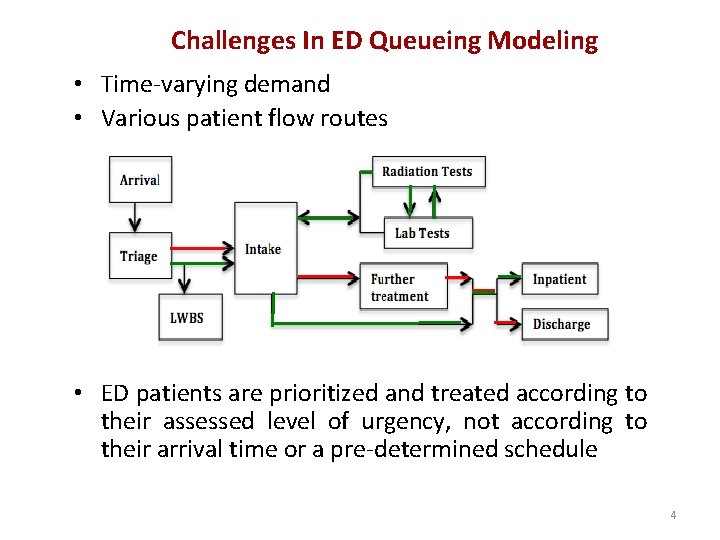
Challenges In ED Queueing Modeling • Time-varying demand • Various patient flow routes • ED patients are prioritized and treated according to their assessed level of urgency, not according to their arrival time or a pre-determined schedule 4
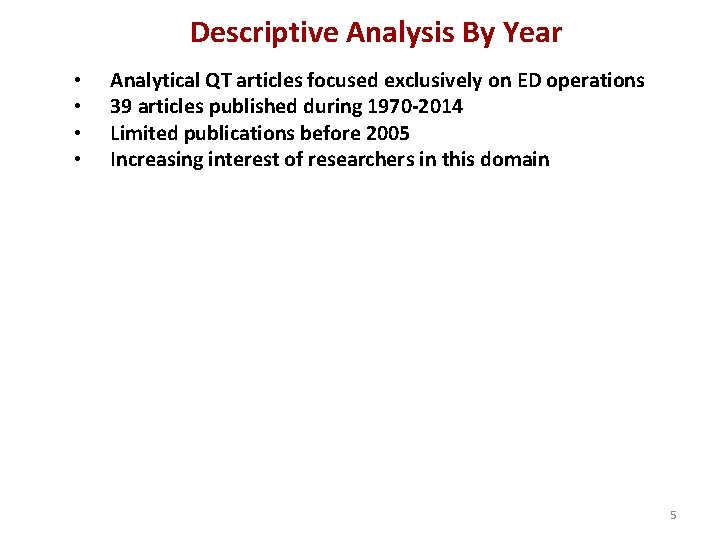
Descriptive Analysis By Year • • Analytical QT articles focused exclusively on ED operations 39 articles published during 1970 -2014 Limited publications before 2005 Increasing interest of researchers in this domain 5
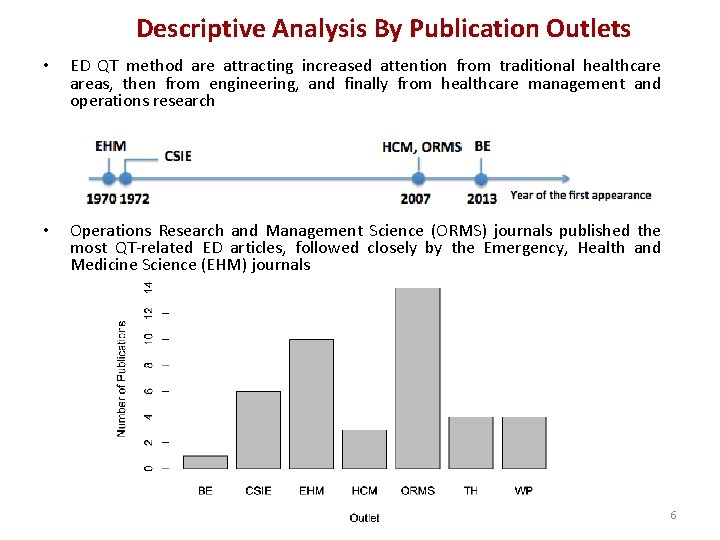
Descriptive Analysis By Publication Outlets • ED QT method are attracting increased attention from traditional healthcare areas, then from engineering, and finally from healthcare management and operations research • Operations Research and Management Science (ORMS) journals published the most QT-related ED articles, followed closely by the Emergency, Health and Medicine Science (EHM) journals 6
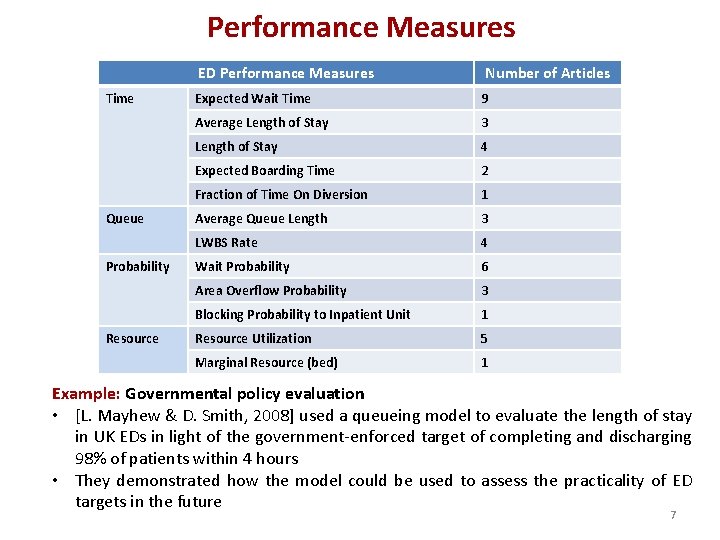
Performance Measures ED Performance Measures Time Queue Probability Resource Number of Articles Expected Wait Time 9 Average Length of Stay 3 Length of Stay 4 Expected Boarding Time 2 Fraction of Time On Diversion 1 Average Queue Length 3 LWBS Rate 4 Wait Probability 6 Area Overflow Probability 3 Blocking Probability to Inpatient Unit 1 Resource Utilization 5 Marginal Resource (bed) 1 Example: Governmental policy evaluation • [L. Mayhew & D. Smith, 2008] used a queueing model to evaluate the length of stay in UK EDs in light of the government-enforced target of completing and discharging 98% of patients within 4 hours • They demonstrated how the model could be used to assess the practicality of ED targets in the future 7
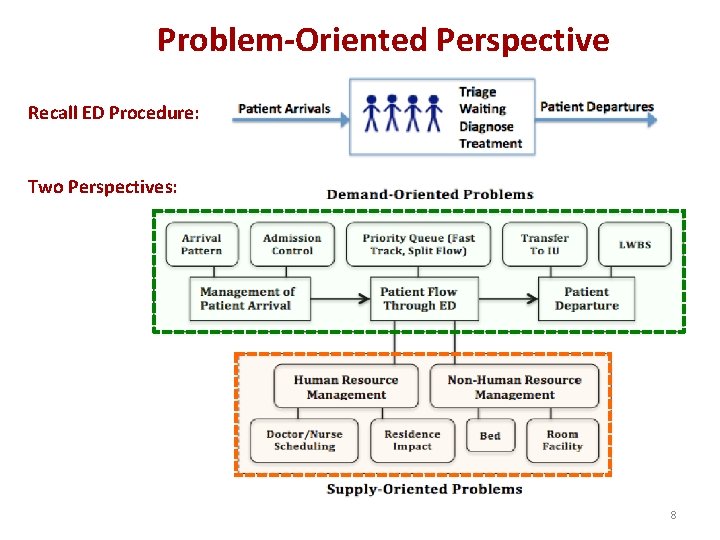
Problem-Oriented Perspective Recall ED Procedure: Two Perspectives: 8
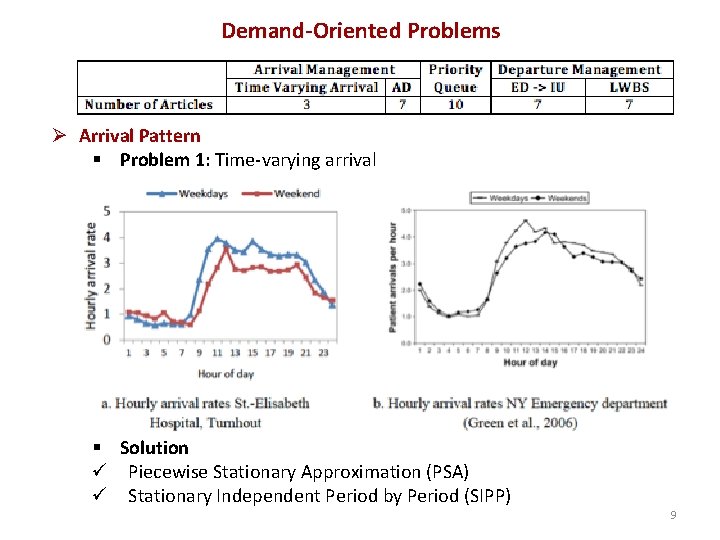
Demand-Oriented Problems Ø Arrival Pattern § Problem 1: Time-varying arrival § Solution ü Piecewise Stationary Approximation (PSA) ü Stationary Independent Period by Period (SIPP) 9
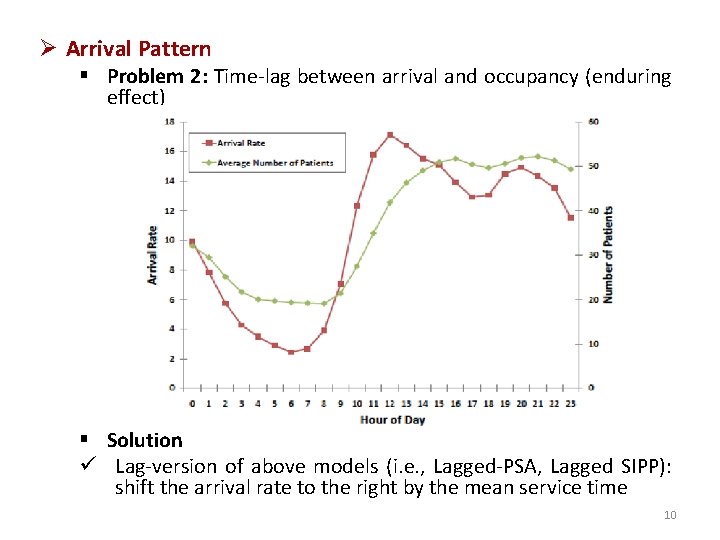
Ø Arrival Pattern § Problem 2: Time-lag between arrival and occupancy (enduring effect) § Solution ü Lag-version of above models (i. e. , Lagged-PSA, Lagged SIPP): shift the arrival rate to the right by the mean service time 10
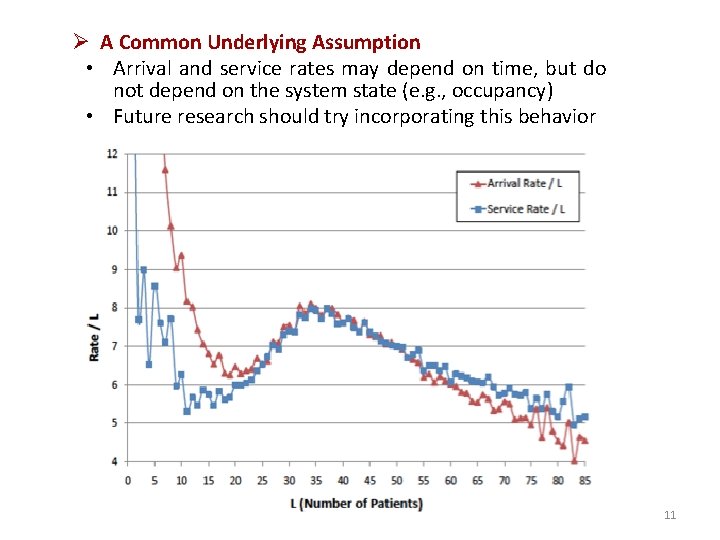
Ø A Common Underlying Assumption • Arrival and service rates may depend on time, but do not depend on the system state (e. g. , occupancy) • Future research should try incorporating this behavior 11
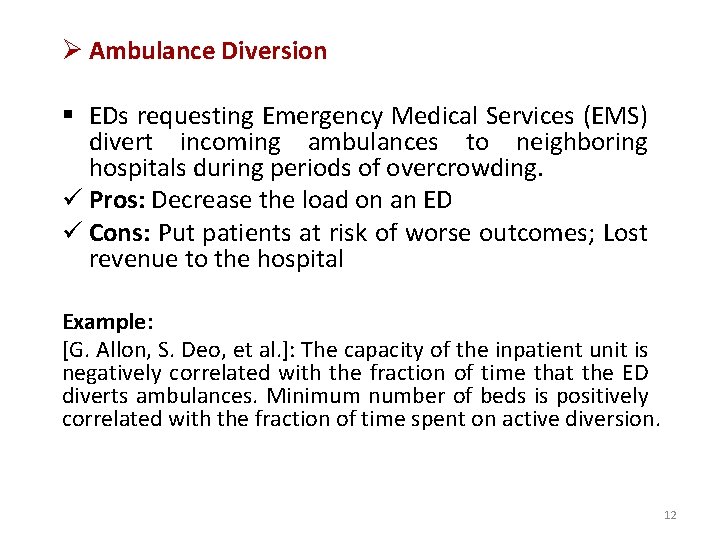
Ø Ambulance Diversion § EDs requesting Emergency Medical Services (EMS) divert incoming ambulances to neighboring hospitals during periods of overcrowding. ü Pros: Decrease the load on an ED ü Cons: Put patients at risk of worse outcomes; Lost revenue to the hospital Example: [G. Allon, S. Deo, et al. ]: The capacity of the inpatient unit is negatively correlated with the fraction of time that the ED diverts ambulances. Minimum number of beds is positively correlated with the fraction of time spent on active diversion. 12
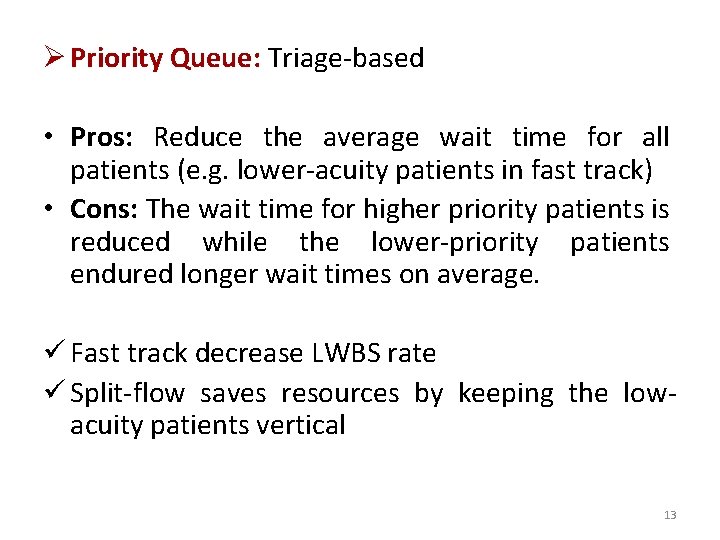
Ø Priority Queue: Triage-based • Pros: Reduce the average wait time for all patients (e. g. lower-acuity patients in fast track) • Cons: The wait time for higher priority patients is reduced while the lower-priority patients endured longer wait times on average. ü Fast track decrease LWBS rate ü Split-flow saves resources by keeping the lowacuity patients vertical 13
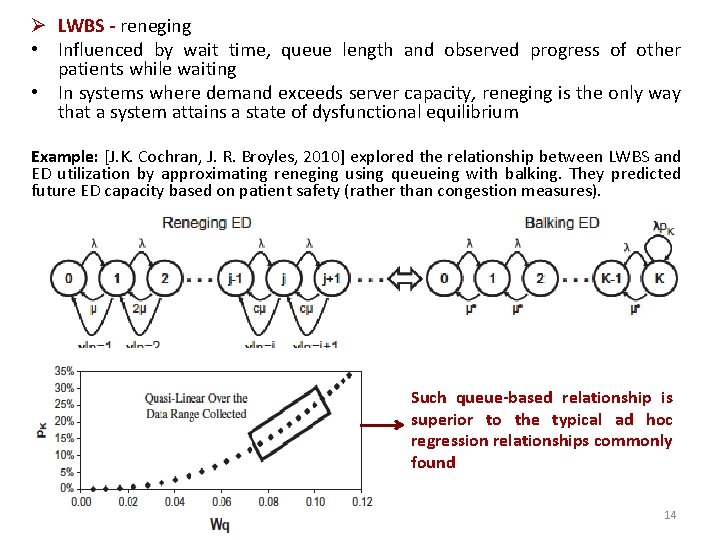
Ø LWBS - reneging • Influenced by wait time, queue length and observed progress of other patients while waiting • In systems where demand exceeds server capacity, reneging is the only way that a system attains a state of dysfunctional equilibrium Example: [J. K. Cochran, J. R. Broyles, 2010] explored the relationship between LWBS and ED utilization by approximating reneging using queueing with balking. They predicted future ED capacity based on patient safety (rather than congestion measures). Such queue-based relationship is superior to the typical ad hoc regression relationships commonly found 14
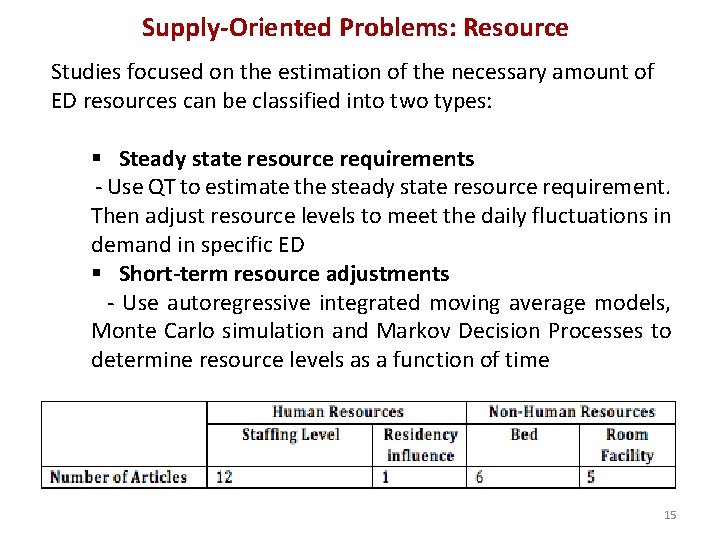
Supply-Oriented Problems: Resource Studies focused on the estimation of the necessary amount of ED resources can be classified into two types: § Steady state resource requirements - Use QT to estimate the steady state resource requirement. Then adjust resource levels to meet the daily fluctuations in demand in specific ED § Short-term resource adjustments - Use autoregressive integrated moving average models, Monte Carlo simulation and Markov Decision Processes to determine resource levels as a function of time 15
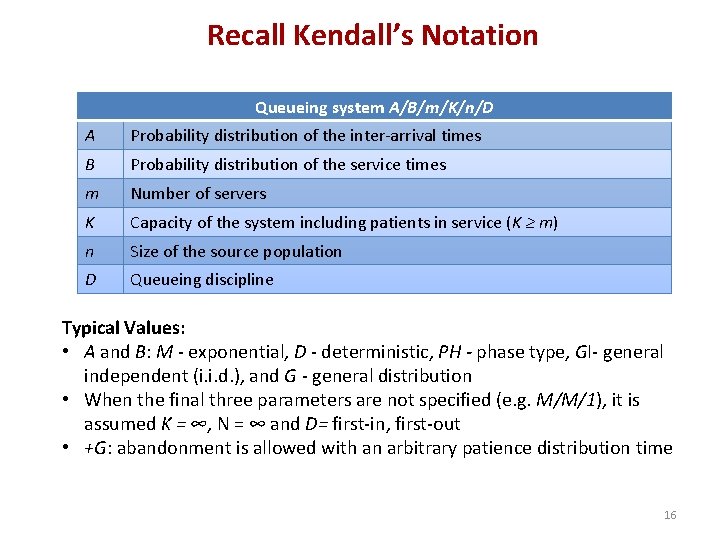
Recall Kendall’s Notation Queueing system A/B/m/K/n/D A Probability distribution of the inter-arrival times B Probability distribution of the service times m Number of servers K Capacity of the system including patients in service (K ≥ m) n Size of the source population D Queueing discipline Typical Values: • A and B: M - exponential, D - deterministic, PH - phase type, GI- general independent (i. i. d. ), and G - general distribution • When the final three parameters are not specified (e. g. M/M/1), it is assumed K = ∞, N = ∞ and D= first-in, first-out • +G: abandonment is allowed with an arbitrary patience distribution time 16
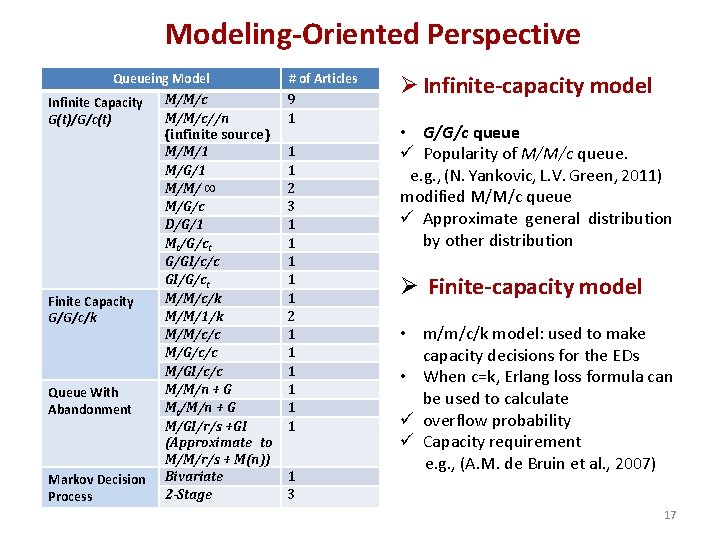
Modeling-Oriented Perspective Queueing Model M/M/c Infinite Capacity M/M/c//n G(t)/G/c(t) (infinite source) M/M/1 M/G/1 M/M/ ∞ M/G/c D/G/1 Mt/G/ct G/GI/c/c GI/G/ct M/M/c/k Finite Capacity M/M/1/k G/G/c/k M/M/c/c M/GI/c/c M/M/n + G Queue With Mt/M/n + G Abandonment M/GI/r/s +GI (Approximate to M/M/r/s + M(n)) Markov Decision Bivariate 2 -Stage Process # of Articles 9 1 1 1 2 3 1 1 1 2 1 1 1 1 3 Ø Infinite-capacity model • G/G/c queue ü Popularity of M/M/c queue. e. g. , (N. Yankovic, L. V. Green, 2011) modified M/M/c queue ü Approximate general distribution by other distribution Ø Finite-capacity model • m/m/c/k model: used to make capacity decisions for the EDs • When c=k, Erlang loss formula can be used to calculate ü overflow probability ü Capacity requirement e. g. , (A. M. de Bruin et al. , 2007) 17
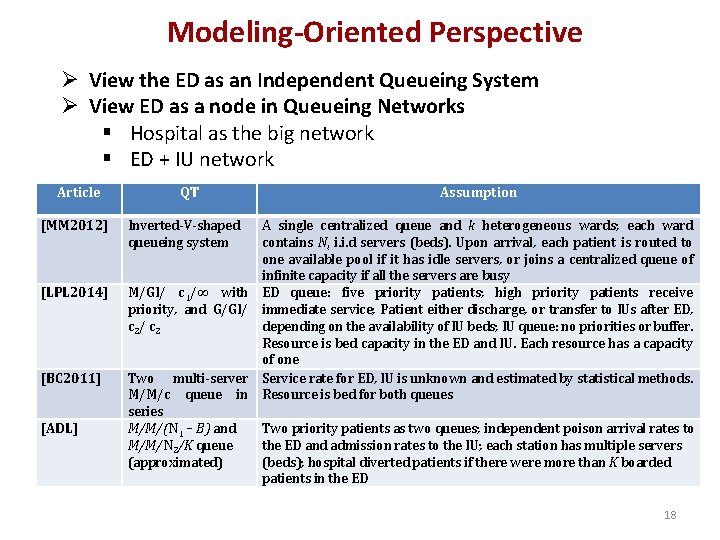
Modeling-Oriented Perspective Ø View the ED as an Independent Queueing System Ø View ED as a node in Queueing Networks § Hospital as the big network § ED + IU network Article QT Assumption [MM 2012] Inverted-V-shaped queueing system [LPL 2014] M/GI/ c 1/∞ with priority, and G/GI/ c 2 [BC 2011] A single centralized queue and k heterogeneous wards; each ward contains Ni i. i. d servers (beds). Upon arrival, each patient is routed to one available pool if it has idle servers, or joins a centralized queue of infinite capacity if all the servers are busy ED queue: five priority patients; high priority patients receive immediate service; Patient either discharge, or transfer to IUs after ED, depending on the availability of IU beds; IU queue: no priorities or buffer. Resource is bed capacity in the ED and IU. Each resource has a capacity of one Service rate for ED, IU is unknown and estimated by statistical methods. Resource is bed for both queues Two multi-server M/M/c queue in series M/M/(N 1 − B) and Two priority patients as two queues; independent poison arrival rates to M/M/N 2/K queue the ED and admission rates to the IU; each station has multiple servers (approximated) (beds); hospital diverted patients if there were more than K boarded patients in the ED [ADL] 18
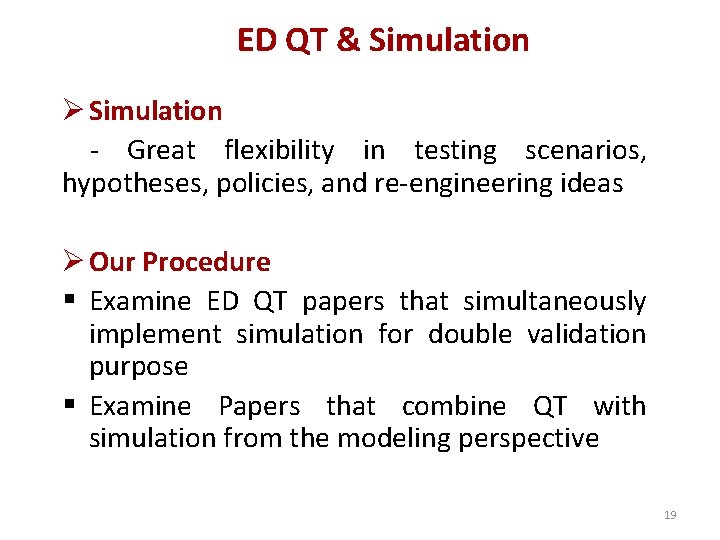
ED QT & Simulation Ø Simulation - Great flexibility in testing scenarios, hypotheses, policies, and re-engineering ideas Ø Our Procedure § Examine ED QT papers that simultaneously implement simulation for double validation purpose § Examine Papers that combine QT with simulation from the modeling perspective 19
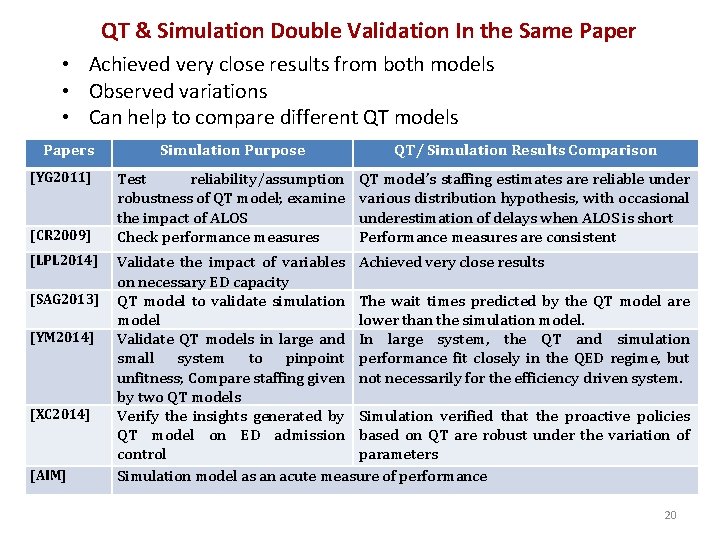
QT & Simulation Double Validation In the Same Paper • Achieved very close results from both models • Observed variations • Can help to compare different QT models Papers [YG 2011] [CR 2009] [LPL 2014] [SAG 2013] [YM 2014] [XC 2014] [AIM] Simulation Purpose QT/ Simulation Results Comparison Test reliability/assumption robustness of QT model; examine the impact of ALOS Check performance measures QT model’s staffing estimates are reliable under various distribution hypothesis, with occasional underestimation of delays when ALOS is short Performance measures are consistent Validate the impact of variables Achieved very close results on necessary ED capacity QT model to validate simulation The wait times predicted by the QT model are model lower than the simulation model. Validate QT models in large and In large system, the QT and simulation small system to pinpoint performance fit closely in the QED regime, but unfitness; Compare staffing given not necessarily for the efficiency driven system. by two QT models Verify the insights generated by Simulation verified that the proactive policies QT model on ED admission based on QT are robust under the variation of control parameters Simulation model as an acute measure of performance 20
![QT Simulation Double Validation In the Same Paper Example AIM Simulation Purpose Test QT & Simulation Double Validation In the Same Paper Example: [AIM] Simulation Purpose: Test](https://slidetodoc.com/presentation_image/401ce25fef3333a8203073b94e8dcc3c/image-21.jpg)
QT & Simulation Double Validation In the Same Paper Example: [AIM] Simulation Purpose: Test how the number of patients in ED depends on time and state of the system for different QT models Results: Compared with the Mt/Mt/ ∞ model which fits well only when ED patient number is small and Mt/Mi/ ∞ model which is even less accurate, the state dependent model Mi/Mi/ ∞ has an overall good fit with simulation and real system performance 21
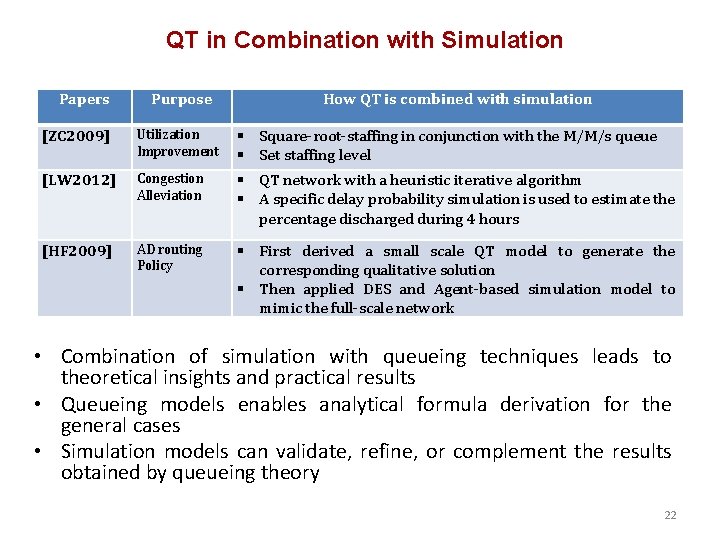
QT in Combination with Simulation Papers Purpose How QT is combined with simulation [ZC 2009] Utilization Improvement § Square-root-staffing in conjunction with the M/M/s queue § Set staffing level [LW 2012] Congestion Alleviation § QT network with a heuristic iterative algorithm § A specific delay probability simulation is used to estimate the percentage discharged during 4 hours [HF 2009] AD routing Policy § First derived a small scale QT model to generate the corresponding qualitative solution § Then applied DES and Agent-based simulation model to mimic the full-scale network • Combination of simulation with queueing techniques leads to theoretical insights and practical results • Queueing models enables analytical formula derivation for the general cases • Simulation models can validate, refine, or complement the results obtained by queueing theory 22
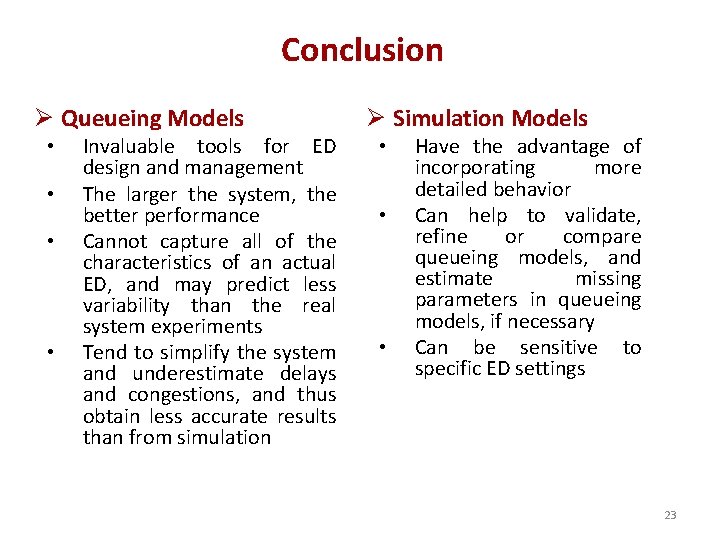
Conclusion Ø Queueing Models • • Invaluable tools for ED design and management The larger the system, the better performance Cannot capture all of the characteristics of an actual ED, and may predict less variability than the real system experiments Tend to simplify the system and underestimate delays and congestions, and thus obtain less accurate results than from simulation Ø Simulation Models • • • Have the advantage of incorporating more detailed behavior Can help to validate, refine or compare queueing models, and estimate missing parameters in queueing models, if necessary Can be sensitive to specific ED settings 23
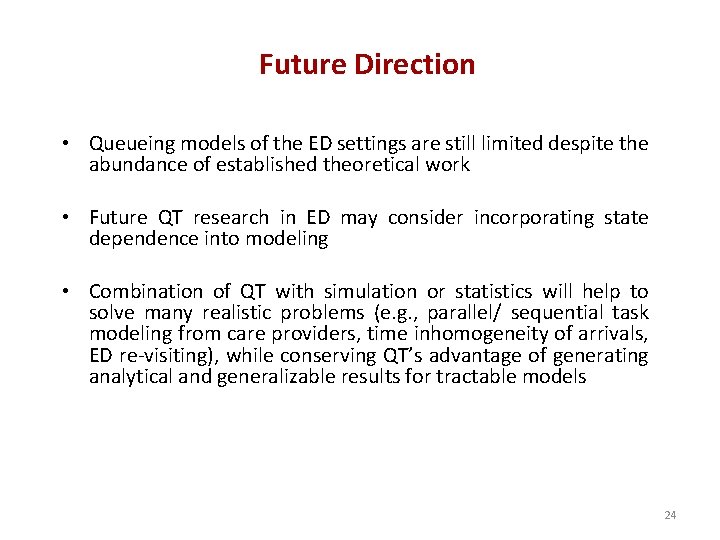
Future Direction • Queueing models of the ED settings are still limited despite the abundance of established theoretical work • Future QT research in ED may consider incorporating state dependence into modeling • Combination of QT with simulation or statistics will help to solve many realistic problems (e. g. , parallel/ sequential task modeling from care providers, time inhomogeneity of arrivals, ED re-visiting), while conserving QT’s advantage of generating analytical and generalizable results for tractable models 24
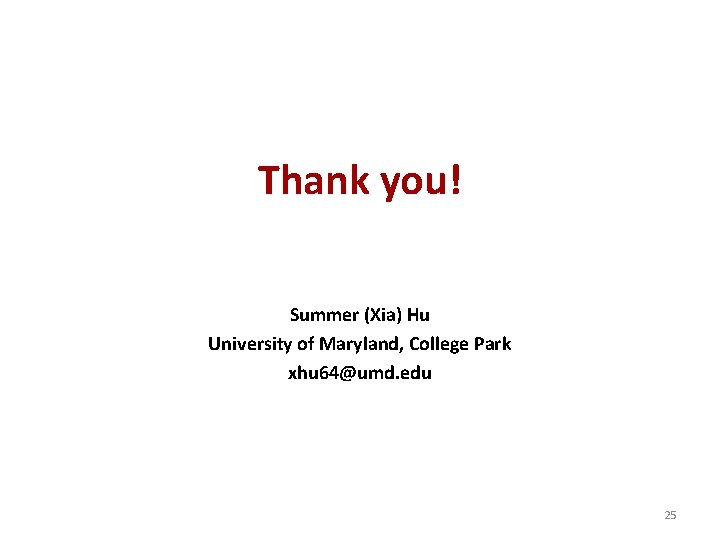
Thank you! Summer (Xia) Hu University of Maryland, College Park xhu 64@umd. edu 25
Modeling role modeling theory
Queuing theory formula
Queueing theory
Mm1 queue
Littles law
Virtual output queueing
Queueing
Predictive queueing
Kleinrock queueing systems
Simulation modeling and analysis law kelton
Pharmaceutical simulation and modeling
Introduction to modeling and simulation
Biomedical modeling and simulation
Contoh proses stokastik dalam kehidupan sehari-hari
Modeling and simulation
Relational modeling vs dimensional modeling
Nature of simulation
Basic simulation modeling
Applying educational theory in practice
Student development theory academic advising
Queuing theory simulation
Chapter 33 applying paints and enamels
Problem 4-1 applying the rules of debit and credit
What is the chemistry behind ml/pp and how does it work
Ch 28 milady
Chapter 17:5 providing first aid for poisoning